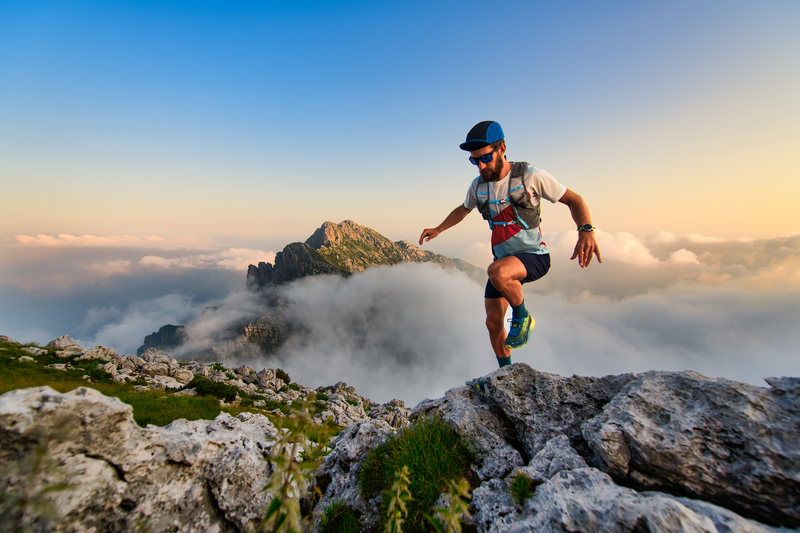
94% of researchers rate our articles as excellent or good
Learn more about the work of our research integrity team to safeguard the quality of each article we publish.
Find out more
REVIEW article
Front. Ecol. Evol. , 29 November 2021
Sec. Models in Ecology and Evolution
Volume 9 - 2021 | https://doi.org/10.3389/fevo.2021.750779
This article is part of the Research Topic Digital Evolution: Insights for Biologists View all 6 articles
In digital evolution, populations of computational organisms evolve via the same principles that govern natural selection in nature. These platforms have been used to great effect as a controlled system in which to conduct evolutionary experiments and develop novel evolutionary theory. In addition to their complex evolutionary dynamics, many digital evolution systems also produce rich ecological communities. As a result, digital evolution is also a powerful tool for research on eco-evolutionary dynamics. Here, we review the research to date in which digital evolution platforms have been used to address eco-evolutionary (and in some cases purely ecological) questions. This work has spanned a wide range of topics, including competition, facilitation, parasitism, predation, and macroecological scaling laws. We argue for the value of further ecological research in digital evolution systems and present some particularly promising directions for further research.
Over the past decade, researchers have become increasingly aware of the profound impact that ecological and evolutionary dynamics have on each other (Schoener, 2011; Hendry, 2016). Ecological interactions shape the underlying fitness landscape that is traversed by evolving populations. At the same time, evolutionary forces continually adjust the composition of organisms that make up that ecosystem. As such, ever-shifting fitness landscapes and fluctuating ecosystems continually fuel each other's change.
At the heart of these dynamics is diversity. Evolution requires diversity within a group for selection to act upon; low-diversity populations typically have low evolutionary potential (McDonald and Linde, 2002; Lavergne and Molofsky, 2007; Walker and Ofria, 2012). Ecosystems, by definition, are made up of diverse species and one of the fundamental questions in ecology is how a multitude of species can stably coexist. Ecosystems that are more diverse are generally more stable, productive, and resilient (Hooper et al., 2005; Tilman et al., 2014). In both ecology and evolution, there are some processes that should clearly reduce diversity and some that should promote—or at least preserve—it. A better understanding of these processes is critical to creating predictive theory for how evolving systems will respond to perturbations. Such theory will improve our ability to anticipate changes in the natural world, restore faltering ecosystems, or engineer novel microbiomes to improve human health. A current lack of strong theoretical foundations has imperiled our remediation efforts, and sometimes led them to backfire (Suding, 2011; Catalano et al., 2019).
However, untangling these dynamics has proven challenging. They are governed by a web of feedback loops, stochastic events, and emergent properties. Often simply adding a property, such as spatial structure, to a system will completely change the outcome (Doebeli and Knowlton, 1998; Vostinar and Ofria, 2019). To add further complications, these processes often play out over vast spatiotemporal scales, making them difficult to manipulate in a controlled experiment. As such, digital organisms are a valuable model system for understanding these complex dynamics (Adami, 2002). They allow us to untangle feedback loops by performing experiments in which we disable focal processes. For example, sometimes it would be useful to disentangle the effects of ecological and evolutionary dynamics so that we can understand the exact factors that produced a result. In a wet lab or field system, experiments that require such a fine level of manipulation are intractable. However, in a digital system mutations can be disabled with the flip of a switch (ecology mode) as can interactions among organisms (evolution mode). Another obstacle to understanding eco-evolutionary dynamics is that evolution experiments often require thousands of generations. Even for rapidly-reproducing organisms like bacteria, it takes nearly a year just to reach 2000 generations (Wiser et al., 2013), but most digital evolution systems can reach this milestone in less than an hour. Similarly, collecting ecological data at a high enough resolution to answer many questions is labor-intensive in biological systems, but can be automated in their digital counterparts. The speed and pellucidity of digital systems enables us to test our intuition in near real-time, allowing us to form precise questions before investing the time to conduct a well-targeted laboratory experiment.
We can situate field research, laboratory experiments, and digital evolution along a continuum (see Figure 1). The richest ecological and evolutionary dynamics are found in nature, and can be studied via field research. However, field research is accompanied by the aforementioned challenges with replication, sample size, and precisely measuring variables of interest. Laboratory experiments trade some of the richness of the field for increased experimental tractability. For most purposes, digital evolution platforms are a step further along this continuum. However, they hardly represent an extreme; other mathematical and computational systems are even faster and more tractable, with the necessary side effect of being more abstract.
Figure 1. Continuum of study system richness vs. ease of interpretation. In choosing a system in which to study ecological or evolutionary questions, there are inherent trade-offs to be made. As systems become more abstract, they can be made faster and easier to interpret. However, they may fail to capture important aspects of the dynamics observed in nature. Conversely, systems with dynamics that better reflect nature are more challenging to study. Indeed, study systems at all points along this continuum can provide useful insights into different aspects of ecology and evolution.
The next step toward speed/transparency/abstraction are simulations. Like digital evolution, simulations are computer programs in which ecological and/or evolutionary dynamics play out. However, simulations are simpler and more targeted at a specific question or real-world system. Indeed, the goal of a simulation is usually to predict which of a limited set of outcomes will occur in a given real-world scenario, while digital evolution systems strive for open-ended dynamics and outcomes. Simulations may be individual-based like digital evolution, or they may focus on a different level of abstraction. Because simulations and digital evolution are both broad categories that span a considerable range of the speed/transparency/abstraction continuum, the boundary between them can sometimes be fuzzy. In the context of computational evolution research, the fundamental distinction between simulations and digital evolution is that simulations study changes in the frequency of predefined traits whereas digital evolution systems instantiate evolution (Pennock, 2007).
Lastly, there are mathematical models. These are generally written in pure math and are maximally fast, transparent, and abstract. When they are able to capture the relevant dynamics of a system, they are an incredibly powerful tool. However, constructing a mathematical model generally requires abstracting away many of the intricacies found in systems with more natural dynamics (Adami et al., 2016). Such simplification is necessary and important, but there is an inherent risk of excluding dynamics that turn out to be essential. Dynamics that are known to be important, but are often excluded for the sake of model tractability include spatial structure, low-level organism-organism interactions, and the capacity for subtle variations among individuals across a population. Mathematical models are also a broad category (which overlaps with simulations), with different types of models serving different purposes and falling at different points along the speed/transparency/abstraction continuum (Evans et al., 2013).
Ultimately, all of these types of systems complement each other. Testing the predictions of a mathematical model in a digital evolution system gives us added confidence that the model is a useful abstraction. Similarly, exploring a concept in a digital evolution system can help us gain useful intuition for crafting experiments in a laboratory or field system before we put months or years into data collection. Conversely, a hypothesis to explain an unexpected outcome in a laboratory experiment might lead us to build a simulation to test that hypothesis. Such synergies exist between all steps along the continuum. Each is a useful tool in our toolbox.
We argue that digital evolution systems have a number of properties that make them well-suited to the task of studying eco-evolutionary dynamics:
Because digital evolution systems are instantiations of evolution (as opposed to merely simulating evolutionary dynamics) (Pennock, 2007), they are necessarily individual-based. Each member of the population is an individual with some amount of agency, allowing for a broad range of survival traits to evolve. Experiments are conducted by modifying the rules governing the behavior of individuals or their interactions with each other and the environment. This approach is in contrast to systems that represent the population at a more abstract level, such as a Gaussian distribution of trait values or as a set of categorical traits. In these non-individual-based systems, experiments are more often performed by changing the equations that govern how the population changes over time. These two approaches generally target different levels of abstraction. Because digital evolution systems try to avoid introducing any preconceptions about the range of potential outcomes that could result from a given set of starting conditions, individual-based models are more appropriate in cases where the cumulative impact of individual behavior can lead to complex and nuanced population-level effects. Most open questions in eco-evolutionary dynamics fall squarely into this category, as they largely concern the large-scale trends that result from simple rules governing interactions between a large number of individuals.
In thinking about what individual-level properties should be encoded in an eco-evolutionary system, it is helpful to consider which rules are necessary and/or sufficient for which dynamics. Variation, inheritance, and differential reproductive success are necessary to instantiate evolution by natural selection, but are not sufficient for the formation of stable ecological communities. Even the capacity for ecological interaction is insufficient, although it is necessary. Stable ecological communities require that there be multiple potential strategies for survival (i.e., niches) and that it be possible for groups of organisms using different strategies to coexist. There are a vast number of ways to add this property to a system, many of which will be discussed later in this review.
The underlying genetic representation of organisms in most digital evolution systems are computer programs, making them fundamentally different from DNA-based organisms. While this fact means that digital evolution is generally not a good fit for modeling any specific system on earth, it also provides a valuable framework for producing insights that generalize across systems (Maynard Smith, 1992; Ofria and Wilke, 2004; Kawecki et al., 2012; MacPherson and Gras, 2016). Traditionally, general inferences about biology are drawn by observing the same behavior across multiple model systems (e.g., Drosophila, E. coli, and Arabadopsis). The more distinct these systems are from each other the better, as any differences reduce the chance that the observed results were caused by an idiosyncrasy of a specific subset of the phylogeny of life on earth. However, all of life on earth shares some common ancestry (Maynard Smith, 1992). Thus, even results that can be replicated across many model systems are not necessarily unavoidable outcomes of evolution. If we observe a consistent pattern across digital and natural systems, however, that is strong evidence that it will be true for most evolving systems.
The problem of how to achieve generality has historically been a greater source of concern in ecology than in evolutionary biology (Fox, 2019). There are a variety of approaches that ecologists use to obtain general results (Fox, 2019), a number of which involve a potentially arduous process of comparison between a large number of distinct ecosystems. We can accelerate this process by starting with comparisons to digital systems. This technique allows us to quickly determine whether results are likely to generalize before putting in the substantial work required to conduct a comparable field study. More importantly, if we do not observe the same results in biology as we do in digital evolution, that is an indication that there is an important factor at play that we have not noticed. Such disparities help us identify mistaken preconceptions and either direct us toward building more realistic digital environments or identify critical areas where ecological theory may need further development.
Being able to generalize eco-evolutionary findings is important for predicting the future of ecosystems on earth. This importance is heightened by climate change. As our planet enters states that are increasingly dissimilar from any that we have previously observed, relying purely on knowledge specific to any given ecosystem is becoming less viable. Additionally, understanding the general principles that organize all eco-evolutionary systems is a critical tool for astrobiologists seeking to identify signatures that would suggest the presence of life on another planet.
