- 1Department of Zoology, School of Natural and Mathematical Sciences, University of Venda, Thohoyandou, South Africa
- 2Afromontane Unit, Department of Zoology and Entomology, University of the Free State, Phuthaditjhaba, South Africa
Bats are known to be sensitive to changes in their environment. The impact of natural vegetation cover, artificial light intensity and noise (dBA) were investigated on the bat community on the opencast Venetia diamond mine using mixed-effects models. Clutter-feeding bats were virtually absent compared to surrounding natural habitats, suggesting the negative impact of vegetation removal and/or light and/or noise pollution. Mixed-effect models revealed that natural vegetation was the most important factor impacting species richness and overall bat activity. In general, bat activity of both open-air and clutter-edge foragers was negatively impacted over areas close to mining operations that were devoid of vegetation cover. Artificial light only significantly affected feeding activity with less feeding activity in the lit areas. Anthropogenic noise had no significant impact on bat activity and species richness. Our study highlights the importance of vegetation cover and the complexity of the interaction between bats and the environment incorporating anthropogenic factors (artificial lighting, continuous noise, and habitat degradation) and natural factors such as minimum temperature, moon phase, and season that confound trends in bat species richness and responses in relation to opencast mining.
Introduction
Anthropogenic developments expose bats to habitat alterations and a range of pollutants, to which bats are known to be sensitive (Jones et al., 2009; Naidoo et al., 2016; Frick et al., 2019). Opencast mining allows the study of the effects of habitat degradation due to the physical removal of natural habitat and continual noise and nighttime light pollution. It is generally accepted that bats exhibit trait-based responses to these habitat-specific environmental changes based on morphology, such as differences in wing shape and associated echolocation call (Aldridge and Rautenbach, 1987; Schoeman, 2015; Jung and Threlfall, 2018), as well as sensitivities of audiological and visual systems (Schaub et al., 2008; Eklöf et al., 2014; Luo et al., 2015; Haddock et al., 2019; Finch et al., 2020), and by shifts in behaviour and community structure due to differential roosting and feeding preferences of different foraging groups: open air, clutter edge, and clutter (Norberg and Rayner, 1987; Bader et al., 2015; Monadjem et al., 2020). Combined, these aspects governing responses of bats to alterations in their environment could result in physiological changes. For example, bats that benefit from foraging around artificial light sources have shown higher blood metabolite levels (ß-hydroxybutyrate) early in the evening compared to species that do not forage around lights (Cravens and Boyles, 2019).
Many studies have focused on the impacts of noise and light individually on bat behaviour, predominantly in a laboratory setting, focusing on a single or select few species. Some exceptions have investigated these impacts in the field in habitats that are naturally dark and lit for experimental purposes (Stone et al., 2009; Minnaar et al., 2014; Bailey et al., 2019) or areas that experience temporary lighting depending on human activities, e.g., at stadiums (Schoeman, 2015). The response of bats to light and noise in their environment is species-specific (Schaub et al., 2008; Stone et al., 2009).
Artificial night time lighting delays and reduces the number of bats emerging from their roosts (Boldogh et al., 2007), influences species-specific foraging behaviour (Minnaar et al., 2014; Stone et al., 2015; Lewanzik, 2017; Bailey et al., 2019; Salinas-Ramos et al., 2021), affects commuting behaviour (Stone et al., 2009, 2015; Gaston et al., 2013; Lewanzik, 2017), interferes with navigation (Lewanzik, 2017; Lewanzik and Voigt, 2017), and could affect the stability of bat communities through competitive exclusion (Arlettaz et al., 2000; Stone et al., 2015). If adults have to forage further afield from maternity roosts, the resulting higher energetic cost and decreased foraging time could negatively impact the growth rates of young bats (Stone et al., 2015; Lewanzik, 2017). Artificial lighting can thus create “barriers” that may limit the effective dispersal of species, isolating habitat patches and populations from immigration and reduce the connectivity of habitats in the landscape, e.g., Rhinolophus hipposideros (Stone et al., 2009; Gaston and Bennie, 2014). Many bat species appear to be intolerant of light and avoid lit areas, particularly slow-flying highly manoeuvrable species that feed within cluttered spaces (clutter foragers) of the genera Rhinolophus and Hipposideros (Lewanzik and Voigt, 2017). In general, they are accepted to be intolerant of light and avoid lit areas due to the sensitivity of their eyes to light (and ultraviolet) that may lead to potential vision impairment in lit areas (Jones et al., 2009; Lewanzik, 2017).
Bats are known auditory specialists (Lattenkamp et al., 2020), with excellent hearing over several octaves, with the greatest sensitivity in species-specific high-frequency ultrasonic echolocation call spectral ranges, although there is evidence for aural sensitivity to lower frequency sounds to eavesdrop on prey-generated sounds or to hear low-frequency isolation calls of pups, e.g., Rhinolophus ferrumequinum (Bohn et al., 2006). Foraging success can be impeded by auditory conflicts resulting from anthropogenic or natural noise that has spectral ranges similar to that of any given species of echolocating bat or species that rely on the sounds produced by prey (mating calls and movement) (Simmons et al., 1978; Schaub et al., 2008; Gomes et al., 2016). Sounds that overlap with bats auditory cues, and sounds with properties (including loudness and intensity) that fall within the sensitive auditory range of bats could be intolerable to bats and are avoided. These sounds can cause stress, reduce attention, disrupt biological processes (e.g., communication) and mask auditory perceptions (acoustic masking) (Bunkley and Barber, 2015; Luo et al., 2015; Gomes et al., 2016; Geipel et al., 2019).
The response of bats to noise seems to be not only species and individual specific but is also dependent on the behavioural context (Schaub et al., 2008; Luo et al., 2014, 2015). For example, under laboratory conditions, M. myotis avoided the playback sound stimulus and actively avoided foraging areas that were heavily impacted by noise (Schaub et al., 2008). Conversely, during rest (torpid period), M. myotis quickly habituated to anthropogenic noise exposure (Luo et al., 2014). Species that rely on passive listening to locate prey are expected to avoid foraging habitats degraded by anthropogenic noise such as M. blythii, M. evotis, M. septentrionalis, Euderma maculatum, and species from the genera Plecotus and Corynorhinus (Schaub et al., 2008; Bunkley and Barber, 2015). Few field studies show how traffic noise (Luo et al., 2014; Finch et al., 2020) and noise associated with natural gas extraction (Bunkley et al., 2015) impacts bat ecology. Ambient noise (less than and greater than 20 kHz) can provide a greater challenge for insectivorous bats which have been shown to actively avoid noise stimulus even if the noise characteristics does not overlap with echolocation calls, resulting in reduced foraging activity (Luo et al., 2015; Finch et al., 2020).
Studies on the effects of large-scale opencast mining activities on bat communities in tropical regions are scant and few exist for temperate regions (see Armstrong, 2010; Duarte et al., 2015; Theobald et al., 2020). Factors shown to influence total bat activity and species richness included distance from the boundary of the mine, woodland cover, climatic variables (Theobald et al., 2020) and machinery noise negatively impacting soundscape complexity (Duarte et al., 2015).
We investigated the impact of artificial lighting, noise and natural vegetation cover on bat activity and behaviour in relation to opencast diamond mining at Venetia Mine in the Limpopo River Valley. Compared with most studies which investigate light and noise separately, we investigated the effects of light and noise together in the field, taking vegetation structure, moon phase, minimum temperature (°C) and season into account. Based on the literature above, we predicted that clutter and clutter-edge forager bats might avoid well-lit, noisy areas with low vegetation cover. In contrast, we expect open-air foragers that fly above the canopy may be unaffected by noise and low vegetation cover but that they might increase feeding activity over well-lit areas due to an increase in insects attracted to lights.
Materials and Methods
Study Site
The study was conducted on the footprint of the opencast Venetia diamond mine (−22.427708°, 29.324158°) over 21 nights during March 2019 (early autumn) and September 2019 (early spring). The Venetia diamond mine is situated in the northern Limpopo River Valley, approximately 60 km north of the Soutpansberg mountain range (Figure 1). Mining-related activities began in 1984 and the mine was fully operational since 1992.1 Mining operations are active 24 h a day. The mine is located in the Limpopo Ridge Bushveld (Mucina and Rutherford, 2011) and is restricted to a kimberlite pipe containing the diamonds (Brown et al., 2009). The Limpopo Ridge Bushveld is dominated by Mopane (Colophospermum mopane), Red Bushwillow (Combretum apiculatum), and Purple-pod Cluster-leaf (Terminalia prunoides) with a handful of other iconic tree species such as Knobthorn (Senegalia nigrescens), Marula (Sclerocarya birrea), and Baobab (Adansonia digitata) (Mucina and Rutherford, 2011). The area is considered subtropical and semi-arid. The climate is characterised by hot dry winters and hot summers with mean annual precipitation between 300 and 400 mm falling predominantly during the summer (Mucina and Rutherford, 2011).
