- 1Leibniz Institute for Zoo and Wildlife Research, Berlin, Germany
- 2Institute of Ecology, Technische Universität Berlin, Berlin, Germany
- 3Berlin-Brandenburg Institute of Advanced Biodiversity Research, Berlin, Germany
The prevailing trend of increasing urbanization and habitat fragmentation makes knowledge of species’ habitat requirements and distribution a crucial factor in conservation and urban planning. Species distribution models (SDMs) offer powerful toolboxes for discriminating the underlying environmental factors driving habitat suitability. Nevertheless, challenges in SDMs emerge if multiple data sets - often sampled with different intention and therefore sampling scheme – can complement each other and increase predictive accuracy. Here, we investigate the potential of using recent data integration techniques to model potential habitat and movement corridors for Eurasian red squirrels (Sciurus vulgaris), in an urban area. We constructed hierarchical models integrating data sets of different quality stemming from unstructured on one side and semi-structured wildlife observation campaigns on the other side in a combined likelihood approach and compared the results to modeling techniques based on only one data source - wherein all models were fit with the same selection of environmental variables. Our study highlights the increasing importance of considering multiple data sets for SDMs to enhance their predictive performance. We finally used Circuitscape (version 4.0.5) on the most robust SDM to delineate suitable movement corridors for red squirrels as a basis for planning road mortality mitigation measures. Our results indicate that even though red squirrels are common, urban habitats are rather small and partially lack connectivity along natural connectivity corridors in Berlin. Thus, additional fragmentation could bring the species closer to its limit to persist in urban environments, where our results can act as a template for conservation and management implications.
Introduction
Although urban sprawl is among the main drivers of habitat loss and degradation, cities also offer suitable habitat islands (Angold et al., 2006; Niesner et al., 2021) and can function as stepping stones (Saura et al., 2014; Lynch, 2019), if functional connectivity - exchange of genes, biomass or energy - is prevailing (Baker and Harris, 2007; Braaker et al., 2014). However, urban wildlife faces increasing challenges due to growing anthropogenic pressure and predicting the distribution of species in anthropogenic areas is therefore a crucial factor for urban wildlife conservation and should build the basis for urban planning (Fajardo et al., 2014; Casazza et al., 2021). Species distribution models (SDMs) quantify the relationship between observations of a species and the underlying environmental gradients (Araújo and Guisan, 2006; Guisan et al., 2017), and they encompass occupancy frameworks, machine-learning algorithms and hierarchical modeling frameworks (Elith and Leathwick, 2009; Kéry et al., 2010; Dorazio, 2014; Hefley and Hooten, 2016). Occupancy models can be considered as SDMs, as they allow for the estimation of the probability that an area is occupied by a species based on (environmental) covariates (Guillera-Arroita et al., 2015).
In order to receive accurate predictions by SDMs, it is important to collect many observations, which allow a spatial coverage of the heterogeneous, underlying landscape variables (Phillips et al., 2006a; Guillera-Arroita et al., 2015; Guisan et al., 2017). SDM methods are versatile, but routinely rely on information about sampling locations and protocols to model the species distribution as a function of environmental covariates (Renner et al., 2015; Guisan et al., 2017). Structured sampling, which determines spatial and temporal sampling design for participants, is a formal procedure in distribution modeling that offers high quality data by adding information about the detection process (Royle et al., 2009; Guisan et al., 2017). On the contrary, unstructured presence-only data that has been collected opportunistically is often strongly biased and needs correction before being considered for SDMs (Kramer-Schadt et al., 2013; Guillera-Arroita et al., 2015). However, results of SDMs based solely on unstructured data sets strongly differ in their predictive performance compared to structured designs (Tiago et al., 2017; Kelling et al., 2019; Planillo et al., 2021).
Referring to SDMs, making use of multiple data sources simultaneously is a promising avenue to improve ecological inferences and predictions on dynamics of wildlife populations (Koshkina et al., 2017; Farr et al., 2021). Therefore, data integration frameworks have been developed linking multiple data sources via combined likelihood estimation (Fletcher et al., 2016; Farr et al., 2019). Although unified frameworks remain rare, models using thinned point processes, which remove or retain points according to probabilistic rules, showed superior performance of combining unstructured and structured data sources compared to inferences obtained from single data sources (Dorazio, 2014; Fletcher et al., 2016; Koshkina et al., 2017; Guilbault et al., 2021). A recent hierarchical modeling approach by Renner et al. (2019) utilizes multiple data sources while accounting for overfitting and spatial dependence of observations via combined likelihood maximization.
The presence of multiple data sources is often fueled by the increasing interest of people to participate in citizen science, or, in a wider and more inclusive sense, community science projects (CS, see also: National Audubon Society., 2018), often in urban areas. With wider and more consistent availability of smartphones and internet access, CS projects offer opportunities to create valuable data sets by non-academic scientists, through mobile apps for instance (Bonney et al., 2009; McKinley et al., 2017). Even though structured designs are possible in CS projects (e.g., Sullivan et al., 2009; Louvrier et al., 2021; Planillo et al., 2021), the majority follow unstructured designs (van Strien et al., 2013; Kamp et al., 2016; Arazy and Malkinson, 2021). A common bias in unstructured CS projects is that they follow a gradient toward easily accessible areas, which are sampled intensely by participants, while other areas stay non-sampled (Boakes et al., 2010; Geldmann et al., 2016). Therefore, results of SDMs based solely on CS unstructured projects are potentially distorted and often less accurate. Recent efforts combine CS data with structured sampled data sets (Sullivan et al., 2014; Starkey et al., 2017) and use supplementary sightings to validate models from regularly sampled data sets for example, but also to train model algorithms and boost their overall predictive power in combined modeling frameworks (Fletcher et al., 2019; Renner et al., 2019; Isaac et al., 2020). Here, we assessed the predictive accuracy of combined penalized hierarchical models using semi-structured and unstructured mammal monitoring data from CS projects in the metropolitan area of Berlin, Germany. We selected Eurasian red squirrels (Sciurus vulgaris, henceforth: red squirrel) as our model species given their wide distribution across Berlin, their presence in a range of CS projects, respectively, and their unmistakable appearance, which makes them easy to identify by non-scientists, thus subsequent results could be verified reliably.
Here, we asked how multiple available data sets could be best used for constructing SDMs and how well models would perform if based only on one data source. We hypothesized that hierarchical SDM methods using data-integration techniques would outperform those only considering a single data source. We predict that models based on only one data source show a clear bias to the conditions they were collected in despite bias correction techniques. Furthermore, we hypothesized that more reliable spatial predictions would allow us to determine important movement corridors that maintain connectivity.
Methods
Study area
The city of Berlin (52°31′ N, 13°24′ E), located in Eastern Germany, is completely surrounded by the federal state Brandenburg, covers an area of 892 km2 with a population of more than 3.75 million people (Statistical Office for Berlin-Brandenburg., 2021). The green areas of Berlin vary from highly frequented parks in the dense city center to large fragmented forest remnants closer to the administrative border. The built-up area and streets contribute to 58.8% of the surface, greenspaces 12.2%, forest 18.1%, water bodies 6.7 and 4.1% agriculture (Senate Department for the Environment., 2019). Berlin is located in a zone of moderate continental climate with a low mean annual precipitation of 570 mm and mean temperatures of 8°C-10°C (Senate Department for Urban Development und Housing., 2018).
Study species
The red squirrel is a small, diurnal mammalian species with a widespread distribution throughout the forests in Europe and northern Asia (Thorington et al., 2012). The International Union for Conservation of Nature lists the species as least concern, but highlights a decreasing population trend, mainly because of loss of natural habitats (Thorington et al., 2012; Turkia et al., 2018). However, red squirrels successfully dwell in urban habitats, where they can yield high abundances (Reher et al., 2016; Hämäläinen et al., 2018, 2020). Red squirrels are an example of a common urban adapter (Fischer et al., 2015), although urban habitat suitability in the face of climate change and increasing urbanization is still an open question. Red squirrels are appreciated (e.g., fed and taken care of) by many people due to their charismatic appearance (Lurz and Bosch, 2012; Shuttleworth et al., 2015). In our study area red squirrels occur frequently, however without any reliable estimate of the population size. They are accompanied by other typical urban dwellers of all orders, including four mesocarnivores like marten species (Louvrier et al., 2021) and a community of ∼90 bird species with raptors such as northern goshawk (Accipiter gentilis) (Planillo et al., 2020).
Data collection
We analyzed red squirrel observations from two CS projects with different sampling schemes (Figure 1):
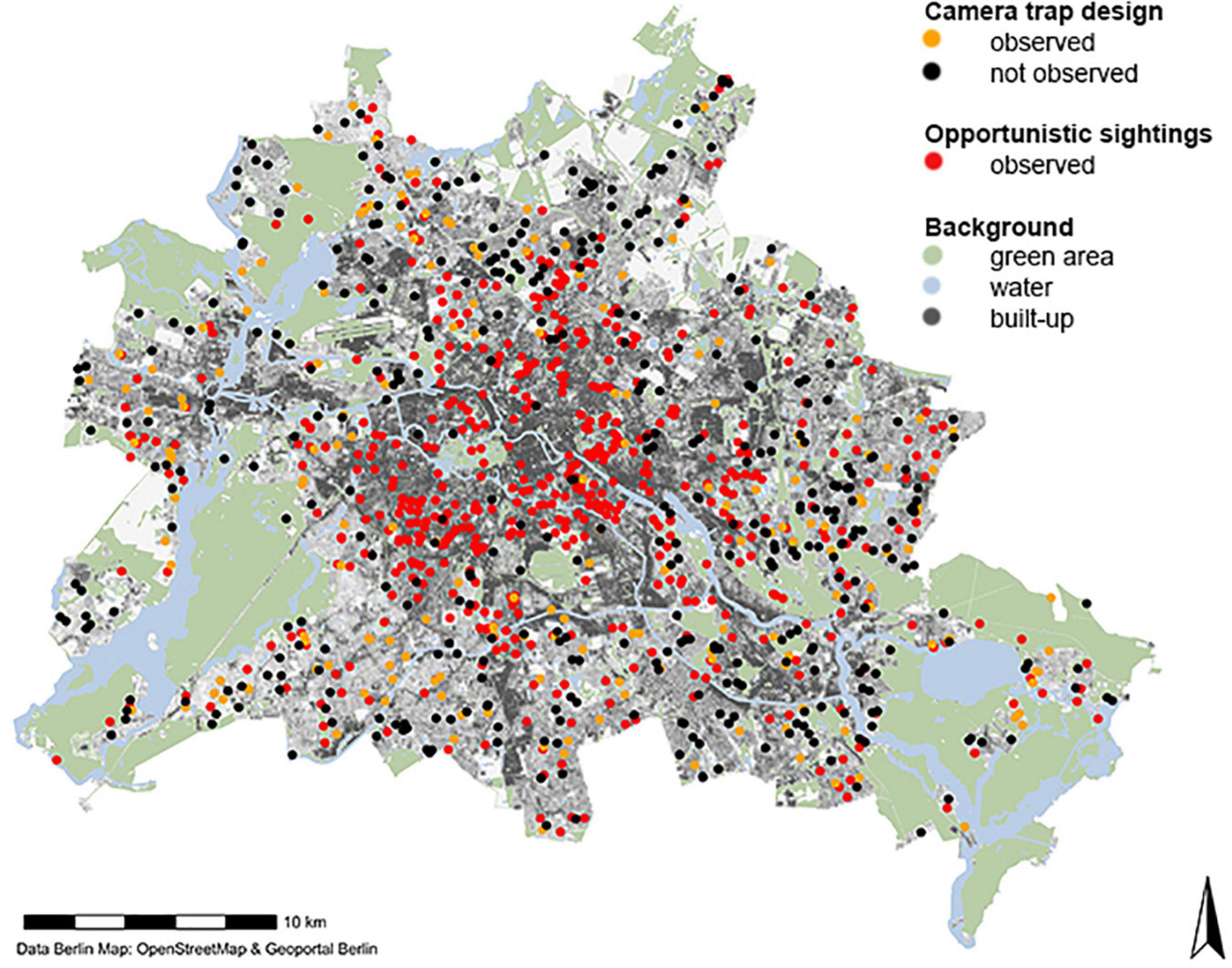
Figure 1. Study area with sampling locations of red squirrels. Camera trap surveys (black and orange dots) represent a semi-structured sampling design. Unstructured presence-only data (red dots). Background map of Berlin created with R package d6berlin (Scherer 2021).
