- 1Department of Ecoscience, Aarhus University, Aarhus, Denmark
- 2Natural History Museum, Aarhus, Denmark
- 3Stiftung Naturschutz Schleswig-Holstein, Molfsee, Germany
- 4Stiftung Wildtiere im Norden, Molfsee, Germany
- 5Center for Wildlife Genetics, Senckenberg Research Institute, Frankfurt, Germany
- 6Department of Biology, Aarhus University, Aarhus, Denmark
Attacks by large predators on livestock are an important driver of conflicts. Consequently, knowledge about where predators occur, where livestock depredation takes place and what factors influence it will aid the mitigation of stakeholder conflicts. Following legal protection, wolves (Canis lupus) in Central Europe are recently spreading to areas dominated by agriculture, bringing them in closer contact with livestock. Here, we analyzed habitat selection and livestock depredation rates of 43 wolves identified by genotyping on the Jutland peninsula, consisting of mainland Denmark and the northernmost German federal state Schleswig-Holstein. Occupancy by resident wolves correlated positively with forest and other non-forested semi-natural land cover (habitat for natural ungulate prey), whereas occupancy by non-resident wolves correlated with increasing forest cover and sheep density. The latter effect likely reflected increased sampling probability of highly mobile dispersers killing livestock. We recorded 565 livestock depredation events (85 in Denmark and 480 in Schleswig-Holstein), of which 42% (55 in DK and 185 in SH) could be assigned to 27 individual wolves based on DNA evidence. Livestock (mostly sheep) were killed by wolves in 16% of the study area. Our results indicate that wolves mostly killed livestock as a context-dependent response, i.e., being dispersers in agricultural areas with low availability of wild ungulate prey and high livestock densities, and not because of behavioral preferences for sheep. Moreover, the livestock depredation was lower in areas with livestock protection measures (implemented in areas with established pairs/packs). We conclude that while wolf attacks on livestock in established wolf territories generally can be reduced through improvement of fences, livestock depredation by non-resident wolves in agricultural areas constitutes a bigger challenge. Albeit technically possible, the economic costs of implementing predator-proof fences and other preventive measures in such pastoral areas infrequently visited by wolves will be considerable. Experiences so far further indicate that lethal removal of identified “problem wolves” may be inefficient in practice.
Introduction
Following severe historic persecution leading to the absence of large carnivores in many areas of Central Europe during most of the nineteenth and mid-twentieth century, large carnivore populations have increased over recent decades (Chapron et al., 2014). The gray wolf (Canis lupus) has received legal protection in the 1980s in most European countries, and has since increased its geographic range (Nowak and Myslajek, 2016; Reinhardt et al., 2019). In general, wolves can persist in human-modified landscapes as long as human tolerance and policy are favorable (Boitani and Linnell, 2015; Reinhardt et al., 2019). Apart from legal protection and supportive public opinion, the main factors for large carnivore recovery (Chapron et al., 2014), habitat suitability for wolves has increased over the last two decades in some European areas, including Central and Northern Europe, which is correlated with decreasing human population density and increasing forest cover (Cimatti et al., 2021). Moreover, wolf occurrence depends on social status, with wolf pairs/packs occupying higher-quality habitats characterized by lower anthropogenic impacts like forests, whereas dispersing individuals can be found in a broad array of habitat types (Nowak et al., 2017).
The natural recolonization process by wolves has resulted in socio-political conflicts that may jeopardize conservation outcomes if not adequately managed. One of the most challenging conservation issues is that wolves predate on livestock, especially when they have returned to areas (after long periods of absence) where people are not habituated to animal husbandry practices that prevent damage (Linnell, 2013). Consequently, wolf predation on livestock can lead to social conflicts between conservationists, farmers and other stakeholder groups (Bautista et al., 2019). Livestock damage is often restricted to few farms (Gazzola et al., 2008), and depends on landscape structure and availability of natural prey (Treves et al., 2004; Suryawanshi et al., 2013; Imbert et al., 2016). Additionally, the social status or family history of individual wolves can affect livestock depredation rates. For example, in Northern Italy dispersing wolves killed more livestock compared to resident pairs and packs (Imbert et al., 2016). Sometimes single individuals or packs are responsible for disproportionately high livestock damage, which often results in public pressure for such “problem individuals” to be culled. From a management perspective, it is essential to identify why wolves predate on livestock. They either do so because of the ambient settings (being in the wrong place) or due to individual behavioral inclinations to kill livestock (compared to other individuals in the same setting) (Linnell et al., 1999). This distinction is important, because the EU Habitats Directive only allows lethal management of the latter type of wolves, whereas losses caused by normally behaving wolves must be solved otherwise, e.g., through protective measures. Prey specialization is a well-recognized phenomenon in generalist predators (Araújo et al., 2011; Dickman and Newsome, 2015) and problem-behaviors are known to vary individually (Swan et al., 2017), which might be transferred socially from parents to offspring, as shown in Grizzly bears (Ursus arctos) (Morehouse et al., 2016). However, to our knowledge, individual versus context dependent variation in livestock depredation rates have not been rigorously analyzed in wolves.
Importantly, livestock depredation by large carnivores can impact the attitudes of different stakeholder groups, which can influence effective conservation (Dressel et al., 2015). In Europe, attitudes toward large carnivores tended to become more negative with perceived increases in large carnivore abundance and risk of damage, especially in areas where people have to co-exist with large carnivores (Ericsson and Heberlein, 2003; Eriksson et al., 2015). Hence, to reduce conflict levels, keeping damage to livestock at low levels is important (Bautista et al., 2019). To do so, it is crucial to gather knowledge on wolf distribution and habitat use (Reinhardt et al., 2019; Cimatti et al., 2021), impact of livestock density on colonization patterns, as well as identifying spatial centers of livestock predation. Moreover, it is important to evaluate existing livestock protection measures (Eklund et al., 2017).
In this study, we investigated patterns of wolf settlement and predation on livestock in Jutland peninsula, one of Europe’s most intensively cultivated regions, consisting of the northernmost German federal state Schleswig-Holstein (SH) and mainland Denmark (DK). We hypothesized that wolf settlement would generally be associated to land cover and human impact, as previously shown (Cimatti et al., 2021), and predicted that wolf occupancy increased with increasing forest and heathland cover (and other natural areas), and with decreasing human impact (human population density and road density). Additionally, we hypothesized that wolf occupancy depends on the social status of wolves, and predicted that non-residents (dispersers), occur in more landscape types than resident wolves (Nowak et al., 2017).
We then investigated depredation rates on livestock by individual wolves and related these patterns to sheep density, land cover, season, wolf social status, and livestock protection measures. Specifically, we predicted that livestock depredation rate decreases with increasing forest and heathland cover, because these land covers are associated with higher abundance of wild ungulates (Borowik et al., 2013), and depredation rate increases in areas with higher densities of sheep that are unprotected by predator-proof fences. Further, we predicted that non-resident wolves have higher livestock depredation rates compared to residents, because (1) non-residents occur more frequently in landscapes that facilitate livestock depredation, and (2) possibly because they are more prone to kill livestock due to unfamiliarity with the local area and/or inexperience. We also tested the extent to which implementation of livestock protection measures (predator-proof fences) lead to a decrease in livestock depredation rate. Finally, we evaluated the magnitude of individual variation in livestock kill rates. If wolf predation on livestock is primarily determined by ambient conditions and social status we expected little individual variation in depredation rates, whereas substantial individual variation should remain if personality and individual behavior had a major influence on a wolf’s inclination to kill livestock.
Materials and Methods
Study Area and Wolf Population Monitoring
The 470-km long Jutland peninsula belongs to Schleswig-Holstein (SH) and Denmark (DK; Figure 1). SH and Hamburg cover an area 16,430 km2, inhabited by 4.47 million people (average population density: 272 per km2), and are covered by 68% farmland, 13% forest, 10% developed, and 9% other land cover. The Danish region of Jutland (29,778 km2, 2.58 million people, average population density: 87 people per km2) is covered by 61% farmland, 13% forest, 12% developed, 10% heathland, and 4% other land cover. Jutland connects to the Central European mainland through a 60-km wide stretch between the North Sea and the Baltic Sea down to the city of Hamburg. In SH, the majority of the human population is located in the southern part of the state that connects to the federal states Niedersachsen and Mecklenburg-Vorpommern.
