- Center for Ecological Research, Kyoto University, Otsu, Japan
Bacteria, fungi, and nematodes are major components of soil ecosystems, playing pivotal roles in belowground material cycles and biological community processes. A number of studies have recently uncovered the diversity and community structure of those organisms in various types of soil ecosystems based on DNA metabarcoding (amplicon sequencing). However, because most previous studies examined only one or two of the three organismal groups, it remains an important challenge to reveal the entire picture of soil community structure. We examined how we could standardize DNA extraction protocols for simultaneous DNA metabarcoding of bacteria, fungi, and nematodes. Specifically, in an Illumina sequencing analysis of forest and farmland soil samples, we performed DNA extraction at five levels of soil-amount (0.5, 2, 5, 10, and 20 g). We then found that DNA extraction with the 0.5 g soil setting, which had been applied as default in many commercial DNA extraction kits, could lead to underestimation of α-diversity in nematode community. We also found that dissimilarity (β-diversity) estimates of community structure among replicate samples could be affected by soil sample amount. Based on the assays, we conclude that DNA extraction from at least 20 g of soil is a standard for comparing biodiversity patterns among bacteria, fungi and nematodes.
Introduction
Bacteria, fungi, and nematodes occupy a large amount of biomass in the soil, playing pivotal ecosystem functions (Fierer et al., 2007; Bardgett and van der Putten, 2014; Nielsen et al., 2015; Wall et al., 2015; Bahram et al., 2018; Bar-On et al., 2018; Flemming and Wuertz, 2019; van den Hoogen et al., 2019). These soil organisms have also been recognized as indicators of the soil environment, targeted, for example, in assays of soil contamination by anthropogenic activities (Bongers, 1990; Bongers and Bongers, 1998; Bongers and Ferris, 1999). In uncovering and evaluating the diversity of those soil organisms, DNA metabarcoding (amplicon sequencing) based on high-throughput sequencing has been increasingly applied as a standard approach (Caporaso et al., 2012; Reuter et al., 2015). Through the recent enrichment of reference DNA databases of bacterial 16S rRNA, fungal internal transcribed spacer (ITS), and nematode 18S rRNA sequences, it has become possible to gain large datasets of soil biodiversity without longtime taxonomic expertise or experiences in microbial isolation (Quast et al., 2013; Nilsson et al., 2019; Kawanobe et al., 2021).
In expanding the use of DNA metabarcoding of soil biodiversity, it is of particular importance to develop standard protocols applicable to broad ranges of situations and organisms. For example, universal primers with high taxonomic coverage have been proposed for each of the bacterial 16S rRNA (Klindworth et al., 2013; Thijs et al., 2017), fungal ITS (Schoch et al., 2012; Lindahl et al., 2013; Op De Beeck et al., 2014), and nematode 18S rRNA regions (Sapkota and Nicolaisen, 2015; Ahmed et al., 2019; Waeyenberge et al., 2019; Sikder et al., 2020; Kenmotsu et al., 2021). For the bioinformatic processes, rapid and accurate algorithms for inferring operational taxonomic units (OTUs) or amplicon sequence variants (ASVs) have been implemented (Callahan et al., 2016). Likewise, theories on reference database search (Tanabe and Toju, 2013) and taxonomic assignment (Huson et al., 2007) have been proposed to allow automatic molecular identification. While all these processes are keys to reliability and reproducibility of DNA metabarcoding, results of molecular-based biodiversity inventories to depend greatly on DNA extraction methods (Thompson et al., 2017; Zielińska et al., 2017; Francioli et al., 2021). In particular, the amount of the soil subjected to DNA extraction possibly has major impacts on the α- and β-diversity estimates of metabarcoding samples.
Because soil properties such as pH and nitrogen concentrations vary within ecosystems, the taxonomic compositions of soil organisms often show high spatial heterogeneity (Franklin and Mills, 2003; Fierer, 2017; Flemming and Wuertz, 2019). Therefore, DNA extraction from small amounts of soil samples may lead to the underestimation of biodiversity or low reproducibility in the reconstruction of community structure. To date, a number of studies have been conducted to evaluate how soil amounts in DNA extraction can affect DNA metabarcoding results of bacteria and/or fungi (Martin-Laurent et al., 2001; Ellingsøe and Johnsen, 2002; Kang and Mills, 2006; Song et al., 2015; Penton et al., 2016). In the Earth Microbiome Project (Gilbert et al., 2014), for example, DNA extraction from 0.5 g of soil was described as a standard protocol.1 For nematode, in contrast, studies based on real-time PCR and T-RFLP concluded that 100 g of soil could be required for inferring community structure (Wiesel et al., 2015). Because DNA extraction from 100 g of soil is impractical for high-throughput analyses with standard laboratory equipment, developing protocols of DNA extraction from smaller amount of soil is an important step for expanding the application of nematode DNA metabarcoding. Moreover, because soil ecosystem processes are driven by complex interactions between bacteria, fungi, and nematodes (Bonfante and Anca, 2009; Toju and Tanaka, 2019; Detrey et al., 2022), standardized DNA extraction protocols commonly applicable to the three organismal groups will provide a platform for reshaping our understanding of ecosystem functions and dynamics.
In this study, we examined how DNA metabarcoding data could be influenced by the amount of the soil subjected to DNA extraction. We collected soil in forest and farmland (soybean field) ecosystems and then performed DNA extraction at five levels of soil-amount settings (0.5, 2, 5, 10, and 20 g). Given the large body size and mobility of nematodes (Ferris et al., 1990; Ettema, 1998; Liu et al., 2019), DNA extraction protocols optimized for bacteria may lead to the failure of nematode diversity profiling (Wiesel et al., 2015). Thus, we tested the hypothesis that standard DNA extraction protocol optimized for bacteria (i.e., extraction from 0.5 g of soil) could result in potential biases in the estimation of nematode diversity. Specifically, based on the Illumina sequencing outputs, α- and β-diversity estimates were compared among the five soil-amount classes for each ecosystem type (forest or farmland) for each of the bacterial 16S rRNA, fungal ITS, and nematode 18S rRNA regions. We then explored the minimum soil amount enabling the simultaneous analysis of bacteria, fungi, and nematodes. The results shown in this study will provide a basis for designing DNA metabarcoding protocols commonly applicable to major taxa in soil ecosystems.
Materials and methods
Study site and soil sampling
Soil samples were collected on October 29, 2021, from a temperate secondary forest (34.972 °N, 135.959 °E) and an experimental soybean field (34.972 °N, 135.958 °E) of the Center for Ecological Research, Kyoto University. In the secondary forest, which was dominated by Quercus serrata (Fagaceae), litter on the soil surface was removed before sampling. Then, at each of the eight sampling positions set at 8-m intervals, three soil core (diameter = 3 cm, depth = 10 cm) samples collected within a 2 m × 18 m area (32 m2) were mixed in a plastic bag. The eight soil samples (ca. 100 g/sample) were then immediately stored in a cold storage bag upon sampling at respective positions in order to avoid the degradation of DNA. Likewise, in the soybean field, soil cores were collected at each of the eight sampling positions set at > 8-m intervals within a 2 m × 18 m area (32 m2). The eight forest soil samples and the eight soybean-field soil samples were preserved at –25°C in the laboratory until DNA extraction.
DNA extraction
Each soil sample was well mixed and subjected to the following DNA extraction processes with five alternative soil amount settings: specifically, DNA extraction from 0.5, 2, 5, 10, and 20 g of wet soil was conducted. For the 0.5-g setting, soil DNA was directly processed with Extrap Soil DNA Kit Plus Ver.2 (BioDynamics Laboratory Inc., Tokyo) based on the beads-beating protocol provided by the manufacturer. For the remaining four settings (2, 5, 10, and 20 g), each soil sample soaked in extraction buffer [10 mM Tris–HCl (pH 8.5), 1 mM EDTA, 1% SDS] (2, 5, 10, and 20 ml for the 2, 5, 10, and 20 g setting, respectively) was shaken with 4-, 1-, and 0.5-mm zirconium beads (Asone, Osaka) in a 50-ml centrifuge tube at 6.5 m/sec for 60 s using FastPrep 24 (MP Biomedicals, United States). After centrifugation, 500 μl of the supernatant was subjected to DNA extraction using Extrap Soil DNA Kit without the default beads-beating step. In total, 80 DNA template samples were obtained [2 ecosystem types (forest or soybean field) × 8 samples × 5 soil-amount classes].
