Modulating Effects of Landscape Characteristics on Responses to Warming Differ Among Butterfly Species
- 1PBL Netherlands Environmental Assessment Agency, The Hague, Netherlands
- 2Department of Environmental Science, Radboud Institute for Biological and Environmental Sciences, Radboud University, Nijmegen, Netherlands
- 3Dutch Butterfly Conservation, Wageningen, Netherlands
- 4Butterfly Conservation Europe Foundation, Wageningen, Netherlands
Understanding and predicting biodiversity responses to climate change are vital to inform conservation strategies, but this is not straightforward as climate change responses depend on the landscape context and differ among species. Here, we quantified changes in the distribution and abundance of 30 butterfly species in the Netherlands in relation to climate change and in landscapes that vary in the amount and connectivity of (semi-)natural vegetation (SNV). We obtained yearly counts of well-monitored butterfly species from 327 time series over 27 years (1992–2018). We used these counts to build mixed effect hurdle models to relate species’ occurrence and abundance to temperature and the amount and connectivity of SNV around the sites. For 55% of the butterfly species, an increased amount or connectivity of SNV corresponded with stronger increases or reduced decreases in occurrence in response to warming, indicating that SNV may facilitate range expansion or mitigate extirpations due to climate change. However, for the occurrence of the other species we found no or a negative interaction between warming and SNV. Further, we did not find indications of a mitigating effect of SNV on abundance responses to warming. Our results thus suggest that increasing the amount and connectivity of SNV does not offer a “one-size-fits-all” solution, highlighting the need for additional measures if butterfly diversity is to be conserved.
Introduction
Global biodiversity is currently facing unprecedented and increasing human pressures, resulting in major declines in vertebrate and invertebrate species (Butchart et al., 2010; Böhm et al., 2013; Tittensor et al., 2014; Thomas, 2016; Wagner, 2020). Currently, the most important direct drivers of these declines are overexploitation and habitat loss (Maxwell et al., 2016; Cardoso et al., 2020). Climate change is, however, becoming an increasingly important driver of biodiversity change (Pereira et al., 2012; Platts et al., 2019; Sánchez-Bayo and Wyckhuys, 2019; Forister et al., 2021). Depending on their thermal tolerance, species may respond to warming through physiological or phenological adaptations or by shifting their distribution towards higher altitudes or latitudes (Easterling et al., 2000; Bellard et al., 2012; Oliver and Morecroft, 2014; Rodrigues and Beldade, 2020). If species fail to adapt or shift their range, climate change will result in abundance declines (Bowler et al., 2017; Antão et al., 2020; Halsch et al., 2021).
Understanding and predicting biodiversity responses to climate change are vital to inform conservation strategies. This is, however, not straightforward, as responses may depend on context-specific conditions, including landscape characteristics (Opdam and Wascher, 2004; Sirami et al., 2017; Newbold et al., 2019). For example, in landscapes heavily modified by human activities, climate change impacts might be larger because species may be hindered in tracking suitable climates, particularly if they have a low dispersal capacity (Pöyry et al., 2009; Schloss et al., 2012; Habel et al., 2016; Oliver et al., 2017). In landscapes with a heterogeneous topography, climate change impacts on species might be smaller due to the larger variety of microclimates, which promotes population stability (Papanikolaou et al., 2017a; Suggitt et al., 2018). Furthermore, (semi-)natural vegetation may mitigate the effect of climate change on species’ abundance and diversity by modulating the microclimate (Oliver et al., 2016; Papanikolaou et al., 2017b).
Butterflies offer excellent opportunities to investigate species’ responses to climate change, not only because they are ectotherms but also because they are among the best-monitored insect species (Thomas, 2016). Existing studies assessing how butterfly responses to climate change are influenced by landscape characteristics have typically focused on a single species (Delattre et al., 2013; Fourcade et al., 2017) or on responses aggregated across multiple species (Oliver et al., 2017; Fourcade et al., 2021). While aggregated multi-species indicators or models may offer valuable insights in general patterns or trends and potential overarching mechanisms, it is generally acknowledged that this aggregation may mask considerable variability among species (Schipper et al., 2016; Leung et al., 2020). Hence, there is clear added value in comparative analyses of the interactive effects of climate change and landscape characteristics across multiple butterfly species.
Here, we aimed to quantify changes in distribution and abundance of multiple individual butterfly species in relation to climate change in landscapes that vary in the amount and connectivity of (semi-)natural vegetation. To that end, we used counts of 30 well-monitored non-migratory butterfly species in the Netherlands, obtained from 327 time series of systematic monitoring data over a period of 27 years (1992–2018). We focused on the Netherlands not only because of the high-quality butterfly monitoring data, but also because of the country’s efforts to improve the connectivity of natural areas in a national ecological network since 1989 (Jongman et al., 2004). We established mixed effect hurdle models to relate species occurrence and abundance to the temperature and the amount and connectivity of (semi-)natural vegetation in the proximity of the site. We then analyzed whether the species’ responses were related to their mobility and thermal niche, which are traits related to species’ responses to temperature change (Fourcade et al., 2021). We also assessed whether the responses were related to the commonness of the species in the Netherlands. By providing insights in the extent to which butterfly responses to climate change depend on landscape and species characteristics, our study helps to understand the effectiveness of landscape-level measures to mitigate potential climate-induced declines of butterfly species (Heller and Zavaleta, 2009; Sirami et al., 2017).
Materials and Methods
Species and Site-Level Habitat Data
We retrieved observations of non-migratory butterfly species from the Dutch Butterfly Monitoring Scheme, which started in 1992 and consists of 1,991 fixed transects of around 1 km long distributed across the Netherlands (Supplementary Figure 1; van Swaay et al., 2002; van Strien et al., 2019). In the Dutch Butterfly Monitoring Scheme, all butterflies are counted weekly from April to September within a distance of 2.5 m from each transect. Surveys are performed in favorable weather conditions, i.e., no rain, low wind speed and temperature above 17°C on days with overcast and above 13°C on sunny days (van Swaay et al., 2002). From the resulting database we retrieved for each species the number of butterfly weeks per transect and per year. The number of butterfly weeks is an estimate of the total number of individuals of a species and is equal to the area under the curve of the linearly interpolated weekly counts (van Swaay et al., 2002). We summed the number of butterfly weeks of the first, second and, for several species, third flying period (generation). The number of observed individuals was assumed to be zero at the start and end of each generation of the respective species (van Swaay et al., 2002). The number of butterfly weeks was only determined in years where the transect was observed at least three times per generation and the time between two subsequent observations of the transect was less than half of the duration of the generation. We excluded the first generation of species that hibernate, as these individuals are included in the counts of the previous year (van Swaay et al., 2002). The site-level habitat type of each transect was recorded during the first year of observation (van Swaay et al., 2002). The recorded habitat types of the transects include agriculture, forest, dunes, semi-natural grassland, heathland, urban, and wetland.
Based on monitoring data from 1992 up to and including 2018, we selected transects according to the following criteria: (i) a single habitat type has been recorded on the transect and (ii) the transect has been monitored in at least 5 years (not necessarily consecutive). From the resulting set of transects we then selected only one per 1 km grid cell, choosing the transect with the largest number of monitored years, in order to limit spatial correlation between transects. This selection resulted in 327 transects and a total of 60 species (of the 66 species recorded in the initial dataset) (Supplementary Figure 1). Supplementary Table 1 shows for each species the number of absences and presences and the number of transects recorded within the site-level habitat types.
Environmental Data
To characterize the temperature at each transect and year, we calculated the growing degree days (GDD), i.e., the sum of all daily mean temperature values above 5°C within a year (Hill et al., 2002; Heikkinen et al., 2010). GDD is a commonly used predictor of the distribution and abundance of butterfly species as it influences the development of butterfly larvae (e.g., Heikkinen et al., 2010; WallisDeVries et al., 2011; Pellissier et al., 2012; Aguirre-Gutiérrez et al., 2017; Palmer et al., 2017). We used daily mean temperature measurements of 38 weather stations from 1991 onwards to calculate the GDD per year (KNMI, 2019). We calculated yearly GDD from September of the previous year to August of the year of interest to maximize overlap with a typical butterfly life cycle. Subsequently, we spatially interpolated the GDD measurements of each year to obtain a yearly value for each transect. Following the recommendations of the Royal Netherlands Meteorological Institute, we interpolated GDD by means of universal kriging using the logarithm of the distance to the shore. We used an exponential model with the nugget set to zero (Sluiter, 2012).
We characterized the landscape characteristics as the amount and connectivity of (semi-)natural vegetation (SNV) around each transect. We retrieved the amount and connectivity of SNV using the 100 m resolution CORINE Land Cover maps (European Union, 2019). We defined SNV as all land cover types other than non-vegetated urban area, arable land, sport, and leisure facilities, pastures, open water, and marine and maritime land cover (Oliver et al., 2017). We considered a single aggregated class of SNV adequate assuming that most butterfly species in our analysis can be considered habitat generalists that use a wide range of (semi-)natural vegetation types (Supplementary Table 2; Essens et al., 2017). For each transect and for each year with a land cover map available (1990, 2000, 2006, 2012, and 2018), we calculated the landscape variables within a square radius of 500, 750, and 1000 m, following earlier studies of butterfly responses to landscape characteristics (Perović et al., 2015; Oliver et al., 2017). We calculated the amount of SNV as the area of SNV within the radius relative to the total area within the radius. We calculated the connectivity of SNV according to the clumpiness index from FRAGSTATS (McGarigal et al., 2012; Fourcade et al., 2021), using the lsm_c_clumpy function of the landscapemetrics package version 0 (Hesselbarth et al., 2019). This index indicates the degree of aggregation independent of the amount of SNV (McGarigal et al., 2012). Clumpiness values range from −1, through 0 to 1, indicating landscapes in which the SNV is fully disaggregated, randomly distributed, and fully aggregated, respectively. We assumed a clumpiness index of 1 (fully aggregated) if the amount of SNV was equal to 1. By means of a linear interpolation between the years, we calculated the amount and connectivity of the SNV for the missing years. An overview of the climate and landscape variables is provided in Table 1.
