- 1Vrije Universiteit Amsterdam, Amsterdam, Netherlands
- 2Dutch Butterfly Conservation, Wageningen, Netherlands
Urbanization drastically changes environmental conditions, including the introduction of sensory pollutants, such as artificial light at night (ALAN) and anthropogenic noise. To settle in urban habitats, animals need to cope with this new sensory environment. On a short timescale, animals might cope with sensory pollutants via behavioral adjustments, such as changes in sexual signaling, which can have important fitness consequences. While ALAN and anthropogenic noise generally co-occur in urban habitats and are known to be able to interact to modify behavioral responses, few studies have addressed their combined impact. Our aim was, therefore, to assess the effects of ALAN, anthropogenic noise, and their interaction on sexual signaling in túngara frogs (Engystomops pustulosus). We observed the calling behavior of frogs in urban and forest areas, and subsequently recorded these frogs in a laboratory set-up while independently manipulating light and noise levels. Frogs in urban areas called with a higher call rate and complexity, which was correlated with local sensory conditions. Furthermore, our lab experiment revealed that ALAN can directly alter sexual signaling independently as well as in combination with anthropogenic noise. Exposure to ALAN alone increased call amplitude, whereas a combination of ALAN and anthropogenic noise interacted to lead to a higher call complexity and amplitude. Overall, the response patterns consistently showed that exposure to ALAN and anthropogenic noise led to more conspicuous sexual signals than expected based on the additive effects of single pollutants. Our results support the notion that urban and forest population differences in sexual signaling can be partially explained by exposure to ALAN and anthropogenic noise. Furthermore, by demonstrating interactive effects between light and noise pollution, our study highlights the importance of examining the effects of multisensory pollution, instead of single pollutants, when trying to understand phenotypic divergence in urbanized vs. natural areas.
Introduction
Human populations are increasing rapidly in size, and are simultaneously becoming more concentrated in cities (United Nations, 2018). Consequently, natural areas are being converted into areas specifically designed for humans, characterized by built structures and other infrastructure, a process known as urbanization. Urbanization brings along drastic changes in biotic and abiotic conditions (Santangelo et al., 2022), including altered resource availability, predator abundance, and light and noise pollution. Species must, therefore, adapt to these novel urban conditions (McKinney and Lockwood, 1999; McKinney, 2006), either via an evolutionary response over generations, or via plasticity within their lifetime (reviewed in Candolin and Wong; Sol et al., 2013).
Behavioral plasticity in communication is commonly observed in response to urban conditions, presumably because behavior can be adjusted on very short time scales and the maintenance of effective communication is crucial for species settlement and persistence in a novel environment. Sexual communication, in particular, has important fitness consequences, as it typically determines the outcome of rival competition, mate attraction, and (cryptic) mate choice (Darwin, 1871; Andersson, 1994). The production, transmission, and perception of sexual signals, such as song and pheromones, are known to be affected by the environment (e.g., Brumm and Slabbekoorn, 2005), and drastic environmental changes due to urbanization thereby pose novel pressures on communication systems. However, the production of sexual signals is often flexible. Animals could therefore behaviorally adjust to novel urban environments by changing their sexual signals, potentially improving signal efficacy.
Urban sensory environments are characterized by high levels of artificial light at night (ALAN) and anthropogenic noise (e.g., street lighting and traffic noise) (Barber et al., 2010; Kyba et al., 2017). These sensory pollutants can interfere with the detection, processing, or interpretation of sexual signals (Halfwerk and Slabbekoorn, 2015; Dominoni et al., 2020a). In response to exposure to sensory pollutants, animals can behaviorally adjust the timing or location of signaling, or the signal itself (reviewed by Cronin et al., 2022). For example, when exposed to ALAN, multiple bird species advance the timing of singing (Kempenaers et al., 2010). In response to noise, animals can change sexual signals by, for example, adjusting the amplitude (Halfwerk et al., 2016), frequency (Slabbekoorn and Peet, 2003; Parris, 2009), or use of different signal elements (Halfwerk and Slabbekoorn, 2009; Orci et al., 2016).
While ALAN and anthropogenic noise generally co-occur in urban areas (McMahon et al., 2017; Votsi et al., 2017), few studies have examined their combined effects (“multisensory pollution”) on animal behavior (reviewed in Halfwerk and Slabbekoorn, 2015; Halfwerk and Jerem, 2021). Sensory stimuli, such as visual and auditory cues, are processed by different sensory systems, but can have complex interactions at higher perceptual levels (e.g., Taylor and Ryan, 2013). Consequently, ALAN and anthropogenic noise can have interactive effects that differ from the expected additive effects of these pollutants in isolation, such as enhancing or mitigating the single effects (Piggott et al., 2015; Hale et al., 2017; Dominoni et al., 2020b). Interactive effects of ALAN and anthropogenic noise have been shown, for example, in activity patterns (Dominoni et al., 2020b), abundance patterns (Wilson et al., 2021), and development and survival in birds (Ferraro et al., 2020). Particularly in the context of communication, animals generally rely on multiple modalities (Partan and Marler, 1999), but we currently lack an understanding of how multiple sensory pollutants affect communication. Studying the combined effects of ALAN and anthropogenic noise is therefore essential to obtain more comprehensive insights into how multisensory pollution affects sexual signaling.
The túngara frog (Engystomops pustulosus) produces acoustic sexual signals and occurs throughout the lowlands of Central and South America where it inhabits both urban and forest areas. Males display from puddles on the forest floor or road and start their call with a “whine” (downward frequency sweep), optionally followed by one or more “chucks” (short high amplitude element), which increases call complexity (Ryan, 1985). Males in urban sites produce more complex calls at higher call rates compared to forest sites, making them more attractive to females (Halfwerk et al., 2019). Differences in call complexity partly persist under urban common garden conditions (Halfwerk et al., 2019), but it is unclear to what extent sensory pollutants directly drive this behavioral response.
Our aim was therefore to examine the effects of ALAN, anthropogenic noise and their interaction on calling behavior in túngara frogs. We observed the calling behavior of frogs in urban and forest sites with varying light and noise levels and tested for relationships between sexual signaling and local sensory conditions. Subsequently, we transported these frogs to our standardized recording set-up, in which we manipulated light and noise levels and recorded calling behavior in response to various conspecific playbacks. Since very few previous studies have addressed the interactive effects of light and noise pollution, and those that have done so show complex synergistic, antagonistic, or even emergent effects (Halfwerk and Jerem, 2021), we did not have clear expectations of the interactive effects of light and noise pollution on calling behavior. This study intends to provide insights into the role of (multi)sensory pollution in changes in sexual signaling in response to urban conditions.
Materials and methods
Sample sites and frog collection
We collected 80 male túngara frogs [Engystomops (= Physalaemus) pustulosus] at six urban and seven forest sites. Sampling sites were all located east of the Panama Canal, around Panama City and the canal zone, and in national parks Soberanía and Camino de Cruces (see Figure 1). To obtain estimates of sensory conditions at each site, we took the average of multiple light (Lux meter HT309, HT Instruments, illumination level reading range 0.01–400 lux, upward and four cardinal directions, breast height) and noise measurements (SPL meter, type 407764, Extech Instruments; two opposite directions for 10 s; A and C weighted, fast, low) taken at different calling sites in 2019 and/or 2021 between 20:00 and 00:00. We lacked sensory data from one urban site, which we replaced with the averages of the other urban sites (which did not influence the results). Average light levels ranged from < 0.01 to 3.7 lux (mean ± SD: urban 1.95 ± 1.5, forest 0.03 ± 0.01), and noise levels ranged from 43.1 to 58.2 dBA (mean ± SD: urban 51.7 ± 5.7, forest 50.6 ± 3.5) and 49.4 to 64.6 dBC (mean ± SD: urban 57.8 ± 5.4, forest 54.6 ± 5.7).