DNA amplification and sequencing
Profiling of biodiversity was performed by targeting bacteria, fungi, and nematodes. For the amplification of the 16S rRNA V4 region of bacteria, the set of the forward primer 515f (Caporaso et al., 2011) and the reverse primer 806rB (Apprill et al., 2015) were used. The primers were fused with 3–6-mer Ns for improved Illumina sequencing quality (Lundberg et al., 2013) and Illumina sequencing primers as detailed in a previous study (Toju et al., 2019). PCR was performed at a 10-μl-scale protocol of KOD ONE PCR Master Mix (TOYOBO, Osaka) with the temperature profile of 35 cycles at 98°C for 10 s (denaturation), 60°C for 5 s (annealing of primers), and 68°C for 5 s (extension), and a final extension at 68°C for 2 min. The ramp rate through the thermal cycles was set to 1°C/sec to prevent the generation of chimeric sequences (Stevens et al., 2013). Taking into account the inference of a previous study on high-throughput sequencing technologies (Smith and Peay, 2014), no PCR replication was set.
Likewise, the ITS1 region of fungi was amplified using the set of the forward primer ITS1F_KYO1 and the reverse primer ITS2_KYO2 (Toju et al., 2012). PCR was performed using the Illumina-sequencing fusion primer design mentioned above at a 10-μl-scale protocol of KOD ONE PCR Master Mix (TOYOBO, Osaka) with the temperature profile of 35 cycles at 98°C for 10 s, 52°C for 5 s, and 68°C for 5 s, and a final extension at 68°C for 2 min (ramp rate = 1°C/sec; no PCR replication).
For the amplification of nematodes, we redesigned the primer sets targeting nuclear 18S rRNA region (Kenmotsu et al., 2021) to improve taxonomic coverage. Specifically, using the aligned 18S rRNA sequences of major nematode taxa [downloaded from the NCBI database2 on November 8, 2020; Supplementary Data 2], we designed the new forward primer NF1_rv (5′- GGT GCA TGG CCG TTC TTA GTT –3′) and reverse primer Nem18SV8_rv (5′- GTG TGT ACA AAK GGC AGG GAC –3′). The PCR for Illumina sequencing was performed with the same thermal cycle protocol used in the analysis of the fungal ITS1 region (no PCR replication).
The PCR products of the bacterial 16S rRNA, fungal ITS1, and nematode 18S rRNA regions were, respectively, subjected to the additional PCR step for linking Illumina sequencing adaptors and 8-mer sample identifier indexes (Hamady et al., 2008) with the amplicons as detailed elsewhere (Toju et al., 2019). The temperature profile in the PCR was 7 cycles at 98°C for 10 s, 55°C for 5 s, and 68°C for 5 s, and a final extension at 68°C for 2 min. The PCR products of the 80 samples were then pooled for each of the 16S rRNA, fungal ITS1, and nematode 18S rRNA regions after a purification/equalization process with the AMPure XP Kit (Beckman Coulter, Inc., Brea). Primer dimers, which were shorter than 200 bp, were removed from the pooled library by supplemental purification with AMPure XP: the ratio of AMPure XP reagent to the pooled library was set to 0.8 (v/v) in this process. The sequencing libraries of the three regions were processed in an Illumina MiSeq sequencer (15% PhiX spike-in). Because the quality of forward sequences is generally higher than that of reverse sequences in Illumina sequencing, we optimized the MiSeq run setting in order to use only forward sequences. Specifically, the run length was set 271 forward (R1) and 31 reverse (R4) cycles to enhance forward sequencing data. The reverse sequences were used only for discriminating between bacterial 16S, fungal ITS1, and nematode 18S rRNA sequences in the following bioinformatic procedure.
Bioinformatics
In total, 13,039,352 sequencing reads were obtained in the Illumina sequencing. On average, 14,622 (SD = 4,262), 6,945 (SD = 6,421) and 66,770 (SD = 46,808) reads were obtained per sample in the prokaryote16S rRNA, fungal ITS, and nematode 18S rRNA regions. The raw sequencing data were converted into FASTQ files using the program bcl2fastq 1.8.4 distributed by Illumina. The bacterial 16S rRNA, fungal ITS and nematode 18S rRNA regions were discriminated based on the sequences of the forward and reverse primer positions and the output FASTQ files were demultiplexed using clsplitseq command of Claident v0.9.2022.01.26 (Tanabe and Toju, 2013; Tanabe, 2022). The removal of low-quality sequences and ASV inference were conducted using filterAndTrim function of DADA2 v1.17.5 (Callahan et al., 2016) package of R v3.6.3 (R Core Team, 2021). Taxonomic annotation of bacteria and fungi was conducted based on the SILVA 138 SSU (Quast et al., 2013) and UNITE version8.2 (Tedersoo et al., 2018) using assignTaxonomy function of DADA2. For the taxonomic annotation of nematodes, the five-nearest-neighbor method using clidentseq command of Claident was applied to the NCBI nucleotide sequence database. ASVs that were not assigned to the domain Bacteria, the kingdom Fungi and the phylum Nematoda were removed from the 16S rRNA, ITS and 18S rRNA datasets, respectively. The mean number of filtered sequencing reads obtained per sample was 2,758, 1,851, and 2,113 for the bacterial, fungal and nematode datasets, respectively. For each target organismal group (Bacteria, Fungi and Nematoda), we then obtained a sample × ASV matrix, in which a cell entry depicted the number of sequencing reads of as ASV in a sample (Supplementary Data 1). The sample with less than 1,000 sequencing reads were discarded from the matrices: 60, 61, and 66 samples remained for bacterial, fungal, and nematode datasets, respectively. The sequencing data were deposited DNA Data Bank of Japan (DDBJ) (accession no.: DRA014170).
Statistical analysis
To examine how ASV richness increased with increasing number of sequencing reads, the iNEXT v.2.0.20 package of R for drawing the interpolation and extrapolation curves was used (Hsieh et al., 2016). The Hill numbers of bacterial, fungal, and nematode ASVs were then calculated for each soil-amount class of each soil sample. To compare the diversity of each sample, Hill numbers (taxonomic richness, Shannon diversity and Simpson diversity) were calculated based on interpolation and extrapolation with 0.999 sample coverage using the estimateD function in the iNEXT package in R. Based on the Hill number data, the α-diversity of bacteria, fungi, and nematodes were, respectively, examined in ANOVA models including ecosystem type (forest or soybean field), soil-amount class, and the interaction term of them as explanatory variables. Multiple comparison was then performed with Tukey’s HSD test. The analysis was conducted for the community datasets at the ASV, genus, family and order levels. Note that the results of the ANOVA were consistent irrespective of with and without the coverage-based rarefaction mentioned above.
For each of the bacterial, fungal, and nematode datasets, the ASV, genus, family and order-level taxonomic compositions of each sample was visualized as bar graphs after performing the rarefaction of the data using the vegan v.2.5.7 package of R (1,000 reads per sample). To compare the levels of among-sample heterogeneity of community structure among bacteria, fungi, and nematodes, we calculated the β-diversity (Bray-Curtis dissimilarity) of ASV, genus, family and order level taxonomic compositions among replicate samples at each soil amount class. At each taxonomic level, we constructed an ANOVA including among-sample β-diversity as a response variable and ecosystem type (forest or soybean field), soil-amount class, and the interaction between them as explanatory variables. Multiple comparison was then performed with Tukey’s HSD test.
Results
In total, 4,000 bacterial (220,607 reads), 1,622 fungal (148,058 reads), and 701 nematode (169,073 reads) ASVs were detected across the samples. The number of bacterial, fungal, and nematode genera were 234, 221, and 48, respectively. Likewise, the number of bacterial, fungal, and nematode families were 171, 158, and 38, respectively. The number of bacterial, fungal and nematode order were 151, 88, and 10, respectively. For all the three organismic groups, interpolation and extrapolation curve were saturated: sample coverage was higher than 0.9 in all the analyzed samples (Supplementary Figure 1). α-diversity of the three organismal groups are shown in Figure 1. The taxonomic compositions of three organismic groups in each soil amount setting were visualized as well (Figures 2–4).
