- 1Institute of Vertebrate Biology of the Czech Academy of Sciences, Brno, Czechia
- 2Department of Botany and Zoology, Faculty of Sciences, Masaryk University, Brno, Czechia
Ectothermic organisms respond to rapid environmental change through a combination of behavioral and physiological adjustments. As behavioral and physiological traits are often functionally linked, an effective ectotherm response to environmental perturbation will depend on the direction and magnitude of their association. The role of various modifiers in behavioral-physiological relationships remains largely unexplored. We applied a repeated-measures approach to examine the influence of body temperature and individual variation on the link between resting metabolic rate (RMR) and exploratory locomotor activity (ELA) in juvenile Alpine newts, Ichthyosaura alpestris. We analyzed trait relationships at two body temperatures separately and as parameters, intercepts and slopes, of thermal reaction norms for both traits. Body temperature affected the level of detectable among-individual variation in two different directions. Among-individual variation in ELA was detected at 12°C, while RMR was repeatable at 22°C. We found no support for a link between RMR and ELA at either temperature. While analysis of intercepts revealed among-individual variation in both traits, among-individual variation in slopes was detected in RMR only. Intercepts were positively associated at the individual, but not the whole-phenotypic, level. For ELA, the target of selection should be individual trait values across temperatures, rather than their thermal sensitivities. The positive association between intercepts of thermal reaction norms for ELA and RMR suggests that phenotypic selection acts on both traits in a correlated fashion. Measurements at one body temperature and within-individual variation hide the metabolic-behavioral relations. We conclude that correlative studies on flexible behavioral and physiological traits in ectotherms require repeated measurement at two or more body temperatures in order to avoid misleading results. This approach is needed to fully understand ectotherm responses to environmental change and its impact on their population dynamics.
Introduction
Ectothermic organisms respond to rapid environmental change through a combination of physiological and behavioral adjustments (Bennett and Huey, 1990; Angilletta et al., 2006; Williams et al., 2008; Huey et al., 2012). As these traits are often functionally linked (Careau et al., 2008; Biro and Stamps, 2010; Mathot and Dingemanse, 2015), an effective response to environmental change will depend on the magnitude and direction of their association. For example, metabolic rate fuels functions and processes underlying locomotion, while locomotor activity is involved in both energy expenditure (e.g., mate searching) and energy gain (e.g., foraging) (Mathot and Dingemanse, 2015). Accordingly, locomotor activity level contributes to the acquisition and allocation of energy into maintenance, growth, reproduction, and survival, and thus to individual fitness in a given habitat (Burger et al., 2021). Information on behavioral and physiological covariation in a thermal context is required to understand the mechanistic link between individual traits and population dynamics under environmental change (Johnston et al., 2019); however, this issue has received surprisingly limited attention.
Current theory predicts that the relationship between metabolic rate and locomotor activity will depend on how an organism manages its energy budget (Careau and Garland, 2012; Mathot and Dingemanse, 2015). Under the “allocation model,” which assumes a fixed daily energy expenditure, an individual with a high standard metabolic rate can invest less energy into locomotor activity than an individual with low maintenance costs, and vice versa. The “performance model,” on the other hand, assumes a positive relationship (slope > 1; Halsey et al., 2019) between maintenance metabolic rate and daily energy budget. Under this scenario, locomotor activity should be positively related to standard metabolic rate, irrespective of its influence on the energy budget. Finally, the “independent model” assumes no relationship between maintenance metabolic rate and energy available for other behavioral (e.g., locomotor activity) and physiological tasks (e.g., digestion) under non-restricted total energy expenditure. In this case, a positive relationship is predicted between energy-gaining locomotor activity and maintenance metabolic rate because of a positive association between maintenance metabolic rate and daily energy expenditure (Mathot and Dingemanse, 2015).
A recent meta-analysis revealed no relationship between resting metabolic rate (RMR) and locomotor activity across taxa (Mathot et al., 2019), which was interpreted as being the result of interspecific variation in net energy contribution of this behavioral trait to the total energy budget. However, there are at least two alternative explanations for this result in ectotherms. First, that metabolic-behavioral relationships are affected by environmental stress (e.g., temperature), which may mask trait covariation due to individual variation in the slope or shape of their thermal reaction norms (Killen et al., 2013). Second, trait covariation may be attenuated by low repeatability (i.e., the relative amount of among-individual variation) of one or both traits at a given body temperature. Unfortunately, most studies on metabolic-activity relationships have been performed at one body temperature only and have used phenotypic correlations, which assume thermal independence of the link, perfect repeatability (R = 1) of both traits, and/or identical directions for among- and within-individual correlation. However, available studies have shown that the metabolic-activity link will change with temperature in an endotherm (Chappell et al., 2004), that the average repeatability of behavioral and physiological traits is 0.4 (Bell et al., 2009; White et al., 2013; Holtmann et al., 2017) and that metabolic-behavioral trait correlations vary at different variation levels (Gifford et al., 2014; Videlier et al., 2019), which suggests that temperature and within-individual (residual) variation may attenuate or mask trait correlation at the whole-phenotypic level. The empirical support for this prediction is missing (Dingemanse and Dochtermann, 2013; Killen et al., 2013; Careau and Wilson, 2017).
In this study, we undertook repeated measurements of RMR (energy consumption of post-absorptive and non-active, but immature, individuals during their typical inactivity period) and exploratory locomotor activity (ELA; distance covered in a new open-field arena) in juvenile newts at two body temperatures in the autumn and the following spring. While both traits are repeatable and thermally dependent in newts (Gvoždík and Kristín, 2017; Baškiera and Gvoždík, 2019, 2021), their covariation level is unknown. A recent analysis of metabolic traits has provided support for the allocation model of energy management in newts (Baškiera and Gvoždík, 2020), implying that energy-consuming ELA should be negatively associated with RMR. However, among-individual variation in the slopes of their thermal reaction norms for ELA is negligible (Baškiera and Gvoždík, 2020), suggesting that the masking or attenuating effect of its within individual variation modifies the RMR-ELA relationship between temperatures. To demonstrate the joint effect of body temperature and among-individual variation on the association of RMR and ELA, we first examined trait relationships at two body temperatures in addition to the commonly used “single temperature” approach. As a next step, we analyzed thermal reaction norm parameters for these traits with respect to among- and within-individual variation.
Materials and Methods
Study Organisms and Maintenance
The alpine newt, Ichthyosaura alpestris, is a widespread European tailed amphibian with a body size up to 12 cm. Adults have an aquatic phase from April to June, and a terrestrial phase that lasts the rest of year. From November to April, the newts overwinter in underground shelters. Juveniles metamorphose in August and September and remain strictly terrestrial until maturity. Alpine newts are highly secretive and display mostly crepuscular and/or nocturnal activity. They feed on a wide range of invertebrate prey (Griffiths, 1996).
For this experiment, we used juvenile newts (n = 62) obtained from a large stock of newt larvae (clutches of 15 females) kept in outdoor tanks (n = 30) filled with non-chlorinated well water (90 × 63 × 47 cm; density = 30 ind. m2) under semi-natural conditions (surface water temperature = 16.6 ± 6.0 [SD]°C; light intensity = 6.44 ± 18.13 × 103 lx). Haphazardly chosen metamorphs were weighed to the nearest 0.001 g (KERN EG, Balingen, Germany) and placed in plastic tanks (40 × 26 × 18 cm; n = 4 per tank) in walk-in environmental rooms with a fluctuating temperature regime (night minimum–day maximum = 12–22°C) and a naturally changing light:dark period (16:8–8:16; light intensity = 300 lx). This thermal regime was chosen to match the newts’ preferred body temperatures (Gvoždík and Kristín, 2017) and mean air temperatures they normally experience in the field (Šamajová and Gvoždík, 2010). The bottom of each tank was provided with moistened filter paper and a ceramic shelter. Every 3 days, the tanks were thoroughly washed and new substrate provided. The newts were fed with live Tubifex worms and chironomid larvae two or three times per week. Each newt was individually marked with a combination of implanted fluorescent elastomers (NorthWest Marine Technology, Shaw Island, United States). In November, we stopped feeding the newts and gradually reduced the air temperature to 4°C. Newts wintered under these conditions until the end of March. From April, the newts were exposed to the same temperature and light conditions as during the autumn.
