- 1Department of Zoology, Faculty of Science, Palacký University Olomouc, Olomouc, Czechia
- 2Department of Ecology, Faculty of Science, Charles University, Prague, Czechia
- 3Department of Zoology, Faculty of Natural Science, Comenius University Bratislava, Bratislava, Slovakia
Disentangling regional and local drivers of species richness in communities is a long-term focus of ecology. Regional species pools affect local communities by providing their constituent species. Additionally, the amount and variety of resources enhance diversity locally. Here, we investigated whether the same ecological factor (vegetation complexity) shapes both regional and local species richness and thus drives local diversity both indirectly (via pool size) and directly (via facilitating the coexistence of species). We studied passerine birds of woodlands and forests in eastern Australia. We quantified regional species pool size and sampled local bird communities at 63 transects spanning 3,000 km. We estimated canopy height both regionally using satellite imagery and locally using vegetation sampling in the field. We studied how species pool size changed with regional canopy height and water availability, and how local species richness changed with pool size and local canopy height. Local species richness increased with both local canopy height and the size of the regional species pool. Pool size, in turn, increased with regional canopy height, which itself increased with water availability. Moreover, local species richness expressed as a proportion of the regional pool also increased with local canopy height. In sum, vegetation complexity indexed by canopy height had a doubly positive effect on local species richness: indirectly by promoting a large regional species pool and directly by facilitating the coexistence of disproportionately many species locally. Regional pools were larger in tall forests probably due to the legacy of extensive moist forests that once covered most of Australia, thus providing a sizeable potential for speciation, diversification, and species persistence. Local species richness was greater in tall, more productive forests with more vegetation layers likely due to more and varied resources (i.e., more potential niches), allowing the coexistence of more individuals and species of consumers.
Introduction
Identifying environmental drivers of local species richness in communities is a long-term focus of ecology (Hutchinson, 1959; MacArthur, 1965). Some species are filtered from local communities due to their limited tolerance to prevailing abiotic conditions (Mittelbach and Schemske, 2015). Simultaneously, interactions among species help determine the final composition of communities (HilleRisLambers et al., 2012), possibly modified by local environments (Song et al., 2020). According to this view, local ecological processes drive the number of coexisting species, with a decisive role played by abiotic tolerances and interspecific competition, both limiting the number of coexisting species (Mittelbach and McGill, 2019). Consequently, repeated patterns in the number and composition of coexisting species have been interpreted as a result of local ecological processes (Diamond, 1975; Weiher and Keddy, 1999).
However, ecologists recognized that large-scale historical processes, including speciation, extinction, and colonization, shape local communities (Ricklefs and Schluter, 1993; Vellend, 2016). More specifically, macroevolutionary and biogeographic processes shape the size and composition of a regional species pool, which then affects local communities in a top-down manner by providing constituent species for local communities (Cornell and Harrison, 2014). In support of this claim, many studies revealed strong positive correlations between local and regional species richness (Szava-Kovats et al., 2013). The shape of these relationships, either linear or saturating, was used to infer the relative contribution of local vs. regional processes in driving local species richness (Cornell and Lawton, 1992; Caley and Schluter, 1997; Srivastava, 1999). However, theoretical studies show that inferring underlying processes from the shape of the curve is difficult (He et al., 2005; Fox and Srivastava, 2006; reviewed in Olivares et al., 2018), which is true in ecology in general (McGill, 2003).
Consequently, attention shifted toward relating local species richness simultaneously to both regional species pool and local environmental conditions (Harrison and Cornell, 2008). Studies showed that both the size of species pool and local environment predicted local species richness in corals, plants, fishes, amphibians, birds, and mammals (e.g., Terborgh and Faaborg, 1980; Karlson et al., 2004; Harrison et al., 2006; Belmaker, 2009; White and Hurlbert, 2010; Belmaker and Jetz, 2012). However, the possibility that, independently of the regional enrichment, the same environmental factor affects both local and regional species richness remains unexplored (Ptacnik et al., 2010). Woodland and forest birds are an ideal system to address this question. First, vegetation complexity in terms of canopy height, total volume of foliage, or the diversity of vegetation layers predicts local species richness in birds (MacArthur and MacArthur, 1961; Recher, 1971; Moss, 1978; Mills et al., 1991; Santillán et al., 2020; Remeš et al., 2021a). Second, the size of the regional species pool often increases with canopy height (Coops et al., 2018; Remeš and Harmáčková, 2018; Feng et al., 2020). Thus, an interesting possibility emerges that species richness increases with canopy height both regionally and locally. If confirmed, the same environmental factor would promote local species richness both directly (via local effects) and indirectly (via regional effects on the size of the species pool).
