Single-Cell RNA Sequencing Atlas From a Bivalve Larva Enhances Classical Cell Lineage Studies
- 1Unit for Integrative Zoology, Department of Evolutionary Biology, University of Vienna, Vienna, Austria
- 2Division of Molecular Evolution and Development, Department of Neuroscience and Developmental Biology, University of Vienna, Vienna, Austria
Ciliated trochophore-type larvae are widespread among protostome animals with spiral cleavage. The respective phyla are often united into the superclade Spiralia or Lophotrochozoa that includes, for example, mollusks, annelids, and platyhelminths. Mollusks (bivalves, gastropods, cephalopods, polyplacophorans, and their kin) in particular are known for their morphological innovations and lineage-specific plasticity of homologous characters (e.g., radula, shell, foot, neuromuscular systems), raising questions concerning the cell types and the molecular toolkit that underlie this variation. Here, we report on the gene expression profile of individual cells of the trochophore larva of the invasive freshwater bivalve Dreissena rostriformis as inferred from single cell RNA sequencing. We generated transcriptomes of 632 individual cells and identified seven transcriptionally distinct cell populations. Developmental trajectory analyses identify cell populations that, for example, share an ectodermal origin such as the nervous system, the shell field, and the prototroch. To annotate these cell populations, we examined ontology terms from the gene sets that characterize each individual cluster. These were compared to gene expression data previously reported from other lophotrochozoans. Genes expected to be specific to certain tissues, such as Hox1 (in the shell field), Caveolin (in prototrochal cells), or FoxJ (in other cillia-bearing cells) provide evidence that the recovered cell populations contribute to various distinct tissues and organs known from morphological studies. This dataset provides the first molecular atlas of gene expression underlying bivalve organogenesis and generates an important framework for future comparative studies into cell and tissue type development in Mollusca and Metazoa as a whole.
Introduction
The organization and diversity of cell types constitute key features during the ontogenetic establishment of animal body plans. Distinct transcriptional profiles of cell populations are an important prerequisite for the formation of cell types, and, ultimately, tissues, of multicellular animals (Shapiro et al., 2013; Stegle et al., 2015; Arendt et al., 2016). Accordingly, deciphering the transcriptomic code underlying the ontogeny of these cell types is crucial for sound inferences of the shared evolutionary history of cells and tissues across the animal kingdom. Among the multicellular animals, adult mollusks exhibit a particularly high morphological diversity that has resulted in a number of autapomorphic characters on various phylogenetic levels [e.g., radula, shell(s), foot, neuromuscular systems] (Smith et al., 2011; Wanninger and Wollesen, 2019). However, despite their unique innovations in the adult body plan, numerous mollusks display a conserved, ciliated, trochophore-type larva that is also found in other lophotrochozoans including annelids and platyhelminths (Nielsen, 2018). The molecular pathways underlying the morphological diversification from this common larval type into clade-specific features remains largely unresolved.
Traditional studies have focused on the ontogeny of different tissues by tracing the mitotic history of a cell and generating so-called fate maps, resulting in the reconstruction of so-called cell lineages (Lillie, 1895; Meisenheimer, 1900; Luetjens and Dorresteijn, 1995; Dictus and Damen, 1997; Render, 1997; Henry et al., 2004; Hejnol et al., 2007; Lyons et al., 2017; Farrell et al., 2018). While cell lineages for certain putative homologous structures (e.g., the prototroch) have been identified, striking differences in mitotic history details are evident even between closely related taxa, particularly within Mollusca (Wanninger and Wollesen, 2015). Moreover, some lineage diagrams do not assign fates to all terminal ends of the cell lineage tree, hindering broad comparisons across taxa (Meisenheimer, 1900; Luetjens and Dorresteijn, 1995). For more detailed insights into differences and shared features on the ontogeny of tissues and organ systems, gene expression analyses using in situ hybridization has been widely used on a variety of molluscan taxa (e.g., Hinman et al., 2003; Lee et al., 2003; Wollesen et al., 2015a,b, 2018; Redl et al., 2016; Wang et al., 2017; Salamanca-Díaz et al., 2021). These studies represent a framework for comparative analyses of genes underlying the formation of features such as the shell field, nervous system, foot, sensory structures, and others (Wanninger and Wollesen, 2019). However, this approach is hampered by the fact that only a fraction of genes are known to be active in certain regions and developmental stages of the respective target species. Novel approaches of sequencing live, dissociated cells (single-cell RNA sequencing, scRNA-seq) followed by in silico methods using fine-grained computational pipelines have allowed for characterization of transcriptomic profiles related to cell types and cell states based on their distinct, relative gene expression levels. Such studies have resulted in comprehensive lists of genes that are expressed during ontogeny (Tang et al., 2010; Trapnell et al., 2014; Achim et al., 2015; Satija et al., 2015; Trapnell, 2015; Vergara et al., 2017; Svensson et al., 2018; Zhong et al., 2018). Accordingly, recent studies have uncovered the presence of previously uncharacterized cell types and an unexpected transcriptomic heterogeneity amongst cell populations and have revealed numerous genes with hitherto unknown function (Karaiskos et al., 2017; Achim et al., 2018; Briggs et al., 2018; Farrell et al., 2018; Fincher et al., 2018; Plass et al., 2018; Sebé-Pedrós et al., 2018; Wagner et al., 2018; Foster et al., 2019, 2020; Siebert et al., 2019; Duruz et al., 2020; Wendt et al., 2020; García-Castro et al., 2021; Paganos et al., 2021; Sur and Meyer, 2021).
The quagga mussel Dreissena rostriformis, native to the Dnieper drainage of the Ukrainian Black Sea region (Nalepa and Schloesser, 2019), has managed to rapidly spread across European and North American freshwater bodies (Mills et al., 1993; Heiler et al., 2013; Aldridge et al., 2014; Karatayev et al., 2014; Wakida-Kusunoki et al., 2015; Nalepa and Schloesser, 2019). Together with its congener, the zebra mussel Dreissena polymorpha, they build dense populations which consume large amounts of phytoplankton with often damaging impact on native invertebrate and fish populations (Raikow, 2004; Strayer et al., 2004; McNickle et al., 2006; Karatayev et al., 2014; Nalepa and Schloesser, 2019). This makes the quagga and zebra mussel one of the most prevalent and successful invasive mollusks (Karatayev et al., 2007). Efforts to understand and manipulate the rate of colonization and dispersal for these species have mainly focused on the adult stage when the population is already established, while data on the dispersive larval stages, the trochophore and the veliger, are still lacking (McCartney et al., 2019).
Here, we present the first comprehensive scRNA-seq study on the trochophore larva of any mollusk, the quagga mussel Dreissena rostriformis. We generated a scRNA-seq library from dissociated cells of entire larvae. Using this dataset, we define distinct cell types present in this larval type. We assign identities to identified cell populations based on characterization of distinct transcriptomic signatures and predict relationships between cell types by modeling developmental trajectories within the dataset. We analyze ciliary, neuronal, muscle, and other cell types with potential importance to environment sensing and larval spreading. Through this work, we also provide a comprehensive molecular atlas of gene expression underlying Dreissena rostriformis development, thereby laying the foundation for further research into understanding the features that allow quagga mussel larvae to rapidly conquer new freshwater habitats.
Materials and Methods
Animal Collection and Cultures
Sexually mature individuals of Dreissena rostriformis were collected in the Danube River in Vienna, Austria (N 48°14′45.812″, O 16°23′38.145″) between April and September, 2019. Adults were gathered from underneath stones and transferred to the laboratory where they were cleaned and maintained in aquaria with filtered river water (FRW) at 19°C.
Spawning of animals was induced by exposing sexually mature specimens for 15 min to a 10–3 M solution of serotonin (#H9523, Sigma-Aldrich, Darmstadt, Germany), followed by one wash and subsequent maintenance in FRW. Individuals were kept isolated in FRW inside 50 mL glass beakers and after approximately 30 min, up to 50% of the treated specimens started to spawn. Oocytes and motile sperm were then collected separately from the water column. Fertilization rates were high when three to four drops of sperm-containing water were added to 50 ml glass beakers with oocytes. The cultures were maintained at 23°C. After fertilization, water was changed every half an hour for the first 3 h and then every 6 h to remove excess sperm and avoid bacterial or fungal infection.
10X Single-Cell 3′ RNA-Seq
Sample Preparation
Cell dissociations of Dreissena larvae were generated by first washing 13 h post fertilization (hpf) trochophore larvae over a 20 μm mesh with sterile autoclaved fresh river water (AFRW). Larvae were concentrated into 1.5 mL tubes by centrifugation (1rcf) for 5 min and AFRW was replaced with a pronase enzyme in AFRW (8 mg/mL) (#10165921001, Roche Diagnostics, Mannheim, Germany) and the tubes were subsequently placed in gentle agitation for 30 min on a rocker at the slowest setting in order to keep the cells and reagents in suspension. Larvae were dissociated by first passing them through a syringe with a hypodermic needle with 0.4 mm diameter. This procedure was repeated and monitored until a uniform single cell solution was visible under the microscope. Cell viability was assessed by adding an acridine orange/propidium iodide (AO/PI) staining solution (#CS2-0106; Nexcelom Bioscience, Lawrence, MA, United States) to stain live and dead cells, respectively (Cellometer K2; Nexcelom Bioscience). A single cell suspension measuring 76.8% viability with a concentration of 1000 cells/μL was loaded into a 10x Chromium Controller using Chromium Single Cell 3′ Kit v2 reagents (#120237, 10x Genomics, United States). cDNA synthesis and library construction were made according to specifications from the manufacturer. Library quantification was performed on a Bioanalyzer (#5067-4626, High Sensitivity DNA reagents, Agilent Technology; Agilent 2100 Bioanalyzer) and sequenced on the Illumina platform.
Mapping Tool Preparation and Cell Clustering
The transcriptomes used for creating the mapping tool, together with the reference genome to map the reads against, were previously generated (Calcino et al., 2019). Gene models were elongated 2 kilobases in the 3′direction to account for poorly annotated three-prime ends (Levin et al., 2016). To annotate the transcriptome, we performed a BLASTX search in each individual gene sequence against the human and the Pacific giant oyster (Crassostrea gigas) genomes. For each transcript, the BLAST hit with the highest E-value was selected for annotation. We utilized InterProScan v5.46-81.0 (Jones et al., 2014) to search for gene ontology and allocate domains on the reference genome by surveying publicly available databases such as GO terms, Pfam, and PANTHER (Supplementary Table 1). The reference database was generated using CellRanger MakeRef v3.1.0 with default settings. Afterward, sample libraries were demultiplexed using CellRanger Makefastq v3.1.0 with default settings and filtered according to cell barcode and Unique Molecular Markers (UMIs) using CellRanger Count v3.1.0 with parameters of –force-cells = 1000 –chemistry = SC3Pv2. The resulting cell count gene expression matrix was analyzed in R v3.6.1 (R Developement Core Team, 2015) with the Seurat v4.0.1 package (Satija et al., 2015). Samples were considered to represent a single cell transcriptome if it contained at least 150 genes. Samples with more than 4,000 sequenced genes were removed as these represent multiplets. The count matrix was processed through a standard Seurat pipeline using default parameters as follows: counts were first normalized, and log scaled (NormalizeData and ScaleData functions) and principal component analysis was performed (RunPCA) using only highly variable features (FindVariableFeatures). We visually inspected an elbow plot of standard deviation of the principal components (Supplementary Figure 1) and selected the top 30 principal components for clustering. We then generated a KNN graph based on the Euclidean distance in PCA space following the Louvain algorithm [FindNeighbors (k.param = 10, nn.method = “annoy,” annoy.metric = “cosine”)] and clustered the data [FindClusters (random.seed = 0, res = 0.5)]. For data visualization, we projected all single cell transcriptomes into a uniform space of 2 dimensions [Uniform Manifold Approximation and Projection (UMAP): RunUMAP (n.neighbors = 10 L, spread = 0.45, min.dist = 0.1, local.connectivity = 100)]. Marker genes were identified with the FindAllMarkers function (random.seed = 0), which considers only genes that were enriched and expressed in at least 10% of the cells in each population (min.pct = 0.1) and with a log fold difference larger than 0.6 (logfc.threshold = 0.6). Later, a filter was performed for the top 10 genes with the higher average logarithmic fold change values (Supplementary Tables 2, 3). Additional cutoff thresholds were explored and the whole pipeline was applied to observe how they affect the remaining cells in the dataset (Supplementary Figure 2 and Supplementary Table 5). We applied a graph imputation algorithm with the R package MAGIC (van Dijk et al., 2018) on single cells for de-noising the count matrix and fill in missing transcripts. The R package topGO (Alexa et al., 2006) was implemented to perform a GO terms enrichment analysis on genes from each cluster, determining statistically the molecular activity of a gene (molecular function), the place in the cell where the gene produces an effect (cellular component), and the physiological process influenced by a gene (biological process). Following this, expression of orthologous marker genes from distinct cell types were examined in this dataset to validate cluster identification assigned by GO term results.
