- 1Agroécologie, Institut Agro, INRAE, Université de Bourgogne, University Bourgogne Franche-Comté, Besançon, France
- 2Agroécologie, Institut Agro, CNRS, INRAE, Université de Bourgogne, University Bourgogne Franche-Comté, Besançon, France
Modern wheat varieties that were selected since the Green Revolution are generally grown with synthetic chemical inputs, and ancient varieties released before1960 without. Thus, when changes occur in rhizosphere microbiota structure, it is not possible to distinguish if they are due to (i) changes in wheat genotypes by breeding, (ii) modifications of the environment via synthetic chemical inputs, or (iii) phenotypic plasticity, the interaction between wheat genotype and the environment. Using a crossed factorial design in the field, we evaluated the effects of either modern or ancient wheat varieties grown with or without chemical inputs (a N fertilizer, a fungicide, and an herbicide) on “microbiome as a phenotype.” We analyzed the rhizosphere microbiota by bacterial and fungal amplicon sequencing, coupled with microscope observations of mycorrhizal associations. We found that plant genotype and phenotypic plasticity had the most influence on rhizosphere microbiota, whereas inputs had only marginal effects. Phenotypic plasticity was particularly important in explaining diversity variations in bacteria and fungi but had no impact on the mycorrhizal association. Our results show an interest in considering the interaction between wheat genotype and the environment in breeding programs, by focusing on genes involved in the phenotypic plasticity of plant-microbe interactions.
Introduction
Plant genotypes have an influence on the composition of rhizosphere microbiota (Lundberg et al., 2012; Peiffer et al., 2013) and function (Lemanceau et al., 2017), which in turn affects plant growth, development, and immunity (Mendes et al., 2011; Berendsen et al., 2012). The rhizosphere microbiota recruited by plants is sensitive to changes in root exudate composition (Lebeis et al., 2015; Hu et al., 2018), and recently, plant loci correlated with specific subsets of rhizosphere microbiota were identified by genome-wide association (Deng et al., 2021). Understanding factors favoring crop associations with beneficial microbes may help in maintaining high crop yields while decreasing synthetic chemical inputs (e.g., man-made herbicides, fungicides, and mineral fertilizers). To steer beneficial interactions between plants and microbiota, two strategies are developed: manipulating soil microbial community directly (Jacquiod et al., 2022) and manipulating plant traits involved in microbial interactions (Bakker et al., 2012). In the latter, the goal can be to enhance specific microbial functions (e.g., nitrogen fixation/mineralization) or enhance community intrinsic properties (e.g., diversity, evenness, and composition) associated with beneficial effects on plants (Bakker et al., 2012).
However, plant breeding has changed interactions with soil microorganisms (Milla et al., 2015; Turcotte et al., 2015). An effect of domestication, from wild relatives to cultivated varieties, on rhizosphere microbiota was observed in barley (Bulgarelli et al., 2015), maize (Szoboszlay et al., 2015), foxtail millet (Chaluvadi and Bennetzen, 2018), and common bean (Pérez-Jaramillo et al., 2017), generally leading to microbiota changes between wild relatives and selected varieties (Pérez-Jaramillo et al., 2018). Domestication was also shown to decrease the mycorrhizal associations and yield gain responsiveness of selected wheat varieties (Kapulnik and Kushnir, 1991; Hetrick et al., 1992). A similar effect was observed in relation to a recent selection effort that started with the Green Revolution (Smale, 1997) between varieties released after 1960 (hereafter called “modern”) and those released before 1960 (hereafter called “ancient”) (Leiser et al., 2016). This recent selection effort was linked with a relaxed selective process of wheat microbiota assembly (Hassani et al., 2020), but the effects are small (Özkurt et al., 2020) and apparently more pronounced for fungi (Spor et al., 2020). However, meta-analyses concluded that agricultural and breeding practices are not responsible for a decrease in mycorrhizal responses in modern varieties (An et al., 2010; Lehmann et al., 2012). The first hypothesis tested in our study is that recent selection has changed wheat rhizosphere microbiota community structure via deteriorated ability to maintain high microbial diversity and mycorrhizal levels in modern varieties.
From another perspective, rhizosphere microbiota changes observed since the Green Revolution may be attributed to the field application of synthetic chemical inputs (e.g., fertilizers, herbicides, and fungicides, hereafter called “inputs”). Effects of inputs were linked with decreased microbial diversity while favoring only few taxa (Dunn et al., 2021). Among different inputs, fertilization is often reported in the literature for its impact on soil microbiota. In a meta-analysis, long-term mineral fertilization was linked with increased microbial biomass (Geisseler and Scow, 2014). Fertilization alters soil microbiota composition by promoting copiotroph microorganisms (Ramirez et al., 2010, 2012). Modifications of the bulk soil microbial reservoir instigated by fertilization are associated with altered rhizosphere community assembly in lettuces (Chowdhury et al., 2019), legumes (Weese et al., 2015), and wheat (Ai et al., 2015; Chen et al., 2019). Similarly, fertilization may reduce the diversity (Egerton-Warburton et al., 2007) and cost-benefit balance of arbuscular mycorrhizal fungi (Lambers et al., 2009). Since fertilizers could promote the dominance of copiotrophs and fungicides could prevent fungal diseases, we formulate a second hypothesis: the presence of inputs will decrease the microbial diversity and abundance of arbuscular mycorrhizal fungi in the wheat rhizosphere.
Furthermore, it needs to be emphasized that the recent selection effort and the use of inputs are two interconnected agricultural practices that evolved in parallel: modern varieties were selected and are generally grown with synthetic inputs, whereas ancient varieties have been selected before 1960 at a time where inputs were not used in a systematic way and are generally grown today in organic farming without synthetic inputs. Studies on the effect of recent selection efforts on the wheat rhizosphere microbiota do not explicitly consider inputs (Spor et al., 2020). Likewise, studies focusing on the effect of inputs on the assembly of wheat rhizosphere microbiota do not consider the recent selection efforts between ancient and modern varieties (Chen et al., 2019). This tangled association between genotypes and inputs, even if applied at low doses (e.g., Voss-Fels et al., 2019), prevents the assessment of their relative importance on wheat rhizosphere microbiota assembly. It is thus paramount to assess the interaction between genotypes and inputs, a neglected aspect in rhizosphere microbiota studies. Here, we formulate our third hypothesis: the effect of recent selection efforts on wheat rhizosphere microbiota and mycorrhizal associations is influenced by inputs.
To test our hypotheses, the effects of recent breeding efforts (ancient vs. modern varieties) and inputs (absence vs. presence) on rhizosphere microbiota assembly can be described using the formalism of quantitative genetics “P = G + E + G × E” (Falconer, 1989). Indeed, beyond morphological or physiological traits of plants, rhizosphere microbiota can be considered as an extended phenotype of plants (de la Fuente Cantó et al., 2020) with an important influence on plant fitness (Lemanceau et al., 2017). As such, the structural and functional properties of rhizosphere microbiota can be considered a host phenotype (Oyserman et al., 2021) that contributes to the inclusive fitness of the host (Danchin et al., 2011). Here, we thus consider the diversity of rhizosphere microbiota as a phenotypical trait “P,” which can be determined by (i) plant genotype “G” (hereafter called “breeding type” differentiating modern and ancient varieties), (ii) environment “E” (modified by agricultural practices such as application of inputs, here an herbicide, a fungicide, and a nitrogen fertilizer), and (iii) interaction between wheat genotype and inputs “G × E,” defined as plant phenotypic plasticity. Phenotypic plasticity denotes the ability of a genotype to exhibit changes in a specific trait across different environments (Laitinen and Nikoloski, 2019), usually visualized as a “norm of reaction” where phenotype changes in each genotype are depicted across environments (Schmalhausen, 1949; Stearns, 1989). Phenotypic plasticity could explain discrepancies between studies regarding the microbial diversity associated with ancient and modern varieties due to environmental differences. It is thus mandatory to evaluate the relative importance of G (hypothesis 1), E (hypothesis 2), and the G × E interaction (hypothesis 3). We thus set an experiment with five ancient and five modern wheat varieties with or without inputs (Supplementary Figure 1) and analyzed rhizosphere microbiota with an integrated approach coupling microscopic observations of mycorrhiza with amplicon sequencing of the bacterial (16S rRNA gene) and fungal (ITS2) communities to determine the relative importance of the three terms in explaining variations in wheat rhizosphere microbiota.
Materials and Methods
Field Site and Experimental Design
The field experiment was conducted at Institu Agro Dijon (47° 18′32 ″N 5° 04′02″ E, Dijon, France preceding culture: Vicia faba). The soil is Calcosol (−22 cm: pHH2O = 8; 34.6% clay, 36.2% loam, and 29.2% sand; 46.2 g.kg–1 organic matter, 26.7 g.kg–1 organic carbon, and 2.11 g.kg–1 total nitrogen; 294 g.kg–1 total Ca; 0.02 g.kg–1 phosphorous pentoxide (P2O5); 24.3 cmol+.kg–1 CEC). Plots (1 × 1 m2, separated by 0.8-m-wide paths) were randomized and distributed in three repeated blocks of 20: five plots for ancient varieties without inputs, five plots for ancient varieties with inputs, five plots for modern varieties without inputs, and five plots for modern varieties with inputs for a total of 60 plots across the three blocks, with N = 15 for each breeding type × input modality (Supplementary Figure 2). Seeds were sown manually (3 and 4 November 2016). We selected two groups of varieties, one that has not been submitted to the recent selection effort in high input systems and one that has been selected in such systems. Based on the hypothesis of disruption of plant-microbial symbiosis, our rationale was that genotypes continuously grown without synthetic inputs and exposed to local microbial communities should have kept ancestral interactions with microorganisms, whereas genotypes selected in high-input systems could have lost these ancestral plant-microbe interactions because of evolutionary trade-offs between selected traits and symbiosis traits (Porter and Sachs, 2020). In the group of varieties selected in high-input systems, we chose five of the most common modern varieties currently grown in the Burgundy region from a diversity of seed suppliers (Table 1). In the group of varieties selected and grown without synthetic inputs, we chose five grown locally among 200 owned by the non-governmental organization “Graines de Noé”1, which promotes in situ conservation of wheat landraces. Information on ancient varieties was retrieved from Jacques Le Gouis and François Balfourier (Centre de Ressources Biologiques « Céréales à paille » of the INRAE Clermont-Ferrand, France). The panel (Table 1) is made of two varieties with a name attesting to their local origin (Barbu du Mâconnais and Blé de Saône) and the most commonly grown by organic farmers in Burgundy (Automne Rouge). To avoid a potential bias due to local selection (small population size amplifying stochastic genetic effects or inbreeding), we supplemented the panel with Alauda, a German crossbreed of Probus (1948, Swiss) × Inntaler (< 1960) specifically selected for organic farming (indeed recent but not selected in high-input systems) and einkorn wheat (Triticum monococcum), an external taxon that is likely to share ancestral plant-microbes interactions, since it was also not selected in high-input systems. These ancient varieties are not fixed genetically, but the in situ conservation method and their phenotypical stability suggested a relative genetic homogeneity. Each variety was grown either (i) with inputs (w) or (ii) without inputs (w/o). Inputs included practices typically applied by local farmers in Burgundy: a fertilizer (ammonium nitrate 27%, Dijon Céréales, Longvic, France) for a total of 150 kg N.ha–1, was applied as 50 kg N.ha–1 three times, on week 18 after sowing (9 March 2017), week 25 (24 April 2017), and week 30 (30 March 2017); a herbicide (Bofix™; Dow Agro Science) was supplied once at 0.3 l.ha–1 on week 23 (10 April 2017), and a fungicide (Bell Star™; Dow Agro Science) was applied once at 2.5 kg.ha–1 on week 26 (5 May 2017).
Rhizosphere Soil Sampling
Sampling was conducted before wheat heading. In each plot, two plants were sampled in opposed corners (green circles, Supplementary Figure 2). All field and laboratory equipment was cleaned with ethanol 70% before each use. Root systems with adhering soil were retrieved by loosening the soil with a fork and immediately transferred to the laboratory. Rhizosphere soil was collected using sterilized brushes. Two rhizosphere soils coming from the same plot were pooled to obtain representative samples and stored at −20 °C. Total DNA was extracted from 250 mg of the rhizosphere soil using a DNeasy PowerSoil-htp 96-well DNA isolation kit (Qiagen, France) based on a combined mechanical and alkaline lysis coupled with chromatography purification, which guarantees the molecular-grade recovery of soil metagenomic DNA.