Since evolution is a key component of eco-evolutionary dynamics, it is important to consider the adaptive landscape in which that evolution is occurring. Fitness landscapes are a common tool in evolutionary biology that map known genotypes or phenotypes to their associated fitness; similar traits are close together on the map based on the ease of mutating between them (Wright, 1932). When visualized in three dimensions, values of two traits are generally arranged along the x- and y-axes, with the fitness corresponding to each of these values shown as a surface on the z-axis. This surface generally has peaks that we expect the population to climb over time, and valleys that we expect will be challenging to cross. In reality, fitness landscapes exist in many more than three dimensions, which may impact the validity of the intuitions they provide (Gavrilets and Vose, 2005), but they are generally believed to be a useful mental model nonetheless. In many cases, fitness landscapes change over time, in which case they are often referred to as “seascapes” (Mustonen and Lässig, 2009). The fitness landscape on which a population is evolving plays a large role in determining the population's adaptive trajectory, often in relatively complex ways. Having a well-understood fitness landscape is critical, or at least helpful, for most research on evolutionary theory. The fitness landscape determines which mutations will be beneficial, how easy it is to arrive at the most fit phenotype, and, to some extent, the strength of selection—factors that are often critical to evolution research.
When we study eco-evolutionary dynamics, we are often effectively studying multiple fitness landscapes layered on top of each other. At least one of these landscapes is, in reality, a seascape reflecting the constant shifts in which traits are ecologically advantageous. This seascape changes endogenously, based on (1) the members of the population, and/or (2) interactions between members of the population and the abiotic environment. Every action that any organism takes changes the fitness landscape in which all other organisms are evolving. These changes last for an indefinite length of time.
For some research questions, this ecologically-generated fitness seascape is sufficient to produce the needed evolutionary dynamics. A number of digital evolution platforms use a fitness landscape based purely on ecological interactions and so may be appropriate in this context. Such systems include EcoSim (Gras et al., 2009), Symbulation (Vostinar and Ofria, 2019), Chromaria (Soros, 2018), and Geb (Channon et al., 1998). Other digital evolution systems, such as Avida (Ofria and Wilke, 2004), can be configured to function in this way but are not by default.
Other research questions require the presence of a more static fitness landscape in addition to the ecological seascape. Generally this landscape represents the traits that are necessary to survive and reproduce at all. It is easy to create simple fitness landscapes that we understand well due to their simplicity. However, fitness landscapes in biology tend to be relatively complex, and some evolutionary dynamics are driven by this complexity. Thus, in order to investigate these dynamics, we need a somewhat complex fitness landscape.
Even by themselves, complex ecological seascapes or static fitness landscapes can be unintuitive and yield unexpected results. When combined, these problems compound. Thus, it is advantageous to use systems where we have a good understanding of as many of the relevant fitness landscapes as possible. For example, we may want to study which ecotypes are best at invading a given niche. If we already know that a given ecotype is a common stepping stone on the way to the adaptation associated with the niche, we have a much better sense of what results we should be expecting to see. Thus, if we observe different results, we will be able to recognize that something unexpected is occurring and explore it further.
Because digital evolution systems have been studied from multiple perspectives, their fitness landscapes are often well understood. Indeed, many allow the user to select among multiple static and dynamic fitness landscapes of varying complexity (e.g., Avida Ofria and Wilke, 2004 and MABE Bohm et al., 2017). Thus, they allow for us to examine evolution and ecology in the context of a complex fitness landscape without sacrificing our ability to have intuition for and interpret the results.
Most digital evolution platforms have the capacity to support ecological research, but each has its own strengths and weaknesses. Just as it is advantageous for a community of researchers to use a range of model systems in the laboratory and field, it is also advantageous to use a range of digital platforms. The representation of individuals varies dramatically from platform to platform, making each a distinct substrate for evolution to act on. Every substrate—including biology on earth—has its own quirks. Thus, truly understanding the behavior of a specific system requires studying that system specifically. However, observing the same behavior across multiple distinct substrates provides strong evidence that a result generalizes.
Taken to its extreme, this argument might suggest that everyone should write their own digital evolution system. However, pre-built digital evolution platforms have a number of advantages (Bohm et al., 2017, 2019b; Lalejini et al., 2019), including the improved usability, reliability, and collective knowledge that comes from having a large base of users and contributors. In particular, they have the potential to be a good fit for biologists who may be uncomfortable writing their own code. An important caveat is that running experiments on many of these platforms often requires comfort with compiling custom software, navigating high-performance computing environments, or conducting programmatic data analysis, meaning that computational skills can still be an obstacle for biologists getting started with these systems. Nevertheless, standalone programs provide an easier entry point for many. Another benefit of standalone programs is that they provide greater consistency between experiments, making it easier to build upon the work of others and situate new data within the context of what is already known. This benefit strengthens once a critical mass of research has been done in a system and so the basic behavior is well documented.
Here, we survey the set of prominent digital evolution platforms currently in use (in addition to some historical ones) that have the potential to support ecology research. For consistency with (Vostinar et al., 2021), we limit our scope to systems that meet the following criteria:
1. Instantiates the evolution of populations of individuals in silico,
2. Supports implicit fitness,
3. Has a complex genotype-to-phenotype mapping due (at least in part) to the effects of ecological interactions between individuals, and
4. Has an effectively unlimited number of possible genotypes.
Perhaps the most popular of digital evolution system is the Avida Digital Evolution Platform (Ofria and Wilke, 2004). Avida has all of the properties that we argue are critical for the study of open-ended eco-evolutionary dynamics. Each organism in Avida is a self-replicating computer program, with a genome made up of computer instructions (see Figure 2). Experiments generally begin by seeding the population with a single program containing a sequence of code that copies each instruction in its genome into its offspring. Every time an instruction is copied, there is a small probability that it will mutate to be a different instruction. Organisms in Avida inhabit a lattice of cells, with each cell containing at most one organism. When an organism is done copying its program, the resulting offspring is placed in an adjacent cell, overwriting any previous occupant of that cell. This dynamic creates competition for space, which incentivizes organisms to copy themselves as fast as possible to reduce the chance of being overwritten before they reproduce. Organisms can increase their reproductive rate either by evolving to be more efficient or by evolving to perform computational tasks that the experimenter has associated with a bonus to CPU speed. Evolving these tasks is akin to DNA-based organisms evolving the ability to metabolize new resources. When organisms successfully complete these tasks, they receive bonus CPU cycles each update, meaning that they are able to execute more code per unit time. Since the Avida system has variation, inheritance, and differential reproductive success, these populations of digital organisms evolve by natural selection.
Figure 2. An organism in Avida. Genomes of organisms in Avida are sequences of simple computer code. Each organism has a virtual CPU that is capable of running the code in its genome. This virtual CPU contains some memory, which can be used to store numbers that the organism is operating on. The CPU executes the instructions in the genome in sequence. In this example, the organism is currently executing the “add” instruction, which takes the number in the first memory slot, adds it to the number in the second memory slot, and stores the result in the third memory slot. In addition to performing mathematical computations, the instructions in the genome can allow the organism to make a copy of itself and place that copy elsewhere in the environment. Thus, organisms in Avida are able to reproduce via self-replication.
The fitness landscape of the default environment in Avida is well-studied (Pakanati, 2015) and has been used in a large number of experiments. Moreover, it has some important structural similarities to fitness landscapes in which biological organisms are evolving. The tasks it rewards are computationally related to each other and span a range of complexities. While evolving the ability to do the simpler tasks is useful in its own right, they can also serve as building blocks for the more complex tasks (Lenski et al., 2003).
Avida also makes it easy to incorporate ecology by layering on a fitness landscape based on biotic interactions. By default, the resources associated with tasks in Avida are unlimited. However, we can introduce competitive interactions between organisms by limiting the quantity of these resources. This scarcity creates negative frequency-dependent selection as organisms compete for the resources. Any time an organism uses a resource it consumes a portion of it, making the ability to use that resource less valuable for all organisms. Avida also includes a number of other mechanisms by which organisms can influence each other's fitness landscapes, such as cross-feeding, parasitism, predation, and cooperation.
Ecosim is a well-established system designed for studying ecology in a predator-prey context (Gras et al., 2009; Mashayekhi et al., 2014a; Scott et al., 2019). There are two trophic levels: predators and prey (prey also consumes food, but this food is not represented by an agent). The motivation for including predator-prey dynamics in EcoSim was to create an evolutionary arms race in which both populations are continuously evolving, eliminating the need for a more explicit fitness landscape. Individuals in EcoSim can move around the world, and are controlled by an artificial brain model called a fuzzy cognitive map. Each individual also has a separate genome determining its physical characteristics.
In addition to its predator-prey dynamics, EcoSim is set apart by its support for speciation research. Reproduction in EcoSim is sexual, and occurs when two sufficiently genetically similar individuals in the same location choose to breed. Whether the individuals are sufficiently similar (i.e., whether they are part of the same species) is determined via a clustering algorithm. As a result, species gradually diverge from each other. EcoSim has been used for a variety of research on speciation (Golestani et al., 2012; Gras et al., 2015; Pour et al., 2017; Bhattacharjee et al., 2018), species area relationships (Devaurs and Gras, 2010; Mashayekhi et al., 2014b), and other ecological questions (Pour et al., 2015; Bhattacharjee et al., 2019; Scott and Gras, 2020).
Symbulation is a simpler system targeted at precisely understanding the evolution of interactions between hosts and endosymbionts (Vostinar and Ofria, 2019). Each agent's genome consists of a single number (its “interaction value”) between –1 and 1 that determines the extent to which it helps or harms its partner (host or endosymbiont). In addition to interactions between hosts and endosymbionts, which are in and of themselves a form of ecology, Symbulation supports having multiple endosymbionts within the same host. A variety of implicit ecological interactions occur in this scenario, although little research has been done on them to date. As its name implies, this platform lies on the border between simulation and digital evolution, and represents a rich yet tractable environment for exploring the complex game theoretic interactions that occur in microbiome communities.
MABE (Modular Agent-Based Evolver) is a flexible digital evolution research system designed to allow users to mix and match modules that represent different styles of genome, brain, fitness evaluator, and environment (Bohm et al., 2017, 2019b). MABE seeks to provide the best of both worlds by simultaneously being friendly to new users, facilitating testing hypotheses in multiple configurations, and promoting better software development practices. Thus, far, the only ecological research that has been published with MABE is focused on sexual selection (Bohm et al., 2019a), but the system allows multiple populations that can interact in any way that the user specifies. Because of its flexibility, however, MABE could be used to address any number of ecological questions.
Aevol is a complex digital evolution system designed to be a close analog to DNA-based genetics. Individuals in Aevol have genomes with four bases that go through processes mirroring transcription and translation (Knibbe et al., 2007; Batut et al., 2013). Ultimately, organisms produce a set of triangles that are overlaid on top of each other to approximate a continuous function. Fitness is determined by how closely the triangles match a target function specified by the environment. While this basic set-up does not inherently promote ecological interactions beyond simple competition for space, Aevol has been extended to support more interesting ecologies. In particular, Aevol has been used to study the evolution of cooperation via public goods (Frénoy et al., 2012; Misevic et al., 2012, 2015).
In Chromaria, agents are colorful creatures with appearance and behavior controlled by convolutional pattern-producing networks (CPPNs) (Soros and Stanley, 2014). In order to reproduce, these agents must find a region in which the background color matches the agent's color and “plant” themselves there, at which point they become part of the background. Consequently, ecological interactions are a core component of this platform, as new phenotypes literally create new ecological niches. Indeed, one of the hypotheses that Chromaria was designed to explore is the idea that ecological interactions are a necessary condition for open-ended evolution to occur. Research in Chromaria thus far has supported this hypothesis (Soros, 2018). Chromaria is unique among the systems described here in that the primary ecological interaction it features is facilitation (specifically commensalism), rather than competition or other antagonistic interactions. Thus, it is a promising platform for studying commensalism and its evolutionary implications.
DISHTINY (Distributed Hierarchical Transitions in Individuality) is a system in which agents communicate and cooperate to harvest spatiotemporally fluctuating resources (Moreno and Ofria, 2019, 2021). As a system designed for studying major evolutionary transitions, the organisms in DISHTINY can be far more complex and slower to execute than most of the other systems discussed here (often requiring a super-computer to execute), making it a less ideal choice for most research on eco-evolutionary dynamics. Populations in DISHTINY can exhibit a rich ecological community containing a variety of cooperative and competitive interactions. Cooperative interactions typically become so essential that individual cells in this community ultimately come together to form multi-cellular organisms, sacrificing cellular-level replication for reproduction of the multicellular organism. There is also preliminary evidence of ecological interactions between these multi-cellular organisms (Moreno and Ofria, 2021). In early versions of DISHTINY, all ecological interactions were mediated via a set of numerical genome values (Moreno and Ofria, 2019). Subsequent work has used agents controlled by evolved code (Moreno and Ofria, 2021).