(1) Camera trap data (n = 669 camera trap locations) formed the semi-structured data set and were collected through a CS project (“Wildtierforscher Berlin”). Camera traps were set up in five phases in private or allotment gardens: autumn 2018, spring and autumn 2019, and spring and autumn 2020. Camera trap locations were selected by dividing the study area into 2 × 2 km grid cells (n = 287) to ensure spatial independence. Camera traps were set up by participants following a strict protocol, i.e., in a corner of the garden approx. 50 cm above ground and pointing to an open area, i.e., the lawn, to capture a wide angle (Louvrier et al., 2021). Each phase consisted of four consecutive weeks, resulting in a binary detection/non-detection matrix of four weeks for each of the five phases (Table 2). (2) In parallel, unstructured presence-only data of red squirrel sightings (n = 1450), were collected through the platform ‘‘StadtWildTiere Berlin’’ (SWT)1 in another CS project, containing only opportunistic observations. The Leibniz Institute for Zoo and Wildlife Research collected and verified sightings by participants since September 2018. Data considered here included all observations until November 2020 and was further pre-processed to reduce bias of inaccurate samples (Appendix A: Presence-only data set pre-processing).
Environmental data
Environmental and anthropogenic covariates considered as explanatory variables for the occurrence of red squirrels were obtained from public online resources (Table 1). Selection of covariates was motivated by existing literature and important for red squirrel distribution (Lurz et al., 2005; Kopij, 2014; Krauze-Gryz and Gryz, 2015; Reher et al., 2016; Hämäläinen et al., 2018; Thomas et al., 2018). All covariates were tested for multicollinearity based on Pearson’s correlation and only covariates with (r) < | 0.7| (Boslaugh and Watters, 2008) were considered (Appendix A: Supplementary Figure 6).
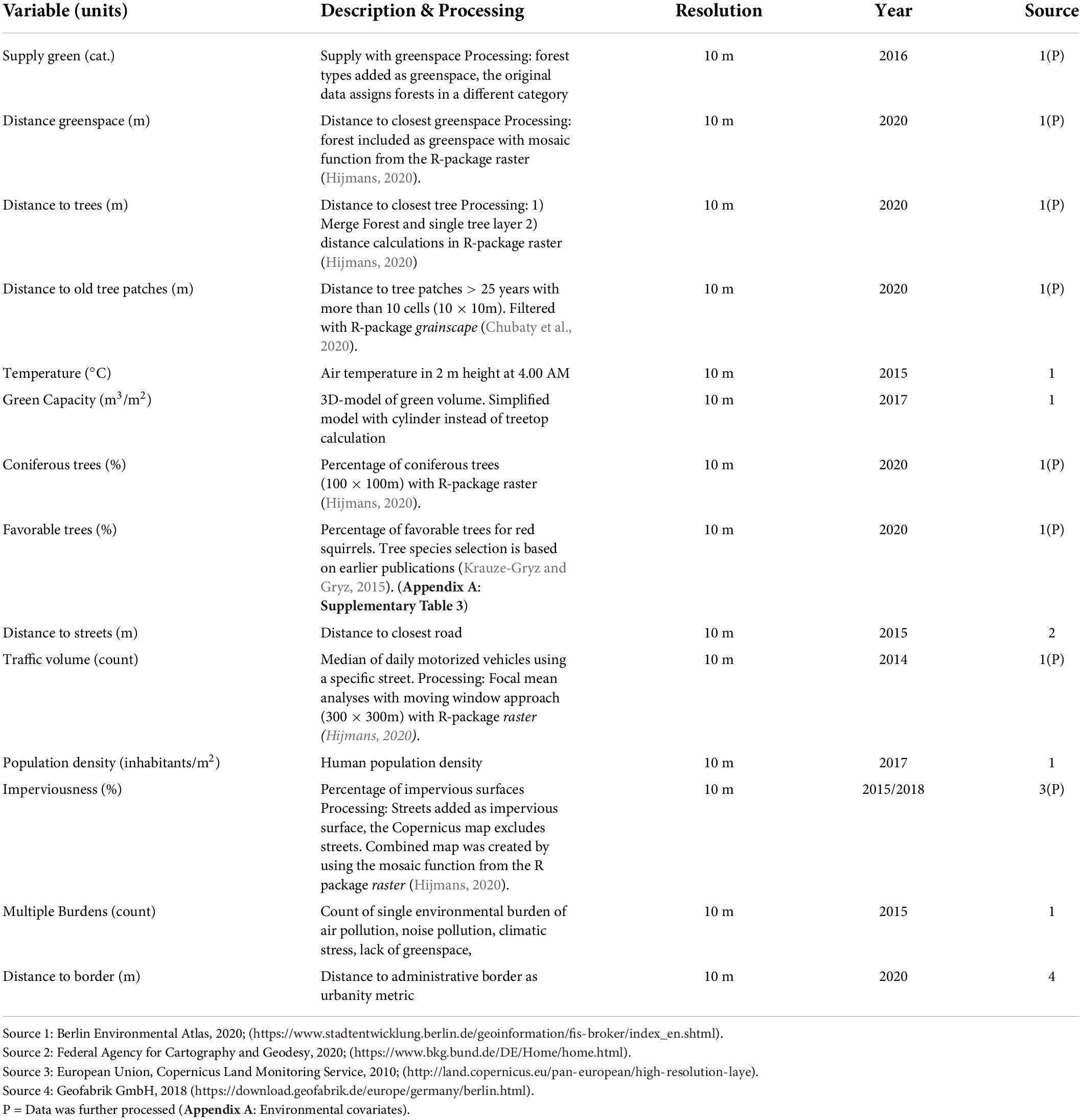
Table 1. Environmental variables considered for the SDMs (plots for all variables in Appendix A: Supplementary Figures 4, 5) based on red squirrel’s needs and human influence factors (see main text).
Species distribution models
We built five SDMs (M1-M5, Figure 2) with the semi-structured and unstructured data sets. (M1) The unstructured data set (presence-only) was fitted to MaxEnt model (Phillips et al., 2006a; M2). A second MaxEnt model was fitted including both the unstructured and the semi-structured data set. (M3) The semi-structured data set was processed in single season occupancy models accounting for imperfect detection (MacKenzie et al., 2002). (M4) A combined likelihood model based on inhomogeneous Poisson-Point-Process models (IPPPM) integrated both data sets, accounted for imperfect detection in both data sets via bias layers and overfitting by adaptive least absolute shrinkage and selection operator (LASSO) penalty and combined both likelihoods via complementary log-log functions (Renner et al., 2019). This model was taken as a reference model to compare the other modeling approaches with. (M5) The same model was also fitted as an area-interaction model (AIM) accounting for spatial dependence of observations (Renner et al., 2019). Models (M1-M5) were fitted with the same full set of environmental covariates subsequently subject to individual model selection processes based on Area Under the Curve (AUC) in MaxEnt models (M1-2); Akaike Information Criterion (AIC) in occupancy models (M3) or Bayesian Information Criterion (BIC) in combined likelihood models (M4-5) respectively. Consequently, the final models vary in their subsets of environmental variables, hence are partially different in model input parameters due to the model selection and fitting process (Appendix B: Model parameters). Furthermore, models vary in resolution, if computational burden required coarser resolutions. If stated, bilinear interpolation was conducted in the package raster (Hijmans, 2020).
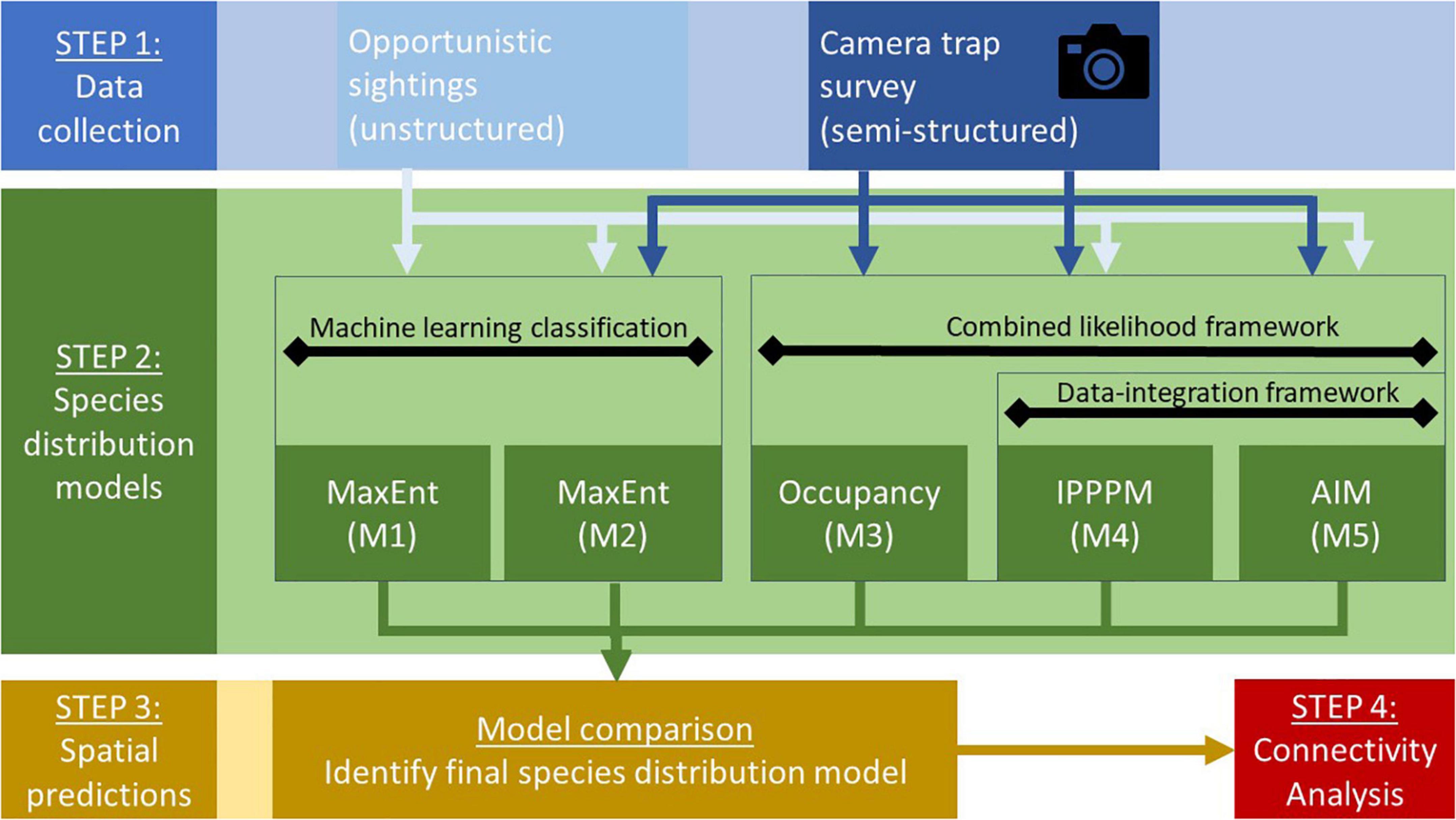
Figure 2. General workflow for generating species distribution models with different data input. *IPPPM: Inhomogeneous point process model (reference model); **AIM: Area interaction model.