Pseudotime Trajectory Analysis
In order to root the pseudotime trajectories in a single place and to calculate the number of transcriptomic changes of each population, we implemented the R package StemID to predict developmentally uncommitted cell populations from maximum transcriptome entropy calculations (Grün et al., 2016). This one cell population, which clustered together based upon the lack of differentially expressed genes compared with the other clusters in the dataset, also received the lowest entropy calculation. This suggests that these cells represent a pool of uncommitted cells within the embryo (Hemmrich et al., 2012; Fincher et al., 2018; Siebert et al., 2019). Cells were ordered along a calculated similarity trajectory with this population defined as the starting point using the Monocle3 R package (Cao et al., 2019), imported from Seurat with the package SeuratWrappers v0.3. Cells were re-clustered using the “cluster_cells” function (resolution = 0.02). The “learn_graph” function was implemented to model the cell differentiation paths throughout development. This principal graph was used to order cells in pseudotime using the “orderCells” function with undifferentiated cells defined as the root. Monocle interprets multiple ends to a trajectory as cellular decisions.
Gene Cloning
A first-strand cDNA Synthesis Kit for RT-PCR (#11483188001, Roche Diagnostics, Mannheim, Germany) was used for cDNA synthesis from the pooled RNA. PCR products were size fractioned by gel electrophoresis and purified with a QIAquick Gel Extraction Kit (#28706, Qiagen, Hilden, Germany). PCR products were cloned in pGEM-T Easy Vectors (#A1380, Promega, Mannheim, Germany). Plasmid minipreps were grown overnight, purified with a QIAprep Spin Miniprep Kit (#27106, Qiagen), and sequenced for verification. Forward and reverse primers for each gene sequence can be found in Supplementary Table 4.
Probe Synthesis and Whole Mount in situ Hybridization
To provide spatial information associated with the transcriptomic signatures and further validate cell type annotations, whole mount in situ hybridization analysis of some of the highly expressed cell population-specific genes were performed on trochophore larvae aged 13–15 hpf. Plasmid products were linearized by PCR amplification using M13 primers as described previously (Wollesen et al., 2015b). Antisense riboprobes were synthesized using digoxigenin-UTP (#11175025910, DIG RNA Labeling Kit, Roche Diagnostics) and SP6/T7 polymerase (#10810274001, #1088176001, Roche Diagnostics).
Larvae were fixed for 1 h in 4% paraformaldehyde (PFA) (20% PFA + 10x PBS; DEPC- treated H2O), followed by three washes of 15 min each in Phosphate Buffer Solution (PBS). For long-term storage of samples, subsequent washing steps for at least 15 min each were performed in different concentrations of methanol (25, 50, and 75%) in 1x PBS. For whole mount in situ hybridization, larvae stored in 100% methanol were rehydrated in 0.1 M PBS and decalcified with PPE (20% PFA + 10x PBS + 0.5 M EGTA at pH 8.0 + diethyl pyrocarbonate; DEPC-treated H2O). Afterward, samples were treated with proteinase-K at 37°C for 10 min (10 μg/mL in PTw: 1x PBS + 0.1% Tween-20). Samples were washed in PTw and post-fixed for 45 min in 4% PFA. Subsequently, samples were washed and transferred to hybridization buffer (50% formamide, 5X SSC, 50 μg/ml heparin, 500 μg/ml yeast tRNA, 0.1% Tween-20, pH 6.0) for 8–10 h at 56–60°C. Probe hybridization was performed at the same temperature with probe concentrations ranging between 1 and 2 ng/μL for 30–48 h. Washing steps after hybridization were done using the following solutions: 75% hybridization buffer + 25% of SSC (3 M NaCl + 0.3 M saline-sodium citrate; SSC buffer + DEPC H2O) for three times 10 min each, then 50% hybridization buffer + 50% SSC twice for 10 min each, 25% hybridization buffer + 75% SSC for 7 min (once) and 1X SSC + 0.1% Tween-20 for three times for 5 min each. Next, three washes in 0.1 M maleic acid buffer (MAB) were performed. A digoxigenin (DIG)-labeled alkaline phosphatase (AP) antibody (#11093274910, Roche Diagnostics) was used at a dilution of 1:5000 in blocking solution (#11096176001, 10x blocking reagent; Roche Diagnostics + 0.1 M MAB) at 4°C overnight. Samples were then washed in PTw three times for 20 min each and twice for 10 min each. Development of the color reaction was done in a NBT/BCIP solution (#11383213001, #11383221001, Roche Diagnostics) diluted in 1x alkaline phosphatase buffer (0.5 M NaCl + 0.5 M Tris at pH 9.5 + 50 mM MgCl2 + 0.1% Tween-20 + DEPC H2O) at 4°C with constant buffer replacement until signal was detected. Color reactions were stopped by washing five times for 10 min each with PTw.
Immunocytochemistry
Trochophore larvae (13 hpf) were fixed in 4% PFA for 1 h, then washed and stored at 4°C in 1x PBS with 0.1% sodium azide added. Afterward, samples were permeabilized by three washing steps of 15 min in 1x PBS + 0.2% Triton X-100 + 0.1% NaN3 (PTA). Blocking was performed in PTA + 6% normal goat serum (NGS) overnight at 4°C. Primary antibody incubation was performed over night at 4°C with anti-acetylated α-tubulin (raised in mouse, monoclonal, #T6793, Sigma-Aldrich) at a concentration of 1:400 diluted in PTA + 6% NGS. Two washes with PTA of 15 min each followed. For the secondary antibody, goat anti-mouse Alexa 568 (#A-11004, Thermo Fisher Scientific, Waltham, MA, United States) was added and the samples were incubated overnight at 4°C. This was followed by two washes with PBS for 15 min each. After that, a mix of CellMask Green Stain (#H32714, Invitrogen, Carlsbad, CA, United States; 1:100) and DAPI (#D1306, Sigma-Aldrich, 1:2000) in PBS was added for 1 h. Then, the samples were washed thrice with PBS for 15 min each and mounted on glass slides with Fluoromount-G (#0100-01, SouthernBiotech, Birmingham, AL, United States) and stored at 4°C for a few days prior to image collection.
Image Collection and Figure Processing
Trochophore larvae (13 hpf) used for in situ hybridization were mounted in 100% glycerol and documented with an Olympus BX53 Microscope (Olympus, Hamburg, Germany). Immunofluorescent antibody preparations were scanned with a Leica TCS SP5 II confocal microscope (Leica Microsystems GmbH, Wetzlar, Germany). FIJI (Schindelin et al., 2012) was used for image analysis and further processing to estimate cell number in a larva through nuclei counting, employing the 3D Object Counter v2 with a threshold of 75. All figures, light micrographs, and graphical representations were prepared, compiled, and adjusted for contrast and brightness using Inkscape version 0.92.4-4 (Inkscape Project, 2020).
Results
De novo Cell Cluster Annotation and Marker Gene Identification in a Bivalve Trochophore Larva
Based on nuclei counts from immunostainings, we estimated that the trochophore larva of the quagga mussel Dreissena rostriformis is composed of approximately 110 cells. Therefore, we aimed to sequence ∼1000 cells with the 10x Genomics platform. In total we sequenced 41325580 reads and 84.9% of these reads mapped to the reference genome, i.e., the remaining percentage could not be mapped to either exonic, intronic, intergenic regions or antisense genes. After filtering out cells with less than 150 molecules and with more than 4000 sequenced molecules, a total of 632 cells were captured, corresponding to around five individuals (Figures 1A,B). The number of genes detected in a cell (nFeatures) have a median of 1054.5 and a mean of 1141.8, while the number of molecules detected within a cell (nCount) have a median of 3253 and a mean value of 4172.8. After processing the cell count matrix with the Seurat pipeline, the heterogeneity of cell states in the trochophore larvae was assessed. Graph-based clustering revealed seven transcriptionally distinct cell populations (Figures 2A–G). Analysis of the genes underlying this clustering, as described below, identified cell populations corresponding to “ciliary/prototroch,” “neuronal,” “endoderm,” “muscle,” “epidermal,” “shell field,” and “undifferentiated” identities (Figures 3A,4A).