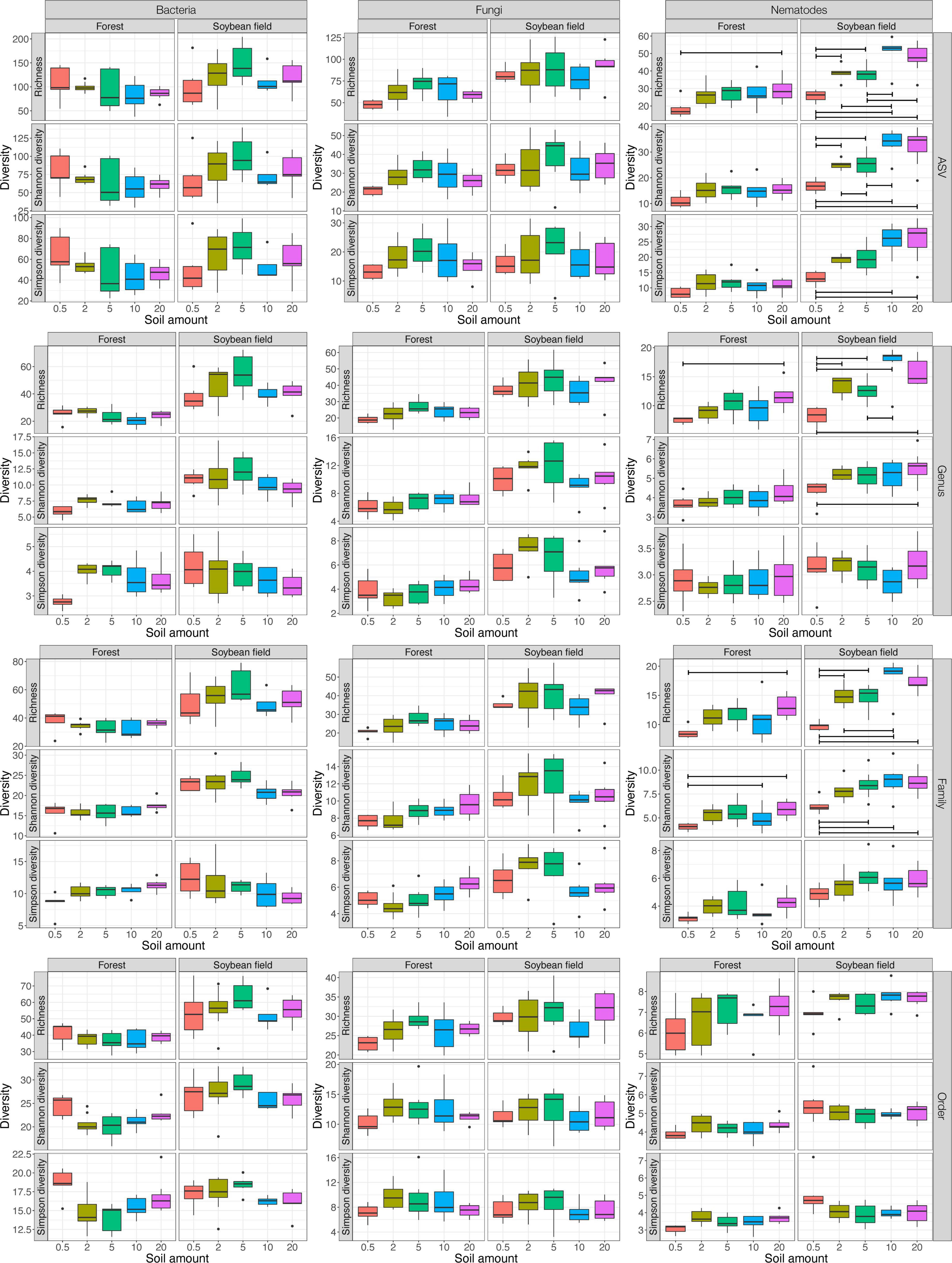
Figure 1. Effects of soil amount in DNA extraction on α-diversity estimates. For each of the bacterial 16S rRNA, fungal ITS, and nematode 18S rRNA datasets, taxonomic richness, Shannon diversity, and Simpson diversity are shown with boxplots at the ASV, genus, family, and order levels, respectively. See Table 1 for ANOVA results. For each ecosystem type (forest or soybean field), statistically significant difference inferred by Tukey’s HSD test is indicated by horizontal bars within the panels (P < 0.05).
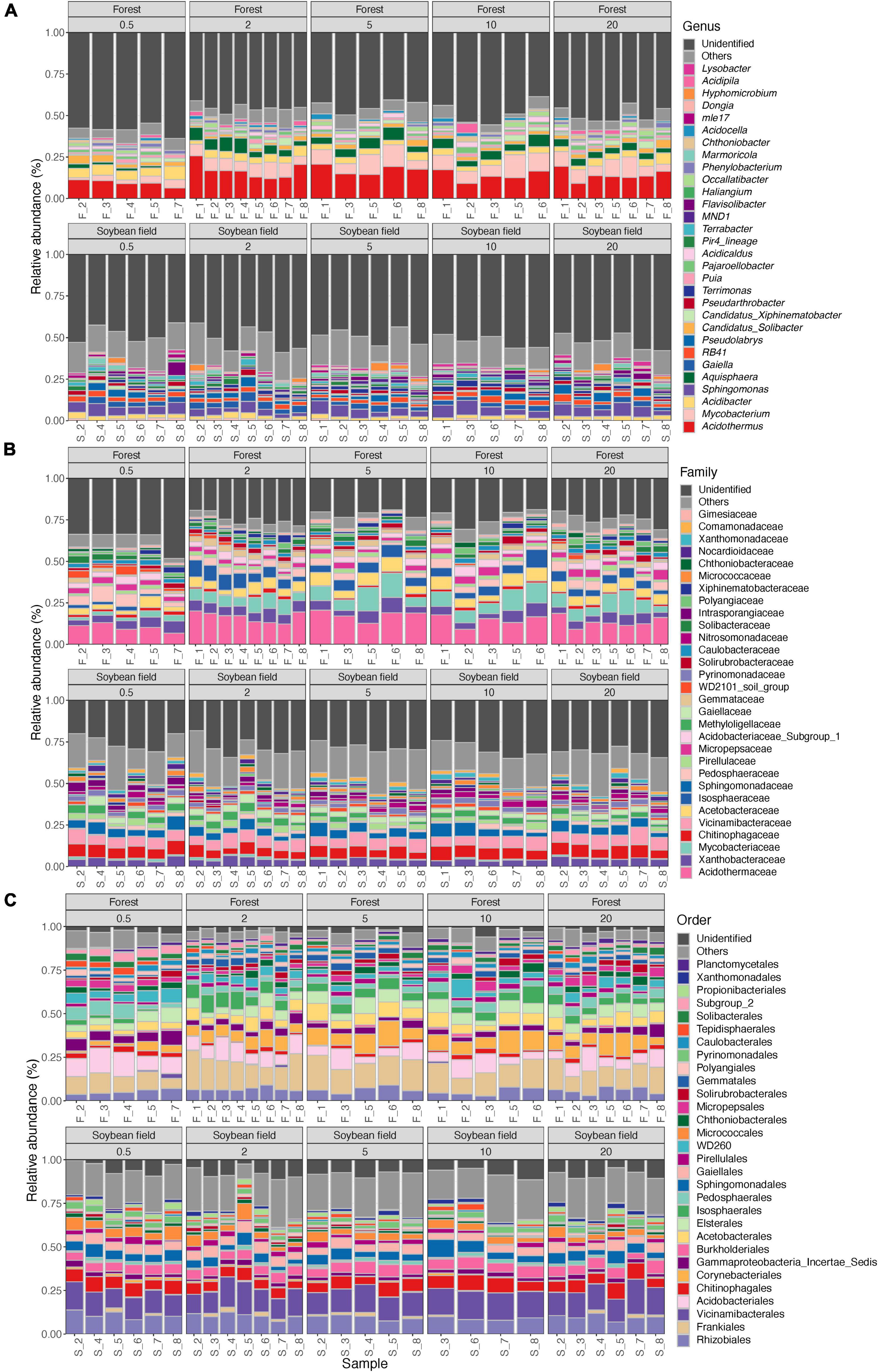
Figure 2. Community compositions of bacteria at the genus (A), family (B), and order (C) levels. The alphabets indicating source ecosystem type (“F_,” forest; “S_,” soybean field) are followed by replicate sample numbers. Samples with less than 1,000 sequencing reads were omitted.