MaxEnt models for presence-only data
(M1) The unstructured data set was sampled irregularly across the study area, hence we used a bias file and restricted background for the MaxEnt models to account for differences in sampling effort and area (Kramer-Schadt et al., 2013; Steen et al., 2019). To account for differences in the sampling effort, we assigned raster cells without observations with a value of 0.1 (10% probability of sampling). Accordingly, areas with overlapping observational buffers have higher probability to be sampled by participants corresponding to counted observations (Planillo et al., 2021). Additionally, we assume areas within a buffer of 500 m around every observation to be sampled by participants (approx. 5 min walking distance; see Planillo et al., 2021), restricting the background from which MaxEnt determines environmental variation (Phillips, 2008). We ran MaxEnt version 3.4.1 (Phillips et al., 2006b), included all environmental variables (resolution: 10m) with the settings as follows: maximum iterations = 2,000, maximum background points = 100,000, replicates = 10 and a logistic model output. The observation points were randomly assigned into 80% training and 20% testing sets for a 5-fold cross-validation to assess model performance and to obtain the AUC value that was used to compared MaxEnt models (Fiedling and Bell, 1997). All analyses were done in R 4.0.3 (R Core Team., 2020), MaxEnt models were built with package dismo (Hijmans et al., 2020). (M2) The second MaxEnt model was run with the previous settings, but with the additional camera trap locations with red squirrel sightings (n = 229). Camera trap locations were not considered to be biased regarding inaccurate samplings and therefore not corrected additionally.
Occupancy model for camera trap data
(M3) We fitted single season occupancy models. We chose a static model without colonization or extinction rates, because camera trap locations varied spatially between sampling seasons [see Appendix B: Occupancy model (M3)]. However, seasonal effects were considered as possible covariates on detection and occupancy. We designed 18 candidate models guided by two main hypotheses: 1) Red squirrels are highly dependent on natural resources, especially older tree patches. 2) Red squirrels prefer to avoid areas with increased human disturbance, such as impervious surfaces. We selected the most promising model within a model selection process (Appendix B: Supplementary Figures 1–3) by AIC corrected for small sample size (AICc, Burnham et al., 2002) and AICc weight (AICcw). Models with ΔAICc > 2 were considered different and models with an AICcw value greater than 0.4 were considered to have more support and relative goodness-of-fit. All occupancy analyses were conducted with unmarked (Fiske and Chandler, 2011). The model with the lowest AICc and highest AICcw was retained for predicting occupancy across the study area. The R-package AICcmodavg (Mazerolle, 2020) was used for a MacKenzie and Bailey goodness-of-fit test, an applied parametric bootstrap approach of 1,000 samples, calculating observed and expected values, a chi-square (X2) test and overdispersion parameters for the most reliable model based on the prior model selection process (MacKenzie et al., 2004; Mazerolle, 2020). In addition to the 10 m resolution of the environmental data, we applied the same set of occupancy candidate models on a coarser grid cell resolution of 500 m to be sure to capture important features of the surroundings as well.
Combined likelihood models (IPPPM + AIM)
(M4) IPPPM: For the combined modeling framework considering both data sets, we fitted the model with a combined penalized likelihood presented in Renner et al. (2019). The assumption of spatial independence of the observation locations was tested via inhomogeneous K-function simulation envelopes (Ripley, 1977; Diggle, 2013) performing Monte-Carlo simulations (n = 10) in R-package spatstat (Baddeley and Turner, 2005). (M5) AIM: A second approach was considered, because presence-only locations indicated minimal spatial dependence. Thus, we fitted an area interaction model (AIM) considering both data sets instead of an IPPPM (Renner et al., 2019) by measuring the overlap of spatial buffers around observation points within a given distance (here: 200m) and fits the likelihood with a maximum pseudo-likelihood (Besag, 1977).
Both models (M4 + M5) were run with the settings of 1,000 model fits with 25 iterations. Detection probabilities were modeled as a complementary log-log function of environmental variables. As penalty function, we used the adaptive lasso penalty pre-supplied with additional information from one prior run with the standard lasso penalty. For the canonical link, the Poisson distribution was selected as the link family and BIC was used for model selection (Schwarz, 1978). To model red squirrel distribution, we considered a smaller set of environmental covariates and used a stepwise selection of input parameters. Due to computational burden, environmental covariates were resampled in a bilinear interpolation into 100 × 100 m grid cells in the raster package (Hijmans, 2020).
Urban habitat connectivity
To identify the most important habitat patches, we used the reference model (M4), delineated patches of 500 × 500 m for connectivity and applied a threshold of MaxKappa after model evaluation with 10,000 random background points in R-package dismo (Hijmans et al., 2020). After applying threshold cut-off values, important habitat patches (n = 384) were assigned and connectivity between these was assessed.
We created a resistance map that relies on the previous habitat suitability map of the reference model and inform this cost layer with additional biological barriers and corridors based on the species’ ecology. We extended the pure habitat suitability map with additional ecological knowledge on our target species, because previous work points out that connectivity models that are based on SDMs are established but rather conservative and provide lower values of connectivity, thus rather underestimate flow between patches (Blazquez-Cabrera et al., 2016). Although connectivity measurements that are solely based on habitat suitability are potentially misleading (Scharf et al., 2018), they can be further informed by expert opinion or biological traits (Stuart et al., 2021). First, we inverted the habitat suitability values to assign low resistance values to highly suitable habitats and vice versa (Poor et al., 2012; Stevenson-Holt et al., 2014; Stuart et al., 2021). Second, we assumed all waterbodies and roads with average daily traffic equals or higher than 25,000 vehicles (approx. 17 cars/min) as complete barriers for red squirrel crossings. On the other hand, we assumed all trees, given as a concrete number by the environmental layer, and forest patches as important corridors for connectivity. We assigned the tree raster cells with low resistance values based on the number of trees, even though the underlying habitat suitability was low in those grid cells. We choose a manipulation of the inverted habitat suitability map to gain finer scaled and more appropriate corridors, which would also connect areas that are unsuitable habitats, but potentially important connectivity corridors. All calculations were conducted using Circuitscape 4.0.5 (McRae et al., 2008) with focus on all possible connections between important habitat patches (see Appendix B: Circuitscape).
Results
The semi-structured camera trap data set consisted of 669 sampling locations, of which 192 camera traps had at least one red squirrel detection. Detection rates differed over the five seasons (proportion of camera trap with detection range from 0.24 to 0.39; Table 2). The unstructured presence-only data set originally covered 1,450 observations, but spatial filtering reduced observations to 800 to be potentially included in SDMs. Underlying environmental gradients differed within the sampling locations regarding the two different sampling schemes with the semi-structured data set being distributed closer to the outskirt areas, while presence-only observations are biased towards urbanized areas (Figure 3).
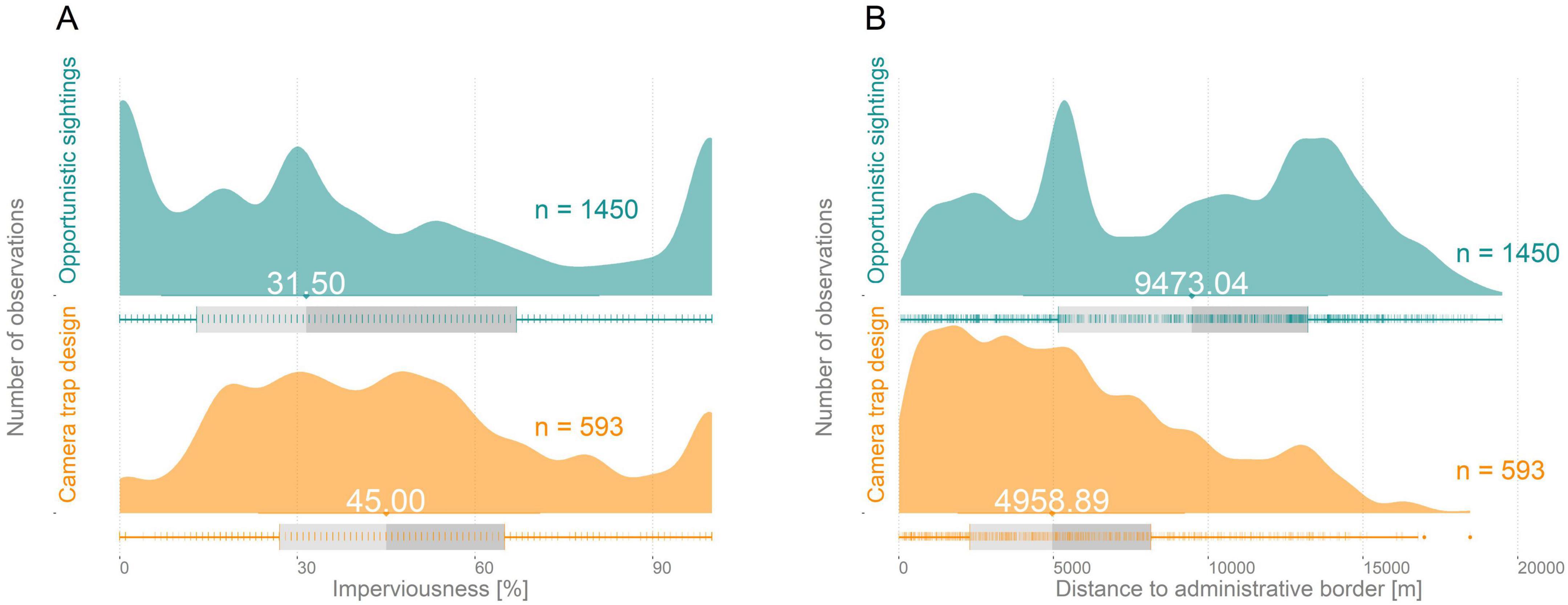
Figure 3. Examples for differences in environmental gradients covered by the different sampling data sets. (A) Impervious surfaces. (B) Distance to administrative border (see Appendix A: Supplementary Figures 7–12 for a complete comparison of sampling locations).