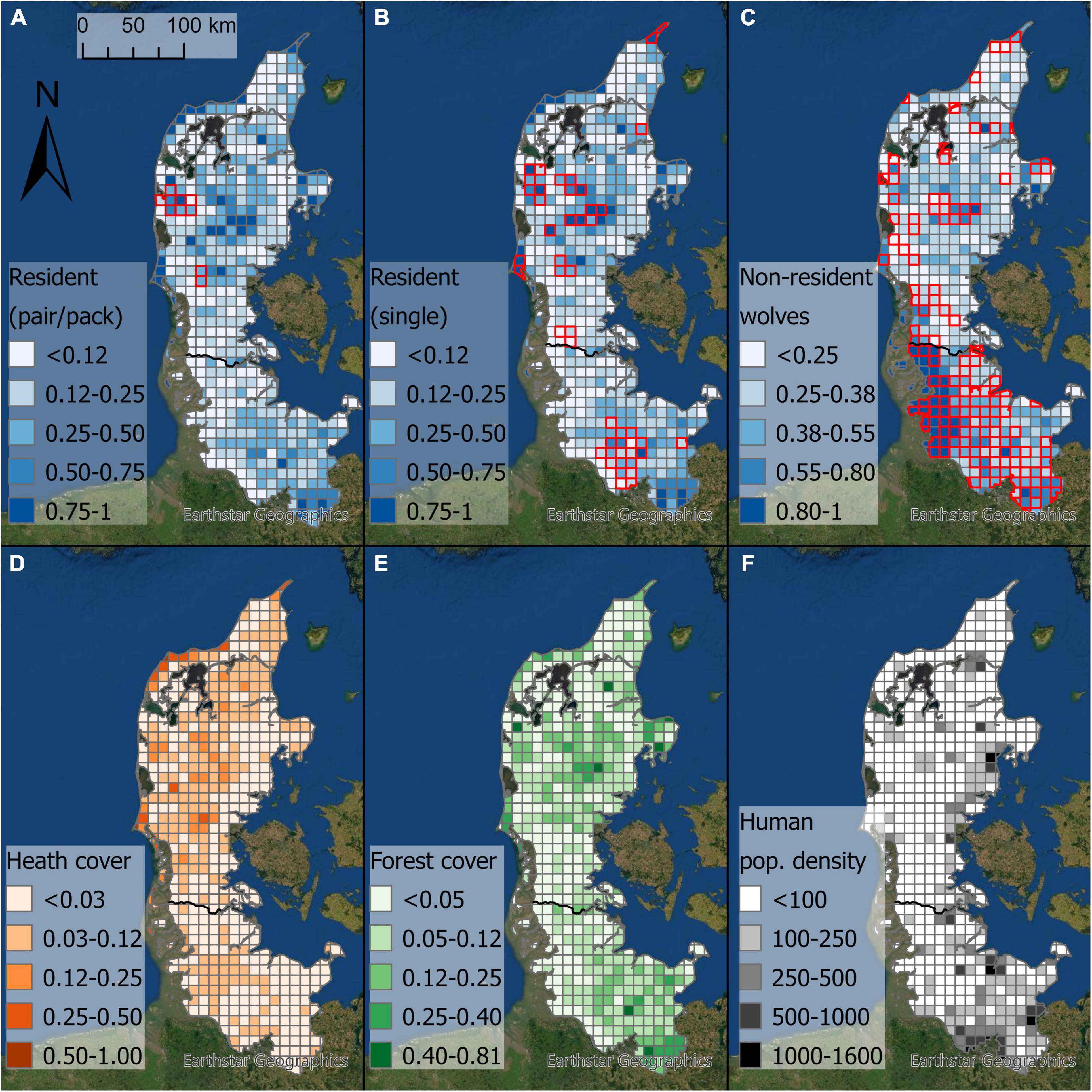
Figure 1. Maps depicting our study area in Jutland separated into 598 grid cells. Red borders show grid cells with observations of (A) resident wolf pairs/packs, (B) single residents, and (C) non-resident wolves. The blue shading depicts the predicted probability of grid cell occupancy by resident wolves [(A,B) pairs/packs and single residents were merged for the analysis], and non-resident wolves (C). (D) Proportion of heathland cover (and other non-forested semi-natural areas in SH) per grid cell. (E) Proportion of forest cover per grid cell. (F) Average human population density (people per km2) per grid cell. The black line represents the border between Denmark in the north and Schleswig-Holstein in the south.
Since the Central European wolf population (to which the wolves in Jutland belong) established in the border region between Eastern Germany and Poland around year 2000 (Nowak and Myslajek, 2016; Reinhardt et al., 2019), wolves have been surveyed by means of genetic markers, enabling to identify individuals and to reconstruct their origin and dispersal paths (Andersen et al., 2015). Governmental agencies and research institutions systematically sample DNA from scats, dead wolves, and livestock kills both in DK and SH. In SH, where sheep farming is common and only recently starting to adapt to wolf presence, livestock depredation has contributed to most genotype identifications. In DK, where wolves kill livestock less frequently, monitoring is primarily undertaken by DNA analyses of scats (Sunde et al., 2021). Because there is only a single wolf observation before 2012 (a roadkill from SH in 2007), we defined the period of our study from 2012 to the first quarter of 2021. Wolf observations were categorized according to the SCALP (Status and Conservation of the Alpine Lynx Population) criteria (Reinhardt et al., 2015), and we only used C1 observations, defined as unambiguously confirmed observations based on facts, for our analyses. Wolves were categorized as being resident when they were observed for ≥6 months in the same area (either as single resident or as wolf pair/pack), and non-residents (usually dispersers) when they were observed <6 months in the same area (Reinhardt et al., 2015). Observations that could be not assigned to an individual wolf were generally coded as non-residents unless we had strong evidence that they were part of a wolf pack/pair (e.g., based on their spatial location).
Land Cover, Human Population Density, and Sheep Density
We created 598 10 km × 10 km grid cells over the entire study area as observational unit to model wolf occurrence (Figure 1), because this size provides a trade-off between information precision and the average home range size of wolves (Chapron et al., 2014; Milanesi et al., 2017; Cimatti et al., 2021). We downloaded land cover data of 2018 from the CORINE land cover database1, and categorized the 44 land cover types into seven biologically relevant categories: (1) built up areas, (2) grassland, (3) cropland, (4) forest, (5) heathland and other (semi)natural land cover (hereafter heathland), (6) water, and (7) other land cover (Supplementary Table 1). For each grid cell we estimated the proportion of forest and heathland (Figure 1), as these land cover types were previously shown to be important for wolves (Sunde and Olsen, 2018). Because there is very little heathland in SH, we additionally included land cover types that were analogous to heathland in DK, based on habitat monitoring data from the Landesamt für Landwirtschaft, Umwelt und ländliche Räume des Landes Schleswig-Holstein2. These land cover types included bushes, woody vegetation outside forests, natural non-forest vegetation not in agricultural use, dune vegetation, moors, and swamps (Supplementary Table 1). We downloaded vector data of roads from the open source database OpenStreetMap3. We only included larger roads (motorways, primary, secondary, and tertiary roads), and intersected these roads with the grid cells to calculate the road density (m road per ha) in each grid cell. Moreover, we obtained data on human population density at 30 arc-second horizontal resolution from the Socioeconomic Data and Applications Center from the Gridded Population of the World (GPW), v.4 dataset for 20204. We then calculated the mean human population density for each 10 km × 10 km grid cell using the ‘‘Zonal Statistics as Table’’ tool in ArcGIS Pro. As a measure of livestock densities, we focused on sheep, because they made up the bulk of livestock kills (see section ‘‘Results’’). Sheep numbers were obtained on municipality level from the Danish central animal register5 for DK and from the Statistikamt Nord6 for SH. Using these numbers, we calculated the sheep density for each municipality (mean ± SD = 0.04 ± 0.06 sheep per ha, median = 0.02, range = 0–0.63 sheep per ha).
Livestock Depredation Patterns
A livestock depredation event was defined as an event where one or more livestock individuals (at the same place within the same day) were killed by a wolf, based on DNA evidence either with (43% of cases) or without (47% of cases) identification of an individual genotype (Reinhardt et al., 2015; Thomsen et al., 2020). To estimate individual livestock depredation rates, we used observations between 1 January 2017 and 15 August 2021, because both countries systematically sampled DNA from saliva left on livestock suspected to be killed by wolves during this period (which was not the case prior to 2017). The individual livestock depredation rate of wolves was estimated as genetically verified predation events relative to the number of days a given wolf was estimated to occur in a given area (grid cell), i.e., the number of livestock depredation events per individual wolf per day. To fill gaps between consecutive observations, we assumed that a wolf would stay in the grid cell where it was observed until the next observation. For example, if a wolf was first observed in grid cell A, and 10 days later in grid cell B to appear in grid cell C after another 5 days, we allocated ten observation days to grid cell A and five observation days to grid cell B. If the observation in grid cell A was based on a DNA profile from a sheep kill, we entered the data as one observation day with livestock depredation and nine observation days without livestock depredation. Accordingly, if the second registration in grid cell B was not based on a predation event (e.g., feces), we recorded 5 days without livestock depredation for grid cell B. For wolves that were not observed for more than 1 year, we assumed that their continued presence was highly unlikely, and estimated the likely day of disappearance as the last observation date plus the average observation interval until its disappearance, adding those days to the last observation (Sunde et al., 2021). We acknowledge that it is not realistic that wolves (especially non-residents) always stayed within the same grid cell for the whole period until the next observation, but consider this approach reasonably unbiased, because observation intervals were short (mean ± SD: 9 ± 13 days in SH and 17 ± 28 days in DK). We obtained 234 observations of identified wolves based on DNA profiles sampled from different livestock depredation events and 689 observations from other sources (Supplementary Table 2), corresponding to 11,960 (88%) observation days in DK and 1,695 (12%) in SH.
Statistical Analyses
Grid Cell Occupancy
We modeled wolf occupancy per 10 km × 10 km grid cell in Jutland, by fitting linear models using Generalized Least Squares of the R package “nlme” (Pinheiro et al., 2017) that allow to account for spatial autocorrelation. For each grid cell, we counted the number of 3-month periods (annual quarters) with confirmed wolf observations out of the total number of quarters monitored (37 quarters from 2012 to 2021) as response variable (i.e., the proportion of quarters with wolf observations). We did this separately for resident wolves (single residents, pairs and packs) and non-residents, because they might select for different land cover and prey (two separate analyses; Supplementary Table 3). As predictor variables, we included the proportion of forest, proportion of heathland, road density, averaged human population density, and sheep density. We modeled spatial effects within an error term, using a spherical function for the correlation matrix (consisting of the x and y coordinate of the top-right corner of each grid cell) of the errors (Beale et al., 2010). Model fitting was performed with restricted maximum likelihood. We scaled all numeric variables (mean = 0; standard deviation = 1) to obtain comparable estimates. There was no collinearity among predictor variables, defined as variance inflation factors (VIF) >3 and Pearson correlation >0.7 (Supplementary Figure 1; Zuur et al., 2010), and no overdispersion in the models. For model selection, we created all possible combinations of the dependent variables using the R package “MuMIn” (Barton, 2016), including five candidate models based on biological hypotheses, a full and a intercept only model (Supplementary Table 3). We selected the most parsimonious model based on Akaike’s Information Criterion corrected for small sample size (AICc; Wagenmakers and Farrell, 2004). Parameters that included zero within their 95% confidence interval were considered uninformative (Arnold, 2010). We assessed the models’ predictive performance based on the receiver operating characteristics, analyzing the area under curve (AUC; Fielding and Bell, 1997). AUC assesses the discrimination ability of the models and its value ranges from 0.5 (equaling random distribution) to 1 (perfect prediction). AUC values >0.75 correspond to high discrimination performances (Fielding and Bell, 1997). As the registered grid cell occupancy depended on wolves present in a grid cell being registered, especially in highly mobile individuals, the result of the occupancy analysis might have been biased toward landscape features that correlate positively with observation frequency (e.g., sheep density that correlates with livestock kill rates), whereas spatial sampling biases was less of an issue for resident individuals that roam within the same few grid cells.
