- 1Department of Wildlife, Fish, and Conservation Biology, University of California, Davis, Davis, CA, United States
- 2Department of Fish, Wildlife, and Conservation Biology, Colorado State University, Fort Collins, CO, United States
- 3Road Ecology Center, Institute of Transportation Studies, University of California, Davis, Davis, CA, United States
- 4School of Veterinary Medicine, One Health Institute, University of California, Davis, Davis, CA, United States
Anthropogenic noise is pervasive across the landscape and can be present at two temporal scales: acute (occurring sporadically and stochastically over the shortest time scales, e.g., milliseconds), and chronic (more persistent than instantaneous and occurring over longer timescales, e.g., minutes, days). Acute and chronic anthropogenic noise may induce a behavioral fear-mediated response in wildlife that is analogous to a prey response to predators. Understanding wildlife responses to anthropogenic noise is especially important in the case of wildlife crossing structures that provide wildlife with access to resources across busy roadways. Focusing on two species common at wildlife crossing structures, mule deer (Odocoileus hemionus) and coyotes (Canis latrans), we addressed the hypotheses that (1) acute traffic noise causes flight behavior; and (2) chronic traffic noise causes changes in a range of behaviors associated with the vigilance–foraging trade-off (vigilance, running, and foraging). We placed camera traps at entrances to ten crossing structures for a period of ∼ 2 months each throughout California, USA. Mule deer and coyotes demonstrated a flight response to acute traffic noise at entrances to crossing structures. Both species demonstrated shifts in behavioral response to chronic traffic noise within and among structures. Coyote behavior was indicative of fear, demonstrating increased vigilance at louder times within crossing structures, and switching from vigilance to running activity at louder crossings. Mule deer responded positively, increasing foraging at both spatial scales, and demonstrating decreased vigilance at louder structures, potentially using crossing structures as a Human Shield. Our results are the first to demonstrate that anthropogenic noise at crossing structures could alter wildlife passage, and that variations in fear response to anthropogenic noise exist across temporal, spatial, and amplitude scales. This dynamic response could alter natural predator-prey interactions and scale up to ecosystem-level consequences such as trophic cascades in areas with roads.
Introduction
Humans have modified the majority of the Earth’s ecosystems, and occupy approximately 50% of the global surface (Strano et al., 2020). Within these human-dominated ecosystems, human activity can elicit fear responses from wildlife species by altering stress physiology, spatiotemporal habitat use, and behavior (Støen et al., 2015; Hammond et al., 2020). Fear is defined in the present study as a reactive, anti-predator behavioral response to an environmental stimulus (Palmer and Packer, 2021) and fear responses to human disturbance are presumed to be analogous to prey responses to predators (Lima and Bednekoff, 1999; Frid and Dill, 2002). These fear responses may result in modification to predator-prey interactions, and lead to trophic cascading effects, ultimately altering ecosystem structure and function (Kuijper et al., 2016; Schmitz et al., 2018; Zanette and Clinchy, 2019).
Anthropogenic disturbances that trigger behavioral fear responses exist across multiple scales. As such, the spatiotemporal scale that an anthropogenic disturbance occupies may determine the scales of fear responses (Dröge et al., 2017; Moll et al., 2017). For example, heterogenous space use by humans can lead to alterations in the movement decisions and distribution of predators and prey at a fine-scale and across a landscape (Suraci et al., 2019, 2020). Moll et al. (2017) and Prugh et al. (2019) recently noted the urgent need for multi-scale frameworks to disentangle the behavioral impacts of fear across scales, demonstrating that prior work examining fear responses has largely focused at a single scale, and inconsistent use of metrics precludes cross-study comparisons.
One anthropogenic disturbance that varies across spatiotemporal scales is noise pollution. Anthropogenic noise pollution is pervasive across urban and wildland areas, and has been linked to behavioral shifts in migration, communication, prey detection, and predator avoidance among wildlife species (Francis et al., 2012; Buxton et al., 2017; Dominoni et al., 2020). Fear responses from noise pollution are commonly reported, though some species demonstrate habituation over time from repeated exposure (Nedelec et al., 2016; Neo et al., 2018; Walthers and Barber, 2020), and some ungulate and small mammal species use anthropogenic noise as a “Human Shield” to seek shelter from predators (Berger, 2007; Shannon et al., 2014b; Suraci et al., 2019). Anthropogenic noise is measured with a number of different weighting scales, each of which vary the importance of amplitude at different sound frequencies. As species differ in their physiological ability to detect sound at certain frequencies, weighting scales can accommodate these differences. For example, A-weighting (dBA) is broadly used for impacts on humans (McKenna et al., 2016) and wildlife (Shannon et al., 2016b), and C-weighting is often used for humans, birds and amphibian effects (Francis et al., 2009; Broner, 2010; Alloush et al., 2011). Low- and higher-frequency sounds are sometimes used in isolation for certain impacts on vertebrates (Qin et al., 2014; Shannon et al., 2016b).
To examine whether a fear response occurs when animals are exposed to various spatial and temporal scales of anthropogenic noise pollution, we need to consider the multi-scale characteristics of the noise pollution and the behavioral response. Acute, infrequent noise (milliseconds to seconds) could startle the animal (acoustic startle response: Koch and Schnitzler, 1997; Francis and Barber, 2013) and induce an escape response (e.g., fleeing; Guiden et al., 2019). Chronic, continuous noise, over longer time periods (minutes, months, and years) could alter anti-predator behavior such as vigilance (predator surveillance: Bednekoff and Lima, 1998; Creel and Winnie, 2005; Francis and Barber, 2013). A behavioral alteration from chronic noise could be due to wildlife perceiving the disturbance as a threat (risk disturbance hypothesis: Frid and Dill, 2002), the disturbance reducing the capability of auditory surveillance, creating the need to increase visual awareness of predators, or the disturbance differentially inhibiting predators more than prey, resulting in lesser need for vigilance on the part of prey (human shield hypothesis: Berger, 2007).
Understanding fear responses can have beneficial implications for the management of applied conservation projects (Prugh et al., 2019; Gaynor et al., 2020). Crossing structures built under or over highways with the aim to mitigate habitat fragmentation, or that were built for another reason (e.g., accommodate water flow), and that wildlife use for passage, may reduce the barrier effects of roads and road-related mortality (Clevenger and Waltho, 2005; Rytwinski et al., 2016). However, the success of crossing structures in facilitating animal passage across roadways depends on specific aspects of animal behavior in response to roads and the structure, especially the likelihood of approaching and entering the structure. Traffic-induced noise pollution that is acute could trigger an escape response and an aversion to enter into the crossing structure (Quinn et al., 2006; Shannon et al., 2014a; Owen et al., 2017). Chronic noise at a crossing structure could increase an animal’s perception of risk, or mask auditory predator cues, thereby increase visual vigilance and lower time spent feeding or moving (Francis and Barber, 2013). Greater levels of chronic noise at crossings could also induce a shift in movement from walking to running, a strategy wildlife may employ to move through riskier situations more quickly (Ydenberg and Dill, 1986; Fryxell et al., 2014). Understanding fear-based avoidance responses at crossing structures is particularly critical if there are no alternative routes available to connect habitats bisected by roads (Gill et al., 2001).
Many studies have demonstrated the acoustic impacts of road noise on behavioral responses in birds, typically under lab or controlled-field settings (McClure et al., 2013; Meillère et al., 2015; Ware et al., 2015), but less is known regarding fear responses in mammals. The few studies that have been conducted on mammals primarily suggest a fear response; vigilance in prairie dogs increases during exposure to simulated vehicular noise (Shannon et al., 2014a) and avoidance activity of white-tailed deer (Odocoileus virginianus) and prairie dogs increases during motorcycle rallies (Buxton et al., 2020). Alongside roads, vigilance in impalas (Aepyceros melampus) increases, while predation efficiency in the greater mouse-eared bat (Myotis myotis) decreases (Siemers and Schaub, 2011; Mtui, 2014). Given the vulnerability of large, mobile mammals to road mortality and habitat fragmentation (Rytwinski and Fahrig, 2015), mammals are often target species for crossing structures. Understanding the impacts of traffic-related noise pollution on behavioral responses in mammals is of particular importance in designing effective crossings.
Using a multi-scale approach of acute (1 s) to chronic (1-week) noise at the site (crossing) and region (California) levels, we tested whether behavioral responses associated with fear exist for two species, coyotes (Canis latrans) and mule deer (Odocoileus hemionus), at crossing structures exposed to traffic noise. We first tested the hypothesis that acute noise levels affect the utilization of crossing structures, predicting that increased noise will be associated with reduced use of the crossing structure. Secondly, we examined whether noise levels alter anti-predator behavior, predicting that noisier traffic conditions would cause an increase in levels of vigilance and/or running, and a decrease in foraging at two spatial scales: within and among crossing structures.
Materials and methods
Study area
Our study was conducted from November 2018 to November 2019 at 10 crossing structures in California, USA: eight culverts and two bridges under state highways (Figure 1). Three of these structures were purpose-built for wildlife crossing, and seven were built for water movement, human access and were opportunistically used by wildlife. Parameters for structural attributes and local conditions would be expected to control for these different uses. Crossing structures were located across Interstate 5 and U.S. Route 97 in northern California; Interstate 80, Interstate 680, and U.S. Route 50 in central California; and State Route 74 in Southern California. Crossing structures were located under highways consisting of one to three lanes of traffic in each direction, in areas classed as low- or medium-intensity development, evergreen forest, or shrub/scrub (Dewitz, 2019).