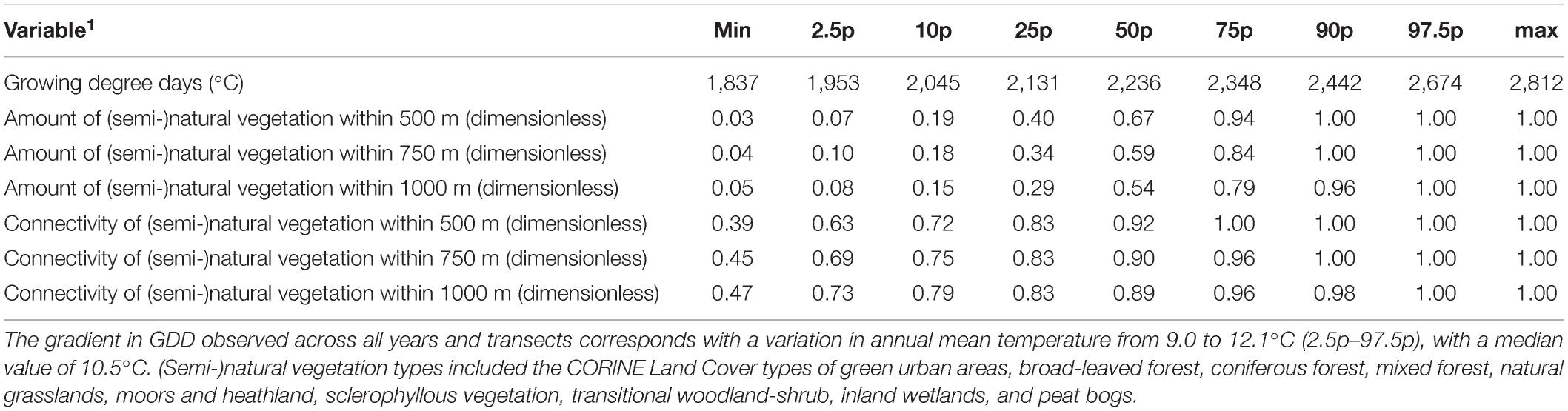
Table 1. Climate and landscape variables included in the analysis and their distributions across all transects and all years.
Hurdle Models and Variable Importance
For each species we fitted a mixed effects hurdle model with the number of butterfly weeks as response variable and a random intercept for transect to account for transect-specific conditions not represented by any of the other variables. A hurdle model consists of two components: a model that assesses the probability of occurrence (presence vs. absence) and a model that estimates the abundance, given the species is present, whereby the set of predictor variables may differ among the two model components (Zuur et al., 2009; Fourcade et al., 2017). The predictor variables included GDD, the amount and connectivity of the SVN and the interaction between the latter two and GDD, allowing responses to climate to differ among landscapes. For GDD we also included a quadratic term in order to account for possible non-linear responses to temperature change. Moreover, we added as predictors the habitat type at each transect to account for species-specific habitat preferences and the year of each observation to account for temporal trends not covered by trends in GDD or landscape characteristics (Nice et al., 2019; Northrup et al., 2019). For further analyses, we considered only species with a minimum of 160 absences and 160 presences, based on 16 predictor variables (linear and quadratic term of GDD, seven habitat types, year, amount, and connectivity of SNV and interactions between the two terms of GDD and the two landscape variables) and requiring 10 observations per predictor variable (Peduzzi et al., 1996). This left us with 30 species in the analysis (Supplementary Table 1).
We modeled occurrence probability with a binomial GLMM and a logit link function and the abundance with a truncated negative binomial GLMM and a log link function. Thus, we accounted for the excess amount of absences (zero inflation) and the overdispersion in the count data (Zuur et al., 2009; Papanikolaou et al., 2017b). To account for the differences in the lengths of the transects, we included the length as offset in the abundance-models (Zuur et al., 2009). To enhance convergence of the models, we standardized all variables to a mean of 0 and a standard deviation of 0.5 (Gelman, 2008). The Spearman correlation between all pairs of variables was below 0.6 (Supplementary Table 3), hence there was no need to exclude any variables from the model fitting.
For both occurrence and abundance, we generated all possible models from the set of predictors, including the intercept-only model, and compared them by means of the second-order Akaike information criterion (AICc). In the model fitting, the two landscape predictors (amount and connectivity of SNV) were mutually exclusive among the three radii (500, 750, 1000 m), i.e., we only allowed landscape predictors corresponding with one radius. For each model component (occurrence and abundance) and each radius we then selected the models for which the AICc-value differed less than 2 AICc units from the top-supported model (Burnham and Anderson, 2002). For each predictor variable j in each model component for each radius, we calculated the weighted average regression coefficient βj according to the method of Burnham and Anderson (2002):
with
Where Δi is equal to the difference between the AICc of the top-supported model and the AIC of model i. We assumed the regression coefficient of variable j in model i (βj,i) to be equivalent to zero when the variable was excluded from model i (Burnham and Anderson, 2002). We also determined the standard error of each weighted average regression coefficient βj, SEj, according to the method of Burnham and Anderson (2002):
Where SEj,i is the standard error of variable j in model i. The model fitting procedure thus resulted in three consensus models for each model component, i.e., one model for each radius. For further analysis we selected per model component the consensus model with the lowest AICc, based on the mean of the AICc values of the sub-models of the consensus model, weighted by wi.
We obtained the hurdle models with the glmmTMB-package version 0.2.3 in the R environment (Brooks et al., 2017; R Core Team, 2018). After model fitting, we retrieved species-specific variable importance values for each variable and model component, following the procedure of the get_variables_importance function from the biomod2 package (Thuiller et al., 2016). According to this method, we calculated the variable importance as 1 minus the Pearson’s correlation between the predictions of the full model and the predictions of the model where the variable of concern was randomized (Supplementary Figure 2).
Butterfly Responses to Climate Change
We used the consensus models of both model components of the hurdle model to calculate for each species the responses of occurrence and abundance to an increasing GDD. To this end, we first applied the consensus models to median and high values of GDD, equal to the 50 and 97.5 percentiles of the GDD values across all transects and all years (Table 1). We used the median rather than a GDD value at the low end of the GDD range, because species may show a unimodal response to warming. We then assessed the response of occurrence to an increasing GDD by calculating the odds ratio (OR), i.e., the ratio between the odds of being present at high (OddsGDDh) and median values (OddsGDDm) of GDD, which is considered an ecologically meaningful method to compare probabilities of occurrence (Rita and Komonen, 2008):
In a similar way we calculated the response of abundance to an increasing GDD as the abundance ratio (AR) between the abundance modeled at high (AbundanceGDDh) and median values (AbundanceGDDm) of GDD:
We calculated the response of occurrence and abundance to warming for nine landscape configurations, including all possible combinations of low, median, or high amount and connectivity of SNV. To that end, we set the amount and connectivity of SNV to the 2.5, 50, or 97.5 percentiles of the values across all transects and all years, considering the species-specific radius according to the best-supported consensus model (Table 1). We fixed the habitat type to equal the habitat type with the highest suitability, i.e., the highest model coefficient (βj) in the model component of interest. We fixed the year-variable at the middle of the range (year 2005).
Species Characteristics
Finally, we evaluated potential relationships between species’ responses to climate change and three species characteristics, reflecting mobility, thermal niche, and commonness within the Netherlands. For the mobility and the thermal niche we used a database of life-history traits and climatic niche traits of 397 European butterfly species (Essens et al., 2017). Essens et al. (2017) reduced six life-history traits into three principal components and five climatic niche traits into two principal components. We used the first principal components of both types of traits, which accounted for 33 and 44% of the variation in life-history and climatic niche traits, respectively. The first principal component of the life-history traits is positively correlated to dispersal capacity, voltinism (i.e., the average number of generations per year), and overwintering stage (an ordinal variable indicating how early in the season species can reproduce). We further refer to this trait as “mobility,” in accordance with Essens et al. (2017). The first principal component of the climatic niche traits is negatively correlated with annual mean temperature and the precipitation range (maximum–minimum monthly precipitation) and positively correlated with the temperature range (maximum–minimum monthly temperature). To facilitate a more intuitive interpretation, we multiplied the values of these principal component scores by −1, such that a higher score reflects a more warm-adapted species. We further refer to this trait as “thermal niche.” As an indicator of the commonness of the species within the Netherlands, we estimated the occupancy and mean abundance per species from the monitoring data, while accounting for the differences in monitoring efforts across years and habitat types. To this end, we calculated for each species the occupancy and mean abundance, given the species is present, across the transects per habitat type and per year, and then calculated the mean of each species across the habitat types and years. We used single linear regression analyses to assess the association between the odds ratio or the abundance ratio (response variable) and the mobility, thermal niche and commonness (predictor variables) for the nine landscape configurations. Because of the positively skewed distributions of the odds and abundance ratios we log-transformed them.
Results
Interactions Between Warming and SNV
For 22 out of the 30 species, we found that the odds ratio (OR) was different in sites with a low, median, and high amount or connectivity of SNV (Figure 1 and Supplementary Figure 3). Thus, for these species the response of occurrence to warming was related to the amount or connectivity of SNV. For the amount of SNV, we found positive interactions with warming for nine species (i.e., OR was larger in sites with a high than a low amount of SNV) and negative interactions for seven species (i.e., OR was larger in sites with a low than a high amount of SNV; Figure 1A). For the connectivity of SNV the majority of the interactions were positive (15 positive vs. 4 negative; Figure 1B). The positive interactions with the connectivity of SNV were mostly larger than the negative interactions (i.e., the difference between OR in sites with a low and high connectivity of SNV was generally larger for species with a positive than a negative interaction). Thus, for 55% (17 out of 30) of the species we found that a larger amount or connectivity of SNV corresponded with a larger increase or smaller decrease in the probability of occurrence in response to warming (Figure 1 and Supplementary Figure 3).