Livestock Depredation Rate
We analyzed depredation rate, using individual days as observation unit (1 = days on which a livestock depredation event by an individual wolf was recorded within a given grid cell versus 0 = days without livestock depredation). We ran generalized linear mixed models using the R package “spaMM,” with a binomial response distribution, and fitting the data with a Matérn correlation model (including the x and y coordinate of the top-right corner of each grid cell as autocorrelated random-slope term) to account for spatial autocorrelation (Rousset, 2017, 2021). To quantify (and test for) individual variation in livestock depredation rates, we included wolf ID as random effect. If certain individuals would have particularly high livestock depredation rates relative to the spatial, seasonal and social circumstances, they would appear as significant outliers. As fixed effects, we included the proportion of forest and heathland cover as predictors of wild ungulate densities, sheep density (all three measures were calculated on grid cell level), season (winter: December–February, spring: March–May, summer: June–August, fall: September–November) to test if wolf predation on livestock changes seasonally, sex, and social status (Supplementary Table 4). Initially, we also included the two-way interactions of social status with the proportion of forest and heathland cover and with sheep density, to test if depredation rates differ between residents and non-residents depending on land cover and livestock prey availability. However, we could not achieve model convergence including the interactions and thus ran separate models for resident and non-resident wolves (Supplementary Table 4). There was no collinearity among predictor variables in any model (Supplementary Figure 2). We scaled all numeric variables (mean = 0; standard deviation = 1) to obtain comparable estimates. We again created all possible combinations of fixed effects, including candidate models based on biological hypotheses, and selected the most parsimonious model based on AICc (Supplementary Table 4).
Additionally, we created a categorical variable describing livestock protection measures. This included three levels: (1) SH, where -- apart from the district in the very southeast -- no funds for preventative livestock protection measures were available until March 2019, (2) no protection measures in DK, and (3) protection measures in place in DK. The protection measures in DK were governmental initiatives to prevent wolf attacks on sheep, typically implemented by predator-proof fences (at least 110 cm high to prevent wolves from entering enclosures) after repeated attacks from resident wolves inside ‘‘wolf management zones’’7. Because livestock protection measures were usually implemented after the establishment of a wolf pair/pack, the protection measures and wolf social status were highly correlated (Pearson correlation = 0.87; Supplementary Figure 2). Consequently, we excluded this variable from the above analysis, but describe the daily depredation rate by wolves before versus after the establishment of the wolf management zone for the subset of wolves whose ranges intersected a wolf management zone in DK. The zone was initially established on 330 km2 in February 2017 and then expanded to 1,730 km2 in April 2021 (another zone was established in 2019, but there were only three wolf observations in the area and no recorded livestock attacks).
Results
Occupancy Patterns
Over the 10-year period from 2012 to 2021, 43 wolves (29 males and 14 females) were identified by genotyping (from 1,793 confirmed observations) in the Jutland peninsula. The number of wolves and wolf observations in the region gradually increased from 2012 to 2021 (Supplementary Figure 3). Of the males, 11 were members of a pair or pack, seven were single residents, and 26 were non-resident (the social status of 12 individuals changed during the study period), and of the females, eight were members of a pair or pack, four were single residents, and eight were non-resident (the social status of four individuals changed). Moreover, 30 individuals were immigrants from Central Europe and 13 were born in DK (7 in 2017, 6 in 2019). Non-residents accounted for 586 observations (503 in SH and 83 in DK), single residents for 381 (97 in SH and 284 in DK), and members of pairs/packs for 826 observations (all in DK; Figure 1).
Resident wolves were observed in 63 grid cells (pairs and packs were observed in 10 grid cells, 2% of the study area, and single residents in 60 grid cells, 10% of the study area). Grid cell occupancy by residents was positively associated with increasing forest (estimate ± SE: 0.24 ± 0.06; 95% confidence interval: 0.12–0.35) and heathland cover (estimate ± SE: 0.22 ± 0.06; 95% confidence interval: 0.09–0.34; Figure 2 and Supplementary Table 3). Human population density, road density, and sheep density were not included in the best model and uninformative in the full model. Based on a 50% predicted probability of resident wolf occurrence in a given grid cell (during the entire study period), 15.9% of the study area was predicted to be suitable for the establishment of resident wolves. Non-resident wolves were observed in 143 grid cells (24% of the study area), and their grid cell occupancy was positively associated with an increasing proportion of forest cover (estimate ± SE: 0.16 ± 0.04; 95% confidence interval: 0.08–0.23) and increasing sheep density (estimate ± SE: 0.24 ± 0.05; 95% confidence interval: 0.14–0.34; Figure 2 and Supplementary Table 3). The proportion of heathland cover, human population density, and road density were not included in the best model and uninformative in the full model.
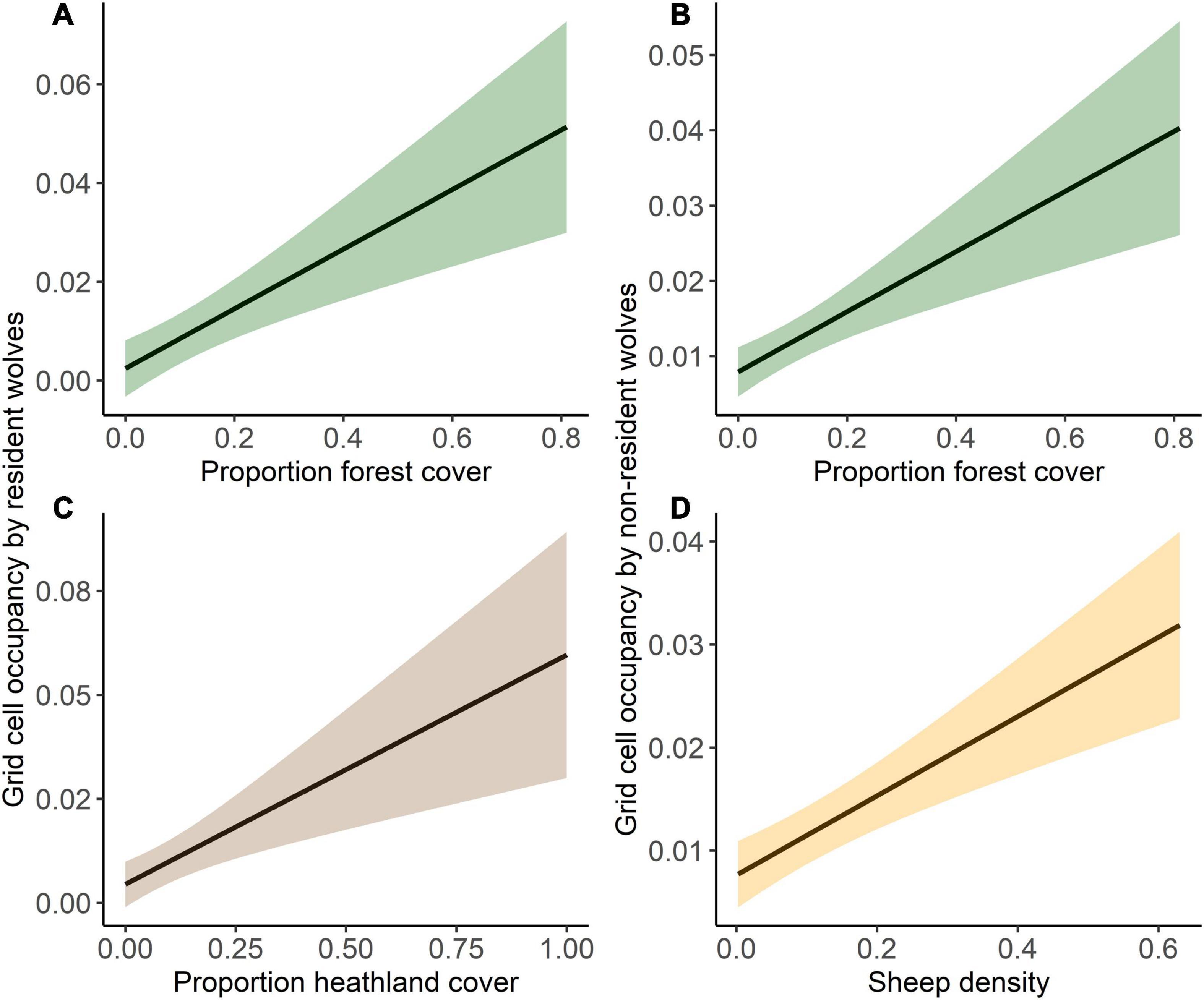
Figure 2. Predicted grid cell occupancy by resident wolves (left panel) in relation to (A) forest cover, and (C) heathland cover, and by non-resident wolves (right panel) in relation to (B) forest cover, and (D) sheep density. 95% confidence intervals are given as shading.