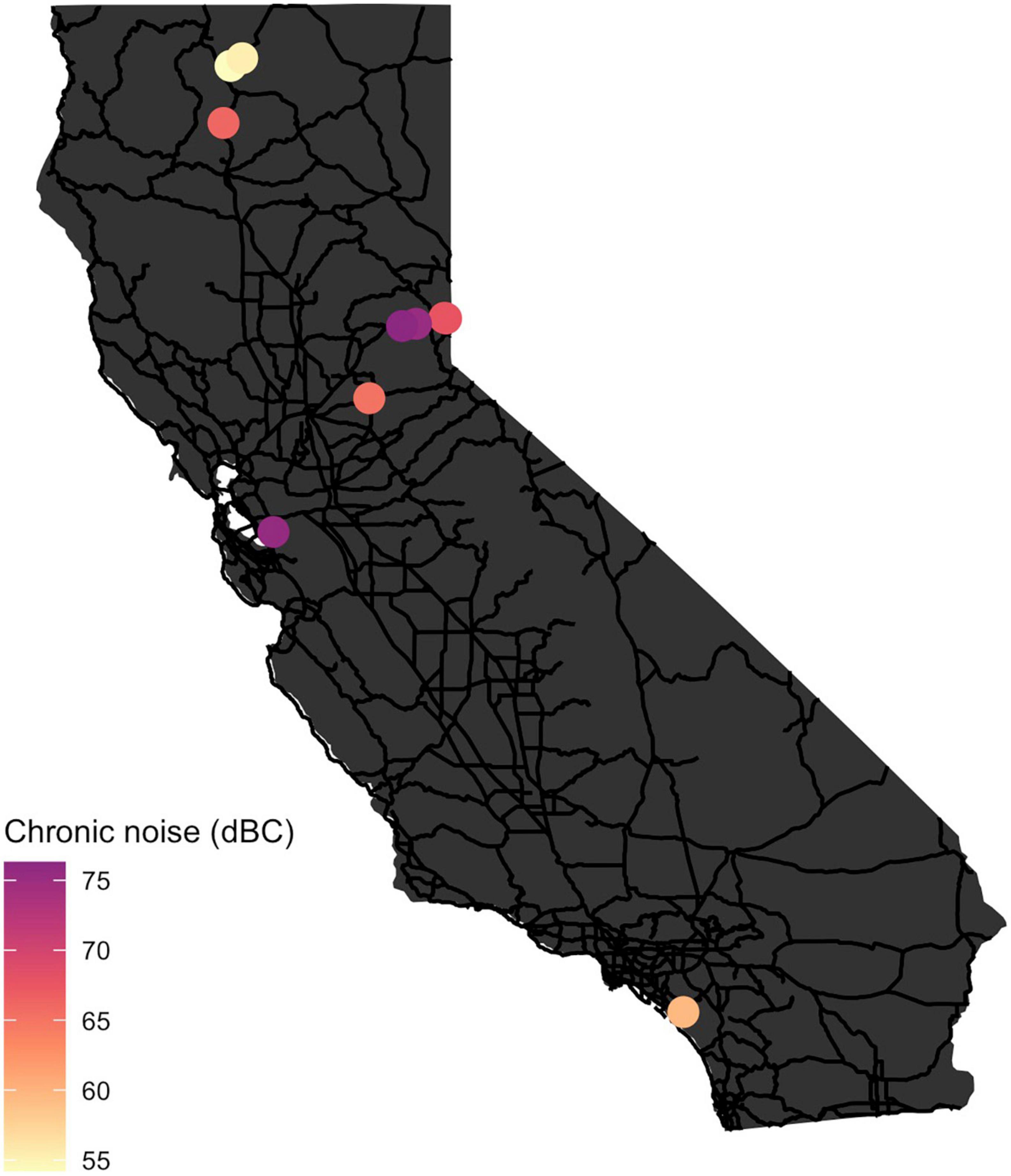
Figure 1. Map of California State Highways (black lines) and the location of each crossing structure sampled in this study (circles). Color of each crossing structure represents the level of chronic noise recorded (1-week period).
Camera trapping
Behavior of coyotes and mule deer was assessed from videos obtained using camera traps. We placed six camera traps (Browning Dark Ops Pro) at each crossing structure and set cameras to record high-quality video and audio, triggered by motion and infrared (no-glow). Once triggered, cameras recorded videos for 20 s, with a 5-s delay between triggers. Cameras were equipped with the “smart IR setting,” i.e., videos during the daytime were recorded beyond 20 s if the camera continued to detect wildlife movement. We placed one camera at each of the two entrances of each crossing structure, ca. 5 m (range: 0–10 m) from the entrance, facing inward. We also placed two cameras at each of the two “approach zones” perpendicular to the crossing, the area ca. 50 m from each crossing structure entry from which wildlife approach the crossing (range: 11–84 m). Cameras remained operational at each crossing structure for an average of 45 days (range: 1–106 days). We captured the location of each camera station and the crossing structure entrance via a handheld GPS device (Garmin eTrex 20×) and estimated the Euclidean distance of each camera station to the entrance of the crossing structure via Google Earth Pro (V7.3.3.7786).
Acute and chronic behavioral responses
We extracted activity from each camera trap video that included mule deer and coyotes using the Behavioral Observation Research Interactive Software (BORIS; Friard and Gamba, 2016). To analyze flight and entry responses associated with acute noise, videos from cameras positioned at the crossing structure entrance (ca. 5 m from entrance) were checked for observations in which animals were facing the direction of the crossing structure entryway or walking toward the crossing structure entryway. Within these observations, we scored each time a movement-related decision-making event occurred: (1) Entry, defined as stepping across the concrete edge of the crossing structure entrance; and (2) Flight, defined as an alteration in body direction and movement away from the crossing structure (Figure 2).
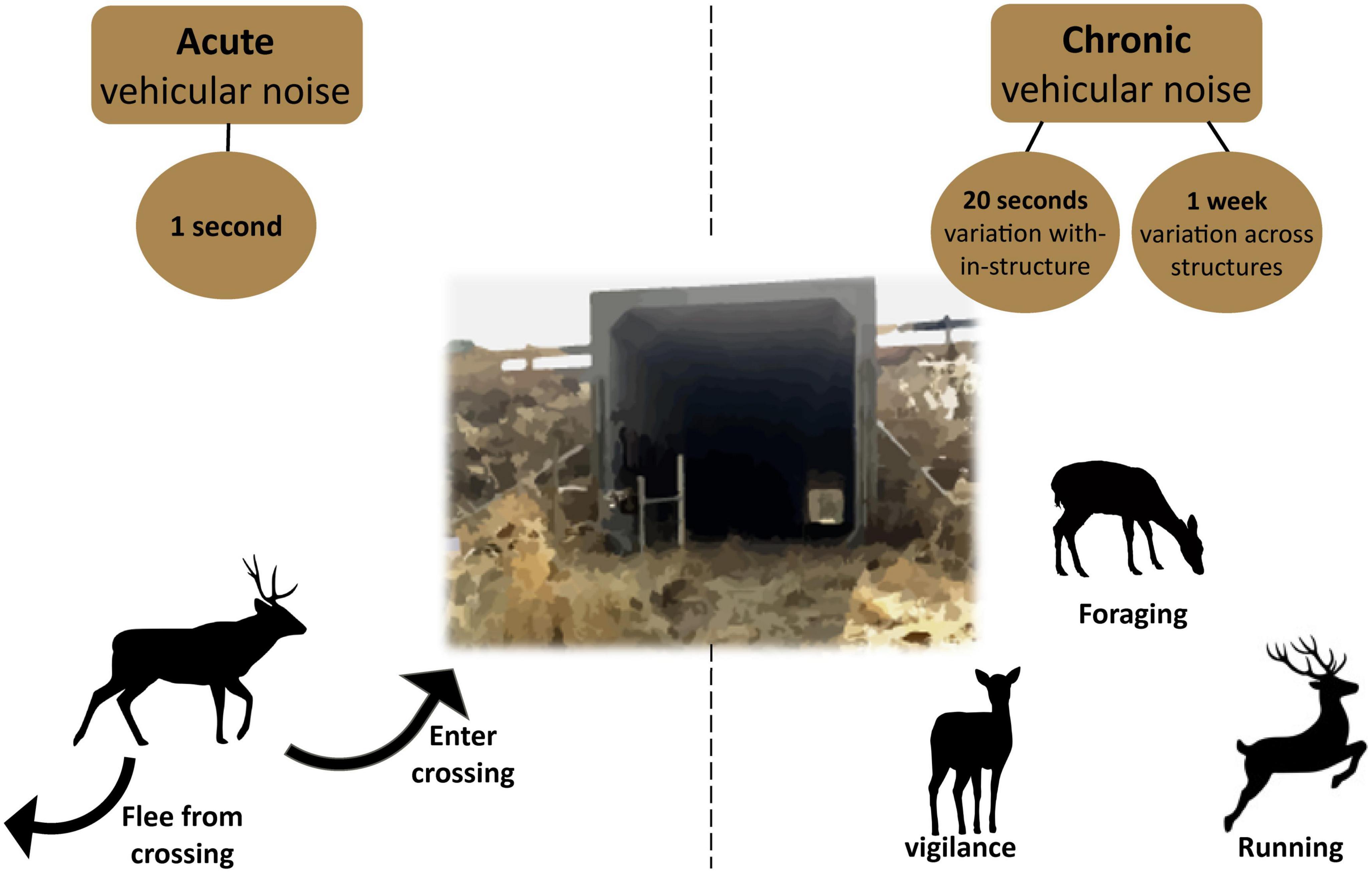
Figure 2. Schematic representing the range of spatial and temporal scales of noise sampled at crossing structures: acute noise (1 s before and after the event), chronic noise across observations within each crossing structure (–20 s) and chronic noise across each structure (1 week). For acute and chronic noise, we illustrate the behavioral responses of mule deer and coyote that were observed.