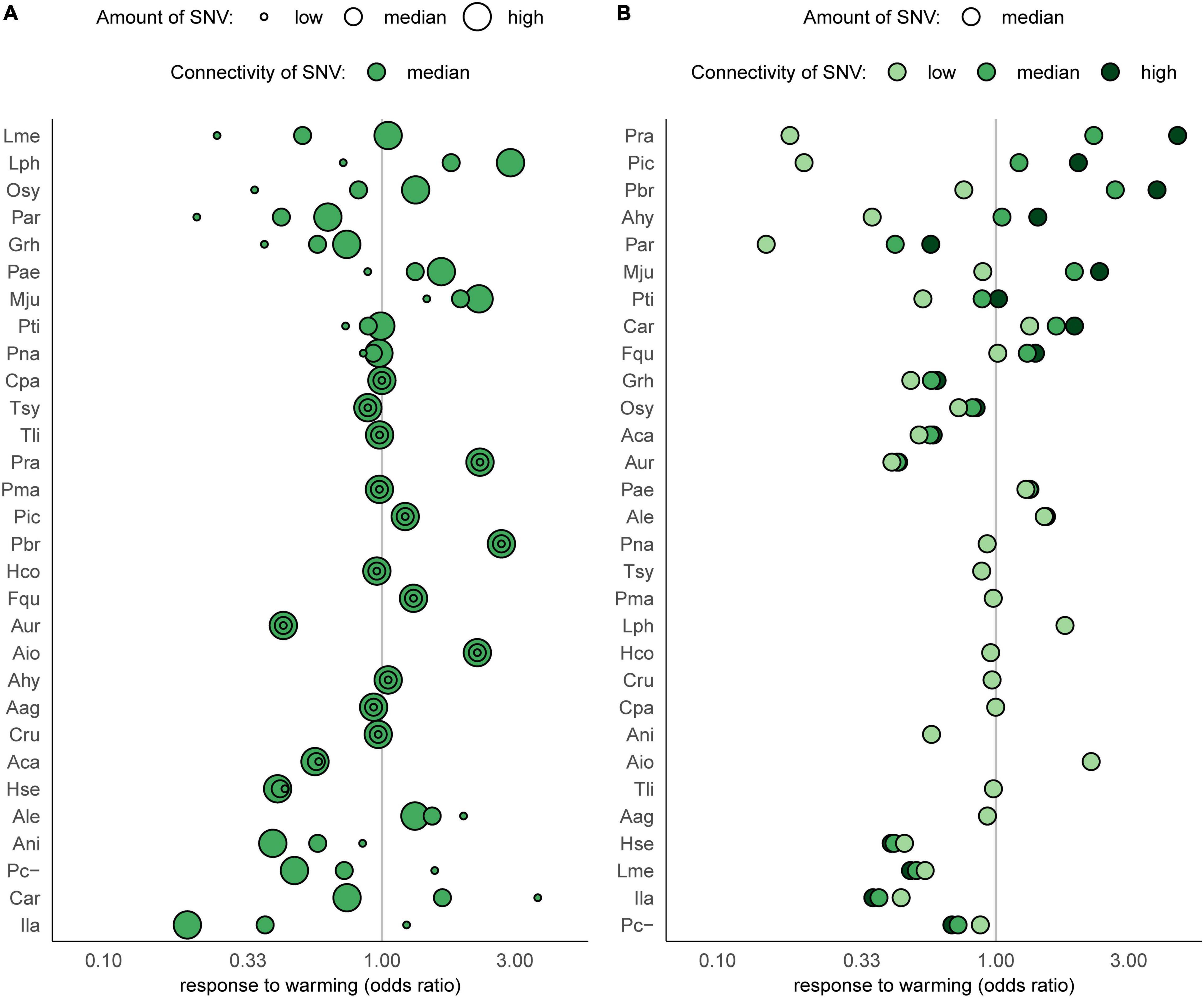
Figure 1. Response of occurrence of butterfly species to an increase in growing degree days in sites with a low, median, and high amount (A) and a low, median, and high connectivity (B) of (semi-)natural vegetation (SNV). The values of connectivity (A) and amount (B) of SNV are fixed to their median values. Response values are expressed as odds ratios (OR) thus values below and above 1 reflect negative and positive responses to warming, respectively. Species are ordered according to the sign and magnitude of the interaction with amount (A) and connectivity (B) of SNV, ranging from positive (top) to negative (bottom) interactions. The increase in GDD corresponds with an increase in annual mean temperature from 10.5 to 12.1°C (Table 1). Low, median, and high amount and connectivity represent the 2.5, 50, and 97.5 percentiles of the distributions of the respective variables across the transects and years (Table 1). The partial dependence plots of the species’ responses to warming in the nine combinations of landscape variables can be found in Supplementary Figure 5. Coefficients of the underlying models are provided in Supplementary Table 4. Aio, Aglais io; Aur, Aglais urticae; Aca, Anthocharis cardamines; Ahy, Aphantopus hyperantus; Ale, Araschnia levana; Ani, Argynnis niobe; Aag, Aricia agestis; Cru, Callophrys rubi; Car, Celastrina argiolus; Cpa, Coenonympha pamphilus; Fqu, Favonius quercus; Grh, Gonepteryx rhamni; Hco, Hesperia comma; Hse, Hipparchia semele; Ila, Issoria lathonia; Lme, Lasiommata megera; Lph, Lycaena phlaeas; Mju, Maniola jurtina; Osy, Ochlodes sylvanus; Pae, Pararge aegeria; Pbr, Pieris brassicae; Pna, Pieris napi; Pra, Pieris rapae; Par, Plebejus argus; Pc-, Polygonia c-album; Pic, Polyommatus icarus; Pma, Pyrgus malvae; Pti, Pyronia tithonus, Tli, Thymelicus lineola, Tsy, Thymelicus sylvestris.
For 26 of the 30 species, the response of abundance to warming was related to the amount or connectivity of SNV (Figure 2 and Supplementary Figure 4). For the amount of SNV, we found positive interactions with warming for 11 species and negative interactions for 8 species (Figure 2A). For the connectivity of SNV we found positive interactions for 6 species and negative interactions for 9 species (Figure 2B).
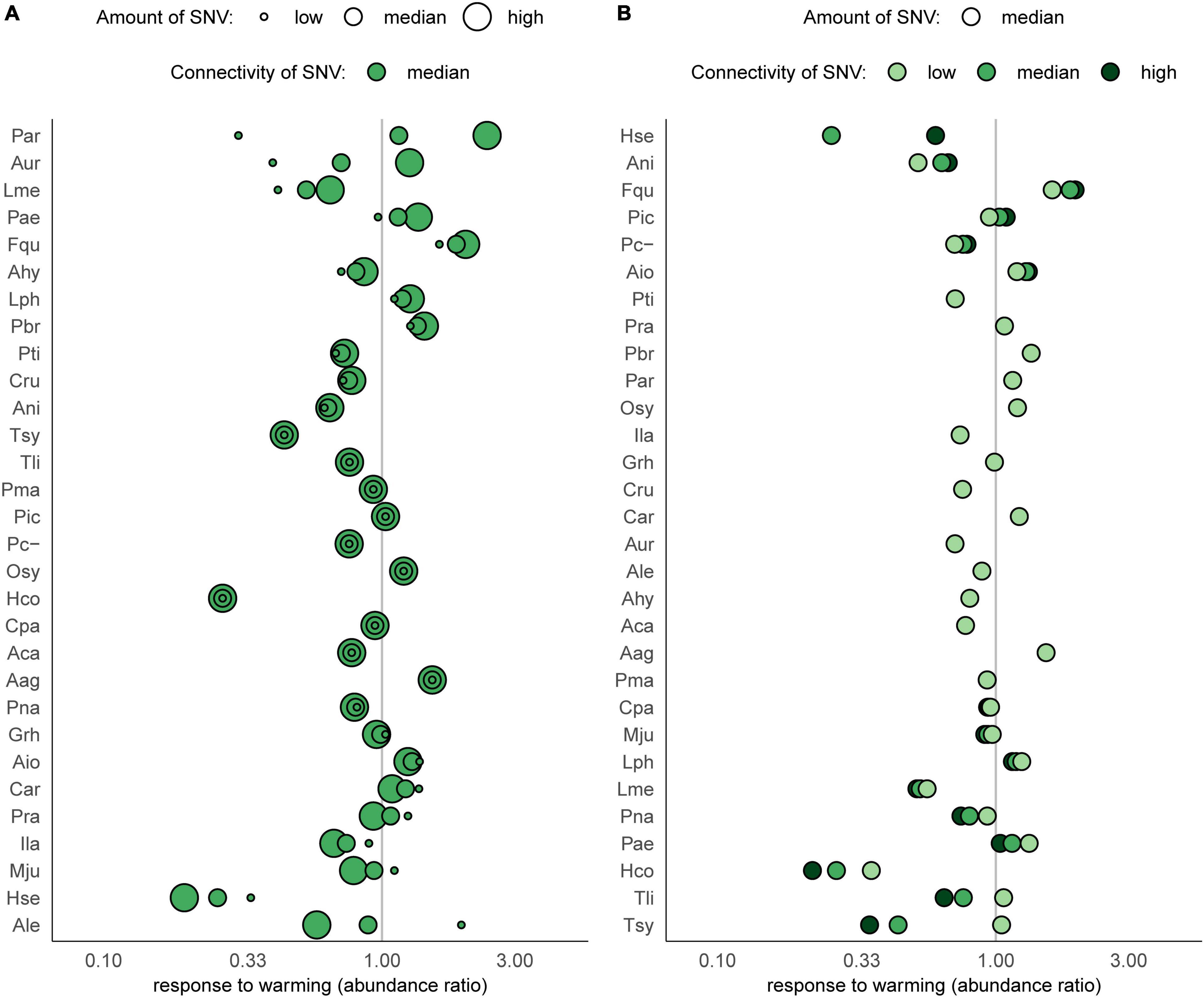
Figure 2. Response of abundance of butterfly species to an increase in growing degree days in sites with a low, median, and high amount (A) and a low, median, and high connectivity (B) of (semi-)natural vegetation (SNV). The values of connectivity (A) and amount (B) of SNV are fixed to their median values. Response values are expressed as abundance ratios (AR), thus values below and above 1 reflect negative and positive responses to warming, respectively. Species are ordered according to the sign and magnitude of the interaction with amount (A) and connectivity (B) of SNV, ranging from positive (top) to negative (bottom) interactions. The increase in GDD corresponds with an increase in annual mean temperature from 10.5 to 12.1°C (Table 1). Low, median, and high amount and connectivity represent the 2.5, 50, and 97.5 percentiles of the distributions of the respective variables across the transects and years (Table 1). The partial dependence plots of the species’ responses to warming in the nine combinations of landscape variables can be found in Supplementary Figure 5. Coefficients of the underlying models are provided in Supplementary Table 5. Aio, Aglais io; Aur, Aglais urticae; Aca, Anthocharis cardamines; Ahy, Aphantopus hyperantus; Ale, Araschnia levana; Ani, Argynnis niobe; Aag, Aricia agestis; Cru, Callophrys rubi; Car, Celastrina argiolus; Cpa, Coenonympha pamphilus; Fqu, Favonius quercus; Grh, Gonepteryx rhamni; Hco, Hesperia comma; Hse, Hipparchia semele; Ila, Issoria lathonia; Lme, Lasiommata megera; Lph, Lycaena phlaeas; Mju, Maniola jurtina; Osy, Ochlodes sylvanus; Pae, Pararge aegeria; Pbr, Pieris brassicae; Pna, Pieris napi; Pra, Pieris rapae; Par, Plebejus argus; Pc-, Polygonia c-album; Pic, Polyommatus icarus; Pma, Pyrgus malvae; Pti, Pyronia tithonus; Tli, Thymelicus lineola; Tsy, Thymelicus sylvestris.