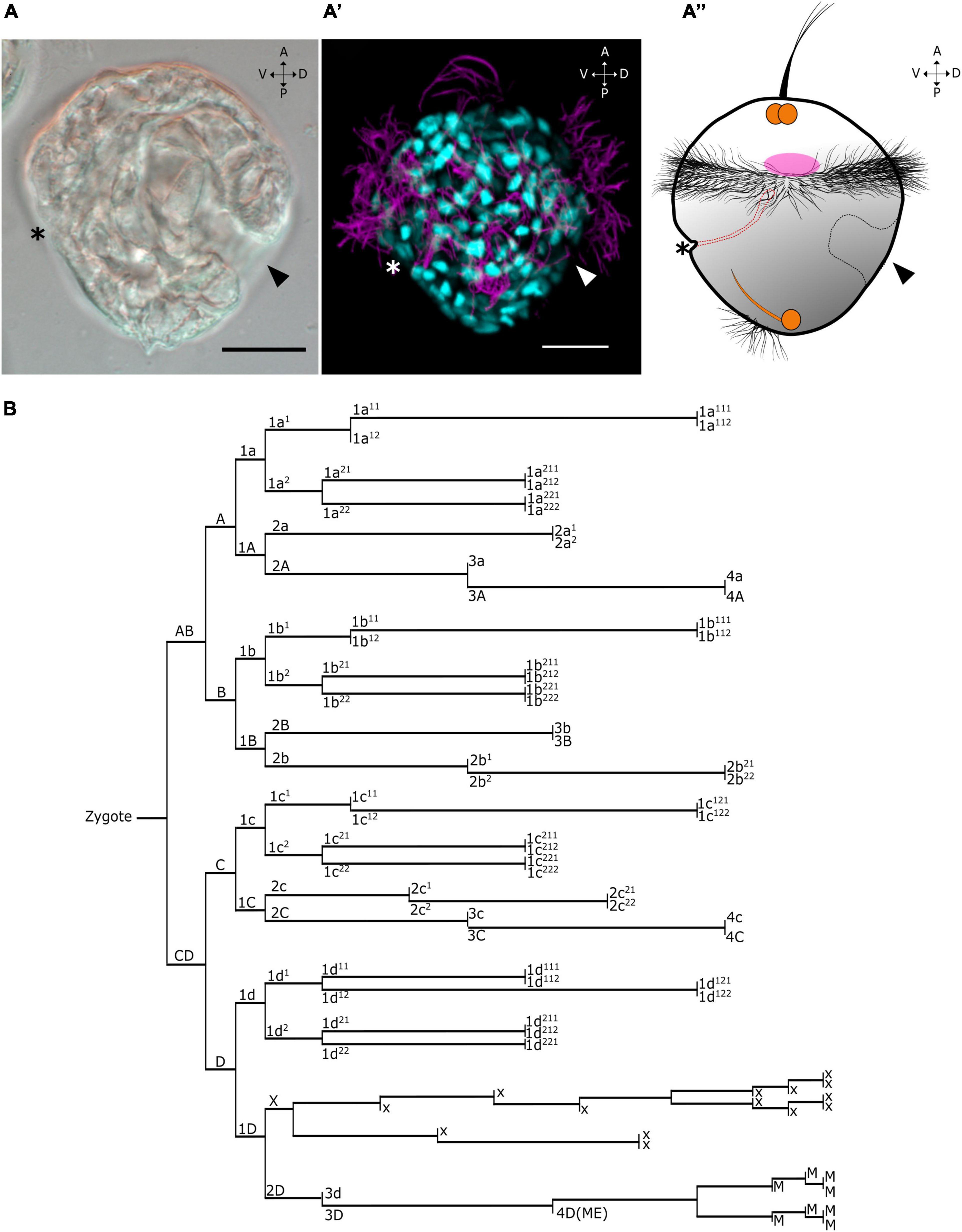
Figure 1. Single-cell transcriptomics of Dreissena rostriformis larvae. Age of larvae is 13 hpf. (A–A”) Lateral view of differential interference contrast (left), alpha-acetylated tubulin/DAPI immunostained trochophores (center), and scheme illustrating the overall morphology of the trochophore larva (right). Asterisk on the larvae indicates the mouth opening. Arrowhead points to the shell field on the right side. Orange marked areas denote the developing nervous system based on data from Pavlicek et al. (2018). Pink marked area denotes the developing muscular system. Dotted black lines highlight shell field cells. Dotted red lines highlight the developing digestive system. Anterior (A), posterior (P), ventral (V), and dorsal (D). Scale bars equal 20 μm. (B) Cell lineage tree of Dreissena modified from Meisenheimer (1900) and Luetjens and Dorresteijn (1995).
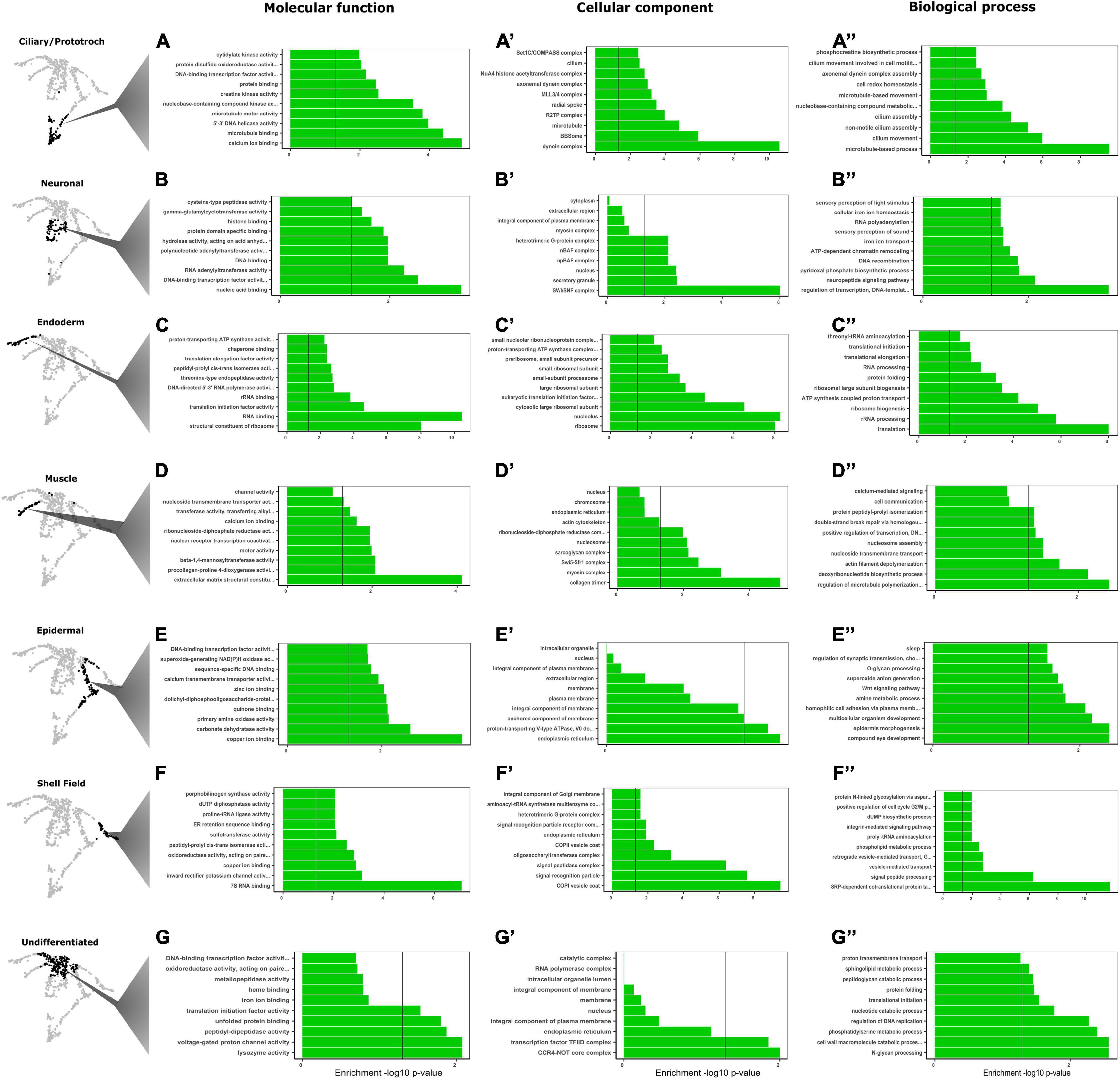
Figure 2. Gene ontology (GO) analyses leads to identification and validation of clusters of differentially expressed genes. (A–G”) GO terms associated with molecular function, cellular component, and biological processes. Classification of the corresponding GO terms form each highlighted cluster on the left side of the panel. Each row represents a cluster with significant transcriptomic signature and each column shows the categories of enrichment by molecular function, cellular component, and biological processes. Vertical black line (p-value = 0.05) in each graph represents statistical significance threshold set after performing a Fisher’s exact test.
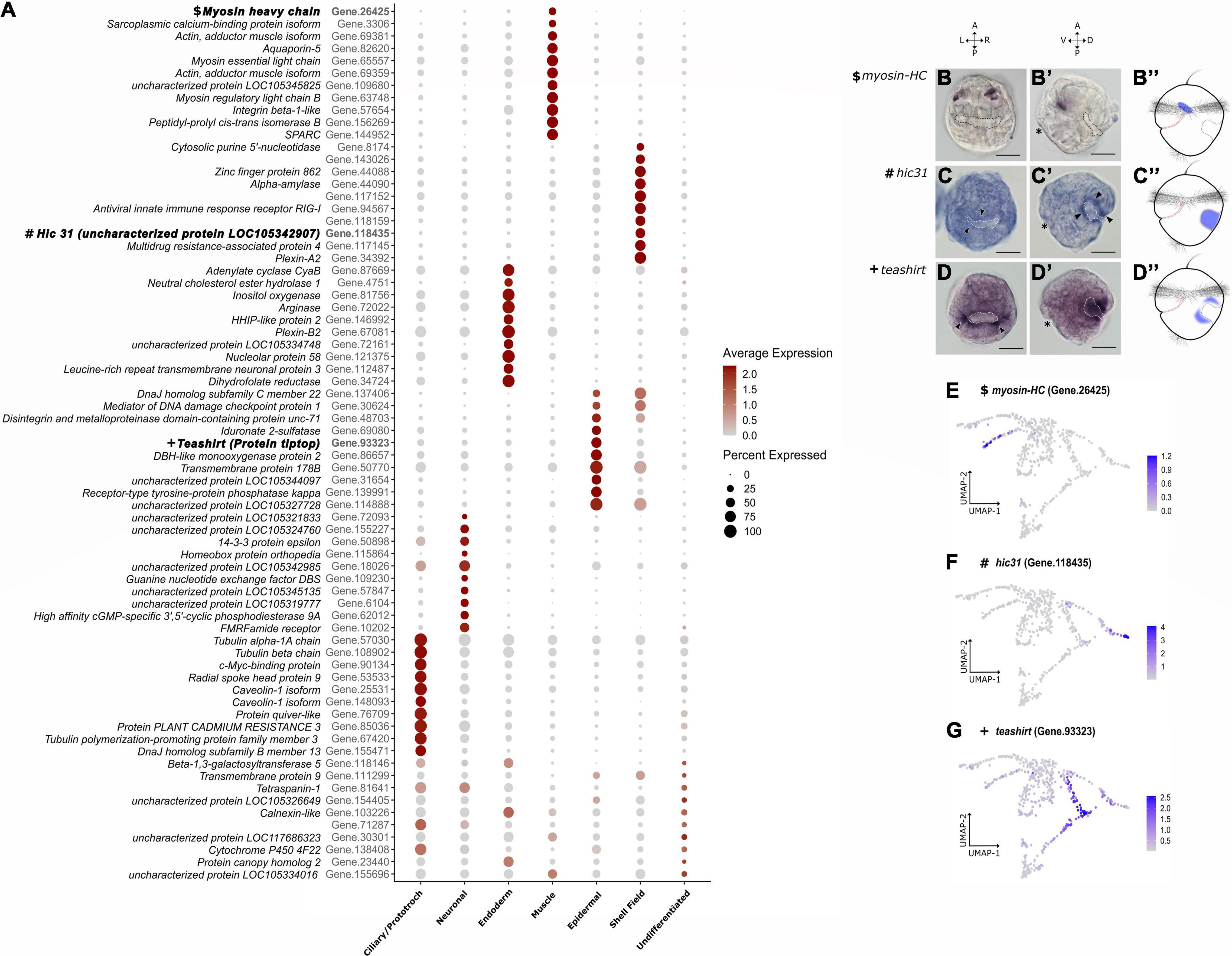
Figure 3. Mapping cell state markers on the trochophore larva. (A) Top 10 highly expressed genes per cluster. On the left side of each gene is the putative ortholog match from Crassostrea gigas. Color intensity represents the average gene expression while circle size is the percentage of cells expressing a certain gene. In bold are the IDs of the genes used for cluster validation through in situ hybridization in (B–D). (B–D”) Expression domains of randomly sampled marker genes from a dorsal (B–D) and a lateral point of view (B’–D’). (B–B”) Mhc expression located in cells from the anterior region between the developing digestive tract and the shell field. Dotted lines highlight the secreted shell field. (C–C”) Hic31 expression located in cells belonging to the outer margin of the shell field (arrowheads). (D–D”) Tsh expression is found in the epidermal cells limiting the outline of the shell field. Asterisk indicates the mouth opening on the left side. Anterior (A), posterior (P), ventral (V), dorsal (D), left (L), and right (R). Scale bars are 20 μm. (E–G) Feature plots of each respective gene used for in situ hybridization and after running the gene imputation algorithm with MAGIC.