To test the performance of our reference model (M4), results of other model approaches were assessed in direct comparison (Figure 5):
The IPPPM (M4) corrected the sampling bias individually, which resulted in a very strong bias towards the city centre in the unstructured data set (Figure 4C) and just a minor bias in the semi-structured data set of camera traps (Figure 4B). However, results of the inhomogeneous K-function simulation envelopes still highlight minimal spatial clustering, even after spatial filtering. Consequently, area-interaction terms instead of point processes were tested additionally (M5, Appendix B: Supplementary Figure 6–8) and selected based on the K.inhom function of R-package spatstat. The adaptive lasso penalty (0.0545) was applied as a safeguard against overfitting, resulting in a reduced influence of covariates in the global model. Model estimates indicate red squirrels to be negatively related to impervious surfaces (β = −0.221 ± 0.098) and areas with high focal green capacities (β = −0.167 ± 0.076), but positively with short distance to greenspaces (β = −0.213 ± 0.089), and short distance to tree patches with older trees (β = −0.175 ± 0.076). Areas with higher human population were tended to be avoided (β = −0.07 ± 0.032), Suitable habitats were largely predicted in suburban areas, but also in larger parks, graveyards or housing areas in more urbanized area (Figure 4A).
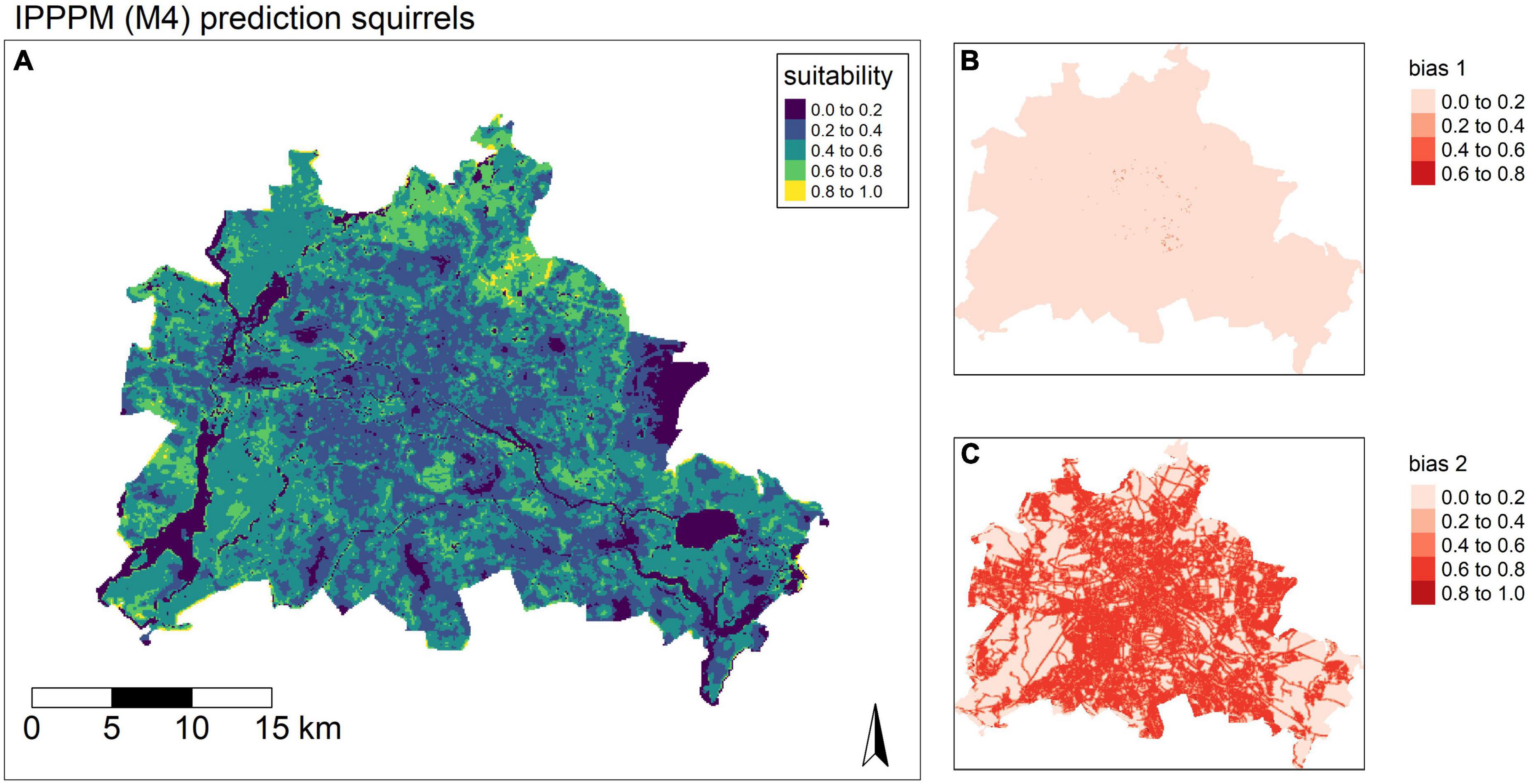
Figure 4. (A) Reference model (M4): Red squirrel distribution model. Data integration approach using an inhomogeneous Poisson-Point-Process-model and a combined likelihood maximization. (B) Bias correction for semi-structured data set. (C) Bias correction for unstructured data set.
(M1/M2) Both MaxEnt models showed similar results, but contrary to the IPPPM (M4): highly suitable habitat identified by the reference model was neglected, but urban areas that are unlikely to suit as considerable habitat were assigned with high suitability values (Figures 5A–D). M1 (sighting data; training AUC = 0.777, test AUC = 0.821) and M2 (sightings + camera trap presences; training AUC = 0.717, test AUC = 0.798) related relative probability of occurrence with young trees and impervious surfaces, but differed in their results for the variables “distance to greenspace” or “distance to administrative border” (Appendix B: MaxEnt).
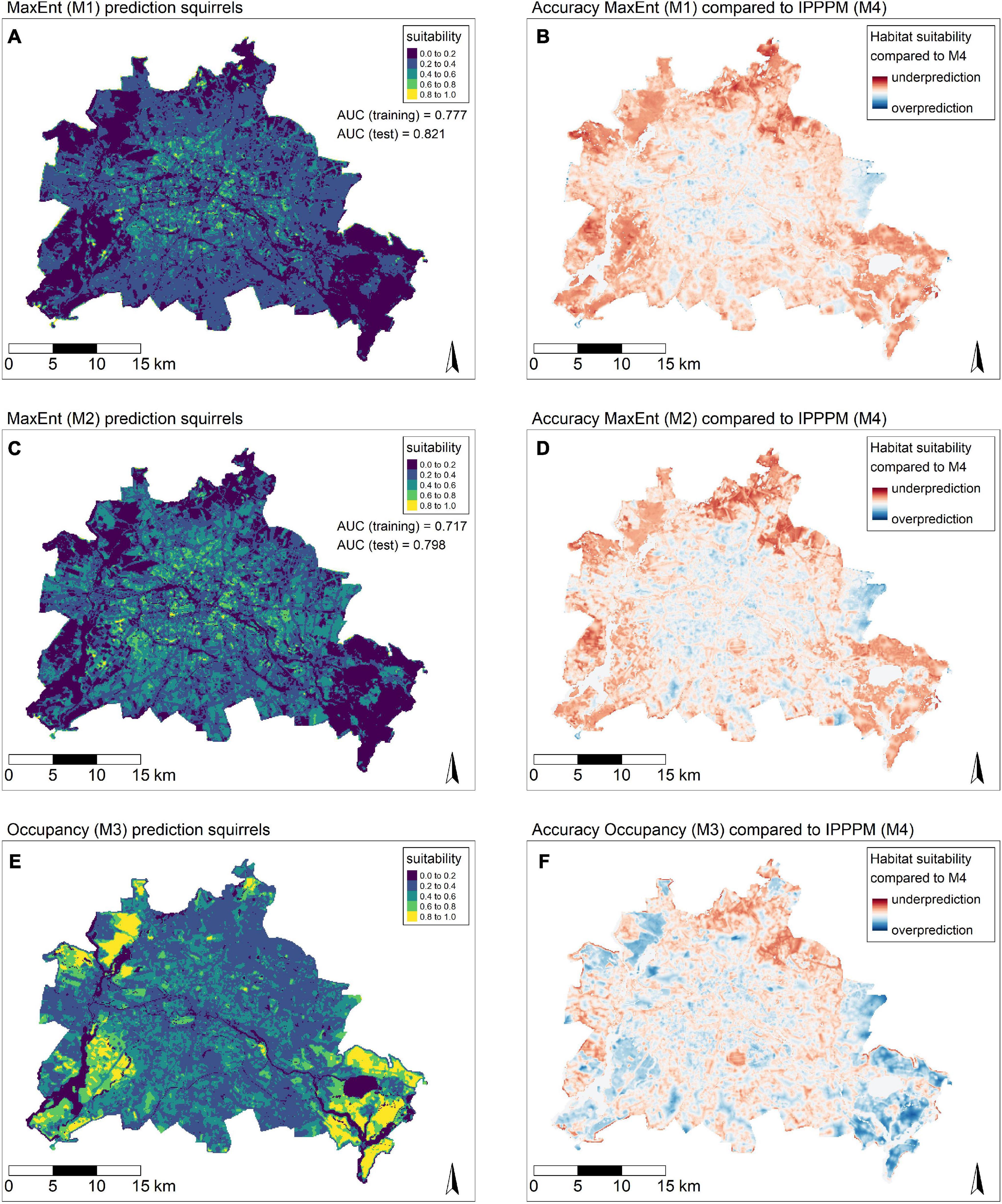
Figure 5. Comparison of the single distribution models for red squirrels in Berlin. Left side: predicted models, right side: Difference between reference model and respective model (overprediction = blue, underprediction = red). (A) MaxEnt model based on unstructured data set provided by participants. (B) Difference between reference model (M4) and MaxEnt model. (C) MaxEnt model based on unstructured data set provided by participants and camera traps with ≥ 1 observations. (D) Difference between reference model (M4) and MaxEnt model. (E) Occupancy model with semi-structured data set. (F) Difference between reference model (M4) and occupancy model.
(M3) In the occupancy model, detection probability averaged over all seasons was 0.437 (± 0.024), increasing in the spring seasons to 0.633 (± 0.036). The modeling results clearly pointed to one global model after the model selection process, indicating the same set of covariates as the reference model to be important for the species distribution. The same global model was also selected in the coarser resolution of 500 m, and had an increased support compared to all other models. Occupancy was positively influenced by high focal green capacities, areas with little impervious surfaces and higher human population densities, but negatively influenced by being close to greenspaces (Figure 5E). However, the global model should be considered with caution as the McKenzie and Bailey goodness-of-fit test showed a high overdispersion with an estimate of c-hat > 4 and a p-value <0.05, indicating the results as highly questionable and not adjustable (Mazerolle, 2020). Comparing this model to the reference model showed a clear neglect of suitable habitats in the urban parts and potential overprediction of suburban areas (Figure 5F).
