The vertical light-gradient and its potential impact on animal distribution and behavior
- The Lund Vision Group, Department of Biology, Lund University, Lund, Sweden
The visual environment provides vital cues allowing animals to assess habitat quality, weather conditions or measure time of day. Together with other sensory cues and physiological conditions, the visual environment sets behavioral states that make the animal more prone to engage in some behaviors, and less in others. This master-control of behavior serves a fundamental and essential role in determining the distribution and behavior of all animals. Although it is obvious that visual information contains vital input for setting behavioral states, the precise nature of these visual cues remains unknown. Here we use a recently described method to quantify the distribution of light reaching animals’ eyes in different environments. The method records the vertical gradient (as a function of elevation angle) of intensity, spatial structure and spectral balance. Comparison of measurements from different types of environments, weather conditions, times of day, and seasons reveal that these aspects can be readily discriminated from one another. The vertical gradients of radiance, spatial structure (contrast) and color are thus reliable indicators that are likely to have a strong impact on animal behavior and spatial distribution.
Introduction
As humans, it is easy to relate to the impact that visual environments have on our mood and its profound effects on what we desire to do. We want to remain in beautiful environments but cannot wait to get away from places we find visually displeasing. Our preferred activities clearly differ between sunny and overcast days, as well as between the intense mid-day sun, the warm light of the setting sun or the blueish light at late dusk. These things feel almost too obvious and natural to realize that it is in fact a superior behavioral control at play.
All animals need to select habitats and continuously pick the right activities from their behavioral repertoire. These choices differ between diurnal, crepuscular, and nocturnal species and depend on their size, food source, main threats, and numerous other factors. The species-specific choice of where to be and what to do under different times of day and different environmental conditions is one of the fundamental pillars shaping ecological systems (Davies et al., 2012). Sensory information from the environment together with internal physiological conditions (hunger, fatigue, etc.) provide the information that sets behavioral states, which in turn determines the activities that each species is prone to engage in at any one time (Gurarie et al., 2016; Mahoney and Young, 2017; McCormick et al., 2020). If an environment changes, it will alter the input to behavioral states, and potentially change the activities or whereabouts in a species-specific manner.
The external information used to set behavioral states is obviously multimodal. Temperature and olfactory cues are undeniably important (Abram et al., 2016; Breugel et al., 2018), but vision arguably provides the largest amount of information for a vast number of species. Animals use vision to find suitable habitats, position themselves optimally in the habitat and select the behaviors that make best sense in the current place, time, and environmental condition. Especially for assessing the type of environment and determining the time of day and the current weather conditions, vision provides rich and reliable information.
However, it is not immediately obvious which aspects of visual information provide input to behavioral states. The position and movement of visible structures are used for orientation behaviors and active interactions with the environment, and they are often considered to be the main or only relevant type of visual information (Carandini et al., 2005; Sanes and Zipursky, 2010; Clark and Demb, 2016). But these aspects of visual information do not say much about the type of environment, time of day, season, or weather conditions. However, the visual structures used for active vision are seen against a background, which is often considered as redundant visual information. To reduce the amount of information and economize on both time and cost of processing, early visual processing in the nervous system is believed to remove much of the visual background (Wandell, 1995; Olshausen and Field, 2004). The absolute intensity and spectral balance, or large gradients of these across the visual field, are typically considered as mere disturbances to the visual information. But the visual background is far from superfluous. It specifically contains information that can be used to read the type of environment and the current conditions.
Until recently, there has been a lack of methods for quantitatively describing the visual background. With the environmental light field (ELF) method (Nilsson and Smolka, 2021), there is now a tool by which visual environments, in particular the general background, can be comprehensively quantified (Figure 1). As a function of elevation angle, the ELF method measures the absolute intensity (photon radiance), the intensity variations (contrasts), and the spectral balance. In principle, the ELF method describes the vertical gradients of intensity, visual structure and spectral balance in the environment. These are the features that remain when scene-specific information is removed. The vertical light gradients depend on the type of environment, its quality, and the current conditions (time of day, weather, season, etc.) (Nilsson and Smolka, 2021). This is exactly the information animals need for assessing their environment and selecting relevant behaviors. If we can quantify the visual background in different habitats and how it varies with time of day and current environmental conditions, we can tap into the information that animals most likely use for deciding where to be and what to do.
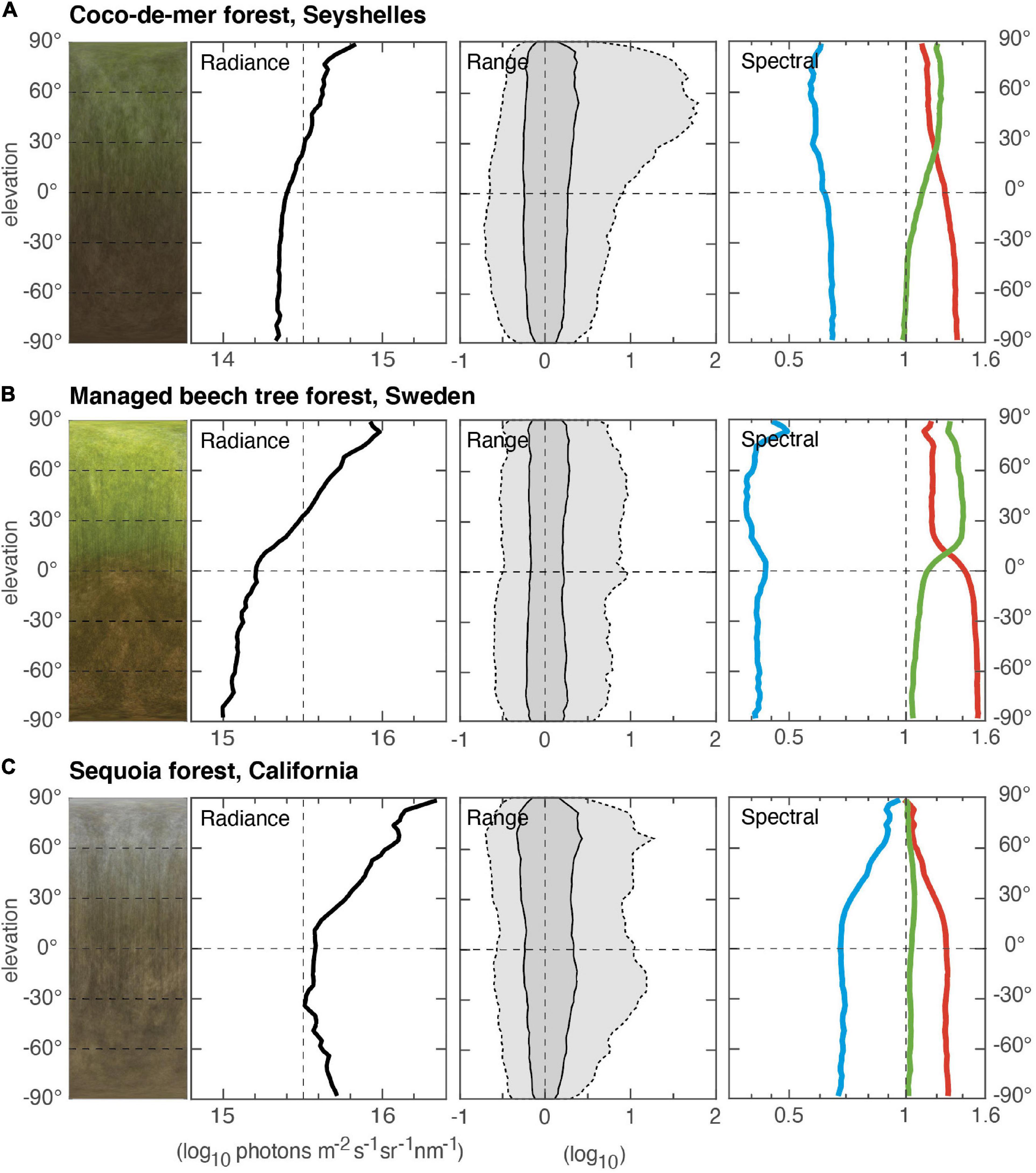
Figure 1. Vertical light gradients in three forest environments recorded with the ELF method (Nilsson and Smolka, 2021). For each environment, radiance data sampled from many different positions (scenes) in the environment is plotted as a function of elevation angle. An average image (compressed in azimuth) from the contributing scenes (180° by 180°) is shown to the left, followed by panels showing the intensity (radiance) on an absolute log-scale, the intensity range on a relative log-scale (dark gray, 50% of all intensities; light gray, 95% of all intensities), and to the right, the contribution of red, green, and blue light plotted on a relative log scale. (A) A wet tropical forest at the center of Praslin Island, the Seychelles (measurement based on 51 scenes, 29 December 2017, start 15:16, duration 51 min). The dense forest is dominated by coco-de-mer and other palm trees with leaves at multiple levels. (B) A managed beech tree forest, close to Maglehem, southern Sweden (measurement based on 13 scenes, 14 May 2015, start 13:55, duration 4 min). Smaller trees and bushes are largely cleared, leaving largely open space under the dense but translucent canopy. (C) Dense sequoia forest, Sierra Nevada, California, United States (measurement based on 31 scenes, 17 April 2015, start 12:02, duration 42 min). All environments, (A–C), were measured on clear, sunny days when the sun was at least 45° above the horizon.
Here, we compare the vertical light gradients from different environments, seasons, times of day, and weather conditions. Based on these comparisons, we characterize the visual background information to identify aspects of the information that animals may use for assessing the environment and setting behavioral states. We specifically test whether different landscapes and their current condition can be reliably determined by information extracted from the vertical gradients of intensity, spatial structure (image contrasts), and spectral balance.