To examine behaviors associated with chronic noise, we also scored activity of individual mule deer and coyotes for all videos from all cameras, including the videos analyzed for fight or entry responses. We focused on three categories of behavior associated with fear: (1) vigilance, defined as an individual standing or sitting upright, (2) running, defined as any movement faster than a walk, and (3) foraging, defined as feeding or drinking (Figure 2 and Supplementary Figure 1). For each of these behaviors, we assigned a start and stop time within each video and calculated time as a proportion of total time the individual was present in the video. The BORIS enabled classification of multiple behaviors recorded simultaneously. To distinguish between individuals within one video, we assigned each conspecific a unique identification based on order of appearance or distance from the camera. To avoid differential periods of exposure to a camera and to ensure observations were independent, video clips longer than 20 s were trimmed, and observations were filtered to include a minimum time interval of 5 min between observations. For each video, we documented whether the video was taken in day (color) or night (black and white) mode, the temperature listed for each video collected by the camera’s internal thermometer, and the maximum group size observed. We recorded the number of humans that passed through every video and quantified the frequency of daily visits by humans for each camera station (0–12/day). Research technicians who classified observations initially received extensive training in the recognition of behaviors across species and data capture using BORIS software.
Noise indices
We considered anthropogenic noise at multiple temporal and spatial scales. We quantified acute traffic-related noise (1 s) during each decision-making event occurring at the entrance of a crossing structure. We quantified chronic traffic-related noise for the duration of wildlife presence at a crossing structure across two spatial scales: (1) in each video (−20 s), representing variation within a crossing structure and (2) at each crossing structure (1 week), representing variation among crossing structures.
Acute noise
Audio waveform data recorded by the camera trap during each video were exported using BORIS software. Using the R function “cut_sels” (warbleR; Araya-Salas and Smith-Vidaurre, 2017) we cut selections of each audio file for a 1-s period before and after each decision-making event (flight or entry). For each cut audio file, we then produced an amplitude envelope of the waveform signal derived from the Hilbert transform using the “env” function (seewave; Sueur et al., 2020). Using this envelope, we then extracted the relative maximum amplitude for each 2-s audio file, representing acute noise.
Chronic noise
We quantified the chronic traffic-induced noise levels two ways. First, we extracted the relative median amplitude for each −20-s audio file that pertained to the start and stop time that we observed an individual animal in each video, using the same method as above. Second, we sampled sound pressure levels at each crossing structure, during July–November 2019 for a 7-day period after removal of camera traps (Figure 2). We used TENMA 72-947 and PCE-322 sound pressure meters, which detect in the 30–130 dB range and were set at the slow setting. We placed two sound meters at the entrance of one side of the crossing structure facing inward on a tripod 0.5 m above the ground. Sound pressure levels were measured in 59-s increments in decibels, with one meter set to a C-weighted filter (dBC), and the other set to an A-weighted filter (dBA). For each crossing structure, we then extracted L50 (median noise) measures. As a C-weighted filter more accurately depicts low-frequency noise commonly associated with traffic noise and owing to an equipment failure of the A-weighted sound meter at one crossing structure, hereafter we report findings for dBC, and provide comparative L50 dBA measure in the (Supplementary Tables 1, 3).
Statistical analysis
We analyzed the effects of acute noise on decision-making at the entrance of the crossing structure (entry or flight response), and chronic noise on behavior (proportion of time being vigilant, running, or foraging).
Acute behavioral response: Flight and entry
To examine the impact of acute noise on whether coyote and mule deer entered or fled from the crossing structure entrance, we employed a hierarchical generalized linear (glm) model using the “glm” function in R. Crossing structure and camera unit were specified as random effects, and a binomial distribution was selected based on the binary structure of the response variable (enter or flee). We included additional environmental and structural predictors for each observation that may also affect behavior: group size, time of day (binary; day/night), temperature recorded on each video for each camera, rate of human daily visitations, distance to crossing structure, and openness ratio of each crossing structure [(Crossing height × Crossing width)/Crossing length]. We performed step-wise removal of non-significant variables, and selected the best fit model based on the lowest AIC score.
Chronic behavioral response: Activity budgets
To test for the behavioral impacts of chronic noise within- and across crossing structures, we again employed hierarchical glm analyses for proportion of time: (1) coyotes spent being vigilant and running and (2) mule deer spent being vigilant, running and foraging, using crossing structure and camera as random effects. As we recorded no foraging by coyotes, a model for coyote foraging was not employed. Due to the data type of the behavioral categories being proportional and to account for overdispersion in the data, we used a quasibinomial distribution. We examined the effect of the same predictors as the acute model above, as well as season and dominant land cover category within a 100 m radius of the crossing structure opening to reflect the broader spatiotemporal structure of the data (Dewitz, 2019). To parse out the within-structure and between-structure effects of chronic noise, we used within-group centering (van de Pol and Wright, 2009). This statistical technique accounts for the interdependency between the two levels of spatially aggregated data by centering (subtracting the mean of among-structure noise) and scaling (dividing centered numbers by standard deviation of among-structure noise) each camera-level measurement of noise (“scale” function).
We checked for model robustness by comparing all models to the respective base model using an ANOVA and plotting the Pearson’s residuals. All analyses were carried out in RStudio (R v. 3.6.1; RStudio Team, 2015).
Results
Acute behavioral response: Flight and entry
For mule deer, we observed 192 decision-making events at 16 cameras and 8 structures. A total of 35 (18%) of the events resulted in a flight response and 157 (82%) resulted in an entry (Supplementary Table 2). For coyotes, we observed 50 decision-making events at 11 cameras and 4 structures, nine (18%) of which resulted in a flight response and 41 (82%) resulted in an entry. For both mule deer and coyote, flight responses were more likely to occur as levels of acute noise increased (deer p ≤ 0.001, SE = 0.23; coyote p = 0.018, SE = 0.52; Table 1 and Figure 3). The best fit model also demonstrated that mule deer were more likely to enter underpasses with a smaller openness ratio (p = 0.001, SE = 0.58; range = 0.2–40.9), and coyotes were more likely to enter underpasses during warmer temperatures (p = 0.016, SE = 0.05). For model AIC and coefficient estimates, see Supplementary Table 3.
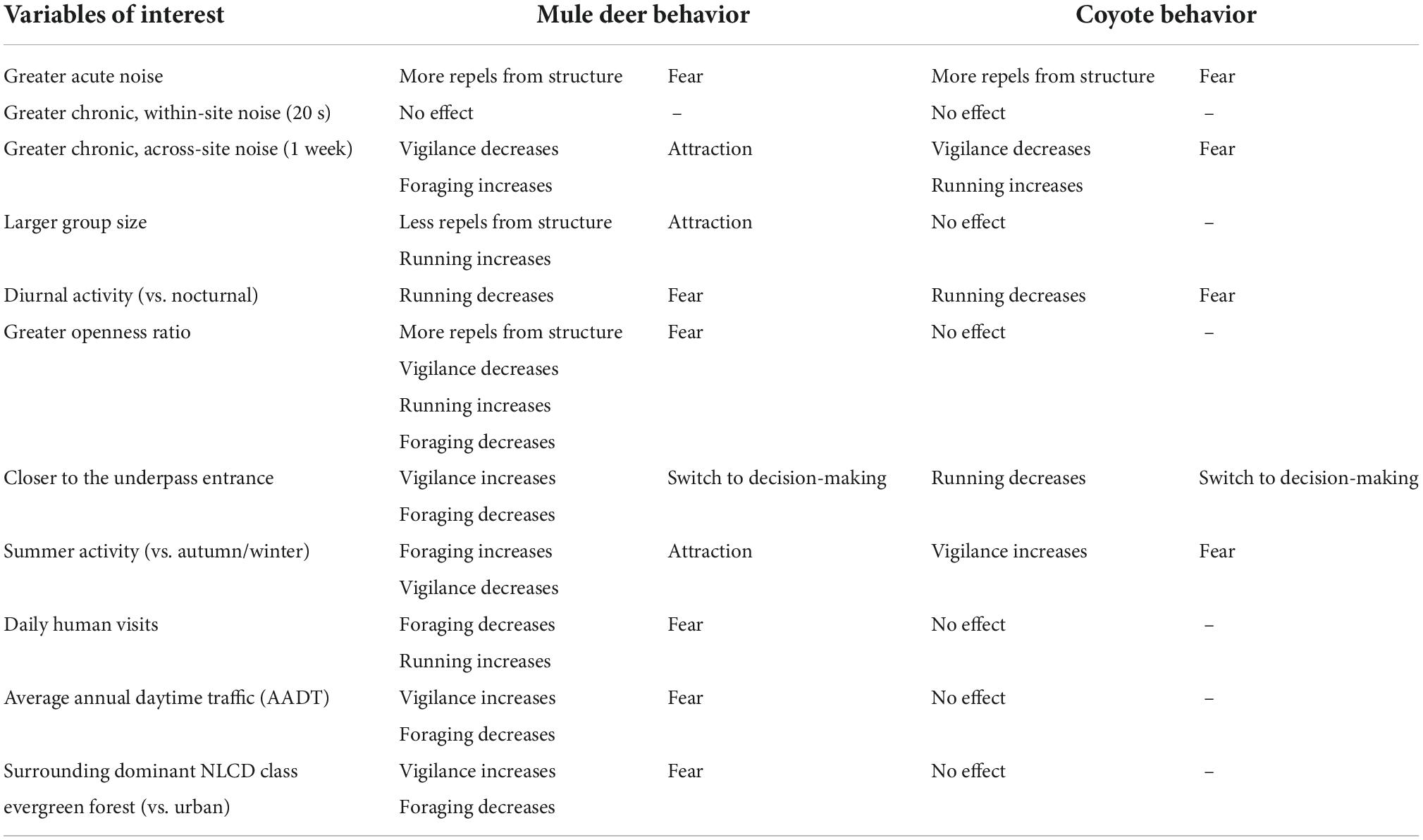
Table 1. A summary of the continuous response variables examined and their effects on coyote and mule deer flight response, vigilance, foraging, and running (mule deer only) behavior.