The connectivity model based on M4 identified corridor networks in the whole metropolitan area of Berlin, but crucial areas for connectivity are mostly identified in areas with higher urbanization, where resources for connectivity are rare (Figure 6A). In these areas, the model highlights the importance of urban parks and higher tree densities. After applying a fixed threshold, areas assigned with high importance for connectivity, a few parks and graveyards could be identified as bottlenecks for connectivity. Other areas crucial for connectivity (Figure 6B) were located mostly at very large streets, where a crossing is only possible at very few intersections (e.g., bridges, tunnels).
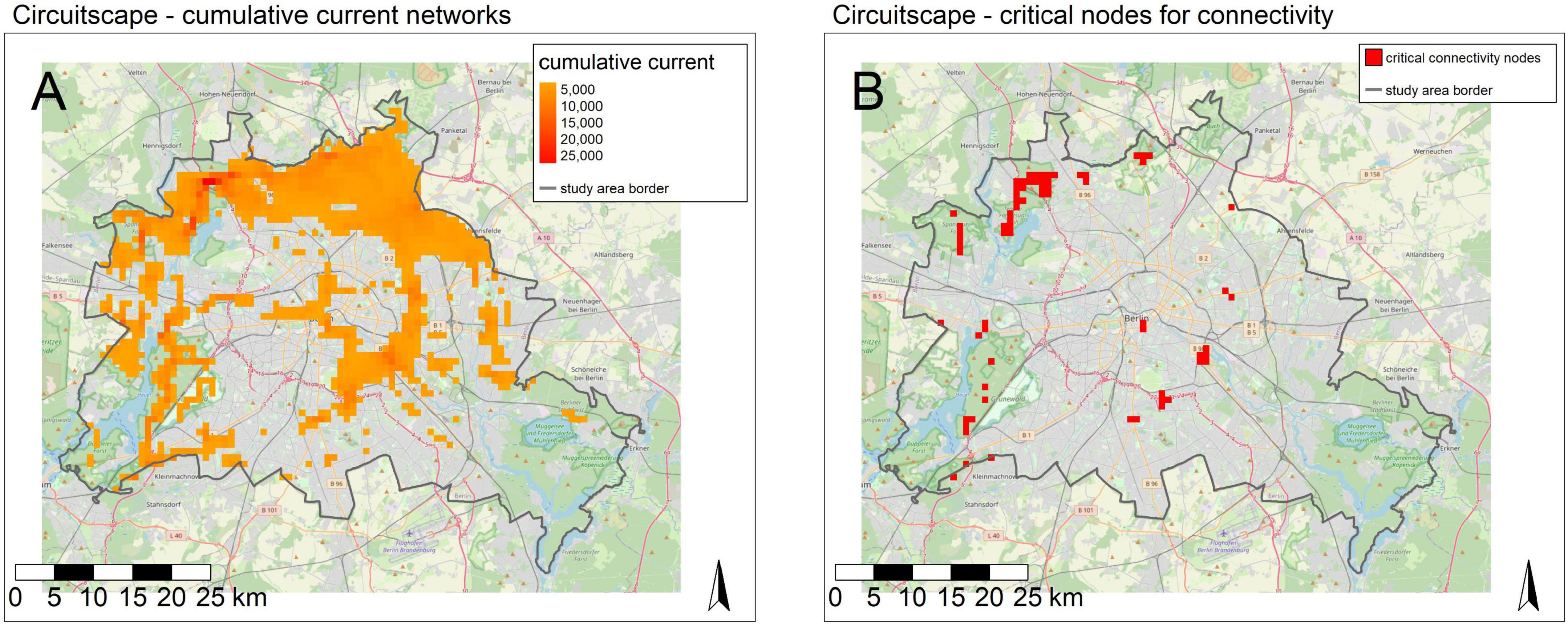
Figure 6. Circuitscape model for connectivity of important habitat patches. (A) Cumulative current network and flow between important habitat patches. (B) Identification of critical nodes after threshold application.
Discussion
In this study, we compared SDMs based on single or multiple CS data sets with different observational biases, data quality and sampling scheme. As expected, the integrated model was more likely to estimate red squirrel distribution and highlighted the advantages of considering multiple data sources for SDMs (Isaac et al., 2020). In the following, we first discuss the novelty of the statistical approach integrating CS in an urban context; and then we discuss the findings in the light of urban wildlife conservation and green infrastructure planning.
Advantages of data-integration approaches
Although data-integration techniques in SDMs are relatively recent approaches, their potential has been shown in both, simulation and applied studies: Dorazio (2014) conducted simulation-based comparisons and found even limited additional observations from a second data source to improve SDMs performance. Koshkina et al. (2017) combined occupancy data and presence-only data via IPPPM and evaluated model performance by using simulated data and subsequent application. Fletcher et al. (2016) combined camera trap data with presence-only observations obtained from a CS project, but ignored imperfect detection in the observation process.
In this study, sparse and potentially biased camera trap data alone estimated red squirrel distributions inadequately. After integrating the presence-only observations sampled by participants, we were able to demonstrate red squirrel habitat being present in both, semi-natural and urban habitats, which – to our knowledge – describes urban red squirrel habitats in Berlin adequately and is furthermore in good agreement with previous studies (Kopij, 2009, 2014; Reher et al., 2016; Thomas et al., 2018). However, model evaluation and validation for integrated models remains rather challenging (Zipkin et al., 2021), especially if known biases exist or prediction errors are likely influenced by data quantity or quality, hence traditional model assessment tools are partially in contrast to the principle of data-integration itself – to include the maximum amount of available data (Isaac et al., 2020).
Differences in suitability predictions
To understand the differences in SDMs and the potential of data-integration approaches for urban planning, it is important to consider differences in sampling schemes and accompanying biases respectively. While unstructured sightings are often biased towards easily accessible (Geldmann et al., 2016) or biodiversity-rich areas, and not evenly distributed over the sampling area, biases in camera traps designs are not as obvious and easy to account for Kays et al. (2009), Kolowski and Forrester (2017). In unstructured sightings, potential bias represents mostly spatial distortion of sampling locations and corrections are well accepted and broadly used. In our case, models based on unstructured data sets neglected suitable habitats and assigned nearly contrary results towards higher number of observations in dense urbanized areas, even though we corrected for sampling biases, as has been shown for other species in urban environments (Planillo et al., 2021). Surprisingly, results based on the semi-structured data set also failed robust distribution estimation and simplified the mechanisms of underlying environmental drivers too much, hence overestimated habitat suitability in the urban outskirt areas and omitted urban habitats in the center. This is possibly due to the bias of the CS project focusing on private gardens and allotments, which are usually not located within the city center (Louvrier et al., 2021). Additionally, aiming at detecting multiple species could have influenced detection rates noticeably (Meek et al., 2014; Dyson et al., 2019).
However, red squirrels are frequent visitors of private gardens (Baker and Harris, 2007), which compared to natural or semi-natural habitat show higher varieties of plant species and partially increased domestic animal densities (Paker et al., 2014; Louvrier et al., 2021). Hämäläinen et al. (2020) found an increase in red squirrel occurrence in urban areas explained by changes in tree species composition and attraction by bird feeders. On the other hand, Magris and Gurnell (2002) found domestic cats to be the main death cause of red squirrels, also corroborated by Fey et al. (2016). Neglecting species interaction effects hence could further bias the results and decrease reliability of SDMs (Kolowski and Forrester, 2017).
Besides spatial sampling effort, it is important to consider detectability of species in surveys (Dickinson et al., 2010; Dorazio, 2014). While species in urban areas are potentially reported more easily in unstructured surveys because they can be observed with less effort by people, it is potentially challenging to observe them in areas with more vegetation cover and higher trees typical of the outskirt of urban areas (Di Cerbo and Biancardi, 2013). Moreover, red squirrels in urban areas tend to be bolder compared to rural individuals; potentially increasing detection probabilities in urban areas, when surrounded by humans (Uchida et al., 2016, 2019; Kostrzewa and Krauze-Gryz, 2020).
Suggestions for incorporating CS projects in urban planning
The reference model using a data-integration approach combining both data sets, showed how to leverage unstructured data in SDMs, but higher accuracy of models could also be generated by improved sampling schemes beforehand (Planillo et al., 2021), for example by including absences, revisitations or transect sampling. Furthermore, sampling locations could be restricted or extended, so that sampling follows the environmental gradients more evenly and represents the background sufficiently. For example, notifications in apps could inform participants about insufficiently sampled areas and ask them to observe these to balance out the sampling design. Nevertheless, there is the risk of integrating too many additional parameters ultimately decreasing the motivation of volunteers (Rotman et al., 2012) and potentially increasing effort for project coordinators as well.
Furthermore, more structured collection of CS project observations in central registers would increase data consistency, as many projects aim for equal species with the same extent (Young et al., 2019). CS data bases, for example the semi-structured eBird project, show that constant and cross-border projects can lead to successful scientific contributions (Sullivan et al., 2009). Walker and Taylor (2017) successfully modeled population changes in migratory bird species based on eBird data, compared it to traditional survey methods and found structured CS projects promising avenues for distribution modeling. The accuracy of SDMs could also be increased by considering other modeling techniques than the ones examined in this study, for example integrating multiple species in joint species distribution models that take information from other species observations into account, or use latent variables to account for additional effects, such as species interactions or missing environmental covariates predictors (Warton et al., 2015). However, in case of multiple data sets collected with an overlapping extent, there is a clear indication that data-integration approaches still outperform single-source models (Fletcher et al., 2016; Koshkina et al., 2017; Isaac et al., 2020; Fidino et al., 2022).
Urban red squirrel habitats and connectivity
The connectivity model identified important habitat patches and bottlenecks in connectivity as expected, highlighting the importance of green structures in urban areas for connectivity for red squirrels. A major corridor crosses the city along the river Spree, combining several parks as suitable habitat patches and potentially linking the city borders. However, the corridor does not fully follow the river line due to massive impervious construction sites, indicating that urban planning has already lost a huge opportunity for wildlife-friendly urban development. This identified most critical area for connectivity could also be beneficial for other urban wildlife and act as a template for implementing crossing sections or further conservation of existing habitat patches.
While habitat fragmentation is a major threat in natural habitats (Lurz et al., 2005), there are likely other factors causing red squirrel occurrence in smaller urban habitat patches. Previous studies on red squirrel habitats found differing importance of fragmentation in urban areas. While Verbeylen et al. (2003) associated permanent occupation only for patches of at least 5 ha, Koprowski (2005) found fragmentation not necessarily being a problem for red squirrels, if the negative consequences be dampened by multiple fragmented, but high quality habitat patches or by supplementary food. Another survey conducted by Hämäläinen et al. (2018) found red squirrel occurrence even in single trees, hence a clear contrast compared to natural habitats. In general, red squirrels in urban areas tend to travel shorter distances for dispersal (Fey et al., 2016; Selonen et al., 2018), due to energy savings or supplementary food, ultimately decreasing the need for longer dispersal. In this study, the identified critical nodes depend largely on the need to travel between patches, resulting in risk to overestimate their importance for overall connectivity. Surveys of urban red squirrels in Paris, showed viable populations with high genetic variations, hence highlighted urban fragmentation to be less important (Rézouki et al., 2014). However, Thomas et al. (2018) analyzed red squirrel habitats and found lowered sensitivity to fragmentation in urban red squirrels, but highlighted that any further fragmentation would decrease populations, indicating the species being close to its limits. Furthermore, the importance of streets as barriers in connectivity shows contrary results and there is a risk of overestimating their importance; Magris and Gurnell (2002) carried out a survey and found 36% of death causes were attributed to roads, but Fey et al. (2016) showed roads would not influence dispersal movements whilst still being avoided and concluded that streets with infrequent traffic are more dangerous, because squirrels are not used to it.