Materials and methods
Vertical light gradients were measured from raw images taken with digital cameras (Nikon D810 or D850) equipped with a 180° lens generating circular images (Sigma 8 mm F3.5, EX DG Fisheye) (see Nilsson and Smolka, 2021 for details). The camera was oriented horizontally, such that the image covered all elevation angles from straight down (−90°) to straight up (+90°). To extend the dynamic range, daylight and twilight scenes were captured using bracketing with three different exposures (automatic exposure ±3 EV steps). Night-time scenes with only starlight were captured with the camera on a tripod and a single exposure of 8 min, followed by another 8 min exposure with the shutter closed (to quantify and subtract sensor-specific dark-noise). For comparisons of different environments and different weather conditions, we sampled some 10–30 different scenes within each environment to produce “average” environments with a minimum of scene-specific bias. For comparisons over time (24 h), we used a single scene with the camera mounted on a tripod. Under-water measurements were made using a custom-built under-water housing with a hemispherical dome port centered around the anterior nodal point of the fisheye lens (to obtain identical angular mapping in terrestrial and aquatic environments).
Images were analyzed using open-source software described in Nilsson and Smolka (2021). This software uses radiometric calibration files to compute the median radiance, together with the 50–95% range of data, as a function of elevation angle. Calculations were made separately for the red, green and blue channels. Calculations were performed at the full resolution of the camera, but the output was binned for 3° bands of elevation (and 180° azimuth), effectively generating a resolution of 3° for elevation angles. Calibrations were made in photon flux per nm wavelength, providing comparable values for white-light and individual spectral channels. Radiances are given as log10 values to provide manageable numbers over the full range of natural intensities from night to day. Using median rather than mean values, returns “typical” radiances for each elevation angle, where any strong light sources are represented mainly as peaks on the upper bound of the 95% range. The computed data was plotted in three different panels showing the vertical gradients of (1) median radiance (intensity) for white-light, (2) range of intensities (50–95% data range, normalized to the median radiance) and (3) the relative radiances (relative color) of red, green and blue light (normalized to the median white-light radiance).
For spectral sensitivity of the red, green and blue channels of Nikon D810, see supplementary information to Nilsson and Smolka (2021). The spectral sensitivities of Nikon D850, used for some of our measurements, is very similar and displayed in Supplementary Figure S1 for this manuscript. For both camera models, the median wavelengths of the spectral channels are: blue 465; green 530; and red 610 nm. As computationally demonstrated in the supplementary information of Nilsson and Smolka (2021), the spectral balance curves for environment measurements are not very sensitive even to larger changes in the spectral sensitivities of the camera, implying that other radiometrically calibrated models or makes of RGB cameras (with color image sensors having red, green, and blue pixels) would provide close to identical measurements. The reason for this robustness is that the spectral curves summed over 180° azimuth bands are always very broad in natural environments.
To give a visual impression of the vertical gradients, the software also generates an equirectangular image projection of the scene or average environment (horizontally compressed in our figures).
Results
For more than a decade, we have used the ELF method to measure vertical light gradients in a large range of natural environments from across the world. The measurements have been performed at different times of day, weather conditions and seasons. From this large collection of data, we here use selected typical measurements to identify the features of vertical light gradients that carry information about the type of environment, time and weather.
In Figure 1 we compare three different forest environments (a dense tropical forest dominated by coco-de-mere and other palm tree species, a managed beech tree forest, and a sequoia forest). The vertical light gradients differ significantly and in many ways between the different forests. The radiance gradient (in the text referred to as intensity gradient) has a steeper slope (more light from above than from below) in the managed forest, with a dense canopy but little undergrowth, compared to the tropical forest with multiple layers of vegetation. In the sequoia forest, the intensity gradient has an obvious C-shape that we have seen also in other coniferous forests with cone-shaped trees (spruce). By a large margin, the tropical forest is the darkest (note that the intensity values are logarithmic, and a difference of one represents 10 times difference in intensity). Other differences are seen in the range plots, where the glossy but opaque leaves of the tropical forest generate a characteristic P-shape caused by high intensity spots of specular reflections centered around the direction of the sun. This phenomenon is not seen in the beech forest with its more translucent leaves. The color gradients are also markedly different between all three types of forest, especially the relative amount of blue light and the inflection of the red and green curves. These spectral differences depend heavily on the fractions of light reflected or transmitted through the foliage.
Semi-open environments (Figure 2) differ from dense forests by significant amounts of direct skylight/sunlight or diffuse light from clouds. This causes a characteristic shift in the spectral balance (color) at the skyline. On clear days (at which all three data sets in Figure 2 were acquired), the sky is always dominated by blue light, with less green and even less red. Below the skyline, this order is reversed because vegetation and other organic material are often poor at reflecting blue light but efficient in reflecting red. Fresh green leaves favor green, whereas red dominates in light reflected from dirt, leaf litter and non-photosynthesizing parts of plants. The intensity and range gradients depend much on the type of vegetation, how tall it is, how far apart trees and bushes are and how dry or lush the vegetation is. The color of the ground is also a matter of great variation. An obvious example (Figure 2) is the Australian bushland environment, which has a bright ground dominated by dry mineral dirt, whereas the environments from Sweden and Cape Verde have a much darker ground dominated by organic matter.
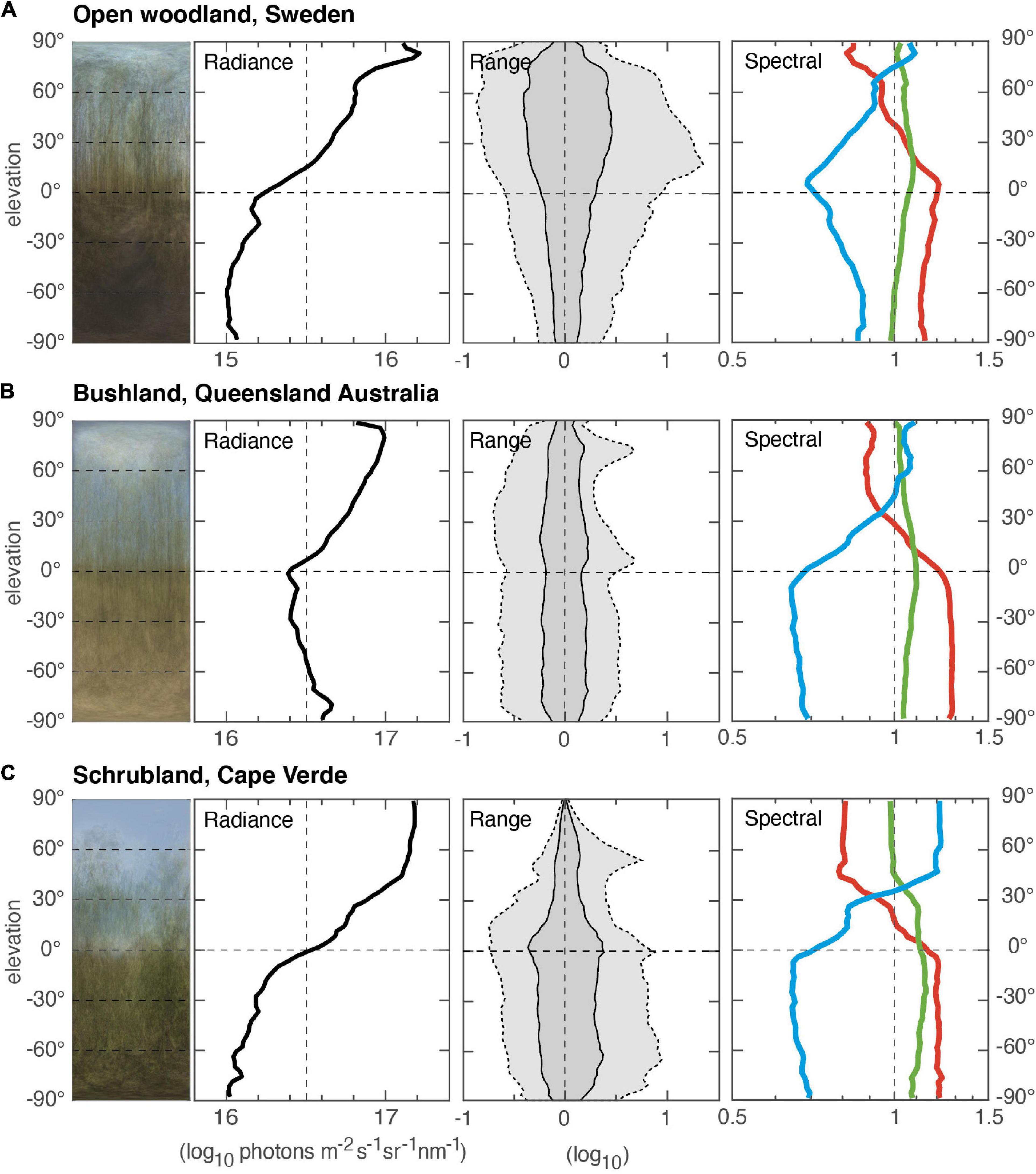
Figure 2. Three different semi-open environments. (A) Open woodland, close to Kivik, southern Sweden (measurement based on 20 scenes, 29 March 2020, start 15:12, duration 5 min). (B) Dry bushland at Mt. Garnet, Queensland, Australia (measurement based on 26 scenes, 3 December 2012, start 10:03, duration 14 min). Here, eucalypt trees are separated by dry grass. (C) Shrubland at Serra Malagueta National Park, Santiago, Cape Verde (measurement based on 14 scenes, 6 December 2016, start 15:08, duration 6 min). In this lush mountainous terrain, low trees are mixed with bushes. All environments, (A–C), were measured on clear, sunny days when the sun was at least 45° above the horizon.