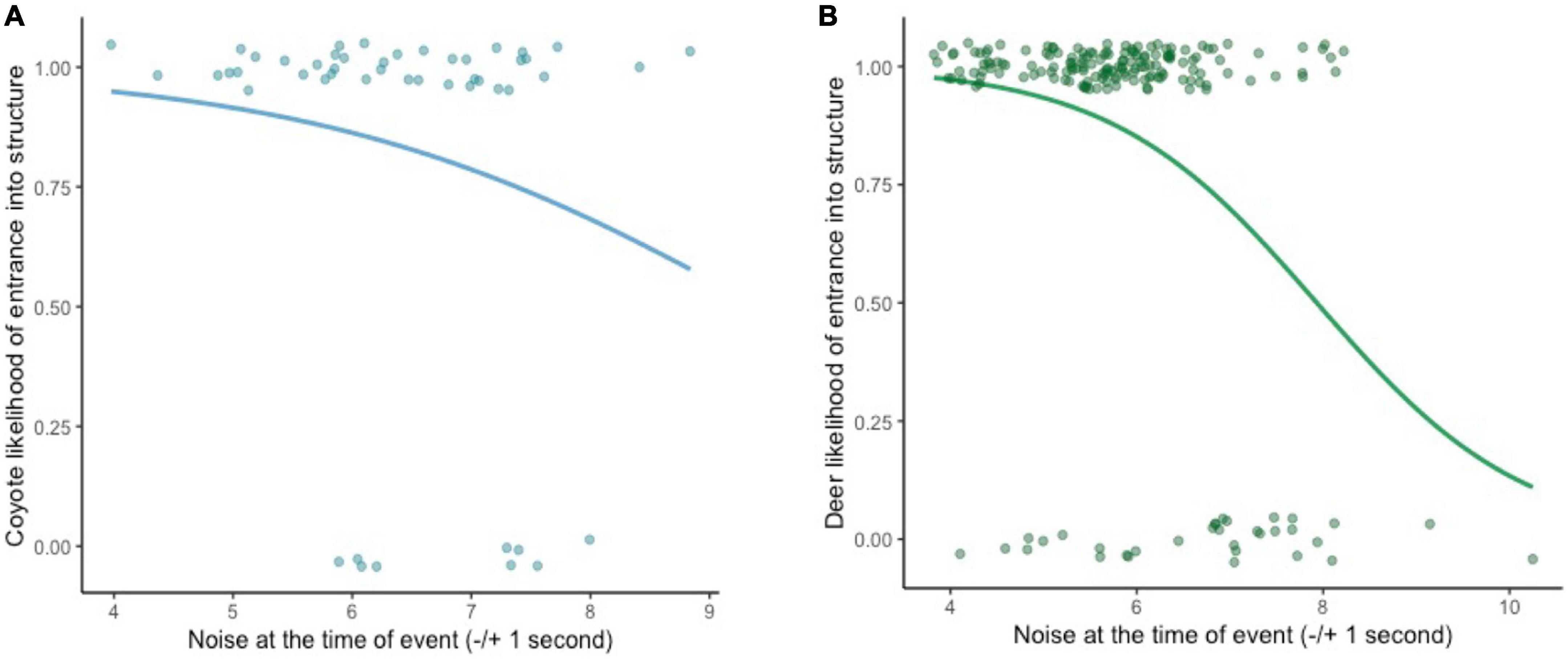
Figure 3. Landscape of fear response: Likelihood of (A) coyotes and (B) deer entering the crossing structure (1 = enter, 0 = repel) in response to acute noise (±1 s) at time of decision-making event.
Chronic behavioral response: Activity budgets
We collected a total of 3 h and 44 min of mule deer observations (n = 1,346) from 28 cameras at 10 structures. In the absence of a minimum 5-min time gap between observations, mule deer foraging increased during greater levels of within-structure noise. However, with a time gap (5 min) to increase independence of observations, we found that vigilance, foraging, and running behavior were not altered during greater levels of within-structure noise. For both sets of analyses, greater levels of among-structure noise demonstrated an increase in mule deer foraging (p < 0.001, SE = 0.02; Figure 4), coupled with a decrease in vigilance (p < 0.001, SE = 0.04; Figure 4).
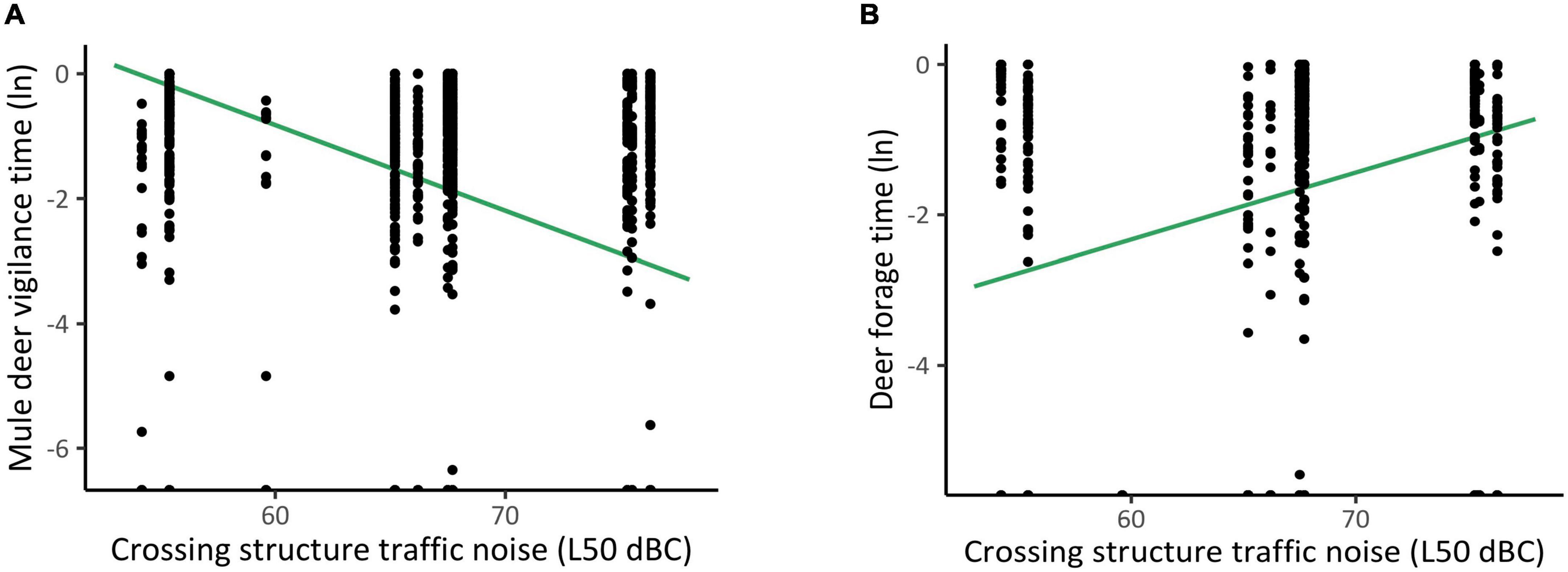
Figure 4. Proportion of time mule deer spent being (A) vigilant and (B) foraging at crossing structures with differing chronic (1 week) noise levels (black dotes). To capture the estimated effect of chronic noise independent of other covariates, the line of best fit was generated using a predictive model.
We collected 20 min of coyote observations (n = 243) from 21 cameras at 8 structures. In the absence of a 5-min time gap between observations, vigilance increased at louder levels of within-structure noise, however, this relationship became non-significant with the addition of a 5-min gap between observations. In response to greater levels of among-structure noise, coyote demonstrated a different response to mule deer; vigilance decreased (p = 0.005, SE = 0.04; Figure 5), and running increased (p < 0.001, SE = 0.14; Figure 5). Other covariates and their associated effect on vigilance, running, and foraging are summarized in Table 1 and Supplementary Table 4.