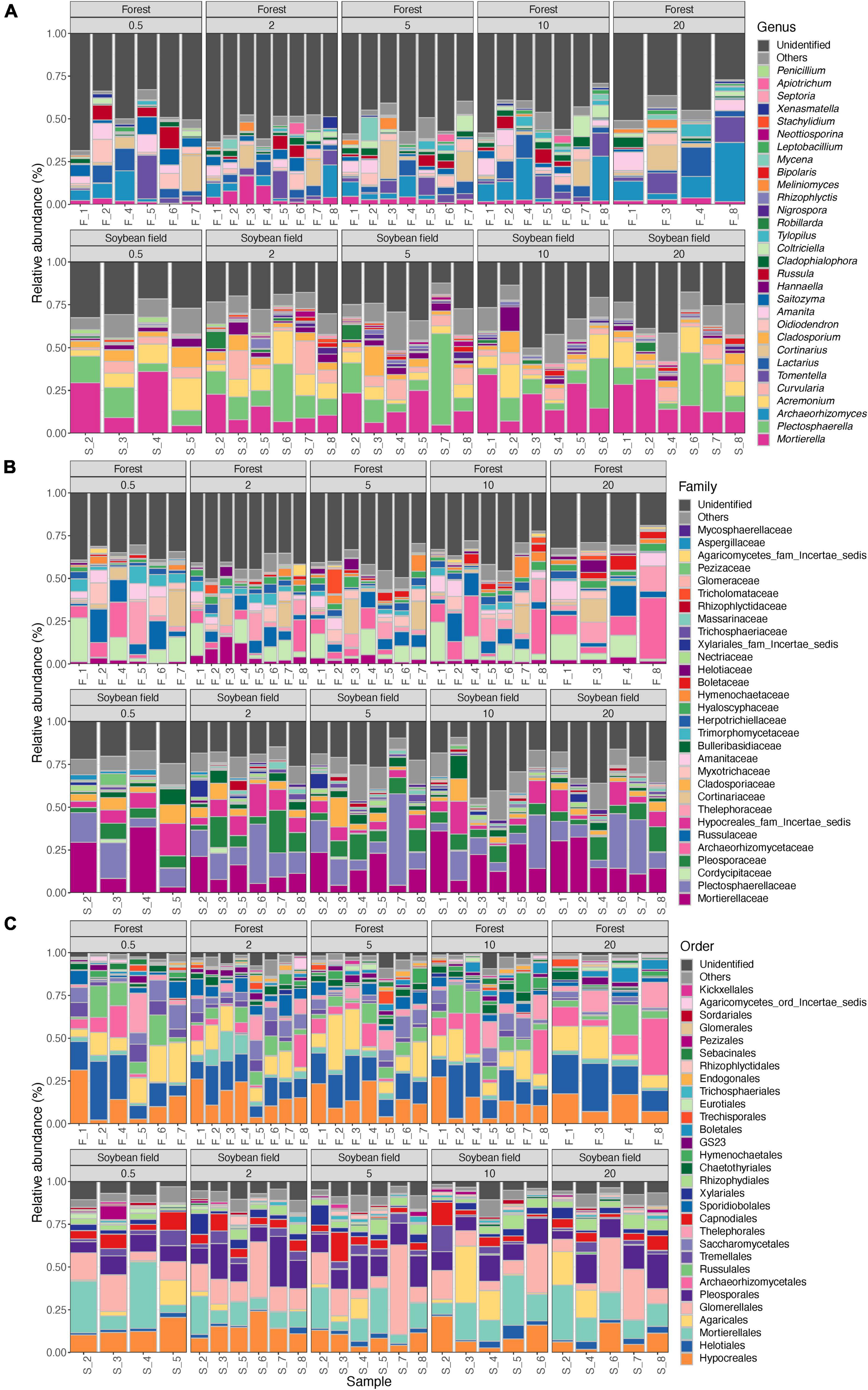
Figure 3. Community compositions of fungi at the genus (A), family (B), and order (C) levels. The alphabets indicating source ecosystem type (“F_,” forest; “S_,” soybean field) are followed by replicate sample numbers. Samples with less than 1,000 sequencing reads were omitted.
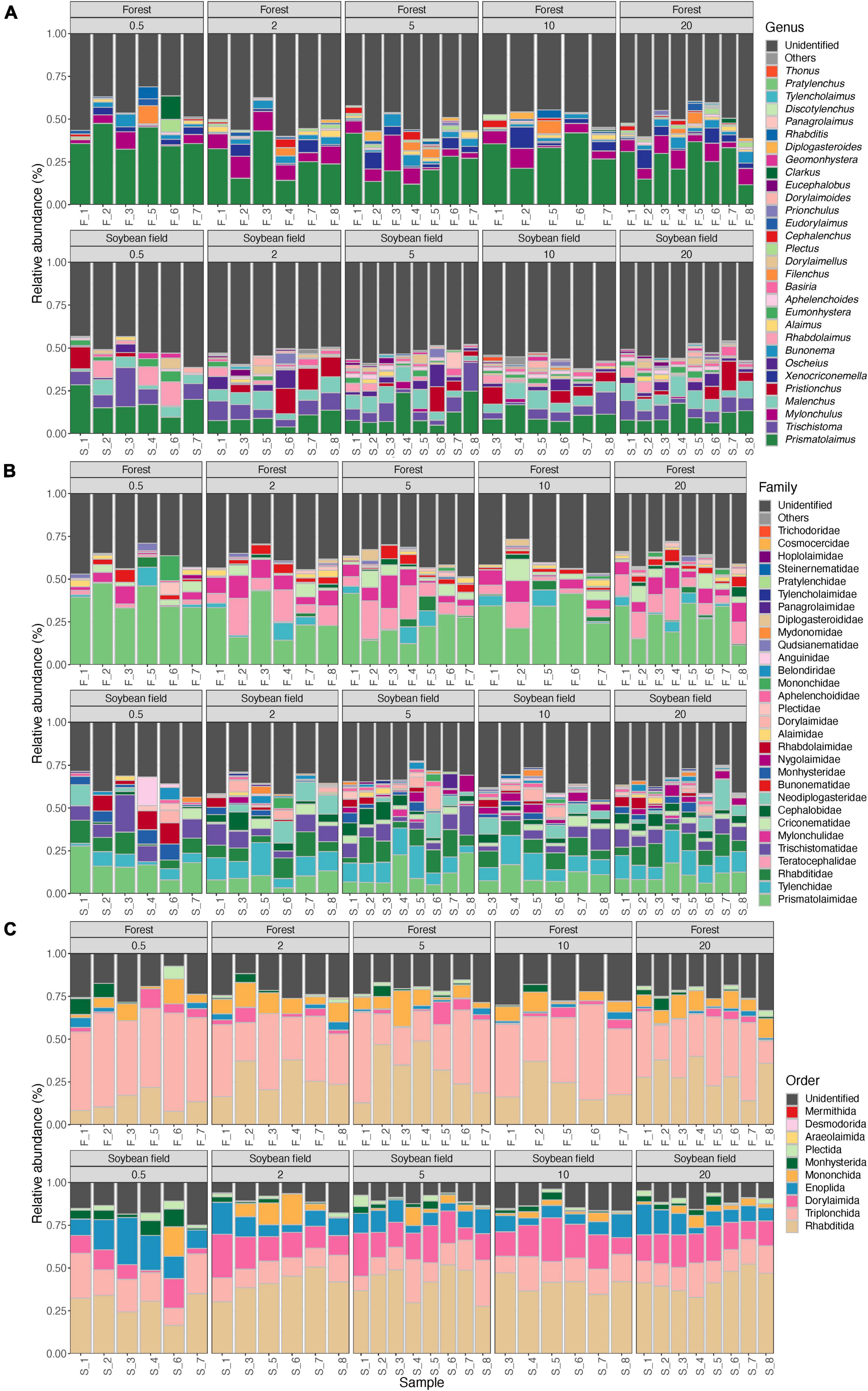
Figure 4. Community compositions of nematodes at the genus (A), family (B), and order (C) levels. The alphabets indicating source ecosystem type (“F_,” forest; “S_,” soybean field) are followed by replicate sample numbers. Samples with less than 1,000 sequencing reads were omitted.