In fully open environments, where little or no vegetation lifts the skyline above the horizon, the vertical light gradients are split sharply by the horizon (Figure 3). On clear days, the sky intensity gradient is revealed in full, with the highest intensities just above the horizon. If the ground reflects much light, as in the Californian lakebed, intensities below the horizon may significantly exceed those of the sky (note that direct sunlight does not contribute much to sky median intensity, but it does illuminate the ground). The elevation of the sun is visible mainly as an intensity peak on the 95% data range of the range graphs. A bare ground also leads to narrow range profiles, whereas vegetated ground, as in a meadow, generates a broader range and also much lower intensity compared to the sky. The color gradients in the sky depend on solar elevation and air humidity, but below the horizon they reveal the color of the ground.
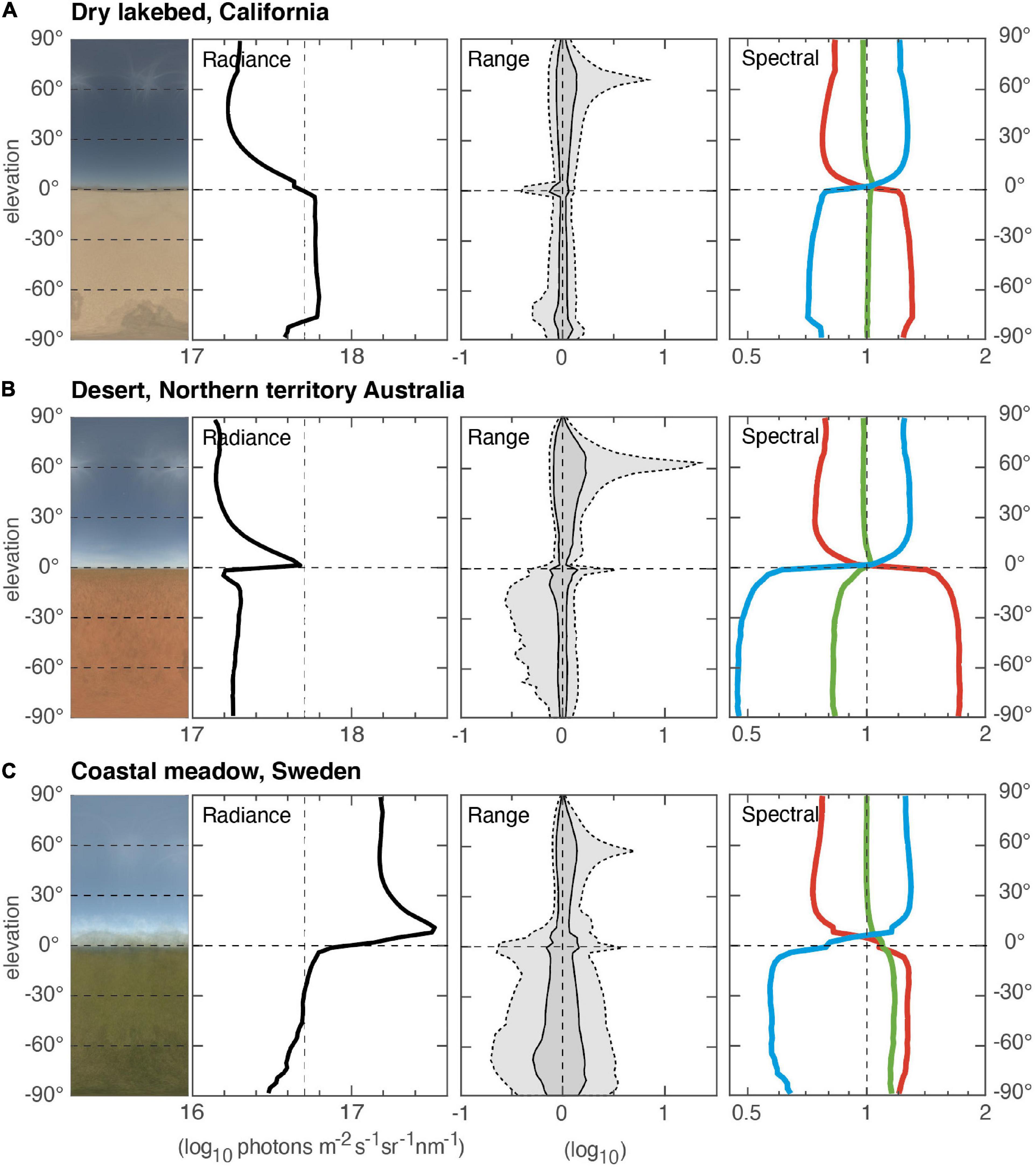
Figure 3. Open environments of different types. (A) Dry lakebed near Lancaster, California, United States (measurement based on 14 scenes, 13 April 2015, start 11:25, duration 7 min). (B) Desert with red dirt near Tilmouth Well, Northern Territory, Australia (measurement based on 23 scenes, 6 April 2019, start 12:37, duration 6 min). (C) Coastal meadow at Hovs Hallar, southern Sweden (measurement based on 22 scenes, 30 May 2020, start 16:17, duration 5 min). All environments, (A–C), were measured on clear, sunny days when the sun was at least 45° above the horizon.
Aquatic visual environments are very different from terrestrial ones (MacIver et al., 2017; Nilsson, 2017), and this is true also for the vertical light gradients (Figure 4). The horizon is typically much less pronounced, but because light is refracted as it enters water, the 180° terrestrial hemisphere is compressed to a cone of 97°, called Snell’s window (see Cronin et al., 2014). In shallow pelagic environments, Snell’s window makes a sharp intensity shift at an elevation angle of 48.5°, although movements of the water surface may blur this shift. In shallow epibenthic environments the lit seafloor makes Snell’s window much less obvious. With increasing depth, Snell’s window is replaced by a smooth vertical gradient, as exemplified in the Cape Cod environment of Figure 4. In general, aquatic environments display much narrower range gradients because light scattering reduces contrasts in water (except for high-amplitude light-flicker at the edge of Snell’s window). The spectral gradients may be very complex in shallow epibenthic habitats, but simpler in pelagic and deeper habitats. Different types of water preferably transmit specific wavelength regions, and this makes the vertical color gradients depend strongly on depth.
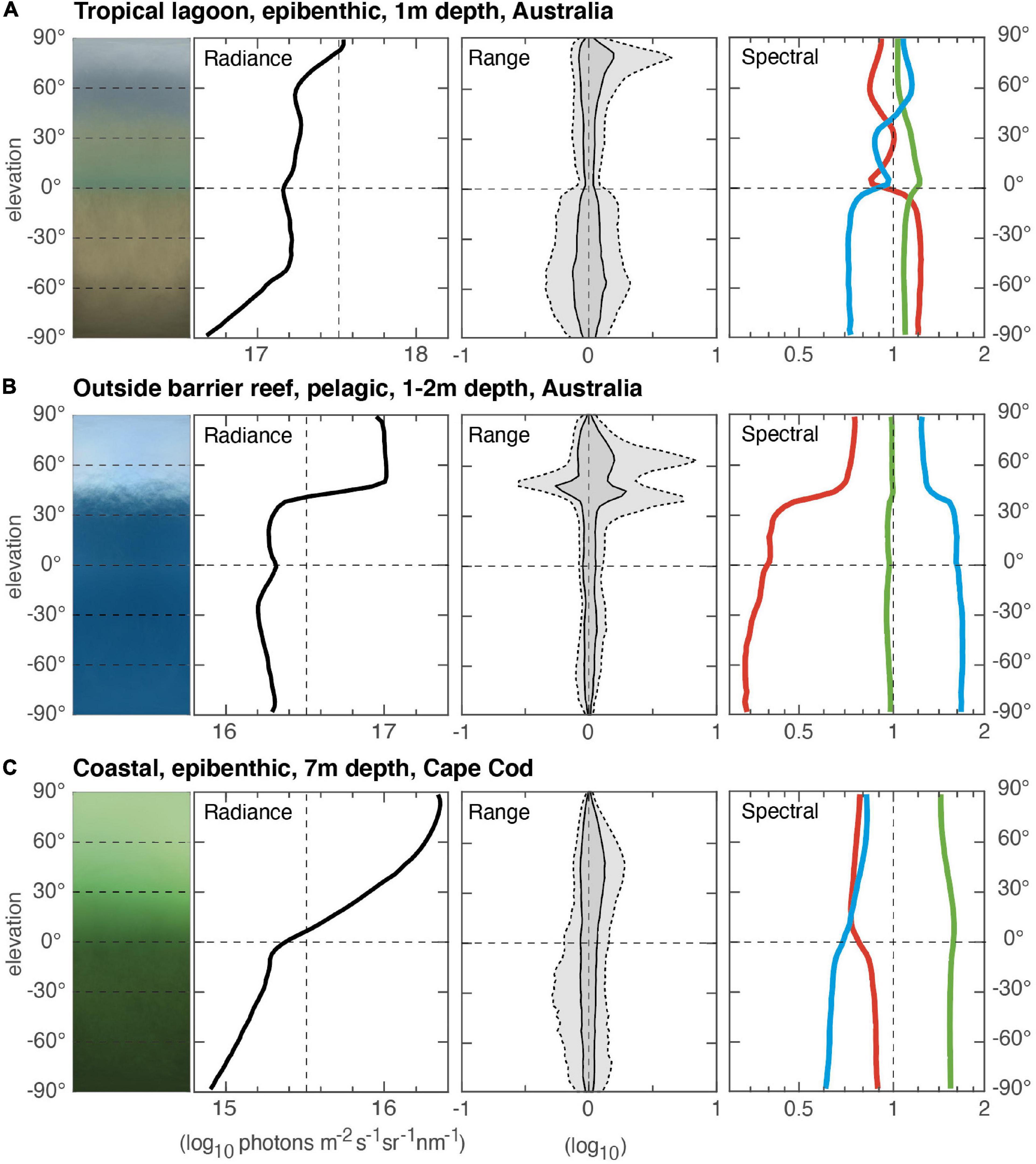
Figure 4. Shallow aquatic environments. (A) A tropical lagoon at Lizard Island, Queensland, Australia (measurement based on 40 scenes, 7 September 2015, start 12:17, duration 12 min). The environment was sampled at 1 m depth close to the bottom in the shallow lagoon. (B) Outside the barrier reef, at 1–2 m from the surface, over deep water with no visible seafloor, Queensland coast, Australia (measurement based on 27 scenes, 4 September 2015, start 14:31, duration 11 min). (C) Coastal epibenthic environment at 7 m depth outside Cape Cod, Massachusetts, United States (measurement based on 24 scenes, 19 October 2016, start 15:52, duration 8 min). All environments, (A–C), were measured on clear, sunny days. In panels (A,B), the sun was at least 45° above the horizon, and in panel (C), 20°C above the horizon.