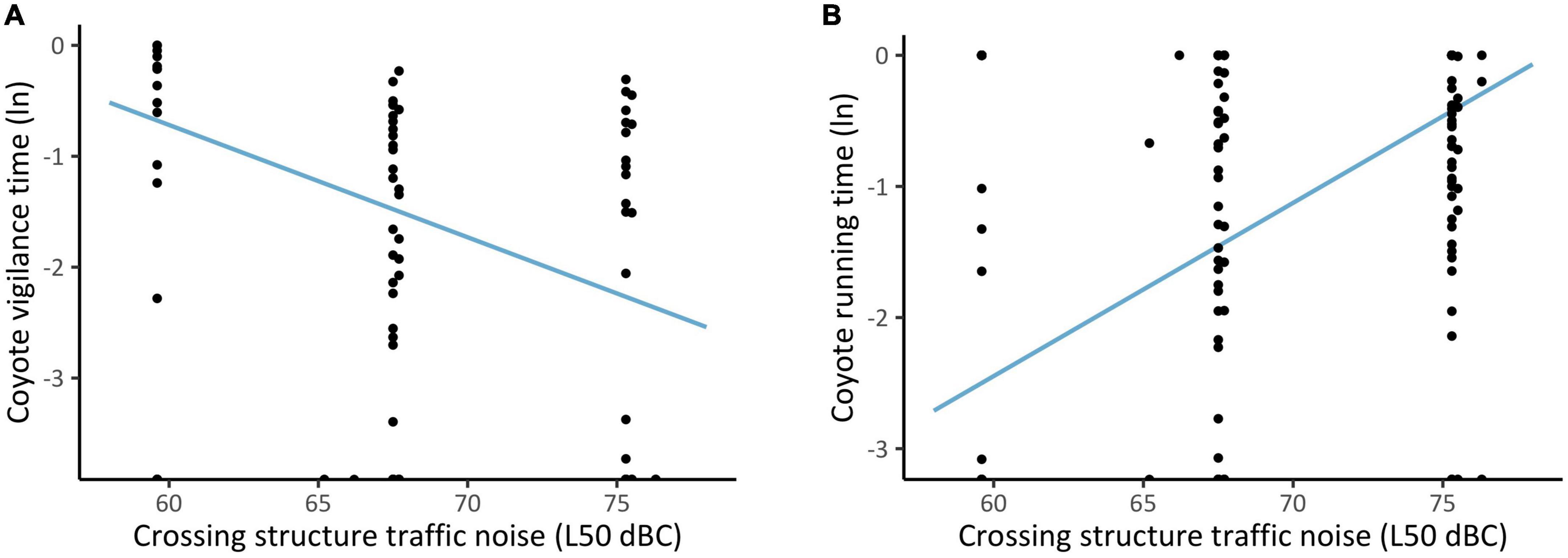
Figure 5. Proportion of time coyotes spent being (A) vigilant and (B) running at crossing structures with differing chronic (1 week) noise levels (black dots). To capture the estimated effect of chronic noise independent of other covariates, the line of best fit was generated using a predictive model.
Discussion
Acute fear responses
We found support for our hypothesis that acute noise from vehicles invokes a flight response (Figure 3). Our results provide additional support to prior findings that a fear response can be induced by sudden increases in noise associated with transportation. We found that at crossing structures where coyotes and mule deer were exposed to higher levels of acute noise from vehicles, both species were more likely to initiate a flight response than they were to use the crossing. Hermit crabs (Pagurus bernhardus) (Walsh et al., 2017; Tidau and Briffa, 2019), fish (Voellmy et al., 2014), black-tailed prairie dogs (Cynomys ludovicianus) (Shannon et al., 2016a), mule deer and mountain sheep (Ovis canadensis) (Weisenberger et al., 1996) have similarly demonstrated startle responses from acute noise, leading to increased physiological stress. Through invoking a flight rather than a crossing-entry response, acute noise pollution resulted in suboptimal behavior (Sih, 2013) for coyote and mule deer; a result that impairs connectivity across roads with potential effects on genetic exchange and access to resources (van der Ree et al., 2009). If other wildlife species experienced similar responses, the consequence would be impaired effectiveness of wildlife crossing structures as mitigation for connectivity losses.
Chronic noise
For coyotes, we detected a switch from vigilance to running at louder crossing structures (Table 1 and Figure 5). These behavioral changes are in accordance with the risk disturbance hypothesis, which posits that alterations to anti-predator behavior are due to wildlife perceiving the disturbance as a threat, and suggest that acute and chronic traffic noise can alter the “Landscape of Fear” in predators (Laundré et al., 2001). Our findings are supported by previous work that has demonstrated exposure to transportation noise can cause decreased foraging for a number of terrestrial mammals (Shannon et al., 2014b; Smith et al., 2017), marine mammals (Blair et al., 2016; Wisniewska et al., 2018), and other taxa (Wale et al., 2013; Castaneda et al., 2020). Though higher levels of chronic noise elicited a behavioral fear response in coyotes, for mule deer, chronic noise among structures elicited reduced anti-predator behavior, suggestive of a Human Shield effect (Table 1). Hence, wildlife responses to noise pollution can vary according to sound levels, temporal scale of sound, and according to species.
Our finding that increased foraging and lowered vigilance by mule deer coincided with higher levels of chronic noise among structures (Figure 4) suggests that zones of greater traffic noise may provide a refuge from predation, consistent with the Human Shield hypothesis (Berger, 2007). Ungulates have displayed similar increases in foraging in response to anthropogenic stressors within U.S. National Parks (Berger, 2007; Brown et al., 2012; Shannon et al., 2014b). Predators of mule deer have been shown to display strong fear responses to noise pollution (Smith et al., 2017). Large-bodied carnivores may also be particularly deterred from areas of high anthropogenic noise due to the low-frequency energy overlapping strongly with the frequencies in which carnivores communicate, or because of an inability to detect prey as a result of cue masking (Warren et al., 2006; Cardoso et al., 2018). Therefore, predation risk could be lower for ungulates during periods of higher noise pollution. However, in the case of roads and traffic, ungulates using the Human Shield to avoid predators may still suffer higher rates of mortality from collisions with vehicles.
Another explanation for mule deer behavior can be taken from the risk allocation hypothesis (Lima and Bednekoff, 1999), which states that wildlife exposed to long-term, continuous threats are unable to sustain anti-predatory behavior, and that anti-predator responses are used only when exposed to unpredictable, infrequent stimuli. Though we did document a fear response in mule deer to acute traffic noise, and no effect of noise when comparing behavioral events within a single crossing structure, under the risk allocation hypothesis, we would expect mule deer to exhibit no difference in anti-predator behavioral responses when exposed to chronic noise across structures. Rather, we detected a decrease in vigilance coupled with an increase in foraging of mule deer, rendering the risk allocation hypothesis a somewhat unlikely explanation for our findings.
Consequences across spatial and temporal scale
Our results indicate a differential effect of noise pollution across temporal (acute vs. chronic noise) scales for deer, and a differential effect of noise pollution across spatial (within-structure vs. among-structure) scales for both species. Previous studies have recommended examining responses to fear across multiple spatiotemporal scales to capture variation in response (Creel and Christianson, 2008; Moll et al., 2017; Prugh et al., 2019). Here, in sampling vehicular noise across different spatial and temporal dimensions, we were able to identify the mechanisms driving behavioral responses that occur across the temporal spectrum of noise. Findings for mule deer demonstrate that fear and Human Shield effects are not mutually exclusive, and that prey species concurrently assess risk at various temporal scales. Employing a hierarchical modeling framework, we also identified that species-level behavioral responses differ across spatial scales when examining chronic noise. Much of our current knowledge surrounding the relation of risk perception and behavior to spatiotemporal scale is based upon herbivore-carnivore interactions. For example patch- and landscape-level fear occur in red deer (Cervus elaphus) when inhabiting wolf territories in Poland, and African ungulates increase vigilance during times of concurrent short-term and long-term risk from predation (Kuijper et al., 2015; Dröge et al., 2017). Here we add to the ecology of fear literature and demonstrate anthropogenic stressors also mediate fear responses of wildlife across multiple temporo-spatial scales.
Additional factors impacting behavior
Our primary goal was to assess the effect of anthropogenic noise on behaviors of mammals at highway crossing structures; however, we also examined several covariates which could alter the behavioral response (Table 1 and Supplementary Tables 3, 4). First, regardless of noise condition, for mule deer in larger groups we detected a lower likelihood of a flight response at the crossing structure. This trade-off between flight response and group size at crossing structures is likely due to individuals requiring less time to scan for predators (many eyes hypothesis) in larger groups, and individuals benefiting from a lowered predation risk through dilution effects (Roberts, 1996). As an example, vigilance of California ground squirrels (Otospermophilus beecheyi) only increased when small groups, but not large groups, were exposed to loud playbacks of natural sounds (e.g., rivers) (Le et al., 2019). The proportion of time mule deer spent running also increased when in larger groups, though this may be due to juveniles playfully interacting with one another.