Responses to Warming in Relation to Species Characteristics
Overall, we found weak but mostly positive relationships between species’ responses to warming and their mobility (Figure 3). Thus, mobile species tend to show stronger increases or less pronounced declines in response to warming than less mobile species (Figure 3). We further found slightly more pronounced relationships (i.e., slightly steeper slopes and lower p-values) between the response of species’ occurrence and mobility for sites with medium to high as opposed to low connectivity of the SNV (Figures 3A–C). This indicates that mobile species profit slightly more from increases in connectivity than less mobile species. We also found less pronounced relationships (i.e., shallower slopes, higher p-values, and a higher intercept) between the response of species’ occurrence and mobility for sites with high as opposed to low amounts of SNV (Figures 3A–C). This indicates that less mobile species profit slightly more from increases in the amount of SNV than mobile species. For abundance, we did not find this differentiation with connectivity and amount of SNV (Figures 3D–F).
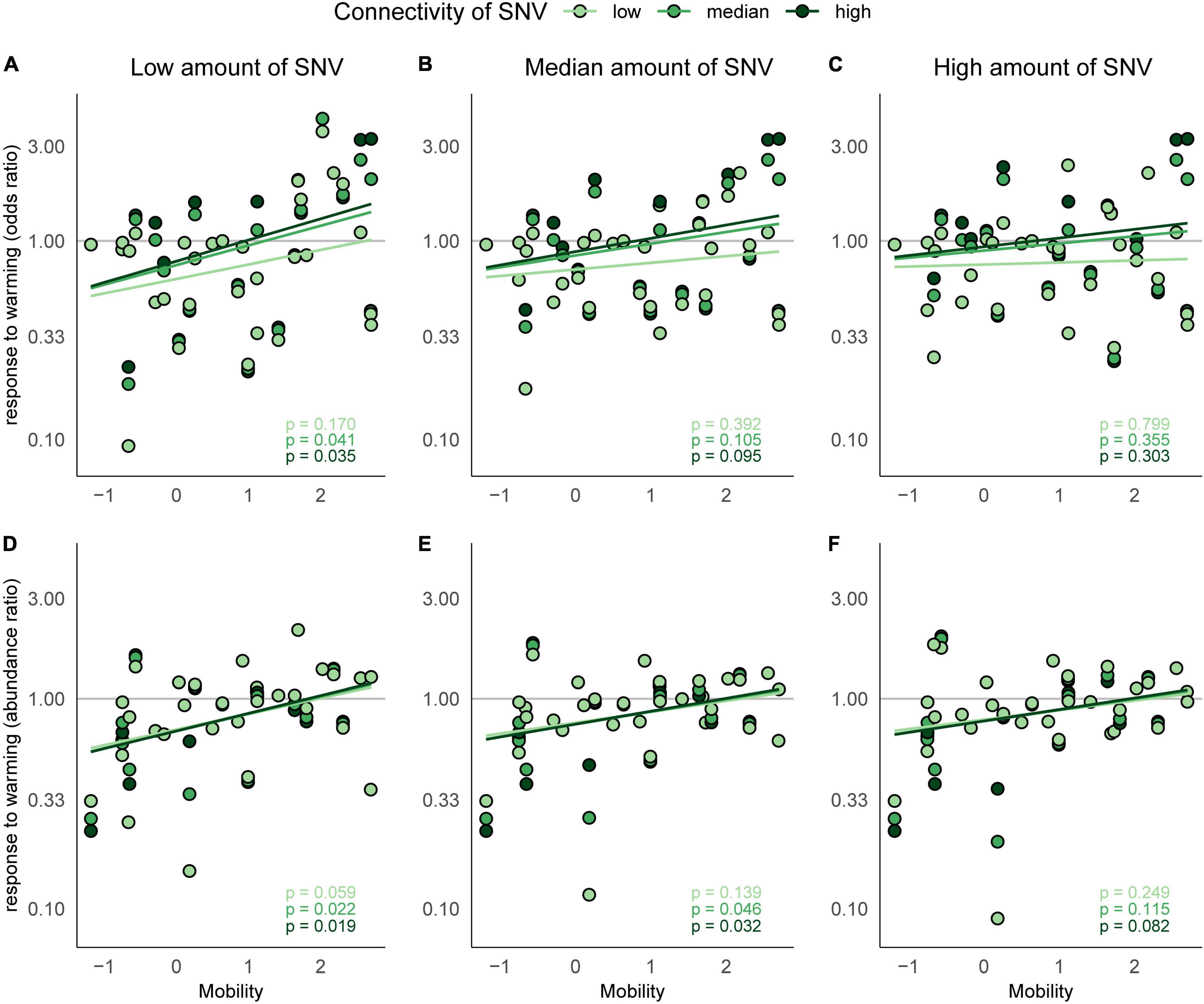
Figure 3. Response of occurrence, expressed as the odds ratio (OR) (A–C), and abundance, expressed as the abundance ratio (AR) (D–F), of butterfly species to an increase in growing degree days in relation to their mobility for nine combinations of amount and connectivity of (semi-)natural vegetation (SNV). The increase in GDD corresponds with an increase in annual mean temperature from 10.5 to 12.1°C (Table 1). The mobility is represented by the species’ scores on the first principal component of a PCA based on six life-history traits, retrieved from Essens et al. (2017). The score is positively correlated to dispersal capacity, voltinism, and overwintering stage. The p-values refer to the significance values of the slopes of the trend lines, which are calculated with linear regression analyses.
We found more positive relationships (i.e., larger slopes and lower p-values) between species’ responses of occurrence to warming and their thermal niche for sites with a median and high amount and connectivity of SNV than for sites with a low amount or connectivity of SNV (Figures 4A–C). This indicates that warm-adapted species show stronger increases or less pronounced declines in occurrence in response to warming in sites with a median to high as opposed to a low amount and connectivity of SNV. We did not find a relationship between species’ response of abundance to warming and their thermal niche (Figures 4D–F).
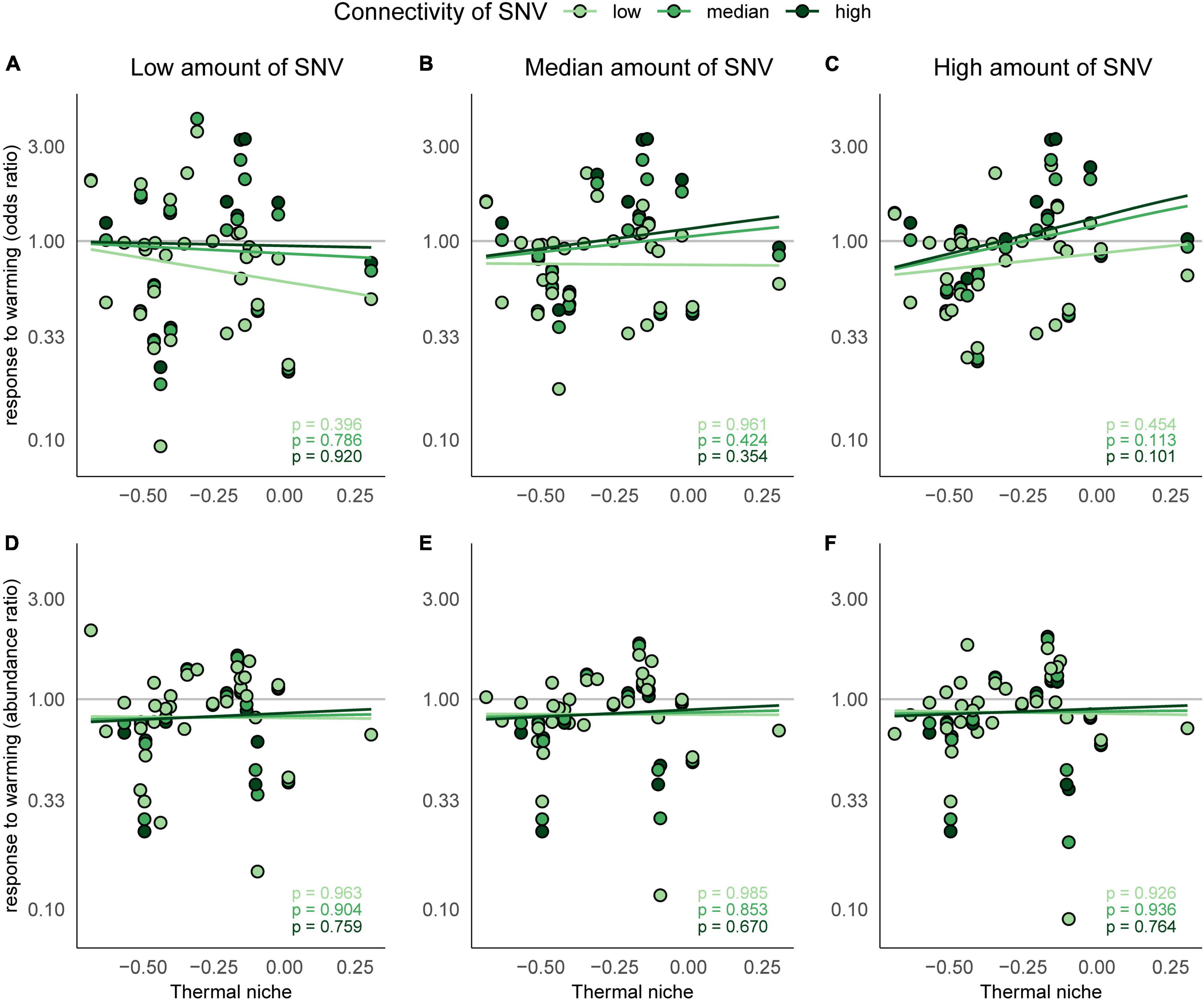
Figure 4. Response of occurrence, expressed as the odds ratio (OR) (A–C), and abundance, expressed as the abundance ratio (AR) (D–F), of butterfly species to an increase in growing degree days in relation to their thermal niche for nine combinations of amount and connectivity of (semi-)natural vegetation. The increase in GDD corresponds with an increase in annual mean temperature from 10.5 to 12.1°C (Table 1). The thermal niche is represented by the species’ scores on the first principal component of a PCA based on five climatic niche traits, retrieved from Essens et al. (2017). The score is positively correlated with the annual mean temperature and precipitation range (maximum–minimum monthly value) and negatively correlated with the temperature range (maximum–minimum monthly value). The p-values refer to the significance values of the slopes of the trend lines, which are calculated with linear regression analyses.