Metabolic Rate Assays
Metabolic rate was measured indirectly as the rate of oxygen consumption, using flow-through intermittent respirometry. A more detailed description of the system, along with its verification and calibration, is provided elsewhere (Kristín and Gvoždík, 2012). In short, we flushed CO2-free and water-saturated air (flow rate = 120 ± 1 ml min–1) through each chamber (30 ml) of a nine-channel (eight chambers and one baseline) respirometry system (Sable Systems, Las Vegas, NV, United States) fitted with a baselining unit and an RM-8 multiplexer, twice per hour (enclosure time = 1,679 s) for a total of 5 h. The expired air was then passed through a Nafion™ desiccator, a CO2 analyzer, a scrubber, and an oxygen analyzer (FoxBox-C, Sable Systems). The respirometry chambers were placed in a water bath at 12 and 22 ± 0.5°C, while room temperature was maintained at 5 ± 2°C higher than the experimental temperature to prevent water condensation inside the respirometry system. The experimental temperatures were chosen to cover the newts’ daily temperature range under rearing conditions.
We measured RMR at both temperatures in October and May, using the simplest repeated-measures design, i.e., two body temperatures with two repeated measurements (Killen et al., 2016), to reduce both newt habituation to experimental settings and the duration of measurement bouts. A 7-month time interval between measurement bouts was chosen to examine among-individual (co)variation before and after the cycle of acclimation to wintering temperatures and re-acclimation to thermal conditions during the following activity season. As newts are mostly crepuscular to nocturnal, all metabolic trials took place during the day (08:00–19:00). For each trial, the order of individuals and experimental temperatures was randomized. Newts were weighed to the nearest 0.001 g (KERN EG, Balingen, Germany) and individually placed into a respirometry chamber. Each newt was starved for 5 days prior to the metabolic trials to induce a post-absorptive state (Gvoždík and Kristín, 2017). Since locomotor activity greatly affects newt metabolic rate (Kristín and Gvoždík, 2012), newt activity was continuously monitored inside the chambers using web cameras connected to a PC with motion detection software (5 fps; iSpy software)1 to ensure that newt activity was negligible during the measurement time interval. The lowest oxygen consumption (ml h–1) of non-moving individuals (> 90% of enclosure time) from each trial was calculated from peak areas (integrals) of inverted raw oxygen measurements, divided by chamber enclosure time (Lighton, 2008; Kristín and Gvoždík, 2012). If locomotor activity violated standard conditions for RMR, they were discarded from further analyses. Data acquisition and calculations were performed using Expedata software v. 1.9.17 (Sable Systems).
Locomotor Activity Assays
All newts were rested for 2 days between RMR and ELA measurements. To measure ELA, circular acrylic arenas (140 × 10 mm; n = 9, illuminated from the underneath by IR light, were used to record newt activity at two body temperatures, 12 and 22°C in October and May. Unlike RMR measurements, which were performed in water-saturated air, newt body temperature during ELA trials can be affected by evaporative cooling. Accordingly, we estimated body temperature by measuring the operative temperature inside agar models of a similar size to the experimental individuals (Navas and Araujo, 2000). Visual communication between newts was prevented by placing opaque walls between each arena. Each trial consisted of a 5 min habituation period, followed by a 15 min recording period. After each trial, the experimental arenas were thoroughly cleaned with 95% ethanol to remove chemical cues from previously tested newts. Newts’ position during the trial was continuously recorded using an automated tracking system (3.75 fps; Ethovision XT, Noldus, Wageningen, Netherlands), with ELA calculated as total distance (cm) covered during a trial. Software tracking was checked for missing values prior to calculation, and trials with > 5% missing samples were discarded from further analyses. All trials were performed in an environmental room without human presence during the newt activity period, i.e., after sunset between 20:00 and 22:00. We used satiated newts for each locomotor trial, with the order of individuals and the order of experimental temperatures randomized.
Statistical Analysis
We obtained information on RMR and ELA (co)variation using Bayesian multivariate mixed-effect modeling. First, we analyzed trait (co)variances at each body temperature separately. The model included month of measurement as a fixed factor, body mass as the linear covariate, and individual identity as a random factor. The variables examined and the covariate were mean-centered and divided by sample standard deviation to standardize variation to the same scale. Next, we analyzed (co)variances in the intercepts and slopes of individual thermal reaction norms. We examined (co)variation between intercepts and slopes for both traits, using the bivariate model with random intercept and slopes (Mitchell and Houslay, 2021). The fixed model structure included body temperature and body mass. The random structure consisted of two factors, individual identity and month, with random slopes.
Models were run with three expanded-parameter priors to evaluate the robustness of the results (Supplementary Table 1 and Figures 1–3). Each model run consisted of 830,000 iterations, with the first 30,000 discarded. The remaining chain was sampled every 200th iteration, which resulted in a sample size of 4,000 estimates for posterior distribution. Each chain was tested for autocorrelation (< 0.1 in all cases) and convergence (Heidelberger and Gelman diagnostics). While all models with different priors produced similar parameter estimates, we chose the one with the best chain convergence for statistical inference. We considered any parameter in which the 95% credible interval (CI) did not include zero as statistically significant. For both variance and repeatability estimates (where values cannot be negative), we considered very low positive values (< 10–7) as zero (Houslay and Wilson, 2017) and checked truncation in their posterior distribution. We then calculated variance explained by fixed model structure (Rm2) and the whole model (Rc2; Nakagawa and Schielzeth, 2013).
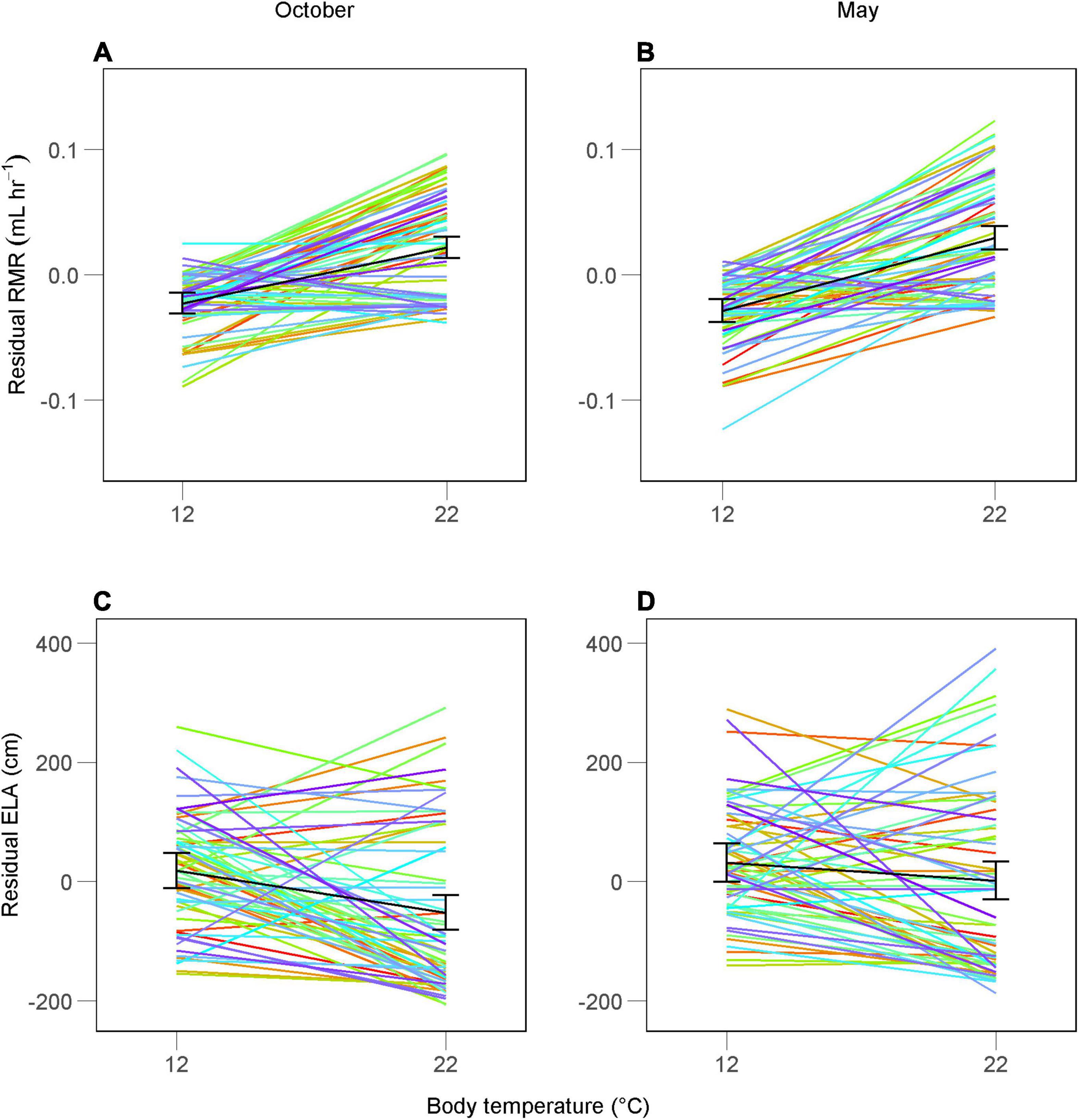
Figure 1. Repeated measurements of mass-adjusted resting metabolic rate (RMR; A,B) and exploratory locomotor activity (ELA; C,D) in juvenile Alpine newts (Ichthyosaura alpestris) at two body temperatures between October and the following May. Each line shows values for one individual, while the black line shows the population mean (95% CI). Values were standardized to zero mean and divided by the sample standard deviation to allow variance comparison between traits.