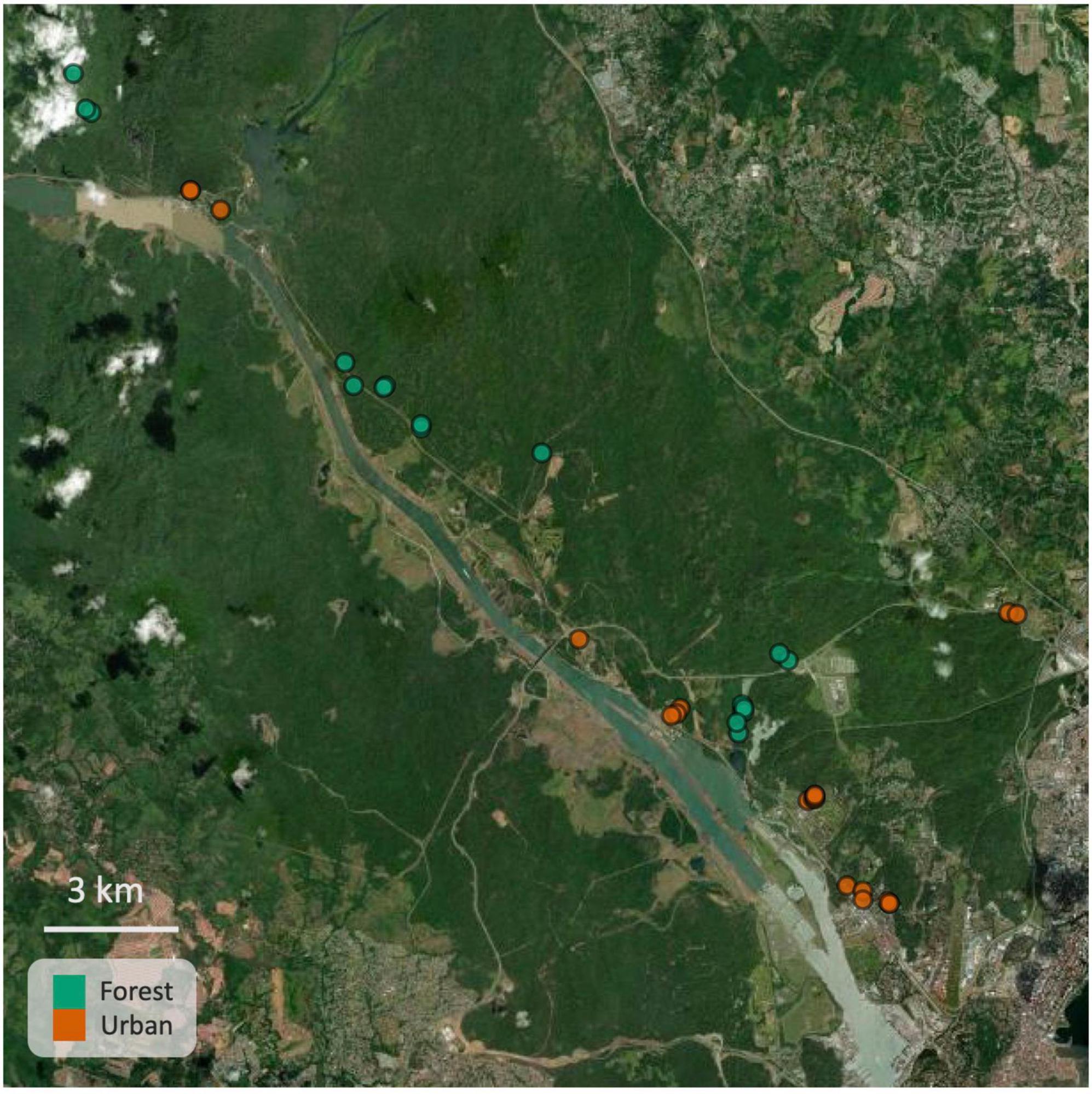
Figure 1. Map showing urban (orange) and forest (green) sampling locations around Panama City, the canal zone and in national forests (Soberanía and Camino de Cruces). Map was created using the leaflet package in R (Cheng et al., 2021).
Per collection night (between 19:15 and 22:15) we caught four calling frogs from an urban site and four from a forest site, and transported them to the lab. To minimize the sampling bias, we decided in a random but balanced manner which site we would collect first. Before catching each frog, we noted its calling behavior in the field for 1 min: the number of calls (“call rate”), the maximum number of chucks per call (“call complexity”), and the number of calling males in the vicinity (“chorus size”).
Frogs were housed individually in a small enclosure (18 × 11.5 × 13 cm, L × W × H), with mesh on all sides except for the bottom, in one of eight custom-built recording boxes lined with sound isolating foam (36 × 25 × 58 cm, L × W × H). The small enclosure contained a shelter and a small bowl (ø 8.5 × height 4.0 cm) with dechlorinated water and a strip of mesh for the frog to hold onto while calling. Recording boxes were situated in the same location and temperature and humidity levels were similar across boxes (∼26°C, ∼85% relative humidity). We fed frogs a fixed amount of termites on the second or third day in the lab.
Experiments were conducted in September and October 2019 in Gamboa, Republic of Panamá, using facilities of the Smithsonian Tropical Research Institute (STRI). Frogs were kept in the lab for a maximum of five nights. Before releasing the frogs at their capture sites, we recorded body size as snout-vent length (SVL, mm) and mass (g), and took toe clip samples for later DNA analyses and to prevent re-testing. All experiments were licensed and approved by STRI (IACUC permit: 2019-0301-2022) and the Autoridad Nacional del Ambiente de Panamá (SE/A-47–19).
Experimental design
Sensory treatments
After one night under baseline conditions (low light levels, < 0.01 lx, no noise playbacks) for acclimatization, we exposed frogs for two nights to one of four treatment combinations: forest light and noise (“Control”), forest light and urban noise (“Noise”), urban light and forest noise (“Light”), and both urban light and noise (“Light + Noise”). Each frog was randomly assigned to a treatment while ensuring each treatment was equally represented across frogs from the different collection sites in each round. To provide standardized but ecologically relevant stimuli, we synthesized noise based on field recordings obtained at four urban and four forest sites, which differed in peak amplitude (∼65 dB for urban noise; and ∼45 dB for forest noise, at the center of the enclosure, SPL meter; A weighted, fast, and low), amplitude modulation (higher for urban noise), and frequency (more energy in frequencies < 2 kHz for urban noise, see Supplementary Figure 1). The noise was looped continuously using small speakers [JBL clip 3, frequency response 120 Hz–20 kHz (-6dB)] at ∼25 cm of the middle of the enclosure, connected to an iPhone or iPod (Apple). We equipped the recording boxes with white broad-spectrum LEDs (Nichia, NSPW500DS, peak ∼460 nm) to set night light levels to mimic urban (1.3 lx) or forest (< 0.01 lx) levels (measured at the middle of enclosure lux meter, HT309, HT Instruments), and to expose the frogs to daylight levels (∼250 lx) between 07:00 and 19:00. During the sensory treatments, 45 out of 80 frogs called in our set-up, which was approximately equally spread across the sensory treatment (Control: 13, Noise: 9, Light: 11, Light + Noise: 12) and origin (Forest: 23, Urban: 22).
To test the effects of sensory treatments across different social environments, we exposed the frogs to different playbacks. At 19:00, a 1-h loop of artificial frog calls started playing at ∼25 cm from the center of the enclosure. For each new round of frogs, we calibrated the speakers (FR8WP, Visaton, frequency response 100 Hz to 20 kHz (-10 dB), connected to Renkforce T21 amplifiers) using a 1 kHz tone set at 74 dB at 50 cm (SPL meter, A—weighted, fast, low). The playback loop started with a chorus consisting of artificial calls of varying complexity, amplitude, and call rate (∼ 74 dB, 3 × 3-min bouts, with 20 s of silence in between bouts). After a 2.5-min break, a single artificial rival call played every 2 s (∼ 80 dB, 3 × 3-min bouts, with 20 s of silence in between bouts). The first bout had whines only, followed by a bout with whines with one chuck, ending with a bout with whines with three chucks, with again 20 s of silence in between bouts. The chorus and rival blocks were repeated once, followed by 15 min of silence to complete the 1-h playback file. On both nights we repeatedly played this 1-h loop to maximize the chance of recording calling behavior in response to each of the playback types. We recorded calling behavior until 05:00 with omnidirectional lavalier microphones (AKG C417 PP, calibrated using a G.R.A.S. 42 AB tone generator, 114 dB at 1 kHz) ∼40 cm above the frog connected to an audio recorder (Zoom F8N multitrack field recorder).
Audio analyses
We detected calls in 40% of the audio files using autodetec in the WarbleR package (Araya-Salas and Smith-Vidaurre, 2017) in R (v 3.6.1, R Core Devlopment Team, 2019), using a bandpass between 2 and 4.5 kHz, amplitude threshold of 8%, window length of 128, and duration between 150 and 600 ms. We checked the detection by verifying that 3% of the files without any detected calls indeed did not have calls. For nights with < 200 calls detected, we manually checked if the recordings contained calls. Next, we annotated a random 1% of the selections based on spectrograms. Using these annotated selections, we trained a neural network using TensorFlow 2 and Keras in Python to determine whether the detected calls were indeed the focal frog’s calls. The network contained three layers, the first and second layers used 128 nodes and a reLU activation function, the final layer had three nodes and a softmax activation function. The network was trained in 50 epochs. The calls with a low certainty (< 0.45, 1.4% of the selections) we annotated manually. We again randomly annotated 1% of the spectrograms to assess the accuracy of the network, based on which we estimate a false positive and false negative rate of 0.28% and 0.49%, respectively. We applied the network to the rest of the data to obtain the total number of calls per frog per night. We corrected for differences in recording length by dividing the number of calls by the number of recorded hours.