Finally, we found more positive relationships (i.e., larger slopes and lower p-values; Figures 5A–C) between species’ responses of occurrence to warming and their commonness for sites with a high amount and connectivity of SNV than for sites with a low amount and connectivity of SNV. This indicates that the commonest species in our dataset benefit more from a high amount and connectivity of SNV than the less common species. We did not find a relationship between species’ response of abundance to warming and their commonness (Figures 5D–F).
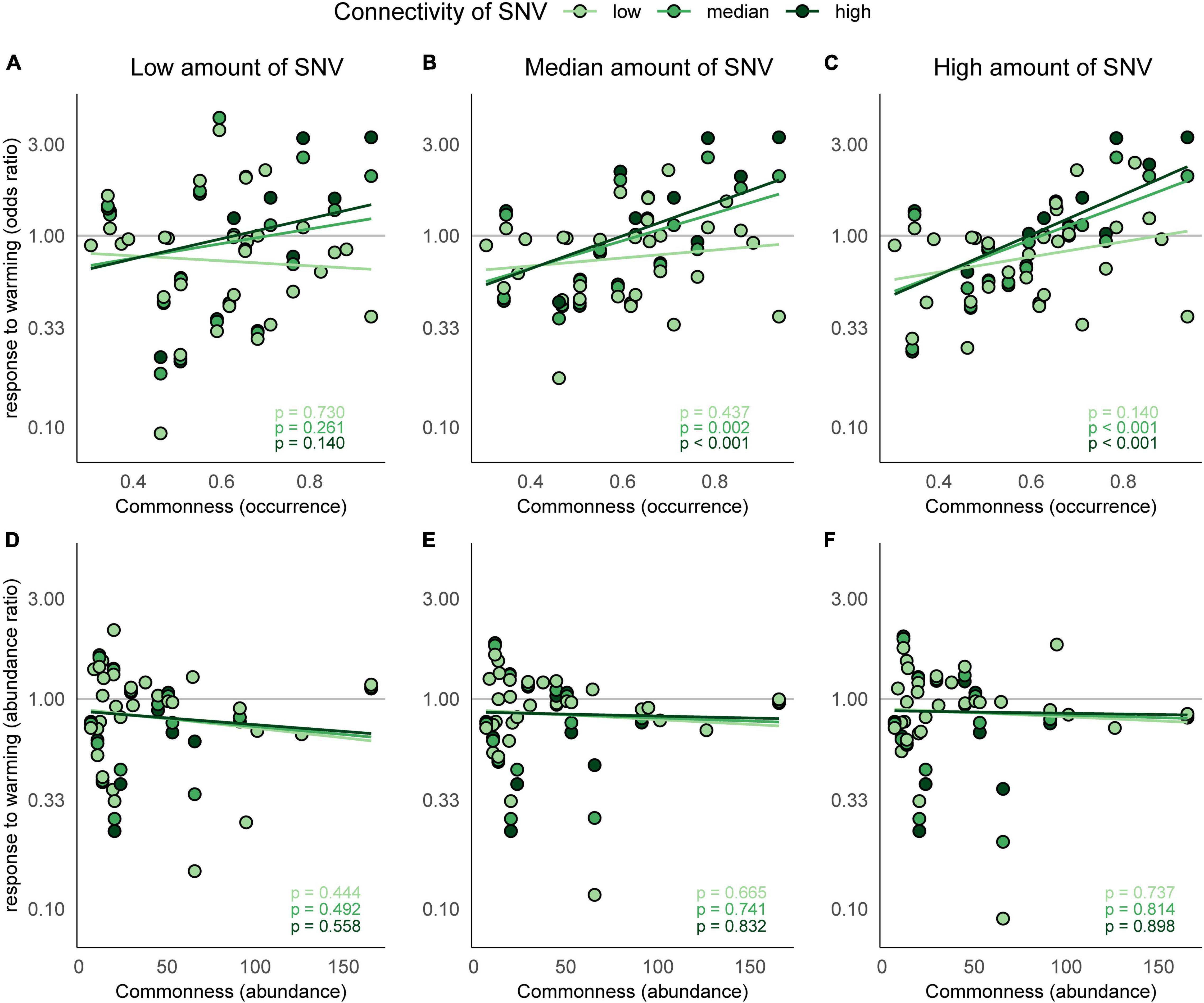
Figure 5. Response of occurrence, expressed as the odds ratio (OR) (A–C), and abundance, expressed as the abundance ratio (AR) (D–F), of butterfly species to an increase in growing degree days in relation to their commonness for nine combinations of amount and connectivity of (semi-)natural vegetation. We estimated the commonness in occurrence and abundance from the monitoring data. The p-values refer to the significance values of the slopes of the trend lines, which are calculated with linear regression analyses.
Discussion
We assessed responses to warming of 30 non-migratory butterfly species in the Netherlands in relation to landscape characteristics. We found that the amount and connectivity of (semi-)natural vegetation (SNV) in the surrounding landscape may have a positive effect on warming-related changes in the distribution of 55% of the assessed butterfly species, in particular the warm-adapted and most common ones. Similar positive interactions between warming and the amount and connectivity of the SNV have been found for the distributions of bird, mammal, and other invertebrate species (Oliver et al., 2016; Gaüzère et al., 2017; Papanikolaou et al., 2017b; Platts et al., 2019; Gaget et al., 2020). However, for the occurrence of the remaining butterfly species in our dataset, we found no or negative interactions between warming and SNV (Figure 1), highlighting the relevance of studying species-level responses. Similarly, the modifying effects of the amount and connectivity of SNV on abundance responses to warming differed among species (Figure 2).
The positive interactions we found between warming and SNV on butterfly distribution may reflect colonization and extinction dynamics. Colonization rates increase with the area and connectivity of SNV, as individuals are more likely to encounter nearby suitable patches and the costs of dispersal to other sites are smaller (Kuussaari et al., 1996; Hill et al., 2001; Dover and Settele, 2009; Bonte et al., 2012). This could facilitate the colonization of new sites where the climate has become suitable and recolonization of sites after extirpation (Estrada et al., 2016; Oliver et al., 2017; Poniatowski et al., 2020). Our results indicate that mobile species having multiple generations per year and hibernating as adult or pupae may particularly profit from SNV connectivity. These traits may favor the dispersal of mobile species through connected sites of SNV, even if low amounts of SNV are available, as these traits could increase the odds of an individual to colonize or recolonize a site (Bocedi et al., 2014; Estrada et al., 2016). Our results seem in contrast with the results of Fourcade et al. (2021), who found an overall negative relationship between the response of warm-adapted and mobile butterfly species occurrence to warming and the connectivity of SNV. However, Fourcade et al. (2021) considered landscape configuration at larger spatial extents (1–50 km from the monitoring sites) and included sites at much higher latitudes (Finland) than our study. As such, their observations may reflect that scattered habitat can facilitate range expansion across large unsuitable areas at species’ leading edge of their distribution range (Crone et al., 2019; Fourcade et al., 2021). Our results may reflect species’ responses closer to the center or trailing edge of their ranges, as the optimal annual mean temperature of 29 of the 30 studied species is below 10.5°C, the median annual mean temperature observed in the transects, which indicates that their distribution extends northward from the Netherlands (Table 1 and Supplementary Table 6; Schweiger et al., 2014).
We found negative interactions between the response of occurrence to warming and SNV in particular for the amount of SNV (Figure 1). This may reflect responses of species with more specific habitat requirements that therefore not necessarily benefit from more SNV. Although all the species in our dataset can be considered habitat generalists within Europe, the species for which we found a negative interaction were generally characterized by somewhat higher habitat specificity scores (SSI), with a median (25th–75th percentile) SSI of 20.4 (17.7–24.2), compared to species with a positive interaction, which had a median (25th–75th percentile) SSI of 13.4 (12.6–18.4) (Supplementary Table 2; Essens et al., 2017). Moreover, for some species the habitat specificity for Europe as a whole may differ from the habitat specificity in parts of their range or may change in time (Warren et al., 2001; Oliver et al., 2009). For example, Issoria lathonia, for which we found a negative interaction, is a habitat generalist in Europe (Supplementary Table 2; Essens et al., 2017), but has a preference for specific dune habitats and increasingly also for arable field margins specifically in the Netherlands. Thus, the species for which we found negative interactions between warming and the amount of SNV often have a stricter dependency on particular types of (semi-)natural or man-made vegetation types in the Netherlands compared to the species for which we found positive interactions. The negative interactions may therefore reflect that a larger amount of SNV goes on the expense of particular vegetation types required to establish new populations (Aguirre-Gutiérrez et al., 2016; Platts et al., 2019; Warren et al., 2021). Specifically, after colonization or recolonization of a site butterflies depend on the availability and density of larval host plants and nectar supply to reproduce and establish (Van Dyck et al., 2009; Fourcade and Öckinger, 2017). While habitat generalist species can often find a sufficient amount of host plants and nectar in the most common vegetation types in the Netherlands, establishment of species with a more specific habitat preference may be hampered by a lack of suitable host plants and nectar.