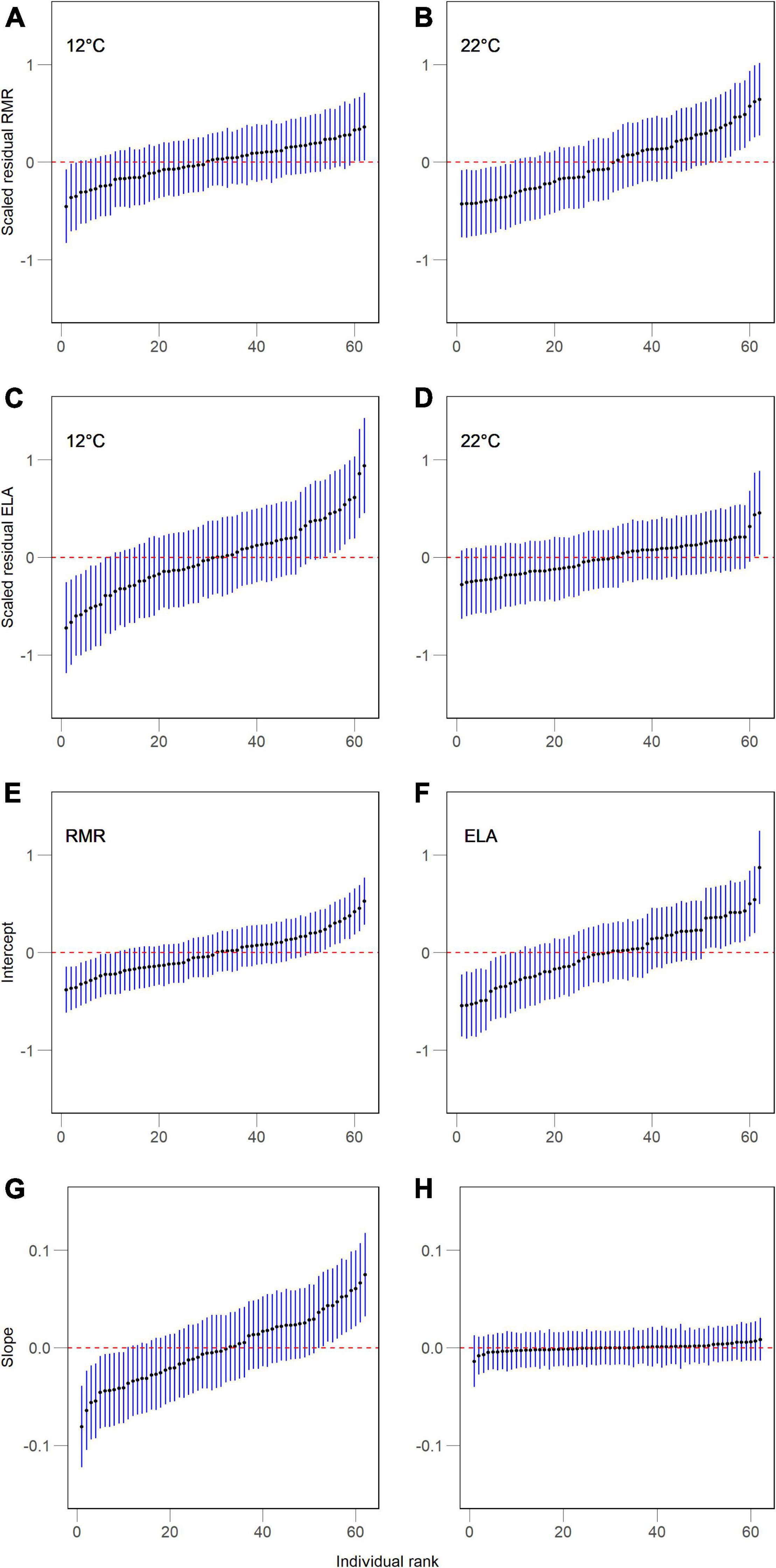
Figure 2. Individual random intercepts of resting metabolic rate (RMR) and exploratory locomotor activity (ELA) at two body temperatures (A–D) and as parameters of their thermal reaction norms, intercepts and slopes (E–H) in juvenile Alpine newts (Ichthyosaura alpestris). Values are estimates (SE) of best linear unbiased predictors (BLUP) for each individual from their respective model (Tables 1, 3).