In all naturally lit environments, the time of day causes major changes to the vertical light gradients. Figure 5 illustrates a single scene from an Australian desert monitored round the clock under a constantly clear sky. Apart from the obvious intensity shifts covering a total of eight orders of magnitude (100 million times difference in intensity), the vertical light gradients in the sky change slope and spectral balance depending on solar elevation. The large intensity shifts occur at solar elevations between −20° (below the horizon) and 10° (above the horizon). For solar elevations above about 10°, the slope of the vertical intensity gradient provides a potential time signal in addition to the solar position. During dusk and dawn (solar elevations between 0° and −20°) there are also changes in the vertical intensity gradient in the sky, as well as a reliable change in the spectral balance between red, green, and blue light. When the sun’s elevation is between −5° and −10° (below the horizon), there is a strong dominance of blue light (the blue hour). Under overcast conditions, changes in the vertical intensity gradient are largely lost, but the changes in spectral balance remain. When the sun is 18° or more below the horizon (astronomical night), and there is no moonlight, stars and airglow are the main light sources, causing a typical dominance of red light (Johnsen, 2012; Jechow et al., 2019; Warrant et al., 2020). During astronomical night, there is no time signal apart from the movement of the celestial hemisphere.
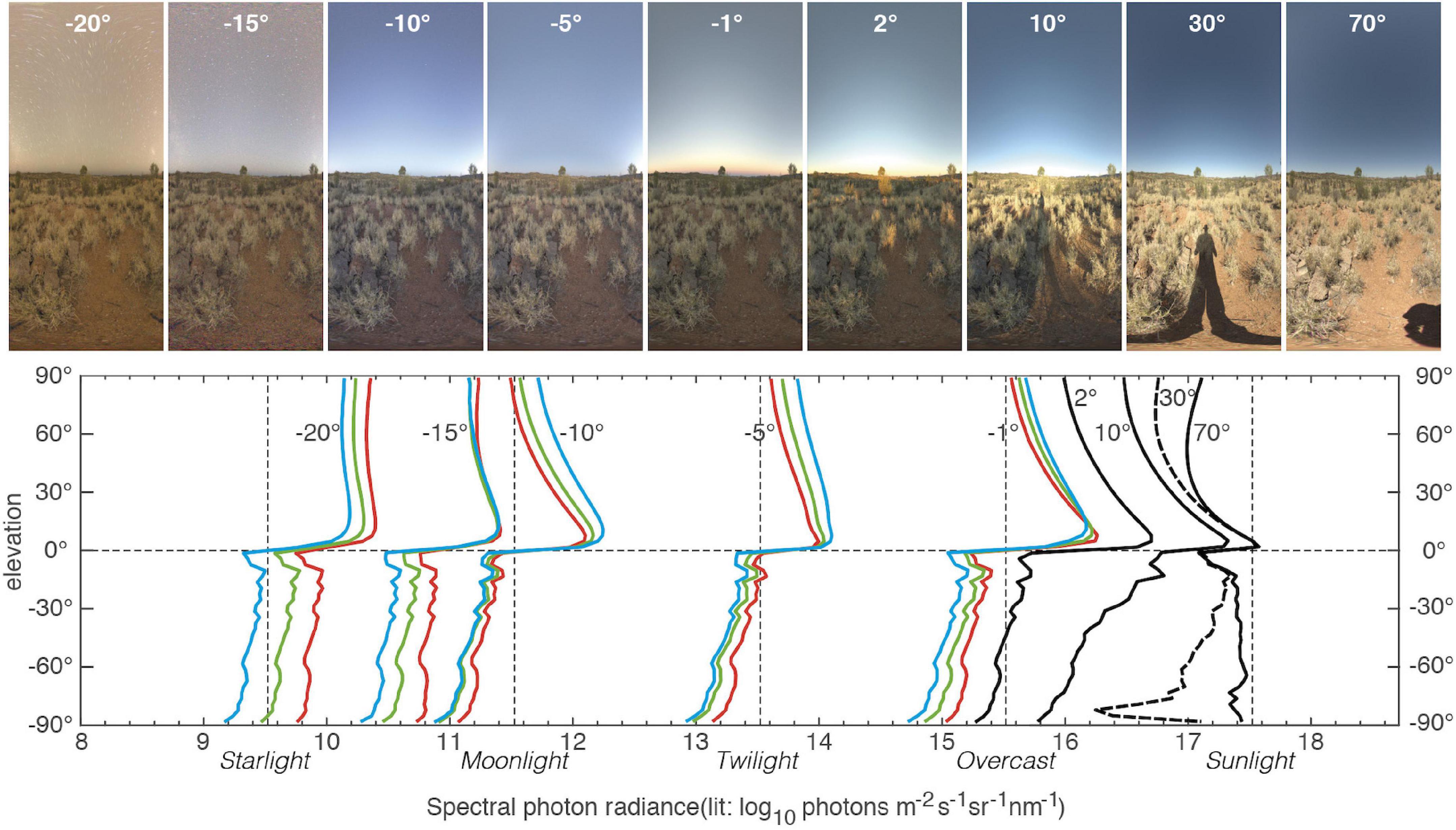
Figure 5. Vertical light gradients at a single scene in arid country south of Alice Springs, Northern Territory, Australia, sampled at different times, both day and night (17–18 October 2017). Images of the scene are shown above for each of the solar elevations plotted in the graph below. Absolute radiances are plotted with the sun at different elevation angles from –20° (below the horizon) at night to 70° above the horizon during the day. Night-time measurements are plotted with separate curves for red, green, and blue to show the shifts in spectral balance. To avoid clutter, daytime measurements are not divided into separate curves for different spectral bands (Full daytime graphs are provided in Supplementary Figure S3). The curve for 30° is shown as a dashed line for clarity. Measurements were taken at new moon to avoid influence of moonlight at night. The location was selected to be entirely free of detectable light pollution. All displayed measurements are of the antisolar hemisphere. The solar hemisphere was also recorded and found to be similar, but at daytime, angles ±20° of the sun’s elevation are noticeably brighter. The labels, Starlight, Moonlight, Twilight, Overcast, and Sunlight are not related to the displayed data, but provided by the analysis software as guides for typical radiances. All measurements provided in the figure were recorded under completely clear skies.
In Figures 1–5 measurements have been made under clear skies. Under overcast conditions, all these measurements would have been different. The changes in vertical light gradients from clear sky to overcast (100% cloud cover) are major and similar in terrestrial and shallow water environments, although dense forests are less affected. In open or semi-open environments during the day, overcast conditions cause a massive reduction of intensity below the skyline (Figure 6), with no corresponding drop of sky radiance. This is caused by the loss of direct sunlight, which under clear skies makes up about 80% of the irradiance (the blue sky making up the remaining 20%). A heavily overcast sky may be darker than a blue sky, but a lightly overcast sky may even be brighter. Typical for overcast conditions is also that the range of intensities is dramatically reduced. A third consequence of overcast skies is that the vertical color gradients change to almost neutral above the skyline. The relative contribution of blue light decreases above the skyline but increases below the skyline. Overcast skies thus cause major changes in vertical light gradients. Partial cloud cover results in intermediate changes, which are closer to clear conditions if the sun or moon is not occluded.