The lack of a consistent positive effect of SNV on the abundance response to warming contrasts with various studies that reported a buffering effect of an increased amount of SNV on the impact of climate change on abundance, including butterflies (Oliver et al., 2015, 2017) as well as other insect and bird species (Oliver et al., 2016; Papanikolaou et al., 2017b; Northrup et al., 2019). In general, a larger amount of SNV is expected to support a larger population, which is more persistent during stochastic extinctions caused by climate change (Opdam and Wascher, 2004; Oliver and Morecroft, 2014; Oliver et al., 2017). Our results suggest, however, that mitigating effects of SNV on distribution do not necessarily correspond with benefits in terms of abundance (Figures 1, 2). Differences between distribution and abundance trends have been reported by Van Dyck et al. (2009) for several common butterfly species in the Netherlands. Uncorrelated trends for abundance and distribution have also been reported for more than 170 species of moth in Scotland by Dennis et al. (2019). These differences suggest that the distribution and abundance of butterflies respond differently to environmental drivers (Fourcade and Öckinger, 2017; Fourcade et al., 2017; Dennis et al., 2019).
Possibly, the inconsistent results for abundance reflect differences in characteristics of the SNV other than mere amount or connectivity, for example the habitat quality and the microclimate (Freckleton et al., 2005; Fourcade and Öckinger, 2017; Oliver et al., 2017). The quality of the habitat within the SNV is considered to be an important driver of abundance changes in Lepidoptera (Van Dyck et al., 2009; Dennis et al., 2019). Abundance trends of butterfly species may vary across different types of (semi-)natural vegetation, possibly due to differences in nitrogen pollution levels and the availability and diversity of host plants and nectar (Van Dyck et al., 2009). Microclimate is also an important determinant of the population growth rate of butterflies (WallisDeVries et al., 2011; Oliver and Morecroft, 2014; Bladon et al., 2020) and varies strongly with vegetation type, soil type, patch size, and the distance from the edge of the patch (Morecroft et al., 1998; Herbst et al., 2007; Suggitt et al., 2011; De Frenne et al., 2019). Local environmental conditions may alter the effect of macroclimatic warming on the microclimatic conditions (WallisDeVries and van Swaay, 2006; De Frenne et al., 2021). Moreover, abundance may be impacted more strongly by warming in more homogeneous landscapes with a low environmental quality (McLaughlin et al., 2002; Oliver and Morecroft, 2014).
Many butterfly species across Western Europe, including common ones, have declined in distribution and abundance over the past decades and century (Van Dyck et al., 2009; Habel et al., 2016; van Strien et al., 2019; Warren et al., 2021), and climatic warming may lead to further declines (Supplementary Figures 3, 4; Settele et al., 2008; WallisDeVries et al., 2011; Wessely et al., 2017). Our results indicate that increasing the amount or connectivity of SNV may facilitate range expansion or mitigate extirpations in response to warming in particular for the most common and warm-adapted butterfly species in Netherlands. However, these measures are not necessarily effective for all butterfly species. Moreover, the effect of warming on abundance is not necessarily ameliorated by increasing the amount and connectivity of SNV. Consequently, increasing the amount and connectivity of SNV does not provide a “one-size-fits-all” strategy for preventing warming-induced declines in butterflies distribution and abundance. We recommend to combine an increase in the amount and connectivity of SNV with other conservation measures, such as increasing the quality of the habitat. Moreover, further declines of butterfly species can be prevented by limiting future warming through actions to mitigate climate change (Schweiger et al., 2012; Warren et al., 2021).
Data Availability Statement
The data analyzed in this study is subject to the following licenses/restrictions: The species and site-level habitat data that underlie the findings of this study are available from Dutch Butterfly Conservation upon reasonable request. Requests should be directed to the corresponding author of this manuscript (marjon.hellegers@pbl.nl). Temperature measurements are openly available from KNMI (2019). CORINE Land Cover maps are openly available from European Union (2019).
Author Contributions
MHe, CS, MHu, and AS conceptualized the research. MHe and CS conducted the analyses. All authors contributed to interpretation of the results and writing of the manuscript and approved the submitted version.
Conflict of Interest
The authors declare that the research was conducted in the absence of any commercial or financial relationships that could be construed as a potential conflict of interest.
Publisher’s Note
All claims expressed in this article are solely those of the authors and do not necessarily represent those of their affiliated organizations, or those of the publisher, the editors and the reviewers. Any product that may be evaluated in this article, or claim that may be made by its manufacturer, is not guaranteed or endorsed by the publisher.
Supplementary Material
The Supplementary Material for this article can be found online at: https://www.frontiersin.org/articles/10.3389/fevo.2022.873366/full#supplementary-material
References
Aguirre-Gutiérrez, J., Kissling, W. D., Biesmeijer, J. C., WallisDeVries, M. F., Reemer, M., and Carvalheiro, L. G. (2017). Historical changes in the importance of climate and land use as determinants of Dutch pollinator distributions. J. Biogeogr. 44, 696–707. doi: 10.1111/jbi.12937
Aguirre-Gutiérrez, J., Kissling, W. D., Carvalheiro, L. G., WallisDevries, M. F., Franzén, M., and Biesmeijer, J. C. (2016). Functional traits help to explain half-century long shifts in pollinator distributions. Sci. Rep. 6:24451. doi: 10.1038/srep24451
Antão, L. H., Bates, A. E., Blowes, S. A., Waldock, C., Supp, S. R., Magurran, A. E., et al. (2020). Temperature-related biodiversity change across temperate marine and terrestrial systems. Nat. Ecol. Evol. 4, 927–933. doi: 10.1038/s41559-020-1185-7
Bellard, C., Bertelsmeier, C., Leadley, P., Thuiller, W., and Courchamp, F. (2012). Impacts of climate change on the future of biodiversity. Ecol. Lett. 15, 365–377. doi: 10.1111/j.1461-0248.2011.01736.x
Bladon, A. J., Lewis, M., Bladon, E. K., Buckton, S. J., Corbett, S., Ewing, S. R., et al. (2020). How butterflies keep their cool: physical and ecological traits influence thermoregulatory ability and population trends. J. Animal Ecol. 89, 2440–2450. doi: 10.1111/1365-2656.13319
Bocedi, G., Zurell, D., Reineking, B., and Travis, J. M. J. (2014). Mechanistic modelling of animal dispersal offers new insights into range expansion dynamics across fragmented landscapes. Ecography 37, 1240–1253. doi: 10.1111/ecog.01041
Böhm, M., Collen, B., Baillie, J. E. M., Bowles, P., Chanson, J., Cox, N., et al. (2013). The conservation status of the world’s reptiles. Biol. Conserv. 157, 372–385. doi: 10.1016/j.biocon.2012.07.015
Bonte, D., Van Dyck, H., Bullock, J. M., Coulon, A., Delgado, M., Gibbs, M., et al. (2012). Costs of dispersal. Biol. Rev. 87, 290–312. doi: 10.1111/j.1469-185X.2011.00201.x
Bowler, D. E., Hof, C., Haase, P., Kröncke, I., Schweiger, O., Adrian, R., et al. (2017). Cross-realm assessment of climate change impacts on species’ abundance trends. Nat. Ecol. Evol. 1:0067. doi: 10.1038/s41559-016-0067
Brooks, M. E., Kristensen, K., Van Benthem, K. J., Magnusson, A., Berg, C. W., Nielsen, A., et al. (2017). glmmTMB balances speed and flexibility among packages for zero-inflated generalized linear mixed modeling. R J. 9, 378–400. doi: 10.32614/RJ-2017-066
Burnham, K. P., and Anderson, D. R. (2002). Model Selection and Multimodel Inference: A Practical Information-Theoretic Approach, 2nd Edn. New York, NY: Springer-Verlag.
Butchart, S. H. M., Walpole, M., Collen, B., van Strien, A., Scharlemann, J. P., Almond, R. E., et al. (2010). Global biodiversity: indicators of recent declines. Science 328, 1164–1168. doi: 10.1126/science.1187512
Cardoso, P., Barton, P. S., Birkhofer, K., Chichorro, F., Deacon, C., Fartmann, T., et al. (2020). Scientists’ warning to humanity on insect extinctions. Biol. Conserv. 242:108426. doi: 10.1016/j.biocon.2020.108426
R Core Team (2018). R: A Language and Environment for Statistical Computing. Vienna: R Foundation for Statistical Computing.