Here, we used circuit theory, based on electric current flow to analyze red squirrel habitat connectivity, yet many other options of modeling are available, including least-cost path modeling, current flow, factorial least-cost path density, resistant kernels, and randomized shortest path algorithm (for an overview see: Simpkins et al., 2018; Diniz et al., 2020), with different applications. However, omnidirectional methods, such as Circuitscape, often show similar results (Phillips et al., 2021); comparing the results to empirical data showed reliable estimates of connectivity corridors, especially when the species uses random exploration of the underground, for example during dispersal movements (McClure et al., 2016). We did not have access to additional movement data (e.g., collared animals) to identify the true range of movements in Berlin (sensu LaPoint et al., 2015), hence research conducted in that context should be ultimately considered for investigating urban population dynamics of red squirrels.”
Conclusion
Combined data-integration approaches are more likely to estimate true biological distribution of species, even if the input data sets lack a structured sampling design. Our study highlights that two common modeling techniques, such as MaxEnt and occupancy, can omit or negate the habitat identified when both techniques are not combined, pointing out the importance of considering multiple data sources for urban planning when conservation decisions should be included. However, data-integration approaches for ecological studies requires unified frameworks and implementation of advanced statistical tools for model validation. Applying the data integration approach, we identified critical hotspots for red squirrels in Berlin and delineated an important corridor bridging the forests outside the urban area. In this study, we showed how data integration approaches could be used as a tool for combining multiple CS projects that often depend on different sampling schemes and efforts.
Data availability statement
Original datasets are available in a publicly accessible repository: These data can be found here: https://github.com/EcoDynIZW/Grabow_2022_FrontEcolEvol.
Ethics statement
The animal study represents a non-invasive monitoring method of free ranging animals without any impact and thus does not require approval or revision.
Author contributions
MG, JL, AP, and SK-S conceived the ideas and designed methodology. SKie, KB, MS, RH, and SKim collected the data and led the citizen science campaign. MG, JL, and AP analyzed the data. SD and TS supported project supervision. MG and SK-S led the writing of the manuscript. All authors contributed critically to the drafts and gave final approval for publication.
Funding
MG, SD, and SK-S are associated with the DFG Research Training Group “BioMove” (DFG-GRK 2118/2). JL was supported by funds from DAAD (Leibniz-DAAD Research Fellowships, 2018—57423756), I.Z.W. and by an IPODI grant from the TU Berlin. SKie and AP were supported by the German Federal Ministry of Education and Research BMBF within the Collaborative Project “Bridging in Biodiversity Science—BIBS” (funding no. 01LC1501). RH, KB, and SKim were supported by the BMBF funded project WTImpact (funding no. 01IO1725). This work was funded by the Deutsche Forschungsgemeinschaft (DFG, German Research Foundation) – project no. 491292795.
Acknowledgments
We thank Miriam Brandt for initiating and leading WTImact. We thank Moritz Wenzler for providing environmental layers and Derk Ehlert and Klemens Steiof (both SenUVK) for valuable discussions. We also like to thankful for all participating citizen scientists in and around Berlin who spent numerous hours in maintaining the camera traps and uploading and classifying camera trap photos in the WTImpact project and the citizen science participants who reported their red squirrel sightings (with pictures and videos) in the framework of the BIBS project.
Conflict of interest
The authors declare that the research was conducted in the absence of any commercial or financial relationships that could be construed as a potential conflict of interest.
Publisher’s note
All claims expressed in this article are solely those of the authors and do not necessarily represent those of their affiliated organizations, or those of the publisher, the editors and the reviewers. Any product that may be evaluated in this article, or claim that may be made by its manufacturer, is not guaranteed or endorsed by the publisher.
Supplementary material
The Supplementary Material for this article can be found online at: https://www.frontiersin.org/articles/10.3389/fevo.2022.881247/full#supplementary-material
Footnotes
References
Angold, P. G., Sadler, J. P., Hill, M. O., Pullin, A., Rushton, S., Austin, K., et al. (2006). Biodiversity in urban habitat patches. Sci.Total Environ. 360, 196–204. doi: 10.1016/j.scitotenv.2005.08.035
Araújo, M. B., and Guisan, A. (2006). Five (or so) challenges for species distribution modelling. J. Biogeogr. 33, 1677–1688. doi: 10.1111/j.1365-2699.2006.01584.x
Arazy, O., and Malkinson, D. (2021). A Framework of Observer-Based Biases in Citizen Science Biodiversity Monitoring: Semi-Structuring Unstructured Biodiversity Monitoring Protocols. Front. Ecol. Evol. 9:693602. doi: 10.3389/fevo.2021.693602
Baddeley, A., and Turner, R. (2005). spatstat An R Package for Analyzing Spatial Point Patterns. J. Stat. Soft. 12, 1–42. doi: 10.18637/jss.v012.i06
Baker, P. J., and Harris, S. (2007). Urban mammals: what does the future hold? An analysis of the factors affecting patterns of use of residential gardens in Great Britain. Mamm. Rev. 37, 297–315. doi: 10.1111/j.1365-2907.2007.00102.x
Besag, J. (1977). Some methods of statistical analysis for spatial data. Bull. Int. Statist. Institute 47, 77–92. doi: 10.1007/s10661-011-2005-y
Blazquez-Cabrera, S., Gastón, A., Beier, P., Garrote, G., Angel Simon, M., Saura, S., et al. (2016). Influence of separating home range and dispersal movements on characterizing corridors and effective distances. Landsc. Ecol. 31, 2355–2366. doi: 10.1007/s10980-016-0407-5
Boakes, E. H., McGowan, P. J. K., Fuller, R. A., Chang-qing, D., Clark, N. E., O’Connor, K., et al. (2010). Distorted views of biodiversity: spatial and temporal bias in species occurrence data. PLoS Biol. 8:e1000385. doi: 10.1371/journal.pbio.1000385
Bonney, R., Cooper, C. B., Dickinson, J., Kelling, S., Phillips, T., Rosenberg, K. V., et al. (2009). Citizen Science: A Developing Tool for Expanding Science Knowledge and Scientific Literacy. BioScience 59, 977–984. doi: 10.1525/bio.2009.59.11.9
Boslaugh, S., and Watters, P. A. (2008). Statistics in a Nutshell: A Desktop Quick Reference, Chap. 7. Sebastopol, CA: O’Reilly Media.
Braaker, S., Moretti, M., Boesch, R., Ghazoul, J., Obrist, M. K., and Bontadina, F. (2014). Assessing habitat connectivity for ground-dwelling animals in an urban environment. Ecol. Appl. 24, 1583–1595. doi: 10.1890/13-1088.1
Burnham, K. P., Burnham, K. P. M. S. A. I, and Anderson, D. R. (2002). Model Selection and Inference: A Practical Information-Theoretic Approach, 2nd Edn. New York, NY: Springer-Verlag, doi: 10.1007/b97636
Casazza, G., Abeli, T., Bacchetta, G., Dagnino, D., Fenu, G., Gargano, D., et al. (2021). Combining conservation status and species distribution models for planning assisted colonisation under climate change. J. Ecol. 109, 2284–2295. doi: 10.1111/1365-2745.13606
Chubaty, A. M., Galpern, P., and Doctolero, S. C. (2020). The r toolbox grainscape for modelling and visualizing landscape connectivity using spatially explicit networks. Methods Ecol Evol. 11, 591–595. doi: 10.1111/2041-210X.13350
Di Cerbo, A. R., and Biancardi, C. M. (2013). Monitoring small and arboreal mammals by camera traps: effectiveness and applications. Acta Theriol. 58, 279–283. doi: 10.1007/s13364-012-0122-9
Dickinson, J. L., Zuckerberg, B., and Bonter, D. N. (2010). Citizen Science as an Ecological Research Tool: Challenges and Benefits. Annu. Rev. Ecol. Evol. Syst. 41, 149–172. doi: 10.1146/annurev-ecolsys-102209-144636
Diniz, M., Cushman, S., Machado, R., and De Marco Júnior, P. (2020). Landscape connectivity modeling from the perspective of animal dispersal. Landsc. Ecol. 35, 41–58. doi: 10.1007/s10980-019-00935-3
Dorazio, R. M. (2014). Accounting for imperfect detection and survey bias in statistical analysis of presence-only data. Glob. Ecol. Biogeogr. 23, 1472–1484. doi: 10.1111/geb.12216
Dyson, K., Ziter, C., Fuentes, T. L., and Patterson, M. S. (2019). Conducting urban ecology research on private property: advice for new urban ecologists. J. Urban Ecol. 5:juz001. doi: 10.1093/jue/juz001
Elith, J., and Leathwick, J. R. (2009). Species Distribution Models: Ecological Explanation and Prediction Across Space and Time. Annu. Rev. Ecol. Evol. Syst. 40, 677–697. doi: 10.1146/annurev.ecolsys.110308.120159
Fajardo, J., Lessmann, J., Bonaccorso, E., Devenish, C., and Muñoz, J. (2014). Combined use of systematic conservation planning, species distribution modelling, and connectivity analysis reveals severe conservation gaps in a megadiverse country (Peru). PLoS One 9:e114367. doi: 10.1371/journal.pone.0114367
Farr, M. T., Green, D. S., Holekamp, K. E., Roloff, G. J., and Zipkin, E. F. (2019). Multispecies hierarchical modeling reveals variable responses of African carnivores to management alternatives. Ecol. Appl. 29:e01845. doi: 10.1002/eap.1845
Farr, M. T., Green, D. S., Holekamp, K. E., and Zipkin, E. F. (2021). Integrating distance sampling and presence-only data to estimate species abundance. Ecology 102:e03204. doi: 10.1002/ecy.3204
Fey, K., Hämäläinen, S., and Selonen, V. (2016). Roads are no barrier for dispersing red squirrels in an urban environment. Behav. Ecol. 27, 741–747. doi: 10.1093/beheco/arv215
Fidino, M., Lehrer, E. W., Kay, C. A. M., Yarmey, N. T., Murray, M. H., Fake, K., et al. (2022). Integrated Species Distribution Models Reveal Spatiotemporal Patterns of Human–Wildlife Conflict. Ecol.Appl. 10:e2647. doi: 10.1002/eap.2647
Fiedling, A. H., and Bell, J. (1997). A review of methods for the assessment of prediction errors in conservation presence/absence models. Envir. Conserv. 24, 38–49. doi: 10.1017/S0376892997000088
Fischer, J. D., Schneider, S. C., Ahlers, A. A., and Miller, J. R. (2015). Categorizing wildlife responses to urbanization and conservation implications of terminology. Conserv. Biol. J. Soc. Conserv. Biol. 29, 1246–1248. doi: 10.1111/cobi.12451
Fiske, I., and Chandler, R. (2011). unmarked An R Package for Fitting Hierarchical Models of Wildlife Occurrence and Abundance. J. Stat. Soft 43, 1–23. doi: 10.18637/jss.v043.i10
Fletcher, R., Hefley, T. J., Robertson, E. P., Zuckerberg, B., McCleery, R. A., and Dorazio, R. M. (2019). A practical guide for combining data to model species distributions. Ecology 100:e02710. doi: 10.1002/ecy.2710
Fletcher, R. J., McCleery, R. A., Greene, D. U., and Tye, C. A. (2016). Integrated models that unite local and regional data reveal larger-scale environmental relationships and improve predictions of species distributions. Landsc. Ecol. 31, 1369–1382. doi: 10.1007/s10980-015-0327-9
Geldmann, J., Heilmann-Clausen, J., Holm, T. E., Levinsky, I., Markussen, B., Olsen, K., et al. (2016). What determines spatial bias in citizen science? Exploring four recording schemes with different proficiency requirements. Divers. Distrib. 22, 1139–1149. doi: 10.1111/ddi.12477
Guilbault, E., Renner, I., Mahony, M., and Beh, E. (2021). How to make use of unlabeled observations in species distribution modeling using point process models. Ecol. Evol. 11, 5220–5243. doi: 10.1002/ece3.7411
Guillera-Arroita, G., Lahoz-Monfort, J. J., Elith, J., Gordon, A., Kujala, H., Lentini, P. E., et al. (2015). Is my species distribution model fit for purpose? Matching data and models to applications. Glob. Ecol. Biogeogr. 24, 276–292. doi: 10.1111/geb.12268
Guisan, A., Thuiller, W., and Zimmermann, N. E. (2017). Habitat Suitability and Distribution Models: With Applications in R. Ecology, Biodiversity and Conservation. Cambridge, CA: Cambridge University Press.