For bacteria and fungi, soil amount in DNA extraction did not have significant effects on the α-diversity estimates at the ASV, genus, family, and order levels (Table 1; Supplementary Table 1 and Figure 1). Meanwhile, the interaction between ecosystem type (forest or soybean field) and soil amount had significant effects on the Shannon and Simpson diversity of bacteria at the genus, family and order levels (Table 1 and Supplementary Table 1). This result suggests that the effects of soil amount varied between ecosystem types, although combined effects of soil amount and ecosystem type were difficult to interpret.
In the nematode dataset, significant effects of soil amount on taxonomic richness, Shannon diversity, and Simpson diversity were observed at the ASV and family levels (Table 1; Supplementary Table 1). Likewise, soil amount had significant effects on taxonomic richness and Shannon diversity at the genus level (Supplementary Table 1). Meanwhile, such effects of soil amount on α-diversity index values were not observed at the order level (Supplementary Table 1). At the ASV, genus, and family levels Tukey’s HSD test indicated that α-diversity scores had higher values at 20 g than at 0.5 g soil DNA extraction settings in more than half of the combinations of ecosystem type and α-diversity indices (Figure 1).
The level of dissimilarity in bacterial community compositions among replicate samples significantly varied depending on the soil amount settings at the ASV, but not at the genus, family and order levels (Table 2; Supplementary Table 2 and Figure 5). In the Tukey’s HSD test, among-sample community dissimilarity of bacteria was lowest at 10 g among the soil amount settings in the soybean field data (Figure 5). For fungi, dependence of community dissimilarity level on the soil amount settings was observed at the ASV, genus, and order levels (Table 2 and Supplementary Table 2), although the pattern was inconsistent between the forest and soybean field ecosystems (Figure 5). Likewise, the level of among-replicate dissimilarity in nematode community compositions was observed at all the examined taxonomic levels (Table 2; Supplementary Table 2 and Figure 5) and there was significant difference among different soil amount in ANOVA test (Table 2). Moreover, Tukey’s HSD test indicate that the community composition dissimilarity had significantly lower values at 10 and 20 g soil amount setting (Figure 5).
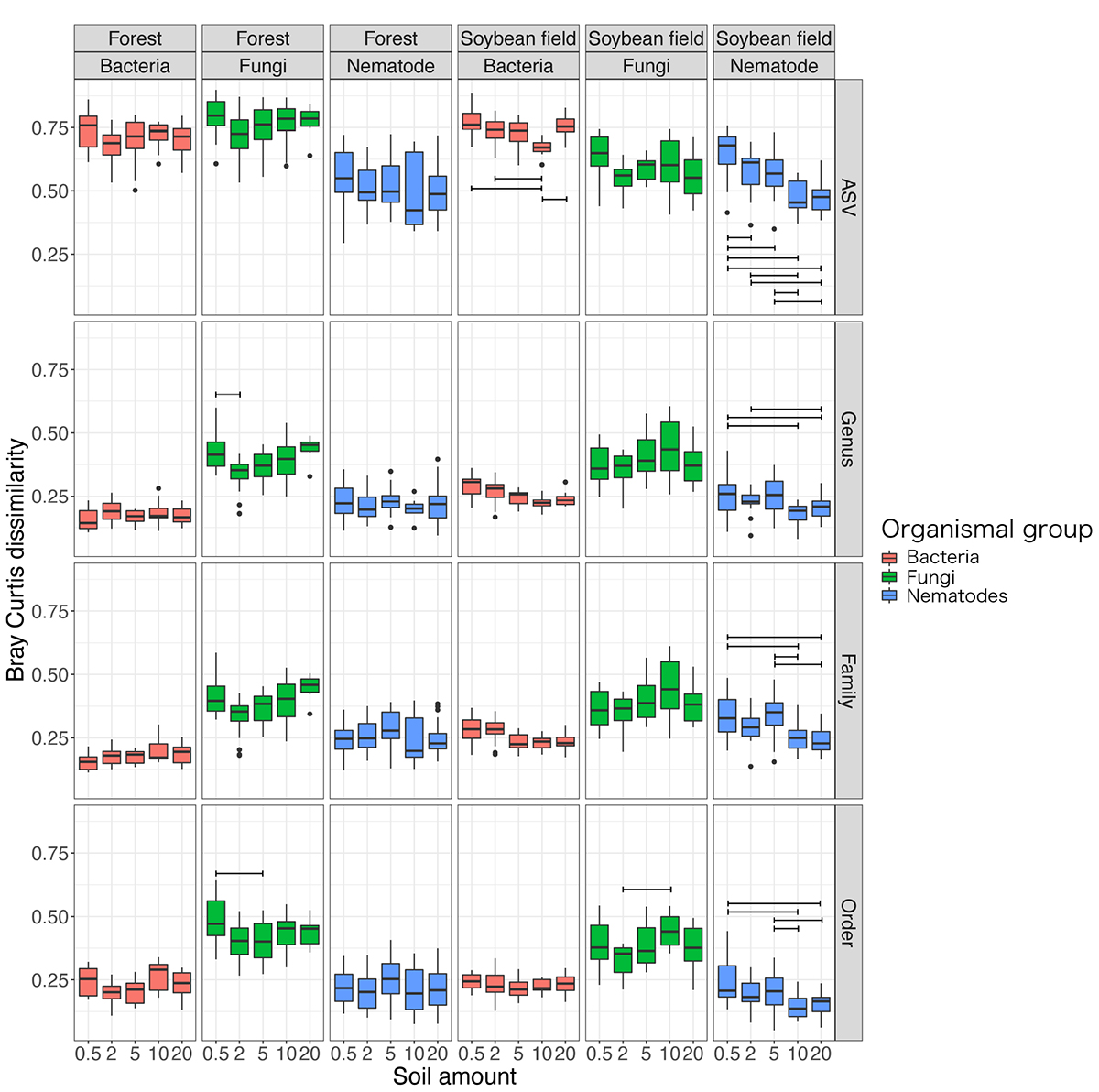
Figure 5. Dissimilarity of community compositions among replicate samples. For each organismal group (bacteria, fungi, and nematodes) in each ecosystem type (forest or soybean field), Bray-Curtis dissimilarity (β-diversity) among replicate samples are shown at each of the ASV, genus, family, and order levels. An ANOVA analysis testing potential effects of soil amount in DNA extraction on β-diversity estimates was performed for each panel. See Table 2 and Supplementary Table 2 for the results of the ANOVAs in which we included soil amount, ecosystem type (forest or soybean field), and the interaction of the two variables.
The among-replicate community dissimilarity, which represented heterogeneity of community structure, varied depending also on organismal groups (Figure 5). Specifically, fungi showed the highest level of among-sample community heterogeneity at the genus, family, and order levels. At the ASV level, bacteria showed comparable and higher among-sample community heterogeneity in the forest and soybean field ecosystems, respectively (Figure 5). Such heterogeneity level of community structure was moderate for nematodes (Figure 5).
Discussion
We here examined how the amount of soil subjected to DNA extraction affects the results of biodiversity surveys targeting three major groups of soil organisms, namely, bacteria, fungi, and nematodes. We then found that DNA metabarcoding protocols optimized for bacteria (and fungi) could result in potential biases in the estimation of α- and β-diversity of nematodes in the soil as detailed below.