Second, as expected, a greater number of humans present at the crossing structure caused a decrease in deer foraging and an increase in running, particularly during the daytime. This supports previous findings demonstrating that wildlife fear human presence generally, though differentially in scale (human shield effect) and will often shift to more nocturnal activity as a way to avoid humans (Stankowich, 2008; Ciuti et al., 2012; Gaynor et al., 2018). Coyotes also demonstrated less running activity during nocturnal hours, which could be associated with exposure to artificial light from headlights and streetlamps at crossing structures. For example, mule deer use brightly lit urban areas as a human shield (Ditmer et al., 2021). Future research should include experimental playbacks of anthropogenic sound to disentangle the relationship between noise and visual disturbances.
Third, coyote running decreased and mule deer vigilance increased at camera stations closer to the crossing structure, which is indicative of a switch to individuals assessing whether to enter the structure. At camera stations farther away from the crossing structure, mule deer foraging increased. In addition, during warmer summer months, mule deer foraging increased while vigilance decreased. These behavioral shifts are likely due to higher densities of vegetation during warmer seasons and in areas farther from the concrete crossing structure. In contrast, coyote vigilance increased during warmer temperatures, potentially due to greater activity of their predators. For example, black bears (Ursus americanus) are only a predation risk for coyotes during the spring and summer months when they are not hibernating (Rogers, 1992).
Fourth, mule deer increased vigilance and lowered foraging time at crossing structures associated with greater levels of traffic volume and evergreen forest (dominant NLCD category within 100 m buffer of the crossing). It is likely that deer perceive more forested habitats, often synonymous with dark edges, and highly trafficked areas, often synonymous with greater lanes of traffic and higher speeds, to be more risky (Altendorf et al., 2001; Blackwell et al., 2014; Knopff et al., 2014; Ditmer et al., 2021).
Finally, mule deer behavior was affected by the openness ratio of the crossing structure—for more open crossings, deer were less vigilant, but also less likely to forage, more likely to run and more likely to flee away from the entrance. Previous studies have typically observed a higher propensity for deer to use structures with adequate visibility and larger openness ratios (Clevenger and Waltho, 2005; Bissonette and Rosa, 2012). The structures in California (present study) potentially had greater associated traffic volumes than the studies in Clevenger and Waltho (2005) and Bissonette and Rosa (2012), which were in the Rockies. This could contribute to differences between the present study and previous published studies. Our finding could also be driven by certain unmeasured characteristics of the structure or surrounding landscape, and future studies should go beyond measuring the openness ratio and include measures such as brightness within the structure to tease this effect apart.
Though we filtered the dataset to include a minimum time interval of 5 min between observations, and identified unique individuals within each video, we did not monitor behavior at the individual level across the entire study. To determine the impact that behavioral alterations can have on individual fitness, and whether unsuccessful crossings have a population-level impact, future research should examine whether flight is the consistent response of certain individuals, or a proportion of decisions made by all individuals. Individuals could be monitored through GPS telemetry data or software that enables individual recognition from data collected by camera traps. Monitoring individual responses could also shed light on whether fear responses are characteristic of certain personalities. For instance, Eastcott et al. (2020) found among-individual behavioral differences in vigilance behavior of dwarf mongoose to playbacks of traffic noise.
Road networks are expanding globally, with the addition of 25 million km predicted by 2,050 (Dulac, 2013; Laurance et al., 2014). With this expansion comes increased interactions between wildlife and humans. Our work demonstrates that a fear response of wildlife to anthropogenic noise stressors at wildlife crossing structures is likely to differ depending on temporal scale, species, and characteristics of the stressor. This dynamic, behavioral response to noise pollution could alter predator-prey interactions, which can scale up to ecosystem-level consequences such as trophic cascades (Smith et al., 2017; Gaynor et al., 2019; Zanette and Clinchy, 2019). Furthermore, crossing structures are proposed as the primary conservation response to road impacts to wildlife. Ineffective crossing structures, as measured by wildlife use, could result in changes in wildlife species distributions and resource availability and use, which could degrade species interactions and ecosystem health. For example, differential responses of large, common ungulates (e.g., mule deer) and their predators (e.g., mountain lions, coyotes) could lead to spatial variation in grazing and browsing intensity based on prey and predators’ responses to traffic conditions, which could lead in turn to landscape-scale changes in plant communities and wildfires. To ensure a landscape of coexistence, additional research is needed to understand what anthropogenic stressors drive fear responses in wildlife and how spatiotemporal scale plays a role. Our work suggests that to ensure the viability of wildlife crossing structures, vehicular noise pollution should be considered during the design phase of these structures.
Data availability statement
The original contributions presented in this study are included in the article/Supplementary material, further inquiries can be directed to the corresponding author.
Ethics statement
Ethical review and approval was not required for the animal study because passive remote sensing methods were used for observation only.
Author contributions
FS conceived of the study. AC and FS collected the data, designed, and performed the analysis. AC wrote the manuscript. FS and TV contributed to the data and edited the manuscript. All authors contributed to the article and approved the submitted version.
Funding
This project was made possible by a Dissertation grant to AC from the National Center for Sustainable Transportation (NCST), University of California, Davis and a research grant to FS from the NCST, supported by U.S. Department of Transportation and the California Department of Transportation through the University Transportation Centers program.
Acknowledgments
We thank David Waetjen, Rich Codington, Jamie Bourdon, Collin Raff, Catherine Le, Annabelle Louderback-Valenzuela, Mia Guarnieri, Parisa Farman, Mia Macneill, Jeffrey Scott, Pao Perez, and Vivian Lei for assistance with data collection and Rachel Alsheikh, Tricia Nguyen, and Mia Guarnieri for assistance with video tagging.
Conflict of interest
The authors declare that the research was conducted in the absence of any commercial or financial relationships that could be construed as a potential conflict of interest.
Publisher’s note
All claims expressed in this article are solely those of the authors and do not necessarily represent those of their affiliated organizations, or those of the publisher, the editors and the reviewers. Any product that may be evaluated in this article, or claim that may be made by its manufacturer, is not guaranteed or endorsed by the publisher.
Supplementary material
The Supplementary Material for this article can be found online at: https://www.frontiersin.org/articles/10.3389/fevo.2022.891595/full#supplementary-material
References
Alloush, M., Scofield, D., Marczak, S., Jones, R., Kaiser, K., Oliva, M., et al. (2011). When sounds collide: the effect of anthropogenic noise on a breeding assemblage of frogs in Belize, Central America. Behaviour 148, 215–232. doi: 10.1163/000579510X551660
Altendorf, K. B., Laundre, J. W., Gonzales, C. A. L., and Brown, J. S. (2001). Assessing effects of predation risk on foraging behavior of mule deer. J. Mammal. 82, 430–439. doi: 10.1644/1545-15422001082<0430:AEOPRO<2.0.CO;2
Araya-Salas, M., and Smith-Vidaurre, G. (2017). warbleR: An R package to streamline analysis of animal acoustic signals. Methods Ecol. Evol. 8, 184–191.
Bednekoff, P. A., and Lima, S. L. (1998). Randomness, chaos and confusion in the study of antipredator vigilance. Trends Ecol. Evol. 13, 284–287. doi: 10.1016/S0169-5347(98)01327-5
Berger, J. (2007). Fear, human shields and the redistribution of prey and predators in protected areas. Biol. Lett. 3, 620–623. doi: 10.1098/rsbl.2007.0415
Bissonette, J. A., and Rosa, S. (2012). An evaluation of a mitigation strategy for deer-vehicle collisions. Wildl. Biol. 18, 414–423. doi: 10.2981/11-122
Blackwell, B. F., Seamans, T. W., and DeVault, T. L. (2014). White-tailed deer response to vehicle approach: Evidence of unclear and present danger. PLoS One 9:e109988. doi: 10.1371/journal.pone.0109988
Blair, H. B., Merchant, N. D., Friedlaender, A. S., Wiley, D. N., and Parks, S. E. (2016). Evidence for ship noise impacts on humpback whale foraging behaviour. Biol. Lett. 12:20160005. doi: 10.1098/rsbl.2016.0005
Broner, N. (2010). A simple criterion for low frequency noise emission assessment. J. Low Freq. Noise Vib. Act. Control 29, 1–13.
Brown, C. L., Hardy, A. R., Barber, J. R., Fristrup, K. M., Crooks, K. R., and Angeloni, L. M. (2012). The effect of human activities and their associated noise on ungulate behavior. PLoS One 7:e40505. doi: 10.1371/journal.pone.0040505
Buxton, R. T., McKenna, M. F., Brown, E., Ohms, R., Hammesfahr, A., Angeloni, L. M., et al. (2020). Varying behavioral responses of wildlife to motorcycle traffic. Glob. Ecol. Conserv. 21:e00844. doi: 10.1016/j.gecco.2019.e00844
Buxton, R. T., McKenna, M. F., Mennitt, D., Fristrup, K., Crooks, K., Angeloni, L., et al. (2017). Noise pollution is pervasive in U.S. protected areas. Science 356, 531–533. doi: 10.1163/9789004313507_013
Cardoso, G. C., Hu, Y., and Francis, C. D. (2018). The comparative evidence for urban species sorting by anthropogenic noise. R. Soc. Open Sci. 5:172059. doi: 10.1098/rsos.172059
Castaneda, E., Leavings, V. R., Noss, R. F., and Grace, M. K. (2020). The effects of traffic noise on tadpole behavior and development. Urban Ecosyst. 23, 245–253.