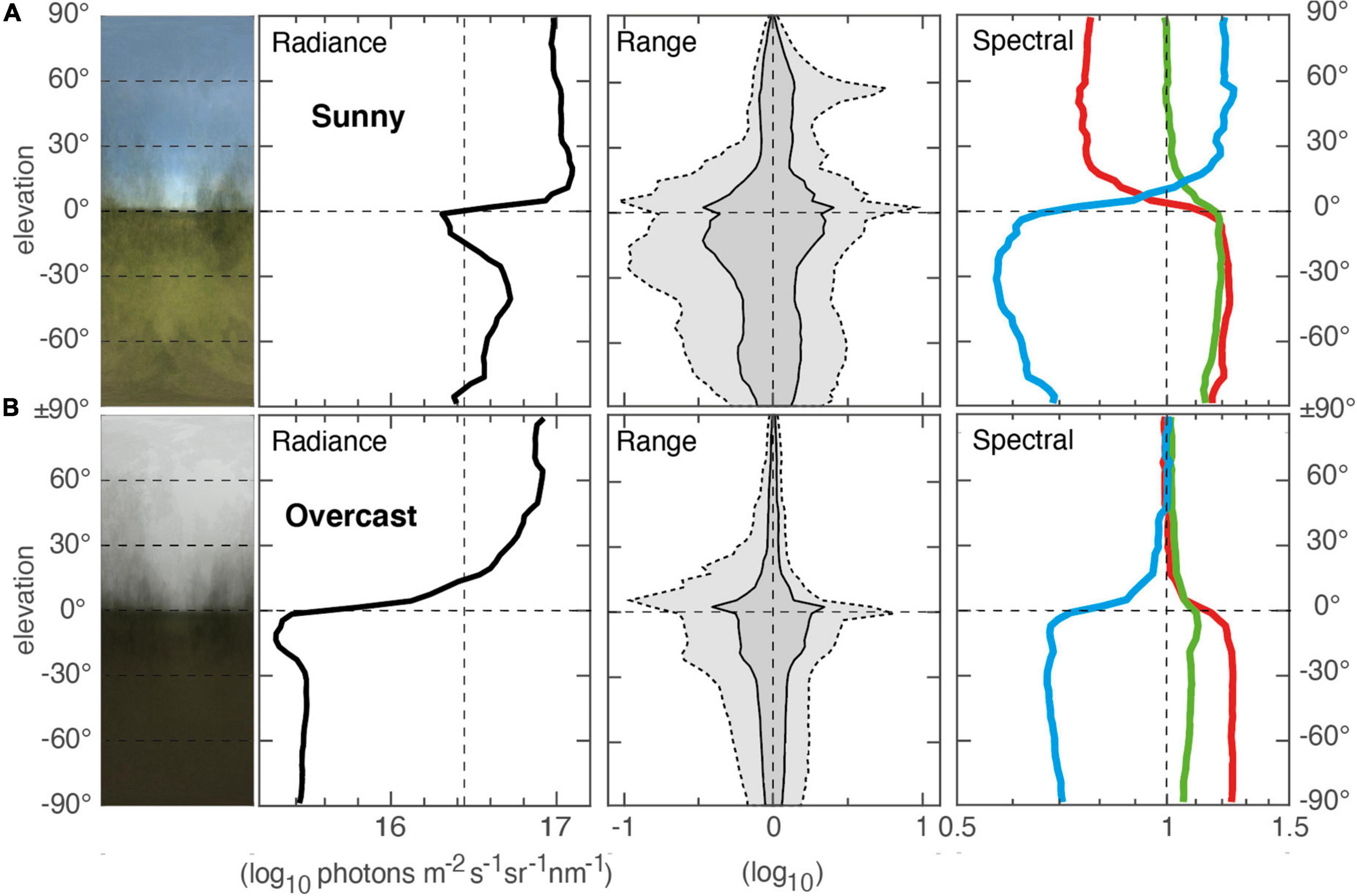
Figure 6. Vertical light gradients in a semi-open environment near Dalby, southern Sweden measured in clear sunny conditions (A) and overcast conditions (B). Measurements were based on 21–25 scenes, sampled on 21 July 2016, start 13:30 m, duration 7 min (A) and 17 September 2016, start 12:44, duration 6 min (B). A single scene at another environment, recorded at sunny, partially cloudy and fully overcast is provided in Supplementary Figure S4.
Vertical light gradients change also with the seasons, especially in areas of deciduous vegetation or winter snow cover (Figure 7). Leaves in deciduous forest canopy change the spectral balance as the leaves mature and eventually acquire autumn colors. Springtime vegetation on the ground, before the canopy takes too much of the light, is obvious in the vertical gradients of April and May in Figure 7. Dry or wet leaf litter on the forest floor causes additional variations in the vertical light gradient, and snow cover obviously has a major effect.
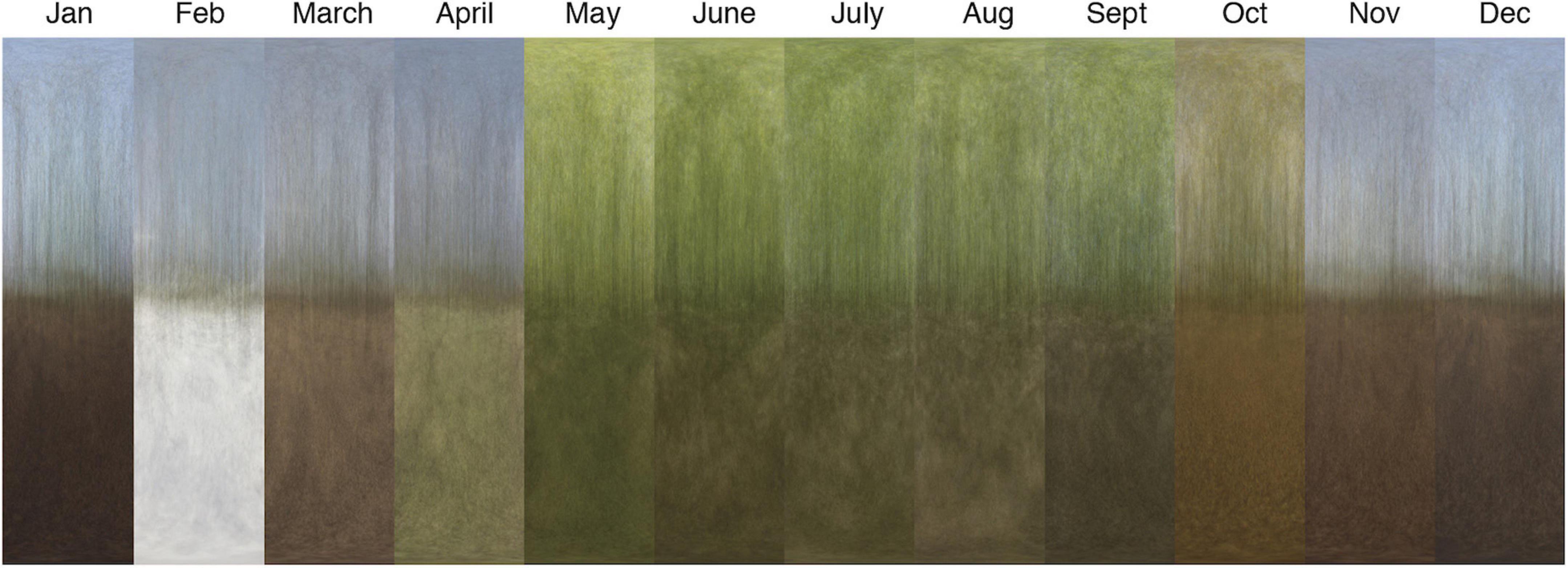
Figure 7. Average images (±90° elevation) of a beech-tree forest near Dalby, southern Sweden, sampled in clear sunny conditions each month of the year. The images were sampled randomly from a trail through the forest, resulting in 30–36 scenes contributing to each image. For these measurements, full results with light gradient graphs are found in Supplementary Figure S5.
Discussion
Visual assessment of the environment
It is obvious that animals equipped with vision can be expected to use this sense to assess their environment for choosing their whereabouts and activities. But which kinds of visual information do animals use for assessing their environment? There can be two principal answers to this question: identified objects and non-object-based visual information (Nilsson, 2022). Seeing identified objects, such as prey or other food items, conspecifics, predators, or objects that can be associated with any of these, are all potentially very useful for assessing the situation and choosing suitable activities (Hein, 2022). There can be no doubt that animals capable of object discrimination also use this visual modality to assess their environment.
However, detection, identification and classification of objects require several layers of devoted neural circuits for visual processing as well as cognitive abilities to translate the information into adaptive behaviors. Non-object based visual information is simpler, requires fewer levels of neural processing and was present before object vision evolved (Nilsson, 2020, 2021). The ancient visual roles that are not based on object vision must have involved assessment of the environment, and because they exploit very different types of visual information, such visual mechanisms are likely to remain important even after object vision evolved.
Visible structures that are not identified objects are fundamental for orientation, control of movement and many other visually guided behaviors (Rodiek, 1998; Tovée, 2008). For assessing the visual environment, non-object-based vision can help determine the type and quality of the environment to aid in habitat selection and choice of activity. It may also be used to determine weather conditions and time of day, all of which are important factors for choosing the most appropriate location and behavior. Without object identification, the visual world is a spatial distribution of intensities. These may vary from up to down and from left to right, i.e., with elevation angle and with azimuth. It is the variations with elevation angle, i.e., the vertical light gradients, that can be expected to differ systematically between different types of environment, times of day, or weather conditions. The azimuth direction provides additional information on the range of intensities at each elevation angle, which is a proxy for the distribution and abundance of visible structures.
Vertical light gradients thus represent much of the non-object based visual information that is available for assessing the environment. Being both simple and informative, vertical light gradients are potentially essential for a wide range of animals in their ability to assess the type of environment, the time of day and the weather conditions. It follows that vertical light gradients may have a fundamental impact on the distribution and activity of animals.
In this manuscript we have presented measurements of vertical light gradients from different environments, terrestrial as well as aquatic, different weather conditions, and times of day and seasons. The gradients were divided into three components: intensity, intensity range (a proxy for spatial structure), and spectral composition. The intensity component can be further divided into the absolute position on the intensity scale, and the relative intensities at different elevation angles. In total, we thus identify four different components of information contained in the vertical light gradients (Table 1). With access only to one of these four components, it would be difficult or impossible to discriminate between different environments and tell them apart from differences that are due to weather or time of day. But combining all four components, such discrimination is generally straightforward. Color vision is needed for determining the spectral balance, and it makes the assessment of vertical light gradients more robust. Many vertebrates and arthropods, from both aquatic and terrestrial environments, can quickly relocate over longer distances, and in these two animal groups, color vision is common.
Many insects, reptiles and birds have excellent color vision which includes a UV channel (Kelber and Osorio, 2010; Cronin et al., 2014; Osorio, 2019). Our measurements were made with a consumer camera and are thus restricted to red, green, and blue channels, but it would clearly be interesting to include also the UV band (300–400 nm) in the measurements. Non-primate mammals are dichromats and would not be able to discriminate between red and green, and many nocturnal species have reduced or lost their color vision (Osorio and Vorobyev, 2008; Jacobs, 2009).