Call behavior analyses
For each frog, we manually selected two call bouts per playback type per night for analyses using Audacity (version 2.3.3). To cover within-frog variation, we selected these bouts from the recording with the first calling activity as well as the recording with peak activity, as indicated by the algorithm output. We selected 1-min call bouts, which started after the first 15 s of calling (“warm-up”), after which males typically call steadily. In a few cases, the frogs did not call steadily and only produced short bouts, which we included if they were minimally 30 s and had at least six calls with an interval of maximally 10 s. For the short bouts, we did not exclude a warm-up period as this was representative of the calling behavior.
For each bout, we noted the number of calls (“call rate”) and the total number of chucks (“call complexity”). Additionally, for the rival playback (W, WC, WCCC), we counted the number of calls that overlapped with the playback (“call overlap”), which are known to be important parameters for rival interactions and mate attraction (Ryan, 1980; Tárano, 2015; Smit et al., 2019). In total, we analyzed 460 min (C: 107, N: 99, L: 109, L + N: 145) from 45 frogs exposed to sensory treatments.
Amplitude measurements
Per frog per night, we selected up to three calls for amplitude measurements in each playback type. If available, we selected these calls from call bouts during the activity peak, and otherwise from call bouts during the first activity. Calls were selected based on the oscillogram using Avisoft SASLab Pro (Avisoft Bioacoustics, version 5.2), after filtering the recordings with a high pass filter (> 300 Hz). Calls were randomly selected, with the limitation that the calls needed to be non-overlapping with at least a 0.1-s gap until the next artificial rival call. We selected a total of 794 calls from 45 different frogs. From these calls, we obtained peak-to-peak amplitude (P2P) and root mean square (RMS) using the seewave package (v 2.1.6, Sueur et al., 2008). For RMS, we corrected for varying levels of background noise, by subtracting RMS of 0.1 s (0.3 s in case of chorus playback) before and after the call using the RMS method (also see Halfwerk et al., 2016). RMS values were transformed to absolute dB values, using an average RMS of a 1 kHz calibrator tone of 114 dB for each microphone separately. For analyses, absolute values were back-transformed to a linear scale.
Statistical analyses
We carried out statistical analyses using (generalized) linear mixed models using the lme4 (v 1.1-26, Bates et al., 2015) and glmmTMB (v 1.0.2.1., Brooks et al., 2017) packages in R (v 3.6.1, R Core Devlopment Team, 2019). We tested for significance by comparing the model with and without the factor of interest using log-likelihood ratio tests. We verified the absence of heterogeneity and residual patterns by plotting the residuals against the fitted values, and against each covariate in the model and not in the model (Zuur and Ieno, 2016). Additionally, for models with (generalized) Poisson and (negative) binomial distributions, we checked for overdispersion and zero-inflation using the DHARMa package (Hartig, 2021). Numerical covariates were standardized in all models.
For field data, we assessed the effects of site type (urban/forest) and light and noise levels, and their interaction, on call rate and maximum complexity. Since we had slightly under- or over-dispersed count data, we used generalized linear mixed models with generalized Poisson distributions with log-link functions. Site type (urban/forest) was collinear with light and noise levels, and therefore we ran separate models for the effects of site type and the effects of light and noise levels. Noise levels measured on the dBA and dBC scales were highly collinear, and we decided to run the analyses only on noise on dBA scale. All models contained the number of simultaneously calling frogs (“chorus size”) as a covariate since this is known to affect calling behavior. We added site ID as a random intercept to account for multiple observations per site.
For the lab data, we analyzed calling behavior in each of the three playback types (silence, rival, and chorus) separately, because calling behavior varied greatly according to the social environment. All initial models contained light and noise treatments, their interaction, and frog origin, which all were factors with two levels (urban/forest). Additionally, we added the night number (factor; 2 or 3) as a covariate. In the case of the rival playback, we added the call complexity of the rival playback (“intensity”; 0, 1, or 3 chucks) as an ordered covariate. To account for repeated measurements of frog ID nested in site ID, we added frog ID and site ID as random intercepts. In the case of peak-to-peak amplitude, the microphone number was added to account for variation between the microphones used.
For both the number of calls per hour and call rate (per min), we first fitted a Poisson model (log link). Because we encountered problems with the heterogeneity of model residuals vs. fitted values, we switched to a gaussian model (identity link). Additionally, we log-transformed a total number of calls per hour in order to meet model assumptions, and for call rate we divided the number of calls by bout length to correct for variation in the length of scored segments. Call complexity was modeled during the silence playback as binary data (chucks present vs. chucks absent) in a binomial model (logit link), because of zero inflation and convergence issues with a Poisson distribution. For the other playback types, we compared negative binomial, Poisson, and generalized Poisson models with and without zero-inflation to find the model with the lowest AIC score to continue with the analyses. The best model for both the chorus and rival playbacks were negative binomial models (log link) with zero inflation. The proportion of overlapping calls was modeled using a beta binomial distribution (log link), since overdispersion was indicated when running a model with a binomial distribution. In all models with either the number of chucks or the proportion of overlapping calls as response variables, we added the number of produced calls as a covariate.
For call amplitude, we averaged up to three RMS or peak-to-peak (P2P) amplitude measurements per analyzed call bout to account for similarities within bouts. To assess the effect of sensory treatment on RMS or P2P, we added the frog’s body mass as a covariate to the model, since this has been shown to explain a significant amount of variation in whine amplitude (James et al., 2021). To assess origin effects on amplitude, we did not include mass in the initial model, as mass seemed to slightly differ between urban and forest frogs, which could be an important factor underlying origin effects. In case of a significant effect of origin, we added mass to the model to examine its role in the difference found. For estimates and visual representation, we back-transformed amplitude estimates to the dB scale.
We started by testing the statistical significance of the interaction between light and noise treatment, followed by testing the effects of light and noise treatment separately using the model without interaction. For subsequent model comparisons, we removed the interaction term when it was found not to be statistically significant. Any significant interaction effect was followed up by a comparison of the estimates from the model with interaction (interactive effects) and without interaction (expected additive effects), and interactions were characterized as either antagonistic (AB < A + B) or synergistic (AB > A + B) (following Hale et al., 2017 and Halfwerk and Jerem, 2021). Because of a lack of power, we did not test the interaction between sensory treatment and frog origin. We set statistical significance levels to p < 0.05, and we report trends (p < 0.1) in the main text if along similar lines with statistically significant findings. Graphs were made using the ggplot package (v. 3.3.5, Wickham, 2009).
Results
Calling behavior and sensory conditions in the field
Frogs produced twice as many calls (+100.3%) (p < 0.001, χ2 = 14.870; Figures 2A,D and Supplementary Table 1) with a higher maximum complexity (+0.4 chucks, p = 0.04, χ2 = 4.3657) in urban sites compared to forest sites. Local light and noise levels did not have interactive effects on call rate or complexity (all p > 0.60; see Supplementary Table 1). Call rate increased with light levels (p = 0.01, χ2 = 5.9942, Figure 2B) and tended to increase with noise levels (p = 0.05, χ2 = 3.7720, Figure 2C). For maximum call complexity, there was no statistically significant association with light or noise levels (all p > 0.10, Figures 2E,F).