Livestock Depredation Patterns
From 2012 to August 2021, 85 livestock depredation events from DK and 480 from SH were attributed to wolves (Table 1). In DK, 88% of the depredation events were on sheep, with an average of 5.4 ± 6.5 (SD) individuals killed per depredation event. In SH, sheep comprised 98% of all livestock attacks (the number of individuals killed per attack was not consistently reported). The 565 livestock depredation events occurred in 99 grid cells (16% of the total area; Figure 3), with 337 events (60%) located within 16 grid cells (13 in SH, 3 in DK) with >10 livestock depredation events each.
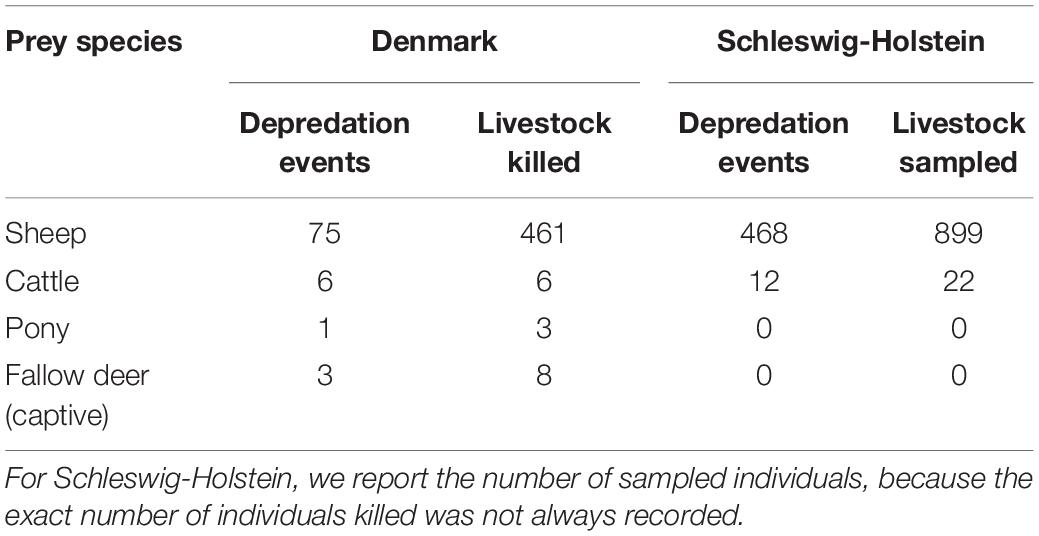
Table 1. The number of confirmed livestock depredation events and number of individuals killed (Denmark) or sampled (Schleswig-Holstein) by wolves between 2012 and 2021.
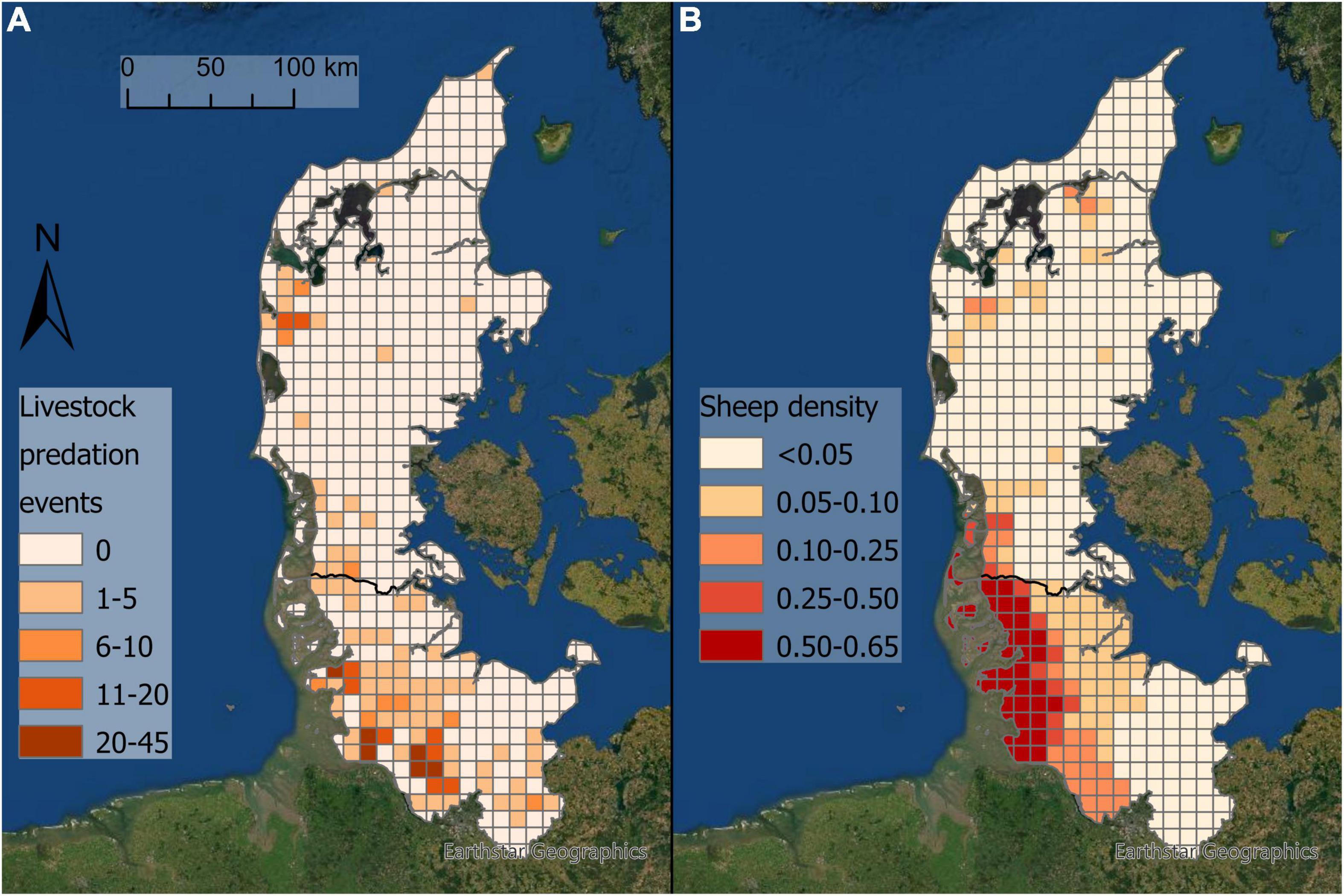
Figure 3. (A) Distribution of 565 livestock depredation events in Jutland peninsula attributed to wolves by state authorities, 2013–August 2021. (B) The sheep density (number of individuals per ha) per grid cell in Jutland peninsula. The black line represents the border between Denmark in the north and Schleswig-Holstein in the south.
From January 2017 to August 2021 (when depredation events were systematically reported), we could assign 234 depredation events (55 in DK and 179 in SH) to 25 individual wolves based on DNA evidence (out of a total of 37 individually identified wolves). Of these depredation events, 25 were caused by pair or pack members (all in DK), 78 by single residents (17 in DK, 61 in SH), and 137 by non-residents (13 in DK, 118 in SH; Table 2). Three male siblings (GW900m, GW924m, and GW932m), born in DK in 2017, were responsible for 108 (46%) of the depredation events assigned to individuals. All three individuals dispersed to SH in 2018 where they stayed for 3–16 months before either dispersing out of the state (GW900m: 19 January–22 March 2019, 19 livestock attacks during this period; GW924m: 8 July 2018–21 October 2019, 64 attacks) or disappearing (GW932m: 6 May–27 August 2018, 17 attacks). GW924m was categorized as single resident after establishing in a fixed area in SH, whereas the other two individuals were categorized non-residents. After 10 months in SH, wolf GW924m was categorized as a “problem wolf” by the federal state office for nature conservation after it had overcome predator-proof fences several times. Despite a shooting permit being issued, the wolf lived on in SH for additional 6 months before dispersing south, where it died in a traffic collision in Niedersachsen 3 months later.

Table 2. The number of individual wolf observation days (of identified individuals), livestock depredation events (number, events per day, events per year), and number of individuals from January 2017 to August 2021 separately for non-residents, single residents, and pairs/packs in Denmark and Schleswig-Holstein.
On average, individual wolves were registered killing livestock every 0.005 ± 0.007 (SD) days in DK compared to every 0.136 ± 0.135 days in SH. In other words, wolves were recorded to predate on livestock on average 1.8 times per year in DK and 49.6 times per year in SH, corresponding to a 27 times higher individual livestock depredation rate in SH than in DK. Moreover, livestock depredation rates differed among individuals of different social status, being highest for non-residents in SH and lowest for pairs/packs in DK (Table 2). The aforementioned three individuals (GW900m, GW924m, and GW932m) were registered killing livestock every 0.21, 0.13, and 0.12 days, respectively, once they had dispersed to SH.