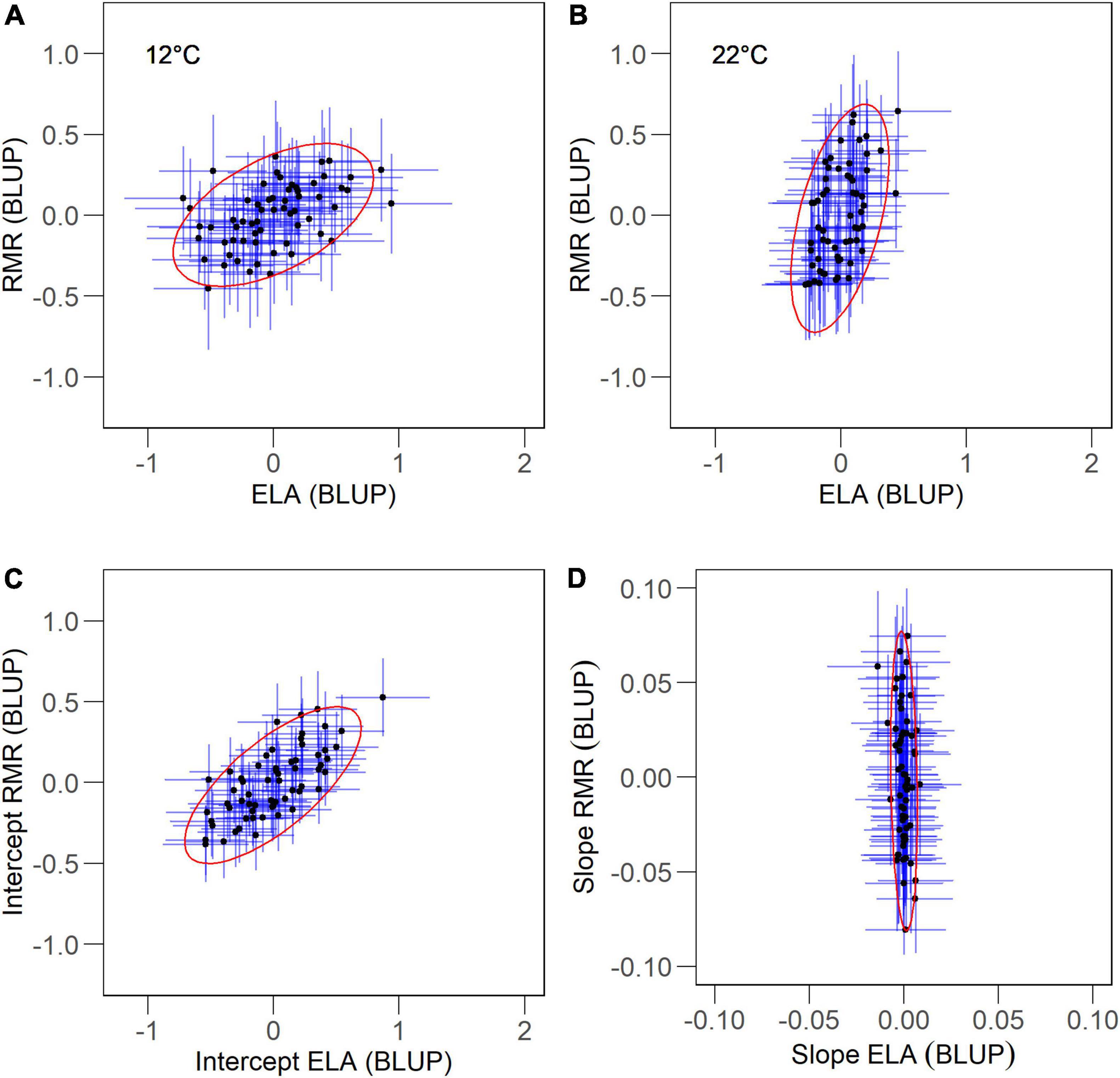
Figure 3. Individual-level associations between resting metabolic rate (RMR) and exploratory locomotor activity (ELA) at two body temperatures (A,B) and as parameters of their thermal reaction norms, intercepts and slopes (C,D), in juvenile Alpine newts (Ichthyosaura alpestris). Values are estimates (SE) of best linear unbiased predictors (BLUP) for each individual from the respective model (Tables 1, 3). Values are fitted with 95% confidence ellipses.
We calculated trait repeatability (R) and correlations (r) from their individual and residual variances (V) and covariances (Cov). Trait repeatability (intraclass correlation) for trait x was calculated as , where Vind,x is among-individual variation and Ve,x is residual variation. In the case of bivariate model with two random factors, we calculated trait repeatability as , where Vmonth,x is between-month variation (Araya-Ajoy et al., 2015). It represents the amount of among-individual variation due to permanent environmental effects and genetic differences. Finally, we used the formula after Araya-Ajoy et al. (2015) to calculate the repeatability of slopes of thermal reaction norms as . Note that Rxslope is not directly comparable to Rx, because slopes and intercepts have different units, and thus the formula lacks residual variance in the denominator. Unlike Rx, this metric shows the amount of among-individual variation due to long-term consistency. Individual correlation (rind) was calculated as , where Covind,xy is among-individual covariation between trait x and y. Similarly, residual correlation (re) was calculated as , where Cove,xy is residual covariation between trait x and y. Finally, phenotypic correlation (rp) was calculated as or in the case of model with two random factors. The statistical significance of R and r were inferred using the same approach as for model parameters. We used the best linear unbiased predictors (BLUP) obtained from multivariate mixed models to visualize trait associations at the individual level (Houslay and Wilson, 2017). All statistical calculations were performed in the R statistical environment (R Development Core Team, 2010, Vienna, Austria), using the “MCMCGlmm” (Hadfield, 2010) and “CODA” (Plummer et al., 2006) packages.
Results
We obtained 496 repeated measures of RMR and ELA from 62 individuals (Figures 1A,B). Both traits were affected by body mass [October: 0.76 ± 0.12 (SD) g; May: 1.04 ± 0.24 g] at both body temperatures, with RMR increasing with body mass and ELA decreasing (Table 1). Mass-adjusted mean RMRs were similar between months at both body temperatures (Figures 1A,B). While mass-adjusted mean ELA showed similar values between months at 12°C, however, newts increased their average locomotor activity at 22°C in May (Figures 1C,D).
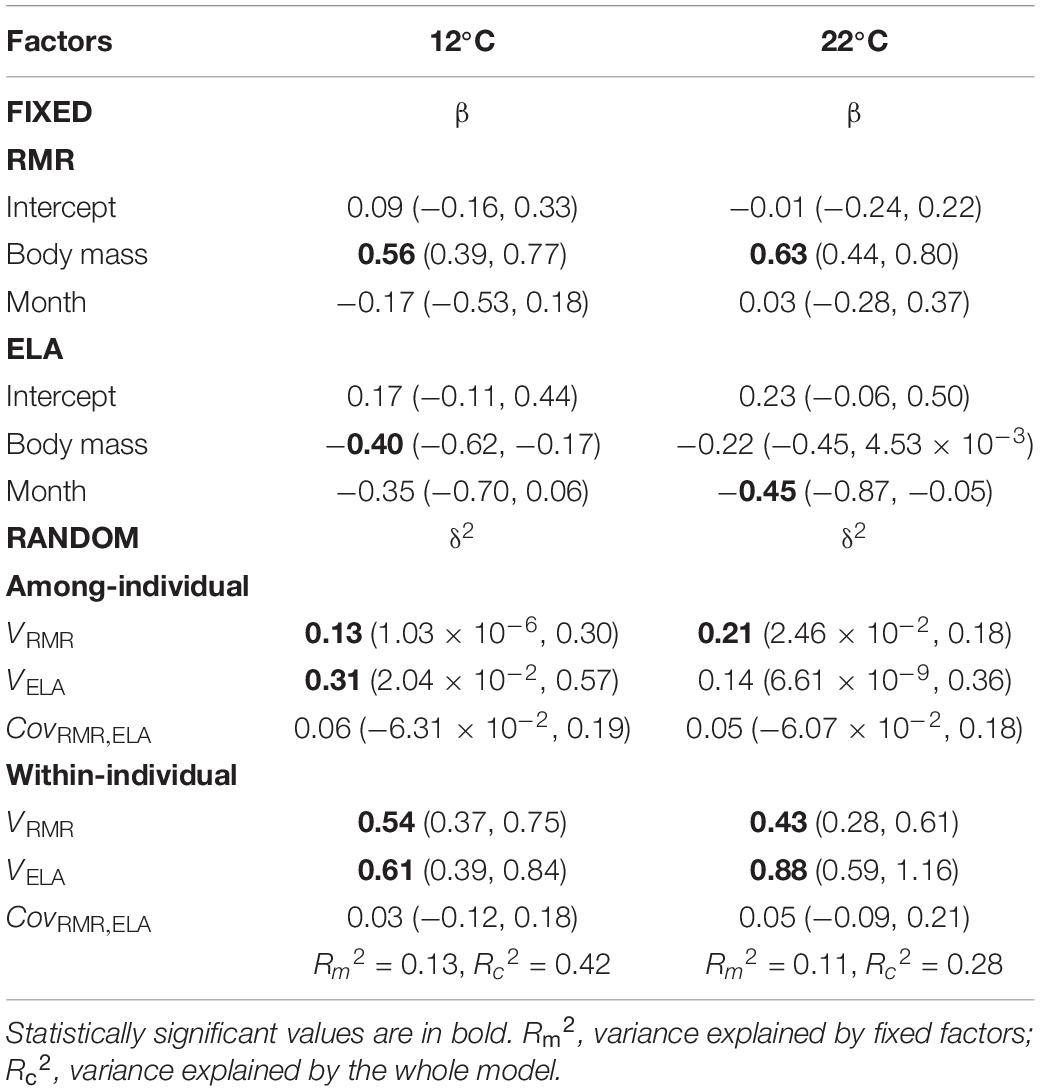
Table 1. Parameters [estimates with 95% credibility intervals (CI)] of multivariate mixed models examining co(variation) between resting metabolic rate (RMR) and exploratory locomotor activity (ELA) in juvenile Alpine newts (Ichthyosaura alpestris) at two body temperatures.