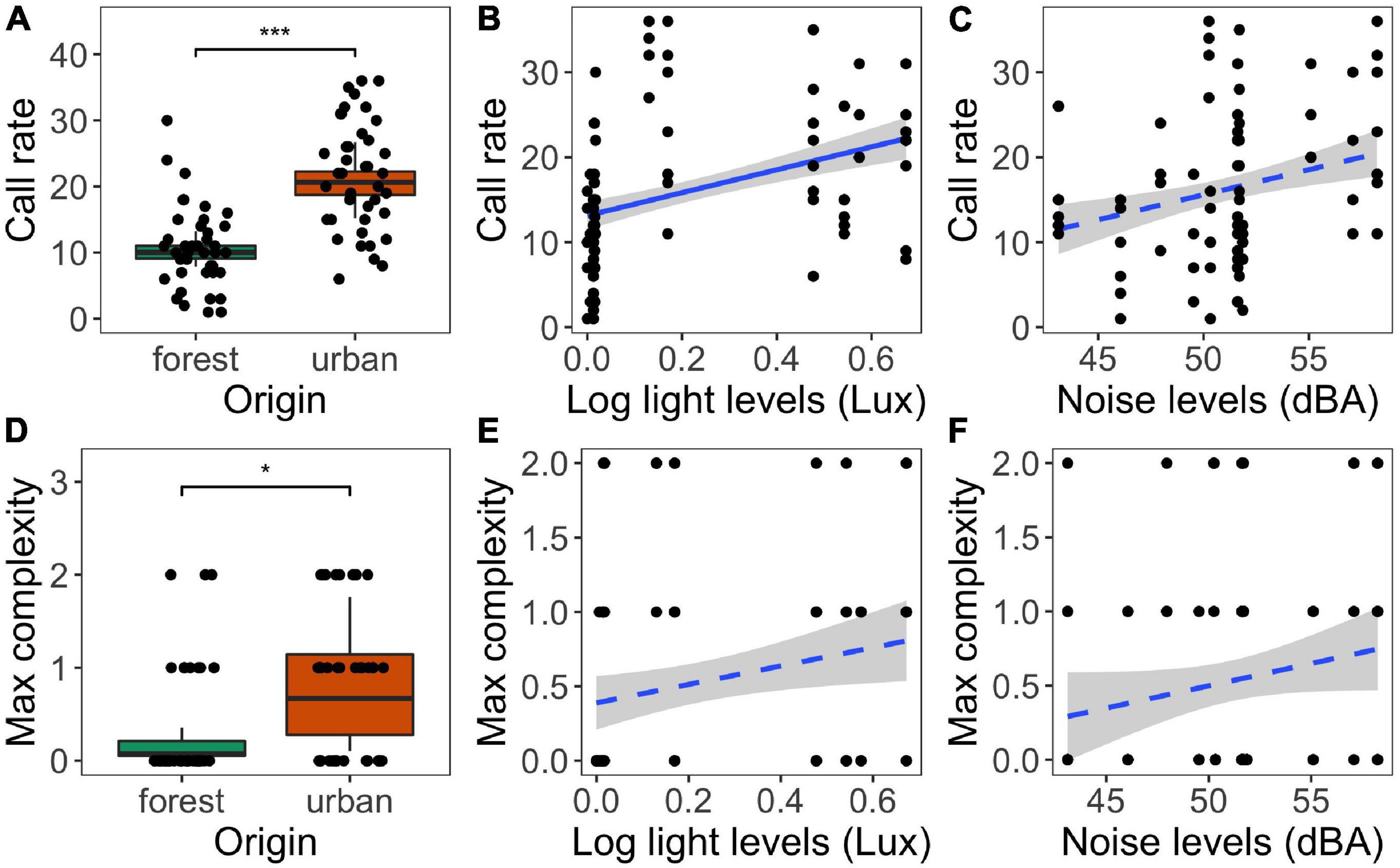
Figure 2. Associations between calling behavior (call rate and maximum call complexity) and origin (A,D), and local light (B,E) and noise levels (C,F). Significant differences between urban and forest sites are indicated with an asterisk (*< 0.05, ***< 0.001). Solid and dashed regression lines (with 95% CI) indicate significant and non-significant relations, respectively. Boxplots and regression lines are based on model predictions (excluding the interaction between light and noise in case that was not statistically significant), data points shown are raw data. See text and Supplementary Table 1 for statistics.
Calling behavior in urban and forest frogs in the lab
Total number of calls and call rate did not differ between frogs from urban vs. forest sites (all p > 0.19; Supplementary Figures 2A,B and Supplementary Tables 2, 3). Call overlap with the rival playback, however, was significantly higher in urban frogs compared to forest frogs (24.8% vs. 12.6%, p = 0.03, χ2 = 4.779; Supplementary Figures 2A,D). Forest frogs, on the other hand, called with a 24.0% higher call complexity than urban frogs during the rival playback (p = 0.01, χ2 = 6.505; Supplementary Figure 2C and Supplementary Table 4), but not during the other playback types (all p > 0.55). Additionally, forest frogs called with a 4.6 dB higher peak-to-peak amplitude (P2P) compared to urban frogs during the rival playback (p = 0.04, χ2 = 4.3706; Supplementary Figure 2E and Supplementary Table 7). Body mass seemed to partially explain the difference in P2P, as adding mass to the model decreased the estimated difference between urban and forest frogs to 3.7 dB, which was no longer statistically significant (p = 0.06, χ2 = 3.5383). We found no statistically significant differences between urban and forest frogs in the P2P in other playback types (all p > 0.23), nor when we looked at the RMS (root mean square) of calls (all p > 0.24; Supplementary Figure 2F and Supplementary Table 6).
The effects of sensory treatments on calling behavior
Sensory treatment did not affect the total number of calls, call rate, or call overlap (all p > 0.08; Figures 3A,B,D and Supplementary Tables 2, 3 and 5). Urban light and noise did have a significant interaction effect on call complexity during the rival playback (χ2 = 5.388, p = 0.02; Figure 3C and Supplementary Table 4). In the urban light and noise treatment, frogs produced 17.2% more chucks compared to the control conditions, which is higher and in a different direction than the expected effect under additivity of exposure to the separate pollutants (14.0%; Figure 4C). Therefore, we classify the interaction effect of urban light and noise as “antagonistic.” Similarly, the interaction between urban light and noise on call complexity had a nearly statistically significant antagonistic effect during the chorus playback (p = 0.06, χ2 = 3.6816). No other treatment effects on call complexity were found during the chorus playback or silence (all p > 0.35).
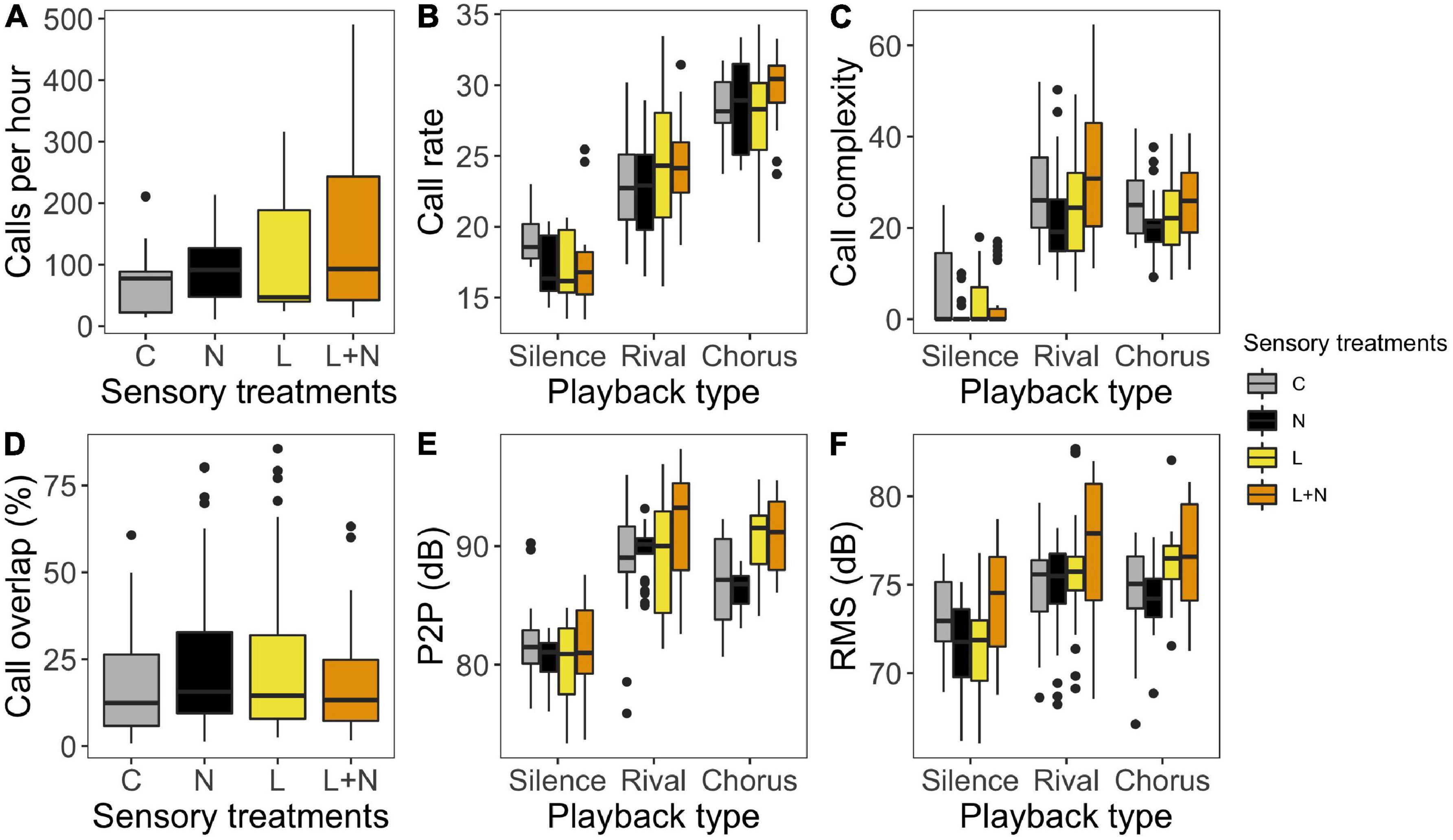
Figure 3. Calling behavior in response to sensory treatments (Control, Noise, Light, Light + Noise; grey, black, yellow and orange) in different playback types (silence, rival and chorus). (A) Total number of calls per hour. (B) Number of calls per minute. (C) Number of chucks per minute. (D) Percentage of calls overlapping with the played rival call. (E) Peak-to-peak amplitude of whole call. (F) RMS of whole call. Graphs are based on model predictions (excluding the interaction between light and noise in case that was not statistically significant), except for call complexity during silence, which is based on raw data since we ran the model on binary data (chucks/no chucks). See text and Supplementary Tables 2–6 for statistics.