Hämäläinen, S., Fey, K., and Selonen, V. (2018). Habitat and nest use during natal dispersal of the urban red squirrel (Sciurus vulgaris). Landsc. and Urban Planning 169, 269–275. doi: 10.1016/j.landurbplan.2017.09.011
Hämäläinen, S., Fey, K., and Selonen, V. (2020). Search strategies in rural and urban environment during natal dispersal of the red squirrel. Behav Ecol Sociobiol 74:124. doi: 10.1007/s00265-020-02907-z
Hefley, T. J., and Hooten, M. B. (2016). Hierarchical Species Distribution Models. Curr Landsc. Ecol Rep 1, 87–97. doi: 10.1007/s40823-016-0008-7
Hijmans, R. J., Phillips, S., Leathwick, J., and Elith, J. (2020). dismo: Species Distribution Modeling R package version 1.3-3.
Isaac, N. J. B., Jarzyna, M. A., Keil, P., Dambly, L. I., Boersch-Supan, P. H., Browning, E., et al. (2020). Data Integration for Large-Scale Models of Species Distributions. Trends Ecol. Evol. 35, 56–67. doi: 10.1016/j.tree.2019.08.006
Kamp, J., Oppel, S., Heldbjerg, H., Nyegaard, T., and Donald, P. F. (2016). Unstructured citizen science data fail to detect long-term population declines of common birds in Denmark. Divers Distrib. 22, 1024–1035. doi: 10.1111/ddi.12463
Kays, R., Kranstauber, B., Jansen, P., Carbone, C., Rowcliffe, M., Fountain, T., et al. (2009). Camera traps as sensor networks for monitoring animal communities. IEEE Confer. Local Comput. Netw. 10, 811–818. doi: 10.1109/LCN.2009.5355046
Kelling, S., Johnston, A., Bonn, A., Fink, D., Ruiz-Gutierrez, V., Bonney, R., et al. (2019). Using Semistructured Surveys to Improve Citizen Science Data for Monitoring Biodiversity. BioScience 69, 170–179. doi: 10.1093/biosci/biz010
Kéry, M., Royle, J. A., Schmid, H., Schaub, M., Volet, B., Häfliger, G., et al. (2010). Site-occupancy distribution modeling to correct population-trend estimates derived from opportunistic observations. Conserv. Biol. J.Soc. Conserv. Biol. 24, 1388–1397. doi: 10.1111/j.1523-1739.2010.01479.x
Kolowski, J. M., and Forrester, T. D. (2017). Camera trap placement and the potential for bias due to trails and other features. PLoS One 12:e0186679. doi: 10.1371/journal.pone.0186679
Kopij, G. (2009). Habitat and drey sites of the red squirrel Sciurus vulgaris Linnaeus 1758 in suburban parks of Wrocław, SW Poland. Acta Zool. Cracoviensia Series A 52, 107–114. doi: 10.3409/azc.52a_1-2.107-114
Kopij, G. (2014). Distribution and abundance of the Red Squirrel Sciurus vulgaris in an urbanised environment. Acta Musei Silesiae. Sci. Naturales 63, 255–262. doi: 10.2478/cszma-2014-0022
Koprowski, J. L. (2005). The response of tree squirrels to fragmentation: a review and synthesis. Anim. Conserv. 8, 369–376. doi: 10.1017/S1367943005002416
Koshkina, V., Wang, Y., Gordon, A., Dorazio, R. M., White, M., and Stone, L. (2017). Integrated species distribution models: combining presence-background data and site-occupancy data with imperfect detection. Methods Ecol. Evol. 8, 420–430. doi: 10.1111/2041-210X.12738
Kostrzewa, A., and Krauze-Gryz, D. (2020). The choice and handling of supplemental food by red squirrels in an urban park. Behav. process. 178:104153. doi: 10.1016/j.beproc.2020.104153
Kramer-Schadt, S., Niedballa, J., Pilgrim, J. D., Schröder, B., Lindenborn, J., Reinfelder, V., et al. (2013). The importance of correcting for sampling bias in MaxEnt species distribution models. Divers. Distrib. 19, 1366–1379. doi: 10.1111/ddi.12096
Krauze-Gryz, D., and Gryz, J. (2015). “A review of the diet of the red squirrel (Sciurus vulgaris) in different types of habitats,” in Red Squirrels: Ecology, Conservation & Management in Europe, eds C. M. Shuttleworth, P. W. W. Lurz, and M. W. Hayward (European Squirrel Initiative), 39–50.
LaPoint, S., Balkenhol, N., Hale, J., Sadler, J., and van der Ree, R. (2015). Ecological connectivity research in urban areas. Funct. Ecol. 29, 868–878. doi: 10.1111/1365-2435.12489
Louvrier, J. L. P., Planillo, A., Stillfried, M., Hagen, R., Börner, K., Kimmig, S., et al. (2021). Spatiotemporal interactions of a novel mesocarnivore community in an urban environment before and during SARS-CoV-2 lockdown. J. Anim. Ecol. 91, 367–380. doi: 10.1111/1365-2656.13635
Lurz, P. W. W., and Bosch, S. (2012). The European Red Squirrel: Sciurus vulgaris, 1., ed. ed. NBB English Edition 183. Hohenwarsleben: Westarp Wissenschaftenverlagsgesellschaft.
Lurz, P. W. W., Gurnell, J., and Louise, M. (2005). Sciurus vulgaris. Mammalian Species 769, 1–10. doi: 10.2307/3504523
Lynch, A. J. (2019). Creating Effective Urban Greenways and Stepping-stones: Four Critical Gaps in Habitat Connectivity Planning Research. J. Planning Lit. 34, 131–155. doi: 10.1177/0885412218798334
MacKenzie, D. I., Bailey, L. L., and Nichols, J. D. (2004). Investigating species co-occurrence patterns when species are detected imperfectly. J. Anim. Ecol. 73, 546–555. doi: 10.1111/j.0021-8790.2004.00828.x
MacKenzie, D. I., Nichols, J. D., Lachman, G. B., Droege, S., Andrew Royle, J., and Langtimm, C. A. (2002). Estimating site occupancy rates when detection probabilities are less than one. Ecology 83, 2248–2255. doi: 10.1890/0012-96582002083
Magris, L., and Gurnell, J. (2002). Population ecology of the red squirrel (Sciurus vulgaris) in a fragmented woodland ecosystem on the Island of Jersey, Channel Islands. J. Zool. 256, 99–112. doi: 10.1017/S0952836902000134
Mazerolle, M. J. (2020). AICcmodavg: Model Selection and Multimodel Inference Based.on (Q)AIC(c). Available online at :https://cran.r-project.org/package=AICcmodavg (accessed 05, June 2022).
McClure, M. L., Hansen, A. J., and Inman, R. M. (2016). Connecting models to movements: testing connectivity model predictions against empirical migration and dispersal data. Landsc. Ecol. 31, 1419–1432. doi: 10.1007/s10980-016-0347-0
McKinley, D. C., Miller-Rushing, A. J., Ballard, H. L., Bonney, R., Brown, H., Cook-Patton, S. C., et al. (2017). Citizen science can improve conservation science, natural resource management, and environmental protection. Biol. Conserv. 208, 15–28. doi: 10.1016/j.biocon.2016.05.015
McRae, B. H., Dickson, B. G., Keitt, T. H., and Shah, V. B. (2008). Using circuit theory to model connectivity in ecology, evolution, and conservation. Ecology 89, 2712–2724. doi: 10.1890/07-1861.1
Meek, P. D., Ballard, G., Claridge, A., Kays, R., Moseby, K., O’Brien, T., et al. (2014). Recommended guiding principles for reporting on camera trapping research. Biodivers. Conserv. 23, 2321–2343. doi: 10.1007/s10531-014-0712-8
National Audubon Society. (2018). Equity, Diversity, and Inclusion: With a Plurality of Voices, we will Inspire more People and Conserve more Habitats.. Available online at: https://www.audubon.org/ (accessed June 05, 2022).
Niesner, C. A., Blakey, R. V., Blumstein, D. T., and Abelson, E. S. (2021). Wildlife Affordances of Urban Infrastructure: A Framework to Understand Human-Wildlife Space Use. Front. Conserv. Sci. 2:774137. doi: 10.3389/fcosc.2021.774137
Paker, Y., Yom-Tov, Y., Alon-Mozes, T., and Barnea, A. (2014). The effect of plant richness and urban garden structure on bird species richness, diversity and community structure. Landsc. Urban Planning 122, 186–195. doi: 10.1016/j.landurbplan.2013.10.005
Phillips, P., Clark, M. M., Baral, S., Koen, E., and Bowman, J. (2021). Comparison of methods for estimating omnidirectional landscape connectivity. Landsc. Ecol. 36, 1647–1661. doi: 10.1007/s10980-021-01254-2
Phillips, S. J. (2008). Transferability, sample selection bias and background data in presence-only modelling: a response to Peterson et al. (2007). Ecography 31, 272–278. doi: 10.1111/j.0906-7590.2008.5378.x
Phillips, S. J., Anderson, R. P., and Schapire, R. E. (2006a). Maximum entropy modeling of species geographic distributions. Ecol. Model. 190, 231–259. doi: 10.1016/j.ecolmodel.2005.03.026
Phillips, S. J., Dudík, M., and Schapire, R. E. (2006b). Maxent Software for Modeling Species Niches and Distributions (Version 3.4.1).