At 12°C, we detected repeatable among-individual variation in ELA, but not in RMR (Table 2 and Figures 2A,C). Association between both traits was statistically non-significant at all variation levels (Table 2 and Figure 3A). At 22°C, we found support for repeatability of RMR, but not ELA (Table 2 and Figures 2B,D). Further, we found no evidence for trait association at this body temperature (Table 2 and Figure 3B).
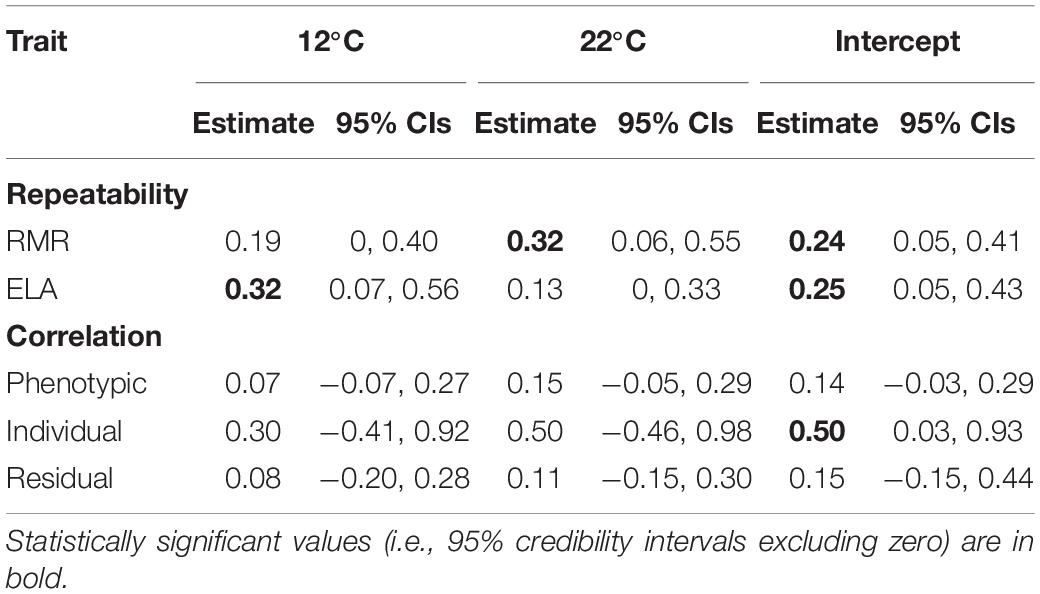
Table 2. Repeatability of resting metabolic rate (RMR) and exploratory locomotor activity (ELA) and their correlation coefficients measured at two body temperatures and as intercepts of their reaction norms in juvenile Alpine newts (Ichthyosaura alpestris).
The fixed structure of random intercept and slope model showed that RMR increased with body mass, while ELA decreased (Table 3). Intercepts of thermal reaction norms for both traits and slopes for RMR contained detectable amounts of among-individual variation (Table 3 and Figures 2E–H). There was no significant covariation between intercepts and slopes among individuals and between months in either trait (Table 3). Intercepts of thermal reaction norms for RMR and ELA were positively associated at the individual level, but not at the whole-phenotypic or residual levels (Table 2 and Figure 3C). As the credible interval for rind was broad, we were unable to obtain any firm conclusion on the exact magnitude of this association. Slope values indicated a disparate effect of body temperature on RMR and ELA (Table 3), with positive values indicating that RMR increased with temperature (Figures 1A,B), while ELA decreased (Figures 1C,D). We found no support for the repeatability of slopes for ELA and its between-trait association at any variation level (Table 3 and Figure 3D).
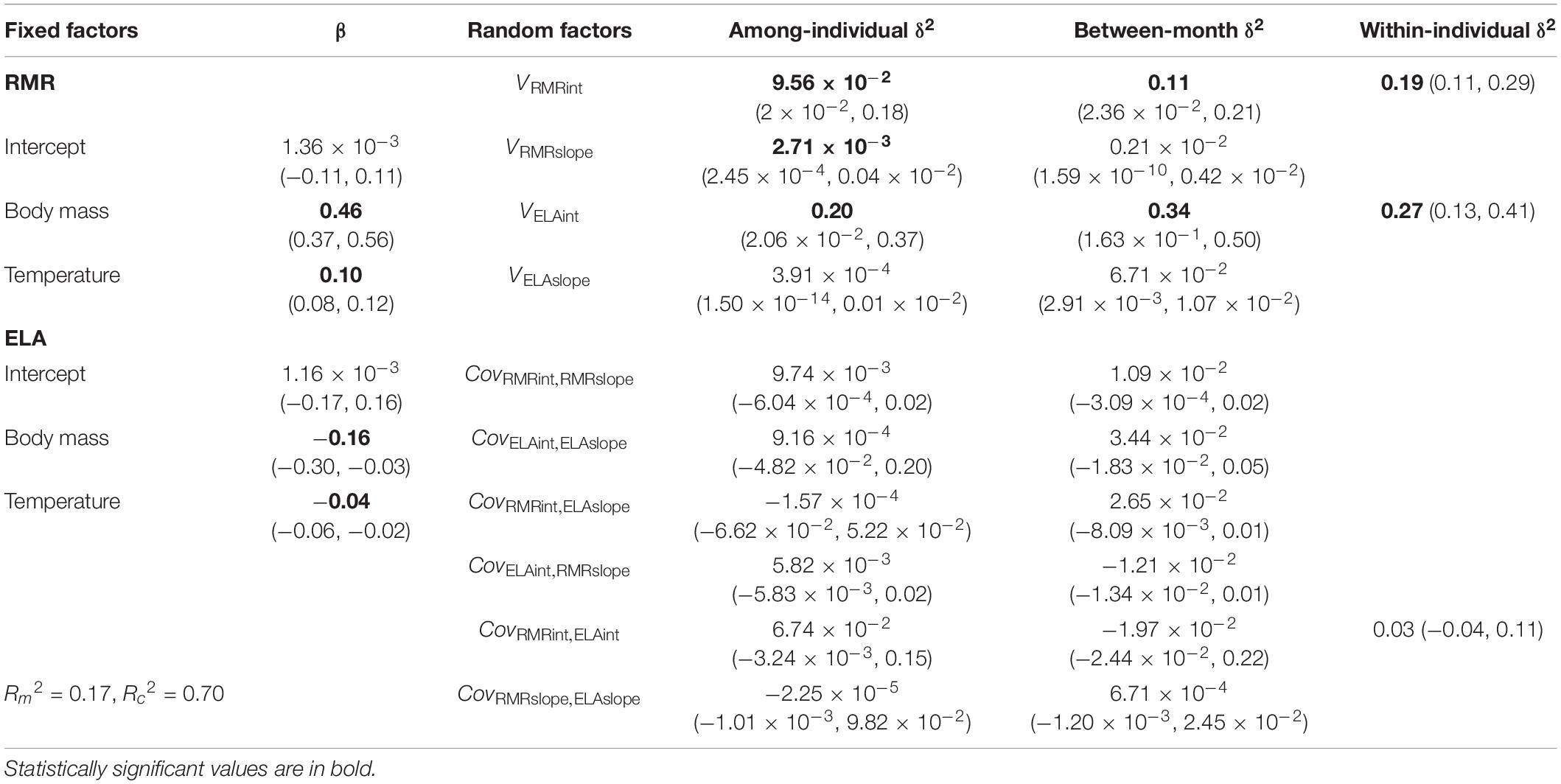
Table 3. Parameters [estimates with 95% credibility intervals (CI)] for multivariate mixed models examining co(variation) among intercepts and slopes of reaction norms for resting metabolic rate (RMR) and exploratory locomotor activity (ELA) in juvenile Alpine newts (Ichthyosaura alpestris).