Urdar is an artificial life platform for studying eco-evolutionary dynamics in a crossfeeding environment (Gerlee and Lundh, 2010). In contrast to the other systems we have discussed so far, Urdar has neither predator-prey dynamics nor movement, giving it the potential to be substantially more tractable for addressing questions that do not require such properties. Urdar consists of a population of organisms that process binary strings. Each organism is an update rule for a one-dimensional cellular automaton. On each evaluation, each organism receives a random string from the environment, processes it according to its rule, and places the resulting string in the environment. The increase in the string's Shannon entropy as a result of this transformation is the amount of "energy" that the organism was able to extract from the resource. Organisms that extract enough energy are allowed to replicate. This rule results in a system that self-organizes into a cross-feeding ecological community, with stable coexistence of multiple species promoted by negative frequency dependence.
Polyworld is one of the oldest standalone artificial life platforms, and includes ecological interactions at its core (Yaeger, 1993). Organisms in Polyworld are controlled by Artificial Neural Networks that use Hebbian learning over the course of each individual's lifetime. Organisms in Polyworld receive visual sensory input, move freely around the world, forage for food, and attack other organisms. They can also signal a desire to mate, which will occur if another nearby organism also signals this desire. Lastly, they can adjust their visual appearance. Each of these behaviors is associated with an output neuron; the activation levels of these neurons determine which behaviors are performed. Polyworld is an open-ended system for exploring the evolution of various life history strategies within a complex network of interaction. It is likely a particularly good choice for research that deals with within-lifetime learning. However, the complexity of the learning and interaction systems comes with trade-offs. For many questions, Polyworld's complexity makes it harder to understand the drivers of a given result, and some have suggested that the effect of learning may be disproportionately strong (Channon et al., 1998). Perhaps as a result, despite the inherent ecological dynamics built in to Polyworld, it has not been used for much ecology research.
Geb is a similar system to Polyworld, but removes lifetime learning from the mix, forcing all intelligent behavior to be the result of evolution (Channon et al., 1998). Organisms in Geb are controlled by recurrent neural networks encoded genotypically as context-free L-systems. The process of building the neural network from the genome mirrors development in nature (Channon and Damper, 2000). Interactions are also more constrained in Geb than in Polyworld, although they are still complex enough to be potentially challenging to analyze. Each time step, organisms choose between five actions: 1) attempt to mate with the organism in front of them, 2) attempt to fight the organism in front of them, 3) turn clockwise, 4) turn counterclockwise, or 5) move forward. This set-up yields complex movement-based interactions between agents, often involving spinning in circles while attempting to mate and fight (Channon et al., 1998). Because analyzing the ecology of Geb requires analyzing the movements of its agents, little ecological research has been done in it to date. However, it could be especially effective for addressing questions about competitive interactions in movement ecology.
Perhaps the first digital evolution platform to foster a complex ecology, Echo was proposed by Holland (1995). The central currency in Echo is a set of limited resources. Genomes in Echo are made up of these resources, and individuals can only reproduce when they have obtained the necessary resources to copy their genome. To obtain these resources, individuals can uptake them directly from the environment, take them from another organism via combat (akin to predation), or trade another organism for them. Interactions between individuals in Echo are controlled by the genome, which is divided up into a set of “tags” and “conditions.” An individual's tags are the information about it that other individuals can sense and make decisions based on. An individual's conditions determine how it will behave, and are secret from others. Echo was used to study the generality of ecological community assembly patterns (Hraber and Milne, 1997; Hraber et al., 1997) and to provide early confirmation that digital evolution systems could produce complex ecologies (Smith and Bedau, 1997).
Tierra, the precursor to Avida, was the first digital evolution system (Ray, 1991) to exhibit strong ecological dynamics. As in Avida, individuals in Tierra are self-replicating sequences of assembly-code-like instructions. Unlike in Avida, however, these individuals all exist in one large “soup” of RAM. While individuals do not have write-access to regions belonging to other individuals, they are able to read and execute those areas of memory. This configuration naturally allows for the evolution of parasitism, in the form of programs that use other programs' self-replication code, rather than maintaining their own. In principle, other ecological interactions could occur in Tierra as well, although they can be challenging to identify. In practice, though, the ecology of Tierra tends to consist of only one type of parasite and one type of host at a time, and the dynamics will often stagnate (Ray, 1994).
While evolutionary computation is not a single stand-alone research platform, it is included here because we argue that it is an underappreciated class of model systems for doing eco-evolutionary research. Evolutionary computation falls approximately between simulations and digital evolution along the continuum in Figure 1. While there is overlap between the categories of digital evolution and evolutionary computation, three important caveats are that many evolutionary computation systems (1) allow for no direct interaction among individuals in a population, (2) assign fitness based on an explicit fitness function, and (3) ignore realism or biological plausibility in order to generate high-quality solutions as rapidly as possible. As such, they rarely exhibit ecological dynamics (with notable exceptions discussed below) and fail to meet key criteria for inclusion in this review.
Evolutionary computation is a sub-field of machine learning in which principles from evolutionary biology are applied to digital populations in an effort to solve computational problems. The populations are composed of candidate solutions to a target problem and the fitness of these solutions is evaluated based on how well they solve that problem. Individuals are selected to reproduce based on how well they solve problem instances and their offspring are mutated. The general category of evolutionary computation encompasses a number of more specific types of evolutionary algorithms, including genetic algorithms, genetic programming, and evolutionary strategies.
More advanced evolutionary algorithms do rely on underlying ecological interactions (Dolson et al., 2018a,b). Evolutionary computation researchers have devoted substantial attention to understanding how to promote coexistence among lineages exploring different regions of a fitness landscape (Goldberg et al., 1987; Mahfoud, 1995; Mouret and Doncieux, 2009; Pugh et al., 2015). Promoting diversity in an evolving population is important for evolutionary computation because it allows part of the population to sit on top of a fitness peak (referred to as “exploitation” in machine learning) while the rest of the population continues searching the rest of the fitness landscape (referred to as “exploration” in machine learning). The techniques that they have developed for achieving this goal all map back to ecological dynamics (Dolson et al., 2018b). Thus, evolutionary algorithms can be used as a testing ground for high-level hypotheses about ecological coexistance mechanisms and their interplay with evolutionary dynamics.
A number of highly effective evolutionary-computation techniques owe their success to ecological dynamics. These largely fall into four categories: niching/speciation (e.g., Goldberg et al., 1987; Stanley and Miikkulainen, 2004), parent selection (e.g., De Jong, 1975; Mahfoud, 1992), dividing the population into subpopulations (e.g., Hu et al., 2005; Hornby, 2006), and adjusting the objective function to favor diversity and/or novelty (e.g., Mouret and Doncieux, 2009). In particular, fitness sharing and Eco-EA, both of which fall into the niching/speciation category, show promise as model systems for studying ecology.
Fitness sharing, one of the first ecology-based diversity maintenance approaches, is by far the simplest of these techniques (Goldberg et al., 1987). Under this regime, the fitness of each individual is reduced based on the number of similar individuals in the population. As such, constant pressure to diversify is imposed. Coexistence dynamics under fitness sharing are mathematically identical to coexistence dynamics under modern coexistence theory in ecology (Dolson et al., 2018b).
Eco-EA is an approach, originally built on top of Avida, to solving complex problems by associating limited resources with simpler challenges that can be used as stepping stones (Goings and Ofria, 2009; Goings, 2010; Goings et al., 2012). These challenges can be sub-problems of the larger problem, individual test cases, or other related tasks that may be used as components of a global solution. Resources remain plentiful until a solution to the corresponding sub-problem is discovered. When a population first solves a sub-problem, the accumulated resource dramatically boosts the fitness of individuals that use it. As the number of individuals using the resource increases, it become less valuable. As long as there is some cost to attempting to use each resource, negative frequency dependence fosters small, but stable sub-populations capable of solving each sub-problem. Coexistence dynamics under fitness sharing are mathematically identical to coexistence dynamics under R* theory in ecology (Dolson et al., 2018b).
In general, evolutionary computation systems are a particularly good fit for eco-evolutionary questions centering around ecology's role in facilitating the evolution of solutions to complex problems. They run quickly, feature a variety of well-understood but non-trivial fitness landscapes, and instantiate a wide range of simple ecological dynamics.
For ease of reference when attempting to choose a system for research, we have assembled a summary table (see Figure 3) comparing the extent to which each system supports various research directions. Note that most of these systems are under active development, and many of these dynamics could easily be added to more systems in the future.
Figure 3. Comparison of digital evolution platforms. This table rates digital evolution platforms on the extent to which they can be used to study specific dynamics. A rating of “central” indicates that a dynamic is a critical component of a system and has likely been studied in that system. A rating of “optional” indicates that the dynamic is not present by default but can be turned on. A rating of “incidental” indicates that the dynamic may occur in the system, but likely not in a particularly straightforward way. A rating of “impossible” indicates that a dynamic is excluded from that system, as it currently exists. The speciation rating specifically refers to speciation in the context of sexual recombination. The “Complex Dynamics” and “Simple Dynamics” columns reflect each system's position along the continuum in Figure 1. “Complex Dynamics” indicates the extent to which the system supports dynamics toward the rich and natural end of the spectrum. “Simple Dynamics” indicates the extent to which the system supports being configured to be simple and tractable. Note: Endosymbiosis is rated "central" to Tierra, due to the ubiquitous evolution of parasites. However, these parasites are unconventional in that they do not disproportionately harm the host.
While the first major discoveries to come out of digital evolution research fell squarely into the category of pure evolutionary theory (Wilke et al., 2001; Lenski et al., 2003), simple ecological dynamics were soon explored. Cooper and Ofria demonstrated that limiting the quantity of resources available was sufficient to evolve a stable and diverse ecosystem (Cooper and Ofria, 2003). They set up this experiment by associating computational tasks in Avida with a resource that had a limited availability and inflow rate. Every time an organism performed a task and received the reward, it used up some of the resource, resulting in a situation where digital organisms performing tasks is effectively equivalent to biological organisms metabolizing chemical resources. This arrangement created negative frequency dependence; tasks being performed by fewer organisms were more valuable, just as inhabiting any underutilized niche in an ecosystem is advantageous. In prior experiments, where resources were unlimited, some phenotypes in the population were simply more fit than the others; the fitnesses did not change over time. Layering the shifting biotic fitness seascape and the static abiotic landscape created pressure for adaptive radiation and subsequent stabilizing selection on the different ecotypes. These results were consistent with mathematical theory being contemporaneously developed by theoretical ecologists (Chesson, 2000), and demonstrated that this theory holds up when populations are allowed to evolve.
Chow et al. expanded on this work, exploring the continuum from environments with scarce resources to ones where resources were effectively unlimited (Chow et al., 2004). They found that ecotypic diversity peaks at intermediate levels of resource availability. Resource availability, in this context, is roughly analogous to primary productivity; it determines the carrying capacity of each niche. Previous field research had found that species richness tends to be highest in environments with intermediate productivity, but there had been a lack of consensus over which properties of environments were driving this effect. Chow et al. replicated this finding in Avida, purely by varying the amounts of each resource that were present in the environment. This result demonstrated that limited resources alone are sufficient to explain the productivity-diversity relationship. This ability to isolate potential drivers of a phenomenon and determine which ones are sufficient to produce a given result is a major strength of individual-based digital systems.
The finding that competition can drive adaptive radiation has since been corroborated in many other digital ecosystems. Research in Urdar also found stable coexistence between species specialized on different types of food, although in this case the negative frequency dependence may have partially resulted from mutualism in addition to competition (Gerlee and Lundh, 2010). Similarly, in Ecosim, different predator species evolve to specialize on different types of prey, leading to physical and behavioral differences (Mashayekhi et al., 2014a). Eldridge and Kiefer found further evidence of competition-driven niche partitioning, this time in the context of agents evolving to vocalize at different frequencies (Eldridge and Kiefer, 2018). Lastly, continuing with the perspective that all of evolutionary computation can be viewed as a model system for studying biology as well, the widespread success of niching algorithms is a clear final confirmation of the generality of this result (Dolson et al., 2018b).