The probability of wolf depredation on livestock (on a given observation day) was best explained by sex (lower in females, but uninformative), season (highest in winter and lowest in summer), proportion of heathland (negative correlation), proportion of forest (negative uninformative correlation), and sheep density (positive uninformative correlation) (Table 3 and Supplementary Table 4). Social status (resident versus non-resident) was not included in the best model and uninformative in the full model, indicating that after accounting for the land cover variables, sheep density, and spatial autocorrelation, there was no statistical difference between predation rates of resident and non-resident wolves. The estimated random effect (wolf ID) differed significantly from zero for six individuals (Figure 4). Two individuals had lower depredation rates than expected by the fixed effects and four had higher depredation rates (including the officially declared “problem wolf” GW924m), with the latter four wolves accounting for 96 (41%) of all livestock depredation events. The random effects of GW924m’s two siblings did not significantly differ from zero (Figure 4). Depredation rates by resident wolves were better predicted by our analysis (AUC: 0.93) than depredation rates by non-residents (AUC: 0.85). The probability of resident wolf depredation on livestock was lowest in summer (though seasonal differences were generally small), decreased with increasing proportion of heathland, and increased with sheep density (Table 3, Supplementary Table 4, and Figure 5). Proportion of forest was included in the best model, but uninformative (Table 3 and Supplementary Table 4), and sex was not included in the best model and uninformative in the full model. The probability of non-resident wolf depredation on livestock was best explained by season only, but this effect was uninformative (Table 3, Supplementary Table 4, and Figure 5). The proportion of heathland and forest, sheep density, and sex were not included in the best model and uninformative in the full model.
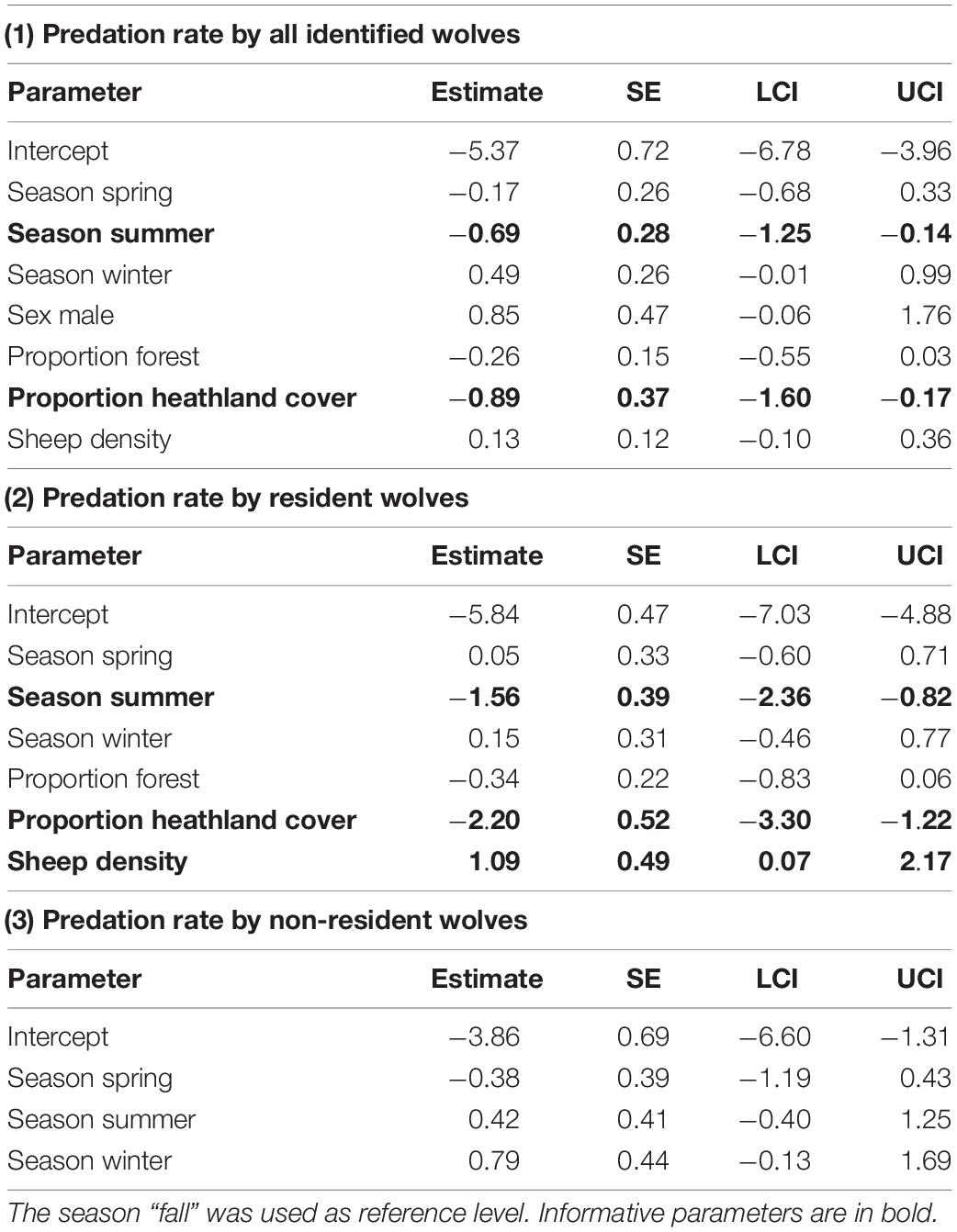
Table 3. The estimate, standard error (SE), lower (LCI), and upper (UCI) 95% confidence interval for the analysis of wolf predation rate, separately for (1) all individually identified wolves, (2) resident wolves, and (3) non-resident wolves.
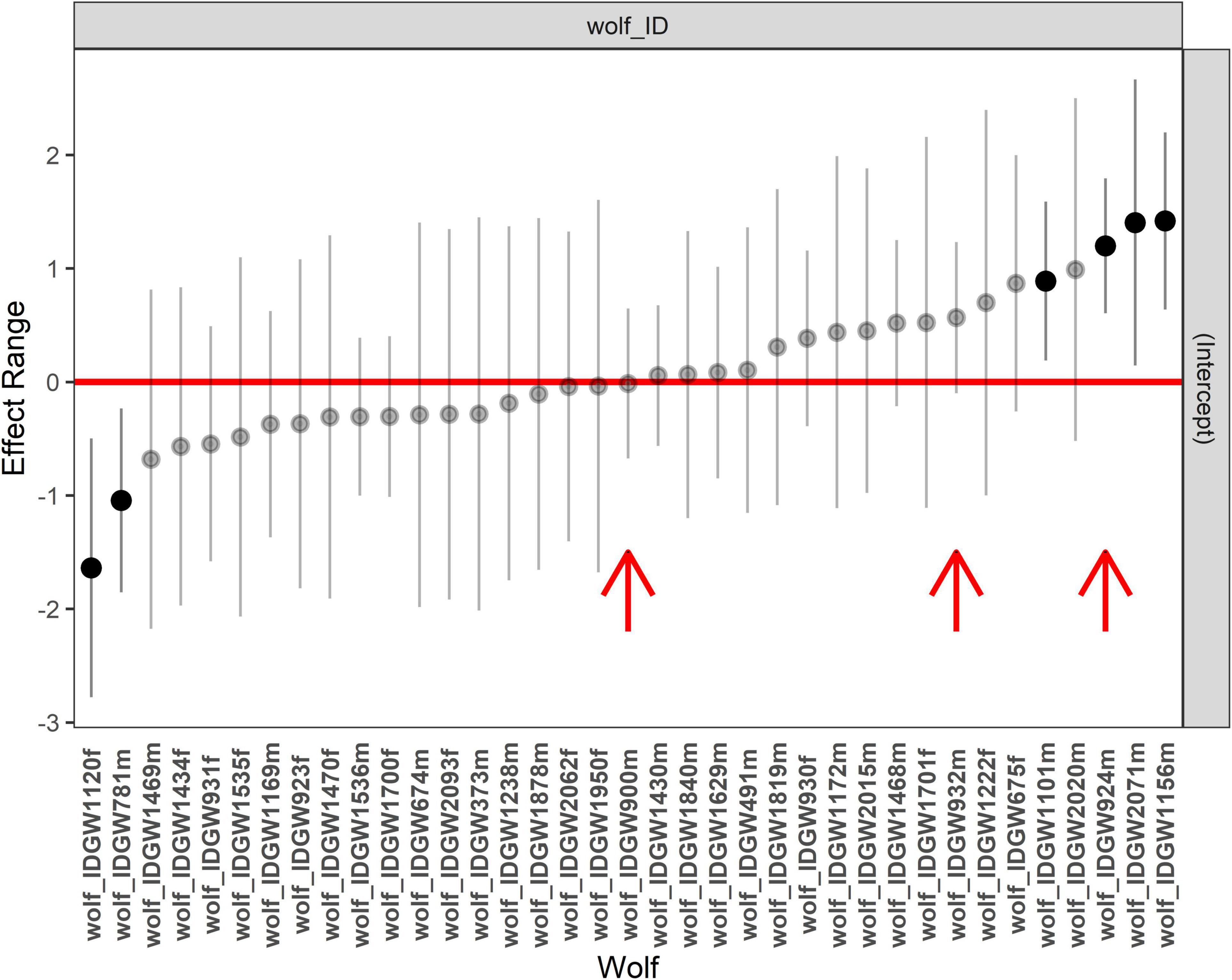
Figure 4. Estimated random effect (of each individual wolf) of the model describing the daily livestock depredation rates by individual wolves in Jutland peninsula (landscape and season as fixed effects). The red arrows indicate the three male siblings that contributed 45% of all livestock attacks. Mean estimates are shown as dots, and 95% confidence intervals as bars. Intervals that do not include zero are in bold.