Microbial Community Sequencing
Bacterial/fungal amplicon sequencing was adapted from previously described methods (Spor et al., 2020). Total bacterial and fungal diversity and composition were obtained by sequencing the V3-V4 hypervariable regions of the bacterial 16S rRNA gene (small subunit of the ribosomal operon, Baker et al., 2003; Herlemann et al., 2011) and the ITS2 region (Internal Transcribed Spacer 2 in the ribosomal operon, White et al., 1990; Ihrmark et al., 2012) by Illumina Miseq 2 × 250-bp paired-end analysis. For consistency, we analyzed both the 16S rRNA and ITS2 sequences using an OTU pipeline instead of Amplicon Sequence Variants (ASV), since the latter is currently not optimal for fungal ITS (Estensmo et al., 2021). Plant contaminations were removed from 16S rRNA gene amplicon sequences by systematically removing OTUs sharing at least 98% identity with a wheat plastid DNA sequence (Triticum aestivum chloroplast; accession: NC_002762.1:c123842-122352 and NC_002762.1:91051-92542). A summary of sequenced samples is provided in Supplementary Table 1. Raw sequences have been deposited to the SRA (Sequence Read Archive, bacteria: SUB9063594, Fungi: SUB9104701) public repository.
Statistical Analysis
Data analysis was performed with the Rstudio software (R Core Team, 2020). We used the following model: “P ∼ E + G/Variety + G*E + block,” where “P” is the rhizosphere microbiota parameter considered (e.g., diversity indices), “G” is the genotype, defined as the breeding type (either modern or ancient varieties), and “E” is the environment, defined as inputs (either with or without). We added a “variety” factor to consider the random variance that could arise from the difference between varieties inside the modern or ancient groups (“G/variety”). The effect of spatial heterogeneity in our experimental site was assessed through the “block” factor with an additive effect. Since observations of mycorrhiza were conducted in two batches, we specifically included an additive “batch” factor in the model (refer to the Mycorrhizal colonization section). Normality and homoscedasticity were assessed by Shapiro and Bartlett tests. Normally distributed data were analyzed with ANOVA models, followed by post hoc Tukey’s honest significant difference test (Tukey’s HSD, p < 0.05, package ‘agricolae,’ de Mendiburu and Yaseen, 2020). Data that were not normally distributed were analyzed with the non-parametric Scheirer-Ray-Hare tests followed by post hoc false discovery rate test correction to account for multiple testing (FDR, p < 0.05, “rcompanion” and “FSA,” Sokal and Rohlf, 1995). In the Results section, we present the percentage of variance explained and associated p-values from the ANOVA and Scheirer-Ray-Hare models.
Alpha Diversity
Sequencing completeness and quality were verified with rarefaction curves (“vegan”; Dixon, 2003; Supplementary Figure 3), enabling the identification of eight outlier samples coming from the same plate column harboring significantly higher sequence counts but relatively lower OTU discovery rates than the others (p < 0.001). The samples were excluded from further analyses, leaving N = 11–14 in each G × E modality, with at least four biological replicates per variety (Supplementary Table 1). Sequencing profiles were resampled at 14,000 counts to normalize diversity discoveries (Schöler et al., 2017), and the following indices were used: observed taxon richness (S: the number of OTUs detected), Chao-1 (estimation of real taxon richness based on rare taxon occurrence represented by one sequence in a sample), ACE (abundance-based coverage estimator: estimation of real taxon richness based on rare taxon occurrence represented by 10 sequences in a sample), Simpson Reciprocal index (1/D, D = dominance, the probability of picking the same taxon twice from two random drawings), Shannon index (H: a measure of species diversity and evenness based on the sum of all species contribution in a community), and Pielou’s equitability (J = H/ln(S), an index ranging from 0 to 1 indicating the level of evenness in the community). The models are available in Supplementary Table 2 (Bacteria) and Supplementary Table 3 (Fungi). A PERMANOVA was performed on the six diversity indices (Supplementary Table 4). The distribution and phylogenetic composition of core OTU fractions were visualized in Venn diagrams (Supplementary Figure 4).
Beta Diversity
Resampled sequencing profiles were analyzed by PERMANOVA (“vegan,” Bray-Curtis dissimilarity, Supplementary Table 4). As only breeding type and block had significant effects, a partial distance-based redundancy analysis (db-RDA) was performed to visualize the profiles with the model: “breeding type + condition (block)” (“capscale” function, 10,000 permutations). OTUs whose abundance was significantly altered among breeding types (G), inputs (E), and phenotypic plasticity (G x E) were detected using non-rarefied data by quasi-likelihood F-test (QLFT) under negative binomial distributions and generalized linear models (nb-GLM, package “edgeR,” Robinson et al., 2009), as recommended in the literature (Schöler et al., 2017; Jacquiod et al., 2020). Only OTUs with abundance fold-change > 2, overall occurrence > 20 times, and FDR-adjusted p-value < 0.05 were considered (Supplementary Table 5).
Network Analysis
Modern and ancient wheat variety rhizosphere microbiota were visualized as networks using Poisson Log Normal (PLN) models, a performant method for sparse count data that enables control of dependency effects arising from compositional profiles (PLN, package “PLNmodels,” Chiquet et al., 2019). Partial correlation matrices integrating both core bacterial and fungal OTUs in the ancient (N = 26/26) and modern varieties (N = 24/24) were computed using the “Total Sum Scaling” offset criteria (TSS) and the following model: “∼ 1 + block + input + offset.” PLN models were validated with the Bayesian Information Criterion (BIC) to set the appropriate sparsifying penalty levels. The complexity of networks was investigated by means of the degree index (average number of edges per node), node betweenness (average shortest node path between node pairs), and edge betweenness (average shortest edge path between edge pairs). We identified keystone species, i.e., species that have a disproportionate deleterious effect on the community network structure upon their removal (Berry and Widder, 2014), as those having the highest degree, reaching 40% of the total proportion of edges. Edge arithmetic was applied with the “igraph” package function (Csardi and Nepusz, 2006; Jacquiod et al., 2020) to intersect the modern and ancient networks. Visualization of the finalized network was performed with the “Cytoscape” software (Shannon et al., 2003). Separated networks obtained from the modern and ancient varieties are presented in supporting information (Supplementary Figure 5).
Mycorrhizal Colonization
Two root systems per plot were collected and pooled together 29 weeks after sowing (22–24 May 2017). The root material was washed and stained (Vierheilig et al., 1998). Roots were cleared (90 °C, 5–10 min, 10% KOH), dipped in black ink (90 °C, 5 min, 5% in acetic acid), rinsed (with water), dipped in acetic acid (8%, 25 min, room temperature), rinsed (with water), dipped in pure glycerol, and stored (at 4 °C). For microscopic observation, roots were cut (1 cm), and 15 fragments were mounted with glycerol between slides (see pictures in Supplementary Figure 6). To avoid discoloration during conservation, the root samples were divided into two staining batches. A significant difference in staining intensity was found between the two batches (Supplementary Figure 7). To account for coloration fluctuations, the two batches were identified in the statistical model as a “+ batch” factor. Mycorrhization rates were assessed according to Trouvelot et al. (1986) via the following indices: frequency of root fragments with mycorrhizal structures on the root system scale (F), the intensity of mycorrhizal colonization on the root system scale (M) or restricted to mycorrhizal root fragments (m), and arbuscular abundance on the root system scale (A) or restricted to mycorrhizal root fragments (a). Univariate models and the global PERMANOVA model are available in supporting data (Supplementary Tables 4, 6).
Results
Sequencing Statistics
From the 60 initial rhizosphere samples, 51 (bacteria) and 50 (fungi) amplicon profiles passed the quality control for downstream analysis. For bacterial profiles, 2,099,307 raw sequences were recovered, averaging 41,163 ± 1,049 sequences per sample (standard error of the mean). For fungal profiles, 3,993,837 raw sequences were recovered, averaging 79,877 ± 2,120 sequences per sample. After assembly and cleaning, the bacterial profiles yielded 1,076,714 raw sequences, averaging 21,112 ± 597 sequences per sample. Clean fungi profiles yielded 1,333,569 raw sequences, averaging 26,671 ± 1,001 sequences per sample. All the bacterial and fungal profiles could be resampled at a minimal depth of 14,000 sequences.
Effects on the Bacterial Community
We first performed a global analysis on all diversity indices to detect main effects by PERMANOVA (Table 2 and Supplementary Table 4), and 63.5% of the variance was explained by our model, with a significant effect of breeding type (7.4%, p = 0.013), the G × E interaction (5.7%, p = 0.034), and different varieties nested in each breeding type (48.8%, p = 0.015). In detail, breeding type (ancient vs. modern varieties) had a significant effect on all the diversity indices: richness (explaining 15.57% of the total variance, p < 0.001), Chao-1 (6.89%, p = 0.025), ACE (5.87%, p = 0.044), Simpson reciprocal (9.18%, p = 0.032), Shannon (20.08%, p = 0.001), and equitability (13.82%, p = 0.009) (Table 3 and Supplementary Table 2). The modern varieties had higher diversity than the ancient varieties (Figure 1). The presence of inputs had a significant negative effect on community evenness via the Simpson reciprocal (7.83%, p = 0.048). The G × E interaction had a significant effect on richness (7.7%, p = 0.011), ACE (6.8%, p = 0.031), and Shannon (8.64%, p = 0.038), as the addition of inputs led to a significant decrease in diversity only for the ancient varieties (Figure 1). The different varieties nested in each breeding type was the main source of variation in the richness (46.1%, p = 0.009), Chao1 (49.9%, p = 0.015), and ACE (47.5%, p = 0.028) indices. No effect of spatial heterogeneity was detected (block: p > 0.05). The visual distribution of bacterial taxa in Venn diagrams across G × E treatments allowed the detection of distinct OTUs associated with breeding type and inputs and their interaction and covering a broad phylogenetic diversity (Supplementary Figure 4). The modern varieties had more unique taxa (61 including unique representatives from Gemmatimonadetes and TM7) than the ancient ones (34 including unique representatives from Planctomycetes). Similar numbers of unique OTUs were detected without (42) and with inputs (46 including unique representatives from Gemmatimonadetes and Planctomycetes). The G × E interaction yielded 98 (without inputs) and 92 (with inputs) unique taxa in the ancient varieties, while 86 (without inputs) and 110 (with inputs) unique taxa with similar phylogenetic signatures in all fractions were observed in the modern varieties.
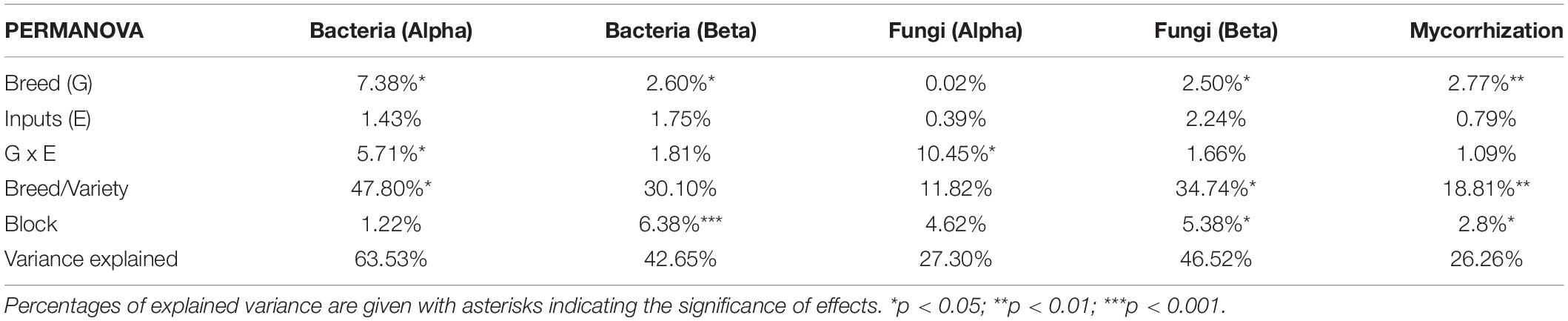
Table 2. Permutational analysis of variance for bacterial and fungal alpha diversity (based on the six diversity indices: richness, Chao-1, ACE, Simpson reciprocal, Shannon, and equitability), beta diversity (based on Bray-Curtis dissimilarity index), and for parameters describing mycorrhizal colonization (F, M, m, A, and a, refer to Materials and methods for a description).