These initial digital eco-evolutionary dynamics papers tell a compelling story about how evolution guides the long-term assembly of ecosystems. However, ecology also effects long-term evolutionary trends. Walker and Ofria explored how continued evolutionary innovation in Avida plays out differently within ecological communities assembled at different levels of productivity (Walker and Ofria, 2012). Previous research on the connection between population diversity and evolutionary potential (as measured by the probability of evolving complex traits) suggested that there is generally a positive correlation between these two variables. This hypothesis makes sense, as a more diverse population is necessarily more spread out across the fitness landscape, increasing the probability that some members of the population will be close to an adaptive peak. However, Walker and Ofria found that the relationship between diversity and evolutionary potential is more complicated; evolutionary potential peaked at a different level of productivity than diversity did. Follow-up work in Avida has yielded consistent results (Dolson and Ofria, 2017; Dolson et al., 2017). This observation is an example of the difficulty of predicting eco-evolutionary feedbacks a priori. Digital systems give us the fine-grained control necessary to find such deviations from our expectations.
So far, we have discussed ecosystems assembled purely on the basis of competitive interactions. While these model ecosystems are useful for gaining basic insights into eco-evolutionary dynamics, ecosystems in nature involve a wide variety of different types of interactions among organisms. These include various forms of facilitation, in which species make it easier for other species to survive.
Among the simplest of facilitation interactions is cross-feeding (also called syntrophy), wherein organisms of one ecotype feed off of byproducts generated by organisms of a different ecotype. Yet, even such a simple interaction can have unpredictable eco-evolutionary dynamics. Johnson and Wilke built an incredibly simple cross-feeding ecosystem in Avida by providing two resources, each of which created the other as a byproduct when it was metabolized (Johnson and Wilke, 2004). This type of environment produces an even stronger form of stabilizing selection than occurs with purely competitive interactions; increased competition for one resource is combined with a decrease in the production of that resource (assuming a fixed population size). The result is a pair of ecotypes that exhibit either Lotka-Volterra-like oscillatory dynamics or a more stable equilibrium. Which one of these regimes occurs appears to be largely determined by stochastic factors. More complex hierarchical cross-feeding food webs have also been constructed in Avida (Yedid et al., 2008, 2009, 2012) and Urdar (Gerlee and Lundh, 2010). Additionally, more recent digital evolution research has shown that the existence of seasonality or temporal niches can facilitate the evolution of stable cross-feeding interactions (Rocabert et al., 2017).
Cross-feeding is a relatively simple form of facilitation as it is not susceptible to cheating. Cooperation, in which cheating is a possibility, poses a more challenging evolutionary problem. Nevertheless, cooperative species interactions play a critical role in many ecosystems (Frederickson et al., 2005; Goudard and Loreau, 2008). Understanding what evolutionary pressures can lead to such scenarios is critical to understanding this component of ecosystem assembly, but is, again, challenging to address in vitro or in vivo. Digital evolution has facilitated a deeper exploration of the intricate mechanisms that allow for the evolution of cooperative behaviors.
For example, based on inclusive fitness theory we would expect that genes that code for altruistic behavior should be more evolutionarily successful if they more effectively direct that altruistic behavior at other individuals with the same gene. Clune et al. tested this hypothesis in Avida by providing various altruistic instructions that could be included in organisms' genomes by mutation (Clune et al., 2010). When organisms executed these instructions, they would lose some of their CPU cycles and a neighboring organism would receive some number of CPU cycles. The neighbor that received this benefit was determined by the specific altruistic instruction that was used. The available instructions fell along a continuum of how accurately they could target the benefit at organisms likely to be altruistic back. The least accurate (other than the random controls) was kin targeting, which donated the CPU cycles to a parent or offspring. Slightly more accurate was similarity targeting, in which the organism receiving the CPU cycles had to have a genome that had a high level of overall similarity to the donating organism (as measured by the quality of the best possible sequence alignment between their two genomes). Lastly, there was greenbeard targeting, named for the idea that altruistic organisms can display visual markers (like a green beard) that are tied to their altruism and thus provides a reliable signal to other organisms (Hamilton, 1964; Dawkins, 1976). With this method, only other organisms that have previously executed the greenbeard altruism instruction can receive benefit from an organism executing it. Theoretically, this greenbeard approach should be the most accurate form of altruism targeting, as, in the Avida implementation, it literally allows organisms to help only those that express the same gene. Surprisingly, however, while similarity targeting was favored over kin targeting, greenbeard targeting was not selected over the other forms. Further experimentation revealed that the key difference was that greenbeard targeting did not provide a way to distinguish mildly altruistic organisms from highly altruistic organisms (those that executed the instruction repeatedly). This result may explain the fact that most greenbeard altruism observed in nature tends to relate to binary rather than continuous forms of altruism. Indeed, when the greenbeard instruction was adjusted to only benefit other organisms exhibiting the same level of altruism, it did become the preferred mechanism.
Some traits, such as releasing a beneficial chemical into the surrounding environment (i.e., public goods), do not have a specific other organism being targeted. For these traits to be evolutionarily favored, there must be some mechanism for ensuring that their benefit primarily goes to kin. In many cases, this guarantee can come from living in a sufficiently spatially-structured environment, such that the majority of neighboring organisms are kin. Another option is quorum-sensing, wherein altruistic organisms signal their presence to each other, allowing them to base their actions on the number of kin that are present (Diggle et al., 2007). Beckmann and McKinley demonstrated that digital organisms in Avida are capable of evolving quorum sensing if allowed to pass messages to each other (Beckmann and McKinley, 2009). In collaboration with biologists, they were then able to use this system to understand how bacteria might evolve resistance to efforts to interfere with their quorum sensing (Beckmann et al., 2012). Vostinar (née Johnson) et al. expanded on this research, finding that not only is the evolution of quorum sensing in Avida possible, it also increases the range of conditions in which altruism will evolve and the extent to which it is beneficial (Johnson et al., 2014). This work provided the basis for a more precisely targeted digital system that directly modeled quorum sensing in a species of bacteria (Vostinar et al., 2018).
The evolution of public goods cooperation has also been studied in Aevol (Misevic et al., 2012). Taking advantage of Aevol's rich genome system, Frenoy et al. studied the interactions between genetic architecture and the stability of cooperation in the form of secreting a public good (Frénoy et al., 2013). They found that in populations where cooperation was robust, the genes governing public good secretion were interconnected with genes supporting the organism's basic metabolism. In essence, the populations had evolved a genetic architecture that made the evolution of cheating challenging. This phenomenon seemed to occur more frequently when cooperation was costly. These findings highlight the value of studying these questions in complex digital systems; such behavior is only possible due to Aevol's elaborate genomes.
Subsequent research in Aevol investigated the effect of a population's shape within physical space on the evolution of public goods cooperation (Misevic et al., 2015). Specifically, they compared populations in long narrow worlds to populations in more evenly-proportioned worlds. Counter-intuitively, despite the fact that a long and narrow world should reduce interactions between cooperators and cheaters, evenly-proportioned worlds promoted greater levels of cooperation.
Predation is a fundamentally different interaction than those we have discussed so far, and a critical component of many ecological communities in nature. There is an asymmetry in predator-prey relationships that is not necessarily present in competition or facilitation, which results in a system with fundamentally different behavior (Johnson and Wilke, 2004). Studying predation is complicated by the fact that most predators need to be motile in order to reach enough prey to sustain themselves. As such, systems that allow predation generally allow organisms to move around the world.
Of the systems considered here, EcoSim is the one to which predation is most central. Thus, it is unsurprising that EcoSim has been used for a number of interesting studies related to predation. For example, Khater et al. addressed the non-lethal impacts of predators on prey (Khater et al., 2016), showing that the presence of predators has a strong impact on evolved prey behavior. Prey that coexist with predators spend a substantial portion of their time evading predators, leading them to forage dramatically less than prey in the absence of predators. These findings are consistent with prior field research, and support the idea that non-lethal impacts of predators on prey play an important role in structuring multi-trophic communities.
In related work, Khater et al. investigated the impact of predator removal (Khater et al., 2014), finding evidence that it can have a variety of impacts. In particular, the decrease in predator-evasion behavior by prey dramatically alters their spatial distribution. The reduction in movement results in reduced gene flow among prey, ultimately producing higher levels of genetic diversity. Interestingly, this study also involved a condition in which predators were added to a naive population. If this introduction was performed too late into a run of the system, the predator population was generally unable to stabilize, suggesting that, even in the absence of predators, prey evolved properties that posed an increased challenge to the predators.
Predation has also been studied in Avida. Here, movement instructions are enabled, as are instructions that give them sensory information about the environment, making for far more complex (and thus slower) experimental worlds. Prey organisms forage for abiotic resources in the environment and store them in their cells (initial foraging research was conducted by Walker, 2011). Predators can attack prey and receive a portion of their stored resources by executing a specific instruction while facing them.
Because of the huge impact that movement has on the strategies evolved by digital organisms, a lot of predation research in Avida deals with the evolution of movement strategies and sensory behaviors. As it turns out, these behaviors profoundly affect and are affected by the presence of predators. Wagner et al. (2020) compared the behavior of prey evolved with and without a co-evolving population of predators and found that predators force the prey to explore a much wider space of strategies. They also evolved to make better use of their sensory capabilities, which in turn made them better at foraging as well. We hypothesize that the fact that ecosystems often include a variety of related-but-different survival pressures, many of which consistently become more challenging as a population adapts to them, makes them particularly effective at promoting the evolution of more complex organisms.
O'Donnell et al. expanded on some of these questions by investigating properties of prey communities that make them better at evolving to avoid novel predators (O'Donnell et al., 2014). They looked at two factors: the amount of standing genetic variation in a prey population, and the evolutionary history of that population (i.e., whether the lineages had been exposed to different predators in the past). Surprisingly, they found that standing genetic variation had little impact on the extent to which the prey population was able to evolve resistance to predators. Evolutionary history, on the other hand, had a dramatic effect. Despite the fact that the predators used in the experimental phase were from a completely different population than the ones that the prey had co-evolved with, prey populations that had historically been exposed to predators were substantially more likely to evolve resistance to novel predators.
Further research on the specific techniques used to avoid predators has addressed the evolution of mimicry and prey grouping. Mimicry requires a fascinating and complex set of ecological interactions: for example, a poisonous prey species that signals its toxicity to potential predators, a predator species that responds to this signal, and an additional non-poisonous prey species that mimics the signal so that predators will avoid it as well. This set-up creates an interesting form of density-dependence, where the efficacy of the mimicry decreases as the relative abundance of the mimic species increases. Lehmann et al. created such an ecosystem in Avida, and studied the scenarios under which it is stable (Lehmann et al., 2014). They found that the degree to which the toxin harms predators and the accuracy of the mimicry are both important variables in the evolution of a stable population of mimics. The more harmful the toxin, the greater the density of mimics the system can support.
Prey grouping is a more complex predator avoidance strategy, as it requires some degree of coordination among prey organisms. Biswas et al. used Avida to untangle the mechanisms that drive the evolution of such behavior (Biswas et al., 2014). Two different potential mechanisms had previously been proposed: dilution and predator confusion. Dilution is the simple observation that being in a group can lower an individual's chances of being selected by predators for attack, while predator confusion is the idea that predators may have trouble visually perceiving individual prey to hunt if those prey are in a group. To study this phenomenon, the authors observed the extent to which prey organisms evolved grouping behavior under various conditions. While the presence of predators did significantly increase prey grouping behavior, the effect was no different when predators' visual sensors were inhibited. This result suggests that the dilution effect is sufficient to evolve prey grouping behavior, and that the more complex explanation of predator confusion is unnecessary.
The full spectrum of symbiotic interactions (e.g., parasitism, commensalism, mutualism, etc.) contains a variety of different dynamics. While some of these dynamics fall into previously-discussed categories, they are worth considering collectively due to their fluid nature. When species are living in close association with each other (symbiosis), they can rapidly shift between different types of interactions in response to changing evolutionary or ecological forces. As a thorough review of work on studying symbiosis with digital evolution has already been performed (Vostinar et al., 2021), we will restrict this section to a few highlights and direct the reader to Vostinar et al. for further detail.