Planillo, A., Fiechter, L., Sturm, U., Voigt-Heucke, S., and Kramer-Schadt, S. (2021). Citizen science data for urban planning: comparing different sampling schemes for modelling urban bird distribution. Landsc. Urban Planning 211:104098. doi: 10.1016/j.landurbplan.2021.104098
Planillo, A., Kramer-Schadt, S., Buchholz, S., Gras, P., von der Lippe, M., and Radchuk, V. (2020). Arthropod abundance modulates bird community responses to urbanization. Divers. Distrib. 27, 34–49. doi: 10.1111/ddi.13169
Poor, E. E., Loucks, C., Jakes, A., and Urban, D. L. (2012). Comparing Habitat Suitability and Connectivity Modeling Methods for Conserving Pronghorn Migrations. PLoS One 7:e49390. doi: 10.1371/journal.pone.0049390
R Core Team. (2020). R: A Language and Environment for Statistical. R Foundation for Statistical Computing. Vienna, Aust. Available online at: https://www.r-project.org/ (accessed April 02, 2021).
Reher, S., Dausmann, K. H., Warnecke, L., and Turner, J. M. (2016). Food availability affects habitat use of Eurasian red squirrels (Sciurus vulgaris) in a semi-urban environment. JMAMMAL 97, 1543–1554. doi: 10.1093/jmammal/gyw105
Renner, I. W., Elith, J., Baddeley, A., Fithian, W., Hastie, T., Phillips, S. J., et al. (2015). Point process models for presence-only analysis. Methods Ecol. Evol. 6, 366–379. doi: 10.1111/2041-210X.12352
Renner, I. W., Louvrier, J., and Gimenez, O. (2019). Combining multiple data sources in species distribution models while accounting for spatial dependence and overfitting with combined penalized likelihood maximization. Methods Ecol. Evol. 10, 2118–2128. doi: 10.1111/2041-210X.13297
Rézouki, C., Dozières, A., Le Cśur, C., Thibault, S., Pisanu, B., Chapuis, J.-L., et al. (2014). A viable population of the European red squirrel in an urban park. PloS One 9:e105111. doi: 10.1371/journal.pone.0105111
Ripley, B. D. (1977). Modelling Spatial Patterns. J.R. Statist. Soc. 39, 172–192. doi: 10.1111/j.2517-6161.1977.tb01615.x
Rotman, D., Preece, J., Hammock, J., Procita, K., Hansen, D., Parr, C., et al. (2012). “Dynamic changes in motivation in collaborative citizen-science projects,” in Proceedings of the ACM 2012 Conference on Computer Supported Cooperative Work - CSCW ‘12, eds S. Poltrock, C. Simone, J. Grudin, G. Mark, and J. Riedl (New York, NY: ACM Press), 217.
Royle, J. A., Nichols, J. D., Karanth, K. U., and Gopalaswamy, A. M. (2009). A hierarchical model for estimating density in camera-trap studies. J. Appl. Ecol. 46, 118–127. doi: 10.1111/j.1365-2664.2008.01578.x
Saura, S., Bodin, Ö, and Fortin, M.-J. (2014). Stepping stones are crucial for species’ long-distance dispersal and range expansion through habitat networks. J. Appl. Ecol. 51, 171–182. doi: 10.1111/1365-2664.12179
Scharf, A. K., Belant, J. L., Beyer, D. E., Wikelski, M., and Safi, K. (2018). Habitat suitability does not capture the essence of animal-defined corridors. Mov. Ecol. 6:18. doi: 10.1186/s40462-018-0136-2
Schwarz, G. (1978). Estimating the Dimension of a Model. Ann. Statist. 6, 461–464. doi: 10.1214/aos/1176344136
Selonen, V., Fey, K., and Hämäläinen, S. (2018). Increased differentiation between individuals, but no genetic isolation from adjacent rural individuals in an urban red squirrel population. Urban Ecosyst. 21, 1067–1074. doi: 10.1007/s11252-018-0794-2
Senate Department for the Environment. (2019). Transport and Climate Protection, 2019. Proportion of Public Green Areas in Berlin. Available online at: https://www.berlin.de/senuvk/umwelt/stadtgruen/gruenanlagen/de/daten_fakten/downloads/ausw_5.pdf. (accessed April 02, 2021).
Senate Department for Urban Development und Housing. (2018). Berlin Environmental Atlas. Available online at: https://www.stadtentwicklung.berlin.de/umwelt/umweltatlas/ (accessed April 02, 2021).
Shuttleworth, C. M., Lurz, P. W. W., Hayward, M. W., and Bertolino, S. (2015). “Developing integrated red squirrel conservation in Europe,” in Red Squirrels - Ecology Conservation & Management in Europe, eds C. M. P. Shuttleworth and >Lurz, W. W. M. W. Hayward (Suffolk: European Squirrel Initiative), 319–330.
Simpkins, C. E., Dennis, T. E., Etherington, T. R., and Perry, G. L. (2018). Assessing the performance of common landscape connectivity metrics using a virtual ecologist approach. Ecol. Model. 367, 13–23. doi: 10.1016/j.ecolmodel.2017.11.001
Starkey, E., Parkin, G., Birkinshaw, S., Large, A., Quinn, P., and Gibson, C. (2017). Demonstrating the value of community-based (‘citizen science’) observations for catchment modelling and characterisation. J. Hydrol. 548, 801–817. doi: 10.1016/j.jhydrol.2017.03.019
Statistical Office for Berlin-Brandenburg. (2021). Einwohnerinnen und Einwohner im Land Berlin am 31. Dezember 2020. Potsdam: Grunddaten.
Steen, V. A., Elphick, C. S., and Tingley, M. W. (2019). An evaluation of stringent filtering to improve species distribution models from citizen science data. Divers. Distrib. 25, 1857–1869. doi: 10.1111/ddi.12985
Stevenson-Holt, C. D., Watts, K., Bellamy, C. C., Nevin, O. T., and Ramsey, A. D. (2014). Defining Landscape Resistance Values in Least-Cost Connectivity Models for the Invasive Grey Squirrel: A Comparison of Approaches Using Expert-Opinion and Habitat Suitability Modelling. PLoS One 9:e112119. doi: 10.1371/journal.pone.0112119
Stuart, C., Wedding, L., Pittman, S., and Green, S. (2021). Habitat Suitability Modeling to Inform Seascape Connectivity Conservation and Management. Diversity 13:465. doi: 10.3390/d13100465
Sullivan, B. L., Aycrigg, J. L., Barry, J. H., Bonney, R. E., Bruns, N., Cooper, C. B., et al. (2014). The eBird enterprise: An integrated approach to development and application of citizen science. Biol. Conserv. 169, 31–40. doi: 10.1016/j.biocon.2013.11.003
Sullivan, B. L., Wood, C. L., Iliff, M. J., Bonney, R. E., Fink, D., and Kelling, S. (2009). eBird: a citizen-based bird observation network in the biological sciences. Biol. Conserv. 142, 2282–2292. doi: 10.1016/j.biocon.2009.05.006
Thomas, L. S., Teich, E., Dausmann, K., Reher, S., and Turner, J. M. (2018). Degree of urbanisation affects Eurasian red squirrel activity patterns. Hystrix Italian J. Mammal. 29, 175–180. doi: 10.4404/hystrix-00065-2018
Thorington, R. W., Koprowski, J. L., Steele, M. A., and Whatton, J. F. (2012). Squirrels of the World. Baltimore: The Johns Hopkins University Press.
Tiago, P., Pereira, H. M., and Capinha, C. (2017). Using citizen science data to estimate climatic niches and species distributions. Basic Appl. Ecol. 20, 75–85. doi: 10.1016/j.baae.2017.04.001
Turkia, T., Selonen, V., Danilov, P., Kurhinen, J., Ovaskainen, O., Rintala, J., et al. (2018). Red squirrels decline in abundance in the boreal forests of Finland and NW Russia. Ecography 41, 1370–1379. doi: 10.1111/ecog.03093
Uchida, K., Suzuki, K., Shimamoto, T., Yanagawa, H., and Koizumi, I. (2016). Seasonal variation of flight initiation distance in Eurasian red squirrels in urban versus rural habitat. J. Zool. 298, 225–231. doi: 10.1111/jzo.12306
Uchida, K., Suzuki, K. K., Shimamoto, T., Yanagawa, H., and Koizumi, I. (2019). Decreased vigilance or habituation to humans? Mechanisms on increased boldness in urban animals. Behav. Ecol. 30, 1583–1590. doi: 10.1093/beheco/arz117
van Strien, A. J., van Swaay, C. A., and Termaat, T. (2013). Opportunistic citizen science data of animal species produce reliable estimates of distribution trends if analysed with occupancy models. J. Appl. Ecol. 50, 1450–1458. doi: 10.1111/1365-2664.12158
Verbeylen, G., Bruyn, L., De Adriaensen, F., and Matthysen, E. (2003). Does matrix resistance influence Red squirrel (Sciurus vulgaris L. 1758) distribution in an urban landscape? Landsc. Ecol. 18, 791–805. doi: 10.1023/B:LAND.0000014492.50765.05
Walker, J., and Taylor, P. D. (2017). Using eBird data to model population change of migratory bird species. Avian Conserv. Ecol. 12:4. doi: 10.5751/ACE-00960-120104
Warton, D. I., Blanchet, F. G., O’Hara, R. B., Ovaskainen, O., Taskinen, S., Walker, S. C., et al. (2015). So Many Variables: Joint Modeling in Community Ecology. Trends Ecol. Evol. 30, 766–779. doi: 10.1016/j.tree.2015.09.007
Young, B. E., Dodge, N., Hunt, P. D., Ormes, M., Schlesinger, M. D., and Shaw, H. Y. (2019). Using citizen science data to support conservation in environmental regulatory contexts. Biol. Conserv. 237, 57–62. doi: 10.1016/j.biocon.2019.06.016
Keywords: species distribution models, data-integration models, occupancy models, point process models, urban ecology, circuitscape analysis, MaxEnt models, connectivity
Citation: Grabow M, Louvrier JLP, Planillo A, Kiefer S, Drenske S, Börner K, Stillfried M, Hagen R, Kimmig S, Straka TM and Kramer-Schadt S (2022) Data-integration of opportunistic species observations into hierarchical modeling frameworks improves spatial predictions for urban red squirrels. Front. Ecol. Evol. 10:881247. doi: 10.3389/fevo.2022.881247
Received: 22 February 2022; Accepted: 11 July 2022;
Published: 03 August 2022.
Edited by:
Luciano Bosso, Università degli Studi di Napoli Federico II, ItalyReviewed by:
Margaret Gold, Leiden University, NetherlandsAlireza Mohammadi, University of Jiroft, Iran
Danial Nayeri, Cal Poly Humboldt Arcata, United States, in collaboration with reviewer AM
Copyright © 2022 Grabow, Louvrier, Planillo, Kiefer, Drenske, Börner, Stillfried, Hagen, Kimmig, Straka and Kramer-Schadt. This is an open-access article distributed under the terms of the Creative Commons Attribution License (CC BY). The use, distribution or reproduction in other forums is permitted, provided the original author(s) and the copyright owner(s) are credited and that the original publication in this journal is cited, in accordance with accepted academic practice. No use, distribution or reproduction is permitted which does not comply with these terms.
*Correspondence: Marius Grabow, grabow@izw-berlin.de
†Present address: Sarah Kiefer and Sophia Kimmig, Leibniz-Institute of Freshwater Ecology and Inland Fisheries, Berlin, Germany