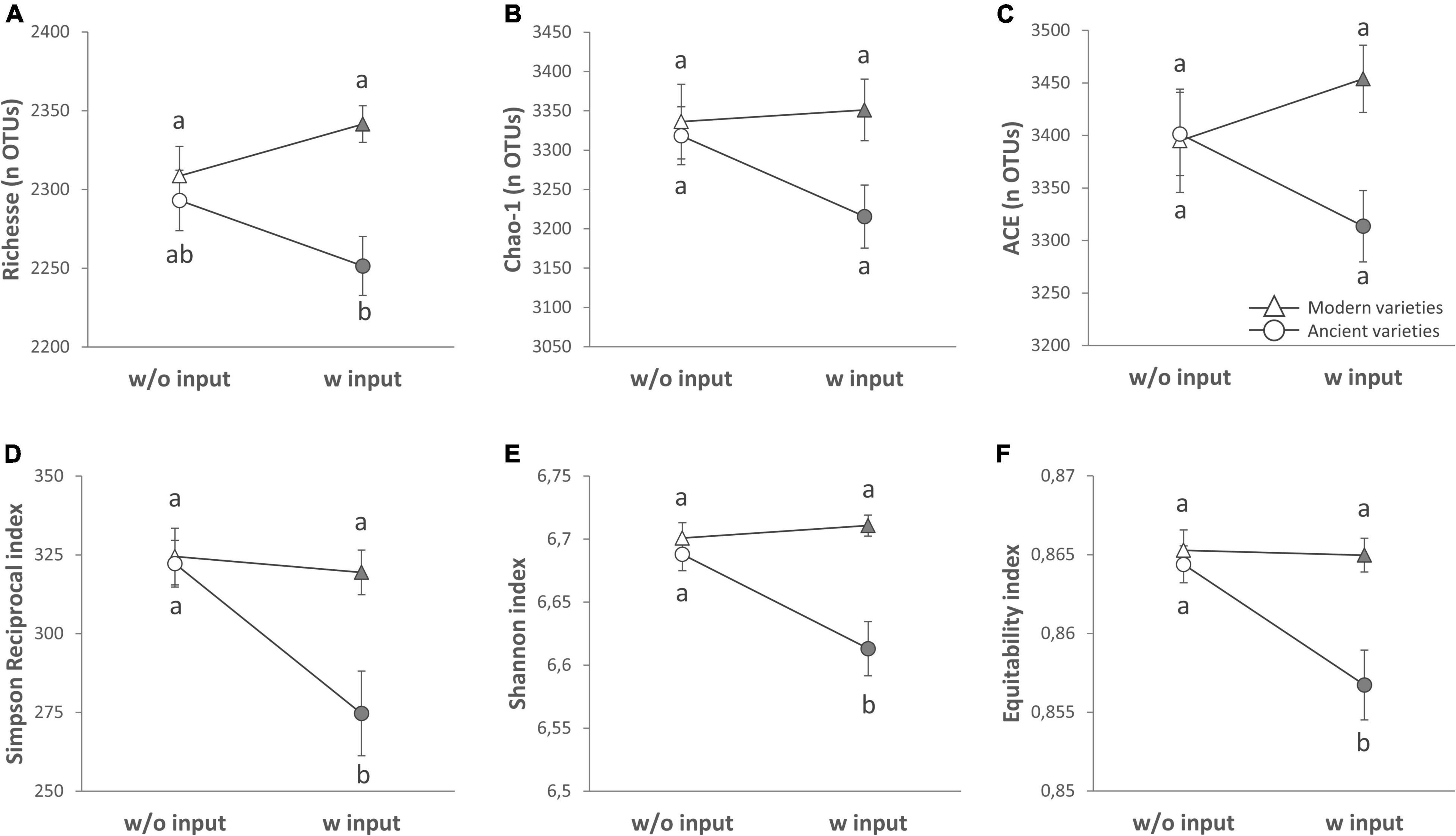
Figure 1. Alpha diversity of the rhizosphere bacterial community (16S rRNA gene amplicon sequencing) presented as reaction norms of ancient and modern wheat genotypes to environment modification by inputs. Panels are respectively showing: the (A) richness, (B) Chao-1 estimator, (C) ACE estimator, (D) Simpson Reciprocal index, (E) Shannon index, and (F) Pielou’s equitability. Data are shown as the interaction between breeding type and the presence/absence of inputs (w/o: without inputs; w: with inputs, N = 11–14 per treatment, error bars are standard error of the mean). Significance was inferred with an ANOVA under Tukey’s HSD post hoc test for normally distributed data (honest significant differences, p < 0.05). Data that were not normally distributed were analyzed by Scheirer-Ray-Hare test followed by post hoc false discovery rate test correction to account for multiple testing (FDR, p < 0.05).
At the beta diversity level (Table 2), the PERMANOVA showed a weak but significant effect on breeding type (2.6%, p = 0.036) but not inputs (1.8%, p = 0.701). Spatial heterogeneity had a significant effect as well (block: 6.5%, p < 0.001). A separation between the modern and the ancient varieties was clearly observed on the constrained axis (CAP1: 2.5%, Figure 2A). A total of 23 bacterial OTUs significantly responded to G, E, and G × E and were mainly from Bacteroidetes, Proteobacteria, Actinobacteria, and Verrucomicrobia (Supplementary Table 5). Eleven OTUs mainly from Flavobacteriaceae (Flavobacterium succinicans), Cytophagaceae, Cellulomonadaceae, Caulobacteraceae, Hyphomicrobiaceae, and Verrucomicrobiaceae had higher abundance in the ancient varieties, while only one unclassified Gemmatimonadetes OTUs was enriched in the modern varieties. Only one OTU from Cytophagaceae was altered by input addition in all the varieties. Ten OTUs from various families were altered by the G × E interaction, mostly being lowered in the modern varieties (with or without inputs) than in the ancient varieties (with or without inputs). Noteworthy is that a Corynebacteriaceae (OTU_17410, Actinobacteria) and a Haliangiaceae (OTU_41432, Proteobacteria) were decreased 11 folds by inputs in the ancient varieties.
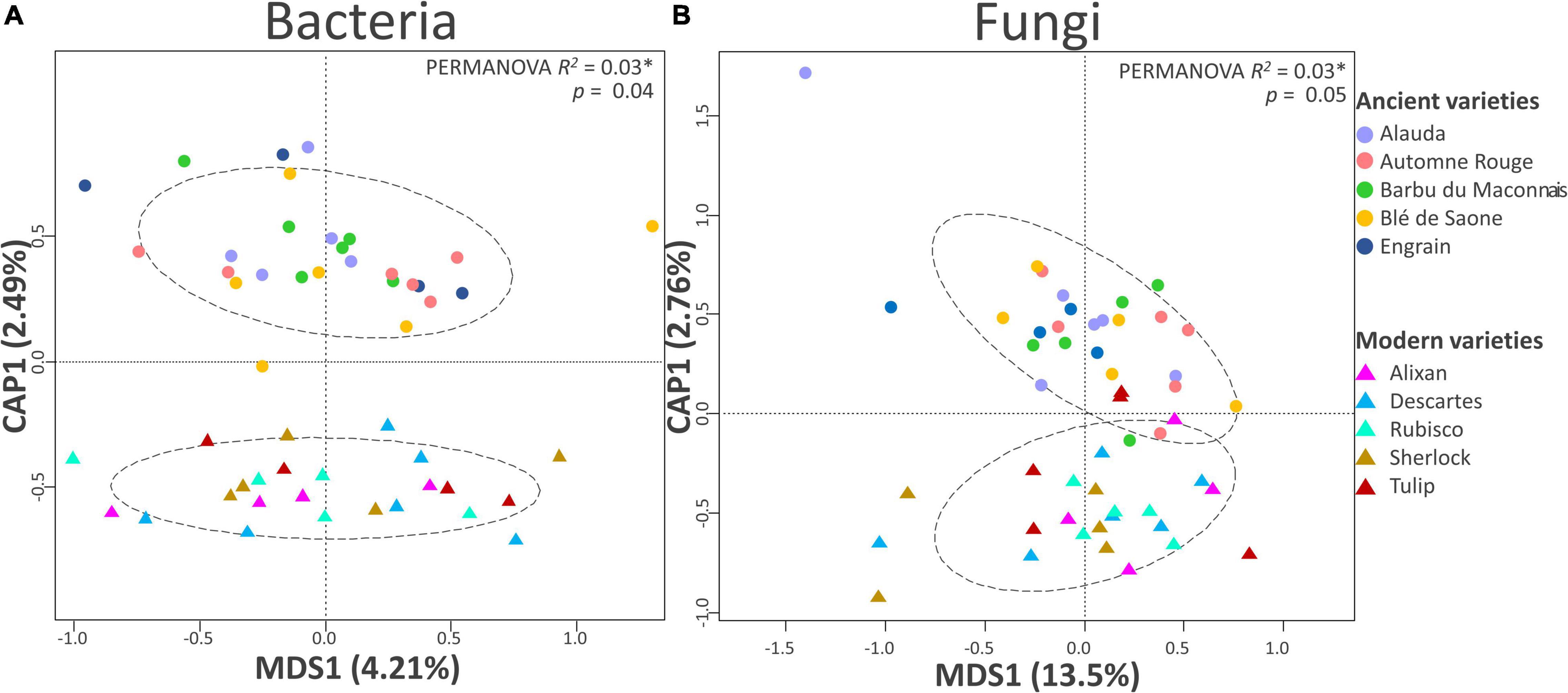
Figure 2. Beta diversity analysis of the (A) bacterial and (B) fungal communities by partial distance-based redundancy analysis (db-RDA). Partial db-RDAs were conducted to output the following constrained models: breeding type + condition (block) (10,000 permutations, N = 24 for modern varieties; N = 27 and 26 for ancient varieties in the bacteria and fungi panels, respectively).
To summarize (Supplementary Table 7), the main factor that significantly altered the bacterial component of the rhizosphere microbiota (P) was a breeding type (G), with higher diversity and different community structure in the modern varieties. Phenotypic plasticity (G × E) was the second most structuring factor of the microbiota with (i) lower richness and evenness with inputs in the ancient varieties, (ii) three OTUs altered by the interaction, and (iii) important changes in presence-absence of OTUs in each G × E modality. The effect of input (E) was marginal.
Effects on the Fungal Community
A global analysis of all the diversity indices was performed to detect the main effects by PERMANOVA (Table 2 and Supplementary Table 4), and 27.3% of the variance was explained by our model, enabling the detection of an overall significant effect of the G × E effect (10.5%, p = 0.053), whereas breeding type (0.02%, p = 0.995), inputs (0.4%, p = 0.765), and varieties (11.8%, p = 1) had no effect. No effect of spatial heterogeneity was detected (block: 4.6%, p = 0.444). In detail, the breeding type had indeed no effect on the fungal diversity indices (Figure 3, Table 4 and Supplementary Table 3). Unlike the bacterial profiles, spatial heterogeneity was detected in fungal evenness indices, including the Shannon index (6.57%, p = 0.005) and equitability index (17.99%, p = 0.007). The presence of inputs had a significant negative effect on the Shannon index (6.7%, p = 0.044), mostly driven by the ancient varieties (Figure 3). The effect of varieties on each breeding type was not significant despite a relatively high percentage of variance (up to 30.7%). For most of the indices, the G × E interaction induced a decrease in fungal diversity for the ancient varieties with inputs and, conversely, an increase in the modern varieties, as illustrated by the crossing of reaction norms (Figure 3) although supported statistically only for the Chao-1 estimator (13.9%, p = 0.025, Table 4).