Crone, E. E., Brown, L. M., Hodgson, J. A., Lutscher, F., and Schultz, C. B. (2019). Faster movement in nonhabitat matrix promotes range shifts in heterogeneous landscapes. Ecology 100:e02701. doi: 10.1002/ecy.2701
De Frenne, P., Lenoir, J., Luoto, M., Scheffers, B. R., Zellweger, F., Aalto, J., et al. (2021). Forest microclimates and climate change: importance, drivers and future research agenda. Glob. Change Biol. 27, 2279–2297. doi: 10.1111/gcb.15569
De Frenne, P., Zellweger, F., Rodríguez-Sánchez, F., Scheffers, B. R., Hylander, K., Luoto, M., et al. (2019). Global buffering of temperatures under forest canopies. Nat. Ecol. Evol. 3, 744–749. doi: 10.1038/s41559-019-0842-1
Delattre, T., Baguette, M., Burel, F., Stevens, V. M., Quenol, H., and Vernon, P. (2013). Interactive effects of landscape and weather on dispersal. Oikos 122, 1576–1585. doi: 10.1111/j.1600-0706.2013.00123.x
Dennis, E. B., Brereton, T. M., Morgan, B. J. T., Fox, R., Shortall, C. R., Prescott, T., et al. (2019). Trends and indicators for quantifying moth abundance and occupancy in Scotland. J. Insect Conserv. 23, 369–380. doi: 10.1007/s10841-019-00135-z
Dover, J., and Settele, J. (2009). The influences of landscape structure on butterfly distribution and movement: a review. J. Insect Conserv. 13, 3–27. doi: 10.1007/s10841-008-9135-8
Easterling, D. R., Meehl, G. A., Parmesan, C., Changnon, S. A., Karl, T. R., and Mearns, L. O. (2000). Climate extremes: observations, modeling, and impacts. Science 289, 2068–2074. doi: 10.1126/science.289.5487.2068
Essens, T., van Langevelde, F., Vos, R. A., Van Swaay, C. A. M., and WallisDeVries, M. F. (2017). Ecological determinants of butterfly vulnerability across the European continent. J. Insect Conserv. 21, 439–450. doi: 10.1007/s10841-017-9972-4
Estrada, A., Morales-Castilla, I., Caplat, P., and Early, R. (2016). Usefulness of species traits in predicting range shifts. Trends Ecol. Evol. 31, 190–203. doi: 10.1016/j.tree.2015.12.014
European Union (2019). Copernicus Land Monitoring Service, European Environment Agency (EEA). Available online at: https://land.copernicus.eu/pan-european/corine-land-cover.
Forister, M. L., Halsch, C. A., Nice, C. C., Fordyce, J. A., Dilts, T. E., Oliver, J. C., et al. (2021). Fewer butterflies seen by community scientists across the warming and drying landscapes of the American West. Science 371, 1042–1045. doi: 10.1126/science.abe5585
Fourcade, Y., and Öckinger, E. (2017). Host plant density and patch isolation drive occupancy and abundance at a butterfly’s northern range margin. Ecol. Evol. 7, 331–345. doi: 10.1002/ece3.2597
Fourcade, Y., Ranius, T., and Ockinger, E. (2017). Temperature drives abundance fluctuations, but spatial dynamics is constrained by landscape configuration: implications for climate-driven range shift in a butterfly. J. Animal Ecol. 86, 1339–1351. doi: 10.1111/1365-2656.12740
Fourcade, Y., WallisDeVries, M. F., Kuussaari, M., van Swaay, C. A. M., Heliölä, J., and Öckinger, E. (2021). Habitat amount and distribution modify community dynamics under climate change. Ecol. Lett. 24, 950–957. doi: 10.1111/ele.13691
Freckleton, R. P., Gill, J. A., Noble, D., and Watkinson, A. R. (2005). Large-scale population dynamics, abundance–occupancy relationships and the scaling from local to regional population size. J. Animal Ecol. 74, 353–364. doi: 10.1111/j.1365-2656.2005.00931.x
Gaget, E., Galewski, T., Jiguet, F., Guelmami, A., Perennou, C., Beltrame, C., et al. (2020). Antagonistic effect of natural habitat conversion on community adjustment to climate warming in nonbreeding waterbirds. Conserv. Biol. 34, 966–976. doi: 10.1111/cobi.13453
Gaüzère, P., Princé, K., and Devictor, V. (2017). Where do they go? The effects of topography and habitat diversity on reducing climatic debt in birds. Glob. Change Biol. 23, 2218–2229. doi: 10.1111/gcb.13500
Gelman, A. (2008). Scaling regression inputs by dividing by two standard deviations. Stat. Med. 27, 2865–2873. doi: 10.1002/sim.3107
Habel, J. C., Segerer, A., Ulrich, W., Torchyk, O., Weisser, W. W., and Schmitt, T. (2016). Butterfly community shifts over two centuries. Conserv. Biol. 30, 754–762. doi: 10.1111/cobi.12656
Halsch, C. A., Shapiro, A. M., Fordyce, J. A., Nice, C. C., Thorne, J. H., Waetjen, D. P., et al. (2021). Insects and recent climate change. Proc. Natl. Acad. Sci. 118:e2002543117. doi: 10.1073/pnas.2002543117
Heikkinen, R. K., Luoto, M., Leikola, N., Pöyry, J., Settele, J., Kudrna, O., et al. (2010). Assessing the vulnerability of European butterflies to climate change using multiple criteria. Biodivers. Conserv. 19, 695–723. doi: 10.1007/s10531-009-9728-x
Heller, N. E., and Zavaleta, E. S. (2009). Biodiversity management in the face of climate change: a review of 22 years of recommendations. Biol. Conserv. 142, 14–32. doi: 10.1016/j.biocon.2008.10.006
Herbst, M., Roberts, J. M., Rosier, P. T. W., Taylor, M. E., and Gowing, D. J. (2007). Edge effects and forest water use: a field study in a mixed deciduous woodland. For. Ecol. Manag. 250, 176–186. doi: 10.1016/j.foreco.2007.05.013
Hesselbarth, M. H. K., Sciaini, M., With, K. A., Wiegand, K., and Nowosad, J. (2019). landscapemetrics: an open-source R tool to calculate landscape metrics. Ecography 42, 1648–1657. doi: 10.1111/ecog.04617
Hill, J. K., Collingham, Y. C., Thomas, C. D., Blakeley, D. S., Fox, R., Moss, D., et al. (2001). Impacts of landscape structure on butterfly range expansion. Ecol. Lett. 4, 313–321. doi: 10.1046/j.1461-0248.2001.00222.x
Hill, J. K., Thomas, C. D., Fox, R., Telfer, M. G., Willis, S. G., Asher, J., et al. (2002). Responses of butterflies to twentieth century climate warming: implications for future ranges. Proc. R. Soc. B Biol. Sci. 269, 2163–2171. doi: 10.1098/rspb.2002.2134
Jongman, R. H. G., Külvik, M., and Kristiansen, I. (2004). European ecological networks and greenways. Landsc. Urban Plan. 68, 305–319. doi: 10.1016/S0169-2046(03)00163-4
KNMI (2019). KNMI Daily Quality Controlled Climate Data KNMI, the Netherlands. Available online at: https://dataplatform.knmi.nl/dataset/etmaalgegevensknmistations-1.
Kuussaari, M., Nieminen, M., and Hanski, I. (1996). An experimental study of migration in the glanville fritillary butterfly Melitaea cinxia. J. Animal Ecol. 65, 791–801. doi: 10.2307/5677
Leung, B., Hargreaves, A. L., Greenberg, D. A., McGill, B., Dornelas, M., and Freeman, R. (2020). Clustered versus catastrophic global vertebrate declines. Nature 588, 267–271. doi: 10.1038/s41586-020-2920-6
Maxwell, S. L., Fuller, R. A., Brooks, T. M., and Watson, J. E. M. (2016). Biodiversity: the ravages of guns, nets and bulldozers. Nature 536, 143–145. doi: 10.1038/536143a
McGarigal, K., Cushman, S. A., and Ene, E. (2012). FRAGSTATS v4: Spatial Pattern Analysis Program for Categorical and Continuous Maps. Amherst: University of Massachusetts.
McLaughlin, J. F., Hellmann, J. J., Boggs, C. L., and Ehrlich, P. R. (2002). The route to extinction: population dynamics of a threatened butterfly. Oecologia 132, 538–548. doi: 10.1007/s00442-002-0997-2
Morecroft, M. D., Taylor, M. E., and Oliver, H. R. (1998). Air and soil microclimates of deciduous woodland compared to an open site. Agric. For. Meteorol. 90, 141–156. doi: 10.1016/S0168-1923(97)00070-1
Newbold, T., Adams, G. L., Robles, G. A., Boakes, E. H., Braga Ferreira, G., Chapman, A. S. A., et al. (2019). Climate and land-use change homogenise terrestrial biodiversity, with consequences for ecosystem functioning and human well-being. Emerg. Top. Life Sci. 3, 207–219. doi: 10.1042/etls20180135
Nice, C. C., Forister, M. L., Harrison, J. G., Gompert, Z., Fordyce, J. A., Thorne, J. H., et al. (2019). Extreme heterogeneity of population response to climatic variation and the limits of prediction. Glob. Change Biol. 25, 2127–2136. doi: 10.1111/gcb.14593
Northrup, J. M., Rivers, J. W., Yang, Z. Q., and Betts, M. G. (2019). Synergistic effects of climate and land-use change influence broad-scale avian population declines. Glob. Change Biol. 25, 1561–1575. doi: 10.1111/gcb.14571
Oliver, I., Dorrough, J., Doherty, H., and Andrew, N. R. (2016). Additive and synergistic effects of land cover, land use and climate on insect biodiversity. Landsc. Ecol. 31, 2415–2431. doi: 10.1007/s10980-016-0411-9
Oliver, T., Hill, J. K., Thomas, C. D., Brereton, T., and Roy, D. B. (2009). Changes in habitat specificity of species at their climatic range boundaries. Ecol. Lett. 12, 1091–1102. doi: 10.1111/j.1461-0248.2009.01367.x
Oliver, T. H., Gillings, S., Pearce-Higgins, J. W., Brereton, T., Crick, H. Q. P., Duffield, S. J., et al. (2017). Large extents of intensive land use limit community reorganization during climate warming. Glob. Change Biol. 23, 2272–2283. doi: 10.1111/gcb.13587
Oliver, T. H., Marshall, H. H., Morecroft, M. D., Brereton, T., Prudhomme, C., and Huntingford, C. (2015). Interacting effects of climate change and habitat fragmentation on drought-sensitive butterflies. Nat. Clim. Change 5, 941–945. doi: 10.1038/nclimate2746