Discussion
Despite their fundamental importance in behavioral and physiological ecology (Careau et al., 2008; Biro and Stamps, 2010; Careau and Garland, 2012; Mathot and Dingemanse, 2015; Killen et al., 2016), factors affecting metabolic-behavioral relationships remain poorly understood. We identified body temperature and among-individual trait variation (repeatability) as important modifiers of the relationship between RMR and ELA in juvenile newts and showed that detection of among-individual variation in RMR and ELA varies with body temperature. At 12°C, we only detected repeatability in ELA, while at 22°C, we detected repeatability in RMR but not ELA. Accordingly, we were only able to obtain poor estimates for their association at each body temperature. In contrast, intercepts of thermal reaction norms across these temperatures showed repeatability in both traits, with the traits being positively associated at the individual, but not the whole-phenotypic, level.
Our results provide evidence for a positive association between RMR and ELA in juvenile newts at the individual level. While a recent meta-analysis found no support for this relationship across taxa, which was interpreted as the result of the ambiguous impact of locomotor activity on individual energy budget (Mathot et al., 2019), our results suggest a previously unconsidered explanation, i.e., that the inconclusive link between metabolic rate and locomotor activity results from the opposing effect of body temperature on among-individual variation in each trait. In our study, the variation pattern produced poor estimates for correlation coefficients at both temperatures; however, “averaging” trait values across temperatures (intercepts of thermal reaction norm) stabilized individual rank for each trait, which improved estimates of individual correlation coefficient. This demonstrates the necessity to examine metabolic-behavioral relationships using repeated measurements from at least two body temperatures to obtain representative values for ectotherm taxa.
We observed that the relationship between RMR and ELA remained inconclusive at both body temperatures in juvenile newts. The body temperatures used here were within the range of preferred body temperatures for this species (Gvoždík and Kristín, 2017), suggesting that the newts in our study were not thermally stressed. According to a recent theory, the absence of thermal stress should not affect physio-behavioral associations (Killen et al., 2013). However, our results showed that even in the absence of stress, temperature changes altered the amount of among-individual variation in opposite directions for each trait, which resulted in similarly weak support for their association. At present, it is not known how thermal stress alters the association between metabolism and behavior in other ectotherms. While it is known that experimental temperature is an important modifier of the relationship between metabolism and behavior in endotherms (Chappell et al., 2004), the results are not directly comparable between endotherms and ectotherms due to the disparate influence of environmental temperature on their metabolic rate (McNab, 2002). Although further empirical studies are needed to fully understand the effects of environmental stressors on trait (co)variation, it is already clear that trait (co)variation is more sensitive to environmental changes than has previously been thought.
In our study, unlike intercepts, slopes contained significant among-individual variation in RMR only. Previously published results provide mixed support for this finding (Careau et al., 2014; Mitchell and Biro, 2017; Baškiera and Gvoždík, 2019; Kar et al., 2021), suggesting that the amount of among-individual variation in thermal sensitivity varies substantially among taxa and traits. Among-individual variation in slopes appears to be affected by the disparate effects of temperature on physiological and behavioral traits (Abram et al., 2017). The thermal dependence observed in RMR is a typical example of a kinetic effect, which is determined by the amount of kinetic energy in a physiological system. In contrast, ELA is mostly affected by the integrated effect of temperature, which results from sensed thermal information integrated by the neural system. Accordingly, the shape of reaction norms for ELA varies between newt life stages and differs substantially from thermal performance curves for forced locomotion (Baškiera and Gvoždík, 2019; Gvoždík and Boukal, 2021). Given the presence of among-individual variation is the basic assumption for evolution by natural selection, different amount of this variation in slopes for RMR and ELA suggests that thermal sensitivities of physiological and behavioral traits evolve in disparate rates in newts (Gvoždík, 2015) as in other long-lived ectotherms (Muñoz et al., 2014; Bodensteiner et al., 2021).
One could argue that our estimates of among-individual variation are confounded by the experimental design used. Thermal reaction norms, for example, are often non-linear (Huey and Stevenson, 1979; Amarasekare, 2015; Little and Seebacher, 2021), whereas our measurements at two body temperatures assume a linear thermal dependence of the traits studied. Although the thermal dependence of ELA is U-shaped across the broad body temperature gradient, both ELA and RMR are linear within the limited temperature range used in the present study (Gvoždík and Kristín, 2017; Baškiera and Gvoždík, 2019). Accordingly, our “two-temperature” approach provided unbiased estimates of the variation in the slopes of thermal reaction norms. Another source of bias could arise from neglecting the maternal effect on examined traits (Bernardo, 1996; Mousseau and Fox, 1998; Uller, 2008). For logistical reasons, we could not raise newts from each clutch separately, and therefore consider maternal identity as an additional random factor. Therefore, we cannot exclude the possibility that among-individual variation in the traits studied was overestimated compared to values obtained from randomly selected individuals in a large population. Despite this shortcoming, the phenotypic similarity between offspring should not affect the main result of our study, i.e., the differential effect of body temperature on among-individual variation in physiological and behavioral traits.
The positive association between RMR and ELA has both ecological and evolutionary implications. From an ecological point of view, the positive RMR-ELA link suggests that juvenile newts manage their energy budget according to the performance model (Careau and Garland, 2012; Mathot and Dingemanse, 2015). This is contrary to recent findings showing a negative relationship between maintenance metabolic rate and energy available for other tasks, but is in accordance with the positive association between maintenance and maximum metabolic rate, in adult newts across body temperatures (Baškiera and Gvoždík, 2020). The likely explanation for this discrepancy is the context-dependency of energy management (Halsey et al., 2019). Juvenile newts, for example, show variation in their RMR and growth rates in the presence of intra- and interspecific competition (Janča and Gvoždík, 2017), which may corroborate this view. However, further experimental support is needed for a definite conclusion. From an evolutionary point of view, this finding suggests that phenotypic selection should act on RMR and ELA in a correlative manner. Theory suggests that the adaptive significance of RMR is highly context-dependent (Careau et al., 2008; Burton et al., 2011; Norin and Metcalfe, 2019). Positive RMR-ELA covariation means that if resources are widely available, selection should favor metabolically fast individuals with high locomotor activity level, while low metabolic rate and locomotor activity should be beneficial under a scarcity of resources. In ectotherms, context-dependent adaptive significance is complicated by variation in the thermal dependency of both traits. For example, under limited resources, high body temperatures disproportionally increase mandatory metabolic costs relative to locomotor activity level, which provides a further penalization to metabolically-fast individuals. As such, climate warming is likely to erode metabolic-behavioral variation within ectotherm populations in the near future.
To sum up, we demonstrated that body temperature and individual variation hide metabolic-behavioral relationships in an ectotherm, with body temperature modifying the link not through disparate thermal dependency of mean trait values but the amount of among-individual variation. By focusing on parameters of reaction norms rather than trait values at a single body temperature, we were able to identify the link between metabolic rate and locomotor activity. This clearly indicates the importance of a repeated measures design across at least two body temperatures for obtaining meaningful information on metabolic-behavioral links in ectotherms (Killen et al., 2016). The minimalistic approach could be further improved by adding more temperatures and repeated measures to increase the accuracy of parameter estimates (van de Pol, 2012; Dingemanse and Dochtermann, 2013; Mitchell and Houslay, 2021); however, finding an optimal solution among the statistical needs, logistical issues, and confounding effects of habituation or acclimation provides a challenging task in this field. Despite these issues, we believe that adopting the repeated measurements approach over two or more temperatures will substantially improve our understanding of the role of energy budget (Careau and Garland, 2012), pace of life syndrome (Goulet et al., 2017), and trade-offs (Careau and Wilson, 2017) in shaping ectotherm responses to environmental change.