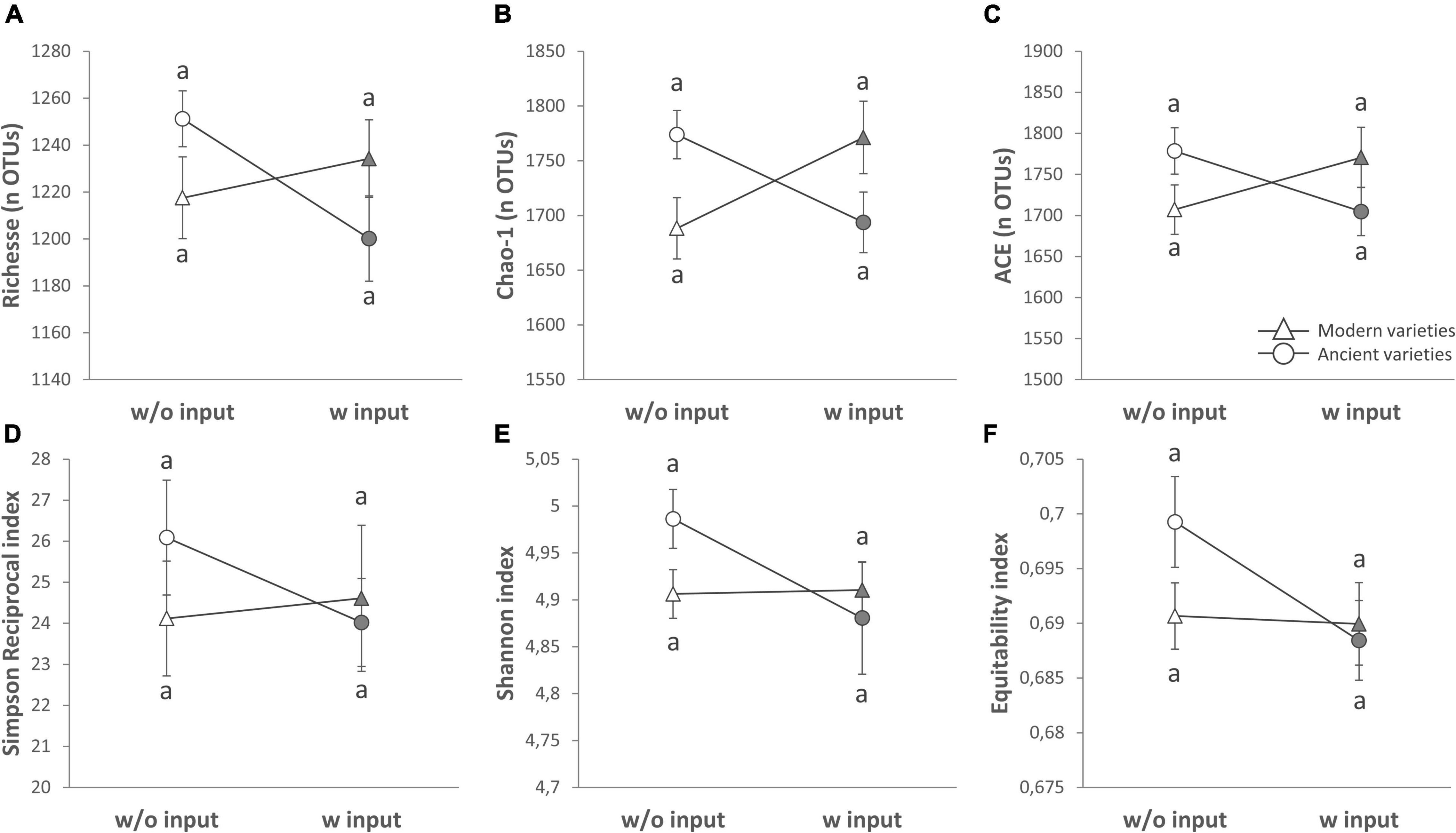
Figure 3. Alpha diversity of the rhizosphere fungal community (ITS2 gene amplicon sequencing) presented as reaction norms of ancient and modern wheat genotypes to environment modification by inputs. Panels are respectively showing: the (A) richness, (B) Chao-1 estimator, (C) ACE estimator, (D) Simpson reciprocal index, (E) Shannon index, and (F) Pielou’s equitability. Data are shown as the interaction between breeding type and the presence/absence of inputs (w/o: without inputs; w: with inputs; N = 11–14, error bars are standard error of the mean). Significance was inferred with an ANOVA under Tukey’s HSD post hoc test for normally distributed data (honest significant differences, p < 0.05). Data that are not normally distributed were analyzed by Scheirer-Ray-Hare test followed by post hoc FDR test correction to account for multiple testing (FDR, p < 0.05).
The visual distribution of taxa in Venn diagrams across G × E treatments allowed for the detection of distinct OTUs associated with breeding type and inputs and their interaction, showing a clear dominance of Ascomycota (Supplementary Figure 4). The ancient varieties had more unique taxa (54, mainly from Chaetomiaceae, Ascobolaceae, Nectriaceae, and Trichocomaceae) than the modern ones (38 mainly from Nectriaceae, Ascobolaceae, and Trebouxiaceae including a unique Mortierellasarnyensis). Without inputs, more unique taxa were detected (56 mainly from Chaetomiaceae, Lasiosphaeriaceae, Nectriaceae, and Trebouxiaceae and including unique representatives from Basidiomycota and Chytridiomycota) than with inputs (36 mainly from Helotiales, Nectriaceae, Pyronemataceae, and Trichocomaceae and including unique representatives from Glomeromycota). The G × E interaction yielded unique OTUs in each category from families Ascobolaceae, Chaetomiaceae, Trebouxiaceae, and Mortierellaceae. More specifically, in the ancient varieties, 132 unique OTUs were found without inputs (featuring families Cladosporiaceae, Pleosporaceae, Pyronemataceae, and Trichocomaceae) and 115 with inputs (featuring families Herpotrichiellaceae, Hypocreales, Nectriaceae, Ophiostomataceae, Pyronemataceae, and Tremellaceae). In the modern varieties, 130 unique OTUs were found without inputs (featuring families Cladosporiaceae, Hypocreales, Nectriaceae, Ophiostomataceae, Plectosphaerellaceae, and Pyronemataceae) and 128 with inputs (featuring families Herpotrichiellaceae, Hypocreales, Nectriaceae, Ophiocordycipitaceae, Ophiostomataceae, Plectosphaerellaceae, and Trichocomaceae). Noteworthy was the unique occurrence of Glomeromycota in ancient varieties without inputs and modern varieties with inputs. More specifically, the modern varieties with inputs have recruited six unique Glomeromycota OTUs from different phylogenetic origins: Claroideoglomus, Diversispora, Gigaspora, one unclassified Claroideoglomeraceae, and one unclassified Glomerales. In the ancient varieties without inputs, only one unique Claroideoglomus OTU (not the same as in modern with inputs) was recruited.
At the level of beta diversity (Table 2), the PERMANOVA showed a significant spatial heterogeneity (block, 5.4%, p = 0.011). Breeding type (2.5%, p = 0.052) and variety (34.7%, p = 0.02) had significant effects but not inputs (2.2%, p = 0.14). A separation between the modern and ancient varieties was observed on the constrained axis (CAP1: 2.8%, Figure 2B). Thirty-three fungal OTUs significantly responded to G, E, and G × E (Supplementary Table 5) and were mainly from Ascomycota, Basidiomycota, and Chytridiomycota. Twelve fungal OTUs were altered by breeding type, being enriched in the ancient varieties, and were mostly from families Helotiaceae, Erysiphaceae (Blumeriagraminis), Ascobolaceae, Nectriaceae (Fusarium solani), Leptosphaeriaceae, Sporormiaceae (Preussiaflanaganii), Russulaceae, and Lobulomycetaceae (Clydaeavesicula). Only one OTU (Psathyrellapanaeoloides) was enriched in the modern varieties. Six OTUs from various families were enriched by inputs (including Blumeriagraminis, Fusarium solani, and Clydaeavesicula), and three were decreased by inputs (including Psathyrellapanaeoloides). The G × E interaction altered 12 OTUs from various families, mostly between ancient varieties without inputs and modern varieties with inputs (e.g., Blumeriagraminis and Psathyrellapanaeoloides). The G × E interaction was also seen amongst ancient varieties depending on input application (Didymellanegriana and Pseudeurotiumovale favored no inputs, while Cryptococcus uniguttulatus favored inputs). Among noteworthy discriminant OTUs, an Ascobolaceae (OTU_3503, Pezizomycetes, Ascomycota) was found to be impacted by G (ancient > modern), E (without > with inputs), and G × E (ancient varieties without inputs > modern with inputs), a Psathyrellapanaeoloides (OTU_31, Basidiomycota) whose abundance was increased by 178 folds in the modern varieties with inputs compared to the ancient ones without inputs, and a Russulaceae (OTU-113, Basidiomycota) whose abundance was increased by 30 folds in the ancient varieties with inputs compared to those without. To summarize (Supplementary Table 7), breeding type (G) and phenotypic plasticity (G × E) were equally important in the fungi component of the microbiota (P). Breeding type (G) affected the community structure, with 13 OTUs enriched in the ancient varieties and only one in the modern varieties but did not alter the diversity indices. Phenotypic plasticity (G × E) had an overall important effect on alpha diversity, with diversity reduction in inputs only for the ancient varieties and an effect on the abundance of 12 OTUs between the modern varieties with inputs and the ancient ones without inputs.
Mycorrhizal Colonization
The breeding type had an impact on the intensity of mycorrhizal colonization in the root system (M, 4.3%, p = 0.005), on mycorrhizal root fragments (m, 4.5%, p = 0.009), on the arbuscular abundance in the root system (A, 4.7%, p = 0.018), and on mycorrhizal root fragments (a, 3.82%, p = 0.002), all being in favor of higher values in the modern varieties than in the ancient ones (Figure 4, Table 5 and Supplementary Table 6). Inputs had an impact only on M (2.6%, p = 0.027) and m (2.6%, p = 0.046), being higher without inputs. The G × E interaction had no effect. Varieties in each breeding type had an effect on m (22.46%, p = 0.01), M (23.63%, p = 9.6E-4), and a (19.77%, p = 0.03). Spatial heterogeneity slightly affected M (block: 4.13%, p = 0.02). The frequency of mycorrhization “F” was not affected. The PERMANOVA on all the indices revealed a significant effect of breeding type in favor of higher values in the modern varieties (2.8%, p = 0.008, Table 2 and Supplementary Table 4). The effect of varieties nested in each breeding type was also significant (18.8%, p = 0.007), as well as spatial heterogeneity (block: 2.8%, p = 0.046). To summarize (Supplementary Table 7), the mycorrhizal indices were primarily altered by breeding type (G), as mycorrhization was stronger in the modern varieties. The addition of inputs (E) decreased the intensity of mycorrhizal colonization in the root system and root fragments. The G × E interaction had no effects.
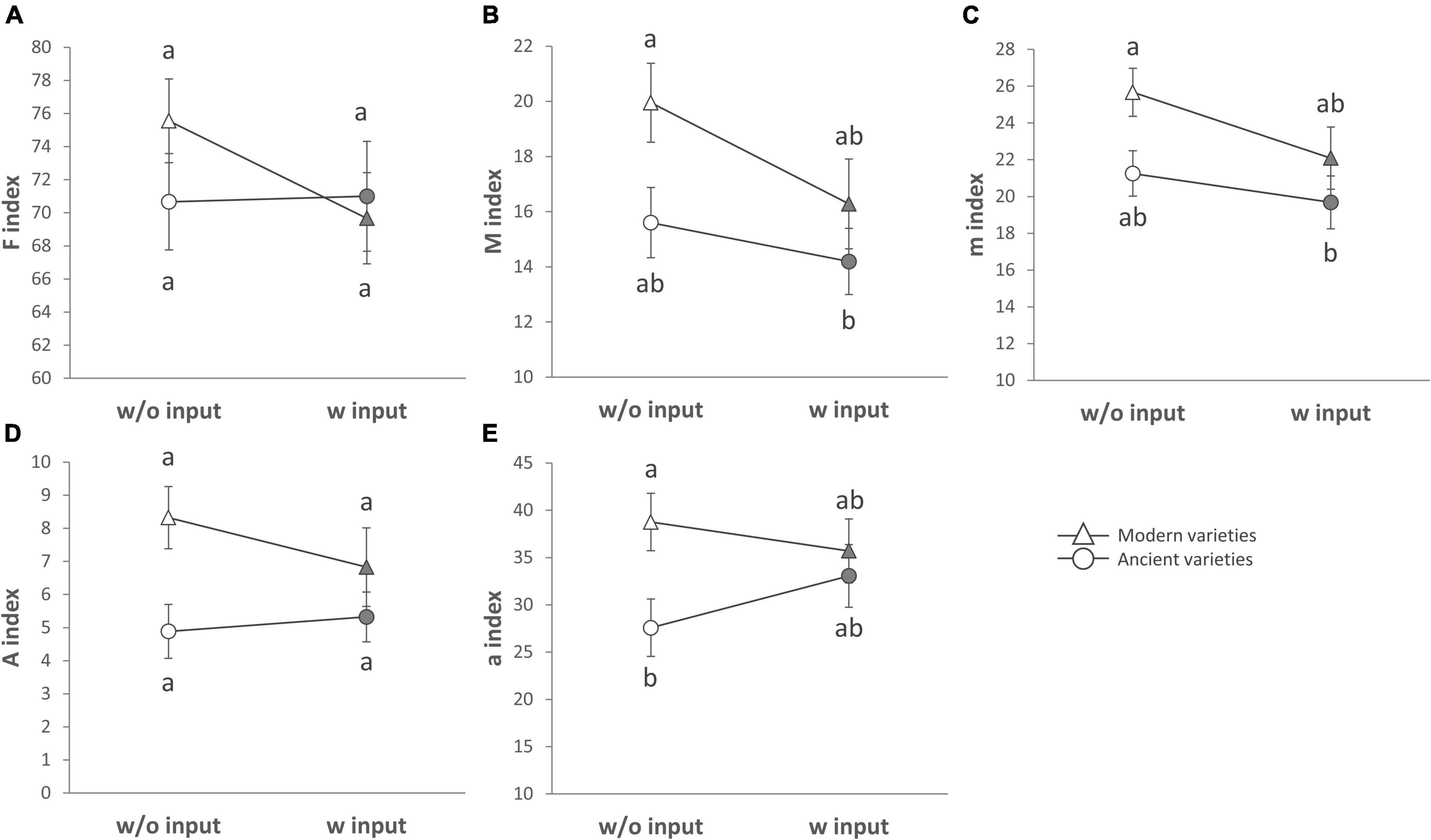
Figure 4. Mycorrhizal colonization for breeding types and inputs that are presented as reaction norms. Panels are respectively showing (A) the frequency of root fragments with mycorrhizal structures on the root system scale, intensity of mycorrhizal colonization on the root (B) system scale, or (C) restricted to mycorrhizal root fragments (D) arbuscule abundance on the root system scale or (E) restricted to mycorrhizal root fragments for each type of breeding (modern varieties with triangles, ancient varieties with circles) in response to the presence of inputs. Significance was inferred by ANOVA under Tukey’s HSD post hoc test for normally distributed data (honest significant differences, p < 0.05). Data that were not normally distributed were analyzed by Scheirer-Ray-Hare test followed by post hoc FDR test correction to account for multiple testing (FDR, p < 0.05).