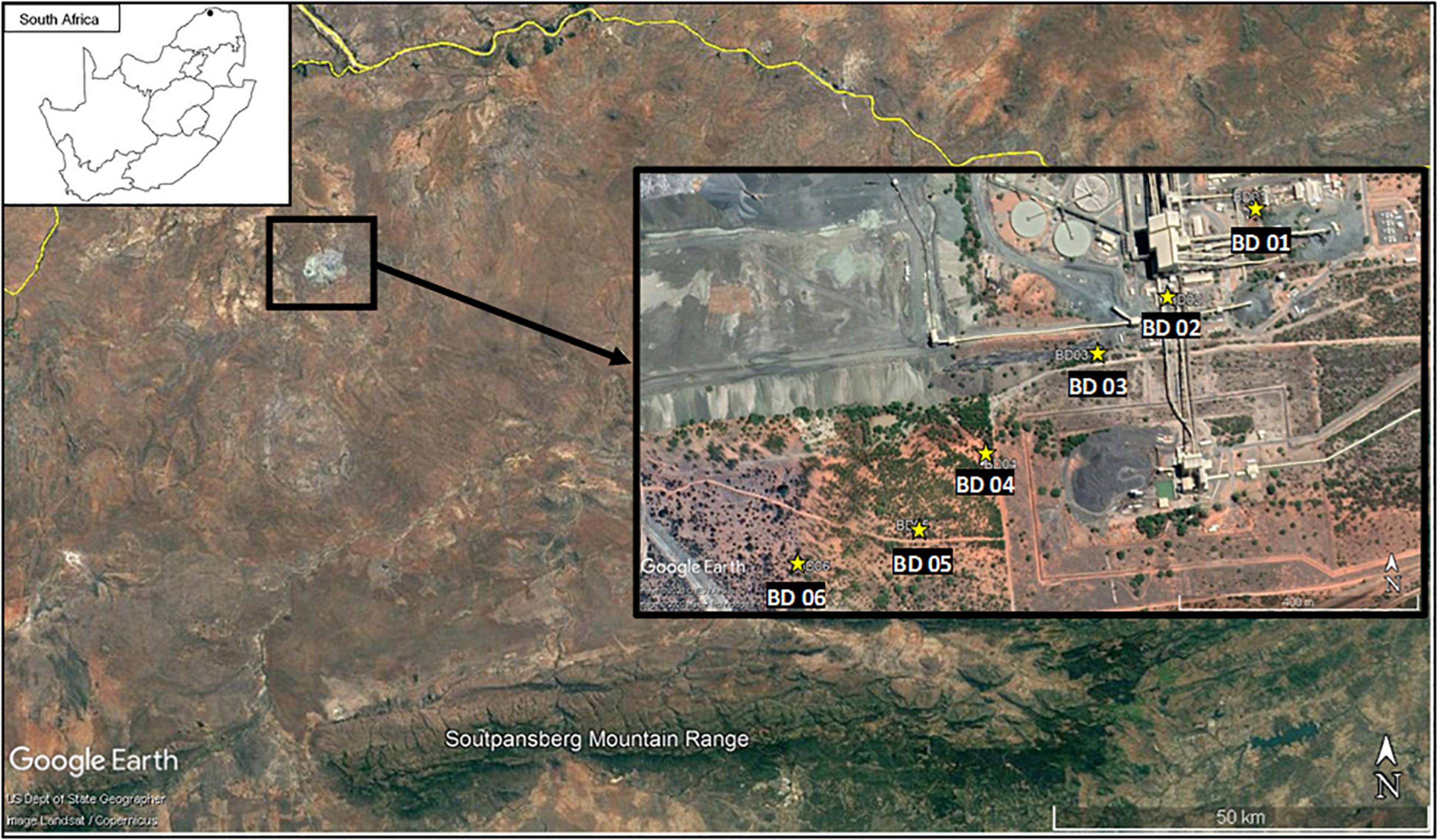
Figure 1. The location of the study site in the northern Limpopo River Valley (dot on map insert) and placement of the bat detectors () on the Venetia diamond mine. BD01 was situated at a workshop, BD02 was at the processing plant itself, BD03 was on the edge of a laydown (open-air storage) area, BD04 was placed along the edge of a road opposite the sorting area, BD05 and BD06 were place furthest from the noise and light of the active mining areas in natural vegetation.
Light Transect Location and Luminosity Measurements
The in situ lighting on the mine was used to identify the impact of light along a gradient. Six SM4BAT FS recorders (Wildlife Acoustics, Inc., Maynard, United States) with SMM-U1 ultrasonic microphones mounted approximately 6 m above the ground and fitted with two 64 GB SDXC cards were placed approximately 100 m apart along a light gradient. The transect began from the floodlights near several workshops and the processing plant and extended in a straight line from the mine into darker areas (Figure 1). The specific positioning of the transect ensured that the effect of water was eliminated to prevent an over-representation of activity at a given site. Bats are known to be attracted to artificial water points in semi-arid regions in the absence of larger, natural water sources (Taylor et al., 2020). Bat detector 01 was placed in an area that was exposed directly to a harsh white light from the workshop buildings as well as an orange floodlight from a nearby conveyer belt system (∼36 m away). Bat detector 02 was positioned in an area that was exposed to direct light from an orange floodlight at the processing area. Bat detector 03 was placed on the edge of the processing plant in an open-air storage area. Bat detector 04 was placed at the edge of the mining footprint to the southwest of the processing plant. Bat detectors 05 and 06 were placed furthest away from the processing plant extending into natural vegetation.
Light intensity (maximum luminosity) at each site was recorded and presented as the measurement lux using a handheld digital lux meter (ME-GM1020 Digital Lux Meter) held ∼2 m above the ground with the light-sensitive sphere pointing toward the light source. Maximum luminosity was recorded as this would in effect be what the bats are exposed to when flying through lit patches. At bat detectors 04, 05, and 06, spill-over light was measured in lit areas within the vegetation as the vegetation had effectively created dark spots where luminosity was recorded as 0.0 lux. Due to logistic constraints, light measurements could not be taken each night. Light intensity readings (maximum lux) were recorded on the initial nights of the transect installations during March and September 2019 after the sun had set and the horizon no longer had the glow of the setting sun.
Noise Frequencies and Sound Pressure Levels
The primary source of noise that bats would be exposed to was the constant noise from the processing plant (crusher and conveyor systems). There would also be intermittent noise from trucks and earth-moving plant (engine noises, reverse alarms) and loading and offloading of material. From the recorded bat call files, the continuous noise levels that the bats would have been exposed to at each bat detector per night were extracted from all the .WAV files. The Noise Analysis tool in Kaleidoscope Pro2 was used to determine the maximum sound pressure level (SPL) of the mine at each bat detector from the.WAV files. The analysis was followed in accordance with the guidelines suggested by Wildlife Acoustics.3 We selected the standard A-weighted frequency band (covering the audible frequency range from 20 Hz to 20 kHz) to be analysed, as this would provide a frequency response curve typical to how a human ear would perceive the ambient noise of the mine and is considered ideal for bat hearing ranges (Bunkley and Barber, 2015). We required the scale of the SPL output results to be in relation to the international reference pressure (auditory threshold) of 0 dB (SPL) = 20 μPa (sound pressure), where 1 Pa is equal to 94 dB relative to 20 μPa, thus 94 dB was entered in the dB adjustment field (Bruneau, 2006). Taking into account the microphone sensitivity gain of +12 dB entered into the settings of the SM4BAT, the software applied a correction factor of 81 dB. From here on, SPL will be referred to as noise (dBA). The noise frequency along the transect that the bats were exposed to was measured in BatSound from sound files with a timestamp as close to 19:00 as possible on each night. The frequency of the background noise was determined over a 2,000 ms period and the mean and standard deviation was calculated.
To determine the acoustic intensity ratio (z) between the “quietest” and “loudest” points along the transect, the equation ΔL = 10 log10(z) was used. ΔL is the difference between two relative intensities and z is the ratio of one sound to another, thus z = 10Δ L/10. To calculate the perceived change in loudness or level change (x), the equation Δ L = 10 log2(x) was used, thus x = 2Δ L/10 (equations by Sengpiel Audio, 2014).
Percentage of Natural Vegetation Estimation
Natural vegetation cover was visually estimated and recorded as a percentage; bat detectors 01 and 02: 0% (completely devoid of vegetation; no trees and no grass), bat detector 03: 25% (in a cleared lay-down area; on the edge of a stand of trees but no grass), bat detector 04: 50% (on the edge of a wooded area bordered by a road and open grass area) and bat detectors 05 and 06: 100% (unaltered natural vegetation). For the mixed-effects model analysis and resulting graphical outputs, each percentage was assigned a letter to ensure it was treated as categorical: A = 0%, B = 25%, C = 50%, D = 100%.