Data Availability Statement
The datasets presented in this study can be found in online repositories. The names of the repository/repositories and accession number(s) can be found below: https://doi.org/10.6084/m9.figshare.17013449.v1.
Ethics Statement
All experimental procedures were approved by the Expert Committee for Animal Conservation of the Institute of Vertebrate Biology of the Czech Academy of Sciences (research protocol no. 135/2016). Permission to capture newts was provided by the Agency for Nature Conservation and Landscape Protection of the Czech Republic (1154/ZV/2008).
Author Contributions
LG conceived the ideas, designed the methodology, and analyzed the data. SB and LG collected the data and wrote the manuscript. Both authors contributed critically to the drafts and gave final approval for publication.
Funding
This research was supported by the Czech Science Foundation (Grant Nos. 17-15480S and 21-29169S to LG), the Institute of Vertebrate Biology of the Czech Academy of Sciences (RVO: 68081766 to LG), and the Department of Botany and Zoology, Faculty of Science, Masaryk University in Brno, Czechia (MUNI/A/1575/2020 to SB).
Conflict of Interest
The authors declare that the research was conducted in the absence of any commercial or financial relationships that could be construed as a potential conflict of interest.
Publisher’s Note
All claims expressed in this article are solely those of the authors and do not necessarily represent those of their affiliated organizations, or those of the publisher, the editors and the reviewers. Any product that may be evaluated in this article, or claim that may be made by its manufacturer, is not guaranteed or endorsed by the publisher.
Acknowledgments
We thank all reviewers for their comments on the previous version of this study, L. Podhajský for his help in completing the experiment, and M. Šugerková for caring for the newts.
Supplementary Material
The Supplementary Material for this article can be found online at: https://www.frontiersin.org/articles/10.3389/fevo.2022.850941/full#supplementary-material
Footnotes
References
Abram, P. K., Boivin, G., Moiroux, J., and Brodeur, J. (2017). Behavioral effects of temperature on ectothermic animals: unifying thermal physiology and behavioural plasticity. Biol. Rev. 92, 1859–1876. doi: 10.1111/brv.12312
Amarasekare, P. (2015). Effects of temperature on consumer-resource interactions. J. Anim. Ecol. 84, 665–679. doi: 10.1111/1365-2656.12320
Angilletta, M. J., Bennett, A. F., Guderley, H., Navas, C. A., Seebacher, F., and Wilson, R. S. (2006). Coadaptation: a unifying principle in evolutionary thermal biology. Physiol. Biochem. Zool. 79, 282–294. doi: 10.1086/499990
Araya-Ajoy, Y. G., Mathot, K. J., and Dingemanse, N. J. (2015). An approach to estimate short-term, long-term and reaction norm repeatability. Methods Ecol. Evol. 6, 1462–1473. doi: 10.1111/2041-210X.12430
Baškiera, S., and Gvoždík, L. (2019). Repeatability of thermal reaction norms for spontaneous locomotor activity in juvenile newts. J. Therm. Biol. 80, 126–132. doi: 10.1016/j.jtherbio.2019.01.010
Baškiera, S., and Gvoždík, L. (2020). Thermal independence of energy management in a tailed amphibian. J. Vertebr. Biol. 69, 1–10. doi: 10.25225/jvb.20057
Baškiera, S., and Gvoždík, L. (2021). Repeatability and heritability of resting metabolic rate in a long-lived amphibian. Comp. Biochem. Physiol. A Mol. Integr. Physiol. 253:110858. doi: 10.1016/j.cbpa.2020.110858
Bell, A. M., Hankison, S. J., and Laskowski, K. L. (2009). The repeatability of behavior: a meta-analysis. Anim. Behav. 77, 771–783. doi: 10.1016/j.anbehav.2008.12.022
Bennett, A. F., and Huey, R. B. (1990). Studying the evolution of physiological performance. Oxford Surv. Evol. Biol. 7, 251–284.
Biro, P. A., and Stamps, J. A. (2010). Do consistent individual differences in metabolic rate promote consistent individual differences in behavior? Trends Ecol. Evol. 25, 653–659. doi: 10.1016/j.tree.2010.08.003
Bodensteiner, B. L., Agudelo-Cantero, G. A., Arietta, A. Z. A., Gunderson, A. R., Muñoz, M. M., Refsnider, J. M., et al. (2021). Thermal adaptation revisited: how conserved are thermal traits of reptiles and amphibians? J. Exp. Zool. A Ecol. Integr. Physiol. 335, 173–194. doi: 10.1002/jez.2414
Burger, J. R., Hou, C., Hall, C. A. S., and Brown, J. H. (2021). Universal rules of life: metabolic rates, biological times and the equal fitness paradigm. Ecol. Lett. 24, 1262–1281. doi: 10.1111/ele.13715
Burton, T., Killen, S. S., Armstrong, J. D., and Metcalfe, N. B. (2011). What causes intraspecific variation in resting metabolic rate and what are its ecological consequences? Proc. R. Soc. B 278, 3465–3473. doi: 10.1098/rspb.2011.1778
Careau, V., and Garland, T. (2012). Performance, personality, and energetics: correlation, causation, and mechanism. Physiol. Biochem. Zool. 85, 543–571. doi: 10.1086/666970
Careau, V., Gifford, M. E., and Biro, P. A. (2014). Individual (co)variation in thermal reaction norms of standard and maximal metabolic rates in wild-caught slimy salamanders. Funct. Ecol. 28, 1175–1186. doi: 10.1111/1365-2435.12259
Careau, V., Thomas, D., Humphries, M. M., and Réale, D. (2008). Energy metabolism and animal personality. Oikos 117, 641–653. doi: 10.1111/j.0030-1299.2008.16513.x
Careau, V., and Wilson, R. S. (2017). Of uberfleas and krakens: detecting trade-offs using mixed models. Integr. Comp. Biol. 57, 362–371. doi: 10.1093/icb/icx015
Chappell, M. A., Garland, T., Rezende, E. L., and Gomes, F. R. (2004). Voluntary running in deer mice: speed, distance, energy costs and temperature effects. J. Exp. Biol. 207, 3839–3854. doi: 10.1242/jeb.01213
Dingemanse, N. J., and Dochtermann, N. A. (2013). Quantifying individual variation in behavior: mixed-effect modelling approaches. J. Anim. Ecol. 82, 39–54. doi: 10.1111/1365-2656.12013
Gifford, M. E., Clay, T. A., and Careau, V. (2014). Individual (Co)variation in standard metabolic rate, feeding rate, and exploratory behavior in wild-caught semiaquatic salamanders. Physiol. Biochem. Zool. 87, 384–396. doi: 10.1086/675974
Goulet, C. T., Thompson, M. B., Michelangeli, M., Wong, B. B. M., and Chapple, D. G. (2017). Thermal physiology: a new dimension of the pace-of-life syndrome. J. Anim. Ecol. 86, 1269–1280. doi: 10.1111/1365-2656.12718
Gvoždík, L. (2015). Mismatch between ectotherm thermal preferenda and optima for swimming: a test of the evolutionary pace hypothesis. Evol. Biol. 42, 137–145. doi: 10.1007/s11692-015-9305-z
Gvoždík, L., and Boukal, D. S. (2021). Impacts of predator-induced behavioral plasticity on the temperature dependence of predator-prey activity and population dynamics. J. Anim. Ecol. 90, 503–514. doi: 10.1111/1365-2656.13383
Gvoždík, L., and Kristín, P. (2017). Economic thermoregulatory response explains mismatch between thermal physiology and behavior in newts. J. Exp. Biol. 220, 1106–1111. doi: 10.1242/jeb.145573
Hadfield, J. (2010). MCMC methods for multi-response generalized linear mixed models: the MCMCglmm R package. J. Stat. Softw. 33, 1–22.