Network Analysis
Poisson Log-Normal (PLN) models reached stable solutions (refer to the two separated networks in Supplementary Figure 5), resulting in two networks with comparable sizes in terms of nodes (modern network: 141 nodes, sparsifying penalty = 0.22, R2 = 0.993; ancient network: 158 nodes, sparsifying penalty = 0.21, R2 = 0.993). To better visualize the specific and common parts between the two networks, we merged them and clustered nodes based on their membership (either unique or common, Figure 5). The intersection revealed 81 OTUs in common, while 77 and 60 were, respectively, specific to the network of either ancient or modern varieties. Similar phylogenetic compositions were found for both networks, with a dominance of Ascomycota, Proteobacteria, Actinobacteria, and Bacteroidetes OTUs. Eight keystone species gathering 40% of edges were identified (Supplementary Figure 5). The network of the ancient varieties had significantly higher complexity than that of the modern ones for all the tested indices. In the network of the ancient varieties, three keystone OTUs centralized most edges, including an Ascobolaceae (OTU_3503, Pezizomycetes, Ascomycota, degree = 34), an unclassified fungus (OTU_123, Ascomycota, degree = 26), and a Cellvibrio (OTU_886, Gammaproteobacteria, degree = 20). In the network of the modern varieties, one keystone OTU centralized most edges, Lectera longa (OTU_37, Sordariomycetes, degree = 54). Among common OTUs, four keystones centralized most edges: an unclassified Sordariomycetes (OTU_71, Ascomycota, degree = 38), a Janthinobacteria (OTU_1532, Oxalobacteraceae, Betaproteobacteria, degree = 18), a Mortierella (OTU_112, Mortierellomycota, degree = 13), a Pedobacter (Sphingobacteriaceae, Bacteroidetes, degree = 11). Six OTUs in this network were previously detected as being “discriminant taxa” (refer to “Network” in Supplementary Table 5). Among them, Ascobolaceae (OTU_3503, Pezizomycetes, Ascomycota, degree = 34) was an important discriminant OTU being affected by G, E, and G × E (Supplementary Table 5). To summarize, each network had its own set of OTUs. The ancient network was more complex, including more keystone species than in the modern network. Among keystone OTUs in the ancient network, the OTU_3503 Ascobolaceae was the most centralized one, being sensitive to G, E, and G × E.
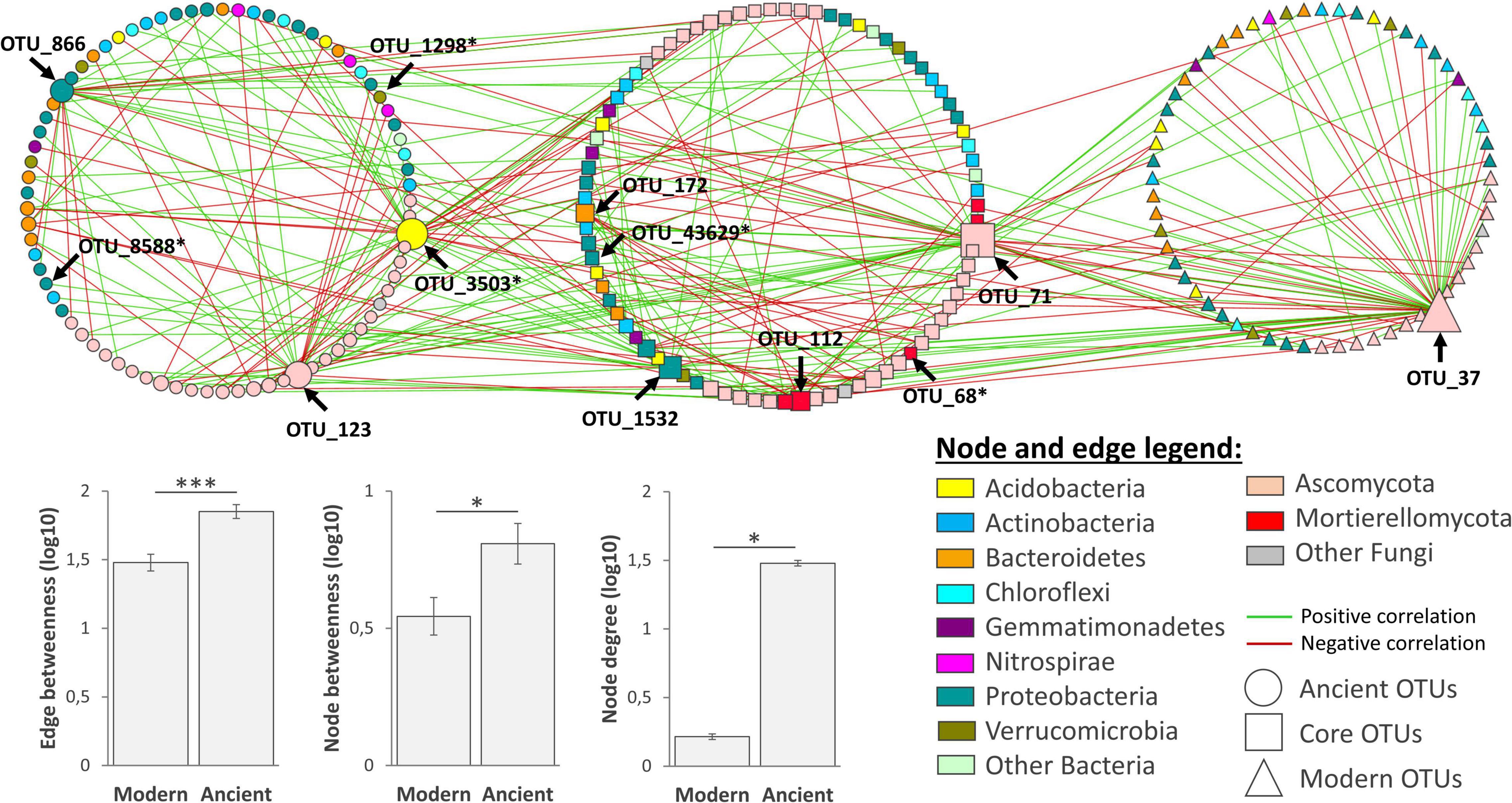
Figure 5. Combined network analysis of rhizosphere microbiota associated to the modern (N = 26) and ancient (N = 24) wheat varieties. The modern and ancient networks were constructed using dominant OTUs based on partial correlations obtained with Poisson Log Normal models combining the bacterial and fungal datasets together (∼1 + block + offset). For visualization, both networks were merged into one and clustered in three to feature: (i) unique OTUs of the ancient network on the left, (ii) common OTUs in the middle, and (iii) modern OTUs on the right. OTUs of interest (keystone or discriminant OTUs from Supplementary Table 5) are indicated with an arrow. Bar charts represent average network complexity indices estimated from edges or nodes (Kruskal–Wallis test, *p < 0.05; ***p < 0.001).
A summary figure was established based on the main results obtained on the bacterial and fungal communities and mycorrizha colonization (Figure 6).
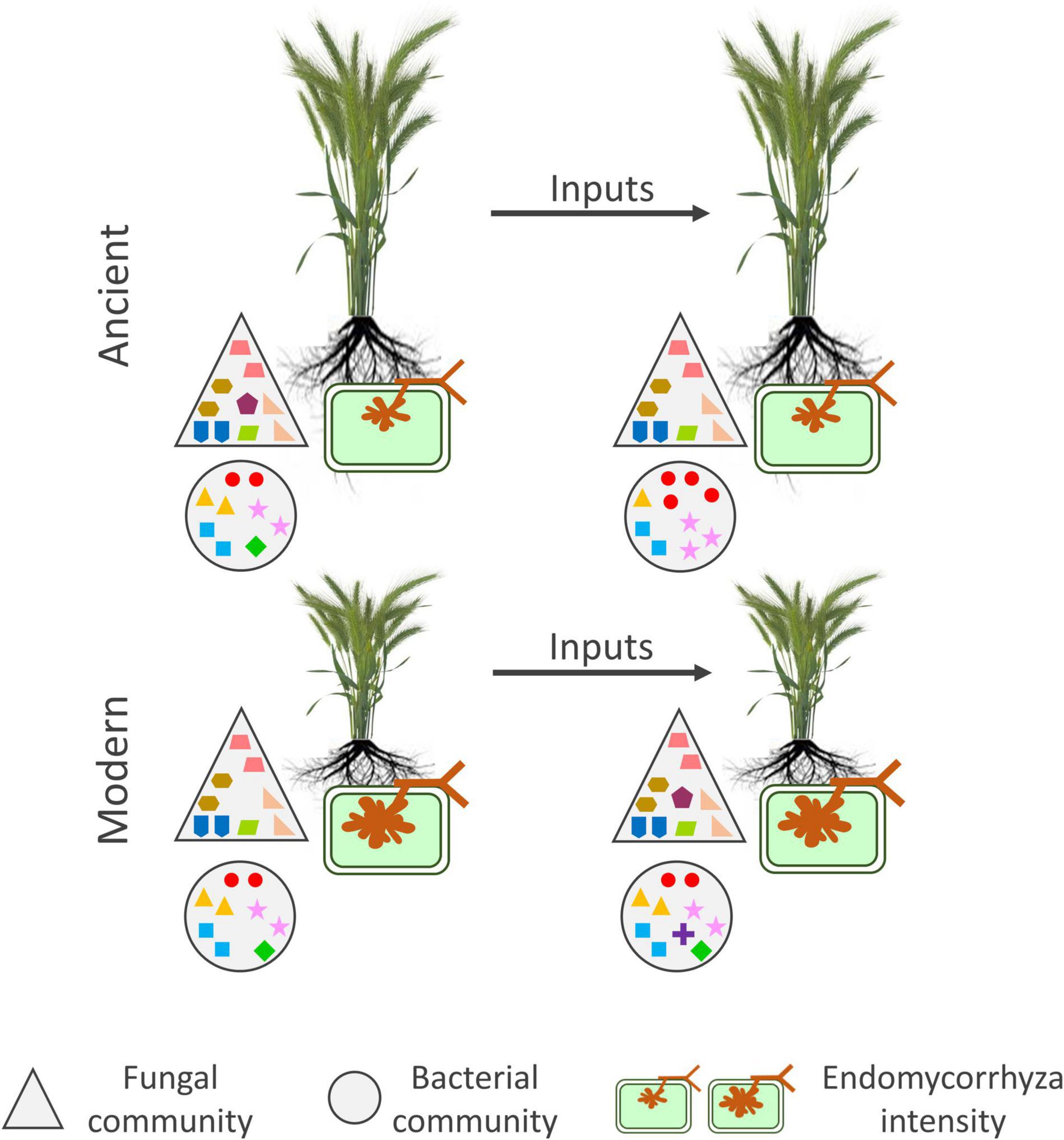
Figure 6. Summary picture of the main effects detected by PERMANOVA on the alpha-diversity indices and mycorrhizal colonization parameters. The figure depicts ancient (tall plants) and modern (short plants) rhizosphere microbiota with and without inputs. The big triangles and circles depict the fungal and bacterial communities, respectively. The mycorrhiza is depicted as a green cell with an arbuscular structure inside. The symbols inside the big triangles and circles represent OTUs. PERMANOVA results are shown in Table 2. Only results from G, E, and G x E are shown. For the fungal community, input addition led to slight reduction in estimated richness for the ancient varieties and slight increase for the modern varieties. For the bacterial community, inputs reduced the richness and evenness for the ancient varieties but led to slight increase in richness for the modern varieties. For the mycorrhiza, the modern varieties had more pronounced colonization than the ancient varieties.
Discussion
Modern wheat varieties were selected and are generally grown with inputs, whereas ancient varieties were selected before 1960, at a time where inputs were not used in a systematic way, and are generally grown today in organic farming without synthetic inputs. This link between genotype (G) and agricultural practices modifying the environment (E) prevents the assessment of the relative importance of individual factors (E and G) and their interaction (G × E) on plant-microbiota relationships. Our results are a first attempt to quantify the importance of these effects in the field. As such, spatial variability was important to consider by setting up three repeated blocks (Supplementary Figure 2) included in statistical models. This spatial variability was expected and had a relatively low effect on our recorded parameters (0.1∼18%). In addition, it was important to assess the variability inside each breeding type by considering variety-specific variations across the modern and ancient genotypes (by multi-stratum variance analysis). Indeed, the variety effect nested inside each breeding type (see model, Supplementary Figure 1) was sometimes important (1.1–49.9%). However, both spatial and variety effects did not prevent the detection of significant effects associated with our factors of interest.