Call Analysis
Kaleidoscope Pro (version 4.5.5, Wildlife Acoustics, Inc.) was used to convert sound files (.WAV) into zero-crossing files (ZC). AnalookW (version 4.5z, Chris Corben) and BatSound (version 3.31, Pettersson Elektronik AB) were used to identify all bat calls. A minimum of four pulses per 15 s was initially filtered from the data set. The filtered sound files were then bulk sorted to species level using filters designed in AnalookW based on call parameters from Taylor et al. (2013) and Monadjem et al. (2020) and were refined using the bat calls recorded on site (Supplementary Table 1). Due to the overlap in call parameters (particularly peak frequencies, durations and bandwidths), all calls were manually checked and adjusted as necessary if the filters had incorrectly identified the calls.
Several bat species could not be confidently differentiated from each other due to the degree of overlap in call parameters. Chaerephon pumilus and Mops condylurus are known to occur on the mine, even sharing the same roosts (pers. Obs.) but could not be reliably distinguished from each other acoustically and thus were placed in the same call group but considered as one species for the analyses. Chaerephon cf. ansorgei and Molossid 19 kHz (possibly Tadarida ventralis) exhibit overlapping call parameters and were grouped as one species. The same procedure was followed for Pipistrellus rusticus and Neoromicia anchietae, and Laephotis capensis and P. rueppellii with each species group counted as a single species for the analysis.
Three categories of bat behaviour were recognized: non-feeding (commuting/searching), feeding attempts (feeding buzzes) and socialising. Feeding buzzes were manually identified from the ZC files and were validated using the associated. WAV file in BatSound since these types of behavioural calls are challenging to confidently classify based on the ZC file. The identification of each echolocation call to bat behavioural category was important to determine if the artificial lighting on the mine provided feeding opportunities. All calls were organised into foraging guilds according to Monadjem et al. (2020); open-air foragers (OAF) that fly and forage above the vegetation (Molossidae and Emballonuridae), clutter-edge foragers (CEF) that forage near/along the edge of vegetation (Vespertilionidae and Miniopteridae) and clutter foragers (CF) that forage within cluttered spaces, often close to the ground (Rhinolophidae and Hipposideridae). All bat passes were standardised to Activity Index (AI) based on Miller (2001). Activity Index was thus represented as one call per specific species over a 1-min interval. The same was done for behaviourally-categorised subsets of calls with special attention paid in instances where conspecifics were performing two types of behaviours during the same 1-min interval, thus no identified calls were lost.
Detectability of the bats across the site does need to be considered. However, the proposed correction factor by Monadjem et al. (2017) was not applied due to several concerns around sample size, bat detector brand and methodology. Until more research has been conducted in this field with new technologies, we are hesitant to apply a correction factor to the current data as it will undoubtedly distort the data (see Taylor et al., 2020 where this detection factor over-compensated for clutter-feeding bats).
Statistical Analysis
R version 3.6.3 and packages “car,” “pscl,” “lme4,” “MuMIn,” “multcomp,” and “mgcv” were used to perform the statistical analyses. Significant differences in artificial light and noise (dBA) between six bat detectors were explored using one-way ANOVAs since these two main variables of particular interest were expected to be significantly different between the bat detectors. Linear mixed-effects models (lmer) and a generalised mixed-effects model (glmer) were used to determine which factors and associated models were most likely responsible for the observed differences in AI (total AI, AI per foraging guild and AI associated with specific behaviours) and species richness along the transect. Date and bat detector was set as the random factor to account for pseudo replication. The fixed factors were light intensity (lux), noise (dBA), minimum temperature (Tmin,°C), percentage of natural vegetation cover, moon phase and season. All AI data were log-transformed to normalise the data suitable for lmer with the exception of feeding. In this instance, a glmer (family Poisson) was used to determine the best fit model. Best-fit models were selected based on the calculated corrected Akaike Information Criterion values (AICc) and associated delta AICc (ΔAICc) values < 2. Collinearity between the fixed factors was tested using the variation of inflation factor (VIF) function in R. As suggested by Fox and Monette (1992), we used generalised VIF (GVIF1/2*df) instead of GVIF. If the GVIF1/2*df < 5, the association between the factors was deemed weak and were included in the mixed-effects models.
Type II Wald Chi-square tests (Anova: lme4) were used on each mixed-effects model (lmer and glmer) to determine any significant differences in the means of the independent variables; moon phase, Tmin (°C), maximum luminosity (lux), noise (dBA) and percentage of natural vegetation cover in relation to the dependent variables; AI and species richness across the six bat detectors. The Wald Chi-square test was chosen as it is not bound by a specific distribution and is thus a suitable non-parametric test that can be used for non-normal variable distributions in mixed-effects models.
Percentage AI of OAF and CEF species was used to determine the dominant species along the transect. One-way ANOVAs were used to explore the differences in AI of the dominant species along the transect in relation to bat detector and percentage natural vegetation cover.
Results
A total of 35,327 files recorded over the 21 nights were identified (species level and activity type) using a combination of AnalookW and BatSound to analyse zero-crossing and wave files, respectively, resulting in a total of 42,028 bat passes. Since zero-crossing files lose intensity and harmonic information, BatSound was used to verify feeding buzzes and identifications where zero-crossing files viewed in AnalookW were unclear or ambiguous. The total activity index was calculated to be 31,563 (Table 1).
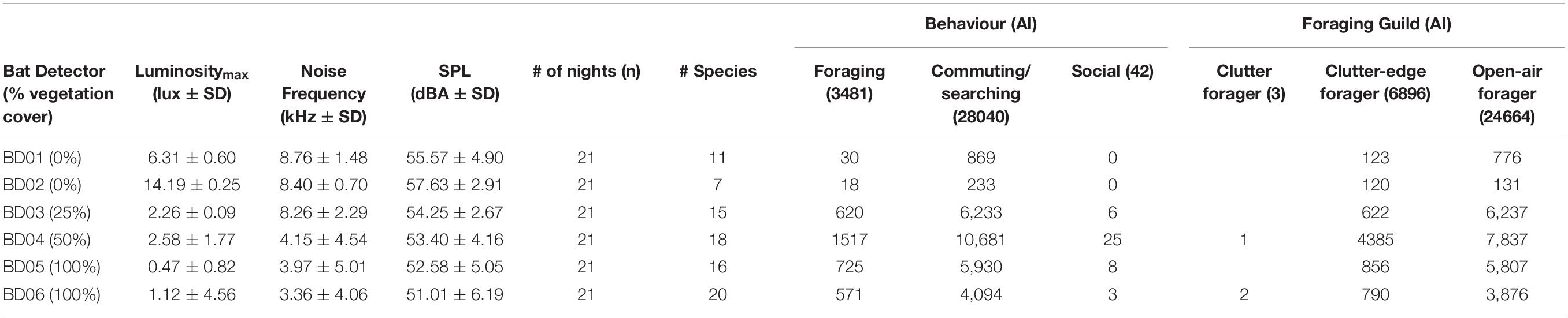
Table 1. Average and standard deviation of maximum luminosity (lux), noise frequency (kHz), and sound pressure level (SPL, dBA) recorded at each bat detector with the associated percentage of natural vegetation cover indicated in brackets. Distribution of activity index (AI) of all bats across the transect indicating behaviours and foraging guilds with the AI per behaviour and foraging guild presented in brackets.
Species Richness and Activity Index
Overall, 19 (potentially 23) bat species/species-groups were acoustically identified (Figure 2). Bat species richness varied along the transect (Table 1). The highest species richness was recorded where percentage natural vegetation cover was 50% and at the end of the transect (18 species), and the lowest species richness was recorded at the beginning of the transect (seven species). For all analyses, 19 species or species-groups were used as listed in Figure 2.