Oliver, T. H., and Morecroft, M. D. (2014). Interactions between climate change and land use change on biodiversity: attribution problems, risks, and opportunities. Wiley Interdiscip. Rev. Clim. Change 5, 317–335. doi: 10.1002/wcc.271
Opdam, P., and Wascher, D. (2004). Climate change meets habitat fragmentation: linking landscape and biogeographical scale levels in research and conservation. Biol. Conserv. 117, 285–297. doi: 10.1016/j.biocon.2003.12.008
Palmer, G., Platts, P. J., Brereton, T. M., Chapman, J. W., Dytham, C., Fox, R., et al. (2017). Climate change, climatic variation and extreme biological responses. Philos. Trans. R. Soc. B Biol. Sci. 372:20160144. doi: 10.1098/rstb.2016.0144
Papanikolaou, A. D., Kuhn, I., Frenzel, M., and Schweiger, O. (2017a). Landscape heterogeneity enhances stability of wild bee abundance under highly varying temperature, but not under highly varying precipitation. Landsc. Ecol. 32, 581–593. doi: 10.1007/s10980-016-0471-x
Papanikolaou, A. D., Kuhn, I., Frenzel, M., and Schweiger, O. (2017b). Semi-natural habitats mitigate the effects of temperature rise on wild bees. J. Appl. Ecol. 54, 527–536. doi: 10.1111/1365-2664.12763
Peduzzi, P., Concato, J., Kemper, E., Holford, T. R., and Feinstem, A. R. (1996). A simulation study of the number of events per variable in logistic regression analysis. J. Clin. Epidemiol. 49, 1373–1379. doi: 10.1016/S0895-4356(96)00236-3
Pellissier, L., Pradervand, J. N., Pottier, J., Dubuis, A., Maiorano, L., and Guisan, A. (2012). Climate-based empirical models show biased predictions of butterfly communities along environmental gradients. Ecography 35, 684–692. doi: 10.1111/j.1600-0587.2011.07047.x
Pereira, H. M., Navarro, L. M., and Martins, I. S. (2012). Global biodiversity change: the bad, the good, and the unknown. Annu. Rev. Environ. Resour. 37, 25–50. doi: 10.1146/annurev-environ-042911-093511
Perović, D., Gámez-Virués, S., Börschig, C., Klein, A., Krauss, J., Steckel, J., et al. (2015). Configurational landscape heterogeneity shapes functional community composition of grassland butterflies. J. Appl. Ecol. 52, 505–513. doi: 10.1111/1365-2664.12394
Platts, P. J., Mason, S. C., Palmer, G., Hill, J. K., Oliver, T. H., Powney, G. D., et al. (2019). Habitat availability explains variation in climate-driven range shifts across multiple taxonomic groups. Sci. Rep. 9:15039. doi: 10.1038/s41598-019-51582-2
Poniatowski, D., Beckmann, C., Löffler, F., Münsch, T., Helbing, F., Samways, M. J., et al. (2020). Relative impacts of land-use and climate change on grasshopper range shifts have changed over time. Glob. Ecol. Biogeogr. 29, 2190–2202. doi: 10.1111/geb.13188
Pöyry, J., Luoto, M., Heikkinen, R. K., Kuussaari, M., and Saarinen, K. (2009). Species traits explain recent range shifts of finnish butterflies. Glob. Change Biol. 15, 732–743. doi: 10.1111/j.1365-2486.2008.01789.x
Rita, H., and Komonen, A. (2008). Odds ratio: an ecologically sound tool to compare proportions. Ann. Zool. Fennici 45, 66–72. doi: 10.5735/086.045.0106
Rodrigues, Y. K., and Beldade, P. (2020). Thermal plasticity in insects’ response to climate change and to multifactorial environments. Front. Ecol. Evol. 8:271. doi: 10.3389/fevo.2020.00271
Sánchez-Bayo, F., and Wyckhuys, K. A. G. (2019). Worldwide decline of the entomofauna: a review of its drivers. Biol. Conserv. 232, 8–27. doi: 10.1016/j.biocon.2019.01.020
Schipper, A. M., Belmaker, J., de Miranda, M. D., Navarro, L. M., Böhning-Gaese, K., Costello, M. J., et al. (2016). Contrasting changes in the abundance and diversity of North American bird assemblages from 1971 to 2010. Glob. Change Biol. 22, 3948–3959. doi: 10.1111/gcb.13292
Schloss, C. A., Nuñez, T. A., and Lawler, J. J. (2012). Dispersal will limit ability of mammals to track climate change in the Western Hemisphere. Proc. Natl. Acad. Sci. U.S.A. 109, 8606–8611. doi: 10.1073/pnas.1116791109
Schweiger, O., Harpke, A., Wiemers, M., and Settele, J. (2014). Climber: climatic niche characteristics of the butterflies in Europe. ZooKeys 367, 65–84. doi: 10.3897/zookeys.367.6185
Schweiger, O., Heikkinen, R. K., Harpke, A., Hickler, T., Klotz, S., Kudrna, O., et al. (2012). Increasing range mismatching of interacting species under global change is related to their ecological characteristics. Glob. Ecol. Biogeogr. 21, 88–99. doi: 10.1111/j.1466-8238.2010.00607.x
Settele, J., Kudrna, O., Harpke, A., Kühn, I., van Swaay, C., Verovnik, E., et al. (2008). Climatic Risk Atlas of European Butterflies. Sofia: Pensoft.
Sirami, C., Caplat, P., Popy, S., Clamens, A., Arlettaz, R., Jiguet, F., et al. (2017). Impacts of global change on species distributions: obstacles and solutions to integrate climate and land use. Glob. Ecol. Biogeogr. 26, 385–394. doi: 10.1111/geb.12555
Sluiter, R. (2012). Interpolation Methods for the Climate Atlas. De Bilt: Royal Netherlands Meteorological Institute (KNMI).
Suggitt, A. J., Gillingham, P. K., Hill, J. K., Huntley, B., Kunin, W. E., Roy, D. B., et al. (2011). Habitat microclimates drive fine-scale variation in extreme temperatures. Oikos 120, 1–8. doi: 10.1111/j.1600-0706.2010.18270.x
Suggitt, A. J., Wilson, R. J., Isaac, N. J. B., Beale, C. M., Auffret, A. G., August, T. A., et al. (2018). Extinction risk from climate change is reduced by microclimatic buffering. Nat. Clim. Change 8, 713–717. doi: 10.1038/s41558-018-0231-9
Thomas, J. A. (2016). Butterfly communities under threat: butterfly populations are declining worldwide as a result of habitat loss and degradation. Science 353, 216–218. doi: 10.1126/science.aaf8838
Thuiller, W., Georges, D., Engler, R., and Breiner, F. (2016). biomod2: Ensemble Platform for Species Distribution Modeling. R Package Version 3.3-7.1.
Tittensor, D. P., Walpole, M., Hill, S. L. L., Boyce, D. G., Britten, G. L., Burgess, N. D., et al. (2014). A mid-term analysis of progress toward international biodiversity targets. Science 346, 241–244. doi: 10.1126/science.1257484
Van Dyck, H., Van Strien, A. J., Maes, D., and Van Swaay, C. A. M. (2009). Declines in common, widespread butterflies in a landscape under intense human use. Conserv. Biol. 23, 957–965. doi: 10.1111/j.1523-1739.2009.01175.x
van Strien, A. J., van Swaay, C. A. M., van Strien-van Liempt, W. T. F. H., Poot, M. J. M., and WallisDeVries, M. F. (2019). Over a century of data reveal more than 80% decline in butterflies in the Netherlands. Biol. Conserv. 234, 116–122. doi: 10.1016/j.biocon.2019.03.023
van Swaay, C. A. M., Plate, C. L., and van Strien, A. J. (2002). Monitoring butterflies in the Netherlands: how to get unbiased indices. Proc. Exp. Appl. Entomol. 13, 21–27.
Wagner, D. L. (2020). Insect declines in the anthropocene. Annu. Rev. Entomol. 65, 457–480. doi: 10.1146/annurev-ento-011019-025151
WallisDeVries, M. F., Baxter, W., and Van Vliet, A. J. (2011). Beyond climate envelopes: effects of weather on regional population trends in butterflies. Oecologia 167, 559–571. doi: 10.1007/s00442-011-2007-z
WallisDeVries, M. F., and van Swaay, C. A. M. (2006). Global warming and excess nitrogen may induce butterfly decline by microclimatic cooling. Glob. Change Biol. 12, 1620–1626. doi: 10.1111/j.1365-2486.2006.01202.x
Warren, M. S., Hill, J. K., Thomas, J. A., Asher, J., Fox, R., Huntley, B., et al. (2001). Rapid responses of British butterflies to opposing forces of climate and habitat change. Nature 414, 65–69. doi: 10.1038/35102054
Warren, M. S., Maes, D., van Swaay, C. A. M., Goffart, P., Van Dyck, H., Bourn, N. A. D., et al. (2021). The decline of butterflies in Europe: problems, significance, and possible solutions. Proc. Natl. Acad. Sci. 118:e2002551117. doi: 10.1073/pnas.2002551117
Wessely, J., Hülber, K., Gattringer, A., Kuttner, M., Moser, D., Rabitsch, W., et al. (2017). Habitat-based conservation strategies cannot compensate for climate-change-induced range loss. Nat. Clim. Change 7:823. doi: 10.1038/nclimate3414
Keywords: climate adaptation, nature conservation, biodiversity, fragmentation, insects, Lepidoptera, landscape composition
Citation: Hellegers M, van Swaay CAM, van Hinsberg A, Huijbregts MAJ and Schipper AM (2022) Modulating Effects of Landscape Characteristics on Responses to Warming Differ Among Butterfly Species. Front. Ecol. Evol. 10:873366. doi: 10.3389/fevo.2022.873366
Received: 10 February 2022; Accepted: 25 March 2022;
Published: 23 May 2022.
Edited by:
David Jack Coates, Department of Biodiversity, Conservation and Attractions (DBCA), AustraliaReviewed by:
Martin Konvicka, University of South Bohemia in České Budějovice, CzechiaAndrew Wilby, Lancaster University, United Kingdom
Copyright © 2022 Hellegers, van Swaay, van Hinsberg, Huijbregts and Schipper. This is an open-access article distributed under the terms of the Creative Commons Attribution License (CC BY). The use, distribution or reproduction in other forums is permitted, provided the original author(s) and the copyright owner(s) are credited and that the original publication in this journal is cited, in accordance with accepted academic practice. No use, distribution or reproduction is permitted which does not comply with these terms.
*Correspondence: Marjon Hellegers, marjon.hellegers@pbl.nl