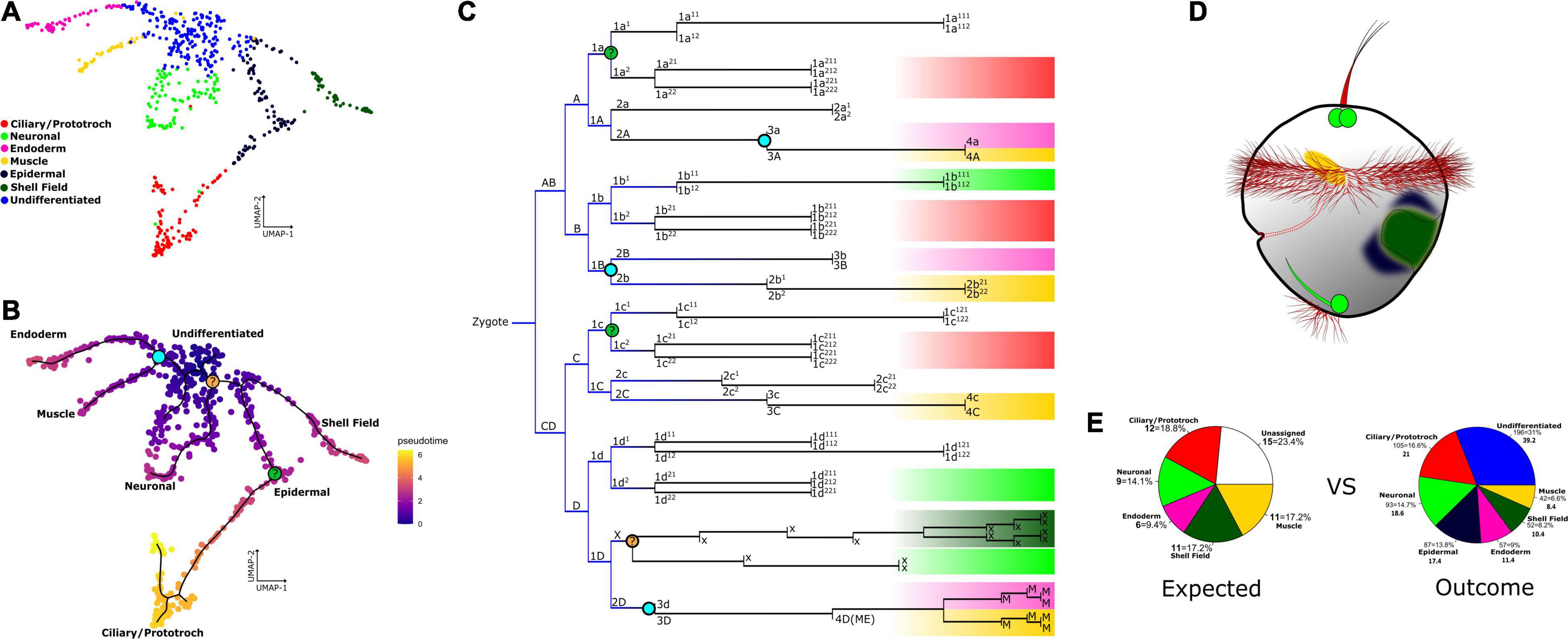
Figure 4. Cell clusters mapped onto the Dreissena cell lineage tree and their location in the trochophore larva. (A) Resulting UMAP graph with cluster identification result of our annotation pipeline. Identified cell clusters are named and color-coded. (B) Calculated pseudotime showing recovered differentiation trajectories among extant clusters. Black lines represent the learned pseudotime trajectories. Colored nodes marked in the trajectory pathways and in the cell lineage tree represent the possible developmental decisions inferred from pseudotime and are drawn into the cell lineage map. The aquamarine node represents the developmental decision between endodermal and muscle cells. Light orange and light green represent the nodes that do not have a certain position on the fate map. (C) Cell lineage tree of Dreissena modified from Meisenheimer (1900) and Luetjens and Dorresteijn (1995). Highlighted are the putative terminal cell identities with the same color code from the UMAP projection. Blue gradient on the lines of early cell lineages represents the multipotency of the cells, which is lost in further cell divisions to acquire identity and could still be present in some lineages. Cell lineages not colored here mean the fate of these cell lineages was not identified in the fate map of Dreissena. (D) Putative location of clusters on the larva based on annotation methods described here. (E) Pie charts showing the percentage of cell types based on counts from cell lineage studies for one single larva (left, “expected”) vs. the percentage of cell types based on counts of this study from five larvae (right, “outcome”).
Transcriptional Domains Confirm Cell Type Identity in the Larva
Ciliary cells in Dreissena start to develop early in the gastrula stage. GO terms analysis revealed one cluster of cells with a statistically significant enrichment in genes involved in processes related to cilia assembly, movement and cell motility, and microtubule-based processes (Figures 2A–A″), thus identifying a “ciliary/prototroch” cell population within the dataset. Moreover, further sub-structure within this group is exhibited when the clustering resolution parameter is increased (Supplementary Figures 1I–K and Supplementary Table 3). In the larva, there are several subtypes of ciliated cells such as prototroch, telotroch, and apical tuft cells (Meisenheimer, 1900; Pavlicek et al., 2018). The structure seen in this cluster could reflect the different subtypes of ciliary cells in the live animal.
Cells identified as “neuronal” present highly expressed genes where there is an enrichment of neurogenesis-related processes such as neuropeptide signaling pathway, proton transmembrane transport (iron ion transport), and acetylcholine receptor regulator activity (Figures 2B–B″ and Supplementary Tables 1, 2). When increasing the resolution for finding clusters, results revealed subclusters within this population (Supplementary Figures 1I–K and Supplementary Tables 2, 3). Even though the two resulting subclusters have equal enrichment for neuronal processes, they have differential expression of markers setting them apart from each other (Supplementary Tables 2, 3). For example, while the red subcluster has predominant expression of FMRFamide receptor, the turquoise one on the right presents high expression of neuronal acetylcholine receptor subunit alpha-6 (Supplementary Figures 1I, 4C,D). Annotations from transcriptomic signatures in the cluster “neuronal” indicate that neuropeptides are secreted from these cells. Accordingly, in-depth analysis of gene expression signatures of this cluster appears particularly promising for characterizing the gene regulatory networks responsible for the differentiation of neuronal cells.
The cluster “endoderm” shows all related cells share gene annotations from human and Crassostrea orthologs related to enzymatic reactions that are likely to occur in the developing digestive system, e.g., arginase-1 (Gene.72022), cathepsin K preproprotein (Gene.17238), neutral cholesterol ester hydrolase-1 (Gene.4751) (Kirschke et al., 1995; Duruz et al., 2020), but also included immune response-related genes, e.g., galectin-8 (Gene.117090) (Vasta et al., 2015). Moreover, GO terms for processes related to ribosome biogenesis, RNA processing, translation, and ATP synthesis-coupled proton transport show enrichment in this cluster (Figures 2C–C″). Since all these activities are crucial for cell survival, one could assume the enriched processes are crucial for all cell states at this stage and some of the genes are expressed in other clusters. However, the annotations from the top differentially expressed genes identified in this cluster suggest that these cells are involved in endoderm development and immune responses (Supplementary Figure 4). Altogether, cells in this cluster are highly likely to have endodermal fates in the quagga mussel larva.
Bivalves have two predominant adductor muscles of which the anterior adductor and some larval retractor systems already start to form in the trochophore larva (unpublished observation). We identified cells with muscle identity in our dataset on the “muscle” cluster where there is gene ontology enrichment with processes associated with muscle system formation such as calcium ion binding, actin filament depolymerization, and protein peptidyl-prolyl isomerization (protein folding) (Figures 2D–D″ and Supplementary Table 1). In addition, the commonly expressed marker during animal myogenesis, myosin heavy chain (mhc; Castellani and Cohen, 1987; Ladurner and Rieger, 2000; Dyachuk and Odintsova, 2009; Li et al., 2019), is expressed in this cluster. In the Dreissena trochophore, transcripts of mhc are found in the anterior mesoderm. They form spot-like expression domains in the anterior region between the developing digestive tract and the shell field (Figures 3B–B″,E). Hence, in silico annotations and in situ hybridization data support the identity of muscle cells for this cluster.
Cells with an “epidermal” identity formed a cluster with GO terms enrichment in processes that play a role as integral components of the membrane, such as epidermis morphogenesis and homophilic cell adhesion via plasma membrane (Figures 2E–E″ and Supplementary Table 1). To visualize these cells in the larva, in situ hybridization of one of the top marker genes was performed. The gene teashirt (tsh) has an expression domain in cell populations adjacent to the anterior and posterior margins of the cells forming the shell gland (Figures 3D–D″,G). This gene expression domain and GO term annotations suggest that this cluster contributes to the formation of various types of epithelia and epidermal tissue in the trochophore larva of Dreissena.
Dreissena trochophores exhibit a developing shell field which grows and envelops the visceral region in later stages. We identified cells belonging to the cluster “shell field,” which express several genes with unknown ontology term or ortholog match. Cells belonging to this cluster express numerous short-numbered-kilobases genes, which match hitherto uncharacterized molluscan genes (Supplementary Table 1). Nevertheless, the few GO terms collected show enrichment in processes that interact with the extracellular matrix, such as integrin-mediated signaling pathway and positive regulation of cell cycle G2/M phase progress (Figures 2F–F″ and Supplementary Table 1). From the set of highly expressed genes forming the transcriptomic signature in this cluster, hic31 was chosen to visualize the cluster in the Dreissena larva because it was previously shown to be involved in establishing the protein matrix prior to shell secretion (Liu et al., 2015, 2018). In Dreissena trochophores, hic31 expression is present around the margin of the shell field (Figures 3C–C″,F). Thus, gene annotations and in situ hybridization data support the identity of shell field cells for this cluster.
We identified one cluster devoid of a unique transcriptional signature (Figure 2G). These cells were considered “undifferentiated” because their GO term annotations showed that they have significant levels of regulation of DNA replication, protein synthesis, and translational initiation in the Dreissena trochophore (Figures 2G–G″). The predominant expression of such “housekeeping genes” as well as the shared expression signatures of these genes with all clusters, in combination with a lack of distinct expression of marker genes (Supplementary Figure 4 and Supplementary Tables 1–3), is a typical feature of animal cells that have not yet undergone differentiation into developmental fates and is often observed in whole-organism single cell RNA-seq datasets (Hemmrich et al., 2012; Fincher et al., 2018; Siebert et al., 2019; Duruz et al., 2020). This cluster is composed of cells with low gene counts (151–1332), suggesting that these cells lack sufficient information to robustly cluster with similar cell populations and thus could represent a clustering artifact. In this case, this cell population would be expected to disappear with more stringent filtering (min. 500 detected genes), allowing for more robust clustering of the remaining cell populations. However, removing these low information cells does not improve clustering but rather shifts the cluster boundary, resulting in a cluster of cells with less information (Supplementary Data 2). Although this may represent a technical artifact, it could also be interpreted as a real biological signal at this stage of development wherein many of the embryonic cells are in a permissive cell state prior to activating cell differentiation pathways. In addition, results from StemID, an algorithm for predicting multipotent cell identities (Grün, 2020), suggest a statistically supported link of all clusters to these cells in the trochophore stage and indicate there is a starting point for differentiation trajectories in this cluster (Supplementary Figure 3). Altogether, in silico analyses support the notion that this cluster is composed of cells which have not yet developed a unique transcriptomic signature and thus the identified developmental trajectories are differentiated from this cluster.