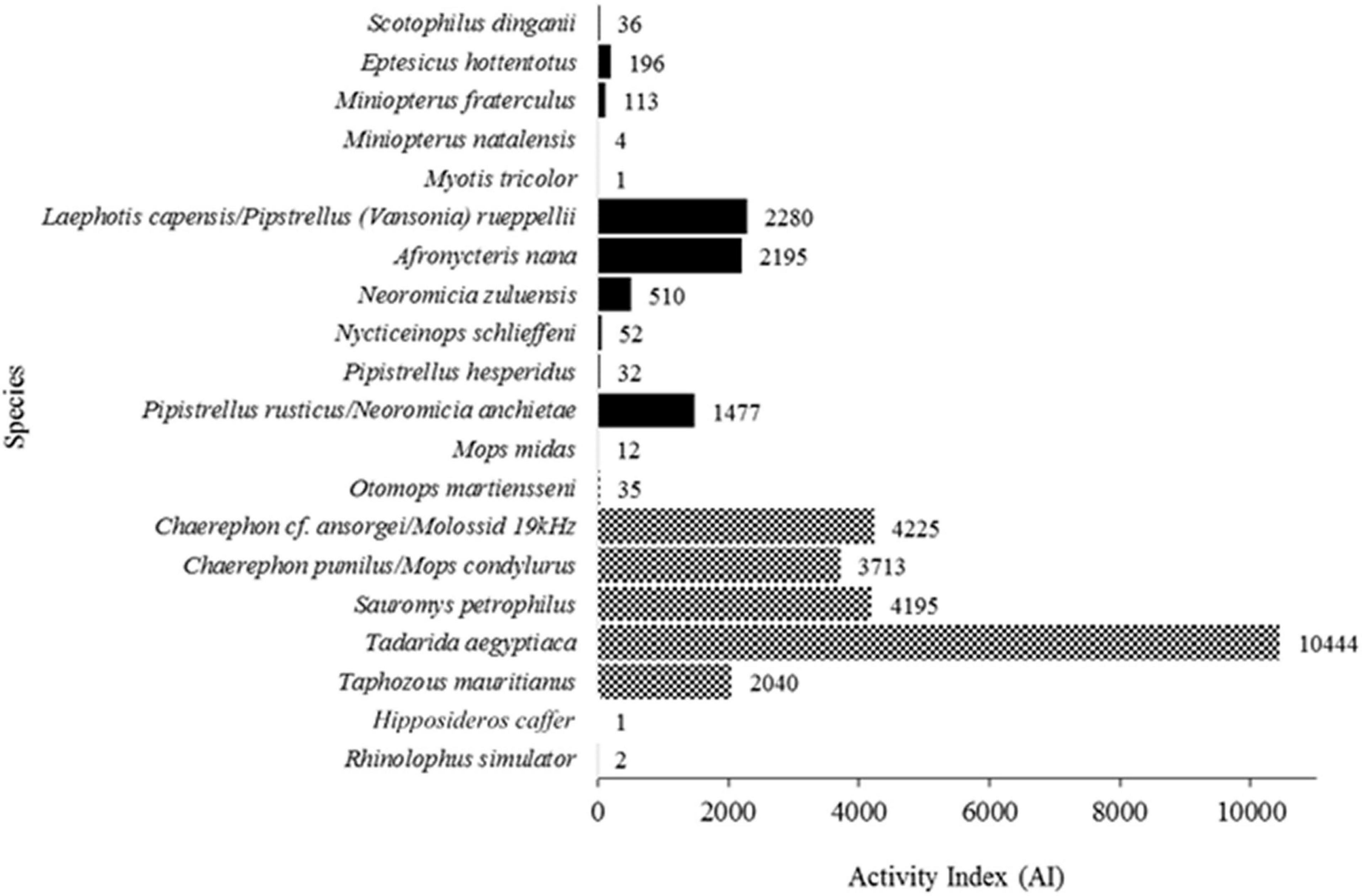
Figure 2. Species identified with the associated sum of behavioural AI categories. Solid filled bars indicate clutter-edge foragers and the checkered bars indicate open-air foragers. Rhinolophus simulator and Hipposideros caffer are clutter foragers but were represented in such low numbers that a fill is not visible.
Total AI, AI per foraging guild and behaviour (feeding attempts, non-feeding, and social) were all significantly different along the transect (all P < 0.05; Supplementary Figure 1). Open-air foragers (OAF) accounted for the highest overall AI (24,664), as well as AI associated with social (29), feeding (2,594) and non-feeding (22,041) activity (Table 1 and Supplementary Figure 1). Total OAF AI was dominated by T. aegyptiaca (42.35%), cf. C. ansorgei/Molossid 19 kHz (17.13%), Sauromys petrophilus (17.01%), and C. pumilus/M. condylurus (15.05%). Clutter-edge foragers (CEF) accounted for the second-highest overall AI (6,896), social (13), feeding (887), and non-feeding (5,996) activity (Table 1 and Supplementary Figure 1). Laephotis capensis/P. rueppellii, A. nana, and P. rusticus/N. anchietae dominated with 33.08, 31.83, and 21.42% of the total CEF AI, respectively. Finally, clutter foragers (CF) were poorly represented with only three recorded bat passes (Table 1) of two individuals of R. simulator and a single H. caffer.
Artificial Light and Anthropogenic Noise
Light intensity and noise (dBA) were significantly different along the transect (P < 0.05), decreasing both with distance away from the mine and the percentage of natural vegetation (highest at BD01 and BD02 with zero cover and lowest at BD05 and BD06 with 100% cover (Table 1). All noise (dBA) fell into the range that would be considered to be moderate to seriously annoying, particularly to humans (Berglund et al., 2000). Noise frequencies that were recorded at the beginning of the transect at and near the processing plant could have only overlapped with O. martiensseni, which is known to produce a narrow bandwidth (6.4 ± 2.3 kHz) and long duration (24 ± 14.8 ms) echolocation calls with a peak frequency of 10.8 ± 2 kHz (Monadjem et al., 2020). With only 35 calls recorded of O. martiensseni, noise frequency was not used in any of the analyses since the chance of acoustic masking of the remaining species of bats would be negligible to absent.
Tests of Collinearity, Analysis of Variance, and Mixed-Effects Models
The tests of collinearity on each linear and generalised mixed-effect regression model (lmer and glmer) showed that all factors had fairly weak associations when considering GIVF1/2*df since all values were <5 (Supplementary Table 2). The results of the analysis of variance (Anova: lme4) are presented in Supplementary Table 2 indicating the effect of light intensity (lux), noise (dBA), Tmin (°C), percentage of natural vegetation cover (% Nat. veg), moon phase and season on the dependent variables.
The best fit model selection, model estimates and associated cftest results are presented in Supplementary Table 3. The percentage of natural vegetation cover was significantly important for all 12 best-fit models, with significantly higher AI, species richness, forager guild activity and specific activities of different behaviour over 25, 50, and 100% natural vegetation cover than areas devoid of natural vegetation (Supplementary Table 3). Differences in AI were best explained by three best-fit models which included, in addition to percentage of vegetation cover and Tmin, moon phase, season and light intensity (lux) (Figure 3A). Species richness was best explained by two best-fit models which showed a significant increase with percentage vegetation cover ≥25% and increasing Tmin (°C), and was affected by moon phase and season, although not significantly (Supplementary Table 3 and Figure 3B). Open-air forager activity was best explained by two best-fit models indicating significant effects of Tmin and percentage vegetation cover as well as non-significant effects of moon phase, season, and noise (dBA) (Supplementary Table 3 and Figure 4A). Clutter-edge forager activity was shown only to be significantly affected by percentage of natural vegetation cover in both best-fit models although season (not significant) was included in the first model from the best-fit selection table (Supplementary Table 3 and Figure 4B). Light intensity, percentage natural vegetation cover, moon phase and season were all significant factors that described feeding activity by a single best-fit model (Supplementary Table 3 and Figure 5A) with higher feeding attempts during early autumn over the dimly lit areas that were exposed to spill-over from the lights of the mine, had natural vegetation cover ≥ 25% and during periods of darker moon phases (Table 1 and Supplementary Table 3). Lastly, three best-fit models indicated that Tmin and percentage natural vegetation cover were significant factors for bats non-feeding behaviour with the inclusion of light intensity in the third model (Supplementary Table 3 and Figure 5B). Moon phase and season were included in the models but did not have a significant effect on non-feeding behaviour (Supplementary Table 3).