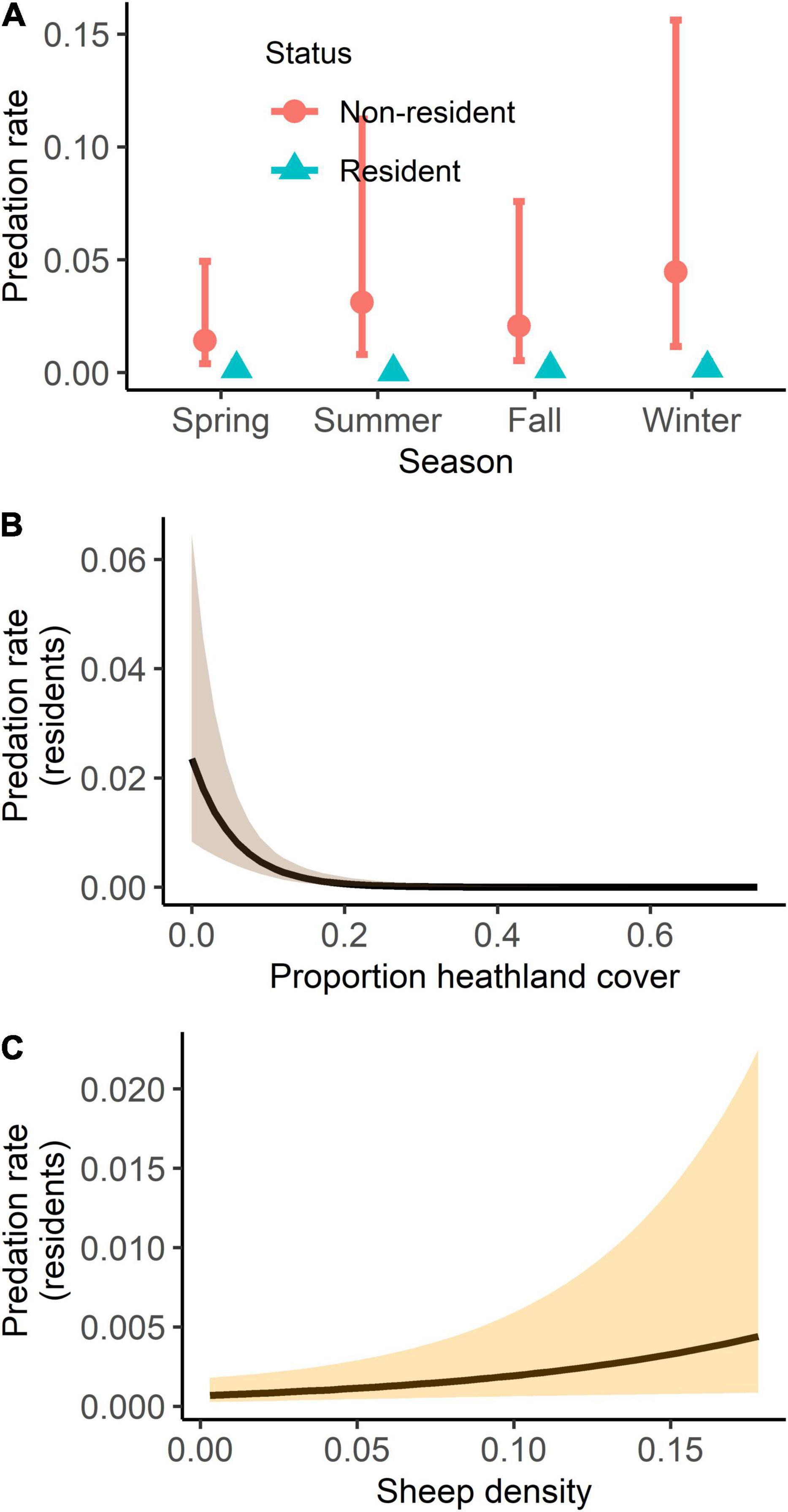
Figure 5. Predicted probability of daily depredation events by individual wolves on livestock in relation to (A) season, shown separately for resident and non-resident wolves. Moreover, the predicted probability of daily depredation events by resident wolves on livestock in relation to the (B) proportion of heathland cover and (C) sheep density. Mean estimates are shown as dots (A) and lines (B,C), and 95% confidence intervals as bars (A) and shading (B,C).
In the area where a wolf management zone was established in 2017 (and expanded in 2021), 17 livestock depredation events were assigned to identified wolves (all members of a pair or pack). Daily depredation rate decreased from 0.009 (two depredation events during 220 wolf days) before the establishment of the zone to 0.002 (15 depredation events during 7175 wolf days) after the zone had been established (estimate ± SD: −3.88 ± 1.39; 95% confidence interval: −6.59; −1.16). Within the wolf management zone, no attacks were registered within intact and functional predator-proof fences to date.
Discussion
Our data suggest that resident wolves in Jutland settled in habitats with high forest and heathland cover (habitat mostly found in DK), whereas grid cell occupancy by non-residents was positively associated with sheep density and forest cover. Further, resident wolves killed fewer livestock per day than non-residents. This was likely related to dispersers occurring in a much wider geographical area with a broader range of landscape types and more often in the southern part of the peninsula, where sheep are more abundant and thus available as prey. Importantly, the variation in individual livestock predation rates was largely related to spatial patterns of wolf occurrence and environmental settings, and less to individual variation and social status, suggesting that livestock depredation was generally context dependent rather than the result of personality differences (such as individuals selecting for livestock). Differences in land cover and sheep density explain why the total number of livestock attacks was almost 6-fold higher in SH than in DK, despite ca. four times more wolf observation days in DK compared to SH. Hence, so far livestock damage inflicted by residents appears to be manageable, which might also be related to the introduction of governmentally supported livestock protection initiatives in areas where wolf pairs/packs established (Gervasi et al., 2021; Oliveira et al., 2021), whereas dispersing individuals pose a greater challenge. Below, we discuss how our findings can be used to predict the future expansion of wolves and to manage livestock depredation in cultivated landscapes.
Occurrence Patterns
Overall, wolves were more likely to occur in grid cells with high forest cover. The pattern of resident wolves selecting grid cells with high coverage of forest and heathland was in accordance with habitat selection patterns from other European countries (Jędrzejewski et al., 2008; Fechter and Storch, 2014; Nowak et al., 2017; Grilo et al., 2019). Forest is positively associated with wild ungulates (Borowik et al., 2013), the main prey of wolves in Central Europe, which explains why wolf grid cell occupancy increased with forest and heathland cover (Sunde and Olsen, 2018; Roder et al., 2020). Our model predicted that about 16% of the region’s grid cells, almost all of them positioned in DK, could be considered suitable for wolf establishment. Lack of suitable habitat in SH explains why so few individuals stayed resident in SH, and in such cases not permanently. However, once higher quality habitats are occupied, wolves might nevertheless establish territories in lower quality habitats (Nowak et al., 2017).
Our models performed slightly worse in explaining grid cell occupancy by non-resident wolves compared to residents, suggesting that predicting the occurrence of dispersing wolves is challenging because they often traverse landscapes unsuitable for settlement (Blanco and Cortés, 2007). From a methodological perspective, we argue that the occurrence patterns of resident wolves provide a reliable representation of their true occupancy in the study area, as resident wolves were usually registered multiple times within each 3-month period. Moreover, at least in DK where the majority of the resident wolves were registered, resident wolves were monitored actively, independently of passive registration based on livestock kills (in DK 7% of all wolf observations came from livestock depredation events). In comparison, monitoring of non-resident wolves was more prone to be biased toward areas with high livestock densities where the per capita livestock depredation rate is higher. This is especially true for SH, where >90% of wolf observations came from livestock depredation events. In this light, the positive association between non-resident wolf occupancy and sheep density may reflect disproportionately high sampling frequency of highly mobile dispersers in quadrates with high sheep density (where the probability of killing sheep as opposed to wild prey is higher), rather than dispersers being attracted to areas with high sheep density.
Livestock Depredation
As only 64% (DK) and 39% (SH) of genetically registered livestock depredation events from 2017 to 2020 could be assigned to wolf individuals, and not all livestock attacks may be sampled genetically, the depredation rates estimated from individually assigned kills likely underestimate true depredation rates. Livestock kills without a genetically assigned predator individual could in theory also be caused by undetected wolves that lived in the region before they were registered, hence underestimating the number of wolf observation days and consequently overestimating individual depredation rates. However, we consider this potential bias rather small due to the high observation frequency and detection rate of wolves in the region (Sunde et al., 2021).
Despite DK harboring >80% of the wolves in the region, measured in wolf observation days, the total number of livestock attacks in SH was six times higher than in DK. The annual livestock depredation rate of wolves estimated for DK (approximately two depredation events resulting in eight dead animals per wolf per year if estimated from assigned kills) was slightly above the median for European countries at roughly ∼5 kills per wolf per year (Linnell and Cretois, 2018). In contrast, the annual depredation rates in SH of approx. 50 livestock depredations per wolf per year in our study period based on individually assigned livestock kills exceed the highest reported livestock depredation rate from any European country (Norway: 34 sheep and goats killed per wolf per year 2012–2016) (Linnell and Cretois, 2018).
Our analysis suggests that differences in depredation rates were mainly attributed to spatial differences where residents and non-residents occurred, i.e., related to land cover (more forest and heathland in DK) and sheep density (more sheep in SH), than to social status per se. Lower depredation rates in DK were likely also related to the implementation of local protective measures (predator-proof fences) after which livestock depredation decreased. This result is in line with previous findings that predator-proof fences can be a successful livestock protection measure in areas were wolf pairs or packs establish (Reinhardt et al., 2011; Gervasi et al., 2021; Oliveira et al., 2021), although our data have to be taken cautiously due to the limited sample size. Livestock depredation by wolves decreased with increasing heathland, but surprisingly not forest. Areas that sustain wild ungulate prey, like heathland and forest (Kuiters and Slim, 2002; Borowik et al., 2013), are generally selected by wolves (Lesmerises et al., 2012; Milleret et al., 2019). It is therefore conceivable that availability of heathland buffered livestock attacks because the proportion of time wolves spent in these habitats correlated positively with their availability. Conversely, areas with very low coverage of forest and heathland likely forced wolves to cross open land with sheep pastures more often than would be the case in areas with more (semi)natural land cover. This is especially true for dispersers attempting to establish a territory, thereby roaming over large areas. The different depredation rates among individuals of different social status (being highest in non-residents and lowest for members of pairs/packs) were largely explained by differences in where residents and non-residents occur. Moreover, we cannot exclude the possibility that lack of familiarity with an area might influence the propensity of non-residents to attack livestock, as shown in other areas and species (Mizutani, 1993; Linnell et al., 1999). Independent of the proximate mechanism, increased predation on livestock in areas with reduced availability of natural prey and increased availability of livestock was also shown in other areas (Suryawanshi et al., 2013; Imbert et al., 2016). We have no clear explanation why livestock depredation rates by resident wolves were higher during winter and lower during summer, but it might relate to prey switching related to the presence of dependent offspring. These seasonal patterns contrast with other studies that found increased livestock depredation during summer (Bradley et al., 2015) and fall (Iliopoulos et al., 2009), respectively, and might be caused by geographic differences in seasonal variation of wild and livestock prey availability.