Although color vision is arguably an asset for evaluation of the environment, vertical light gradients are useful also for color-blind species. Most cephalopods (octopus, squid, and cuttlefish) are color blind, but instead have polarization vision (Shashar, 2014). This is a common modality also in arthropod vision (Labhart, 2016). For these animals, the polarization properties of light, as a function of elevation angle, is a potential additional component of the vertical light gradient.
Our measurements were computed for 3° resolution of elevation angles. To make reliable assessments of place, time, and weather, it would suffice to sample with much lower resolution. For nearly all vertebrates and many arthropods, the visual acuity would allow at least the same resolution as in our measurements. Many invertebrates, such as polychaetes, gastropod snails, onychophorans, and millipedes have small low-resolution eyes with an angular resolution in the range of 10°–40° (Nilsson and Bok, 2017), and even for these animals, vertical light gradients would contain useful information for habitat selection and behavioral choice. Interestingly, such low-resolution vision excludes the possibility of object vision, making vertical light gradients the only option for visual assessment of the environment (Nilsson, 2022). In many insects, the dorsal ocelli, and not just the compound eyes, provide sufficient spatial resolution to be used for reading vertical light gradients (Berry et al., 2007a,b; Hung and Ibbotson, 2014; Taylor et al., 2016).
Vertical light gradients from forests, semi-open and open environments (Figures 1–3) reveal large and obvious differences between types of environment and significant differences also between different environments of the same general type. This implies that robust visual cues are available to animals for habitat selection and choice of activities. Within a forest, the vertical light gradients may also differ significantly between different locations, such as the forest edge, local open patches and areas with different plant compositions. Consequently, vertical light gradients are potentially important cues determining the distribution of animals over a large range of spatial scales.
In addition to terrestrial environments, we also presented a small selection of aquatic environments (Figure 4). These are clearly very different to the terrestrial world, largely because of the noticeable loss of contrast with distance. This means that aquatic visual scenes have much fewer objects and other structural details, and are far more dominated by the vertical light gradients of intensity and spectral balance. In aquatic habitats, the vertical light gradients have additional dependencies on depth and water quality, which adds new types of important information. This also means that type of environment, time and weather may be more entangled and more difficult to separate in aquatic light gradients. Here we only present a small sample of aquatic measurements to show that variations are large also in the under-water world. A more rigorous analysis of vertical light gradients in aquatic habitats will be published separately elsewhere.
To make measurements comparable in Figures 1–3, they were all made close to noon and under clear skies. The measurements would be partly different at other times of day or other weather conditions, but the different environments can still be discriminated from each other because time of day and weather affect the vertical light gradients in very specific ways (Figures 5, 6). This means that information about the time and weather can be extracted from the vertical light gradients irrespective of the type of environment. However, there are exceptions where the type of environment, time or weather cannot be entirely separated, or some aspects cannot be read from the vertical light gradients. In dense forests the vertical light gradients change rather little with weather, and also with time, if dusk and dawn are excluded. Cloud cover can mask changes that depend on time, and at starlight intensities most animals are color blind (Cronin et al., 2014; Warrant et al., 2020). Under these conditions, animals may resolve the ambiguities by other sensory modalities or by their biological clock.
The difference between clear and overcast sky (Figure 6) is obvious, but there are of course other weather conditions affecting the visual world. Partial cloud cover, with or without the exposed sun, thin high-altitude clouds, different amounts of air humidity, precipitation, fog and suspended particles will all cause specific changes to the vertical light gradients. At night, the lunar phase will have an impact on the vertical light gradients, offering cues for regulating nocturnal behavior and setting lunar rhythms. Vertical light gradients also contain information on seasons (Figure 7), which are strongly linked to the conditions of the vegetation. Likewise, extended droughts or wet periods will cause changes in the vegetation that are detectable in the vertical light gradient. Finally, anthropogenic alterations of the environment or climate change will influence the vertical light gradients and this may mediate effects on animal distribution and behavior. We conclude that vertical light gradients provide simple but reliable visual cues that can be used by animals to assess a broad range of essential qualities and conditions in their environment.
With an exposed sun or moon, vertical light gradients depend on the azimuth angle. When the sun or moon is far from zenith, solar and antisolar (or lunar and antilunar), directions display different sky gradients as well as different gradients below the horizon. This can be used by animals for directional cues, but for information on the type of environments, time of day or weather conditions, averaging over azimuth orientations (compass directions) offers a way to eliminate such bias. Recording many scenes at random azimuths then corresponds to animals integrating vertical light gradients with time constants much longer than those used for active vision.
How can the information content of vertical light gradients be further investigated?
Our database of vertical light gradients currently includes about 1,200 terrestrial and 100 aquatic environments distributed across the globe, at different times of day and different weather conditions. The material presented in this manuscript and in Nilsson and Smolka (2021) are samples from this large databank of measurements. The technique is simple to use, and many other labs are now contributing to these quantifications of the visual world. How can this wealth of new information be exploited?
There are of course limits to the conclusions that can be drawn from samples selected manually from a large database. A more systematic and unbiased approach would improve the use of vertical light gradients as a tool in various disciplines of ecology, physiology and neurobiology. However, it is not immediately clear how to analyze an entire database of vertical light gradients. The approach of choice will depend on the questions that are asked. The ability to independently determine the type of environment, weather, depth, etc., from the vertical light gradients is of great biological significance. A relevant question is thus whether vertical light gradients can be used to independently classify the type of environment, time of day and weather, and for aquatic environments also depth and possibly even water quality. It would further be important to know if there are conditions where it is difficult or impossible to fully read any of the factors that contribute to the vertical light gradients.
One approach is to apply software that quantifies similarities between different vertical light gradients to generate a phylogenetic tree of measurements. The degree by which measurements from different environments, times of day, weather, etc., assemble in different clades would thus quantify the reliability of vertical light gradients for informing about the environment and its conditions. Failures to place measurements in clades that correspond to different environments and conditions would indicate the limits of useful information from vertical light gradients. We have made preliminary test with this approach and found that existing Matlab routines for phylogenetic analysis can be adapted to successfully classify different types of terrestrial environments (see Supplementary Figure S2). An advantage of this approach is that environment classification is part of the results and is thus unbiased. It is of course also possible to use the same approach to test the support for arbitrary classifications made beforehand.
A principally different approach would be to tag measurements with environment type, time of day, degree of cloud cover, etc., and train a neural network to classify measurements from a subset of the database, and then validate how well it can classify the remaining database. This approach can be adapted to arbitrary measurement classifications and allows investigations of how well different classes can be discriminated. There will of course always be a potential bias introduced by subjective classifications of weather or type of environment (whereas time of day or water depth can be objectively classified). This implies that strict classification criteria would have to be established.
Both the above approaches allow for quantification of the minimum amount of information on the vertical light gradients that is needed for successful discrimination between types of environments, time of day, weather, depth in water, etc. Our measurement routines generate a 3° resolution of elevation angles, but it is quite possible that much lower resolution suffices. It is also possible that some components of the vertical light gradients or angular spans are more important than others for reliable classifications. It would be of particular interest to identify the parts (elevation angles and intensity/range/spectral components) of vertical light gradients that are most essential for biologically relevant discrimination of environments and conditions. This would facilitate behavioral experiments on the effects of vertical light gradients.
The biological significance of vertical light gradients
We have concluded that vertical light gradients carry rich and essential information about the environment. This information is available to all animals with spatial vision, and it would be remarkable if it does not have a major influence on animal behavior and choice of habitat. The spatial distribution of light in natural environments is known to be reflected in the retinal design of both vertebrate and insect eyes (Zimmermann et al., 2018; Lancer et al., 2020; Qiu et al., 2021), but how animals use the overall distribution of light to assess their environment is practically unknown. The amount of light illuminating the environment (the illuminance or irradiance) is well-known to regulate behavior and to entrain biological rhythms across the animal kingdom (Hertz et al., 1994; Cobcroft et al., 2001; Kristensen et al., 2006; Chiesa et al., 2010; Tuomainen and Candolin, 2011; Pauers et al., 2012; Alves-Simoes et al., 2016; Farnworth et al., 2016; Kapogiannatou et al., 2016; Blume et al., 2019; Storms et al., 2022). Accordingly, artificial light at night (light pollution) is emerging as a major factor influencing the spatiotemporal distribution of animals (Polak et al., 2011; Owens et al., 2020; Hölker et al., 2021; Miller et al., 2022). However, with rare exceptions such as Jechow et al. (2019), it is the ambient light intensity (illuminance), not the spatial distribution of light, that is documented as the environmental cue. In contrast, many established effects of light may very well be sensed as vertical light gradients by the animals. It is clear that illuminance readings cannot be used to discriminate between the effects of time, weather, environments, or water depth, but the combined components of vertical light gradients can resolve these ambiguities. There are thus reasons to believe that, for many animal species, vertical light gradients represent the actual cue of which the general illuminance is just one component related to the absolute position of the intensity profile on the radiance axis. It is therefore possible that the majority of known responses to light intensity are in fact responses to vertical light gradients.
Given the potentially major and important effects on animal behavior, responses to vertical light gradients deserve attention, but how can such responses be investigated? For the vast majority of animal species, it can be expected that the spatiotemporal distribution and behavioral choices depend on the input from multiple senses, previous experiences and internal physiological states. To isolate the contributions of vertical light gradients, the first option would be to monitor behavioral choice in laboratory environments where the vertical light gradients can be manipulated when all else is kept constant. However, reproducing natural light gradients in a laboratory setting is not entirely trivial. Projection of light on a planetarium hemisphere is problematic because reflected light will illuminate all other elevation angles. Full control of the vertical light gradients requires bright light emitting diode (LED) monitors in all directions, at least above the horizontal plane, in addition to a direct light source simulating the sun or moon, and carefully controlled reflective properties of the ground and surrounding objects. The emission of red, green, and blue light must be individually controllable, and the produced light environments must be calibrated by measurements. The controlled environment must further be large enough to allow for a natural choice of behaviors of the tested species.