Pseudotime Trajectories Infer Relationships Between Cell Populations
We aimed to identify trajectories and reconstruct lineages from the cell clusters of Dreissena rostriformis trochophore larvae. While running the Monocle3 algorithm without any assumptions a priori, eight major trajectory ends could be recovered to model a differentiation tree with all cell populations and relate them to one single root, the “undifferentiated” cluster (Figure 4B). Two trajectory ends correspond to the clusters enriched with processes related to muscle development, endoderm development, and ribosomal activity (Figures 2C–D′, 3B). “Muscle” and “endoderm” originate from a common cell lineage, the corresponding cell division can be observed on the node linking these two cell types (Figure 4C). The remaining trajectories show terminal ends in neuronal and ciliary clusters (Figure 4B). The presence of multiple differentiation pathways in these clusters supports further structure within the respective cell groups. There is one trajectory linking shell field and several epidermal cells (Figure 4B). This suggests a specification pathway that employs a subset of epithelial cells destined to contribute to the shell field. To assign putative terminal fates on the lineage tree of Dreissena and link them to our annotated clusters, we analyzed defined fate maps from other mollusks and made an estimation on the expected cell types per individual (Figure 4C). We then compared the number of expected clusters from the revised lineage tree of Dreissena to the actual number of cells obtained in our dataset (Figure 4E). Thereby, the “expected” cluster cells were counted from a developmental stage that was approximately one cell division cycle younger (67 cells) than that of the “outcome” cluster (110 cells). This was done to assess whether the same cell types described in classical cell lineage studies were also recovered by our single cell RNA-seq approach. While the proportions of expected versus outcome match well for the “neuronal,” “ciliary,” and “endoderm,” the proportion of “shell field” and “muscle” cells show a higher proportion in the expected than in the actual outcome analysis. This could be due to the annotation of previously unrecognized cell types on the lineage tree from Dreissena that might affect the cluster proportions of the “outcome” (Figure 4E). For example, concerning the shell field and epidermal cells, that both derive from the ectoderm, these might not yet have undergone fate specification in the analyzed developmental stage and thus might all fall in one category (here: shell field) in the “expected” analysis. The higher value for “muscle” cells in the “expected” chart relative to the “outcome” one might be because considerably fewer cells have undergone differentiation into muscle cells, a situation that is congruent with morphological analyses that showed a strikingly simple myoanatomy of Dreissena trochophores (Meisenheimer, 1900; Pavlicek et al., 2018, unpublished observations). Overall, the trajectories traced across the whole dataset position extant clusters uniformly along each respective path. This shows that developmental trajectories are well sampled in our dataset. In addition, it confirms that our cluster annotations recover the correct proportions of expected cell types from the lineage tree and that the pseudotime analysis is a good indicator of differentiation paths in the live larva.
Discussion
Verification of Cluster Identity by Ortholog Comparison Across Metazoa
The trochophore larva of the quagga mussel is comprised of well-defined features such as a shell field, a ciliated prototroch, telotroch and apical tuft, a simply-built nervous system, and a developing digestive tract (Pavlicek et al., 2018; Salamanca-Díaz et al., 2021). Our single cell transcriptomic atlas of the Dreissena trochophore provides the first detailed analysis of the transcriptomic toolkit of a larval bivalve mollusk at single cell resolution, capturing cells corresponding to each of these morphological features (Figures 4A,D). Overall, our transcriptomic annotations in the quagga mussel Dreissena rostriformis confirm that key molecular factors expressed in all clusters are comparable to other species. As such, the cells identified as “ciliary/prototroch” are enriched in genes involved in ciliogenesis and cilia movement and the development of the dynein complex known to be a crucial part of locomotion in many metazoans (King, 2012). Ciliated cells found in the annelids Platynereis dumerilii, Hydroides elegans, and Capitella teleta similarly express orthologs of Caveolin, Forkhead box J (foxJ), rshp4, dynein heavy chain 9 (DNAH9), tubulin polymerization-promoting family member 3, and tektin4 (Supplementary Figure 4 and Supplementary Tables 1–3; Arenas-Mena et al., 2007; Achim et al., 2018; Sur and Meyer, 2021). Furthermore, genes expressed in ciliary cells of Dreissena are also present in motor cilia of more distantly related organisms such as the acoel Isodiametra pulchra (i.e., Dynein heavy chain and tubulin homologs) and in ciliary band cells of the sea urchin Strongylocentrotus purpuratus (e.g., foxJ) (Duruz et al., 2020; Paganos et al., 2021). This shows that the “ciliary/prototroch” cells in our data possess a distinct transcriptomic signature indicative of the ability to produce cilia, and that at least some of this molecular program was present in the last common ancestor of bivalves, annelids, sea urchins, and xenacoelomorphs.
We identified cells that have ontology enrichment of neuroreceptors crucial for peptide signaling and factors related to mechanosensation and photosensation (Figures 2B–B″). Additionally, this cluster shows high expression of orthologs that play a role in the differentiation of the anlagen for the developing larval neurosecretory cells, e.g., FMRFamide receptor, neuronal acetylcholine receptor subunit alpha-6 and neuregulin 2, in the bivalves Macrocallista nimbosa, Mizuhopecten yessoensis, and Mytilus galloprovincialis (Supplementary Figure 4 and Supplementary Tables 1–3; Price and Greenberg, 1977; Wang et al., 2017; Gerdol et al., 2020). This indicates the presence of a neurosecretory center in this cluster that contains the key machinery for neuronal differentiation and suggests that the neuroanatomy of Dreissena trochophore larvae may be more complex than currently appreciated by morphological data using fluorescence-coupled antibody staining (Pavlicek et al., 2018).
Cells identified as “endoderm” expresses orthologs linked to protein breakdown processes and catalytic reactions which take place in the digestive system (Figures 2C–C″; Kirschke et al., 1995; Duruz et al., 2020). This is supported by the expression of crucial functional orthologs such as hepatocyte nuclear factor 4 (hnf4), hematopoietically expressed homeobox gene (hhex), and galectin. Galectin has been reported to promote phagocytosis of pathogens as a defense mechanism present in all cells and in hemocytes in adult stages, playing a role in innate immune responses and intracellular digestion in aquatic mollusks (Vasta et al., 2015). Hnf4 is considered a marker of developing endoderm since its expression has been found in gut stem cells in several metazoans (Achim et al., 2018; Wendt et al., 2020; Paganos et al., 2021; Sur and Meyer, 2021) and was highly expressed exclusively in this cluster (in a limited number of cells only). Hhex is considered a regulator of hepatocyte development in deuterostomes (Wallace et al., 2001; Evseeva et al., 2021; Paganos et al., 2021) and was also found to be expressed in cells of this cluster (Supplementary Figure 4 and Supplementary Tables 1–3). Although detection of these transcriptional markers is restricted to few cells, this is not uncommon for transcription factors, and overall the molecular fingerprint of this cluster is coherent with the developing gastrodermis.
Dreissena muscle progenitor cells consistently express common muscle and mesodermal markers. Among the gene orthologs identified in the “muscle” cluster, expression of twist, Hand, Mox, and myosin heavy chain (mhc) were detected (Figures 3A,E and Supplementary Figure 4). These genes are typically involved in mesoderm and/or muscle formation across metazoan clades (Castellani and Cohen, 1987; Ladurner and Rieger, 2000; Nederbragt et al., 2002; Dyachuk and Odintsova, 2009; Dobi et al., 2015; Achim et al., 2018; Li et al., 2019). In addition, there is gene ontology enrichment of several processes associated with muscle differentiation such as calcium ion binding and actin filament depolymerization (Figures 2D–D″).
The cluster “epidermal” shows enrichment of epidermis-related processes such as its morphogenesis and cell adhesion (Figures 2E–E″). Moreover, this cluster expresses orthologs of epidermal cells from the acoel Isodiametra pulchra and the annelid Capitella teleta that are associated with extracellular matrix factors and tight junctions such as annexin, cadherins, and claudin family members (Supplementary Figure 4 and Supplementary Tables 1–3; Duruz et al., 2020; Sur and Meyer, 2021). Claudin is a transmembrane protein that is a crucial component of tight junctions in cells and cadherins are a type of cell adhesion molecules important for epithelium formation (Broders and Thiery, 1995; Krause et al., 2008; Piontek et al., 2008; Shapiro, 2016). For annexin, while playing a role in calcium-dependent membrane-binding and constituting an ectodermal marker in several metazoans (Meyer and Seaver, 2009; Duruz et al., 2020; Sur and Meyer, 2021), our results find this gene expressed also in ciliary and shell field cells (Supplementary Figure 4 and Supplementary Tables 1–3). Hence, these shared transcriptomic signatures suggest that the precursor for both clusters have an ectodermal origin. Overall, the ortholog search supports the identity of this cluster by allowing one to one comparisons of cluster markers from different animals.
Orthologs of genes expressed in the shell field cluster that are known to play a role in early molluscan shell formation include Hox1, chitin binding protein, and hic31 (Supplementary Figure 4 and Supplementary Tables 1–3; Kakoi et al., 2008; Kin et al., 2009; Tan et al., 2017; Wollesen et al., 2018; Zhao et al., 2018; Liu et al., 2020; Salamanca-Díaz et al., 2021). Transcripts expressed here show ontology enrichment in processes such as peptide signaling and plasma membrane interactions, linking the activity of these cells to shell building mechanisms (Figures 2F–F″). However, most of the upregulated genes in this cluster do not have a known ontology term or an ortholog match with other metazoans (Supplementary Tables 1–3). Previous studies analyzing gene products expressed in shell secreting mantle tissues of various bivalves and gastropods found that, despite having a common biomineralization toolbox, the shell matrix expresses many novel, duplicated, and reorganized genes. These features have led to the conclusion that molluscan shells are “rapidly evolving” (Jackson et al., 2006; Aguilera et al., 2017; Zhao et al., 2018). This opens the possibility for further analyses in Dreissena shell field cells and to test whether these markers constitute hitherto unknown genes.
Enhancing the Cell Fate Map of Dreissena
Cell groups were linked together by tracing pseudotime trajectories across our dataset (Figure 4B). As these trajectories are represented in function of pseudotime, they can be used as a bioinformatical approximation of developmental decisions. By comparing terminal fates from other molluscan lineage trees to our results, we were able to link scRNA-seq data to the cellular lineage tree of Dreissena (Meisenheimer, 1900; Figure 1B). The “muscle” and “endoderm” clusters are separated here by a node in the scRNA-seq dataset (Figure 4B). According to cell fate maps from Dreissena and other mollusks, there is a significant portion of the endoderm that originates from mesodermal lineages (Nielsen, 2005; Kurita et al., 2009; Chan and Lambert, 2011; Gharbiah et al., 2013; Lyons et al., 2017). Therefore, since these clusters have an origin in a common cell lineage, the node between these two groups may mark the corresponding cell division visualized in the cell fate map (Figure 4C). On the other hand, the clusters seen in the rest of the built trajectories, i.e., shell field and epidermal, neuronal and ciliary/prototroch (Figures 4B,C), have their origin in macromeres composed mainly of the ectodermal germ layer (Nielsen, 2005; Kurita et al., 2009; Chan and Lambert, 2011; Gharbiah et al., 2013; Lyons et al., 2017). Furthermore, after increasing cluster resolution and analyzing ortholog gene expression in both, ciliary and neuronal cells, there was occurrence of more than one subtype in each cluster (Supplementary Figures 1I–K). This was supported by the trajectory analyses that display multiple ends in both ciliary and neuronal cells (Figure 4B). We suggest that this diversity within a cluster could represent the different subtypes of ciliary and neuronal cells seen in the live larva, i.e., apical tuft, prototroch, telotroch, FMFRamide receptor-positive and neuronal acetylcholine receptor subunit alpha-6-positive cells, respectively (Supplementary Figure 4 and Supplementary Tables 1–3). Similar cluster diversity in neuronal cell types is found in single cell datasets from other metazoans such as the annelid Capitella teleta and the sea urchin Strongylocentrotus purpuratus. All neuronal cell types of these species share a baseline transcriptomic signature, with only enough differences in expression to subdivide the overall cluster into different categories, e.g., 12 neuronal subtypes in the sea urchin and four neural subgroups in C. teleta (Achim et al., 2018; Foster et al., 2020; Paganos et al., 2021). Moreover, when analyzing the cell lineage tree of Dreissena, our data highlights that the underlying transcriptomic signature is similar across lineages that give rise to the same fate (Figure 3C).