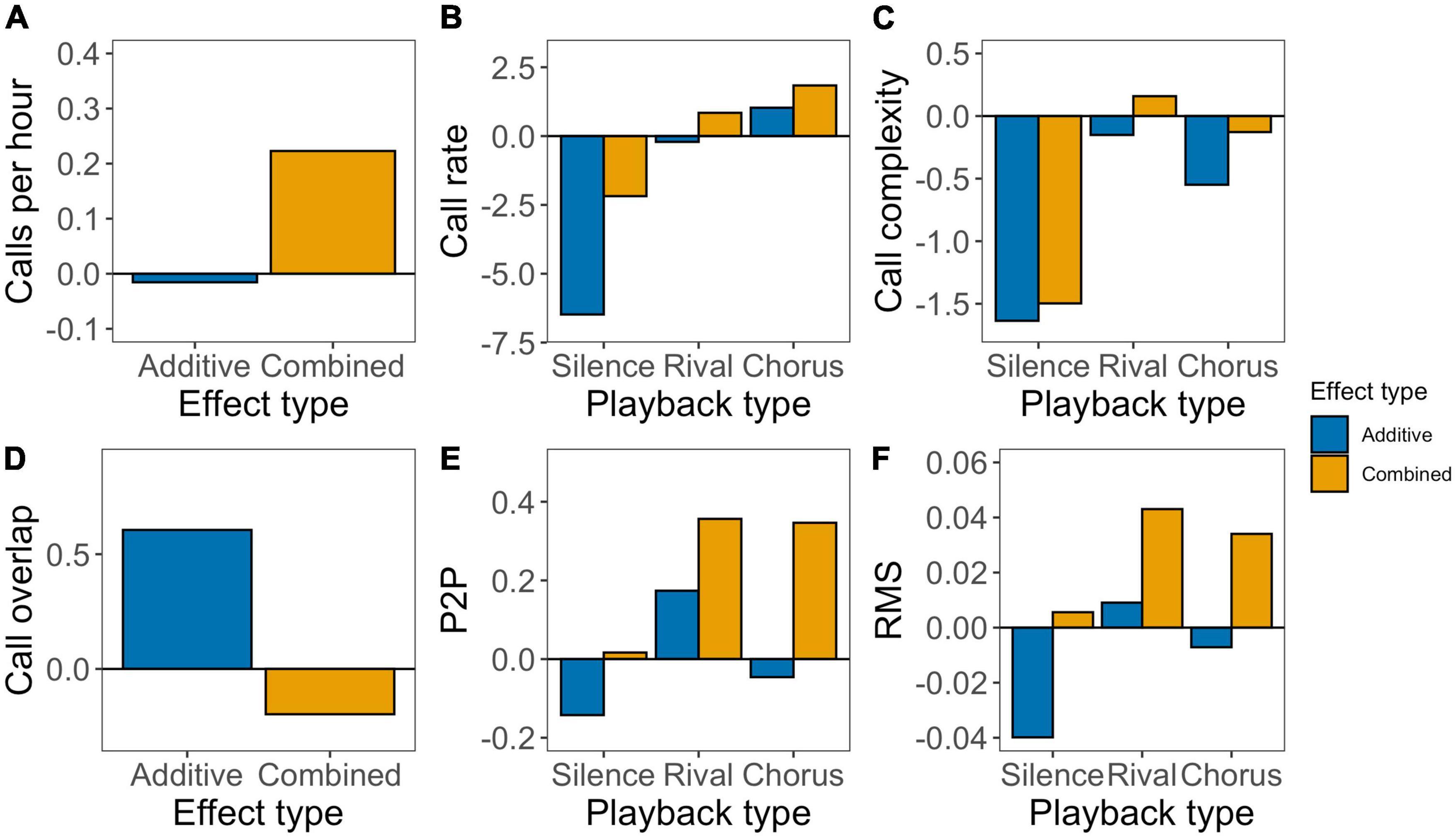
Figure 4. Expected additive effects (the sum of the observed single effects, blue), and the observed combined effects (orange) of light and noise treatments in different playback types (silence, rival and chorus). Estimates are obtained from the models including interaction between light and noise treatment and are not back transformed. (A) Total number of calls. (B) Number of calls per minute. (C) Number of chucks per minute. (D) Percentage of calls overlapping with the played rival call. (E) Peak-to-peak amplitude of whole call. (F) RMS of whole call. See text and Supplementary Table 8 for statistics.
Next, we looked into the effects of sensory treatment on call amplitude. Urban light and noise had a statistically near-significant interaction effect on peak-to-peak amplitude (P2P) during the chorus (p = 0.05, χ2 = 3.7696; Figure 3E and Supplementary Table 7). A similar pattern was visible in the silence (p = 0.09, χ2 = 2.9126), but not in the rival playback (p = 0.41, χ2 = 0.6917). Additionally, during the chorus playback, exposure to urban light, independently of noise treatment, led to an increase of 4.7 dB in P2P compared to exposure to forest light (p = 0.01, χ2 = 6.1385). We did not find any other effects of urban light or noise on P2P (all p > 0.06). The root mean square (RMS) of the calls was affected by a significant interaction between urban light and noise during silence (p = 0.047, χ2 = 3.9315; Figure 3F and Supplementary Table 6). Exposure to the combination of urban light and noise increased RMS compared to the control conditions (+ 0.7 dB), which was again an antagonistic effect (expected additive effects: -5.5 dB; Figure 4F). We found no other statistically significant treatment effects on RMS during any of the other playback types (all p > 0.10). Because of a lack of power, we did not test the interaction between sensory treatment and frog origin.
While only statistically significant in two cases, in 13 out of 14 comparisons observed effects were higher under combined urban light and noise exposure than expected additive effects based on exposure to the single pollutants (Figure 4 and Supplementary Table 8). This pattern was visible during all playback types in the number of calls per hour, call rate, complexity, P2P, and RMS (Figures 4A–C,E,F), but not in call overlap (Figure 4D). When the expected additive effects were positive, we generally found the observed combined effects to be more positive (“synergism”). Alternatively, in case the expected additive effects were negative, we mostly observed less negative or even positive combined effects (“antagonism”).
Discussion
Our aim was to assess the effects of ALAN, anthropogenic noise and their interaction on sexual signaling in túngara frogs. We related local light and noise levels to calling behavior in the field as well as experimentally manipulated sensory conditions in our lab set-up. In urban field sites, frogs called with a higher call rate and complexity compared to forest sites, which was partially associated with higher light and noise levels. Manipulating light and noise levels revealed that frogs adjust their calling behavior as a direct response to sensory conditions, although effect sizes appeared to be minor. Exposure to ALAN increased call amplitude, whereas combined exposure to ALAN and anthropogenic noise lead to interactive effects on both call amplitude and call complexity. Last, we found persisting differences in calling behavior between frogs from urban and forest origins in our lab set-up.