There are good reasons to attempt the type of laboratory test outlined above on a broad selection of species. Such experiments will reveal the impact of vertical light gradients and how much it differs between species. By manipulating the vertical light gradients, it will also be possible to uncover the components of the light environment that are most important for behavioral control. With a good knowledge of the effects of vertical light gradients, these can be applied to predict the consequence of changes in both natural and artificial environments, to identify desirable and undesirable changes, and to mitigate negative effects on the spatiotemporal distribution of animals. More specifically, vertical light gradients can be used to identify conditions that elicit swarming, aggregation, or other biologically important behaviors. It is also possible that vertical light gradients are used for endocrine control, to make the animals physiologically prepared for the preferred activities. Knowledge of the influence of vertical light gradients may also be used to create more appropriate conditions for physiological and behavioral animal experiments (Mäthger et al., 2022), and to improve artificial indoor lighting for humans and in animal husbandry.
There is also a neurobiological side of visual assessment. Vertical light gradients are likely to provide access to previously unexplored mechanisms that set behavioral states in animals. All animals constantly have to decide where to be and what to do. The choices could be to forage at the current location or search for a better place, to avoid or engage in aggressive mate interactions, to continue resting or initiate activity. At any moment, depending on the type of environment, time of day, weather condition or other visible cues, some activities are suitable, but others are not. The changing tendency to engage in some activities but not in others is often referred to as “behavioral states.” This concept is gaining ground in both ecology and neurobiology (Berman et al., 2016; Gurarie et al., 2016; Mahoney and Young, 2017; Naässel et al., 2019; Russell et al., 2019; McCormick et al., 2020). The contribution of vertical light gradients in the setting of behavioral states must of course involve the visual system, but the neural processes that result in a choice of activity remains poorly know.
In most animals, the master-control of behavior is likely to involve several rather different processes. A general tendency to prefer some activities and avoid others can be set by internal physiological states together with sensory information about external conditions such as vertical light gradients. Behavioral tendencies are likely to involve a combination of systemic hormones and local release of neuromodulators that together alter the properties of decision-making circuits in the brain, i.e., set an appropriate behavioral state. For animals with at least some cognitive abilities, the actual decision of initiating or terminating a behavior will involve an assessment of the situation. In terms of vision, such an assessment would be based on the distribution of recognized objects (animals or other relevant items), combined with memories and experiences, and the outcome would be used to time and actuate behavioral decisions (Hein, 2022). Most species will also have protective reflexes that can override any behavioral decision. Once a behavior is initiated, it can be guided with continuously updated sensory information in a closed loop (Milner and Goodale, 2008).
The neural circuits that act as a master–control of behavior are poorly known in both vertebrates and arthropods. The neural pathways that carry information on vertical light gradients must be different from “ordinary vision” used for orientation and object vision. The reason for this statement is that “ordinary vision” relies on image contrasts and their movement (Rodiek, 1998), whereas vertical light gradients instead represent the background against which objects and contrasts are seen. To relay information on the movement of objects and other image contrast, the neurons need to be fast and provide high spatial resolution. To record the visual background, the opposite is true: it is contained largely in low spatial frequencies, and the information is extracted by integrating over time. Low spatial resolution means few neurons, and long integration time (slow speed) implies thin neurites. So, even if reading vertical light gradients may be a fundamentally important visual function, its neural correlates may have escaped attention because they are expected to make up only a small and inconspicuous part of animal visual systems.
Conclusion
The mechanisms that animals use to visually assess the environment and its conditions have been largely overlooked. Here we show that vertical light gradients contain vital information on the type of environment, time, weather, and other factors that animals are likely to rely on for finding suitable habitats and optimally deploying their behavioral repertoire.
Responses to vertical light gradients can be expected to be species-specific and contribute to define the niche of each species. Unraveling these responses will open a new dimension in behavioral ecology and provide an understanding of the mechanisms behind choices of habitat and activity. Measurements of vertical light gradients are easily acquired and offer a powerful tool for making predictions on the spatiotemporal distribution of animals, timing of specific behaviors and responses to a changing world.
Data availability statement
The original contributions presented in this study are included in the article/Supplementary material, further inquiries can be directed to the corresponding author.
Author contributions
D-EN conceived the project with input from JS and MB. D-EN wrote the first draft. JS and MB refined the text. D-EN and JS prepared the Supplementary material. All authors contributed data and approved the final version.
Funding
We gratefully acknowledge funding from the Swedish Research Council (grants 2015-04690 and 2019-04813 to D-EN), and the Knut and Alice Wallenberg Foundation (grant KAW 2011.0062 to D-EN).
Conflict of interest
The authors declare that the research was conducted in the absence of any commercial or financial relationships that could be construed as a potential conflict of interest.
Publisher’s note
All claims expressed in this article are solely those of the authors and do not necessarily represent those of their affiliated organizations, or those of the publisher, the editors and the reviewers. Any product that may be evaluated in this article, or claim that may be made by its manufacturer, is not guaranteed or endorsed by the publisher.
Supplementary material
The Supplementary Material for this article can be found online at: https://www.frontiersin.org/articles/10.3389/fevo.2022.951328/full#supplementary-material
References
Abram, P. K., Bovin, G., Moiroux, J., and Brodeur, J. (2016). Behavioural effects of temperature on ectothermic animals: Unifying thermal physiology and behavioural plasticity. Biol. Rev. 92, 1859–1876. doi: 10.1111/brv.12312
Alves-Simoes, M., Coleman, G., and Canal, M. M. (2016). Effects of type of light on mouse circadian behaviour and stress levels. Laborat. Anim. 50, 21–29. doi: 10.1177/0023677215588052
Berman, G. J., Bialek, W., and Shaevitz, J. W. (2016). Predictability and hierarchy in Drosophila behavior. Proc. Natl. Acad. Sci. U.S.A. 113, 11943–11948. doi: 10.1073/pnas.1607601113
Berry, R. P., Stange, G., and Warrant, E. J. (2007a). Form vision in the insect dorsal ocelli: An anatomical and optical analysis of the dragonfly median ocellus. Vision Res. 47, 1394–1409. doi: 10.1016/j.visres.2007.01.019
Berry, R. P., Warrant, E. J., and Stange, G. (2007b). Form vision in the insect dorsal ocelli: An anatomical and optical analysis of the Locust Ocelli. Vision Res. 47, 1382–1393. doi: 10.1016/j.visres.2007.01.020
Blume, C., Garbazza, C., and Spitschan, M. (2019). Effects of light on human circadian rhythms, sleep and mood. Somnologie. 23, 147–156. doi: 10.1007/s11818-019-00215-x
Breugel, F., van, Huda, A., and Dickinson, M. H. (2018). Distinct activity-gated pathways mediate attraction and aversion to CO2 in Drosophila. Nature 564, 420–424. doi: 10.1038/s41586-018-0732-8
Carandini, M., Demb, J. B., Mante, V., Tolhurst, D. J., Dan, Y., Olshausen, B. A., et al. (2005). Do We Know What the Early Visual System Does? J. Neurosci. 16, 10577–10597. doi: 10.1523/JNEUROSCI.3726-05.2005
Chiesa, J. J., Aguzzi, J., Garciìa, J. A., SardaÌ, F., and de la Iglesia, H. O. (2010). Light Intensity Determines Temporal Niche Switching of Behavioral Activity in Deep-Water Nephrops norvegicus (Crustacea: Decapoda). J. Biol. Rhythms. 25, 277–287. doi: 10.1177/0748730410376159
Clark, D. A., and Demb, J. B. (2016). Parallel Computations in Insect and Mammalian Visual Motion Processing. Curr. Biol. 26, R1062–R1072. doi: 10.1016/j.cub.2016.08.003
Cobcroft, J. M., Pankhurst, P. M., Hart, P. R., and Battaglene, S. C. (2001). The effects of light intensity and algae-induced turbidity on feeding behaviour of larval striped trumpeter. J. Fish Biol. 59, 1181–1197. doi: 10.1111/j.1095-8649.2001.tb00185.x
Cronin, T. W., Johnsen, S., Marshall, N. J., and Warrant, E. J. (2014). Visual Ecology. Princeton: Princeton Univ. Press. doi: 10.23943/princeton/9780691151847.001.0001
Davies, N. B., Krebs, J. B., and West, S. A. (2012). An Introduction to Behavioural Ecology, 4th Edn. Oxford: Wiley-Blackwell, 506.