For bacteria and fungi, there have been several studies evaluating potential effects of soil sample amount on DNA metabarcoding-based biodiversity inventories. Among those studies, some suggested that 10 g of soil were required to gain reproducible ASV richness data (Kang and Mills, 2006; Penton et al., 2016), while others concluded smaller amounts of soil were enough for surveys of bacteria and fungi (Ranjard et al., 2003; Song et al., 2015). In our study, the amount of soil subjected to DNA extraction did not directly affect the α-diversity estimates (i.e., taxonomic richness, Shannon diversity, and Simpson diversity) of bacteria and fungi (Table 1; Supplementary Table 1 and Figure 1). Therefore, we conclude that DNA extraction from 0.5 g of soil and that from larger amounts of soil can yield qualitatively similar metabarcoding results in the analyses targeting bacteria and fungi. Meanwhile, taking into account the previous studies reporting potential bias related to DNA extraction from small amounts of soil (Kang and Mills, 2006; Penton et al., 2016), careful management of experimental protocols would be required in DNA extraction from 0.5 g of soil.
While there have been a number of methodological studies on bacterial and fungal DNA metabarcoding, few studies have been conducted to examine the effects of soil sample amount on nematode diversity surveys. In research on soil nematode communities, the traditional approach is to isolate nematodes from the soil matrix by means of the Baermann method, elutriator sucrose centrifugation, and filter separation, followed by microscopic morphological observations (Neher et al., 1995; Yeates et al., 1999; Ferris et al., 2012). This approach is usually taken based on sampling of several hundred grams of soil. Meanwhile, in recent years, DNA-based techniques have been recognized as an alternative approach and nematode community inventories from smaller amount of soil have started to be examined (Wiesel et al., 2015; Treonis et al., 2018; Quist et al., 2019; Zhang et al., 2021).
Our results indicated that soil amount in DNA extraction could considerably affect DNA metabarcoding results of nematodes α-diversity and community composition (Table 1; Supplementary Table 1 and Figure 1). All the α-diversity estimates increased with increasing soil amount, reaching maximum values at 10 g at the ASV, genus, and family levels (Figure 1). These results indicate that biodiversity surveys of nematodes require higher amounts of soil than those of bacteria and fungi. This finding is as expected because nematodes [typically, from 0.1 to 2.5 mm long (Hodda, 2022)] are much larger than bacterial cells and fungal hyphae: thus, their population density in the soil may be much lower than microbes. Even if “environmental DNA” derived from feces or remains of nematodes are detectable simultaneously with DNA from living nematode bodies, the scale of soil samples required for diversity inventories would be larger in nematodes than in bacteria and fungi (Peham et al., 2017). Given our data, we would suggest mixing as much soil as possible and then introducing subsampled 20 g of soil to lysis solutions for DNA extraction. Ultimately, the use of more soil in the lysis step would be ideal. However, 20 g may be the upper limit of molecular biological experiments given the size of centrifugeable tubes (≦ 50 ml). Consequently, including replicate sampling points within each study site is of particular importance in nematode DNA barcoding even if DNA extraction is performed at the 20 g soil scale.
The results of the DNA metabarcoding also indicated that the level of dissimilarity (β-diversity) among replicate samples could vary depending on soil amount settings in DNA extraction, especially in nematodes (Table 2; Supplementary Table 2 and Figure 5). Basically, DNA extraction from small amount of soil is expected to result in higher dissimilarity among replicate samples because inferred community structure is more likely to be influenced by stochastic factors. The expected decrease in β-diversity with increasing soil amount was observed in at the ASV level in the soybean filed ecosystem for bacteria and nematode community (Figure 5). Thus, DNA extraction from 20 g of soil is recommend for analyses including nematodes as target organisms with regard to both α- and β-diversity surveys.
Although the above results are informative, it should be acknowledged that the optimal amount of soil for DNA metabarcoding potentially depend on soil and ecosystem types. For example, the level of among-sample heterogeneity in fungal community structure can vary between grassland ecosystems and farmlands with continuous anthropogenic perturbations (e.g., hyphal disconnection by tillage). In addition, it has been reported that soil nematode density varies across continents depending on soil edaphic factors (van den Hoogen et al., 2019). Thus, for processing soil samples with highly heterogeneous community structure or low organismal densities, mechanical approaches for systematically mixing large amounts of soil before DNA extraction need to be developed in future studies as direct DNA extraction from soil.
Overall, we found that the standard DNA metabarcoding protocols developed for bacteria are not directly applicable to multicellular organisms in terms of the soil amount subjected to DNA extraction. Given the results on α- and β-diversity estimates, we conclude that DNA extraction from 20 g of soil is necessary for simultaneously investigating the biodiversity of bacteria, fungi, and nematodes in below-ground ecosystems. Because DNA-based inventories of biodiversity have become major tools in ecology, a next important step may be integration and comparison of diversity patterns among different groups of organisms. Toward the thorough understanding of metacommunity-scale dynamics and macroecological patterns of soil biodiversity, further attempts of technical standardization are necessary to target whole biomes including not only bacteria, fungi, and nematodes but also other invertebrates and protists.
Data availability statement
The datasets presented in this study can be found in online repositories. The names of the repository/repositories and accession number(s) can be found below: https://www.ddbj.nig.ac.jp/, DRA014170.
Author contributions
TK and HT designed the work and wrote the manuscript. TK carried out the experiments and analyzed the data. Both authors contributed to the article and approved the submitted version.
Funding
This work was financially supported by JSPS Grant-in-Aid for Scientific Research (18H04009), JST FOREST (JPMJFR2048), and NEDO Moonshot Research and Development Program (JPNP18016) to HT.
Acknowledgments
We thank reviewers for their helpful comments that improved the manuscript.
Conflict of interest
The authors declare that the research was conducted in the absence of any commercial or financial relationships that could be construed as a potential conflict of interest.
Publisher’s note
All claims expressed in this article are solely those of the authors and do not necessarily represent those of their affiliated organizations, or those of the publisher, the editors and the reviewers. Any product that may be evaluated in this article, or claim that may be made by its manufacturer, is not guaranteed or endorsed by the publisher.
Supplementary material
The Supplementary Material for this article can be found online at: https://www.frontiersin.org/articles/10.3389/fevo.2022.959945/full#supplementary-material
Footnotes
References
Ahmed, M., Back, M. A., Prior, T., Karssen, G., Lawson, R., Adams, I., et al. (2019). Metabarcoding of soil nematodes: The importance of taxonomic coverage and availability of reference sequences in choosing suitable marker(s). Metabarcoding Metagenom. 3, 37–99. doi: 10.3897/mbmg.3.36408
Apprill, A., Mcnally, S., Parsons, R., and Weber, L. (2015). Minor revision to V4 region SSU rRNA 806R gene primer greatly increases detection of SAR11 bacterioplankton. Aquat. Microb. Ecol. 75, 129–137. doi: 10.3354/ame01753
Bahram, M., Hildebrand, F., Forslund, S. K., Anderson, J. L., Soudzilovskaia, N. A., Bodegom, P. M., et al. (2018). Structure and function of the global topsoil microbiome. Nature 560, 233–237. doi: 10.1038/s41586-018-0386-6
Bardgett, R. D., and van der Putten, W. H. (2014). Belowground biodiversity and ecosystem functioning. Nature 515, 505–511. doi: 10.1038/nature13855
Bar-On, Y. M., Phillips, R., and Milo, R. (2018). The biomass distribution on Earth. Proc. Natl. Acad. Sci. U.S.A. 115, 6506–6511. doi: 10.1073/pnas.1711842115
Bonfante, P., and Anca, I. A. (2009). Plants, mycorrhizal fungi, and bacteria: A network of interactions. Annu. Rev. Microbiol. 63, 363–383. doi: 10.1146/annurev.micro.091208.073504
Bongers, T. (1990). The maturity index: An ecological measure of environmental disturbance based on nematode species composition. Oecologia 83, 14–19. doi: 10.1007/BF00324627
Bongers, T., and Bongers, M. (1998). Functional diversity of nematodes. Appl. Soil Ecol. 10, 239–251. doi: 10.1016/S0929-1393(98)00123-1
Bongers, T., and Ferris, H. (1999). Nematode community structure as a bioindicator in environmental monitoring. Trends Ecol. Evol. 14, 224–228. doi: 10.1016/S0169-5347(98)01583-3
Callahan, B. J., McMurdie, P. J., Rosen, M. J., Han, A. W., Johnson, A. J. A., and Holmes, S. P. (2016). DADA2: High-resolution sample inference from Illumina amplicon data. Nat. Methods 13, 581–583. doi: 10.1038/nmeth.3869
Caporaso, J. G., Lauber, C. L., Walters, W. A., Berg-Lyons, D., Huntley, J., Fierer, N., et al. (2012). Ultra-high-throughput microbial community analysis on the Illumina HiSeq and MiSeq platforms. ISME J. 6, 1621–1624. doi: 10.1038/ismej.2012.8
Caporaso, J. G., Lauber, C. L., Walters, W. A., Berg-Lyons, D., Lozupone, C. A., Turnbaugh, P. J., et al. (2011). Global patterns of 16S rRNA diversity at a depth of millions of sequences per sample. Proc. Natl. Acad. Sci. U.S.A. 108, 4516–4522. doi: 10.1073/pnas.1000080107
Detrey, J., Cognard, V., Djian-Caporalino, C., Marteu, N., Doidy, J., Pourtau, N., et al. (2022). Growth and root-knot nematode infection of tomato are influenced by mycorrhizal fungi and earthworms in an intercropping cultivation system with leeks. Appl. Soil Ecol. 169:104181. doi: 10.1016/j.apsoil.2021.104181
Ellingsøe, P., and Johnsen, K. (2002). Influence of soil sample sizes on the assessment of bacterial community structure. Soil Biol. Biochem. 34, 1701–1707. doi: 10.1016/S0038-0717(02)00156-6
Ettema, C. H. (1998). Soil nematode diversity: Species coexistence and ecosystem function. J. Nematol. 30, 159–169.