Here, we evaluated this possibility in passerine birds of woodlands and forests in eastern Australia along a gradient of canopy height. We quantified regional species richness (species pool size) and sampled local bird communities along a 3,000 km long continental-scale transect. We measured canopy height both regionally using satellite imagery and locally using vegetation sampling in the field. Using multiple regressions and structural equation modeling, we studied (1) how species pool size changes with regional canopy height and water availability. We included water availability, because it has been shown to influence vegetation height and the regional number of species (Hawkins et al., 2003; Zhang et al., 2016; Remeš and Harmáčková, 2018). Next, we studied (2) how local species richness changes with pool size and local canopy height. More specifically, based on previous literature (cited above), we expected that both regional and local species richness increase with canopy height, and that local species richness increases also with larger pool size. We thus evaluated the effects of canopy height on species richness across spatial scales and showed that vegetation complexity indexed by canopy height had both direct and indirect positive effects on avian species richness.
Materials and methods
Data acquisition
We sampled local species richness of passerines and vegetation structure of their habitats in the field. We worked at 21 locations in eucalypt woodlands and forests of eastern Australia (Figure 1A and Supplementary Figure 1) during the austral breeding season from September to December in 2016 and 2017 (Supplementary Table 1). We selected habitats in protected areas with native vegetation and without any pastoral activity. At each site, we delimited three transects. Each transect was 2 km long and 50 m wide (10 ha) and was divided into two 25 m wide sides by a centrally running path or narrow forest road along which we walked. We placed the transects such that they were representative of local vegetation and had homogeneous vegetation cover. Each transect was censused twice by two observers with 0–3 days between the two censuses. The two observers worked on different sides of the transect and swapped sides between the two censuses. We started observations shortly after dawn and walked along a transect with roughly constant speed of 100 m per 12 min for the total time of 4 h, thus standardizing the observation effort. We limited our work to days without rain and strong wind. We censused all passerine birds detected by sight or sound. For our analyses, we used the summed abundance of each species from the two censuses of each transect.
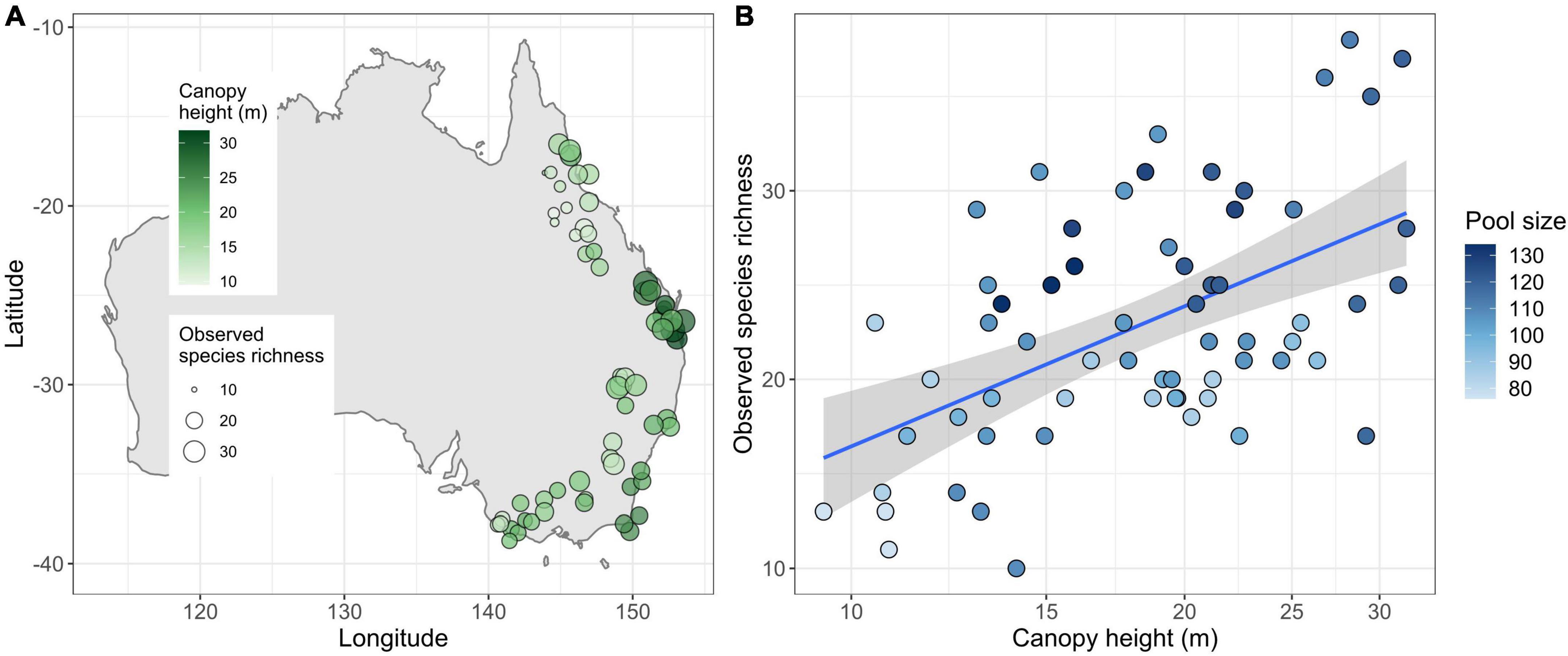