Farnworth, B., Innes, J., and Waas, J. R. (2016). Converting predation cues into conservation tools: The effect of light on mouse foraging behaviour. PLoS One 11:e0145432. doi: 10.1371/journal.pone.0145432
Gurarie, E., Bracis, C., Delgado, M., Meckley, T. D., Kojola, I., and Wagner, C. M. (2016). What is the animal doing? Tools for exploring behavioural structure in animal movements. J. Anim. Ecol. 85, 69–84. doi: 10.1111/1365-2656.12379
Hein, A. M. (2022). Ecological decision-making: From circuit elements to emerging principles. Curr. Opin. Neurobiol. 74:102551. doi: 10.1016/j.conb.2022.102551
Hertz, P. E., Fleishman, L. J., and Armsby, C. (1994). The influence of light intensity and temperature on microhabitat selection in two Anolis lizards. Functional Ecol. 8, 720–729. doi: 10.2307/2390231
Hölker, F., Bollinge, J., Davies, T. W., Gaivi, S., Jechow, A., Kalinkat, G., et al. (2021). 11 Pressing Research Questions on How Light Pollution Affects Biodiversity. Front. Ecol. Evolut. 9:767177. doi: 10.3389/fevo.2021.767177
Hung, Y.-S., and Ibbotson, M. R. (2014). Ocellar structure and neural innervation in the honeybee. Front. Neuroanat. 8:6. doi: 10.3389/fnana.2014.00006
Jacobs, G. H. (2009). Evolution of colour vision in mammals. Phil. Trans. R. Soc. B. 364, 2957–2967. doi: 10.1098/rstb.2009.0039
Jechow, A., Kyba, C. C. M., and Hoölker, F. (2019). Beyond all-sky: Assessing ecological light pollution using multi-spectral full-sphere fisheye lens imaging. J. Imag. 5:46. doi: 10.3390/jimaging5040046
Johnsen, S. (2012). The Optics of Life: A Biologist’s Guide to Light in Nature. Princeton: Princeton University Press. doi: 10.1515/9781400840663
Kapogiannatou, A., Paronis, E., Paschidis, K., Polissidis, A., and Kostomitsopoulos, N. G. (2016). Effect of light colour temperature and intensity on the behaviour of male C57CL/6J mice. Appl. Anim. Behav. Sci. 184, 135–140. doi: 10.1016/j.applanim.2016.08.005
Kelber, A., and Osorio, D. (2010). From spectral information to animal colour vision: Experiments and concepts. Proc. R. Soc. B. 277, 1617–1625. doi: 10.1098/rspb.2009.2118
Kristensen, H. H., Prescott, N. B., Perry, G. C., Ladewig, J., Ersbøll, A. K., Overvad, K. C., et al. (2006). The behaviour of broiler chickens in different light sources and illuminances. Appl. Anim. Behav. Sci. 103, 75–89. doi: 10.1016/j.applanim.2006.04.017
Labhart, T. (2016). Can invertebrates see the e-vector of polarization as a separate modality of light? J. Exp. Biol. 219, 3844–3856. doi: 10.1242/jeb.139899
Lancer, B. H., Evans, B. J. E., and Wiederman, S. D. (2020). The visual neuroecology of Anisoptera. Curr. Opin. Insect Sci. 42, 14–22. doi: 10.1016/j.cois.2020.07.002
MacIver, M. A., Schmitz, L., Mugan, U., Murphey, T. D., and Mobley, C. D. (2017). Massive increase in visual range preceded the origin of terrestrial vertebrates. Proc. Natl. Acad. Sci. U.S.A. 114, E2375–E2384. doi: 10.1073/pnas.1615563114
Mahoney, P. J., and Young, J. K. (2017). Uncovering behavioural states from animal activity and site fidelity patterns. Methods Ecol. Evol. 8, 174–183. doi: 10.1111/2041-210X.12658
Mäthger, L. M., Bok, M. J., Liebich, J., Sicius, L., and Nilsson, D.-E. (2022). Pupil dilation and constriction in the skate Leucoraja erinacea in a simulated natural light field. J. Exp. Biol. 225:jeb243221. doi: 10.1242/jeb.243221
McCormick, D. A., Nestvogel, D. B., and He, B. J. (2020). Neuromodulation of Brain State and Behavior. Ann. Rev. Neurosci. 43, 391–415. doi: 10.1146/annurev-neuro-100219-105424
Miller, C. R., Vitousek, M. N., and Thaler, J. S. (2022). Light at night disrupts trophic interactions and population growth of lady beetles and pea aphids. Oecologia 3, 527–535. doi: 10.1007/s00442-022-05146-3
Milner, A. D., and Goodale, M. A. (2008). Two visual systems re-viewed. Neuropsychologia 46, 774–785. doi: 10.1016/j.neuropsychologia.2007.10.005
Naässel, D. R., Pauls, D., and Huetteroth, W. (2019). Neuropeptides in modulation of Drosophila behavior: How to get a grip on their pleiotropic actions. Curr. Opin. Insect Sci. 36, 1–8. doi: 10.1016/j.cois.2019.03.002
Nilsson, D.-E. (2017). Evolution: An Irresistibly Clear View of Land. Curr. Biol. 27, R702–R719. doi: 10.1016/j.cub.2017.05.082
Nilsson, D.-E. (2020). Eye Evolution in Animals. Ref. Mod. Neurosci. Biobehav. Psychol. 1, 96–121. doi: 10.1016/B978-0-12-805408-6.00013-0
Nilsson, D.-E. (2021). The diversity of eyes and vision. Ann. Rev. Vis. Sci. 7, 8.1–8.23. doi: 10.1146/annurev-vision-121820-074736
Nilsson, D.-E. (2022). The evolution of visual roles – ancient vision versus object vision. Front. Neuroanat. 16:789375. doi: 10.3389/fnana.2022.789375
Nilsson, D.-E., and Bok, M. J. (2017). Low-Resolution Vision—at the Hub of Eye Evolution. Integ. Comp. Biol. 57, 1066–1070. doi: 10.1093/icb/icx120
Nilsson, D.-E., and Smolka, J. (2021). Quantifying biologically essential aspects of environmental light. J. R. Soc. Interf. 18:20210184. doi: 10.1098/rsif.2021.0184
Olshausen, B. A., and Field, D. J. (2004). Sparse coding of sensory inputs. Curr. Opin. Neurobiol. 14, 481–487. doi: 10.1016/j.conb.2004.07.007
Osorio, D. (2019). The evolutionary ecology of bird and reptile photoreceptor spectral sensitivities. Curr. Opin. Behav. Sci. 30, 223–227. doi: 10.1016/j.cobeha.2019.10.009
Osorio, D., and Vorobyev, M. (2008). A review of the evolution of animal colour vision and visual communication signals. Vis. Res. 48, 2042–2051. doi: 10.1016/j.visres.2008.06.018
Owens, A. C. S., Cochard, P., Durrant, J., Farnworth, B., Perkin, E. K., and Seymoure, B. (2020). Light pollution is a driver of insect declines. Biol. Conserv. 241:108259. doi: 10.1016/j.biocon.2019.108259
Pauers, M. J., Kuchenbecker, J. A., Neitz, M., and Neitz, J. (2012). Changes in the colour of light cue circadian activity. Anim. Behav. 83:1143e1151. doi: 10.1016/j.anbehav.2012.01.035
Polak, T., Korine, C., Yair, S., and Holderied, M. W. (2011). Differential effects of artificial lighting on flight and foraging behaviour of two sympatric bat species in a desert. J. Zool. 285, 21–27. doi: 10.1111/j.1469-7998.2011.00808.x
Qiu, Y., Zhao, Z., Klindt, D., Kautzky, M., Szatko, K. P., Schaeffel, F., et al. (2021). Natural environment statistics in the upper and lower visual field are reflected in mouse retinal specializations. Curr. Biol. 31, 3233–3247. doi: 10.1016/j.cub.2021.05.017
Russell, L. E., Yang, Z., Tan, P. L., Fisek, M., Packer, A. M., Dalgleish, H. W. P., et al. (2019). The influence of visual cortex on perception is modulated by behavioural state. bioRxiv [preprint]. doi: 10.1101/706010
Sanes, J. R., and Zipursky, S. L. (2010). Design principles of insect and vertebrate visual systems. Neuron. 66, 15–36. doi: 10.1016/j.neuron.2010.01.018
Shashar, N. (2014). “Polarization Vision in Cephalopods,” in Polarized Light and Polarization Vision in Animal Sciences, Vol. 2, ed. G. Horváth (Heidelberg: Springer), doi: 10.1007/978-3-642-54718-8_8
Storms, M., Jakhar, A., Mitesser, O., Jechow, A., Hölker, F., Degen, T., et al. (2022). The rising moon promotes mate finding in moths. Commun. Biol. 5:393. doi: 10.1038/s42003-022-03331-x
Taylor, G. J., Ribi, W., Bech, M., Bodey, A. J., Rau, C., Steuwer, A., et al. (2016). The dual function of orchid bee ocelli as revealed by X-Ray microtomography. Curr. Biol. 26, 1319–1324. doi: 10.1016/j.cub.2016.03.038
Tovée, M. J. (2008). An Introduction to the Visual System, 2nd Edn. Cambridge: Cambridge University Press, 212. doi: 10.1017/CBO9780511801556
Tuomainen, U., and Candolin, U. (2011). Behavioural responses to human-induced environmental change. Biol. Rev. 86, 640–657. doi: 10.1111/j.1469-185X.2010.00164.x
Warrant, E., Johnsen, S., and Nilsson, D.-E. (2020). Light and visual environments. Ref. Mod. Neurosci. Biobehav. Psychol. 1, 4–30. doi: 10.1016/B978-0-12-805408-6.00002-6
Keywords: vertical light-gradient, spatiotemporal distribution, animal behavior, behavioral choice, vision, behavioral state
Citation: Nilsson D-E, Smolka J and Bok M (2022) The vertical light-gradient and its potential impact on animal distribution and behavior. Front. Ecol. Evol. 10:951328. doi: 10.3389/fevo.2022.951328
Received: 23 May 2022; Accepted: 26 July 2022;
Published: 11 August 2022.
Edited by:
Daniel Marques Almeida Pessoa, Federal University of Rio Grande do Norte, BrazilReviewed by:
Andreas Jechow, Leibniz-Institute of Freshwater Ecology and Inland Fisheries (IGB), GermanyMisha Vorobyev, The University of Auckland, New Zealand
Copyright © 2022 Nilsson, Smolka and Bok. This is an open-access article distributed under the terms of the Creative Commons Attribution License (CC BY). The use, distribution or reproduction in other forums is permitted, provided the original author(s) and the copyright owner(s) are credited and that the original publication in this journal is cited, in accordance with accepted academic practice. No use, distribution or reproduction is permitted which does not comply with these terms.
*Correspondence: Dan-E Nilsson, dan-e.nilsson@biol.lu.se