Ferris, H., Mullens, T. A., and Foord, K. E. (1990). Stability and characteristics of spatial description parameters for nematode populations. J. Nematol. 22, 427–439.
Ferris, H., Sánchez-Moreno, S., and Brennan, E. B. (2012). Structure, functions and interguild relationships of the soil nematode assemblage in organic vegetable production. Appl. Soil Ecol. 61, 16–25. doi: 10.1016/j.apsoil.2012.04.006
Fierer, N. (2017). Embracing the unknown: Disentangling the complexities of the soil microbiome. Nat. Rev. Microbiol. 15, 579–590. doi: 10.1038/nrmicro.2017.87
Fierer, N., Bradford, M. A., and Jackson, R. B. (2007). Toward an ecologica classification of soil bacteria. Ecology 88, 1354–1364. doi: 10.1890/05-1839
Flemming, H. C., and Wuertz, S. (2019). Bacteria and archaea on Earth and their abundance in biofilms. Nat. Rev. Microbiol. 17, 247–260. doi: 10.1038/s41579-019-0158-9
Francioli, D., Lentendu, G., Lewin, S., and Kolb, S. (2021). DNA metabarcoding for the characterization of terrestrial microbiota—pitfalls and solutions. Microorganisms 9:361. doi: 10.3390/microorganisms9020361
Franklin, R. B., and Mills, A. L. (2003). Multi-scale variation in spatial heterogeneity for microbial community structure in an eastern Virginia agricultural field. FEMS Microbiol. Ecol. 44, 335–346. doi: 10.1016/S0168-6496(03)00074-6
Gilbert, J. A., Jansson, J. K., and Knight, R. (2014). The Earth microbiome project: Successes and aspirations. BMC Biol. 12:69. doi: 10.1186/s12915-014-0069-1
Hamady, M., Walker, J. J., Harris, J. K., Gold, N. J., and Knight, R. (2008). Error-correcting barcoded primers for pyrosequencing hundreds of samples in multiplex. Nat. Methods 5, 235–237. doi: 10.1038/nmeth.1184
Hodda, M. (2022). Phylum Nematoda: A classification, catalogue and index of valid genera, with a census of valid species. Zootaxa 5114, 1–289. doi: 10.11646/zootaxa.5114.1.1
Hsieh, T. C., Ma, K. H., and Chao, A. (2016). iNEXT: An R package for rarefaction and extrapolation of species diversity (Hill numbers). Methods Ecol. Evol. 7, 1451–1456. doi: 10.1111/2041-210X.12613
Huson, D. H., Auch, A. F., Qi, J., and Schuster, S. C. (2007). MEGAN analysis of metagenomic data. Genome Res. 17, 377–386. doi: 10.1101/gr.5969107
Kang, S., and Mills, A. L. (2006). The effect of sample size in studies of soil microbial community structure. J. Microbiol. Methods 66, 242–250. doi: 10.1016/j.mimet.2005.11.013
Kawanobe, M., Toyota, K., and Ritz, K. (2021). Development and application of a DNA metabarcoding method for comprehensive analysis of soil nematode communities. Appl. Soil Ecol. 166:103974. doi: 10.1016/j.apsoil.2021.103974
Kenmotsu, H., Ishikawa, M., Nitta, T., Hirose, Y., and Eki, T. (2021). Distinct community structures of soil nematodes from three ecologically different sites revealed by high-throughput amplicon sequencing of four 18S ribosomal RNA gene regions. PLoS One 16:e0249571. doi: 10.1371/journal.pone.0249571
Klindworth, A., Pruesse, E., Schweer, T., Peplies, J., Quast, C., Horn, M., et al. (2013). Evaluation of general 16S ribosomal RNA gene PCR primers for classical and next-generation sequencing-based diversity studies. Nucleic Acids Res. 41:e1. doi: 10.1093/nar/gks808
Lindahl, B. D., Nilsson, R. H., Tedersoo, L., Abarenkov, K., Carlsen, T., Kjøller, R., et al. (2013). Fungal community analysis by high-throughput sequencing of amplified markers–a user’s guide. New Phytol. 199, 288–299. doi: 10.1111/nph.12243
Liu, T., Hu, F., and Li, H. (2019). Spatial ecology of soil nematodes: Perspectives from global to micro scales. Soil Biol. Biochem. 137:107565. doi: 10.1016/j.soilbio.2019.107565
Lundberg, D. S., Yourstone, S., Mieczkowski, P., Jones, C. D., and Dangl, J. L. (2013). Practical innovations for high-throughput amplicon sequencing. Nat. Methods 10, 999–1002. doi: 10.1038/nmeth.2634
Martin-Laurent, F., Philippot, L., Hallet, S., Chaussod, R., Germon, J. C., Soulas, G., et al. (2001). DNA extraction from soils: Old bias for new microbial diversity analysis methods. Appl. Environ. Microbiol. 67, 2354–2359. doi: 10.1128/AEM.67.5.2354-2359.2001
Neher, D. A., Peck, S. L., Rawlings, J. O., and Campbell, C. L. (1995). Measures of nematode community structure and sources of variability among and within agricultural fields. Plant Soil 170, 167–181. doi: 10.1007/BF02183065
Nielsen, U. N., Wall, D. H., and Six, J. (2015). Soil biodiversity and the environment. Annu. Rev. Environ. Resour. 40, 63–90. doi: 10.1146/annurev-environ-102014-021257
Nilsson, R. H., Larsson, K. H., Taylor, A. F. S., Bengtsson-Palme, J., Jeppesen, T. S., Schigel, D., et al. (2019). The UNITE database for molecular identification of fungi: Handling dark taxa and parallel taxonomic classifications. Nucleic Acids Res. 47, D259–D264. doi: 10.1093/nar/gky1022
Op De Beeck, M., Lievens, B., Busschaert, P., Declerck, S., Vangronsveld, J., and Colpaert, J. V. (2014). Comparison and validation of some ITS primer pairs useful for fungal metabarcoding studies. PLoS One 9:e97629. doi: 10.1371/journal.pone.0097629
Peham, T., Steiner, F. M., Schlick-Steiner, B. C., and Arthofer, W. (2017). Are we ready to detect nematode diversity by next generation sequencing? Ecol. Evol. 7, 4147–4151. doi: 10.1002/ece3.2998
Penton, C. R., Gupta, V. V. S. R., Yu, J., and Tiedje, J. M. (2016). Size matters: Assessing optimum soil sample size for fungal and bacterial community structure analyses using high throughput sequencing of rRNA gene amplicons. Front. Microbiol. 7:824. doi: 10.3389/fmicb.2016.00824
Quast, C., Pruesse, E., Yilmaz, P., Gerken, J., Schweer, T., Yarza, P., et al. (2013). The SILVA ribosomal RNA gene database project: Improved data processing and web-based tools. Nucleic Acids Res. 41, D590–D596. doi: 10.1093/nar/gks1219
Quist, C. W., Gort, G., Mooijman, P., Brus, D. J., van den Elsen, S., Kostenko, O., et al. (2019). Spatial distribution of soil nematodes relates to soil organic matter and life strategy. Soil Biol. Biochem. 136:107542. doi: 10.1016/j.soilbio.2019.107542
R Core Team (2021). R: A language and environment statistical computing. Vienna: R Foundation for Statistical Computing.