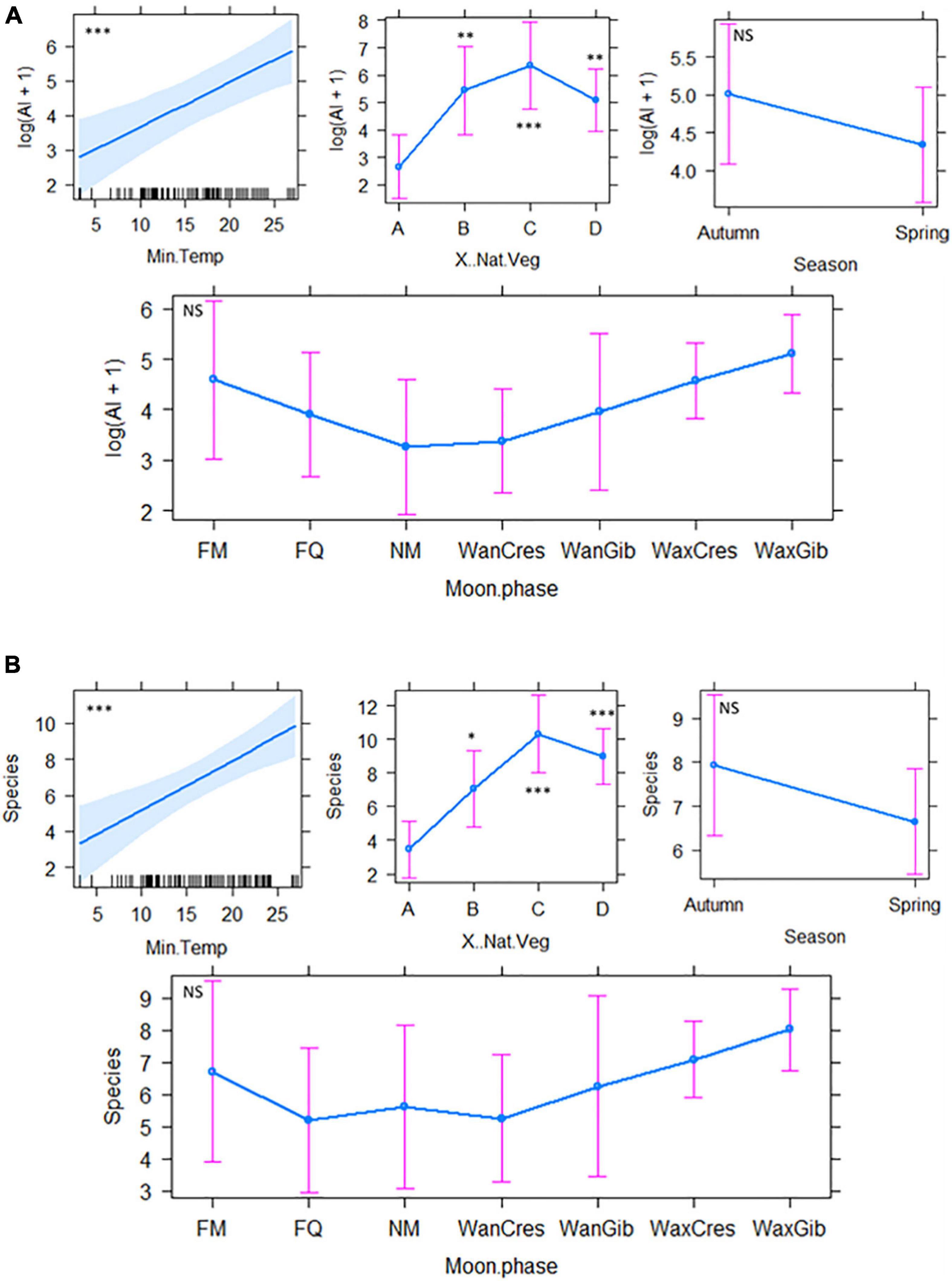
Figure 3. The best fit model outputs for (A) AI (model #2) and (B) species (model #1) showing the influence of season, maximum luminosity, noise (dB), percentage of natural vegetation cover and moon phase on bat richness and activity. Bat detector and date were set as the random factors. Codes in graphs: A = 0%, B = 25%, C = 50%, D = 100%. FM, full moon; FQ, first quarter; NM, new moon; WanCres, waning crescent; WanGib, waning gibbous; WaxCres, waxing crescent; WaxGib, waxing gibbous. NS, Not Significant. *P < 0.05, **P < 0.01, ***P < 0.001.
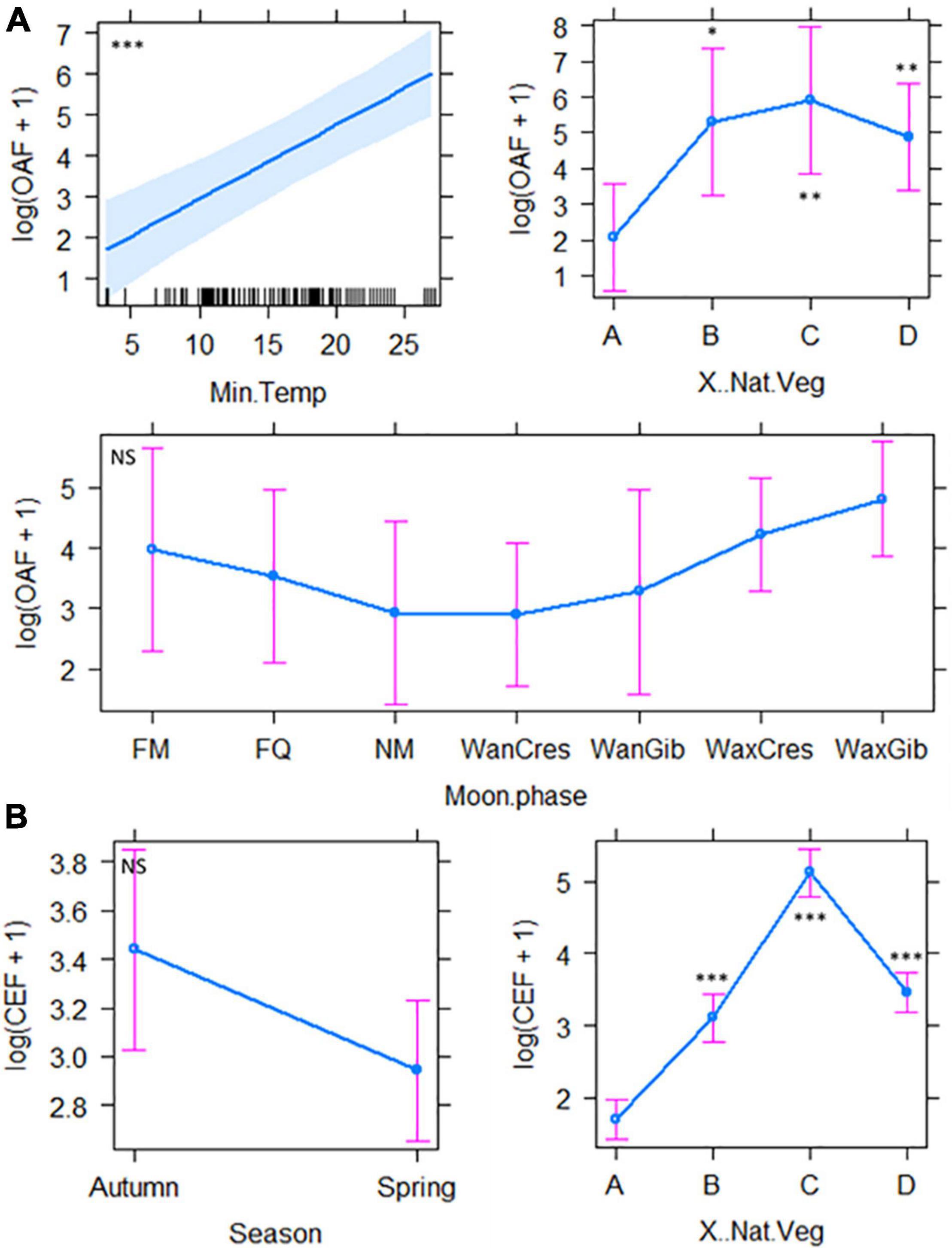
Figure 4. The best fit model outputs indicating the factors responsible for the observed pattern of activity of open-air foragers (A) and clutter-edge foragers (B). Bat detector and date were set as the random factors. A = 0%, B = 25%, C = 50%, and D = 100%. FM, full moon; FQ, first quarter; NM, new moon; WanCres, waning crescent; WanGib, waning gibbous; WaxCres, waxing crescent; WaxGib, waxing gibbous. NS, Not Significant. *P < 0.05, **P < 0.01, ***P < 0.001.