Parasitism is the symbiotic interaction that has received by far the most attention thus far. There is increasing evidence that parasites play a critical role in many ecological communities (Lafferty et al., 2006). Conveniently, parasites fit naturally into Avida. Indeed, the evolution of parasitism is a major finding of research in Avida's precursor, Tierra (Ray, 1994). Since the uncontrolled evolution of parasites in Tierra led to long-term ecological stagnation, Avida implements parasites more intentionally. Like normal organisms in Avida, parasites are a sequence of simple computer code instructions. Unlike normal organisms, parasites cannot survive in a cell on their own. Instead, they inhabit the same cell as a normal organism and steal its CPU cycles (i.e., when the host organism should have been allowed to execute an instruction, the parasite may instead execute one from its own genome). For most parasite experiments in Avida, hosts are required to perform at least one task in order to replicate. Parasites are able to infect hosts that perform the same tasks as them. Thus, there is pressure for hosts to evolve the ability to perform novel tasks and lose the ability to perform the old tasks. This set-up promotes the generation and maintenance of diversity (Zaman et al., 2011), and ultimately leads the hosts to evolve to be more complex than they would otherwise have been (Zaman et al., 2014).
The parasitism-mutualism continuum has been addressed in other digital systems as well. Most notably, Symbulation is designed to simplify the study of these topics by providing a simpler and more targeted platform (Vostinar and Ofria, 2019). Symbulation was recently used to demonstrate that spatial structure has an entirely different impact on host-parasite co-evolution than it does on other forms of potentially-cooperative co-evolution (Vostinar and Ofria, 2019).
All of the ecological forces mentioned above can, of course, be combined. Such studies can quickly become too complex to understand the results of, so it is important to take care in experimental design. However, a number of interesting directions for research on eco-evolutionary dynamics concern the network of interspecific interactions that defines an ecological community. For such lines of research, combining different drivers of ecology in a single experiment is necessary. A number of ideas for approaching such questions have been laid out by Fortuna et al. (2013), and some are already being put into practice, with promising results (Strona and Lafferty, 2016).
Most of our pressing concerns about ecosystems on Earth stem from the fact that many of them are currently facing novel perturbations. Biological data tend to be too limited to allow us to fully understand how an ecological system will be affected by particular perturbations. Digital systems, on the other hand, cannot easily model a specific, real-world ecosystem. In conjunction, however, the combination of natural and digital systems can help us dramatically improve our expectations about the results of a particular perturbation in a natural environment.
The most dramatic types of perturbations an ecosystem can experience are mass extinction events. Mass extinctions are divided into two categories: press and pulse (Erwin, 1998). Press extinctions are those that are induced by a prolonged stressor, to which adaptation is theoretically possible. In Avida, press extinctions can be induced by lowering resource inflow rates to low levels (Yedid et al., 2008). Pulse extinctions, on the other hand, are those that occur too quickly and too intensely for populations to adapt to. In Avida, pulse extinctions can be induced by directly killing a high percentage of the population (Yedid et al., 2009).
Part of the reason that extinctions can be so damaging to ecosystems is that most ecological communities are highly interdependent (Roopnarine et al., 2007). While communities evolved via adaptive radiation in a static environment would almost certainly change if they lost a member, they lack the level of interdependence that a food web creates. As such, understanding the impact of mass extinctions on an ecological community requires us to assemble a more complex community. Most of the research on mass extinctions in Avida has used a complex cross-feeding environment that functions similarly to a trophic pyramid (Yedid et al., 2008, 2009, 2012). In this environment, simpler tasks produce byproduct resources that can be metabolized by performing more complex tasks. Thus, as in most ecological communities, species at higher trophic levels (which are often more complex) are dependent on species at lower trophic levels.
In a press extinction, this dependency means that the most complex ecotypes are generally lost from the population (Yedid et al., 2008). If the amount of resources entering the lowest trophic levels is decreased, less of the byproduct resources can by produced, with a cascading effect across all trophic levels. This deficit percolates up through the trophic pyramid, resulting in minimal resource available for the highest level. What are the long-term of effects of such episodes on a community? Yedid et al. studied the process of regaining complex traits after the press event is over, and found that ecological communities that contained ecotypes with the ability to perform the most complex trait were more likely to re-evolve it than communities that never had such functionality (Yedid et al., 2008). While the mechanisms behind this dynamic were complex and varied, many of them are also present in biological ecosystems, suggesting that this result might generalize.
However, a follow-up study by Yedid et al. (2009) showed that recovery from press extinctions still takes a long time, particularly at higher trophic levels. Recovery from pulse extinctions, on the other hand, occurs much more rapidly. This tendency is believed to be the result of press extinctions providing an alternative selection regime that encourages the loss of more complex traits in favor of simpler traits that will more reliably yield a reward. The authors also observed some parallels between the results of this experiment and observations from the fossil record, further supporting the idea that these same dynamics may occur in biological ecosystems. Further analysis of the phylogenies of populations subjected to these perturbations revealed that stronger perturbations, particularly press events, result in a substantial loss of phylogenetic diversity (Yedid et al., 2012).
Similar research conducted with only a single trophic level suggests that the use of multiple trophic levels in Yedid et al.'s work was important to their results. Communities evolved in an environment in which computational tasks were associated with limited resources and then subjected to extreme bottlenecks (effectively strong pulse extinctions) were able to regain their original levels of consumption of each resource relatively quickly (Olson et al., 2013). This finding is in contrast to the delayed recovery of the most complex task observed in the cross-feeding set-up. Interestingly, although the post-bottleneck communities fulfilled all of the same functions as the original communities, the ecotypes present often partitioned resources very differently. This result reflects the fact that many distinct sets of ecotypes are able to stably co-exist in Avida under limited resources.
A much milder form of perturbation is a localized disruption of some regions within the environment, but not others. Such a scenario is commonly observed in nature in the form of anthropogenically fragmented ecosystems. Often, governments will set aside some regions as biological reserves, where the native ecosystem will, theoretically, be undisturbed. Making such choices determines the spatial structure that populations in this environment will have going forward, a property known to have a dramatic effect on evolutionary dynamics (Tomassini, 2005). While this topic had received a great deal of attention from ecologists, there is a relative lack of knowledge of how evolutionary considerations should inform such decision-making. Some preliminary investigation of this question has been carried out in Avida (Dolson et al., 2016).
A number of large-scale patterns have received a great deal of attention in ecology. Many of these patterns, such as species-area relationships, exist in the form of scaling laws that describe how two related variables collectively change. Others are patterns in the distribution of a variable of interest, such as species-abundance curves. Traditionally, these patterns have existed squarely within the realm of pure ecology. However, as evolution is the ultimate source of ecological variation, studying them from an evolutionary perspective can provide relevant insight. A number of digital evolution experiments have done exactly that.
Species-area relationships are curves relating the size of a region to the number of species found there. Their general shape is remarkably consistent across ecosystems. Thus, an obvious question to ask in the context of a digital ecological community is whether it conforms to this rule. In Echo, the observed species-area curves were positive, but were not a close match to the curves observed in nature (Hraber et al., 1997). Interestingly, this study also examined the species-area relationship in a neutral version of echo, in which all interactions were random. Although the neutral model was not a better approximation of nature, it did behave somewhat differently from standard Echo, suggesting that species interactions do play a role in structuring the species-area relationship.
Mashayekhi et al. undertook a more detailed investigation of species area relationships in EcoSim (Mashayekhi et al., 2014b). They measured species-area relationships under a variety of conditions and using a variety of sampling designs. They compared their results to a variety of mathematical functions that have been proposed to describe the specific shape of species area relationships. Functions in the power function family consistently performed best, although no one function was best across all circumstances. In particular, sampling scale was important in determining which function was the best fit. Additionally, they found a direct relationship between the slope of the species-area curve and the level of beta diversity (variation between spatial locations) in the environment.
Species abundance distributions are another commonly studied macroecological pattern. They are consistently right-skewed, indicating a large number of rare species and much smaller numbers of species that are more common. These patterns were also studied in Echo and EcoSim. Both the traditional version of Echo and the neutral model were found to exhibit species abundance distributions qualitatively similar to those observed in nature, although the neutral model had substantially fewer rare species (Hraber et al., 1997). EcoSim also produces species abundance distributions that are consistent with those observed in nature across a wide variety of spatial scales (Devaurs and Gras, 2010).
Clearly, digital evolution is a powerful tool for studying ecology. Nevertheless, there are a few challenges that it is important to be aware of when applying it:
Many digital evolution platforms have a hard limit on the size of the population they can contain. Often, this restriction is necessary due to resource constraints. However, it is important to be aware that doing so imposes competition for space. Moreover, this competition may occur on a different level than other competitive interactions (i.e., it may be global whereas other interactions are local, or vice versa). Such a discrepancy is not inherently a problem, but it can complicate the interpretation of results, particularly in the case of research on local competitive interactions.
Some ways of implementing space limits are more realistic than others. Density-dependence, in which fitness decreases as population size increases, is a common and important dynamic in ecology (Hendry, 2019). This effect can be achieved by decreasing reproduction rates as a pre-defined carrying capacity is approached. A more organic implementation of density dependence is to make the population dependent on a highly limited resource (Zaman et al., 2011).
When studying feedbacks between evolution and complex ecological networks, it is often desirable to allow the interactions in the network to evolve. However, representing interactions in a genome is not as straightforward as it may initially seem. It is tempting to create a section in the genome for each type of agent; the contents of these sections would control how a given agent interacts with each other type of agent. Unfortunately, this approach is not viable if there are more than a few species, as selection on the interactions rules becomes weaker as the number of agent types increases. This weakened selection causes genetic drift to be the primary driver of most interactions, producing what is most likely a very different eco-evolutionary regime than what generally occurs in nature.
The simplest approach to building interaction networks that do not experience this problem is to allow the environment to mediate them. Environment-mediated interactions can be achieved through resource competition and/or sharing, the implementation of laws of physics, or an artificial brain that can perceive other agents and choose actions based on its perception. In all of these cases, agents affect each other without needing to specifically evolve rules for how to do so.
A promising more general solution to this problem is the idea of tag-based referencing (Spector et al., 2011; Lalejini et al., 2021; Moreno and Ofria, 2021; Moreno et al., 2021). In this paradigm, possible events that an individual might experience, such as interacting with an individual of another type, are assigned “tags.” These tags are sequences of 0 and 1 s that are matched to corresponding tags in the individual's genome, which in turn determine which region of the genome controls the individual's response to the event. Importantly, tag-based referencing can be set up to allow the same response to be associated with multiple events. Thus, a population is able to evolve only as many unique interactions between species as it needs. Because tags are also capable of evolving, stabilizing selection is required to keep a given response associated with a given tag. Thus, in a circumstance where selection is not strong enough to prevent an interaction from being controlled by drift, selection will also likely not be strong enough to keep the response associated with the tag at all. Interestingly, this approach is similar to how interactions worked in John Holland's early Echo model (Holland, 1995). While this solution has not been used much recently, we believe that it is currently the most promising approach to implementing arbitrary evolvable interactions.
Systems for studying evolution and ecology exist along a continuum of biological richness and realism, from mathematical models on one extreme to field research on the other (see Figure 1). Across this continuum, there is an inherent trade-off between the richness of the dynamics in a system and the ease of untangling those dynamics. While digital evolution systems as a whole sit near the middle of this continuum, different digital evolution systems fall at different points along it. Thus, the same factors that one might consider when choosing digital evolution over other research environments should also be considered when choosing among digital evolution platforms.
Within the space of digital evolution platforms, it can often make sense to start with the simplest possible system that can display the dynamics of interest. Anecdotally, it is rare to conduct a digital evolution experiment and find a simpler result than expected. The opposite, however, is quite common. Conducting initial experiments in a simple system facilitates rapid discovery of the most important mechanisms and parameters. Subsequently, scaling up to a more complex system can provide the necessary biological richness to validate and deepen understanding gained with simpler systems. For an example of this approach, compare (Moreno and Ofria, 2019) with (Moreno and Ofria, 2021). Fortunately, many digital evolution systems facilitate such work by supporting a range of complexity levels via changes to their settings.