Ranjard, L., Lejon, D. P. H., Mougel, C., Schehrer, L., Merdinoglu, D., and Chaussod, R. (2003). Sampling strategy in molecular microbial ecology: Influence of soil sample size on DNA fingerprinting analysis of fungal and bacterial communities. Environ. Microbiol. 5, 1111–1120. doi: 10.1046/j.1462-2920.2003.00521.x
Reuter, J. A., Spacek, D. V., and Snyder, M. P. (2015). High-throughput sequencing technologies. Mol. Cell 58, 586–597. doi: 10.1016/j.molcel.2015.05.004
Sapkota, R., and Nicolaisen, M. (2015). High-throughput sequencing of nematode communities from total soil DNA extractions. BMC Ecol. 15:3. doi: 10.1186/s12898-014-0034-4
Schoch, C. L., Seifert, K. A., Huhndorf, S., Robert, V., Spouge, J. L., Levesque, C. A., et al. (2012). Nuclear ribosomal internal transcribed spacer (ITS) region as a universal DNA barcode marker for Fungi. Proc. Natl. Acad. Sci. U.S.A. 109, 6241–6246. doi: 10.1073/pnas.1117018109
Sikder, M. M., Vestergård, M., Sapkota, R., Kyndt, T., and Nicolaisen, M. (2020). Evaluation of metabarcoding primers for analysis of soil nematode communities. Diversity 12:388. doi: 10.3390/d12100388
Smith, D. P., and Peay, K. G. (2014). Sequence depth, not PCR replication, improves ecological inference from next generation DNA sequencing. PLoS One 9:e90234. doi: 10.1371/journal.pone.0090234
Song, Z., Schlatter, D., Kennedy, P., Kinkel, L. L., Kistler, H. C., Nguyen, N., et al. (2015). Effort versus reward preparing samples for fungal community characterization in high-throughput sequencing surveys of soils. PLoS One 10:e0127234. doi: 10.1371/journal.pone.0127234
Stevens, J. L., Jackson, R. L., and Olson, J. B. (2013). Slowing PCR ramp speed reduces chimera formation from environmental samples. J. Microbiol. Methods 93, 203–205. doi: 10.1016/j.mimet.2013.03.013
Tanabe, A. (2022). Claident v0.9.2022.01.26. Available online at: https://github.com/astanabe/Claident (accessed May 19, 2022).
Tanabe, A. S., and Toju, H. (2013). Two new computational methods for universal DNA barcoding: A benchmark using barcode sequences of bacteria, archaea, animals, fungi, and land plants. PLoS One 8:e76910. doi: 10.1371/journal.pone.0076910
Tedersoo, L. Sánchez-Ramírez, S. Kõljalg, U., Bahram, M. Döring, M., Schigel, D., et al. (2018). High-level classification of the Fungi and a tool for evolutionary ecological analyses. Fungal Divers 90, 135–159. doi: 10.1007/s13225-018-0401-0
Thijs, S., de Beeck, M. O., Beckers, B., Truyens, S., Stevens, V., van Hamme, J. D., et al. (2017). Comparative evaluation of four bacteria-specific primer pairs for 16S rRNA gene surveys. Front. Microbiol. 8:494. doi: 10.3389/fmicb.2017.00494
Thompson, L. R., Sanders, J. G., McDonald, D., Amir, A., Ladau, J., Locey, K. J., et al. (2017). A communal catalogue reveals Earth’s multiscale microbial diversity. Nature 551, 457–463. doi: 10.1038/nature24621
Toju, H., Kurokawa, H., and Kenta, T. (2019). Factors influencing leaf- and root-associated communities of bacteria and fungi across 33 plant orders in a grassland. Front. Microbiol. 10:241. doi: 10.3389/fmicb.2019.00241
Toju, H., Tanabe, A. S., Yamamoto, S., and Sato, H. (2012). High-coverage ITS primers for the DNA-based identification of ascomycetes and basidiomycetes in environmental samples. PLoS One 7:e40863. doi: 10.1371/journal.pone.0040863
Toju, H., and Tanaka, Y. (2019). Consortia of anti-nematode fungi and bacteria in the rhizosphere of soybean plants attacked by root-knot nematodes. R. Soc. Open Sci. 6:181693. doi: 10.1098/rsos.181693
Treonis, A. M., Unangst, S. K., Kepler, R. M., Buyer, J. S., Cavigelli, M. A., Mirsky, S. B., et al. (2018). Characterization of soil nematode communities in three cropping systems through morphological and DNA metabarcoding approaches. Sci. Rep. 8:2004. doi: 10.1038/s41598-018-20366-5
van den Hoogen, J., Geisen, S., Routh, D., Ferris, H., Traunspurger, W., Wardle, D. A., et al. (2019). Soil nematode abundance and functional group composition at a global scale. Nature 572, 194–198. doi: 10.1038/s41586-019-1418-6
Waeyenberge, L., de Sutter, N., Viaene, N., and Haegeman, A. (2019). New insights into nematode DNA-metabarcoding as revealed by the characterization of artificial and spiked nematode communities. Diversity 11:52. doi: 10.3390/d11040052
Wall, D. H., Nielsen, U. N., and Six, J. (2015). Soil biodiversity and human health. Nature 528, 69–76. doi: 10.1038/nature15744
Wiesel, L., Daniell, T. J., King, D., and Neilson, R. (2015). Determination of the optimal soil sample size to accurately characterise nematode communities in soil. Soil Biol. Biochem. 80, 89–91. doi: 10.1016/j.soilbio.2014.09.026
Yeates, G. W., Wardle, D. A., and Watson, R. N. (1999). Responses of soil nematode populations, community structure, diversity and temporal variability to agricultural intensification over a seven-year period. Soil Biol. Biochem. 31, 1721–1733. doi: 10.1016/S0038-0717(99)00091-7
Zhang, Y., Ji, L., and Yang, L. (2021). Abundance and diversity of soil nematode community at different altitudes in cold-temperate montane forests in northeast China. Glob. Ecol. Conserv. 29:e01717. doi: 10.1016/j.gecco.2021.e01717
Keywords: biodiversity, DNA extraction, DNA barcoding, high-throughput sequencing, macroecology, microbiomes, soil nematodes, soil volume
Citation: Kageyama T and Toju H (2022) Effects of source sample amount on biodiversity surveys of bacteria, fungi, and nematodes in soil ecosystems. Front. Ecol. Evol. 10:959945. doi: 10.3389/fevo.2022.959945
Received: 03 June 2022; Accepted: 24 August 2022;
Published: 13 September 2022.
Edited by:
Lukasz Lech Stelinski, University of Florida, United StatesReviewed by:
Larry Wayne Duncan, University of Florida, United StatesDorota Porazinska, University of Florida, United States
Copyright © 2022 Kageyama and Toju. This is an open-access article distributed under the terms of the Creative Commons Attribution License (CC BY). The use, distribution or reproduction in other forums is permitted, provided the original author(s) and the copyright owner(s) are credited and that the original publication in this journal is cited, in accordance with accepted academic practice. No use, distribution or reproduction is permitted which does not comply with these terms.
*Correspondence: Takuya Kageyama, a2FnZXlhbWEudGFrdXlhLjg2ZkBzdC5reW90by11LmFjLmpw; Hirokazu Toju, dG9qdS5oaXJva2F6dS40Y0BreW90by11LmFjLmpw