Effects of Breeding on Rhizosphere Microbiota
Breeding type (G) was the most structuring factor explaining the outcome of rhizosphere microbiota (Supplementary Table 7). The recent selection effort from ancient to modern varieties had a more pronounced effect on bacteria than on fungal alpha diversity, with modern varieties having more diversity than ancient ones. All the mycorrhiza indices (except F) were higher with the modern varieties than with the ancient ones. Therefore, our results invalidated the first hypothesis that modern varieties are associated with less diverse communities than ancient ones. This result is in line with the reduced selectivity of modern varieties regarding rhizosphere microbial recruitment as compared to ancient ones (Kiers et al., 2007). Besides, in terms of community structures (beta diversity), breeding had a small effect on both bacterial and fungal communities (2.5–2.6%). Different but not opposite results were reported in the literature about the recent selection effort on rhizosphere microbial community structure: (i) no clear difference in bacterial community structure between ancient (three varieties) and modern (one variety) breeding types (Cangioli et al., 2022); (ii) stronger effect on bacterial community structure between modern (five varieties) and ancient (three varieties) breeding types (Kavamura et al., 2020). However, small effects like ours were already observed with domestication (from wild relatives to cultivated varieties) (Özkurt et al., 2020; Spor et al., 2020) likely associated with a “relaxed” selective force (Hassani et al., 2020). In addition, a very strong effect of breeding was noticed on the structure of the rhizosphere microbiota network, featuring common but also specific OTUs of modern or ancient varieties. For the modern varieties, the organization was less complex than for the ancient ones. The fact that fewer keystone nodes were detected in the modern network, with clear centralization around Lectera longa, could be interpreted as a potential weakness in terms of stability if that one node was to be lost. Noteworthy, while the ancient network had several keystone OTUs, the modern network only had one keystone Lectera OTU. This genus contains crop pathogens (Cannon et al., 2012; Ares et al., 2021) and their abundance was shown to be an important predictor of wheat yield (Yergeau et al., 2020). As for the literature and our study, there is no consensus on the impact of plant breeding on rhizosphere microbiota diversity. A decrease in the diversity of symbiotic rhizobia associations was reported in domesticated legumes (Kim et al., 2014; Sangabriel-Conde et al., 2015). Mutch and Young (2004) reported limited interactions with symbionts in domesticated pea and broad bean compared with wild relatives in non-agricultural soil without inputs. Some studies found more nuanced results: Leff et al. (2017) reported domestication effects on the fungal rhizosphere community of sunflowers but not bacteria. Brisson et al. (2019) reported no effect on diversity but differences in co-occurrence networks among inbred maize lines, modern hybrids, and teosinte. Finally, Kinnunen-Grubb et al. (2020) reported faster bacterial colonization in the rhizosphere of modern wheat cultivars than in that of ancestors, potentially due to the relaxed ability of modern varieties to select beneficial microbial partners (Kiers and Denison, 2008) via the notable changes in root exudate complexity (Pérez-Jaramillo et al., 2017). Our results concur with this interpretation, as the ancient varieties were enriched with specific bacterial and fungal taxa together with a more complex network structure featuring more keystone nodes, unlike the modern ones that had higher diversity but lesser keystones, indicating that their selective force was potentially relaxed. Similarly, Kiers et al. (2007) showed that ancient soybean varieties were more performant in maintaining high seed production when infected with a mixture of effective and ineffective rhizobium strains, unlike modern ones. However, a meta-analysis on the effect of breeding on mycorrhizal association found that modern varieties (> 1950) were more responsive in terms of increased biomass than old varieties (1900–1950) and ancestors (< 1900) (Lehmann et al., 2012). Thus, the fact that modern varieties establish either adaptive or non-adaptive interactions with soil microbiota is still an open question (refer to Ghalambor et al., 2007).
Effects of the Environment on Rhizosphere Microbiota
The presence/absence of inputs (E) had a marginal impact compared to the other effects (Supplementary Table 7) likely due to the narrow range of environmental conditions covered in our study (fixed pedo-climatic conditions, with or without inputs). The abundance of several OTUs was altered by inputs (up to 55.7 fold increase with inputs compared to no inputs, and only up to 4.6 fold increase without inputs compared to inputs). This weak effect might be explained by several reasons, including the preceding crop, soil fertility, application frequencies, and doses. The preceding crop was a legume (Vicia faba), which may have left sufficient organic nitrogen in the soil to hide our mineral fertilization effect. Regarding soil fertility, a study reports shifts in bacterial community composition only in soils with low C and N concentrations (Ramirez et al., 2010, 2012). Since the C and N concentrations in our soil presented a medium value (26.7 g.kg–1 organic carbon and 2.11 g.kg–1 total nitrogen), nitrogen was likely not a limiting factor. Likewise, the effects of inputs on rhizosphere microbiota become noticeable when the treatments were repeated several times (Yang et al., 2012; Schlatter et al., 2017) or when the doses were increased (Kavamura et al., 2018; Chen et al., 2019). Since our purpose was to match local agricultural practices in terms of products and doses, this might explain the observed weak effects. When noted, the effect of inputs was negative on microbial communities in terms of bacterial and fungal evenness, as well as mycorrhizal colonization intensity (likely without functional consequence since arbuscular development was maintained). The results are in line with the literature (Dunn et al., 2021) and support our second hypothesis that the presence of inputs decreases, although slightly, the microbial diversity in the rhizosphere. This decrease in diversity was expected for several reasons. First, the addition of fertilizers can change bacterial communities. Grunert et al. (2019) reported that the addition of an inorganic fertilizer reduced the diversity of tomato rhizosphere microbiota. Second, the effect of fungicide could have suppressed some fungal taxa. Although supposed to target specific fungal pathogens, fungicides may impact the entire rhizosphere fungal community (Esmaeili Taheri et al., 2015). Furthermore, some fungi could benefit from the decreased abundance of dominant species, thus increasing community evenness. Third, we speculated that herbicide application could have reduced microbial diversity by impacting microorganisms associated with weed plant species.
Effects of Phenotypic Plasticity on Rhizosphere Microbiota
We advocate that the lack of consensus in the literature regarding the effect of plant domestication and recent breeding efforts on plant-microbiota association might partly stem from the interaction between genotype and the environment. Indeed, differences in microbiota diversity between modern and ancient varieties can depend on input application. Phenotypic plasticity, a ubiquitous aspect of organisms, describes the ability of a genotype to produce different phenotypes in response to environmental variations (Gause, 1947; Bradshaw, 1965). Variations in the morphology, immunity, or exudation of a given genotype influence the rhizosphere, seen here as an “extended phenotype” (de la Fuente Cantó et al., 2020) or the “microbiota as a phenotype” (Oyserman et al., 2021). However, changes in the environment such as input applications could influence soil microbiota and lead to the recruitment of different rhizosphere microbiota. Indeed, we found that phenotypic plasticity, the G × E interaction, had an important impact on plant-microbiota relationships. It was, after breeding type, the second most structuring force (Supplementary Table 7) and could have potentially been higher provided that a wider range of environmental conditions was investigated. For bacterial alpha diversity, the effect of phenotypic plasticity (5.7%) was in the same range as the breeding type (7.4%), while for fungi, the breeding type had no effect, whereas phenotypic plasticity was significant and relatively important (10.5%). The ancient varieties had a decreased bacterial and fungal diversity due to inputs, whereas the modern varieties were not affected. Furthermore, the presence of core fractions uniquely detected in each G × E modalities reinforces the idea that a real interaction occurred. This was particularly true for Glomeromycota, as previously reported by Lehmann et al. (2012), whose occurrence in our core fractions of breeding types depended on inputs. Furthermore, the discriminant analysis revealed that phenotypic plasticity was altering the same number of OTUs than breeding type, especially between modern varieties with inputs compared to ancient varieties without inputs. This was obvious with OTU_3503, a keystone of the ancient network, whose abundance in the rhizosphere was further decreased by the interaction of breeding and inputs. Thus, the G × E interaction was important in understanding the variations of microbial diversity, validating our third hypothesis that the effects of the recent selection efforts on microbiota diversity depend on the presence of inputs. However, phenotypic plasticity had no impact on mycorrhizal colonization or on the beta diversity of bacterial and fungal communities. Cases of interaction between genotype and environment were reported for other plant species and traits (e.g., maize yield variations across North America: G = 7%, E = 43%, and G × E = 6%; Gage et al., 2017). Regarding plant-microbiota interactions, G × E was shown to affect the phyllosphere, and to a lesser extent, the rhizosphere microbiota of Boechera stricta (Brassicaceae), with stronger effects on evenness than the genotype itself (Wagner et al., 2016). Although not referring to phenotypic plasticity, a study on domestication reported that the fungal community of a wild rice relative was more affected by a fungicide than a cultivated one (Shi et al., 2019), which is consistent with our results. This lower sensitivity of microbiota to the effect of inputs with modern varieties could be explained either by an ecological or a genetic mechanism. In the light of our results and those from Shi et al. (2019), the fungicide treatment might explain that phenotypic plasticity is detrimental to fungi in the ancient varieties but not in the modern varieties perhaps because of the more easily degradable exudates released in the rhizosphere of the modern varieties (Pérez-Jaramillo et al., 2016, 2018). However, since the bacterial community was also affected by phenotypic plasticity, we cannot exclude that plasticity was due to genetic difference: our discriminant OTU analysis suggests that the modern varieties could have lost their ability to regulate rhizosphere microbiota according to soil fertility, which changed with the addition of fertilizer. The lack of regulation in modern varieties is coherent with the reported relaxed selective force they exert on their rhizosphere microbiota (Kiers et al., 2007; Hassani et al., 2020). In that regard, our sequencing results revealed that G × E was responsible for the differential recruitment of unique OTUs among the Glomeromycora phylum, which was more pronounced in the modern varieties with inputs. While likely without functional consequences based on our microscopic mycorrhizal observations, this might indicate that the relaxed selection of the modern varieties is accentuated with inputs. Since they were selected with inputs, the modern varieties might have experienced a lower range of variation in soil fertility than the ancient ones. This modified regulation in plant-microbiota interaction is consistent with results from Gage et al. (2017), showing that variation in phenotypic plasticity is disproportionately controlled by regulatory genes compared to other traits. To prevent the environmental impacts of agriculture, incentives to reduce chemical inputs are increasing. From that perspective, the choice of varieties that promote intrinsic microbial community properties such as richness and evenness is an important lever (Bakker et al., 2012). As reports on the importance of the interaction between genotype and environment on plant microbiota increase, it is high time to put emphasis on plant and microbe genes involved in phenotypic plasticity, for instance through breeding programs. This could allow for the selection of varieties without a priori on agricultural practices they will be exposed to, especially regarding synthetic inputs. This perspective is realistic since genes responsible for yield (i.e., mean trait value) and phenotypic plasticity (i.e., variance) are distinct (Gage et al., 2017), theoretically enabling their concomitant selection by breeders to spawn plastic varieties with enhanced reaction capacity toward a changing environment while maintaining decent yields (Kusmec et al., 2017).
Data Availability Statement
The raw sequence data used in this study has been deposited to the SRA (Sequence Read Archive), https://www.ncbi.nlm.nih.gov/sra, with the following accession numbers: Bacteria: PRJNA701534, Fungi: PRJNA702900. Any further queries should be directed to the corresponding author.
Author Contributions
MB conceived the research. EP, CD, and MB carried out the experiment. DW, LC, and TR did the mycorrhiza observations. SJ dealt with the bioinformatics and statistical analyses and edited the figures and tables. MB wrote the article, with significant contribution of SJ. All authors contributed to the article and approved the submitted version.
Funding
SJ was funded by the University of Bourgogne Franche-Comté via an ISITE-BFC International Junior Fellowship award (AAP3:RA19028.AEC.IS). The salary of TR was funded by the Bourgogne Franche-Comté region via the FABER program (grant no. 2017-9201AAO049S01302).
Conflict of Interest
The authors declare that the research was conducted in the absence of any commercial or financial relationships that could be construed as a potential conflict of interest.
Publisher’s Note
All claims expressed in this article are solely those of the authors and do not necessarily represent those of their affiliated organizations, or those of the publisher, the editors and the reviewers. Any product that may be evaluated in this article, or claim that may be made by its manufacturer, is not guaranteed or endorsed by the publisher.
Acknowledgments
We thank Institut Agro Dijon for the experiment facilities. We are grateful to Jean-Philippe Guillemin, Wilfried Queyrel, and Etienne Gaujour for their advice on wheat culture and the management of the experimental site and Jacques Le Gouis and François Balfourier for providing information on the origins of the ancient varieties. We thank Tariq Shah for his contribution to the bibliographical search. We also thank Graines de Noé and, especially, Hélène Montaz for providing seeds of the ancient varieties, as well as RAGT Semences, Secobra, Saaten Union, and Limagrain for providing the modern varieties.