Even though three individuals were responsible for 45% of the livestock depredations, only for one of them (GW900m), the number of kills per day was more than 10% higher than the average for all wolves in the region and its residual predation rate (adjusted for landscape context) did not differ from the average for all wolves. Hence, the total number of livestock killed by these individuals was mainly caused by the time they stayed in SH. Even though the individual declared as problem wolf (GW924m) had a residual predation rate significantly above average, it was only ranked third in residual predation rate of all individuals in the analysis. This result suggests that even though some individual variation is identifiable in our model, individual preferences appeared to explain a minor part of individual livestock depredation rates compared to the effect of landscape context with no clearly identified outliers that could be ubiquitously assessed as “problem individuals” that were particularly prone to kill livestock [type-II problem individuals following the terminology by Linnell et al. (1999)].
Accordingly, from a management perspective, at least in this study area, removal of identified “problem individuals” would not (or only marginally) reduce local livestock depredation rates more than removal of any other wolf in the same place.
Conclusion
Our results indicate that the wolves in Jutland mostly killed livestock as a context-dependent response, i.e., being dispersers in agricultural areas with low availability of wild ungulate prey and high livestock densities, and not because of behavioral preferences for sheep. Consequently, the removal of so called “problem individuals” likely will not be a viable long-term solution to reduce local livestock depredation rates. From a technical perspective, the incidence that GW924m lived on for 6 month in SH after a shooting permit was issued before finally dispersing from the region, also indicates that targeted lethal management efforts is an inefficient tool to reduce livestock depredation in cultivated landscapes. We conclude that while wolf attacks on livestock in established permanent wolf territories generally can be prevented through improvement of predator-proof fences, livestock depredation by vagrants in pastoral areas where wolves do not settle permanently constitutes a bigger challenge. As the number of vagrant wolves in pastoral habitats like western Schleswig-Holstein is linearly related to the number of wolf packs in the Central European wolf population, a number that is still increasing, depredation rates are likely to increase further in the coming years. Thus, if reducing livestock depredation rates of wolves is a management goal, preventive livestock protection measures, such as predator-proof fences, should be considered in areas where frequent wolf occurrence is likely.
Data Availability Statement
The original contributions presented in the study are included in the article/Supplementary Material. The data that support the findings of this study are openly available at http://doi.org/10.13140/RG.2.2.16625.61289.
Ethics Statement
Ethical review and approval was not required for the animal study because the search for and sampling of genetic material from wolves involved non-intrusive methods that did not affect the sampled subjects. Active monitoring efforts at all times followed the stringent procedures and obligations imposed by the states’ laws and regulations for activities on public and private land.
Author Contributions
PS achieved the funding. PS, MM, and KO conceived the ideas. MM analyzed the data. MM and PS led the writing of the manuscript. JM and BS were coordinating and conducting wolf monitoring in Schleswig-Holstein. KO, CV-S, and PS were responsible for the monitoring in Denmark. CN was responsible for genetic analyses of samples from Germany and partly from Denmark and organized the register of genotyped wolves in Central Europe. MH and PT were responsible for genetic analyses in Denmark since 2017. All authors provided input to the manuscript and its revised versions.
Conflict of Interest
The authors declare that the research was conducted in the absence of any commercial or financial relationships that could be construed as a potential conflict of interest.
Publisher’s Note
All claims expressed in this article are solely those of the authors and do not necessarily represent those of their affiliated organizations, or those of the publisher, the editors and the reviewers. Any product that may be evaluated in this article, or claim that may be made by its manufacturer, is not guaranteed or endorsed by the publisher.
Acknowledgments
We are grateful to the many dedicated volunteers and officials in Germany and Denmark who assisted with the practical wolf monitoring and S. Collet for analysis of genetic data. We thank The Danish Environmental Protection Agency for funding wolf monitoring in DK. The analysis and writing of this manuscript was possible thanks to a donation from 15. Juni Fonden. We also thank Stotra Chakrabarti, Jennifer Lesley Silcock, Ellen Candler, and one anonymous reviewer for their constructive feedback on our manuscript, and Simon Linke for pointing out shortcomings in the review system of the Frontiers journals that hopefully help to improve the system in the future.
Supplementary Material
The Supplementary Material for this article can be found online at: https://www.frontiersin.org/articles/10.3389/fevo.2022.783027/full#supplementary-material
Footnotes
- ^ https://land.copernicus.eu/pan-european/corine-land-cover/clc2018?tab=download
- ^ https://www.schleswig-holstein.de/DE/Fachinhalte/B/biotope/Downloads/kartierschluessel.pdf?__blob=publicationFile&v=2
- ^ https://download.geofabrik.de/europe
- ^ https://sedac.ciesin.columbia.edu/data/set/gpw-v4-population-density-adjusted-to-2015-unwpp-country-totals-rev11/data-download
- ^ https://chr.fvst.dk
- ^ https://www.statistik-nord.de
- ^ https://fvm.dk/nyheder/nyhed/nyhed/miljoeminister-praesenterer-nye-tiltag-for-at-forebygge-ulveangreb/
References
Andersen, L. W., Harms, V., Caniglia, R., Czarnomska, S. D., Fabbri, E., Jedrzejewska, B., et al. (2015). Long-distance dispersal of a wolf, Canis lupus, in northwestern Europe. Mamm. Res. 60, 163–168. doi: 10.1007/s13364-015-0220-6
Araújo, M. S., Bolnick, D. I., and Layman, C. A. (2011). The ecological causes of individual specialisation. Ecol. Lett. 14, 948–958. doi: 10.1111/j.1461-0248.2011.01662.x
Arnold, T. W. (2010). Uninformative parameters and model selection using Akaike’s information criterion. J. Wildlife Manag. 74, 1175–1178. doi: 10.1371/journal.pone.0206711
Bautista, C., Revilla, E., Naves, J., Albrecht, J., Fernández, N., Olszańska, A., et al. (2019). Large carnivore damage in Europe: analysis of compensation and prevention programs. Biol. Conserv. 235, 308–316. doi: 10.1016/j.biocon.2019.04.019
Beale, C. M., Lennon, J. J., Yearsley, J. M., Brewer, M. J., and Elston, D. A. (2010). Regression analysis of spatial data. Ecol. Lett. 13, 246–264.
Blanco, J., and Cortés, Y. (2007). Dispersal patterns, social structure and mortality of wolves living in agricultural habitats in Spain. J. Zool. 273, 114–124. doi: 10.1111/j.1469-7998.2007.00305.x
Boitani, L., and Linnell, J. D. (2015). “Bringing large mammals back: large carnivores in Europe,” in Rewilding European Landscapes, eds H. M. Pereira and L. M. Navarro (Cham: Springer), 67–84. doi: 10.1007/978-3-319-12039-3_4
Borowik, T., Cornulier, T., and Jędrzejewska, B. (2013). Environmental factors shaping ungulate abundances in Poland. Acta Theriol. 58, 403–413. doi: 10.1007/s13364-013-0153-x
Bradley, E. H., Robinson, H. S., Bangs, E. E., Kunkel, K., Jimenez, M. D., Gude, J. A., et al. (2015). Effects of wolf removal on livestock depredation recurrence and wolf recovery in Montana, Idaho, and Wyoming. J. Wildlife Manag. 79, 1337–1346. doi: 10.1002/jwmg.948
Chapron, G., Kaczensky, P., Linnell, J. D., Von Arx, M., Huber, D., Andrén, H., et al. (2014). Recovery of large carnivores in Europe’s modern human-dominated landscapes. Science 346, 1517–1519. doi: 10.1126/science.1257553
Cimatti, M., Ranc, N., Benítez-López, A., Maiorano, L., Boitani, L., Cagnacci, F., et al. (2021). Large carnivore expansion in Europe is associated with human population density and land cover changes. Divers. Distrib. 27, 602–617. doi: 10.1111/ddi.13219
Dickman, C. R., and Newsome, T. M. (2015). Individual hunting behaviour and prey specialisation in the house cat Felis catus: implications for conservation and management. Appl. Anim. Behav. Sci. 173, 76–87. doi: 10.1016/j.applanim.2014.09.021
Dressel, S., Sandström, C., and Ericsson, G. (2015). A meta-analysis of studies on attitudes toward bears and wolves across Europe 1976–2012. Conserv. Biol. 29, 565–574. doi: 10.1111/cobi.12420
Eklund, A., López-Bao, J. V., Tourani, M., Chapron, G., and Frank, J. (2017). Limited evidence on the effectiveness of interventions to reduce livestock predation by large carnivores. Sci. Rep. 7, 1–9.