Ciuti, S., Northrup, J. M., Muhly, T. B., Simi, S., Musiani, M., Pitt, J. A., et al. (2012). Effects of humans on behaviour of wildlife exceed those of natural predators in a landscape of fear. PLoS One 7:e50611. doi: 10.1371/journal.pone.0050611
Clevenger, A. P., and Waltho, N. (2005). Performance indices to identify attributes of highway crossing structures facilitating movement of large mammals. Biol. Conserv. 121, 453–464.
Creel, S., and Christianson, D. (2008). Relationships between direct predation and risk effects. Trends Ecol. Evol. 23, 194–201. doi: 10.1016/j.tree.2007.12.004
Creel, S., and Winnie, J. A. (2005). Responses of elk herd size to fine-scale spatial and temporal variation in the risk of predation by wolves. Anim. Behav. 69, 1181–1189. doi: 10.1016/j.anbehav.2004.07.022
Dewitz, J. (2019). National land cover database (NLCD) 2016 products, US Geological Survey data release. Reston, VA: US Geological Survey. doi: 10.5066/P96HHBIE
Ditmer, M. A., Stoner, D. C., Francis, C. D., Barber, J. R., Forester, J. D., Choate, D. M., et al. (2021). Artificial nightlight alters the predator–prey dynamics of an apex carnivore. Ecography 44, 149–161.
Dominoni, D. M., Halfwerk, W., Baird, E., Buxton, R. T., Fernández-Juricic, E., Fristrup, K. M., et al. (2020). Why conservation biology can benefit from sensory ecology. Nat. Ecol. Evol. 4, 502–511. doi: 10.1038/s41559-020-1135-4
Dröge, E., Creel, S., Becker, M. S., and M’soka, J. (2017). Risky times and risky places interact to affect prey behaviour. Nat. Ecol. Evol. 1, 1123–1128. doi: 10.1038/s41559-017-0220-9
Dulac, J. (2013). Global land transport infrastructure requirements - Estimating road and railway infrastructure capacity and costs to 2050. Paris: International Energy Agency, 54.
Eastcott, E., Kern, J. M., Morris-Drake, A., and Radford, A. N. (2020). Intrapopulation variation in the behavioral responses of dwarf mongooses to anthropogenic noise. Behav. Ecol. 31, 680–691. doi: 10.1093/beheco/araa011
Francis, C. D., and Barber, J. R. (2013). A framework for understanding noise impacts on wildlife: An urgent conservation priority. Front. Ecol. Environ. 11, 305–313. doi: 10.1890/120183
Francis, C. D., Kleist, N. J., and Catherine, P. (2012). Noise pollution alters ecological services: Enhanced pollination and disrupted seed dispersal. Proc. Biol. Sci. 279, 2727–2735. doi: 10.1098/rspb.2012.0230
Francis, C. D., Ortega, C. P., and Cruz, A. (2009). Noise pollution changes Avian communities and species interactions. Curr. Biol. 19, 1415–1419. doi: 10.1016/J.CUB.2009.06.052
Friard, O., and Gamba, M. (2016). BORIS: a free, versatile open-source event-logging software for video/audio coding and live observations. Methods Ecol. Evol. 7, 1325–1330. doi: 10.1111/2041-210X.12584
Frid, A., and Dill, L. (2002). Human-caused disturbance as a form of predation risk. J. Wildl. Manag. 78, 58–67. doi: 10.1002/jwmg.631
Fryxell, J. M., Sinclair, A. R. E., and Caughley, G. (2014). Wildlife ecology, conservation, and management. New York, NY: John Wiley & Sons.
Gaynor, K. M., Brown, J. S., Middleton, A. D., Power, M. E., and Brashares, J. S. (2019). Landscapes of fear: Spatial patterns of risk perception and response. Trends Ecol. Evol. 34, 355–368. doi: 10.1016/j.tree.2019.01.004
Gaynor, K. M., Cherry, M. J., Gilbert, S. L., Kohl, M. T., Larson, C. L., Newsome, T. M., et al. (2020). An applied ecology of fear framework: linking theory to conservation practice. Anim. Conserv. 24, 308–321. doi: 10.1111/acv.12629
Gaynor, K. M., Hojnowski, C. E., Carter, N. H., and Brashares, J. S. (2018). The influence of human disturbance on wildlife nocturnality. Science 360, 1232–1235.
Gill, J. A., Norris, K., and Sutherland, W. J. (2001). Why behavioural responses may not reflect the population consequences of human disturbance. Biol. Conserv. 97, 265–268.
Guiden, P. W., Bartel, S. L., Byer, N. W., Shipley, A. A., and Orrock, J. L. (2019). Predator–prey interactions in the anthropocene: Reconciling multiple aspects of novelty. Trends Ecol. Evol. 34, 616–627. doi: 10.1016/j.tree.2019.02.017
Hammond, T. T., Ortiz-Jimenez, C. A., and Smith, J. E. (2020). Anthropogenic change alters ecological relationships via interactive changes in stress physiology and behavior within and among organisms. Integr. Comp. Biol. 60, 57–69. doi: 10.1093/icb/icaa001
Knopff, A. A., Knopff, K. H., Boyce, M. S., and Clair, C. C. S. (2014). Flexible habitat selection by cougars in response to anthropogenic development. Biol. Conserv. 178, 136–145. doi: 10.1016/j.biocon.2014.07.017
Koch, M., and Schnitzler, H. U. (1997). The acoustic startle response in rats - Circuits mediating evocation, inhibition and potentiation. Behav. Brain Res. 89, 35–49. doi: 10.1016/S0166-4328(97)02296-1
Kuijper, D. P. J., Bubnicki, J. W., Churski, M., Mols, B., and van Hooft, P. (2015). Context dependence of risk effects: Wolves and tree logs create patches of fear in an old-growth forest. Behav. Ecol. 26, 1558–1568. doi: 10.1093/beheco/arv107
Kuijper, D. P. J., Sahlén, E., Elmhagen, B., Chamaillé-Jammes, S., Sand, H., Lone, K., et al. (2016). Paws without claws? Ecological effects of large carnivores in anthropogenic landscapes. Proc. Biol. Sci. 283:20161625. doi: 10.1098/rspb.2016.1625
Laundré, J. W., Hernández, L., and Altendorf, K. B. (2001). Wolves, elk, and bison: reestablishing the “landscape of fear” in Yellowstone National Park, U.S.A. Can. J. Zool. 79, 1401–1409. doi: 10.1139/cjz-79-8-1401
Laurance, W. F., Clements, G. R., Sloan, S., O’Connell, C. S., Mueller, N. D., Goosem, M., et al. (2014). A global strategy for road building. Nature 513, 229–232. doi: 10.1038/nature13717
Le, M. T., Garvin, C. M., Barber, J. R., and Francis, C. D. (2019). Natural sounds alter California ground squirrel, Otospermophilus beecheyi, foraging, vigilance and movement behaviours. Anim. Behav. 157, 51–60. doi: 10.1016/j.anbehav.2019.08.014
Lima, S. L., and Bednekoff, P. A. (1999). Temporal variation in danger drives antipredator behavior: The predation risk allocation hypothesis. Am. Nat. 153, 649–659. doi: 10.1086/303202
McClure, C. J. W., Ware, H. E., Carlisle, J., Kaltenecker, G., and Barber, J. R. (2013). An experimental investigation into the effects of traffic noise on distributions of birds: avoiding the phantom road. Proc. Biol. Sci. 280:20132290. doi: 10.1098/rspb.2013.2290
McKenna, M. F., Shannon, G., and Fristrup, K. (2016). Characterizing anthropogenic noise to improve understanding and management of impacts to wildlife. Endang. Spec. Res. 31, 279–291. doi: 10.3354/esr00760
Meillère, A., Brischoux, F., and Angelier, F. (2015). Impact of chronic noise exposure on antipredator behavior: An experiment in breeding house sparrows. Int. Soc. Behav. Ecol. 26, 569–577. doi: 10.1093/beheco/aru232
Moll, R. J., Redilla, K. M., Mudumba, T., Muneza, A. B., Gray, S. M., Abade, L., et al. (2017). The many faces of fear: A synthesis of the methodological variation in characterizing predation risk. J. Anim. Ecol. 86, 749–765. doi: 10.1111/1365-2656.12680
Mtui, E. K. (2014). Road constraints on impala (Aepyceros melampus) behavior. Trondheim: Norwegian University of Science and Technology.
Nedelec, S. L., Mills, S. C., Lecchini, D., Nedelec, B., Simpson, S. D., and Radford, A. N. (2016). Repeated exposure to noise increases tolerance in a coral reef fish. Environ. Pollut. 216, 428–436. doi: 10.1016/j.envpol.2016.05.058
Neo, Y. Y., Hubert, J., Bolle, L. J., Winter, H. V., and Slabbekoorn, H. (2018). European seabass respond more strongly to noise exposure at night and habituate over repeated trials of sound exposure. Environ. Pollut. 239, 367–374. doi: 10.1016/j.envpol.2018.04.018
Owen, M. A., Swaisgood, R. R., and Blumstein, D. T. (2017). Contextual influences on animal decision-making: Significance for behavior-based wildlife conservation and management. Integr. Zool. 12, 32–48. doi: 10.1111/1749-4877.12235
Palmer, M. S., and Packer, C. (2021). Reactive anti-predator behavioral strategy shaped by predator characteristics. PLoS One 16:e0256147. doi: 10.1371/journal.pone.0256147
Prugh, L. R., Sivy, K. J., Mahoney, P. J., Ganz, T. R., Ditmer, M. A., van de Kerk, M., et al. (2019). Designing studies of predation risk for improved inference in carnivore-ungulate systems. Biol. Conserv. 232, 194–207. doi: 10.1016/j.biocon.2019.02.011
Qin, J., Sun, P., and Walker, J. (2014). “Measurement of field complex noise using a novel acoustic detection system,” in Proceedings of the 2014 IEEE AUTOTEST, (St. Louis, MO), 177–182. doi: 10.1109/AUTEST.2014.6935142
Quinn, J. L., Whittingham, M. J., Butler, S. J., and Cresswell, W. (2006). Predation risk compensation and vigilance in the chaffinch Fringilla coelebs. J. Avian Biol. 37, 601–608.