Impacts of sensory pollutants on calling behavior
Frogs adjusted their sexual signaling according to sensory treatment in our experiment. Sensory pollutants have been described to affect animal sensory systems in three main ways: by interfering with signal detection or discrimination (“masking”), cognitive processing (“distraction”), or interpretation of signals (“misleading”) (Halfwerk and Slabbekoorn, 2015; Dominoni et al., 2020a). In our study, frogs were most likely affected by a combination of these three perceptual mechanisms, which might explain some of the interactive effects we observed.
Anthropogenic noise most likely influences signaling via the mechanism of masking, although we did not find a significant impact of noise exposure when tested in isolation. Other studies have shown changes in response to noise exposure in, for example, signal amplitude (Derryberry et al., 2020), frequency (Slabbekoorn and Peet, 2003; Parris, 2009), and length (Miller et al., 2000). A previous study on túngara frogs also showed responses to noise in terms of call rate, complexity, and amplitude (Halfwerk et al., 2016). In comparison with our study, in Halfwerk et al. (2016), the noise exposure was shorter-term, and the noise stimuli were louder with a higher degree of spectral overlap with the frogs’ calls, which might explain why we did not observe any effect of urban noise exposure.
We did observe a clear effect of ALAN on calling behavior in our study, which led to an increase in call amplitude (+4.7 dB). The effect of light pollution on an acoustic signal illustrates how sensory pollutants can have cross-modal effects, possibly by misleading and distracting (Halfwerk and Slabbekoorn, 2015). Cross-modal effects of ALAN have also been shown, for instance, on the timing of singing in birds (Kempenaers et al., 2010), and the composition of chemical signals in moths (Van Geffen et al., 2015), but in general few studies have examined the effects of ALAN on the production of sexual signals (reviewed by Cronin et al., 2022). In our case, frogs might have been misled by light pollution mimicking favorable signaling conditions related to low predation risk. Higher light levels are, for example, associated with more open areas or the short time window around dusk and dawn. Under high light levels, predation by bats (the main eavesdropping predator on calling males) is expected to be lower (Cronin et al., in preparation), which allows males to take more risk by calling louder.
Combined exposure to ALAN and anthropogenic noise had interactive effects on call complexity and amplitude, which indicates that these effects were different than expected from the sum of the isolated effects of ALAN and anthropogenic noise. Generally, we saw the pattern emerge that frogs produced more conspicuous signals under combined exposure to urban light and noise compared to the expected additive effects of the single pollutants. These interactive effects can be classified as either “synergistic” or “antagonistic” depending on the direction of the expected additive effects (following Hale et al., 2017 and Halfwerk and Jerem, 2021), but it is unclear whether different perceptual or cognitive mechanisms underlie these types of interactions. Only a handful of studies have previously looked into the interactive effects of ALAN and anthropogenic noise (reviewed in Halfwerk and Jerem, 2021), including studies that have shown interaction effects on bird abundance (Wilson et al., 2021) and activity patterns in great tits (Dominoni et al., 2020b), making it difficult to draw general conclusions. Combined exposure to light and noise pollution probably affects different parts of the sensory system, and therefore tends to result in complex and often unexpected patterns for which underlying mechanisms remain in need of clarification (Halfwerk and Jerem, 2021).
Taken together, our experiment shows that exposure to urban sensory conditions, particularly the combination of urban light and noise, results in increased signal conspicuousness, which matches observed patterns in urban areas. The presence of urban sensory pollutants seems to coincide with lower predator and parasite abundances as well as lower mate attraction in this species (McMahon et al., 2017; Halfwerk et al., 2019), leading to less pronounced natural selection pressures and stronger sexual selection pressures on signaling behavior. While producing more conspicuous signals can be costly, both in terms of energy expenditure (Marler and Ryan, 1996) and costs of eavesdroppers (Ryan et al., 1982; Bernal et al., 2006; Akre et al., 2011), it seems to be an adaptive behavioral adjustment to urban conditions in the case of the túngara frog.
Persisting differences between urban and forest frogs
Regardless of the environmental manipulations we employed, differences in calling behavior between urban and forest frogs remained after a few days in our lab set-up. Some of these results are in line with previous findings, whereas others seem contradictory. Similar to Halfwerk et al. (2019), differences between call rates disappeared when changing the environment. For call complexity, on the other hand, we found that forest frogs in our lab set-up produced more complex calls compared to urban frogs, which was the opposite of our field observations and the opposite of previous findings in a translocation experiment (Halfwerk et al., 2019). One key aspect lacking in our experiment was the opportunity for focal males to interact with rival frogs. Our use of static playbacks could therefore explain the discrepancy between field observations and the previous translocation experiment, which we discuss in more detail in the next section. Another difference between urban and forest frogs in our study was that the amplitude of the forest frog calls was higher, which could partly be explained by morphological differences. Urban frogs were slightly smaller than forest frogs, a finding also reported by Halfwerk et al. (2019) and in line with what we would expect for urban ectotherms (Merckx et al., 2018). In túngara frogs, the body size is positively related to vocal sac inflation, which in turn affects call amplitude (James et al., 2021). Last, we found that urban frogs overlapped their calls more often with the rival playback than forest frogs. Signal timing is known to be important for rival interactions and mate choice (Tárano, 2015; Smit et al., 2019), and has been linked to body size in frogs (Höbel, 2011). Because of morphological constraints on call amplitude, increasing call overlap might serve as an alternative strategy for the smaller urban males.
Persisting differences between urban and forest frogs in a controlled environment point toward a role for developmental and/or genetic effects. Developmental plasticity in response to urban conditions can lead to phenotypic differences later in life (e.g., Lampe et al., 2014). Additionally, genetic differences between urban and forest populations could lead to phenotypic differentiation in sexual signals, a topic that is severely understudied (Lambert et al., 2020; Cronin et al., 2022). Increasing knowledge of the underlying mechanisms of urban and forest population divergence is necessary to advance our understanding of the potential for urban evolution and speciation (Lambert et al., 2020; Halfwerk, 2021; Cronin et al., 2022).
Importance of social dynamics
Sexual signals are often produced in a flexible way, depending on both the sensory and social environment (McGregor and Peake, 2000; Brumm and Slabbekoorn, 2005). In our study, we used different types of conspecific playbacks, allowing us to investigate the direct effects of urban sensory pollutants across different social environments. Compared to the effects of the social environment, the sensory treatments had minor direct effects on the calling behavior of the frogs. We mostly found the treatment to affect calling behavior during conspecific playbacks, and not when frogs were calling in isolation. These findings show that variation in sexual signaling becomes more pronounced when competing with rivals, during which animals generally increase their effort.
While static playbacks mimic some aspects of social environments, they provide a very standardized but simplified environment and disregard much of the complexity of interactions in naturalistic settings. The use of interactive playbacks, for example, with birds (McGregor et al., 1992; Mennill and Ratcliffe, 2000; Otter et al., 2002) and frogs (Schwartz, 1994; Bosch et al., 2000; Argo et al., 2010), provides interesting experimental tools to include social dynamics in studying animal communication.
Incorporating social dynamics into the current study could result in larger effects of sensory pollutants, since the social environment would respond both to the sensory treatment and the focal individual. Under urban sensory conditions, for instance, both males might increase their call complexity and amplitude in response to the sensory environment as well as in response to the rival, potentially leading to a positive feedback loop (Argo et al., 2010). Similarly, frogs are known to adjust their signal timing and consequently their call overlap rate depending on the social environment. In green tree frogs (Hyla cinerea), for example, males tend to actively avoid call interference with competitive rivals (Neelon and Höbel, 2019). Frogs in our study could, for example, respond stronger to each other if we had given them the opportunity to adjust the timing of their calls to each other. Especially in an interaction between urban frogs, which overlapped their calls more often, including social dynamics could lead to faster escalations when compared to an interaction between two forest males. Taking into account social dynamics in studying the effects of sensory pollutions therefore provides interesting and more realistic future avenues for studying sexual signaling and its fitness consequences in the context of urbanization.
Conclusion
In conclusion, we demonstrated minor direct effects of urban sensory pollutants on sexual signaling. We found consistent patterns suggesting that exposure to urban sensory pollutants leads to more conspicuous sexual signals, indicating that behavioral adjustments to urban sensory conditions can partially explain observed urban and forest population divergence in sexual signaling. Our study highlights not only the importance of studying the effects of sensory pollutants on communication, but also the potential for complex interactive effects between pollutants. Adopting a multi-stressor approach might therefore challenge the current thinking on how animals respond to urban sensory conditions, which can have important implications for conservation and evolution.