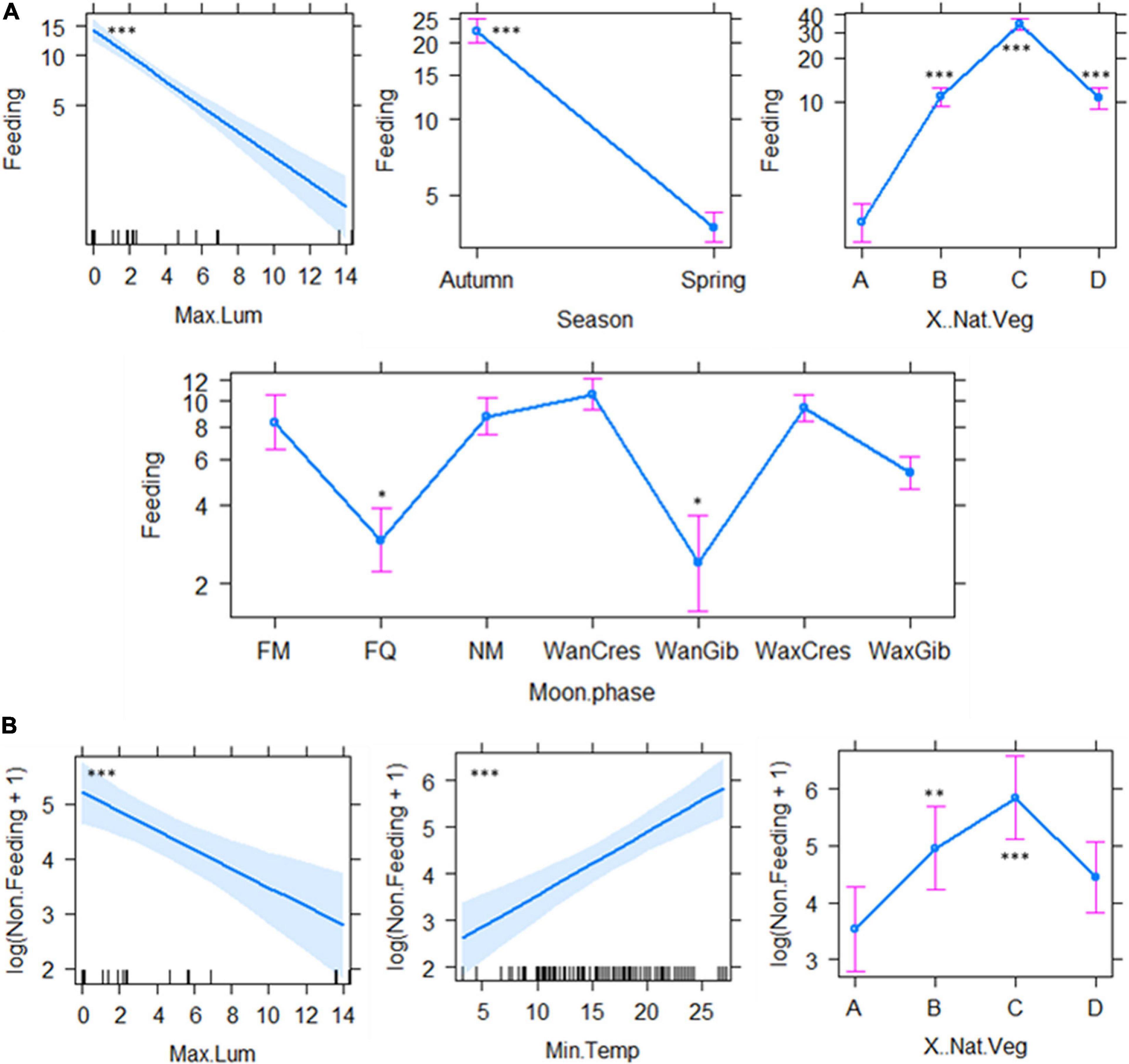
Figure 5. The factors to best explain the observed patterns of feeding (A) and non-feeding (B) activity. Bat detector and date were set as the random factors. A = 0%, B = 25%, C = 50%, D = 100%. FM, full moon; FQ, first quarter; NM, new moon; WanCres, waning crescent; WanGib, waning gibbous; WaxCres, waxing crescent; WaxGib, waxing gibbous. NS, Not Significant. *P < 0.05, **P < 0.01, ***P < 0.001.
Discussion
Species richness recorded along the transect on the Venetia diamond mine is comparable with the species richness recorded by Taylor et al. (2020) from the surrounding area. However, compared to the study of Taylor et al. (2020) conducted only about 14–30 km from Venetia Mine, clutter-feeding bats were conspicuously scarce (AI = 3) in our study. While the activity of clutter feeders was significantly related to the proximity of water bodies and riparian vegetation by Taylor et al. (2020), it is unlikely that the proximity of water accounted for the absence of these bats in our study since a dam used by bats was located close to the sample sites on the mine property. Furthermore, a much higher activity of clutter feeding bats (AI = 150) was recorded during the duration of this study on an undisturbed game farm close to the mine in the same vegetation type (Limpopo Ridge Bushveld) by Cory Toussaint (2021). Thus, we conclude that, as we predicted, these sensitive, clutter-dependent bats have been excluded from our study transect by vegetation removal, light pollution and noise pollution or a combination of these factors. It is known that Rhinolophidae and some Vespertilionidae are sensitive to light (Schoeman, 2015; Stone et al., 2015; Rowse et al., 2016; Azam et al., 2018). Stone et al. (2009) showed that artificial lighting negatively affected R. hipposideros (lesser horseshoe bats) and disrupted commuting routes when hedges were lit (53.09 lux). The unlit side of the hedge (4.17 lux) was also avoided and 0.45 lux was considered to be too bright. Thus the range of light along our transect may have been too bright for the clutter foragers.
With the clutter-edge and open-air feeding guilds, conclusions were less clear-cut. However, the percentage of natural vegetation was the most important factor affecting observed patterns of bat activity. Total AI, foraging guild activity, species richness, feeding and non-feeding behaviour were highest where vegetation cover was at least 50%, as we expected for clutter-edge but not for high-flying open-air bats. Both open-air bat activity in general and that of particular molossid species (see “Results”) were associated with 100% of natural vegetation (furthest from the mine) which was surprising, especially since two of these species are known to roost in buildings on the mine property. It is possible that the low number of open-air feeding bats in well-lit open areas close to the mine infrastructure could have been due to these bats navigating visually using the artificial illumination of the mine. Orientation by sight is not implausible since molossids generally commute and forage in the open, fairly high above the ground where the risk of collision with stationary objects is low. It has been shown that vision takes priority over echolocation when bats are travelling far distances (commuting or migrating) and in instances where a bat may be receiving conflicting information from its sight and echolocation calls (Eklöf, 2003; Gorresen et al., 2015; Liu et al., 2015; Rowse et al., 2016).
As expected for open-air but not clutter-edge feeding bats, neither light nor noise levels had a significant impact on the activity of clutter-edge and open-air feeding bats, separately or combined. A similar pattern was observed by Bunkley et al. (2015) for the open-air feeding molossid, T. brasiliensis where their activity was higher over quieter areas of natural gas extraction. With the clutter-edge feeders, it is possible that habituation to noise has occurred; Bunkley et al. (2015) found that Myotis lucifugus, M. californicus, M. cillolabrum, and Parastrellus hesperus were not affected by noise associated with natural gas extraction. Since they rely more on echolocation to detect and capture prey as opposed to listening for acoustic cues from prey, noise possibly did not impact them in the manner that it would affect gleaning bat species. For example, M. myotis, a gleaner, habituated to traffic noise during torpor but was negatively impacted by traffic noise during foraging activity (Jones, 2008; Schaub et al., 2008; Luo et al., 2014). Organisms can adapt to a particular disturbance regime (Grindal and Brigham, 1998). Since the Venetia diamond mine has been operational since 1992 (27 years at the time of data collection), we assume that the bats inhabiting and using the resources of the Venetia Diamond mine have possibly adapted to a degree to changes in the landscape (including habitat clearing) and possibly habituated to the nightly artificial light and the persistent anthropogenic noise from the mining.
There is no evidence from the current study indicating that either open-air or clutter-edge feeding bats used the flood lights as feeding opportunities. Some species of fast-flying bat species belonging to the genera Tadarida, Myotis, Eptesicus, Pipistrellus, and Vespertilio to name a few, benefit greatly from increased feeding opportunities around street lamps as the bats predated on insects that were attracted by shortwave light (Rydell, 1992; Stone et al., 2009, 2015; Schoeman, 2015). In fact, our results show an opposite trend where foraging activity (of open-air and clutter-edge feeding bats combined) is significantly inversely correlated with light intensity. The observed pattern may have been influenced by the early spring and late summer sampling periods when insect abundance could expectedly be low.
The observed pattern of activity in response to Tmin and moon phase is well known and well documented in the literature (Ciechanowski et al., 2007; Appel et al., 2016; Pech-Canché et al., 2018; Pretorius et al., 2020). Reduced bat activity has often been associated with the energetic costs of flight, reduced prey availability and maintaining stable body temperatures during cooler temperatures and unfavourable weather conditions (Erickson and West, 2002; Bender and Hartman, 2015). Moon phase was shown to be important in our best-fit models but only significantly so for feeding activity where feeding activity was significantly lower during the first quarter, waning gibbous and waxing gibbous (Figure 5A). Whether bats were experiencing true lunar phobia during foraging activities or relying more on visual cues is yet to be investigated.
To our knowledge, no study has simultaneously incorporated the impact of ecological light pollution and continuous noise from mining operations on bat species. The study of bats on the Venetia diamond mine highlights the anthropogenic factors (artificial lighting, noise and habitat degradation) and natural factors that influenced bat activity. As the first case study of the impacts of opencast diamond mining in South Africa (and globally), we hope that the study highlights the need for rigorous scientific studies, inspires students, researchers and consultants alike to investigate the impacts of large-scale developments, particularly mines. It is crucial to understand how African bats respond or adapt to mining developments in the field.
Data Availability Statement
The raw data supporting the conclusions of this article will be made available by the authors, without undue reservation.
Ethics Statement
The animal study was reviewed and approved by Research Ethics Committee, University of Venda, Thohoyandou.