Ericsson, G., and Heberlein, T. A. (2003). Attitudes of hunters, locals, and the general public in Sweden now that the wolves are back. Biol. Conserv. 111, 149–159. doi: 10.1016/s0006-3207(02)00258-6
Eriksson, M., Sandström, C., and Ericsson, G. (2015). Direct experience and attitude change towards bears and wolves. Wildlife Biol. 21, 131–137. doi: 10.3390/ani11061735
Fechter, D., and Storch, I. (2014). How many wolves (Canis lupus) fit into Germany? The role of assumptions in predictive rule-based habitat models for habitat generalists. PLoS One 9:e101798. doi: 10.1371/journal.pone.0101798
Fielding, A. H., and Bell, J. F. (1997). A review of methods for the assessment of prediction errors in conservation presence/absence models. Environ. Conserv. 24, 38–49. doi: 10.1017/s0376892997000088
Gazzola, A., Capitani, C., Mattioli, L., and Apollonio, M. (2008). Livestock damage and wolf presence. J. Zool. 274, 261–269. doi: 10.1111/j.1469-7998.2007.00381.x
Gervasi, V., Linnell, J. D. C., Berce, T., Boitani, L., Cerne, R., Ciucci, P., et al. (2021). Ecological correlates of large carnivore depredation on sheep in Europe. Glob. Ecol. Conserv. 30:e01798. doi: 10.1016/j.gecco.2021.e01798
Grilo, C., Lucas, P. M., Fernández-Gil, A., Seara, M., Costa, G., Roque, S., et al. (2019). Refuge as major habitat driver for wolf presence in human-modified landscapes. Anim. Conserv. 22, 59–71. doi: 10.1111/acv.12435
Iliopoulos, Y., Sgardelis, S., Koutis, V., and Savaris, D. (2009). Wolf depredation on livestock in central Greece. Mamm. Res. 54, 11–22. doi: 10.1007/bf03193133
Imbert, C., Caniglia, R., Fabbri, E., Milanesi, P., Randi, E., Serafini, M., et al. (2016). Why do wolves eat livestock?: factors influencing wolf diet in northern Italy. Biol. Conserv. 195, 156–168. doi: 10.1016/j.biocon.2016.01.003
Jędrzejewski, W., Jędrzejewska, B., Zawadzka, B., Borowik, T., Nowak, S., and Mysłajek, R. (2008). Habitat suitability model for Polish wolves based on long-term national census. Anim. Conserv. 11, 377–390. doi: 10.1111/j.1469-1795.2008.00193.x
Kuiters, A., and Slim, P. (2002). Regeneration of mixed deciduous forest in a Dutch forest-heathland, following a reduction of ungulate densities. Biol. Conserv. 105, 65–74. doi: 10.1016/s0006-3207(01)00204-x
Lesmerises, F., Dussault, C., and St-Laurent, M.-H. (2012). Wolf habitat selection is shaped by human activities in a highly managed boreal forest. For. Ecol. Manag. 276, 125–131. doi: 10.1016/j.foreco.2012.03.025
Linnell, J. (2013). From Conflict to Coexistence: Insights from Multi-Disciplinary Research into the Relationships Between People, Large Carnivores and Institutions. Rome: Istituto di Ecologia Applicata.
Linnell, J. D., and Cretois, B. (2018). The Revival of Wolves and Other Large Predators and Its Impact on Farmers and Their Livelihood in Rural Regions of Europe. Brussels: European Parliament’s Committee on Agriculture and Rural Development.
Linnell, J. D., Odden, J., Smith, M. E., Aanes, R., and Swenson, J. E. (1999). Large carnivores that kill livestock: do “problem individuals” really exist. Wildlife Soc. Bull. 27, 698–705.
Milanesi, P., Breiner, F. T., Puopolo, F., and Holderegger, R. (2017). European human-dominated landscapes provide ample space for the recolonization of large carnivore populations under future land change scenarios. Ecography 40, 1359–1368. doi: 10.1111/ecog.02223
Milleret, C., Ordiz, A., Sanz-Pérez, A., Uzal, A., Carricondo-Sanchez, D., Eriksen, A., et al. (2019). Testing the influence of habitat experienced during the natal phase on habitat selection later in life in Scandinavian wolves. Sci. Rep. 9, 1–11. doi: 10.1038/s41598-019-42835-1
Mizutani, F. (1993). “Home range of leopards and their impact on livestock on Kenyan ranches,” in Proceedings of the Symposia of the Zoological Society of London, London, 425–439.
Morehouse, A. T., Graves, T. A., Mikle, N., and Boyce, M. S. (2016). Nature vs. nurture: evidence for social learning of conflict behaviour in grizzly bears. PLoS One 11:e0165425. doi: 10.1371/journal.pone.0165425
Nowak, S., and Myslajek, R. W. (2016). Wolf recovery and population dynamics in Western Poland, 2001-2012. Mamm. Res. 61, 83–98. doi: 10.1007/s13364-016-0263-3
Nowak, S., Myslajek, R. W., Szewczyk, M., Tomczak, P., Borowik, T., and Jedrzejewska, B. (2017). Sedentary but not dispersing wolves Canis lupus recolonizing western Poland (2001-2016) conform to the predictions of a habitat suitability model. Divers. Distrib. 23, 1353–1364.
Oliveira, T., Treves, A., ópez-Bao, J. V. L., and Krofel, M. (2021). The contribution of the LIFE program to mitigating damages caused by large carnivores in Europe. Glob. Ecol. Conserv. 31:e01815.
Pinheiro, J., Bates, D., DebRoy, S., Sarkar, D., Heisterkamp, S., Van Willigen, B., et al. (2017). Package ‘nlme’. Linear and Nonlinear Mixed Effects Models, Version 3.
Reinhardt, I., Kluth, G., Nowak, C., Szentiks, C. A., Krone, O., Ansorge, H., et al. (2019). Military training areas facilitate the recolonization of wolves in Germany. Conserv. Lett. 12:e12635.
Reinhardt, I., Kluth, G., Pierużek-Nowak, S., and Mysłajek, R. W. (2015). Standards for the Monitoring of the Central European Wolf Population in Germany and Poland. Bonn: BfN Federal Agency for Nature Conservation.
Reinhardt, I., Rauer, G., Kluth, G., Kaczensky, P., Knauer, F., and Wotschikowsky, U. (2011). Livestock protection methods applicable for Germany–a Country newly recolonized by wolves. Hystrix Ital. J. Mammal. 23, 62–72.
Roder, S., Biollaz, F., Mettaz, S., Zimmermann, F., Manz, R., Kéry, M., et al. (2020). Deer density drives habitat use of establishing wolves in the Western European Alps. J. Appl. Ecol. 57, 995–1008.
Rousset, M. F. (2021). Package ‘spaMM’ Version 3.9.25. Available online at: https://cran.r-project.org/web/packages/spaMM/spaMM.pdf
Sunde, P., Collet, S., Nowak, C., Thomsen, P. F., Hansen, M. M., Schulz, B., et al. (2021). Where have all the young wolves gone? Traffic and cryptic mortality create a wolf population sink in Denmark and northernmost Germany. Conserv. Lett. 14:e12812.
Sunde, P., and Olsen, K. (2018). Ulve (Canis lupus) i Danmark 2012-2017. Oversigt og Analyse af Tilgængelig Bestandsinformation. Aarhus: Aarhus Universitet.
Suryawanshi, K. R., Bhatnagar, Y. V., Redpath, S., and Mishra, C. (2013). People, predators and perceptions: patterns of livestock depredation by snow leopards and wolves. J. Appl. Ecol. 50, 550–560. doi: 10.1111/1365-2664.12061
Swan, G. J., Redpath, S. M., Bearhop, S., and McDonald, R. A. (2017). Ecology of problem individuals and the efficacy of selective wildlife management. Trends Ecol. Evol. 32, 518–530. doi: 10.1016/j.tree.2017.03.011
Thomsen, P. F., Hansen, M. M., Olsen, K., and Sunde, P. (2020). Genetiske Analysemetoder I Den Nationale Overvågning Af Ulv (Canis lupus) i Danmark. Aarhus: Aarhus Universitet.
Treves, A., Naughton-Treves, L., Harper, E. K., Mladenoff, D. J., Rose, R. A., Sickley, T. A., et al. (2004). Predicting human-carnivore conflict: a spatial model derived from 25 years of data on wolf predation on livestock. Conserv. Biol. 18, 114–125.
Wagenmakers, E.-J., and Farrell, S. (2004). AIC model selection using Akaike weights. Psychon. Bull. Rev. 11, 192–196. doi: 10.3758/bf03206482
Keywords: Canis lupus, human-wildlife conflicts, large carnivores, livestock protection, Ovis aries, predation, spatial ecology
Citation: Mayer M, Olsen K, Schulz B, Matzen J, Nowak C, Thomsen PF, Hansen MM, Vedel-Smith C and Sunde P (2022) Occurrence and Livestock Depredation Patterns by Wolves in Highly Cultivated Landscapes. Front. Ecol. Evol. 10:783027. doi: 10.3389/fevo.2022.783027
Received: 25 September 2021; Accepted: 20 January 2022;
Published: 21 February 2022.
Edited by:
Stotra Chakrabarti, Macalester College, United StatesReviewed by:
Jennifer Lesley Silcock, The University of Queensland, AustraliaEllen Candler, University of Minnesota Twin Cities, United States
Copyright © 2022 Mayer, Olsen, Schulz, Matzen, Nowak, Thomsen, Hansen, Vedel-Smith and Sunde. This is an open-access article distributed under the terms of the Creative Commons Attribution License (CC BY). The use, distribution or reproduction in other forums is permitted, provided the original author(s) and the copyright owner(s) are credited and that the original publication in this journal is cited, in accordance with accepted academic practice. No use, distribution or reproduction is permitted which does not comply with these terms.
*Correspondence: Martin Mayer, bWFydGluLm1heWVyQGVjb3MuYXUuZGs=
†These authors have contributed equally to this work