Beyond the richness vs. tractability trade-off, other important considerations in selecting a system include: 1) which types of ecological interactions it allows and promotes, 2) ease of use, and 3) the extent to which it has been used to address similar questions. This last point may seem counter-intuitive; as scientists, we are often encouraged to constantly seek out novelty. However, when first examining a new corner of a digital evolution platform, there is generally some “digital naturalism” (Fahmy, 2014) style work to be done. Every system has quirks that need to be understood before meaningful experiments can be conducted. There is value in this work, but if the goal is to answer a specific question it is generally more efficient to study it in a system where these quirks are already understood.
The range of promising future directions for ecology research in digital evolution platforms is as vast as the set of open questions in eco-evolutionary theory. However, there are some specific directions that we think this approach is particularly well-suited to:
Ecological theory is often developed with the simplifying assumption that evolution—at least in the sense of the evolution of novel traits and new species—is not occurring. Sometimes this assumption is justified with the argument that all species have already achieved optimal fitness. However, based on the trajectories of fitness even in incredibly simple systems (Wiser et al., 2013; Wiser, 2015), it seems unlikely that this justification is valid. Furthermore, the changing seascape of fitnesses in any ecological system will ensure that even a seemingly stable fitness peak does not remain so forever. Thus, much of ecological theory could benefit from being tested in a system that relaxes these assumptions, such as digital evolution. Indeed, much of the work reviewed in this paper stemmed from efforts to do exactly that, often yielding interesting and counter-intuitive discoveries in the process (Bohm et al., 2019a; Vostinar and Ofria, 2019).
A particularly likely source of discrepancies between theory and nature is that ecological theory often requires making assumptions about the distributions of variables of interest. In some cases, distributions that seem like reasonable null models have proven to differ in important ways from the distributions observed in nature (Lurgi et al., 2016). Because evolutionary processes play a key role in generating many of the patterns ecologists study, digital evolution platforms can, in some cases, produce distributions of these variables that better match those observed in nature.
In a recent critique of current research on eco-evolutionary dynamics, Hendry pointed out the importance of moving beyond research that focuses on a single species, and instead studying eco-evolutionary dynamics at the community level (Hendry, 2019)1. Relatedly, the extent to which selection operates at a community level was recently highlighted as an unsolved problem in ecology (Loreau, 2020). We heartily agree with the importance of this direction. Most long-term practical applications of eco-evolutionary theory —such as steering evolution in human microbiomes, pathogens, or synthetic ecosystems —require an understanding of how evolution will proceed within an entire community, not a single species within that community.
One of the primary obstacles to understanding community-level evolution is the sheer complexity of the dynamics involved and our lack of theoretical frameworks for understanding them. Even given a world where we have perfect knowledge of a fitness landscape and all possible ecological interactions, we are usually unable to point toward “rules” describing how we expect a community to evolve (i.e., we lack what economists would call “stylized facts” Abad and Khalifa, 2015). These challenges magnify the benefits of studying ecology in digital evolution platforms. The ability to tune the level of complexity in a system to exactly the level that is currently being studied is always useful, but it is invaluable for problems with as many interacting components as this one.
As climate change progresses, eco-evolutionary dynamics will become increasingly important to study for practical reasons. In order to forecast changes in ecosystems that are important to humanity, we will need to study eco-evolutionary dynamics in species that are not conducive to such research. These challenges may stem from long generation times, difficulty in collecting data, or insufficient confidence in experimental success. We propose that these issues can be mitigated via digital pilot experiments. Whereas much digital evolution research is designed to investigate general theory, digital pilot experiments would be tailored closely to specific lab or field experiments planned for the near future. This general approach has already been used in medical research, where it is referred to as a phase i clinical trial (Scott, 2012). Digital pilot experiments would provide an opportunity to (1) confirm that experimental hypotheses hold up under idealized in silico conditions, (2) determine which variables are the most important to prioritize measuring, and (3) obtain preliminary estimations of effect sizes, to facilitate more accurate power analysis and select appropriate experimental scales (spatial, tmeporal, and number of replicates). We suggest that, where lab or field experiments are risky or costly, digital pilot experiments should become a standard first step.
There is increasing evidence from both evolutionary computation and from more traditional digital evolution to suggest that ecology plays an important role in the evolution of complexity (Walker and Ofria, 2012; Dolson et al., 2018a). As has been clear since the early days of evolutionary computation, mutation, inheritance, and selection alone are insufficient to generate solutions to complex problems (Goldberg et al., 1987); additional dynamics and properties are necessary. For the most part, the dynamics that have helped achieve this goal have been ecological in nature (Dolson et al., 2018b). However, it is still unclear whether ecological dynamics are truly necessary for the evolution of complexity, and, if so, which ecological dynamics in particular.
Digital evolution has previously proven to be a powerful tool for understanding the evolution of complexity (Lenski et al., 2003). It has also been a powerful tool for untangling ecology from evolution, via experiments in which mutations and/or ecological interactions are turned on and off (Zaman et al., 2011). Thus, it seems an ideal platform for untangling questions at the intersection of ecology and the evolution of complexity.
Digital evolution offers a rich environment for asking ecological questions, particularly as they intersect with evolutionary questions. We have summarized a number of promising software systems to use for this purpose, and reviewed ecologically-relevant research carried out to date using them. While much of the research thus far has centered on eco-evolutionary dynamics, we believe that digital evolution platforms also have potential as a platform for studying more traditional ecology questions. To aid in such research, as well as to further research into eco-evolutionary dynamics, we have spelled out some suggestions for how best to study ecology in a digital evolution system. Lastly, we have provided suggestions for future research directions. We hope that this review will assist and encourage others to take advantage of the powerful tool-set offered by digital evolution.
Both authors listed have made a substantial, direct, and intellectual contribution to the work and approved it for publication.
This research was supported by the National Science Foundation (NSF) through the BEACON Center (Cooperative Agreement DBI-0939454) and grant DEB-1655715 to CO.
The authors declare that the research was conducted in the absence of any commercial or financial relationships that could be construed as a potential conflict of interest.
All claims expressed in this article are solely those of the authors and do not necessarily represent those of their affiliated organizations, or those of the publisher, the editors and the reviewers. Any product that may be evaluated in this article, or claim that may be made by its manufacturer, is not guaranteed or endorsed by the publisher.
We thank members of the Michigan State University Digital Evolution Lab, the Cleveland Clinic Theory Division, the Michigan State University Evolutionary Control of Digital Ecologies (ECODE) Lab, and two reviewers for useful conversations and feedback on this work.
1. ^Hendry also advocates for the importance of more “real-world” experiments, but notes that not all of his critiques can be addressed within the same studies. Thus, we do not think it unreasonable to suggest that digital experiments can play a complementary role in addressing his critiques.
Abad, L. A., and Khalifa, K. (2015). What are stylized facts? J. Econ. Methodol. 22, 143–156. doi: 10.1080/1350178X.2015.1024878
Adami, C. (2002). Ab initio modeling of ecosystems with artificial life. Nat. Resour. Model. 15, 133–145. doi: 10.1111/j.1939-7445.2002.tb00083.x
Adami, C., Schossau, J., and Hintze, A. (2016). Evolutionary game theory using agent-based methods. Phys. Life Rev. 19, 1–26. doi: 10.1016/j.plrev.2016.08.015
Batut, B., Parsons, D. P., Fischer, S., Beslon, G., and Knibbe, C. (2013). In silico experimental evolution: a tool to test evolutionary scenarios. BMC Bioinformatics 14:S11. doi: 10.1186/1471-2105-14-S15-S11
Beckmann, B. E., Knoester, D. B., Connelly, B. D., Waters, C. M., and McKinley, P. K. (2012). Evolution of resistance to quorum quenching in digital organisms. Artif. Life 18, 291–310. doi: 10.1162/artl_a_00066
Beckmann, B. E., and McKinley, P. K. (2009). “Evolving quorum sensing in digital organisms,” in Proceedings of the 11th Annual Conference on Genetic and Evolutionary Computation (Montreal, QC: ACM), 97–104.
Bhattacharjee, S., MacPherson, B., and Gras, R. (2018). A comparison of sexual selection versus random selection with respect to extinction and speciation rates using individual based modeling and machine learning. Ecol. Complex. 36, 126–137. doi: 10.1016/j.ecocom.2018.08.004
Bhattacharjee, S., MacPherson, B., Wang, R. F., and Gras, R. (2019). Animal communication of fear and safety related to foraging behavior and fitness: an individual-based modeling approach. Ecol. Inform. 54:101011. doi: 10.1016/j.ecoinf.2019.101011
Biswas, R., Bryson, D., Ofria, C., and Wagner, A. (2014). “Causes vs benefits in the evolution of prey grouping,” in ALIFE 14: Proceedings of the Fourteenth International Conference on the Synthesis and Simulation of Living Systems (New York, NY), 641–648.
Bohm, C., Ackles, A. L., Ofria, C., and Hintze, A. (2019a). “On sexual selection in the presence of multiple costly displays,” in ALIFE 2019: The 2019 Conference on Artificial Life (Newcastle: MIT Press), 247–254.
Bohm, C., Lalejini, A., Schossau, J., and Ofria, C. (2019b). “MABE 2.0: an introduction to MABE and a road map for the future of MABE development,” in Proceedings of the Genetic and Evolutionary Computation Conference Companion, GECCO '19 (New York, NY: Association for Computing Machinery), 1349–1356.
Bohm, C. G., Nitash, C. G., and Hintze, A. (2017). “MABE (Modular Agent Based Evolver): a framework for digital evolution research,” in ECAL 2017, the Fourteenth European Conference on Artificial Life (Lyon: MIT Press), 76–83.
Catalano, A. S., Lyons-White, J., Mills, M. M., and Knight, A. T. (2019). Learning from published project failures in conservation. Biol. Conserv. 238:108223. doi: 10.1016/j.biocon.2019.108223
Channon, A. D., and Damper, R. I. (2000). Towards the evolutionary emergence of increasingly complex advantageous behaviours. Int. J. Syst. Sci. 31, 843–860. doi: 10.1080/002077200406570
Channon, A. D., and Damper, R. I. (1998). others Perpetuating evolutionary emergence. From Anim. Animats 5, 534–539.
Chesson, P. (2000). Mechanisms of maintenance of species diversity. Annu. Rev. Ecol. Syst. 31, 343–366. doi: 10.1146/annurev.ecolsys.31.1.343
Chow, S. S., Wilke, C. O., Ofria, C., Lenski, R. E., and Adami, C. (2004). Adaptive radiation from resource competition in digital organisms. Science 305, 84–86. doi: 10.1126/science.1096307
Clune, J., Goldsby, H. J., Ofria, C., and Pennock, R. T. (2010). Selective pressures for accurate altruism targeting: evidence from digital evolution for difficult-to-test aspects of inclusive fitness theory. Proc. R. Soc. B Biol. Sci. 278, 666–674. doi: 10.1098/rspb.2010.1557
Cooper, T. F., and Ofria, C. (2003). “Evolution of stable ecosystems in populations of digital organisms,” in Artificial Life VIII: Proceedings of the Eighth International Conference on Artificial Life, eds R. K. Standish, M. A. Bedau, and H. A. Abbass (Cambridge, MA: MIT Press), 227–232.
De Jong, K. A. (1975). An Analysis of the Behavior of a Class of Genetic Adaptive Systems (Ph.D. Thesis), University of Michigan, Ann Arbor, MI.
Devaurs, D., and Gras, R. (2010). Species abundance patterns in an ecosystem simulation studied through Fisher's logseries. Simulat. Modell. Pract. Theory 18, 100–123. doi: 10.1016/j.simpat.2009.09.012
Diggle, S. P., Griffin, A. S., Campbell, G. S., and West, S. A. (2007). Cooperation and conflict in quorum-sensing bacterial populations. Nature 450, 411–414. doi: 10.1038/nature06279
Doebeli, M., and Knowlton, N. (1998). The evolution of interspecific mutualisms. Proc. Nat. Acad. Sci. U.S.A. 95, 8676–8680. doi: 10.1073/pnas.95.15.8676
Dolson, E., Banzhaf, W., and Ofria, C. (2018a). “Applying ecological principles to genetic programming,” in Genetic Programming Theory and Practice XV, eds W. Banzhaf, R. S. Olson, W. Tozier, and R. Riolo (Cham: Springer International Publishing), 73–88.