Supplementary Material
The Supplementary Material for this article can be found online at: https://www.frontiersin.org/articles/10.3389/fevo.2022.903008/full#supplementary-material
Footnotes
References
Ai, C., Liang, G., Sun, J., Wang, X., He, P., Zhou, W., et al. (2015). Reduced dependence of rhizosphere microbiome on plant-derived carbon in 32-year long-term inorganic and organic fertilized soils. Soil Biol. Biochem. 80, 70–78. doi: 10.1016/j.soilbio.2014.09.028
An, G. H., Kobayashi, S., Enoki, H., Sonobe, K., Muraki, M., Karasawa, T., et al. (2010). How does arbuscular mycorrhizal colonization vary with host genotype? An example based on maize (Zea mays) germplasms. Plant Soil 327, 441–453. doi: 10.1007/s11104-009-0073-3
Ares, A., Costa, J., Joaquim, C., Pintado, D., Santos, D., Monika, M., et al. (2021). Effect of Low-Input Organic and Conventional Farming Systems on Maize Rhizosphere in Two Portuguese Open-Pollinated Varieties (OPV), “Pigarro” (Improved Landrace) and “SinPre” (a Composite Cross Population). Front. Microbiol. 12:636009. doi: 10.3389/fmicb.2021.636009
Baker, G. C., Smith, J. J., and Cowan, D. A. (2003). Review and re-analysis of domain-specific 16S primers. J. Microbiol. Methods 55, 541–555. doi: 10.1016/j.mimet.2003.08.009
Bakker, M. G., Manter, D. K., Sheflin, A. M., Weir, T. L., and Vivanco, J. M. (2012). Harnessing the rhizosphere microbiome through plant breeding and agricultural management. Plant Soil 360, 1–13. doi: 10.1007/s11104-012-1361-x
Berendsen, R. L., Pieterse, C. M. J., and Bakker, P. (2012). The rhizosphere microbiome and plant health. Trends Plant Sci. 17, 478–486. doi: 10.1016/j.tplants.2012.04.001
Berry, D., and Widder, S. (2014). Deciphering microbial interactions and detecting keystone species with co-occurrence networks. Front. Microbiol. 5:219. doi: 10.3389/fmicb.2014.00219
Bradshaw, A. D. (1965). Evolutionary significance of phenotypic plasticity in plants. Adv. Genet. 13, 115–155. doi: 10.1016/S0065-2660(08)60048-6
Brisson, V. L., Schmidt, J. E., Northen, T. R., Vogel, J. P., and Gaudin, A. C. (2019). Impacts of maize domestication and breeding on rhizosphere microbial community recruitment from a nutrient depleted agricultural soil. Sci. Rep. 9, 1–14. doi: 10.1038/s41598-019-52148-y
Bulgarelli, D., Garrido-Oter, R., Münch, P. C., Weiman, A., Dröge, J., Pan, Y., et al. (2015). Structure and function of the bacterial root microbiota in wild and domesticated barley. Cell Host Microbe 17, 392–403. doi: 10.1016/j.chom.2015.01.011
Cangioli, L., Mancini, M., Napoli, M., Fagorzi, C., Orlandini, S., Vaccaro, F., et al. (2022). Differential Response of Wheat Rhizosphere Bacterial Community to Plant Variety and Fertilization. Int. J. Mol. Sci. 23:3616. doi: 10.3390/ijms23073616
Cannon, P. F., Buddie, A. G., Bridge, P. D., Neergaard, E. D., Lübeck, M., and Askar, M. M. (2012). Lectera, a new genus of the Plectosphaerellaceae for the legume pathogen Volutella colletotrichoides. MycoKeys 3:28. doi: 10.3897/mycokeys.3.3065
Chaluvadi, S., and Bennetzen, J. L. (2018). Species-associated differences in the below-ground microbiomes of wild and domesticated Setaria. Front. Plant Sci. 9:1183. doi: 10.3389/fpls.2018.01183
Chen, S., Waghmode, T. R., Sun, R., Kuramae, E. E., Hu, C., and Liu, B. (2019). Root-associated microbiomes of wheat under the combined effect of plant development and nitrogen fertilization. Microbiome 7:136. doi: 10.1186/s40168-019-0750-2
Chiquet, J., Robin, S., and Mariadassou, M. (2019). “Variational inference for sparse network reconstruction from count data,” in International Conference on Machine Learning, eds K. Chaudhuri and R. Salakhutdinov (Long Beach, CA: PMLR), 1162–1171.
Chowdhury, S. P., Babin, D., Sandmann, M., Jacquiod, S., Sommermann, L., Sørensen, S. J., et al. (2019). Effect of long-term organic and mineral fertilization strategies on rhizosphere microbiota assemblage and performance of lettuce. Environ. Microbiol. 21, 1462–2920. doi: 10.1111/1462-2920.14631
Csardi, G., and Nepusz, T. (2006). The igraph software package for complex network research. InterJ. Complex Syst. 1695, 1–9.
Danchin, É, Charmantier, A., Champagne, F., Mesoudi, A., Pujol, B., and Blanchet, S. (2011). Beyond DNA: integrating inclusive inheritance into an extended theory of evolution. Nat. Rev. Genet. 12, 475–486. doi: 10.1038/nrg3028
de la Fuente Cantó, C., Simonin, M., King, E., Moulin, L., Bennett, M. J., Castrillo, G., et al. (2020). An extended root phenotype: the rhizosphere, its formation and impacts on plant fitness. Plant J. 103, 951–964. doi: 10.1111/tpj.14781
de Mendiburu, F., and Yaseen, M. (2020). agricolae: Statistical Procedures for Agricultural Research”. R package version 1.4.0. Available Online at: https://myaseen208.com/agricolae/
Deng, S., Caddell, D. F., and Coleman-Derr, D. (2021). Genome wide association study reveals plant loci controlling heritability of the rhizosphere microbiome. ISME J. 15, 3181–3194. doi: 10.1038/s41396-021-00993-z
Dixon, P. (2003). VEGAN, a package of R functions for community ecology. J. Veg. Sci. 14, 927–930. doi: 10.1111/j.1654-1103.2003.tb02228.x
Dunn, L., Lang, C., Marilleau, N., Terrat, S., Biju-Duval, L., Lelièvre, M., et al. (2021). Soil microbial communities in the face of changing farming practices: a case study in an agricultural landscape in France. PLoS One 16:e0252216. doi: 10.1371/journal.pone.0252216
Egerton-Warburton, L. M., Johnson, N. C., and Allen, E. B. (2007). Mycorrhizal community dynamics following nitrogen fertilization: a cross-site test in five grasslands. Ecol. Monogr. 77, 527–544. doi: 10.1890/06-1772.1
Esmaeili Taheri, A., Hamel, C., and Gan, Y. (2015). Pyrosequencing reveals the impact of foliar fungicide application to chickpea on root fungal communities of durum wheat in subsequent year. Fungal Ecol. 15, 73–81. doi: 10.1016/j.funeco.2015.03.005
Estensmo, E. L. F., Sundy, M., Morgado, L., Martin-Sanchez, P. M., Skrede, I., and Kauserud, H. (2021). The influence of intraspecific sequence variation during DNA metabarcoding: a case study of eleven fungal species. Mol. Ecol. Resour. 21, 1141–1148. doi: 10.1111/1755-0998.13329
Gage, J. L., Jarquin, D., Romay, C., Lorenz, A., Buckler, E. S., Kaeppler, S., et al. (2017). The effect of artificial selection on phenotypic plasticity in maize. Nat. Commun. 8:1348. doi: 10.1038/s41467-017-01450-2
Geisseler, D., and Scow, K. M. (2014). Long-term effects of mineral fertilizers on soil microorganisms - A review. Soil Biol. Biochem. 75, 54–63. doi: 10.1016/j.soilbio.2014.03.023
Ghalambor, C. K., McKay, J. K., Carroll, S. P., and Reznick, D. N. (2007). Adaptive versus non-adaptive phenotypic plasticity and the potential for contemporary adaptation in new environments. Funct. Ecol. 21, 394–407. doi: 10.1111/j.1365-2435.2007.01283.x
Grunert, O., Robles-Aguilar, A. A., Hernandez-Sanabria, E., Schrey, S. D., Reheul, D., Van Labeke, M. C., et al. (2019). Tomato plants rather than fertilizers drive microbial community structure in horticultural growing media. Sci. Rep. 9, 1–15. doi: 10.1038/s41598-019-45290-0
Hassani, M. A., Özkurt, E., Franzenburg, S., and Stukenbrock, E. H. (2020). Ecological Assembly Processes of the Bacterial and Fungal Microbiota of Wild and Domesticated Wheat Species. Phytobiomes J. 4, 217–224. doi: 10.1094/PBIOMES-01-20-0001-SC
Herlemann, D. P., Labrenz, M., Jurgens, K., Bertilsson, S., Waniek, J. J., and Andersson, A. F. (2011). Transitions in bacterial communities along the 2000 km salinity gradient of the Baltic Sea. ISME J. 5, 1571–1579. doi: 10.1038/ismej.2011.41
Hetrick, B. A. D., Wilson, G. W. T., and Cox, T. S. (1992). Mycorrhizal dependence of modern wheat varieties, landraces, and ancestors. Can. J. Bot. 70, 2032–2040. doi: 10.1139/b92-253
Hu, L., Robert, C. A. M., Cadot, S., Zhang, X., Ye, M., Li, B., et al. (2018). Root exudate metabolites drive plant-soil feedbacks on growth and defense by shaping the rhizosphere microbiota. Nat. Commun. 9:2738. doi: 10.1038/s41467-018-05122-7
Ihrmark, K., Bödeker, I. T. M., Cruz-Martinez, K., Friberg, H., Kubartova, A., Schenck, J., et al. (2012). New primers to amplify the fungal ITS2 region – evaluation by 454-sequencing of artificial and natural communities. FEMS Microbiol. Ecol. 82, 666–677. doi: 10.1111/j.1574-6941.2012.01437.x
Jacquiod, S., Puga-Freitas, R., Spor, A., Mounier, A., Monard, C., Mougel, C., et al. (2020). A core microbiota of the plant-earthworm interaction conserved across soils. Soil Biol. Biochem. 144:107754. doi: 10.1016/j.soilbio.2020.107754
Jacquiod, S., Spor, A., Wei, S., Munkager, V., Bru, D., Sørensen, S. J., et al. (2022). Artificial selection of stable rhizosphere microbiota leads to heritable plant phenotype changes. Ecol. Lett. 25, 189–201. doi: 10.1111/ele.13916
Kapulnik, Y., and Kushnir, U. (1991). Growth dependency of wild, primitive and modern cultivated wheat lines on vesicular-arbuscular mycorrhiza fungi. Euphytica 56, 27–36. doi: 10.1007/BF00041740
Kavamura, V. N., Hayat, R., Clark, I. M., Rossmann, M., Mendes, R., Hirsch, P. R., et al. (2018). Inorganic Nitrogen Application Affects Both Taxonomical and Predicted Functional Structure of Wheat Rhizosphere Bacterial Communities. Front. Microbiol. 9:1074. doi: 10.3389/fmicb.2018.01074
Kavamura, V. N., Robinson, R. J., Hughes, D., Clark, I., Rossmann, M., Soares de Melo, I., et al. (2020). Wheat Dwarfing Influences Selection of the Rhizosphere Microbiome. Sci. Rep. 10:1452. doi: 10.1038/s41598-020-58402-y
Kiers, E. T., and Denison, R. F. (2008). Sanctions, cooperation, and the stability of plant-rhizosphere mutualisms. Annu. Rev. Ecol. Evol. Syst. 39, 215–236. doi: 10.1146/annurev.ecolsys.39.110707.173423
Kiers, E. T., Hutton, M. G., and Denison, R. F. (2007). Human selection and the relaxation of legume defences against ineffective rhizobia. Proc. R. Soc. B Biol. Sci. 274, 3119–3126. doi: 10.1098/rspb.2007.1187
Kim, B. H., Ramanan, R., Cho, D. H., Oh, H. M., and Kim, H. S. (2014). Role of Rhizobium, a plant growth promoting bacterium, in enhancing algal biomass through mutualistic interaction. Biomass Bioenergy 69, 95–105. doi: 10.1016/j.biombioe.2014.07.015
Kinnunen-Grubb, M., Sapkota, R., Vignola, M., Nunes, I. M., and Nicolaisen, M. (2020). Breeding selection imposed a differential selective pressure on the wheat root-associated microbiome. FEMS Microbiol. Ecol. 96:fiaa196. doi: 10.1093/femsec/fiaa196
Kusmec, A., Srinivasan, S., Nettleton, D., and Schnable, P. S. (2017). Distinct genetic architectures for phenotype means and plasticities in Zea mays. Nat. Plants 3, 715–723. doi: 10.1038/s41477-017-0007-7