Author Contributions
DC-T and PJT contributed to conception and design of the study. DC-T wrote the first draft of the manuscript, organised the database, and performed the statistical analysis with assistance from PJT. Both authors contributed to manuscript revision, read, and approved the submitted version.
Funding
PJT acknowledges the financial support of the University of Venda, the National Research Foundation and the Department of Science and Technology under the South African Research Chair Initiative (SARChI) on Biodiversity Value and Change within the Vhembe Biosphere Reserve hosted at University of Venda and co-hosted by the Centre for Invasion Biology at University of Stellenbosch (Grant No. 87311).
Conflict of Interest
The authors declare that the research was conducted in the absence of any commercial or financial relationships that could be construed as a potential conflict of interest.
Publisher’s Note
All claims expressed in this article are solely those of the authors and do not necessarily represent those of their affiliated organizations, or those of the publisher, the editors and the reviewers. Any product that may be evaluated in this article, or claim that may be made by its manufacturer, is not guaranteed or endorsed by the publisher.
Acknowledgments
We thank the Venetia Diamond Mine Environmental Department who provided crucial support and logistics for the undertaking of this study for which we are incredibly grateful. Many thanks to Duncan McFayden and Gavin Anderson for allowing the research to be conducted on the Venetia Diamond Mine. A huge thanks to Ibart Janse van Rensburg and Cecile Cloete for their invaluable assistance beyond the scope of their work roles and responsibilities by assisting DC-T with setting up and decommissioning the bat detectors and organising access to the mine and sorting out all necessary logistics (I. Janse van Rensberg).
Supplementary Material
The Supplementary Material for this article can be found online at: https://www.frontiersin.org/articles/10.3389/fevo.2022.752665/full#supplementary-material
Supplementary Figure 1 | AI across the transect for the two main foraging guilds, open-air foragers (right bar) and clutter-edge foragers (left bar), and for two different behaviours, foraging (lower bars) and commuting (upper bars). The activity index of the clutter foragers and social activity were excluded from the current plot as clutter forager activity social calls were minute in comparison to the other two foraging guilds and activity types (see Table 1 for detail).
Footnotes
- ^ https://www.debeersgroup.com/the-group/our-history
- ^ www.wildlifeacoustics.com
- ^ https://www.wildlifeacoustics.com/resources/video-tutorials/kaleidoscope-pro-software/en/kaleidoscope-pro-software-noise-level-analysis-english
References
Aldridge, H. D. J. N., and Rautenbach, I. L. (1987). Morphology, echolocation and resource partitioning in insectivorous bats. J. Anim. Eco. 56, 763–778.
Appel, G., López-Baucells, A., Magnusson, W. E., and Bobrowiec, P. E. D. (2016). Aerial insectivorous bat activity in relation to moonlight intensity. Mamm. Bio. 85, 37–46. doi: 10.1016/j.mambio.2016.11.005
Arlettaz, R., Godat, S., and Meyer, H. (2000). Competition for food by expanding pipistrelle bat populations (Pipistrellus pipistrellus) might contribute to the decline of lesser horseshoe bats (Rhinolophus hipposideros). Bio. Cons. 93, 55–60. doi: 10.1016/S0006-3207(99)00112-3
Armstrong, K. N. (2010). Assessing the short-term effect of minerals exploration drilling on colonies of bats of conservation significance: a case study near Marble Bar. Western Australia J. R. Soc. West. Aust. 93, 165–174.
Azam, C., Le Viol, I., Julien, J., Bas, Y., Zissis, G., Vernet, A., et al. (2018). Evidence for distance and illuminance thresholds in the effects of artificial lighting on bat activity. Landsc. Urban Plan 175, 123–135. doi: 10.1016/j.landurbplan/2018.02.011
Bader, E., Jung, K., Kalko, E. K. V., Page, R. A., Rodriguez, R., and Sattler, T. (2015). Mobility explains the response of aerial insectivorous bats to anthropogenic habitat change in the Neotropics. Bio. Cons. 186, 97–106. doi: 10.1016/j.biocon.2015.02.028
Bailey, L. A., Brigham, R. M., Bohn, S. J., Boyles, J. G., and Smit, B. (2019). An experimental test of the allotonic frequency hypothesis to isolate the effects of light pollution on bat prey selection. Oecologia 190, 367–374. doi: 10.1007/s00442-019-04417-w
Bender, M. J., and Hartman, G. D. (2015). Bat activity increases with barometric pressure and temperature during autumn in central Georgia. Southeast. Nat. 14, 231–242.
Berglund, B., Lindvall, T., and Schwela, D. H. (2000). New WHO Guidelines for Community Noise. Noise Vib. Worldw. 31, 24–29. doi: 10.1260/0957456001497535
Bohn, K. M., Moss, C. F., and Wilkinson, G. S. (2006). Correlated evolution between hearing sensitivity and social calls in bats. Biol. Lett. 2, 561–564.
Boldogh, S., Dobrosi, D., and Samu, P. (2007). The effects of the illumination of buildings on house-dwelling bats and its conservation consequences. Acta Chiropt. 9, 527–534.
Brown, R. J., Tait, M., Field, M., and Sparks, R. S. J. (2009). Geology of a complex kimberlite pipe (K2 pipe, Venetia Mine, South Africa): insights into conduit processes during explosive ultrabasic eruptions. Bull. Volcanol. 71, 95–112. doi: 10.1007/s00445-008-0211-4
Bunkley, J. P., and Barber, J. R. (2015). Noise reduces foraging efficiency in Pallid bats (Antrozous pallidus). Int. Behav. Biol. Ethol. 121, 1116–1121. doi: 10.1111/eth.12428
Bunkley, J. P., McClure, C. J. W., Kleist, N. J., Francis, C. D., and Barber, J. R. (2015). Anthropogenic noise alters bat activity levels and echolocation calls. Glob. Ecol. Conserv. 3, 62–71.
Ciechanowski, M., Zając, T., Biłas, A., and Dunajski, R. (2007). Spatiotemporal variation in activity of bat species differing in hunting tactics: effects of weather, moonlight, food abundance, and structural clutter. Can. J. Zool. 85, 1249–1263. doi: 10.1139/Z07-090
Cory Toussaint. (2021). The Potential of bats as Bioindicators for Areas Currently Transformed by Opencast Mining within the Vhembe Biosphere Reserve, Limpopo Province, South Africa. [Ph.D thesis].Thohoyandou: University of Venda.
Cravens, Z. M., and Boyles, J. G. (2019). Illuminating the physiological implications of artificial light on an insectivorous bat community. Oecologia 189, 69–77. doi: 10.1007/s00442-018-4300-6
Duarte, M. H. L., Sousa-Lima, R. S., Young, R. J., Farina, R. J., Vasconcelos, M., Rodrigues, M., et al. (2015). The impact of noise from open-cast mining on Atlantic forest biophony. Biol. Conserv. 191, 623–631. doi: 10.1016/j.biocon.2015.08.006
Eklöf, J., Šuba, J., Petersons, G., and Rydell, J. (2014). Visual acuity and eye size in five European bat species in relation to foraging and migration strategies. Environ. Exp. Biol. 12, 1–6.
Erickson, J. L., and West, S. D. (2002). The influence of regional climate and nightly weather conditions on activity patterns of insectivorous bats. Acta Chiropt. 4, 17–24. doi: 10.3161/001.004.0103
Finch, D., Schofield, H., and Mathews, F. (2020). Traffic noise playback reduces the activity and feeding behaviour of free-living bats. Environ. Pollut. 263:114405. doi: 10.1016/j.envpol.2020.114405
Fox, J., and Monette, G. (1992). Generalized collinearity diagnostics. J. Am. Stat. Assoc. 87, 178–183.