Dolson, E., and Ofria, C. (2017). “Spatial resource heterogeneity creates local hotspots of evolutionary potential,” in ECAL 2017: The Fourteenth European Conference on Artificial Life, Vol. 29, eds C. Knibbe, G. Beslon, D. Parsons, D. Misevic, J. Rouzaud-Cornabas, N. Bredeche, S. Hassas, O. Simonin, and H. Soula (Lyon: MIT Press), 122–129.
Dolson, E., Perez, S., Olson, R., and Ofria, C. (2017). Spatial resource heterogeneity increases diversity and evolutionary potential. bioRxiv. doi: 10.1101/148973
Dolson, E., Wiser, M. J., and Ofria, C. A. (2016). “The effects of evolution and spatial structure on diversity in biological reserves,” in Artificial Life XV: Proceedings of the Fifteenth International Conference on Artificial Life, eds C. Gershenson, T. Froese, J. M. Siqueiros, W. Aguilar, E. J. Izquierdo, and H. Sayama (Cancun, NM: MIT Press), 434–440.
Dolson, E. L., Banzhaf, W., and Ofria, C. (2018b). Ecological theory provides insights about evolutionary computation. PeerJ Preprints 6:e27315ve27311. doi: 10.7287/peerj.preprints.27315v1
Eldridge, A., and Kiefer, C. (2018). “Toward a synthetic acoustic ecology: sonically situated, evolutionary agent based models of the acoustic niche hypothesis,” in ALIFE 2018: The 2018 Conference on Artificial Life (Tokyo: MIT Press), 296–303.
Erwin, D. H. (1998). The end and the beginning: recoveries from mass extinctions. Trends Ecol. Evolut. 13, 344–349. doi: 10.1016/S0169-5347(98)01436-0
Evans, M. R., Grimm, V., Johst, K., Knuuttila, T., de Langhe, R., Lessells, C. M., et al. (2013). Do simple models lead to generality in ecology? TrendsEcol. Evolut. 28, 578–583. doi: 10.1016/j.tree.2013.05.022
Fahmy, M. (2014). “Artificial life and the philosophy of science,” in ALIFE 14: The Fourteenth International Conference on the Synthesis and Simulation of Living Systems (New York, NY: MIT Press), 596–601.
Fortuna, M. A., Zaman, L., Wagner, A. P., and Ofria, C. (2013). Evolving digital ecological networks. PLoS Comput. Biol. 9:e1002928. doi: 10.1371/journal.pcbi.1002928
Fox, J. W. (2019). The many roads to generality in ecology. Philos. Top. 47, 83–103. doi: 10.5840/philtopics20194715
Frederickson, M. E., Greene, M. J., and Gordon, D. M. (2005). Ecology: “Devil's gardens” bedevilled by ants. Nature 437, 495–496. doi: 10.1038/437495a
Frénoy, A., Taddei, F., and Misevic, D. (2012). “Robustness and evolvability of cooperation,” in Artificial Life 13 (East Lansing: MIT Press), 53–58.
Frénoy, A., Taddei, F., and Misevic, D. (2013). Genetic architecture promotes the evolution and maintenance of cooperation. PLoS Comput. Biol. 9:e1003339. doi: 10.1371/journal.pcbi.1003339
Gavrilets, S., and Vose, A. (2005). Dynamic patterns of adaptive radiation. Proc. Natl. Acad. Sci. U.S.A. 102, 18040–18045. doi: 10.1073/pnas.0506330102
Gerlee, P., and Lundh, T. (2010). Productivity and diversity in a cross-feeding population of artificial organisms. Evolution 64, 2716–2730. doi: 10.1111/j.1558-5646.2010.01020.x
Goings, S. (2010). Natural niching: Applying ecological principles to evolutionary computation (Ph.D). Michigan State University, United States-Michigan.
Goings, S., Goldsby, H. J., Cheng, B. H., and Ofria, C. (2012). An ecology-based evolutionary algorithm to evolve solutions to complex problems. Artif. Life 13, 171–177. doi: 10.7551/978-0-262-31050-5-ch024
Goings, S., and Ofria, C. (2009). “Ecological approaches to diversity maintenance in evolutionary algorithms,” in IEEE Symposium on Artificial Life, 2009. ALife '09 (Nashville, TN: IEEE), 124–130.
Goldberg, D. E., Richardson, J., and Grefenstette, J. J. (1987). “Genetic algorithms with sharing for multimodal function optimization,” in Genetic algorithms and their applications: Proceedings of the Second International Conference on Genetic Algorithms (Hillsdale, NJ: Lawrence Erlbaum), 41–49.
Golestani, A., Gras, R., and Cristescu, M. (2012). Speciation with gene flow in a heterogeneous virtual world: can physical obstacles accelerate speciation? Proc. R. Soc. B Biol. Sci. 279, 3055–3064. doi: 10.1098/rspb.2012.0466
Goudard, A., and Loreau, M. (2008). Nontrophic interactions, biodiversity, and ecosystem functioning: an interaction web model. Am. Nat. 171, 91–106. doi: 10.1086/523945
Gras, R., Devaurs, D., Wozniak, A., and Aspinall, A. (2009). An individual-based evolving predator-prey ecosystem simulation using a fuzzy cognitive map as the behavior model. Artif. Life 15, 423–463. doi: 10.1162/artl.2009.Gras.012
Gras, R., Golestani, A., Hendry, A. P., and Cristescu, M. E. (2015). Speciation without Pre-Defined fitness functions. PLoS ONE 10:e0137838. doi: 10.1371/journal.pone.0137838
Hamilton, W. D. (1964). The genetical evolution of social behaviour. II. J. Theor. Biol. 7, 17–52. doi: 10.1016/0022-5193(64)90039-6
Hendry, A. P. (2016). Eco-evolutionary Dynamics. Princeton University Press. Google-Books-ID: QMtIDAAAQBAJ.
Hendry, A. P. (2019). A critique for eco-evolutionary dynamics. Funct. Ecol. 33, 84–94. doi: 10.1111/1365-2435.13244
Holland, J. H. (1995). Hidden Order: How Adaptation Builds Complexity. New York, NY: Addison Wesley.
Hooper, D. U., Chapin, F. S., Ewel, J. J., Hector, A., Inchausti, P., Lavorel, S., et al. (2005). Effects of biodiversity on ecosystem functioning: a consensus of current knowledge. Ecol. Monogr. 75, 3–35. doi: 10.1890/04-0922
Hornby, G. S. (2006). “ALPS: the age-layered population structure for reducing the problem of premature convergence,” in Proceedings of the 8th Annual Conference on Genetic and Evolutionary Computation, GECCO '06 (New York, NY: ACM), 815–822.
Hraber, P. T., Jones, T., and Forrest, S. (1997). The ecology of echo. Artif. Life 3, 165–190. doi: 10.1162/artl.1997.3.3.165
Hraber, P. T., and Milne, B. T. (1997). Community assembly in a model ecosystem. Ecol. Modell. 103, 267–285. doi: 10.1016/S0304-3800(97)00111-7
Hu, J., Goodman, E., Seo, K., Fan, Z., and Rosenberg, R. (2005). The hierarchical fair competition (HFC) framework for sustainable evolutionary algorithms. Evol. Comput. 13, 241–277. doi: 10.1162/1063656054088530
Johnson, A., Strauss, E., Pickett, R., Adami, C., Dworkin, I., and Goldsby, H. (2014). “More bang for your buck: quorum-sensing capabilities improve the efficacy of suicidal altruism,” in ALIFE 14: Proceedings of the Fourteenth International Conference on the Synthesis and Simulation of Living Systems (New York, NY), 120–128.
Johnson, T. J., and Wilke, C. O. (2004). Evolution of resource competition between mutually dependent digital organisms. Artif. Life 10, 145–156. doi: 10.1162/106454604773563577
Kawecki, T. J., Lenski, R. E., Ebert, D., Hollis, B., Olivieri, I., and Whitlock, M. C. (2012). Experimental evolution. Trends Ecol. Evolut. 27, 547–560. doi: 10.1016/j.tree.2012.06.001
Khater, M., Murariu, D., and Gras, R. (2014). Contemporary evolution and genetic change of prey as a response to predator removal. Ecol. Inform. 22, 13–22. doi: 10.1016/j.ecoinf.2014.02.005
Khater, M., Murariu, D., and Gras, R. (2016). Predation risk tradeoffs in prey: effects on energy and behaviour. Theor. Ecol. 9, 251–268. doi: 10.1007/s12080-015-0277-5
Knibbe, C., Coulon, A., Mazet, O., Fayard, J.-M., and Beslon, G. (2007). A long-term evolutionary pressure on the amount of noncoding DNA. Mol. Biol. Evol. 24, 2344–2353. doi: 10.1093/molbev/msm165
Lafferty, K. D., Dobson, A. P., and Kuris, A. M. (2006). Parasites dominate food web links. Proc. Natl. Acad. Sci. U.S.A. 103, 11211–11216. doi: 10.1073/pnas.0604755103
Lalejini, A., Dolson, E., Bohm, C., Ferguson, A. J., Parsons, D. P., Rainford, P. F., et al. (2019). “data standards for artificial life software,” in ALIFE 2019: The 2019 Conference on Artificial Life (Newcastle: MIT Press), 507–514.
Lalejini, A., Moreno, M. A., and Ofria, C. (2021). Tag-based regulation of modules in genetic programming improves context-dependent problem solving. Genet. Program. Evol. Mach. arXiv: 2012.09229. doi: 10.1007/s10710-021-09406-8
Lavergne, S., and Molofsky, J. (2007). Increased genetic variation and evolutionary potential drive the success of an invasive grass. Proc. Natl. Acad. Sci. U.S.A. 104, 3883–3888. doi: 10.1073/pnas.0607324104
Lehmann, K. D. S., Goldman, B. W., Dworkin, I., Bryson, D. M., and Wagner, A. P. (2014). From cues to signals: evolution of interspecific communication via aposematism and mimicry in a predator-prey system. PLoS ONE 9:e91783. doi: 10.1371/journal.pone.0091783
Lenski, R. E., Ofria, C., Pennock, R. T., and Adami, C. (2003). The evolutionary origin of complex features. Nature 423, 139–144. doi: 10.1038/nature01568
Loreau, M. (2020). “The ecosystem: superorganism, or collection of individuals?” in Unsolved Problems in Ecology, eds A. Dobson, R. D. Holt, and D. Tilman (Princeton University Press), 218–224.
Lurgi, M., Montoya, D., and Montoya, J. (2016). The effects of space and diversity of interaction types on the stability of complex ecological networks. Theor. Ecol. 9, 3–13. doi: 10.1007/s12080-015-0264-x
MacPherson, B., and Gras, R. (2016). Individual-based ecological models: adjunctive tools or experimental systems? Ecol. Modell. 323, 106–114. doi: 10.1016/j.ecolmodel.2015.12.013
Mashayekhi, M., Golestani, A., Farahani, Y., and Gras, R. (2014a). “An enhanced artificial ecosystem: investigating emergence of ecological niches,” in ALIFE 14: The Fourteenth International Conference on the Synthesis and Simulation of Living Systems (New York: The MIT Press), 693–700.
Mashayekhi, M., MacPherson, B., and Gras, R. (2014b). Species–area relationship and a tentative interpretation of the function coefficients in an ecosystem simulation. Ecol. Complex. 19, 84–95. doi: 10.1016/j.ecocom.2014.05.011
McDonald, B. A., and Linde, C. (2002). Pathogen population genetics, evolutionary potential, and durable resistance. Annu. Rev. Phytopathol. 40, 349–379. doi: 10.1146/annurev.phyto.40.120501.101443
Misevic, D., Frénoy, A., Lindner, A. B., and Taddei, F. (2015). Shape matters: lifecycle of cooperative patches promotes cooperation in bulky populations. Evolution 69, 788–802. doi: 10.1111/evo.12616
Misevic, D., Frénoy, A., Parsons, D. P., and Taddei, F. (2012). “Effects of public good properties on the evolution of cooperation,” in ALIFE 2012: The Thirteenth International Conference on the Synthesis and Simulation of Living Systems (East Lansing, MI: MIT Press), 218–225.