Conclusion
Our analyses identified all expected major cell populations in the trochophore larva of the quagga mussel, Dreissena rostriformis (Figure 4E). When comparing our results to studies on other metazoans, we were able to support previous morphological annotations from cell groups such as “ciliary,” “neuronal,” “muscle,” “endoderm,” and “shell field.” Our cluster validations, such as the BLASTX search, GO term annotations, and in situ hybridization identified marker genes for each of these cell types. Moreover, we were able to gain a first glimpse into developmental decisions, e.g., “endoderm” + “muscle,” and cluster structure within already known cell lineages, e.g., “ciliary/prototroch” and “neuronal.” Our data demonstrate that the levels of differentially expressed genes can be used as markers to identify clusters of cells in early developmental (larval) stages of (bivalve) mollusks, thus adding these animals to the suite of taxa that can now be accessed for comparative evolutionary studies on cell type level using scRNA-seq techniques. This should ultimately lead to a better understanding as to how animals form and which genes and cell types are involved in shaping larval and adult life cycle stages across Metazoa.
Data Availability Statement
The data presented in this study are deposited in NCBI’s Gene Expression Omnibus and are accessible through the GEO series accession number GSE192624 (https://www.ncbi.nlm.nih.gov/geo/query/acc.cgi?acc=GSE192624).
Author Contributions
DS-D, AC, and AW designed the research and contributed to interpretation of data. DS-D performed the experiments, generated data with contribution of AC, performed the data analysis with assistance of AC, and drafted the manuscript with input from AW and AC. AC generated the sequencing library from cell suspensions provided by DS-D. SS contributed to gene annotation from the muscle cluster and performed in situ hybridization of myosin heavy chain. All authors read and approved the final version of the manuscript.
Funding
This work was supported by a completion grant of the Vienna Doctoral School of Ecology and Evolution (VDSEE) to DS-D and by the Austrian Science Fund (FWF) (grant P29455-B29 to AW and P31018-B29 to AC).
Conflict of Interest
The authors declare that the research was conducted in the absence of any commercial or financial relationships that could be construed as a potential conflict of interest.
Publisher’s Note
All claims expressed in this article are solely those of the authors and do not necessarily represent those of their affiliated organizations, or those of the publisher, the editors and the reviewers. Any product that may be evaluated in this article, or claim that may be made by its manufacturer, is not guaranteed or endorsed by the publisher.
Acknowledgments
The authors thank Julia Steger and Oliver Link (Vienna) for their support and valuable input when developing the dissociation suspension and assisting the first experimental trials, and Hannah Schmidbaur and Elena A. Ritschard (Vienna) for bioinformatics advice on the gene annotations. The authors also thank Uli Technau for hosting DS-D during this work.
Supplementary Material
The Supplementary Material for this article can be found online at: https://www.frontiersin.org/articles/10.3389/fevo.2021.783984/full#supplementary-material
Supplementary Figure 1 | (A) Barcode plot showing the cumulative distribution of UMIs in function of barcodes. Violin plots show feature distribution for the overall data before (B) and after (C) setting thresholds to the data, and in every cluster (D) from the dataset. (E) Scatter plot showing the correlation between features, Pearson correlation is shown on top of the plot. (F) Top 2000 most variably expressed genes used for further calculation of significant principal components. Cells were filtered out based on less than 150 and more than 4000 features. (G) Elbow plot showing the standard deviations of the calculated principal components. (H) Statistics table on the single cell transcriptome libraries showing sequencing, mapping, and cell metrics summary for this study. (I–K) UMAP graphs result of an increased cluster-finding algorithm resolution with 0.7, 1, and 2 as resolution threshold, respectively.
Supplementary Figure 2 | Dataset exploration on different filtering thresholds. (A,C,D,G,H,K) Represent the data after filtering out cells with less than 300 and more than 4000 genes, resulting in 490 cells. (B,E,F,I,J,L) Represent the data after filtering out cells with less than 500 and more than 4000 genes, resulting in 430 cells. (A,B) Violin plots showing feature and count distribution for the filtered data, with more than 300 and 500 genes, respectively. (C,E) Original clustering highlighted in the filtered data, with more than 300 and 500 genes, respectively. (D–J) UMAP graphs result of an increased cluster-finding algorithm resolution in filter settings of more than 300 and 500 genes, with 0.5, 1, and 5 as resolution threshold, respectively. (K,L) Pseudotime trajectory calculations for each respective filtered data threshold.
Supplementary Figure 3 | Prediction of a stem cell-like cluster at the root of the differentiation pathways based on the initial clustering. The entropy values are shown as color of the vertices. The color of the link represents the −log10 p-value. Thickness of the link indicates the score, reflecting cell density coverage on a link.
Supplementary Figure 4 | (A) Orthologs known to be stem cell markers identified in our dataset, expressed across all clusters. (B) Dot plot of ortholog gene expression per cluster. (C) Orthologs identified in the dataset used to annotate cluster identities. (D) Dot plot of ortholog gene expression per cluster. Color intensity represents the average gene expression while circle size is the percentage of cells expressing a certain gene.
Supplementary Figure 5 | Negative controls (sense riboprobes) of in situ hybridization experiments for each randomly sampled marker gene used for cluster annotation. (A–C) Lateral views of mhc, hic31, and tsh, respectively. Anterior (A), posterior (P), ventral (V), and dorsal (D). Scale bars are 20 μm. Dotted lines indicate the outline of the shell field. Asterisk indicates the mouth opening on the left side.
Supplementary Table 1 | Gene annotations of the transcriptome used for this dataset. Annotations from Pfam, PANTHER, GO terms, and BLASTX hit from human and Crassostrea gigas orthologs are indicated in each column accordingly.
Supplementary Table 2 | Differentially expressed markers per cluster result from the output FindAllMarkers function with a cluster threshold set up to 0.5.
Supplementary Table 3 | Top 10 differentially expressed markers per cluster result from the output FindAllMarkers function with a cluster threshold set up to 0.7.
Supplementary Table 4 | List of primers and nucleotide sequence for each gene used for cloning. Forward and reverse primers used for amplifying each gene by PCR are indicated.
Supplementary Table 5 | Explored cutoffs in the dataset and the number of remaining cells of each cluster after each filtering. Thresholds were set from more than 100 genes and less than 4000 to more than 500 genes and less than 2500, respectively. Dotted lines indicate the outline of the shell field and the symbol * indicates the mouth opening on the left side.
References
Achim, K., Eling, N., Vergara, H. M., Bertucci, P. Y., Musser, J., Vopalensky, P., et al. (2018). Whole-body single-cell sequencing reveals transcriptional domains in the annelid larval body. Mol. Biol. Evol. 35, 1047–1062. doi: 10.1093/molbev/msx336
Achim, K., Pettit, J.-B., Saraiva, L. R., Gavriouchkina, D., Larsson, T., Arendt, D., et al. (2015). High-throughput spatial mapping of single-cell RNA-seq data to tissue of origin. Nat. Biotechnol. 33, 503–509.
Aguilera, F., McDougall, C., Degnan, B. M., and Irwin, D. (2017). Co-option and de novo gene evolution underlie molluscan shell diversity. Mol. Biol. Evol. 34, 779–792. doi: 10.1093/molbev/msw294
Aldridge, D. C., Ho, S., and Froufe, E. (2014). The ponto-caspian quagga mussel, Dreissena rostriformis bugensis (Andrusov, 1897), invades Great Britain. Aquat. Invasions 9, 529–535.
Alexa, A., Rahnenführer, J., and Lengauer, T. (2006). Improved scoring of functional groups from gene expression data by decorrelating GO graph structure. Bioinformatics 22, 1600–1607. doi: 10.1093/bioinformatics/btl140
Arenas-Mena, C., Wong, K. S.-Y., and Arandi-Forosani, N. (2007). Ciliary band gene expression patterns in the embryo and trochophore larva of an indirectly developing polychaete. Gene Expr. Patterns 7, 544–549. doi: 10.1016/j.modgep.2007.01.007
Arendt, D., Musser, J. M., Baker, C. V. H., Bergman, A., Cepko, C., Erwin, D. H., et al. (2016). The origin and evolution of cell types. Nat. Rev. Genet. 17, 744–757. doi: 10.1038/nrg.2016.127
Briggs, J. A., Weinreb, C., Wagner, D. E., Megason, S., Peshkin, L., Kirschner, M. W., et al. (2018). The dynamics of gene expression in vertebrate embryogenesis at single-cell resolution. Science 360:eaar5780. doi: 10.1126/science.aar5780
Broders, F., and Thiery, J. P. (1995). “Structure and function of cadherins BT,” in Organization of the Early Vertebrate Embryo, eds N. Zagris, A. M. Duprat, and A. Durston (Berlin: Springer), 183–208. doi: 10.1007/978-1-4899-1618-1_16
Calcino, A. D., de Oliveira, A. L., Simakov, O., Schwaha, T., Zieger, E., Wollesen, T., et al. (2019). The quagga mussel genome and the evolution of freshwater tolerance. DNA Res. 26, 411–422. doi: 10.1093/dnares/dsz019
Cao, J., Spielmann, M., Qiu, X., Huang, X., Ibrahim, D. M., Hill, A. J., et al. (2019). The single-cell transcriptional landscape of mammalian organogenesis. Nature 566, 496–502. doi: 10.1038/s41586-019-0969-x
Castellani, L., and Cohen, C. (1987). Myosin rod phosphorylation and the catch state of molluscan-muscles. Science (New York, N.Y.) 235, 334–337. doi: 10.1126/science.3026049
Chan, X. Y., and Lambert, J. (2011). Patterning a spiralian embryo: a segregated RNA for a Tis11 ortholog is required in the 3a and 3b cells of the Ilyanassa embryo. Dev. Biol. 349, 102–112. doi: 10.1016/j.ydbio.2010.10.001
Dictus, W. J. A. G., and Damen, P. (1997). Cell-lineage and clonal-contribution map of the trochophore larva of Patella vulgata (Mollusca)1Both authors contributed equally to this work.1. Mech. Dev. 62, 213–226. doi: 10.1016/S0925-4773(97)00666-7
Dobi, K. C., Schulman, V. K., and Baylies, M. K. (2015). Specification of the somatic musculature in Drosophila. Wires Dev. Biol. 4, 357–375. doi: 10.1002/wdev.182
Duruz, J., Kaltenrieder, C., Ladurner, P., Bruggmann, R., Martìnez, P., and Sprecher, S. G. (2020). Acoel single-cell transcriptomics: cell-type analysis of a deep branching bilaterian. BioRxiv [Preprint]. doi: 10.1101/2020.07.10.196782 BioRxiv 2020.07.10.196782,
Dyachuk, V., and Odintsova, N. (2009). Development of the larval muscle system in the mussel Mytilus trossulus (Mollusca, Bivalvia): original article. Dev. Growth Differ. 51, 69–79. doi: 10.1111/j.1440-169X.2008.01081.x
Evseeva, M. N., Dyikanov, D. T., Karagyaur, M. N., Prikazchikova, T. A., Sheptulina, A. F., Balashova, M. S., et al. (2021). Hematopoietically-expressed homeobox protein HHEX regulates adipogenesis in preadipocytes. Biochimie 185, 68–77. doi: 10.1016/j.biochi.2021.02.011
Farrell, J. A., Wang, Y., Riesenfeld, S. J., Shekhar, K., Regev, A., and Schier, A. F. (2018). Single-cell reconstruction of developmental trajectories during zebrafish embryogenesis. Science 360:eaar3131. doi: 10.1126/science.aar3131
Fincher, C. T., Wurtzel, O., de Hoog, T., Kravarik, K. M., and Reddien, P. W. (2018). Cell type transcriptome atlas for the planarian Schmidtea mediterranea. Science 360:eaaq1736. doi: 10.1126/science.aaq1736
Foster, S., Oulhen, N., and Wessel, G. (2020). A single cell RNA sequencing resource for early sea urchin development. Development 147:dev191528. doi: 10.1242/dev.191528
Foster, S., Teo, Y. V., Neretti, N., Oulhen, N., and Wessel, G. M. (2019). Single cell RNA-seq in the sea urchin embryo show marked cell-type specificity in the Delta/Notch pathway. Mol. Reproduct. Dev. 86, 931–934. doi: 10.1002/mrd.23181
García-Castro, H., Kenny, N. J., Iglesias, M., Álvarez-Campos, P., Mason, V., Elek, A., et al. (2021). ACME dissociation: a versatile cell fixation-dissociation method for single-cell transcriptomics. Genome Biol. 22:89. doi: 10.1186/s13059-021-02302-5
Gerdol, M., Moreira, R., Cruz, F., Gómez-Garrido, J., Vlasova, A., Rosani, U., et al. (2020). Massive gene presence-absence variation shapes an open pan-genome in the Mediterranean mussel. Genome Biol. 21:275. doi: 10.1186/s13059-020-02180-3
Gharbiah, M., Nakamoto, A., and Nagy, L. M. (2013). Analysis of ciliary band formation in the mollusc Ilyanassa obsoleta. Dev. Genes Evol. 223, 225–235. doi: 10.1007/s00427-013-0440-1
Grün, D. (2020). Revealing dynamics of gene expression variability in cell state space. Nat. Methods 17, 45–49. doi: 10.1038/s41592-019-0632-3
Grün, D., Muraro, M. J., Boisset, J.-C., Wiebrands, K., Lyubimova, A., Dharmadhikari, G., et al. (2016). De novo prediction of stem cell identity using single-cell transcriptome data. Cell Stem Cell 19, 266–277. doi: 10.1016/j.stem.2016.05.010
Heiler, K. C. M., Bij de Vaate, A., Ekschmitt, K., von Oheimb, P. V., Albrecht, C., and Wilke, T. (2013). Reconstruction of the early invasion history of the quagga mussel (Dreissena rostriformis bugensis) in Western Europe. Aquat. Invasions 8, 53–57.