Frick, W. F., Kinston, T., and Flanders, J. (2019). A review of the major threats and challenges to global bat conservation. Ann. N. Y. Acad. Sci. 1469, 5-25. doi: 10.1111/nyas.14045
Gaston, K. J., and Bennie, J. (2014). Demographic effects of artificial nighttime lighting on animal populations. Environ. Rev. 22, 323–330. doi: 10.1139/er-2014-0005
Gaston, K. J., Bennie, J., Davies, T. W., and Hopkins, J. (2013). The ecological impacts of nighttime light pollution: a mechanistic appraisal. Biol. Rev. 88, 912–927. doi: 10.1111/brv.12036
Geipel, I., Smeekes, M. J., Halfwerk, W., and Page, R. A. (2019). Noise as an information cue for decision-making: the sound of rain delays bat emergence. J. Exp. Biol. 222:192005. doi: 10.1242/jeb.192005
Gomes, D. G. E., Page, R. A., Geipel, I., Taylor, R. C., Ryan, M. J., and Halfwerk, W. (2016). Bats perceptually weight prey cues across sensory systems when hunting in noise. Science 353, 1277–1280. doi: 10.1126/science.aaf7934
Gorresen, P. M., Cryan, P. M., Dalton, D. C., Wolf, S., and Bonaccorso, F. J. (2015). Ultraviolet vision may be widespread in bats. Acta Chiropt. 17, 193–198. doi: 10.3161/15081109ACC2015.17.1.017
Grindal, S. D., and Brigham, R. M. (1998). Short-term effects of small-scale habitat disturbance on activity by insectivorous bats. J. Wildl. Manag. 62, 996–1003. doi: 10.2307/3802552
Haddock, J. K., Threlfall, C. G., Law, B., and Hochuli, D. F. (2019). Light pollution at the urban forest edge negatively impacts insectivorous bats. Biol. Conserv. 236, 17–28. doi: 10.1016/j.biocon.2019.05.016
Jones, G. (2008). Sensory Ecology: noise annoys foraging bats. Curr. Biol. 18, R1098–R1100. doi: 10.1016/j.cub.2008.10.005
Jones, G., Jacobs, D. S., Kunz, T. H., Willig, M. R., and Racey, P. (2009). Carpe noctem: the importance of bats as bioindicators. Endanger. Species Res. 8, 93–115. doi: 10.3354/esr00182
Jung, K., and Threlfall, C. G. (2018). Trait-dependent tolerance of bats to urbanization: a global meta-analysis. Proc. R. Soc. B. 285:20181222. doi: 10.1098/rspb.2018.1222
Lattenkamp, E. Z., Nagy, M., Drexl, M., Vernes, S. C., Wiegrebel, L., and Knörnschild, M. (2020). Hearing sensitivity and amplitude coding in bats are differentially shaped by echolocation calls and social calls. Proc. R. Soc. Lon. B 288:20202600. doi: 10.6084/m9.figshare.c.5237447
Lewanzik, D. (2017). Artificial Light Affects Bats Across Climatic Zones and Feeding Guilds. [Ph.D thesis].Germany: Freie Universität, Berlin doi: 10.17169/REFUBIUM-4256
Lewanzik, D., and Voigt, C. C. (2017). Transition from conventional lighting to light-emitting diode street lighting changes activity of urban bats. J. App. Ecol. 54, 264–271. doi: 10.1111/1365-2664.12758
Liu, H., Wei, J., Li, B., Wang, M., Wu, R., Rizak, J. D., et al. (2015). Divergence of dim-light vision among bats (order: chiroptera) as estimated by molecular and electrophysical methods. Sci. Rep. 5:11531. doi: 10.1038/srep11531
Luo, J., Clarin, B. M., Borissov, I. M., and Siemers, B. M. (2014). Are torpid bats immune to anthropogenic noise? J. Exp. Biol. 217, 1072–1078. doi: 10.1242/jeb.092890
Luo, J., Siemers, B. M., and Koselj, K. (2015). How anthropogenic noise affects foraging. Glob. Change Biol. 21, 3278–3289. doi: 10.1111/gcb.12997
Miller, B. W. (2001). A method for determining relative activity of free flying bats using a new activity index for acoustic monitoring. Acta Chiropt. 3, 93–105.
Minnaar, C., Boyles, J. G., Minnaar, I. A., Sole, C. L., and McKechnie, A. E. (2014). Stacking the odds: light pollution may shift the balance in an ancient predator-prey arms race. J. Appl. Ecol. 52, 522–531. doi: 10.1111/1365-2664.12381
Monadjem, A., Shapiro, J. T., Mtsetfwa, F., Reside, A. E., and Robert, A. (2017). Acoustic call library and detection distances for bats of Swaziland. Acta Chiropt. 19, 175–187. doi: 10.3161/15081109ACC2017.19.1.014
Monadjem, A., Taylor, P. J., Cotterill, F. P. D., and Schoeman, M. C. (2020). Bats of Southern and Central Africa: A Biogeographic and Taxonomic Synthesis. Johannesburg: University of Witwatersrand Press.
Mucina, L., and Rutherford, M. C. (eds) (2011). The vegetation of South Africa, Lesotho and Swaziland. Strelitiza 19. Pretoria: South African National Biodiversity Institute.
Naidoo, S., Vosloo, D., and Schoeman, M. C. (2016). Pollutant exposure at wastewater treatment works affects the detoxification organs of an urban adapter, the banana bat. Environ. Pollut. 208, 830–839. doi: 10.1016/j.envpol.2015.09.056
Norberg, U. M., and Rayner, J. M. V. (1987). Ecological morphology and flight in bats (Mammalia. Phil. Trans. R. Soc. Lond. B. 316, 335–427. doi: 10.1098/rstb.1987.0030
Pech-Canché, J. M., Villegas, P. D. C., Chamorro-Florescano, I. A., Méndes, A., and Lozano-Rodríguez, M. A. (2018). Lunar phobia in phyllostomid bats at La Ceiba, Tuxpan, Veracruz. Ecosist. Y Recur. Agropecuarios 5, 165–170. doi: 10.19136/era.a5n13.1033
Pretorius, M., Van Cakenberghe, V., Broders, H., and Keith, M. (2020). Temperature explains variation in seasonal temporal activity of Miniopterus natalensis more than moonlight or humidity. Mamm. Res. 65, 755–765. doi: 10.1007/s13364-020-00531-w
Rowse, E. G., Lewanzik, D., Stone, E. L., Harris, S., and Jones, G. (2016). “Chapter 7: Dark Matters: The effects of artificial lighting on bats,” in Bats in the Anthropocene: Conservation of Bats in a Changing World, eds C. C. Voigt and T. Kingston, Germany: Freie Universität Berlin. doi: 10.1007/978-3-319-25220-9_7
Rydell, J. (1992). Exploitation of insects around streetlamps by bats in Sweden. Fun. Ecol. 6, 744–750. doi: 10.2307/2389972
Salinas-Ramos, V. B., Ancillotto, L., Cistrone, L., Nastasi, C., Bosso, L., Smeraldo, S., et al. (2021). Artificial illumination influences niche segregation in bats. Environ. Pollut. 284:117187. doi: 10.1016/j.envpol.2021.117187
Schaub, A., Ostwald, J., and Siemers, B. M. (2008). Foraging bats avoid noise. J. Exp. Biol. 211, 3174–3180. doi: 10.1242/jeb.022863
Schoeman, M. C. (2015). Light pollution at stadiums favors urban exploiter bats. Anim. Conserv. 19, 120–130. doi: 10.1111/acv.12220
Sengpiel Audio (2014). Available online at: http://www.sengpielaudio.com/calculator-soundlevel.htm.
Simmons, J. A., Lavender, W. A., Lavender, B. A., and Childs, J. E. (1978). Echolocation by free-tailed bats (Tadarida). J. Comp. Physiol. 125, 291–299.
Stone, E. L., Harris, S., and Jones, G. (2015). Impacts of artificial lighting on bats: a review of challenges. Mamm. Biol. 80, 213–219. doi: 10.1016/j.mambio.2015.02.004
Stone, E. L., Jones, G., and Harris, S. (2009). Street lighting disturbs commuting bats. Curr. Biol. 19, 1123–1127. doi: 10.1016/j.cub.2009.05.058
Taylor, P. J., Nelufule, M., Parker, D. M., Cory Toussaint, D., and Weier, S. M. (2020). The Limpopo River exerts a powerful but spatially limited effect on bat communities in a semi-arid region of South Africa. Acta Chiropt. 22, 75–86. doi: 10.3161/15081109ACC2020.22.1.007
Taylor, P. J., Sowler, S., Schoeman, M. C., and Monadjem, A. (2013). Diversity of bats in the Soutpansberg and Blouberg Mountains of northern South Africa: complementarity of acoustic and non-acoustic survey methods. S. Afr. J. Wildl. Res. 43, 12–26. doi: 10.3957/056.043.0117
Keywords: chiroptera, anthropogenic light, noise, bat behaviour, opencast mining, bat ecology
Citation: Cory-Toussaint D and Taylor PJ (2022) Anthropogenic Light, Noise, and Vegetation Cover Differentially Impact Different Foraging Guilds of Bat on an Opencast Mine in South Africa. Front. Ecol. Evol. 10:752665. doi: 10.3389/fevo.2022.752665
Received: 03 August 2021; Accepted: 16 March 2022;
Published: 27 April 2022.
Edited by:
Ludmilla M. S. Aguiar, University of Brasilia, BrazilReviewed by:
Brock Fenton, Western University, CanadaThiago Vieira, Federal University of Pará, Brazil
Copyright © 2022 Cory-Toussaint and Taylor. This is an open-access article distributed under the terms of the Creative Commons Attribution License (CC BY). The use, distribution or reproduction in other forums is permitted, provided the original author(s) and the copyright owner(s) are credited and that the original publication in this journal is cited, in accordance with accepted academic practice. No use, distribution or reproduction is permitted which does not comply with these terms.
*Correspondence: Dawn Cory-Toussaint, bnljdGVyaXMuY29yeTJzYWludEBnbWFpbC5jb20=