Moreno, M. A., Lalejini, A., and Ofria, C. (2021). Matchmaker, matchmaker, make me a match: geometric, variational, and evolutionary implications of criteria for tag affinity. arXiv:2108.04507 [cs]. arXiv: 2108.04507.
Moreno, M. A., and Ofria, C. (2019). Toward Open-Ended Fraternal Transitions in Individuality. Artif. Life 25, 117–133. doi: 10.1162/artl_a_00284
Moreno, M. A., and Ofria, C. (2021). Exploring evolved multicellular life histories in a open-ended digital evolution system. arXiv:2104.10081 [cs, q-bio]. arXiv: 2104.10081.
Mouret, J.-B., and Doncieux, S. (2009). “Using behavioral exploration objectives to solve deceptive problems in neuro-evolution,” in Proceedings of the 11th Annual Conference on Genetic and Evolutionary Computation, GECCO '09 (New York, NY: ACM), 627–634.
Mustonen, V., and Lässig, M. (2009). From fitness landscapes to seascapes: non-equilibrium dynamics of selection and adaptation. Trends Genet. 25, 111–119. doi: 10.1016/j.tig.2009.01.002
O'Donnell, D. R., Parigi, A., Fish, J. A., Dworkin, I., and Wagner, A. P. (2014). The roles of standing genetic variation and evolutionary history in determining the evolvability of anti-predator strategies. PLoS ONE 9:e100163. doi: 10.1371/journal.pone.0100163
Ofria, C., and Wilke, C. O. (2004). Avida: a software platform for research in computational evolutionary biology. Artif. Life 10, 191–229. doi: 10.1162/106454604773563612
Olson, R., Mirmomeni, M., Brom, T., Bruger, E., Hintze, A., Knoester, D., et al. (2013). “Evolved digital ecosystems: dynamic steady state, not optimal fixed point,” in ECAL 2013: The Twelfth European Conference on Artificial Life (Sicily), 126–133.
Pakanati, A. R. (2015). Structure and Evolutionary Dynamics in Fitness Landscapes (Ph.D). Michigan State University, East Lansing, MI, USA.
Pennock, R. T. (2007). Models, simulations, instantiations, and evidence: the case of digital evolution. J. Exp. and Theor. Artif. Intell. 19, 29–42. doi: 10.1080/09528130601116113
Pour, M. K., Bandehbahman, S., Gras, R., and Cristescu, M. E. (2017). An individual-based modeling approach to investigate sympatric speciation via specialized resource usage. Open J. Ecol. 07, 222. doi: 10.4236/oje.2017.73017
Pour, M. K., Bhattacharjee, S., Gras, R., and Drouillard, K. (2015). “The integration of an individual-based model into toxicokinetics to enhance ecological realism in evaluating population-level impacts of exposure to PCB,” in 2015 Third World Conference on Complex Systems (WCCS) (Marrakech), 1–8.
Pugh, J. K., Soros, L. B., Szerlip, P. A., and Stanley, K. O. (2015). “Confronting the challenge of quality diversity,” in Proceedings of the 2015 Annual Conference on Genetic and Evolutionary Computation, GECCO '15 (New York, NY: ACM), 967–974.
Ray, T. S. (1991). An Approach to the Synthesis of Life. Artificial Life II. Santa Fe, NM: Adison Wesley.
Ray, T. S. (1994). Evolution, complexity, entropy and artificial reality. Physica D 75, 239–263. doi: 10.1016/0167-2789(94)90286-0
Rocabert, C., Knibbe, C., Consuegra, J., Schneider, D., and Beslon, G. (2017). Beware batch culture: seasonality and niche construction predicted to favor bacterial adaptive diversification. PLoS Comput. Biol. 13:e1005459. doi: 10.1371/journal.pcbi.1005459
Roopnarine, P. D., Angielczyk, K. D., Wang, S. C., and Hertog, R. (2007). Trophic network models explain instability of early triassic terrestrial communities. Proc. R. Soc. Lond. B Biol. Sci. 274, 2077–2086. doi: 10.1098/rspb.2007.0515
Schoener, T. W. (2011). The newest synthesis: understanding the interplay of evolutionary and ecological dynamics. Science 331, 426–429. doi: 10.1126/science.1193954
Scott, R., and Gras, R. (2020). A simulation study shows impacts of genetic diversity on establishment success of digital invaders in heterogeneous environments. Ecol. Modell. 431:109173. doi: 10.1016/j.ecolmodel.2020.109173
Scott, R., MacPherson, B., and Gras, R. (2019). “EcoSim, an enhanced artificial ecosystem: addressing deeper behavioral, ecological, and evolutionary questions,” in Cognitive Architectures, Intelligent Systems, Control and Automation: Science and Engineering, eds M. I. Aldinhas Ferreira, J. Silva Sequeira, and R. Ventura (Cham: Springer International Publishing), 223–278.
Smith, R., and Bedau, M. (1997). “Emergence of complex ecologies in ECHO,” in Proceedings of the International Conference on Complex Systems, 9.
Soros, L. (2018). Necessary conditions for open-ended evolution (Electronic Theses and Dissertations).
Soros, L., and Stanley, K. (2014). “Identifying necessary conditions for open-ended evolution through the artificial life world of Chromaria,” in ALIFE 14: Proceedings of the Fourteenth International Conference on the Synthesis and Simulation of Living Systems, eds H. Sayama, J. Rieffel, S. Risi, R. Doursat, and H. Lipson (Cambridge, MA: MIT Press), 793–800.
Spector, L., Martin, B., Harrington, K., and Helmuth, T. (2011). “Tag-based modules in genetic programming,” in Proceedings of the 13th annual conference on Genetic and evolutionary computation, GECCO '11 (New York, NY: Association for Computing Machinery), 1419–1426.
Stanley, K., and Miikkulainen, R. (2004). Competitive coevolution through evolutionary complexification. J. Artif. Intell. Res. 21, 63–100. doi: 10.1613/jair.1338
Strona, G., and Lafferty, K. D. (2016). Environmental change makes robust ecological networks fragile. Nat. Commun. 7:12462. doi: 10.1038/ncomms12462
Suding, K. N. (2011). Toward an era of restoration in ecology: successes, failures, and opportunities ahead. Ann. Rev. Ecol. 42, 465–487. doi: 10.1146/annurev-ecolsys-102710-145115
Tilman, D., Isbell, F., and Cowles, J. M. (2014). Biodiversity and ecosystem functioning. Annu. Rev. Ecol. Evol. Syst. 45, 471–493. doi: 10.1146/annurev-ecolsys-120213-091917
Tomassini, M. (2005). Spatially Structured Evolutionary Algorithms: Artificial Evolution in Space and Time. Natural Computing Series. Berlin; Heidelberg: Springer Berlin Heidelberg.
Vostinar, A. E., Fenton, J., Waters, C., and Ofria, C. (2018). “Signals in the dark: what factors select for the evolution of cooperation controlled by quorum sensing?” in The 2018 Conference on Artificial Life: A Hybrid of the European Conference on Artificial Life (ECAL) and the International Conference on the Synthesis and Simulation of Living Systems (ALIFE) (Tokyo), 651–658.
Vostinar, A. E., and Ofria, C. (2019). Spatial structure can decrease symbiotic cooperation. Artif. Life 24, 229–249. doi: 10.1162/artl_a_00273
Vostinar, A. E., Skocelas, K. G., Lalejini, A., and Zaman, L. (2021). Symbiosis in digital evolution: past, present, and future. Front. Ecol. Evolut. 9:739047. doi: 10.3389/fevo.2021.739047
Wagner, A. P., Zaman, L., Dworkin, I., and Ofria, C. (2020). “Behavioral strategy chases promote the evolution of prey intelligence,” in Evolution in Action: Past, Present and Future: A Festschrift in Honor of Erik D. Goodman, Genetic and Evolutionary Computation, eds W. Banzhaf, B. H. Cheng, K. Deb, K. E. Holekamp, R. F. Lenski, C. Ofria, R. T. Pennock, W. F. Punch, and D. J. Whittaker (Cham: Springer International Publishing), 225–246.
Walker, B. L., and Ofria, C. (2012). “Evolutionary potential is maximized at intermediate diversity levels,” in Proceedings of the 13th International Conference for Artificial Life (East Lansing, MI: MIT Press), 116–120.
Walker, J. C. (2011). “The evolution of optimal foraging strategies in populations of digital organisms,” in Proceedings of the 13th Annual Conference on Genetic and Evolutionary Computation, GECCO '11 (New York, NY: ACM), 203–210.
Wilke, C. O., Wang, J. L., Ofria, C., Lenski, R. E., and Adami, C. (2001). Evolution of digital organisms at high mutation rates leads to survival of the flattest. Nature 412, 331–333. doi: 10.1038/35085569
Wiser, M. J. (2015). An analysis of fitness in long-term asexual evolution experiments (Ph.D. thesis). Michigan State University.
Wiser, M. J., Ribeck, N., and Lenski, R. E. (2013). Long-term dynamics of adaptation in asexual populations. Science 342, 1364–1367. doi: 10.1126/science.1243357
Wright, S. (1932). “The roles of mutation, inbreeding, crossbreeding, and selection in evolution,” in Proceedings of the Sixth International Congress of Genetics (Brooklyn, NY: Brooklyn Botanic Garden), 356–366.
Yaeger, L. (1993). “Computational genetics, physiology, metabolism, neural systems, learning, vision, and behavior or PolyWorld: life in a new context,” in Artificial Life III, Vol. XVII of SFI Studies in the Sciences of Complexity (Santa Fe, NM: Santa Fe Institute).
Yedid, G., Ofria, C., and Lenski, R. (2009). Selective press extinctions, but not random pulse extinctions, cause delayed ecological recovery in communities of digital organisms. Am. Nat. 173, E139–E154. doi: 10.1086/597228
Yedid, G., Ofria, C. A., and Lenski, R. E. (2008). Historical and contingent factors affect re-evolution of a complex feature lost during mass extinction in communities of digital organisms. J. Evol. Biol. 21, 1335–1357. doi: 10.1111/j.1420-9101.2008.01564.x
Yedid, G., Stredwick, J., Ofria, C. A., and Agapow, P.-M. (2012). A comparison of the effects of random and selective mass extinctions on erosion of evolutionary history in communities of digital organisms. PLoS ONE 7:e37233. doi: 10.1371/journal.pone.0037233
Zaman, L., Devangam, S., and Ofria, C. (2011). “Rapid host-parasite coevolution drives the production and maintenance of diversity in digital organisms,” in Proceedings of the 13th Annual Conference on Genetic and Evolutionary Computation (Dublin: ACM), 219–226.
Keywords: digital evolution, eco-evolutionary dynamics, competition, predation, multi-trophic communities, crossfeeding
Citation: Dolson E and Ofria C (2021) Digital Evolution for Ecology Research: A Review. Front. Ecol. Evol. 9:750779. doi: 10.3389/fevo.2021.750779
Received: 31 July 2021; Accepted: 05 November 2021;
Published: 29 November 2021.
Edited by:
György Barabás, Linköping University, SwedenReviewed by:
Rafael D'Andrea, Stony Brook University, United StatesCopyright © 2021 Dolson and Ofria. This is an open-access article distributed under the terms of the Creative Commons Attribution License (CC BY). The use, distribution or reproduction in other forums is permitted, provided the original author(s) and the copyright owner(s) are credited and that the original publication in this journal is cited, in accordance with accepted academic practice. No use, distribution or reproduction is permitted which does not comply with these terms.
*Correspondence: Emily Dolson, ZG9sc29uZW1AbXN1LmVkdQ==
Disclaimer: All claims expressed in this article are solely those of the authors and do not necessarily represent those of their affiliated organizations, or those of the publisher, the editors and the reviewers. Any product that may be evaluated in this article or claim that may be made by its manufacturer is not guaranteed or endorsed by the publisher.
Research integrity at Frontiers
Learn more about the work of our research integrity team to safeguard the quality of each article we publish.