Halsey, L. G., Green, J. A., Twiss, S. D., Arnold, W., Burthe, S. J., Butler, P. J., et al. (2019). Flexibility, variability and constraint in energy management patterns across vertebrate taxa revealed by long-term heart rate measurements. Funct. Ecol. 33, 260–272. doi: 10.1111/1365-2435.13264
Holtmann, B., Lagisz, M., and Nakagawa, S. (2017). Metabolic rates, and not hormone levels, are a likely mediator of between-individual differences in behavior: a meta-analysis. Funct. Ecol. 31, 685–696. doi: 10.1111/1365-2435.12779
Houslay, T. M., and Wilson, A. J. (2017). Avoiding the misuse of BLUP in behavioral ecology. Behav. Ecol. 28, 948–952. doi: 10.1093/beheco/arx023
Huey, R. B., Kearney, M. R., Krockenberger, A., Holtum, J. A. M., Jess, M., and Williams, S. E. (2012). Predicting organismal vulnerability to climate warming: roles of behavior, physiology and adaptation. Proc. R. Soc. B 367, 1665–1679. doi: 10.1098/rstb.2012.0005
Huey, R. B., and Stevenson, R. D. (1979). Integrating thermal physiology and ecology of ectotherms: a discussion of approaches. Am. Zool. 19, 357–366. doi: 10.1093/icb/19.1.357
Janča, M., and Gvoždík, L. (2017). Costly neighbours: heterospecific competitive interactions increase metabolic rates in dominant species. Sci. Rep. 7:5177. doi: 10.1038/s41598-017-05485-9
Johnston, A. S. A., Boyd, R. J., Watson, J. W., Paul, A., Evans, L. C., Gardner, E. L., et al. (2019). Predicting population responses to environmental change from individual-level mechanisms: towards a standardized mechanistic approach. Proc. R. Soc. B 286:20191916. doi: 10.1098/rspb.2019.1916
Kar, F., Nakagawa, S., Friesen, C. R., and Noble, D. W. A. (2021). Individual variation in thermal plasticity and its impact on mass-scaling. Oikos 130, 1131–1142. doi: 10.1111/oik.08122
Killen, S. S., Adriaenssens, B., Marras, S., Claireaux, G., and Cooke, S. J. (2016). Context dependency of trait repeatability and its relevance for management and conservation of fish populations. Conserv. Physiol. 4:cow007. doi: 10.1093/conphys/cow007
Killen, S. S., Marras, S., Metcalfe, N. B., Mckenzie, D. J., and Domenici, P. (2013). Environmental stressors alter relationships between physiology and behavior. Trends Ecol. Evol. 28, 651–658. doi: 10.1016/j.tree.2013.05.005
Kristín, P., and Gvoždík, L. (2012). Influence of respirometry methods on intraspecific variation in standard metabolic rates in newts. Comp. Biochem. Physiol. A 163:147151. doi: 10.1016/j.cbpa.2012.05.201
Lighton, J. R. B. (2008). Measuring Metabolic Rates: A Manual for Scientists. New York, NY: Oxford University Press.
Little, A. G., and Seebacher, F. (2021). Physiological performance curves: when are they useful? Front. Physiol. 12:805102. doi: 10.3389/fphys.2021.805102
Mathot, K. J., and Dingemanse, N. J. (2015). Energetics and behavior: unrequited needs and new directions. Trends Ecol. Evol. 30, 199–206. doi: 10.1016/j.tree.2015.01.010
Mathot, K. J., Dingemanse, N. J., and Nakagawa, S. (2019). The covariance between metabolic rate and behavior varies across behaviors and thermal types: meta-analytic insights. Biol. Rev. 94, 1056–1074. doi: 10.1111/brv.12491
McNab, B. K. (2002). The Physiological Ecology of Vertebrates: A View from Energetics. Ithaca, NY: Cornell University Press.
Mitchell, D. J., and Biro, P. A. (2017). Is behavioral plasticity consistent across different environmental gradients and through time? Proc. R. Soc. B 284:20170893. doi: 10.1098/rspb.2017.0893
Mitchell, D. J., and Houslay, T. M. (2021). Context-dependent trait covariances: how plasticity shapes behavioral syndromes. Behav. Ecol. 32, 25–29. doi: 10.1093/beheco/araa115
Mousseau, T. A., and Fox, C. W. (1998). The adaptive significance of maternal effects. Trends Ecol. Evol. 13, 403–407. doi: 10.1016/S0169-5347(98)01472-4
Muñoz, M. M., Stimola, M. A., Algar, A. C., Conover, A., Rodriguez, A. J., Landestoy, M. A., et al. (2014). Evolutionary stasis and lability in thermal physiology in a group of tropical lizards. Proc. R. Soc. B 281:20132433. doi: 10.1098/rspb.2013.2433
Nakagawa, S., and Schielzeth, H. (2013). A general and simple method for obtaining R2 from generalized linear mixed-effects models. Methods Ecol. Evol. 4, 133–142. doi: 10.1111/j.2041-210x.2012.00261.x
Navas, C. A., and Araujo, C. (2000). The use of agar models to study amphibian thermal ecology. J. Herpetol. 34, 330–334. doi: 10.2307/1565438
Norin, T., and Metcalfe, N. B. (2019). Ecological and evolutionary consequences of metabolic rate plasticity in response to environmental change. Philos. Trans. R. Soc. 37:20180180. doi: 10.1098/rstb.2018.0180
Plummer, M., Best, N., Cowles, K., and Vines, K. (2006). CODA: convergence diagnosis and output analysis for MCMC. R News 6, 7–11.
R Development Core Team (2010). R: A Language and Environment for Statistical Computing. Vienna: R foundation for statistical computing.
Šamajová, P., and Gvoždík, L. (2010). Inaccurate or disparate temperature cues? Seasonal acclimation of terrestrial and aquatic locomotor capacity in newts. Funct. Ecol. 24, 1023–1030. doi: 10.1111/j.1365-2435.2010.01720.x
Uller, T. (2008). Developmental plasticity and the evolution of parental effects. Trends Ecol. Evol. 23, 432–438. doi: 10.1016/j.tree.2008.04.005
van de Pol, M. (2012). Quantifying individual variation in reaction norms: how study design affects the accuracy, precision and power of random regression models. Methods Ecol. Evol. 3, 268–280. doi: 10.1111/j.2041-210X.2011.00160.x
Videlier, M., Rundle, H. D., and Careau, V. (2019). Sex-specific among-individual covariation in locomotor activity and resting metabolic rate in Drosophila melanogaster. Am. Nat. 194, E164–E176. doi: 10.1086/705678
White, C. R., Schimpf, N. G., and Cassey, P. (2013). The repeatability of metabolic rate declines with time. J. Exp. Biol. 216, 1763–1765. doi: 10.1242/jeb.076562
Keywords: energy management, locomotor activity, metabolic rate, thermal adaptation, amphibians, repeatability
Citation: Baškiera S and Gvoždík L (2022) Individual Variation in Thermal Reaction Norms Reveals Metabolic-Behavioral Relationships in an Ectotherm. Front. Ecol. Evol. 10:850941. doi: 10.3389/fevo.2022.850941
Received: 08 January 2022; Accepted: 24 February 2022;
Published: 01 April 2022.
Edited by:
Jose A. Masero, University of Extremadura, SpainReviewed by:
Anthony Herrel, Muséum National d’Histoire Naturelle, FranceSylvain Pincebourde, UMR 7261 Institut de Recherche sur la Biologie de l’Insecte (IRBI), France
Copyright © 2022 Baškiera and Gvoždík. This is an open-access article distributed under the terms of the Creative Commons Attribution License (CC BY). The use, distribution or reproduction in other forums is permitted, provided the original author(s) and the copyright owner(s) are credited and that the original publication in this journal is cited, in accordance with accepted academic practice. No use, distribution or reproduction is permitted which does not comply with these terms.
*Correspondence: Lumír Gvoždík, Z3ZvemRpa0Bicm5vLmNhcy5jeg==
†ORCID: Senka Baškiera, orcid.org/0000-0001-6045-5726; Lumír Gvoždík, orcid.org/0000-0002-6495-2233