Roberts, G. (1996). Why individual vigilance declines as group size increases. Anim. Behav. 51, 1077–1086. doi: 10.1006/anbe.1996.0109
Rytwinski, T., and Fahrig, L. (2015). “The impacts of roads and traffic on terrestrial animal populations,” in Handbook of road ecology, eds R. van der Ree, D. J. Smith, and C. Grilo (New York, NY: John Wiley & Sons), 237–246.
Rytwinski, T., Soanes, K., Jaeger, J. A. G., Fahrig, L., Findlay, C. S., Houlahan, J., et al. (2016). How effective is road mitigation at reducing road-kill? A meta-analysis. PLoS One 11:e0166941. doi: 10.1371/journal.pone.0166941
Schmitz, O. J., Wilmers, C. C., Leroux, S. J., Doughty, C. E., Atwood, T. B., Galetti, M., et al. (2018). Animals and the zoogeochemistry of the carbon cycle. Science 362:eaar3213. doi: 10.1126/science.aar3213
Shannon, G., Cordes, L. S., Hardy, A. R., Angeloni, L. M., and Crooks, K. R. (2014b). Behavioral responses associated with a human-mediated predator shelter. PLoS One 9:e94630. doi: 10.1371/journal.pone.0094630
Shannon, G., Angeloni, L. M., Wittemyer, G., Fristrup, K. M., and Crooks, K. R. (2014a). Road traffic noise modifies behaviour of a keystone species. Anim. Behav. 94, 135–141. doi: 10.1016/j.anbehav.2014.06.004
Shannon, G., McKenna, M. F., Angeloni, L. M., Crooks, K. R., Fristrup, K. M., Brown, E., et al. (2016b). A synthesis of two decades of research documenting the effects of noise on wildlife. Biol. Rev. 91, 982–1005. doi: 10.1111/brv.12207
Shannon, G., Crooks, K. R., Wittemyer, G., Fristrup, K. M., and Angeloni, L. M. (2016a). Road noise causes earlier predator detection and flight response in a free-ranging mammal. Behav. Ecol. 27, 1370–1375. doi: 10.1093/beheco/arw058
Siemers, B. M., and Schaub, A. (2011). Hunting at the highway: Traffic noise reduces foraging efficiency in acoustic predators. Proc. R. Soc. Lond. B Biol. Sci. 278, 1646–1652. doi: 10.1098/rspb.2010.2262
Sih, A. (2013). “A behavioral ecology view of decision making: Something old, something borrowed, something new,” in Comparative decision making, eds P. H. Crowley and T. R. Zentall (New York, NY: Oxford University Press), 243–270. doi: 10.1093/acprof:oso/9780199856800.003.0024
Smith, J. A., Suraci, J. P., Clinchy, M., Crawford, A., Roberts, D., Zanette, L. Y., et al. (2017). Fear of the human “super predator” reduces feeding time in large carnivores. Proc. Biol. Sci. 284, 20170433. doi: 10.1098/rspb.2017.0433
Stankowich, T. (2008). Ungulate flight responses to human disturbance: A review and meta-analysis. Biol. Conserv. 141, 2159–2173. doi: 10.1016/j.biocon.2008.06.026
Støen, O. G., Ordiz, A., Evans, A. L., Laske, T. G., Kindberg, J., Fröbert, O., et al. (2015). Physiological evidence for a human-induced landscape of fear in brown bears (Ursus arctos). Physiol. Behav. 152, 244–248. doi: 10.1016/j.physbeh.2015.09.030
Strano, E., Simini, F., De Nadai, M., Esch, T., and Marconcini, M. (2020). Precise mapping, spatial structure and classification of all the human settlements on Earth. arXiv [Preprint] 1–43. arXiv:2006.06584.
Sueur, J., Aubin, T., Simonis, C., Lellouch, L., Aumond, P., Brown, E. C., et al. (2020). Package “seewave” type package title sound analysis and synthesis.
Suraci, J. P., Clinchy, M., Zanette, L. Y., and Wilmers, C. C. (2019). Fear of humans as apex predators has landscape-scale impacts from mountain lions to mice. Ecol. Lett. 22, 1578–1586. doi: 10.1111/ele.13344
Suraci, J. P., Nickel, B. A., and Wilmers, C. C. (2020). Fine-scale movement decisions by a large carnivore inform conservation planning in human-dominated landscapes. Landsc. Ecol. 35, 1635–1649. doi: 10.1007/s10980-020-01052-2
Tidau, S., and Briffa, M. (2019). Distracted decision makers: Ship noise and predation risk change shell choice in hermit crabs. Behav. Ecol. 30, 1157–1167. doi: 10.1093/beheco/arz064
van de Pol, M., and Wright, J. (2009). A simple method for distinguishing within- versus between-subject effects using mixed models. Anim. Behav. 77, 753–758. doi: 10.1016/j.anbehav.2008.11.006
van der Ree, R., Heinze, D., McCarthy, M., and Mansergh, I. (2009). Wildlife tunnel enhances population viability. Ecol. Soc. 14:7. doi: 10.5751/ES-02957-140207
Voellmy, I. K., Purser, J., Flynn, D., Kennedy, P., Simpson, S. D., and Radford, A. N. (2014). Acoustic noise reduces foraging success in two sympatric fish species via different mechanisms. Anim. Behav. 89, 191–198. doi: 10.1016/j.anbehav.2013.12.029
Wale, M. A., Simpson, S. D., and Radford, A. N. (2013). Noise negatively affects foraging and antipredator behaviour in shore crabs. Anim. Behav. 86, 111–118. doi: 10.1016/j.anbehav.2013.05.001
Walsh, E. P., Arnott, G., and Kunc, H. P. (2017). Noise affects resource assessment in an invertebrate. Biol. Lett. 13:20170098. doi: 10.1098/rsbl.2017.0098
Walthers, A. R., and Barber, C. A. (2020). Traffic noise as a potential stressor to offspring of an urban bird, the European Starling. J. Ornithol. 161, 459–467. doi: 10.1007/s10336-019-01733-z
Ware, H. E., McClure, C. J., Carlisle, J. D., and Barber, J. R. (2015). A phantom road experiment reveals traffic noise is an invisible source of habitat degradation. Proc. Natl. Acad. Sci. U.S.A. 112, 12105–12109. doi: 10.1073/pnas.1504710112
Warren, P. S., Katti, M., Ermann, M., and Brazel, A. (2006). Urban bioacoustics: it’s not just noise. Anim. Behav. 71, 491–502.
Weisenberger, M. E., Krausman, P. R., Wallace, M. C., De Young, D. W., and Maughan, O. E. (1996). Effects of simulated jet aircraft noise on heart rate and behavior of desert ungulates. J. Wildl. Manag. 60:52. doi: 10.2307/3802039
Wisniewska, D. M., Johnson, M., Teilmann, J., Siebert, U., Galatius, A., Dietz, R., et al. (2018). High rates of vessel noise disrupt foraging in wild harbour porpoises (Phocoena phocoena). Proc. Biol. Sci. 285:20172314. doi: 10.1098/rspb.2017.2314
Ydenberg, R. C., and Dill, L. M. (1986). “The economics of fleeing from predators,” in Advances in the study of behavior, eds J. S. Rosenblatt, C. Beer, M.-C. Busnel, and P. J. B. Slater (Cambridge, MA: Academic Press), 229–249. doi: 10.1016/S0065-3454(08)60192-8
Keywords: wildlife crossing structures, noise, fear effects, multi-scale, human-wildlife coexistence, habitat connectivity, road ecology
Citation: Collins AC, Vickers TW and Shilling FM (2022) Behavioral responses to anthropogenic noise at highways vary across temporal scales. Front. Ecol. Evol. 10:891595. doi: 10.3389/fevo.2022.891595
Received: 07 March 2022; Accepted: 04 November 2022;
Published: 30 November 2022.
Edited by:
Orsolya Valkó, Hungarian Academy of Sciences, HungaryReviewed by:
Aaron Wirsing, University of Washington, United StatesMriganka Shekhar Sarkar, Govind Ballabh Pant National Institute of Himalayan Environment and Sustainable Development, India
Copyright © 2022 Collins, Vickers and Shilling. This is an open-access article distributed under the terms of the Creative Commons Attribution License (CC BY). The use, distribution or reproduction in other forums is permitted, provided the original author(s) and the copyright owner(s) are credited and that the original publication in this journal is cited, in accordance with accepted academic practice. No use, distribution or reproduction is permitted which does not comply with these terms.
*Correspondence: Amy C. Collins, YWNjb2xsaW5zQHVjZGF2aXMuZWR1