Hejnol, A., Martindale, M. Q., and Henry, J. Q. (2007). High-resolution fate map of the snail Crepidula fornicata: The origins of ciliary bands, nervous system, and muscular elements. Dev. Biol. 305, 63–76. doi: 10.1016/j.ydbio.2007.01.044
Hemmrich, G., Khalturin, K., Boehm, A.-M., Puchert, M., Anton-Erxleben, F., Wittlieb, J., et al. (2012). Molecular signatures of the three stem cell lineages in hydra and the emergence of stem cell function at the base of multicellularity. Mol. Biol. Evol. 29, 3267–3280. doi: 10.1093/molbev/mss134
Henry, J. Q., Okusu, A., and Martindale, M. Q. (2004). The cell lineage of the polyplacophoran, Chaetopleura apiculata: variation in the spiralian program and implications for molluscan evolution. Deve. Biol. 272, 145–160. doi: 10.1016/j.ydbio.2004.04.027
Hinman, V. F., O’Brien, E. K., Richards, G. S., and Degnan, B. M. (2003). Expression of anterior Hox genes during larval development of the gastropod Haliotis asinina. Evol. Dev. 5, 508–521.
Inkscape Project (2020). Inkscape. Available online at: https://inkscape.org (accessed March 3, 2021).
Jackson, D. J., McDougall, C., Green, K., Simpson, F., Wörheide, G., and Degnan, B. M. (2006). A rapidly evolving secretome builds and patterns a sea shell. BMC Biol. 4:40. doi: 10.1186/1741-7007-4-40
Jones, P., Binns, D., Chang, H.-Y., Fraser, M., Li, W., McAnulla, C., et al. (2014). InterProScan 5: genome-scale protein function classification. Bioinformatics (Oxford, England) 30, 1236–1240. doi: 10.1093/bioinformatics/btu031
Kakoi, S., Kin, K., Miyazaki, K., and Wada, H. (2008). Early development of the japanese spiny oyster (Saccostrea kegaki): characterization of some genetic markers. Zool. Sci. 25, 455–464. doi: 10.2108/zsj.25.455
Karaiskos, N., Wahle, P., Alles, J., Boltengagen, A., Ayoub, S., Kipar, C., et al. (2017). The Drosophila embryo at single-cell transcriptome resolution. Science 358, 194–199. doi: 10.1126/science.aan3235
Karatayev, A. Y., Burlakova, L. E., Pennuto, C., Ciborowski, J., Karatayev, V. A., Juette, P., et al. (2014). Twenty five years of changes in Dreissena spp. populations in Lake Erie. J. Great Lakes Res. 40, 550–559.
Karatayev, A. Y., Padilla, D. K., Minchin, D., Boltovskoy, D., and Burlakova, L. E. (2007). Changes in global economies and trade: the potential spread of exotic freshwater bivalves. Biol. Invasions 9, 161–180. doi: 10.1007/s10530-006-9013-9
Kin, K., Kakoi, S., and Wada, H. (2009). A novel role for dpp in the shaping of bivalve shells revealed in a conserved molluscan developmental program. Dev. Biol. 329, 152–166. doi: 10.1016/j.ydbio.2009.01.021
King, S. M. (2012). Integrated control of axonemal dynein AAA+ motors. J. Struct. Biol. 179, 222–228. doi: 10.1016/j.jsb.2012.02.013
Kirschke, H., Barrett, A. J., and Rawlings, N. D. (1995). Proteinases 1: lysosomal cysteine proteinases. Protein Profile 2, 1581–1643.
Krause, G., Winkler, L., Mueller, S. L., Haseloff, R. F., Piontek, J., and Blasig, I. E. (2008). Structure and function of claudins. Biochim. Biophys. Acta 1778, 631–645. doi: 10.1016/j.bbamem.2007.10.018
Kurita, Y., Deguchi, R., and Wada, H. (2009). Early development and cleavage pattern of the Japanese Purple Mussel, Septifer virgatus. Zool. Sci. 26, 814–820. doi: 10.2108/zsj.26.814
Ladurner, P., and Rieger, R. (2000). Embryonic muscle development of Convoluta pulchra (Turbellaria–Acoelomorpha, Platyhelminthes). Dev. Biol. 222, 359–375.
Lee, P. N., Callaerts, P., De Couet, H. G., and Martindale, M. Q. (2003). Cephalopod Hox genes and the origin of morphological novelties. Nature 424, 1061–1065. doi: 10.1038/nature01872
Levin, M., Anavy, L., Cole, A. G., Winter, E., Mostov, N., Khair, S., et al. (2016). The mid-developmental transition and the evolution of animal body plans. Nature 531, 637–641. doi: 10.1038/nature16994
Li, H., Li, Q., Yu, H., and Du, S. (2019). Developmental dynamics of myogenesis in Pacific oyster Crassostrea gigas. Comp. Biochem. Physiol. Part B 227, 21–30.
Lillie, F. R. (1895). The embryology of the unionidae. A study in cell-lineage. J. Morphol. 10, 1–100. doi: 10.1002/jmor.1050100102
Liu, G., Huan, P., and Liu, B. (2020). Identification of three cell populations from the shell gland of a bivalve mollusc. Dev. Genes Evol. 230, 39–45. doi: 10.1007/s00427-020-00646-9
Liu, X., Jin, C., Li, H., Bai, Z., and Li, J. (2018). Morphological structure of shell and expression patterns of five matrix protein genes during the shell regeneration process in Hyriopsis cumingii. Aquacult. Fish. 3, 225–231. doi: 10.1016/j.aaf.2018.09.005
Liu, X., Zeng, S., Dong, S., Jin, C., and Li, J. (2015). A novel matrix protein hic31 from the prismatic layer of hyriopsis cumingii displays a collagen-like structure. PLoS One 10:e0135123. doi: 10.1371/journal.pone.0135123
Luetjens, C. M., and Dorresteijn, A. W. C. (1995). Multiple, alternative cleavage patterns precede uniform larval morphology during normal development of Dreissena polymorpha (Mollusca, Lamellibranchia). Rouxs Arch. Dev. Biol. 205, 138–149. doi: 10.1007/BF00357760
Lyons, D. C., Perry, K. J., and Henry, J. Q. (2017). Morphogenesis along the animal-vegetal axis: fates of primary quartet micromere daughters in the gastropod Crepidula fornicata. BMC Evol. Biol. 17:217. doi: 10.1186/s12862-017-1057-1
McCartney, M. A., Auch, B., Kono, T., Mallez, S., Zhang, Y., Obille, A., et al. (2019). The genome of the Zebra Mussel, Dreissena polymorpha: a resource for invasive species research. BioRxiv [Preprint]. doi: 10.1101/696732 BioRxiv 696732,
McNickle, G. G., Rennie, M. D., and Sprules, W. G. (2006). Changes in benthic invertebrate communities of South Bay, Lake Huron following invasion by zebra mussels (Dreissena polymorpha), and potential effects on lake whitefish (Coregonus clupeaformis) diet and growth. J. Great Lakes Res. 32, 180–193.
Meisenheimer, J. (1900). Entwicklungsgeschichte von Dreissensia polymorpha Pall. Leipzig: Wilhelm Engelmann.
Meyer, N. P., and Seaver, E. C. (2009). Neurogenesis in an annelid: Characterization of brain neural precursors in the polychaete Capitella sp. Dev. Biol. 335, 237–252. doi: 10.1016/j.ydbio.2009.06.017
Mills, E. L., Dermott, R. M., Roseman, E. F., Dustin, D., Mellina, E., Conn, D. B., et al. (1993). Colonization, ecology, and population structure of the “quagga”mussel (Bivalvia: Dreissenidae) in the lower Great Lakes. Can. J. Fish. Aquat. Sci. 50, 2305–2314.
Nalepa, T. F., and Schloesser, D. W. (2019). Quagga and Zebra Mussels: Biology, Impacts, and Control. Boca Raton, FL: CRC Press.