Figure 1. Canopy height, species richness, and pool size in eastern Australia. (A) Map of Australia showing the location of our 63 transects (their geographic position was randomly jittered to decrease point overlap). The latitudinal span of the sites is ca. 2,300 km, while the span along the coast is ca. 3,000 km. (B) Observed species richness increased with both canopy height and size of the regional species pool (note that light-colored pool points lie mostly below the regression line and vice versa for dark-colored points). Here, observed species richness and ecological species pool are depicted. Other options (Chao2 richness, and biogeographic and internal pool) showed almost identical relationships.
To quantify vegetation structure at each transect, we used a systematic sampling design by placing 10 points (200 m apart) along each transect. At each point, we delimited a semi-circle with the radius of 25 m (area ca. 0.1 ha or 1,000 m2) with alternating left and right direction from the transect. Thus, the total area surveyed for vegetation structure was ca. 1 ha (i.e., 10,000 m2) on each transect. At each point, we recorded vegetation cover in five height strata (0–1, 1.1–2.0, 2.1–5, and 5.1–10, >10 m) and vegetation cover and height of four vegetation strata (herbaceous, shrub, subcanopy, and canopy). Height strata were delimited by a priori selected height bands, while vegetation strata were determined by major vertical vegetation layers typical of woodlands and forests. Thus, for vegetation strata, besides cover we also needed to measure the height of individual strata. The height of the herbaceous stratum was estimated visually, while the height of shrub, subcanopy, and canopy strata was measured by taking three measurements by a laser rangefinder (Nikon Forestry). Vegetation cover of each band (height strata) or stratum (vegetation strata) was estimated by eye on the scale ranging from 0 (no vegetation) to 10 (fully covered). The estimate was done independently by two observers who then converged on a consensus estimate. We calculated several measures of vegetation complexity based on our field measurements and confirmed by a principal component analysis (PCA) analysis that canopy height (varying from 9.4 to 31.7 m) was a good proxy for how complex the vegetation was in terms of the number of vegetation layers and the amount of foliage available for birds to forage on (see Supplementary Table 2 and Supplementary Figures 2, 3). We thus used it as a predictor in all our analyses, in accordance with previous studies (e.g., Gouveia et al., 2014; Coops et al., 2018; Remeš and Harmáčková, 2018; Feng et al., 2020; Remešová et al., 2020; Remeš et al., 2021b).