Data availability statement
Data collected during this study and R scripts can be obtained from DataverseNL and can be accessed via: https://doi.org/10.34894/PJAQXZ. Further inquiries can be directed to the corresponding author.
Ethics statement
This animal study was reviewed and approved by the Autoridad Nacional del Ambiente de Panamá (SE/A-47–19) and STRI (IACUC permit: 2019-0301-2022).
Author contributions
WH applied for funding. WH, JE, and JS contributed to the design of the study. JS and AC collected the data. JS, IW, and BO analyzed the audio files. JS ran the statistical analyses. JS, WH, JE, and AC wrote the manuscript. All authors contributed to manuscript revision, read, and approved the submitted version.
Funding
This study was funded by the ERC-Starting Grant—CITISENSE (802460).
Acknowledgments
We thank Alex Baugh for advice on the experiment, Matías Muñoz and Ron Lootens for their contributions to developing the recording set-up, and Rotem Zilber for assistance in making the frog enclosures and collecting frogs. We are grateful for the logistical support that the STRI, specifically Isis Ochoa and Raineldo Urriola, provided. We also thank two reviewers for providing valuable feedback on earlier versions of the manuscript.
Conflict of interest
The authors declare that the research was conducted in the absence of any commercial or financial relationships that could be construed as a potential conflict of interest.
Publisher’s note
All claims expressed in this article are solely those of the authors and do not necessarily represent those of their affiliated organizations, or those of the publisher, the editors and the reviewers. Any product that may be evaluated in this article, or claim that may be made by its manufacturer, is not guaranteed or endorsed by the publisher.
Supplementary material
The Supplementary Material for this article can be found online at: https://www.frontiersin.org/articles/10.3389/fevo.2022.934661/full#supplementary-material
Supplementary Figure 1 | Left: Power spectra of urban and forest noise stimuli, for each noise type we had four 5-min fragments. Right: Power spectrum of the artificial call, as used in playbacks.
Supplementary Figure 2 | Calling behavior of frogs of urban (orange) and forest (green) origin in different playback types (silence, rival and chorus). (A) Total number of calls per hour. (B) Number of calls per minute. (C) Number of chucks per minute. (D) Percentage of calls overlapping with the played rival call. (E) Peak-to-peak amplitude of whole call. (F) RMS of whole call. Graphs are based on model predictions (excluding the interaction between light and noise in case that was not statistically significant), except for call complexity during silence, which is based on raw data since we ran the model on binary data (chucks/no chucks). See main text and Supplementary Tables 2–6 for statistics.
References
Akre, K. L., Farris, H. E., Lea, A. M., Page, R. A., and Ryan, M. J. (2011). Signal perception in frogs and bats and the evolution of mating signals. Science 333, 751–752. doi: 10.1126/science.1205623
Araya-Salas, M., and Smith-Vidaurre, G. (2017). warbleR: An r package to streamline analysis of animal acoustic signals. Methods Ecol. Evol. 8, 184–191. doi: 10.1111/2041-210X.12624
Argo, I. V., Goutte, S., Kime, N. M., and Ryan, M. J. (2010). Calling strategies of male túngara frogs in response to dynamic playback. Behaviour 147, 65–83.
Barber, J. R., Crooks, K. R., and Fristrup, K. M. (2010). The costs of chronic noise exposure for terrestrial organisms. Trends Ecol. Evol. 25, 180–189. doi: 10.1016/j.tree.2009.08.002
Bates, D., Maechler, M., Bolker, B., and Walker, S. (2015). Fitting linear mixed-effects models using lme4. J. Stat. Softw. 67, 1–48. doi: 10.18637/jss.v067.i01
Bernal, X. E., Rand, A. S., and Ryan, M. J. (2006). Acoustic preferences and localization performance of blood-sucking flies (Corethrella Coquillett) to t??ngara frog calls. Behav. Ecol. 17, 709–715. doi: 10.1093/beheco/arl003
Bosch, J., Rand, A. S., and Ryan, M. J. (2000). Acoustic competition in Physalaemus pustulosus, a differential response to calls of relative frequency. Ethology 106, 865–871. doi: 10.1046/j.1439-0310.2000.00587.x
Brooks, M. E., Kristensen, K., van Benthem, K. J., Magnusson, A., Berg, C. W., Nielsen, A., et al. (2017). glmmTMB balances speed and flexibility among packages for zero-inflated generalized linear mixed modeling. R J. 9:378. doi: 10.32614/RJ-2017-066
Brumm, H., and Slabbekoorn, H. (2005). Acoustic communication in noise. Adv. Study Behav. 35, 151–209. doi: 10.1016/S0065-3454(05)35004-2
Cheng, J., Karambelkar, B., and Xie, Y. (2021). leaflet: Create Interactive Web Maps with the JavaScript “Leaflet Library.
Cronin, A. D., Smit, J. A. H., Muñoz, M. I., Poirier, A., Moran, P. A., Jerem, P., et al. (2022). A comprehensive overview of the effects of urbanisation on sexual selection and sexual traits. Biol. Rev. 97, 1325–1345. doi: 10.1111/brv.12845
Derryberry, E. P., Phillips, J. N., Derryberry, G. E., Blum, M. J., and Luther, D. (2020). Singing in a silent spring: Birds respond to a half-century soundscape reversion during the COVID-19 shutdown. Science 370, 575–579. doi: 10.1126/SCIENCE.ABD5777
Dominoni, D. M., Halfwerk, W., Baird, E., Buxton, R. T., Fernández-Juricic, E., Fristrup, K. M., et al. (2020a). Why conservation biology can benefit from sensory ecology. Nat. Ecol. Evol. 4, 502–511. doi: 10.1038/s41559-020-1135-4
Dominoni, D., Smit, J. A. H., Visser, M. E., and Halfwerk, W. (2020b). Multisensory pollution: Artificial light at night and anthropogenic noise have interactive effects on activity patterns of great tits (Parus major). Environ. Pollut. 256:113314. doi: 10.1016/j.envpol.2019.113314
Ferraro, D. M., Le, M.-L. T., and Francis, C. D. (2020). Combined effect of anthropogenic noise and artificial night lighting negatively affect Western Bluebird chick development. Condor 122, 1–12. doi: 10.1093/condor/duaa037
Hale, R., Piggott, J. J., and Swearer, S. E. (2017). Describing and understanding behavioral responses to multiple stressors and multiple stimuli. Ecol. Evol. 7, 38–47. doi: 10.1002/ece3.2609
Halfwerk, W. (2021). How should we study urban speciation? Front. Ecol. Evol. 8:573545. doi: 10.3389/fevo.2020.573545
Halfwerk, W., and Jerem, P. (2021). A systematic review of research investigating the combined ecological impact of anthropogenic noise and artificial light at night. Front. Ecol. Evol. 9:765950. doi: 10.3389/fevo.2021.765950
Halfwerk, W., and Slabbekoorn, H. (2009). A behavioural mechanism explaining noise-dependent frequency use in urban birdsong. Anim. Behav. 78, 1301–1307. doi: 10.1016/j.anbehav.2009.09.015
Halfwerk, W., and Slabbekoorn, H. (2015). Pollution going multimodal: The complex impact of the human-altered sensory environment on animal perception and performance. Biol. Lett. 11:20141051. doi: 10.1098/rsbl.2014.1051
Halfwerk, W., Blaas, M., Kramer, L., Hijner, N., Trillo, P. A., Bernal, X. E., et al. (2019). Adaptive changes in sexual signalling in response to urbanization. Nat. Ecol. Evol. 3, 374–380. doi: 10.1038/s41559-018-0751-8
Halfwerk, W., Lea, A. M., Guerra, M. A., Page, R. A., and Ryan, M. J. (2016). Vocal responses to noise reveal the presence of the Lombard effect in a frog. Behav. Ecol. 27, 669–676. doi: 10.1093/beheco/arv204
Hartig, F. (2021). DHARMa: Residual diagnostics for hierarchical (multi-level/mixed) regression models. R package version 0.3.4. ***q.