Nederbragt, A. J., Lespinet, O., Van Wageningen, S., Van Loon, A. E., Adoutte, A., and Dictus, W. J. A. G. (2002). A lophotrochozoan twist gene is expressed in the ectomesoderm of the gastropod mollusk Patella vulgata. Evol. Dev. 4, 334–343. doi: 10.1046/j.1525-142X.2002.02020.x
Nielsen, C. (2005). Trochophora larvae: cell-lineages, ciliary bands and body regions. 2. Other groups and general discussion. J. Exp. Zool. Part B 304, 401–447. doi: 10.1002/jez.b.21050
Nielsen, C. (2018). Origin of the trochophora larva. R. Soc. Open Sci. 5:180042. doi: 10.1098/rsos.180042
Paganos, P., Voronov, D., Musser, J., Arendt, D., and Arnone, M. I. (2021). Single cell RNA sequencing of the Strongylocentrotus purpuratus larva reveals the blueprint of major cell types and nervous system of a non-chordate deuterostome. Elife 10:e70416. doi: 10.7554/eLife.70416
Pavlicek, A., Schwaha, T., and Wanninger, A. (2018). Towards a ground pattern reconstruction of bivalve nervous systems: neurogenesis in the zebra mussel Dreissena polymorpha. Organ. Divers. Evol. 18, 101–114. doi: 10.1007/s13127-017-0356-0
Piontek, J., Winkler, L., Wolburg, H., Müller, S. L., Zuleger, N., Piehl, C., et al. (2008). Formation of tight junction: determinants of homophilic interaction between classic claudins. FASEB J. 22, 146–158. doi: 10.1096/fj.07-8319com
Plass, M., Solana, J., Wolf, F. A., Ayoub, S., Misios, A., Glažar, P., et al. (2018). Cell type atlas and lineage tree of a whole complex animal by single-cell transcriptomics. Science 360:eaaq1723. doi: 10.1126/science.aaq1723
Price, D. A., and Greenberg, M. J. (1977). Purification and characterization of a cardioexcitatory neuropeptide from the central ganglia of a bivalve mollusc. Prep. Biochem. 7, 261–281. doi: 10.1080/00327487708061643
R Developement Core Team (2015). R: A Language and Environment for Statistical Computing, Vol. 1. Vienna: R Foundation for Statistical Computing, 409. doi: 10.1007/978-3-540-74686-7
Raikow, D. F. (2004). Food web interactions between larval bluegill (Lepomis macrochirus) and exotic zebra mussels (Dreissena polymorpha). Can. J. Fish. Aquat. Sci. 61, 497–504.
Redl, E., Scherholz, M., Wollesen, T., Todt, C., and Wanninger, A. (2016). Cell proliferation pattern and twist expression in an aplacophoran mollusk argue against segmented ancestry of mollusca. J. Exp. Zool. Part B 326, 422–436. doi: 10.1002/jez.b.22714
Render, J. (1997). Cell Fate Maps in theIlyanassa obsoleta Embryo beyond the Third Division. Dev. Biol. 189, 301–310. doi: 10.1006/dbio.1997.8654
Salamanca-Díaz, D. A., Calcino, A. D., de Oliveira, A. L., and Wanninger, A. (2021). Non-collinear Hox gene expression in bivalves and the evolution of morphological novelties in mollusks. Sci. Rep. 11:3575. doi: 10.1038/s41598-021-82122-6
Satija, R., Farrell, J. A., Gennert, D., Schier, A. F., and Regev, A. (2015). Spatial reconstruction of single-cell gene expression data. Nat. Biotechnol. 33, 495–502. doi: 10.1038/nbt.3192
Schindelin, J., Arganda-Carreras, I., Frise, E., Kaynig, V., Longair, M., Pietzsch, T., et al. (2012). Fiji: an open-source platform for biological-image analysis. Nat. Methods 9, 676–682. doi: 10.1038/nmeth.2019
Sebé-Pedrós, A., Saudemont, B., Chomsky, E., Plessier, F., Mailhé, M.-P., Renno, J., et al. (2018). Cnidarian cell type diversity and regulation revealed by whole-organism single-cell RNA-seq. Cell 173, 1520–1534.e20. doi: 10.1016/j.cell.2018.05.019
Shapiro, E., Biezuner, T., and Linnarsson, S. (2013). Single-cell sequencing-based technologies will revolutionize whole-organism science. Nat. Rev. Genet. 14, 618–630. doi: 10.1038/nrg3542
Shapiro, L. (2016). “Structure and function of cadherin extracellular regions BT,” in The Cadherin Superfamily: Key Regulators of Animal Development and Physiology, eds S. T. Suzuki and S. Hirano (Berlin: Springer), 71–91. doi: 10.1007/978-4-431-56033-3_4
Siebert, S., Farrell, J. A., Cazet, J. F., Abeykoon, Y., Primack, A. S., Schnitzler, C. E., et al. (2019). Stem cell differentiation trajectories in Hydra resolved at single-cell resolution. Science 365:eaav9314. doi: 10.1126/science.aav9314
Smith, S. A., Wilson, N. G., Goetz, F. E., Feehery, C., Andrade, S. C. S., Rouse, G. W., et al. (2011). Resolving the evolutionary relationships of molluscs with phylogenomic tools. Nature 480, 364–367. doi: 10.1038/nature10526
Stegle, O., Teichmann, S. A., and Marioni, J. C. (2015). Computational and analytical challenges in single-cell transcriptomics. Nat. Rev. Genet. 16, 133–145. doi: 10.1038/nrg3833
Strayer, D. L., Hattala, K. A., and Kahnle, A. W. (2004). Effects of an invasive bivalve (Dreissena polymorpha) on fish in the Hudson River estuary. Can. J. Fish. Aquat. Sci. 61, 924–941.
Sur, A., and Meyer, N. P. (2021). Resolving transcriptional states and predicting lineages in the annelid capitella teleta using single-Cell RNAseq. Front. Ecol. Evol. 8:523.
Svensson, V., Vento-Tormo, R., and Teichmann, S. A. (2018). Exponential scaling of single-cell RNA-seq in the past decade. Nat. Protoc. 13, 599–604.
Tan, S., Huan, P., and Liu, B. (2017). Expression patterns indicate that BMP2/4 and Chordin, not BMP5-8 and Gremlin, mediate dorsal–ventral patterning in the mollusk Crassostrea gigas. Dev. Genes Evol. 227, 75–84. doi: 10.1007/s00427-016-0570-3
Tang, F., Barbacioru, C., Bao, S., Lee, C., Nordman, E., Wang, X., et al. (2010). Tracing the derivation of embryonic stem cells from the inner cell mass by single-cell RNA-Seq analysis. Cell Stem Cell 6, 468–478.
Trapnell, C. (2015). Defining cell types and states with single-cell genomics. Genome Res. 25, 1491–1498.
Trapnell, C., Cacchiarelli, D., Grimsby, J., Pokharel, P., Li, S., Morse, M., et al. (2014). The dynamics and regulators of cell fate decisions are revealed by pseudotemporal ordering of single cells. Nat. Biotechnol. 32:381.
van Dijk, D., Sharma, R., Nainys, J., Yim, K., Kathail, P., Carr, A. J., et al. (2018). Recovering gene interactions from single-cell data using data diffusion. Cell 174, 716–729.e27. doi: 10.1016/j.cell.2018.05.061
Vasta, G. R., Feng, C., Bianchet, M. A., Bachvaroff, T. R., and Tasumi, S. (2015). Structural, functional, and evolutionary aspects of galectins in aquatic mollusks: From a sweet tooth to the Trojan horse. Fish Shellfish Immunol. 46, 94–106. doi: 10.1016/j.fsi.2015.05.012
Vergara, H. M., Bertucci, P. Y., Hantz, P., Tosches, M. A., Achim, K., Vopalensky, P., et al. (2017). Whole-organism cellular gene-expression atlas reveals conserved cell types in the ventral nerve cord of Platynereis dumerilii. Proc. Natl. Acad. Sci. U.S.A. 114, 5878–5885.
Wagner, D. E., Weinreb, C., Collins, Z. M., Briggs, J. A., Megason, S. G., and Klein, A. M. (2018). Single-cell mapping of gene expression landscapes and lineage in the zebrafish embryo. Science 360, 981–987. doi: 10.1126/science.aar4362
Wakida-Kusunoki, A. T., Wakida, F. T., and De Leon-Sandoval, J. M. (2015). First record of quagga mussel Dreissena rostriformis bugensis (Andrusov, 1897) (Bivalvia, Dreissenidae) from Mexico. BioInvasions Rec. 4, 31–36.
Wallace, K. N., Yusuff, S., Sonntag, J. M., Chin, A. J., and Pack, M. (2001). Zebrafish hhex regulates liver development and digestive organ chirality. Genesis 30, 141–143. doi: 10.1002/gene.1050
Wang, S., Zhang, J., Jiao, W., Li, J., Xun, X., Sun, Y., et al. (2017). Scallop genome provides insights into evolution of bilaterian karyotype and development. Nat. Ecol. Evol. 1:0120. doi: 10.1038/s41559-017-0120
Wanninger, A., and Wollesen, T. (2015). “Evolutionary developmental biology of invertebrates 2: lophotrochozoa (Spiralia),” in Evolutionary Developmental Biology of Invertebrates 2: Lophotrochozoa (Spiralia), ed. A. Wanninger (Berlin: Springer-Verlag), 103–154. doi: 10.1007/978-3-7091-1871-9
Wanninger, A., and Wollesen, T. (2019). The evolution of molluscs. Biol. Rev. 94, 102–115. doi: 10.1111/brv.12439
Wendt, G., Zhao, L., Chen, R., Liu, C., O’Donoghue, A. J., Caffrey, C. R., et al. (2020). A single-cell RNA-seq atlas of andlt;emandgt;Schistosoma mansoniandlt;/emandgt; identifies a key regulator of blood feeding. Science 369, 1644–1649. doi: 10.1126/science.abb7709
Wollesen, T., Rodriguez Monje, S. V., de Oliveira, A. L., and Wanninger, A. (2018). Staggered Hox expression is more widespread among molluscs than previously appreciated. Proc. R. Soc. B 285:20181513. doi: 10.1098/rspb.2018.1513
Wollesen, T., Rodríguez Monje, S. V., McDougall, C., Degnan, B. M., and Wanninger, A. (2015a). The ParaHox gene Gsx patterns the apical organ and central nervous system but not the foregut in scaphopod and cephalopod mollusks. EvoDevo 6:41. doi: 10.1186/s13227-015-0037-z
Wollesen, T., Rodríguez Monje, S. V., Todt, C., Degnan, B. M., and Wanninger, A. (2015b). Ancestral role of Pax2/5/8 in molluscan brain and multimodal sensory system development. BMC Evol. Biol. 15:231. doi: 10.1186/s12862-015-0505-z
Zhao, R., Takeuchi, T., Luo, Y.-J., Ishikawa, A., Kobayashi, T., Koyanagi, R., et al. (2018). Dual gene repertoires for larval and adult shells reveal molecules essential for molluscan shell formation. Mol. Biol. Evol. 35, 2751–2761. doi: 10.1093/molbev/msy172
Keywords: Bivalvia, development, Dreissena, evo-devo, trochophore, evolution, cell type
Citation: Salamanca-Díaz DA, Schulreich SM, Cole AG and Wanninger A (2022) Single-Cell RNA Sequencing Atlas From a Bivalve Larva Enhances Classical Cell Lineage Studies. Front. Ecol. Evol. 9:783984. doi: 10.3389/fevo.2021.783984
Received: 27 September 2021; Accepted: 16 December 2021;
Published: 26 January 2022.
Edited by:
Maria Ina Arnone, Stazione Zoologica Anton Dohrn, ItalyReviewed by:
Jose Maria Martin-Duran, Queen Mary University of London, United KingdomNathan Kenny, University of Otago, New Zealand
Copyright © 2022 Salamanca-Díaz, Schulreich, Cole and Wanninger. This is an open-access article distributed under the terms of the Creative Commons Attribution License (CC BY). The use, distribution or reproduction in other forums is permitted, provided the original author(s) and the copyright owner(s) are credited and that the original publication in this journal is cited, in accordance with accepted academic practice. No use, distribution or reproduction is permitted which does not comply with these terms.
*Correspondence: Andreas Wanninger, andreas.wanninger@univie.ac.at
†ORCID: David A. Salamanca-Díaz, orcid.org/0000-0003-2082-3939; Stephan M. Schulreich, orcid.org/0000-0002-5309-0228; Alison G. Cole, orcid.org/0000-0002-7515-7489; Andreas Wanninger, orcid.org/0000-0002-3266-5838