Next, we obtained species pools and canopy height data for each transect on a regional geographic scale from appropriate datasets available online. First, we determined the species pool for each transect using digital range maps of all Australian passerines excluding non-native species (BirdLife-International and NatureServe, 2020). We created a 50 km buffer zone around each transect location, overlayed it with range maps, and identified species whose ranges intersected the transect buffer zone. The same results of statistical analyses were obtained when 25 or 75 km buffer was used. Second, we extracted mean canopy height and several environmental characteristics related to water availability, productivity, and vegetation structure (see below) in a circle with a radius of 50 km centered on each transect location (very similar results were obtained when 100 km radius was used). For canopy height, we used a global dataset (Simard et al., 2011) while for the environmental characteristics we used datasets from The Atlas of Living Australia (accessed April 24, 2017).1
The water-related characteristics included mean annual precipitation, moisture index, and precipitation deficit. We chose these variables because water availability is a critical predictor of species richness and canopy height on the southern hemisphere (Hawkins et al., 2003; Zhang et al., 2016; Remeš and Harmáčková, 2018). These environmental variables were highly mutually correlated (r = 0.6–0.9) and thus we retained only the moisture index (Remeš and Harmáčková, 2018). It is defined as the annual mean of the monthly ratio of precipitation to potential evaporation (pan and free water surface). It is a numerical indicator of the degree of dryness of the climate at a given location, whereby high values indicate relatively wet locations while low values indicate relatively dry locations, and thus provides a convenient measure of water availability. The productivity-related measure was net primary productivity (NPP). It correlated strongly with canopy height (r = 0.88), confirming that tall forests had higher NPP and thus likely provided more resources for birds. The vegetation structure-related characteristics were the normalized difference vegetation index (NDVI; a measure of vegetation greenness) and leaf area index (LAI), defined as the one-sided green leaf area per unit ground surface area (in m2/m2). Both these indices correlated strongly with canopy height (0.68 for NDVI and 0.84 for LAI). Thus, having made sure that canopy height is a good proxy of productivity and the overall amount of foliage and the number of vegetation layers present, we selected it for further analyses.
In sum, we obtained several variables at the level of individual transects. First, we obtained local bird densities, species lists, and vegetation structure in the field. Second, we obtained regional species pools, canopy height, and several environmental variables (in circles of 50 km radius centered on transects) using online resources. All the data are available online (Remeš et al., 2022).
Data processing
Several methodological problems must be accounted for when analyzing local and regional species richness. A false curvilinear relationship between local and regional species richness (pseudosaturation) might stem from either underestimating local richness or overestimating regional pool richness (Harrison and Cornell, 2008). Local species richness might be underestimated if rare species are undersampled in species-rich sites (Caley and Schluter, 1997). We ran several checks to ensure this was not so in our data. First, we calculated rarefaction based on sample coverage (Chao and Jost, 2012) using the iNEXT package for R software (Hsieh et al., 2016). Subtracting the sample coverage from unity gives the proportion of the community belonging to unsampled species. In other words, it is the probability that a new, previously unsampled species would be found if the sample were enlarged by one individual. Sample coverage across transects was reasonably high with a median of 0.97 (ranging from 0.90 to 1.00), suggesting that the proportion of the community belonging to unsampled species was low (1–0.97 = 0.03). Moreover, it did not correlate with canopy height (r = 0.16, p = 0.20), showing that high canopy transects with thick vegetation were not comparatively undersampled. Second, total density (the number of all individuals recorded during censuses) increased with canopy height (r = 0.59, p < 0.001). Thus, more species recorded in high canopy sites could have been the result of more individuals sampled. We thus calculated species richness adjusted for unobserved species using the non-parametric Chao2 estimator (called Chao2 richness hereafter; Gotelli and Ellison, 2012). The Chao2 richness correlated well with observed species richness (r = 0.85). Moreover, the difference between Chao2 richness and observed richness (“richness bias”) did not correlate with canopy height, again confirming no systematic undersampling of high canopy transects (r = 0.19, p = 0.13).
Regional species pool can be overestimated if it includes species that do not occur in the habitat of interest (Cornell and Lawton, 1992; Srivastava, 1999). An opposite problem is when the pool is underestimated due to its lacking species excluded by filtering or competition, a case when the pool is constructed exclusively from observed communities (Cadotte and Davies, 2016). Thus, a great attention must be paid to the definition of species pool because it can affect study outcomes (Lessard et al., 2012). We followed the advice of using several definitions of species pool as a kind of sensitivity analysis (Cadotte and Davies, 2016) and defined three species pools. First, a biogeographic species pool included all species whose ranges intersected the 50 km buffer around each transect location (Carstensen et al., 2013). Second, an ecological species pool included a subset of species in the biogeographic pool that were able to occupy habitats on our transects (Zobel et al., 1998). For that, we selected only species with reasonable affinity to forests and woodlands. The included species must have had a habitat selection characteristic of a minimum of 25 points out of 100 assigned to Rainforest, Forest or Woodland (for details on habitat data, see Harmáčková et al., 2019). Using threshold values of 10 or 40 points did not change the results. Third, we defined an internal pool as a subsample of species out of the ecological pool belonging to each transect that occurred at least once on at least one of the transects.