Laitinen, R. A. E., and Nikoloski, Z. (2019). Genetic basis of plasticity in plants. J. Exp. Bot. 70, 739–745. doi: 10.1093/jxb/ery404
Lambers, H., Mougel, C., Jaillard, B., and Hinsinger, P. (2009). Plant-microbe-soil interactions in the rhizosphere: an evolutionary perspective. Plant Soil 321, 83–115. doi: 10.3389/fpls.2021.636709
Lebeis, S. L., Paredes, S. H., Lundberg, D. S., Breakfield, N., Gehring, J., McDonald, M., et al. (2015). Salicylic acid modulates colonization of the root microbiome by specific bacterial taxa. Science 349, 860–864. doi: 10.1126/science.aaa8764
Leff, J. W., Lynch, R. C., Kane, N. C., and Fierer, N. (2017). Plant domestication and the assembly of bacterial and fungal communities associated with strains of the common sunflower. New Phytol. 214, 412–423. doi: 10.1111/nph.14323
Lehmann, A., Barto, E. K., Powell, J. R., and Rillig, M. C. (2012). Mycorrhizal responsiveness trends in annual crop plants and their wild relatives-a meta-analysis on studies from 1981 to 2010. Plant Soil 355, 231–250. doi: 10.1007/s11104-011-1095-1
Leiser, W. L., Olatoye, M. O., Rattunde, H. F. W., Neumann, G., Weltzien, E., and Haussmann, B. I. (2016). No need to breed for enhanced colonization by arbuscular mycorrhizal fungi to improve low-P adaptation of West African sorghums. Plant Soil 401, 51–64. doi: 10.1007/s11104-015-2437-1
Lemanceau, P., Blouin, M., Muller, D., and Moënne-Loccoz, Y. (2017). Let the core microbiota be functional. Trends Plant Sci. 22, 583–595. doi: 10.1016/j.tplants.2017.04.008
Lundberg, D. S., Lebeis, S. L., Paredes, S. H., Yourstone, S., Gehring, J., Malfatti, S., et al. (2012). Defining the core Arabidopsis thaliana root microbiome. Nature 488, 86–90. doi: 10.1038/nature11237
Mendes, R., Kruijt, M., de Bruijn, I., Dekkers, E., van der Voort, M., Schneider, J. H. M., et al. (2011). Deciphering the rhizosphere microbiome for disease-suppressive bacteria. Science 332, 1097–1100. doi: 10.1126/science.1203980
Milla, R., Osborne, C. P., Turcotte, M. M., and Violle, C. (2015). Plant domestication through an ecological lens. Trends Ecol. Evol. 30, 463–469. doi: 10.1016/j.tree.2015.06.006
Mutch, L. A., and Young, J. P. W. (2004). Diversity and specificity of Rhizobium leguminosarumbiovar viciae on wild and cultivated legumes. Mol. Ecol. 13, 2435–2444. doi: 10.1111/j.1365-294X.2004.02259.x
Oyserman, B. O., Cordovez, V., Flores, S. S., Leite, M. F. A., Nijveen, H., Medema, M. H., et al. (2021). Extracting the GEMs:Genotype, Environment, and Microbiome Interactions ShapingHost Phenotypes. Front. Microbiol. 11:574053. doi: 10.3389/fmicb.2020.574053
Özkurt, E., Hassani, M. A., Sesiz, U., Künzel, S., Dagan, T., Özkan, H., et al. (2020). Seed-Derived Microbial Colonization of Wild Emmer and Domesticated Bread Wheat (Triticum dicoccoides and T. aestivum) Seedlings Shows Pronounced Differences in Overall Diversity and Composition. mBio 11:e02637–20. doi: 10.1128/mBio.02637-20
Peiffer, J. A., Spor, A., Koren, O., Jin, Z., Tringe, S. G., Dangl, J. L., et al. (2013). Diversity and heritability of the maize rhizosphere microbiome under field conditions. Proc. Natl. Acad. Sci. U.S.A. 110, 6548–6553. doi: 10.1073/pnas.1302837110
Pérez-Jaramillo, J. E., Carrión, V. J., Bosse, M., Ferrão, L. F., de Hollander, M., Garcia, A. A. F., et al. (2017). Linking rhizosphere microbiome composition of wild and domesticated Phaseolus vulgaris to genotypic and root phenotypic traits. ISME J. 11, 2244–2257. doi: 10.1038/ismej.2017.85
Pérez-Jaramillo, J. E., Carrión, V. J., de Hollander, M., and Raaijmakers, J. M. (2018). The wild side of plant microbiomes. Microbiome 6:143. doi: 10.1186/s40168-018-0519-z
Pérez-Jaramillo, J. E., Mendes, R., and Raaijmakers, J. M. (2016). Impact of plant domestication on rhizosphere microbiome assembly and functions. Plant Mol. Biol. 90, 635–644. doi: 10.1007/s11103-015-0337-7
Porter, S. S., and Sachs, J. L. (2020). Agriculture and the disruption of plant–microbial symbiosis. Trends Ecol. Evol. 35, 426–439. doi: 10.1016/j.tree.2020.01.006
R Core Team (2020). R: A Language and Environment for Statistical Computing. Vienna: R Foundation for Statistical Computing.
Ramirez, K. S., Craine, J. M., and Fierer, N. (2012). Consistent effects of nitrogen amendments on soil microbial communities and processes across biomes. Glob. Chang. Biol. 18, 1918–1927. doi: 10.1111/j.1365-2486.2012.02639.x
Ramirez, K. S., Lauber, C. L., Knight, R., Bradford, M. A., and Fierer, N. (2010). Consistent effects of nitrogen fertilization on soil bacterial communities in contrasting systems. Ecology 91, 3463–3470. doi: 10.1890/10-0426.1
Robinson, M. D., McCarthy, D. J., and Smyth, G. K. (2009). edgeR: a bioconductor package for differential expression analysis of digital gene expression data. Bioinformatics 26, 139–140. doi: 10.1093/bioinformatics/btp616
Sangabriel-Conde, W., Maldonado-Mendoza, I. E., Mancera-López, M. E., Cordero-Ramírez, J. D., Trejo-Aguilar, D., and Negrete-Yankelevich, S. (2015). Glomeromycota associated with Mexican native maize landraces in Los Tuxtlas, Mexico. Appl. Soil Ecol. 87, 63–71. doi: 10.1016/j.apsoil.2014.10.017
Schlatter, D. C., Yin, C., Hulbert, S., Burke, I., and Paulitz, T. (2017). Impacts of Repeated Glyphosate Use on Wheat-Associated Bacteria Are Small and Depend on Glyphosate Use History. Appl. Environ. Microbiol. 83:e01354–17. doi: 10.1128/AEM.01354-17
Schmalhausen, I. I. (1949). Factors of Evolution: The Theory of Stabilizing Selection. Philadelphia, PA: Blakiston.
Schöler, A., Jacquiod, S., Vestergaard, G., Schulz, S., and Schloter, M. (2017). Analysis of soil microbial communities based on amplicon sequencing of marker genes. Biol. Fertil. Soils 53, 485–489. doi: 10.1007/s00374-017-1205-1
Shannon, P., Markiel, A., Ozier, O., Baliga, N. S., Wang, J. T., Ramage, D., et al. (2003). Cytoscape: a software environment for integrated models of biomolecular interaction networks. Genome Res. 13, 2498–2504. doi: 10.1101/gr.1239303
Shi, S., Tian, L., Xu, S., Ji, L., Nasir, F., Li, X., et al. (2019). The rhizomicrobiomes of wild and cultivated crops react differently to fungicides. Arch. Microbiol. 201, 477–486. doi: 10.1007/s00203-018-1586-z
Smale, M. (1997). The Green Revolution and wheat genetic diversity: some unfounded assumptions. World Dev. 25, 1257–1269. doi: 10.1016/S0305-750X(97)00038-7
Sokal, R. R., and Rohlf, F. J. (1995). Biometry: The Principles and Practice of Statistics in Biological Research, 3rd Edn. New York, NY: W.H. Freeman.
Spor, A., Roucou, A., Mounier, A., Bru, D., Breuil, M. C., Fort, F., et al. (2020). Domestication-driven changes in plant traits associated with changes in the assembly of the rhizosphere microbiota in tetraploid wheat. Scientific 10:12234. doi: 10.1038/s41598-020-69175-9
Stearns, S. C. (1989). The evolutionary significance of phenotypic plasticity. BioScience 39, 436–445. doi: 10.2307/1311135
Szoboszlay, M., Lambers, J., Chappell, J., Kupper, J. V., Moe, L. A., and McNear, D. H. Jr. (2015). Comparison of root system architecture and rhizosphere microbial communities of Balsas teosinte and domesticated corn cultivars. Soil Biol. Biochem. 80, 34–44. doi: 10.1016/j.soilbio.2014.09.001
Trouvelot, A., Kough, J. L., and Gianinazzi-Pearson, V. (1986). “Mesure du taux de mycorhization VA d’un système radiculaire. Recherche de méthodes d’estimation ayant une signification fonctionnelle,” in Physiological and Genetical Aspects of Mycorrhizae. Proceedings of the 1st European Symposium on Mycorrhizae, eds V. Gianinazzi-Pearson and S. Gianinazzi (Paris: Institut National de la Recherche Agronomique), 217–221.
Turcotte, M. M., Lochab, A. K., Turley, N. E., and Johnson, M. T. (2015). Plant domestication slows pest evolution. Ecol. Lett. 18, 907–915. doi: 10.1111/ele.12467
Vierheilig, H., Coughlan, A. P., Wyss, U., and Piché, Y. (1998). Ink and vinegar, a simple staining technique for arbuscular-mycorrhizal fungi. Appl. Environ. Microbiol. 64, 5004–5007. doi: 10.1128/AEM.64.12.5004-5007.1998
Voss-Fels, K. P., Stahl, A., Wittkop, B., Lichthardt, C., Nagler, S., Rose, T., et al. (2019). Breeding improves wheat productivity under contrasting agrochemical input level. Nat. Plants 5, 706–714. doi: 10.1038/s41477-019-0445-5
Wagner, M. R., Lundberg, D. S., Tijana, G., Tringe, S. G., Dangl, J. L., and Mitchell-Olds, T. (2016). Host genotype and age shape the leaf and root microbiomes of a wild perennial plant. Nat. Commun. 7, 1–15. doi: 10.1038/ncomms12151
Weese, D. J., Heath, K. D., Dentinger, B. T., and Lau, J. A. (2015). Long-term nitrogen addition causes the evolution of less-cooperative mutualists. Evolution 69, 631–642. doi: 10.1111/evo.12594
White, T., Bruns, T., and Lee, S. (1990). “Amplification and direct sequencing of fungal ribosomal RNA genes for phylogenetics,” in PCR Protocols: a Guide to Methods and Applications, eds M. A. Innis, D. H. Gelfand, J. J. Sninsky, and T. White (London: Academic Press), 315–322. doi: 10.1016/B978-0-12-372180-8.50042-1
Yang, C., Hamel, C., Vujanovic, V., and Gan, Y. T. (2012). Non-target effects of foliar fungicide application on the rhizosphere: diversity of nifH gene and nodulation in chickpea field. J. Appl. Microbiol. 112, 966–974. doi: 10.1111/j.1365-2672.2012.05262.x
Keywords: genotype, environment, phenotypic plasticity, rhizosphere microbiota, mycorrhiza, plant breeding
Citation: Jacquiod S, Raynaud T, Pimet E, Ducourtieux C, Casieri L, Wipf D and Blouin M (2022) Wheat Rhizosphere Microbiota Respond to Changes in Plant Genotype, Chemical Inputs, and Plant Phenotypic Plasticity. Front. Ecol. Evol. 10:903008. doi: 10.3389/fevo.2022.903008
Received: 23 March 2022; Accepted: 15 June 2022;
Published: 13 July 2022.
Edited by:
Jennifer L. Matthews, University of Technology Sydney, AustraliaReviewed by:
Alessio Mengoni, University of Florence, ItalyJohannes Helder, Wageningen University and Research, Netherlands
Copyright © 2022 Jacquiod, Raynaud, Pimet, Ducourtieux, Casieri, Wipf and Blouin. This is an open-access article distributed under the terms of the Creative Commons Attribution License (CC BY). The use, distribution or reproduction in other forums is permitted, provided the original author(s) and the copyright owner(s) are credited and that the original publication in this journal is cited, in accordance with accepted academic practice. No use, distribution or reproduction is permitted which does not comply with these terms.
*Correspondence: Manuel Blouin, bWFudWVsLmJsb3VpbkBhZ3Jvc3VwZGlqb24uZnI=