Höbel, G. (2011). Variation in signal timing behavior: Implications for male attractiveness and sexual selection. Behav. Ecol. Sociobiol. 65, 1283–1294. doi: 10.1007/s00265-011-1142-1
James, L. S., Halfwerk, W., Hunter, K. L., Page, R. A., Taylor, R. C., Wilson, P. S., et al. (2021). Covariation among multimodal components in the courtship display of the túngara frog. J. Exp. Biol. 224, 1–9. doi: 10.1242/jeb.241661
Kempenaers, B., Borgström, P., Loës, P., Schlicht, E., and Valcu, M. (2010). Artificial night lighting affects dawn song, extra-pair siring success, and lay date in songbirds. Curr. Biol. 20, 1735–1739. doi: 10.1016/j.cub.2010.08.028
Kyba, C. C. M., Kuester, T., De Miguel, A. S., Baugh, K., Jechow, A., Hölker, F., et al. (2017). Artificially lit surface of Earth at night increasing in radiance and extent. Sci. Adv. 3, 1–9. doi: 10.1126/sciadv.1701528
Lambert, M. R., Brans, K. I., Des Roches, S., Donihue, C. M., and Diamond, S. E. (2020). Adaptive evolution in cities: Progress and misconceptions. Trends Ecol. Evol. 36, 239–257. doi: 10.1016/j.tree.2020.11.002
Lampe, U., Reinhold, K., and Schmoll, T. (2014). How grasshoppers respond to road noise: Developmental plasticity and population differentiation in acoustic signalling. Funct. Ecol. 28, 660–668. doi: 10.1111/1365-2435.12215
Marler, C. A., and Ryan, M. J. (1996). Energetic constraints and steroid hormone correlates of male calling behaviour in the tungara frog. J. Zool. Lond. 240, 397–409.
McGregor, P. K., and Peake, T. M. (2000). Communication networks: Social environments for receiving and signalling behaviour. Acta Ethol. 2, 71–81. doi: 10.1007/s102110000015
McGregor, P., Dabelsteen, T., Shepherd, M., and Pedersen, S. (1992). The signal value of matched singing in great tits: Evidence from interactive playback experiments. Anim. Behav. 43, 987–998. doi: 10.1016/0003-3472(92)90012-X
McKinney, M. L. (2006). Urbanization as a major cause of biotic homogenization. Biol. Conserv. 127, 247–260. doi: 10.1016/j.biocon.2005.09.005
McKinney, M. L., and Lockwood, J. L. (1999). Biotic homogenization: A few winners replacing many losers in the next mass extinction. Trends Ecol. Evol. 14, 450–453. doi: 10.1016/S0169-5347(99)01679-1
McMahon, T. A., Rohr, J. R., and Bernal, X. E. (2017). Light and noise pollution interact to disrupt interspecific interactions. Ecology 98, 1290–1299. doi: 10.1002/ecy.1770
Mennill, D. J., and Ratcliffe, L. M. (2000). A field test of ‘syrinx’ sound analysis software in interactive playback. Bioacoustics 11, 77–86. doi: 10.1080/09524622.2000.9753451
Merckx, T., Souffreau, C., Kaiser, A., Baardsen, L. F., Backeljau, T., Bonte, D., et al. (2018). Body-size shifts in aquatic and terrestrial urban communities. Nature 558, 113–116. doi: 10.1038/s41586-018-0140-0
Miller, P. J. O., Biassoni, N., Samuels, A., and Tyack, P. L. (2000). Whale songs lengthen in response to sonar. Nature 405, 903–903. doi: 10.1038/35016148
Neelon, D. P., and Höbel, G. (2019). Staying ahead of the game—Plasticity in chorusing behavior allows males to remain attractive in different social environments. Behav. Ecol. Sociobiol. 73:124.
Orci, K. M., Petróczki, K., and Barta, Z. (2016). Instantaneous song modification in response to fluctuating traffic noise in the tree cricket Oecanthus pellucens. Anim. Behav. 112, 187–194. doi: 10.1016/j.anbehav.2015.12.008
Otter, K. A., Ratcliffe, L., Njegovan, M., and Fotheringham, J. (2002). Importance of frequency and temporal song matching in black-capped chickadees: Evidence from interactive playback. Ethology 108, 181–191. doi: 10.1046/j.1439-0310.2002.00764.x
Partan, S., and Marler, P. (1999). Communication goes multimodal. Science 283, 1272–1273. doi: 10.1126/science.283.5406.1272
Piggott, J. J., Townsend, C. R., and Matthaei, C. D. (2015). Reconceptualizing synergism and antagonism among multiple stressors. Ecol. Evol. 5, 1538–1547. doi: 10.1002/ece3.1465
R Core Devlopment Team (2019). A language and environment for statistical computing. R foundation for statistical computing. Vienna: R Foundation for Statistical Computing.
Ryan, M. J. (1980). Female mate choice in a Neotropical frog. Science 209, 523–525. doi: 10.1126/science.209.4455.523
Ryan, M. J., Tuttle, M. D., and Rand, A. S. (1982). Bat predation and sexual advertisement in a Neotropical anuran. Am. Nat. 199, 136–139.
Santangelo, J. S., Ness, R. W., Cohan, B., Fitzpatrick, C. R., Innes, S. G., Koch, S., et al. (2022). Global urban environmental change drives adaptation in white clover. Science 375, 1275–1281. doi: 10.1126/science.abk0989
Schwartz, J. J. (1994). Male advertisement and female choice in frogs: Recent findings and new approaches to the study of communication in a dynamic acoustic environment. Integr. Comp. Biol. 34, 616–624. doi: 10.1093/icb/34.6.616
Slabbekoorn, H., and Peet, M. (2003). Birds sing at a higher pitch in urban noise. Nature 424, 267–267. doi: 10.1038/424267a
Smit, J. A. H., Loning, H., Ryan, M. J., and Halfwerk, W. (2019). Environmental constraints on size-dependent signaling affects mating and rival interactions. Behav. Ecol. 30, 724–732. doi: 10.1093/beheco/arz009
Sol, D., Lapiedra, O., and González-Lagos, C. (2013). Behavioural adjustments for a life in the city. Anim. Behav. 85, 1101–1112. doi: 10.1016/j.anbehav.2013.01.023
Sueur, J., Aubin, T., and Simonis, C. (2008). seewave: A free modular tool for sound analysis and synthesis. Bioacoustics 18, 213–226.
Tárano, Z. (2015). Choosing a mate in a cocktail party-like situation: The effect of call complexity and call timing between two rival males on female mating preferences in the túngara frog physalaemus pustulosus. Ethology 121, 749–759. doi: 10.1111/eth.12387
Taylor, R. C., and Ryan, M. J. (2013). Interactions of multisensory components perceptually rescue túngara frog mating signals. Science 341, 273–274. doi: 10.1126/science.1237113
United Nations, Department of Economic and Social Affairs, Population Division (2018). The World’s cities in 2018 – data booklet (ST/ESA/SER.A/417). New York, NY: United Nations, 34.
Van Geffen, K. G., Groot, A. T., Van Grunsven, R. H. A., Donners, M., Berendse, F., and Veenendaal, E. M. (2015). Artificial night lighting disrupts sex pheromone in a noctuid moth. Ecol. Entomol. 40, 401–408. doi: 10.1111/een.12202
Votsi, N. E. P., Kallimanis, A. S., and Pantis, I. D. (2017). An environmental index of noise and light pollution at EU by spatial correlation of quiet and unlit areas. Environ. Pollut. 221, 459–469. doi: 10.1016/j.envpol.2016.12.015
Wilson, A. A., Ditmer, M. A., Barber, J. R., Carter, N. H., Miller, E. T., Tyrrell, L. P., et al. (2021). Artificial night light and anthropogenic noise interact to influence bird abundance over a continental scale. Glob. Change Biol. 27, 3987–4004. doi: 10.1111/gcb.15663
Keywords: communication, sexual signals, urbanization, artificial light at night (ALAN), anthropogenic noise, multisensory pollution
Citation: Smit JAH, Cronin AD, van der Wiel I, Oteman B, Ellers J and Halfwerk W (2022) Interactive and independent effects of light and noise pollution on sexual signaling in frogs. Front. Ecol. Evol. 10:934661. doi: 10.3389/fevo.2022.934661
Received: 02 May 2022; Accepted: 15 July 2022;
Published: 15 August 2022.
Edited by:
Melissa Lin Grunst, Université de la Rochelle, FranceReviewed by:
Jean Secondi, Université d’Angers, FranceCristiano Bertolucci, University of Ferrara, Italy
Copyright © 2022 Smit, Cronin, van der Wiel, Oteman, Ellers and Halfwerk. This is an open-access article distributed under the terms of the Creative Commons Attribution License (CC BY). The use, distribution or reproduction in other forums is permitted, provided the original author(s) and the copyright owner(s) are credited and that the original publication in this journal is cited, in accordance with accepted academic practice. No use, distribution or reproduction is permitted which does not comply with these terms.
*Correspondence: Judith A. H. Smit, ai5hLmguc21pdEB2dS5ubA==