Data analyses
We related local species richness to canopy height measured on transects and the size of the regional species pool. We also related the proportion of pool size that was observed on each transect to local canopy height. We included this latter analysis, because it explicitly shows whether canopy height affects local species richness independently of pool size or not. Furthermore, we related the size of the regional species pool to regional canopy height (mean canopy height in a circle with a radius of 50 km centered on each transect location) and the moisture index. We always used both measures of species richness (observed and Chao2 richness) and all three types of the species pool (biogeographic, ecological, and internal). For statistical modeling, we used linear mixed models as implemented in the nlme package for R software (Pinheiro et al., 2021). We included study location (n = 21) as a random effect. There was no remaining spatial autocorrelation in residuals from our models, so we did not model it. The proportion of variation explained by the mixed models (Rsq) was calculated using the r2beta function of the r2glmm package (Jaeger, 2017) with the “nsj” option giving estimates according to Nakagawa and Schielzeth (2013).
Next, we followed a conceptual framework of Harrison and Cornell (2008) and modeled species richness using a multiscale structural equation models. The reason for using both multiple regressions and structural equation models was that first we wanted to present separately analyses for the two spatial scales (using multiple regressions), and only then to join them into a cross-scale structural equation model. We find this presentation logical and feel that it makes our presentation comprehensive. To fit the structural equation models, we used a piecewise approach in which the causal relationships among variables were statistically defined and evaluated as mutually interconnected equations using the piecewiseSEM package for R (Lefcheck, 2016). We fitted following equations with fixed and random parts using mixed models in the nlme package. The random effect of Site has 21 levels, with three census transects on each site.
We then united the equations into a single structural model using the psem function of the piecewiseSEM package. We extracted standardized regression coefficients and their statistical significance from the resulting R object using the summary function. We did not evaluate the overall model fit, as our aim was not model selection but rather parameter estimation.
We treated values of species richness as continuous data, because they were reasonably large and it is a common practice (e.g., Hawkins et al., 2003; White and Hurlbert, 2010). In all models, we included quadratic terms for each variable to account for potential non-linear relationships. However, when the quadratic term was not statistically significant, we excluded it from the final model. This was the case in all our models. Otherwise, we retained all predictors in our models. If appropriate, variables were transformed to approach the normal distribution, and these transformations are apparent in figures and tables. We scaled all predictors by subtracting the mean and dividing by one standard deviation.
Results
Across transects, we observed from 10 to 38 species of passerine birds (median = 22). The median size of the species pool ranged from 86 in internal (range 58–104) to 106 in ecological (range 76–134) to 118 in biogeographic pool (range 89–152). Local species richness represented 26.0% of internal, 21.0% of ecological, and 18.6% of biogeographic species pool (median values). Local canopy height ranged from 9.4 to 31.7 m (median = 19.1 m) while regional canopy height ranged from 1.8 to 17.4 m (median = 7.3 m). Lower regional canopy height was caused by interior transects encompassing broad swathes of relatively arid areas with low vegetation (Supplementary Figure 4).
Local species richness increased linearly with both canopy height and the size of the regional species pool (Table 1 and Figure 1B). This was true for both observed and Chao2 species richness and irrespective of the definition of the species pool. The only exception was internal species pool, where the effect of canopy height was no longer statistically significant (Table 1). The size of the species pool increased with canopy height [Figure 2A; biogeographic pool: estimate (SE) = 4.3 (2.0), p = 0.031; ecological pool: 4.6 (1.9), p = 0.017; internal pool: 6.7 (1.4), p < 0.001]. On the contrary, its relationship with the moisture index was never statistically significant (all p-values > 0.05). We then calculated the proportion of species in the species pool that were observed on the transects in the field. This proportion increased linearly with canopy height for both observed and Chao2 species richness and for all three types of the species pool. This increase was always statistically significant (all p-values < 0.05; Supplementary Table 3 and Figure 2B). The likely reason was that while the increase of local species richness with canopy height was linear, the increase of regional species pool was strong until canopy height of ca. 15 m, while it leveled off thereafter (Figure 2A and Supplementary Figure 6).
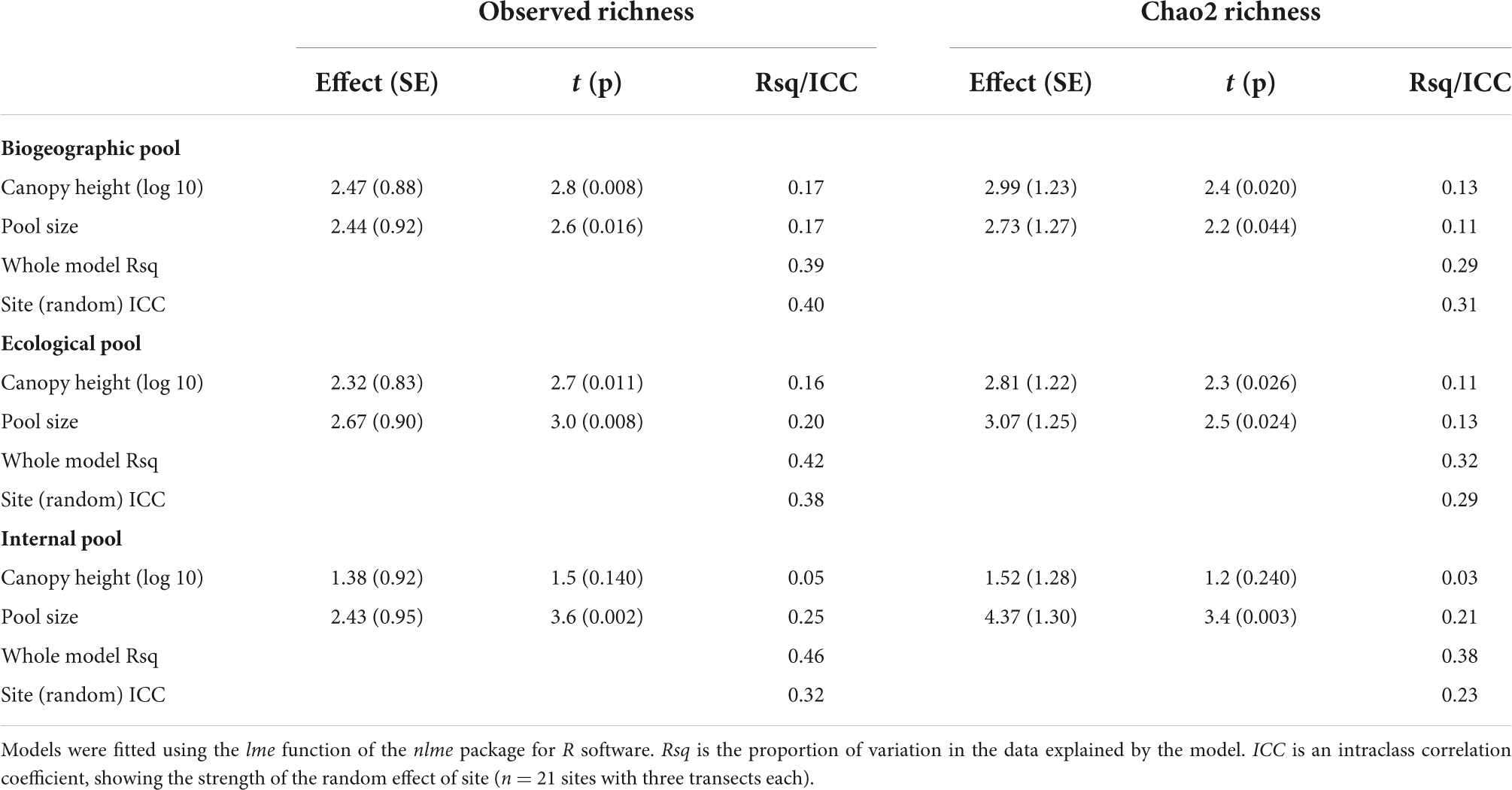
Table 1. Results of linear mixed models relating local species richness to canopy height and the size of the regional species pool.
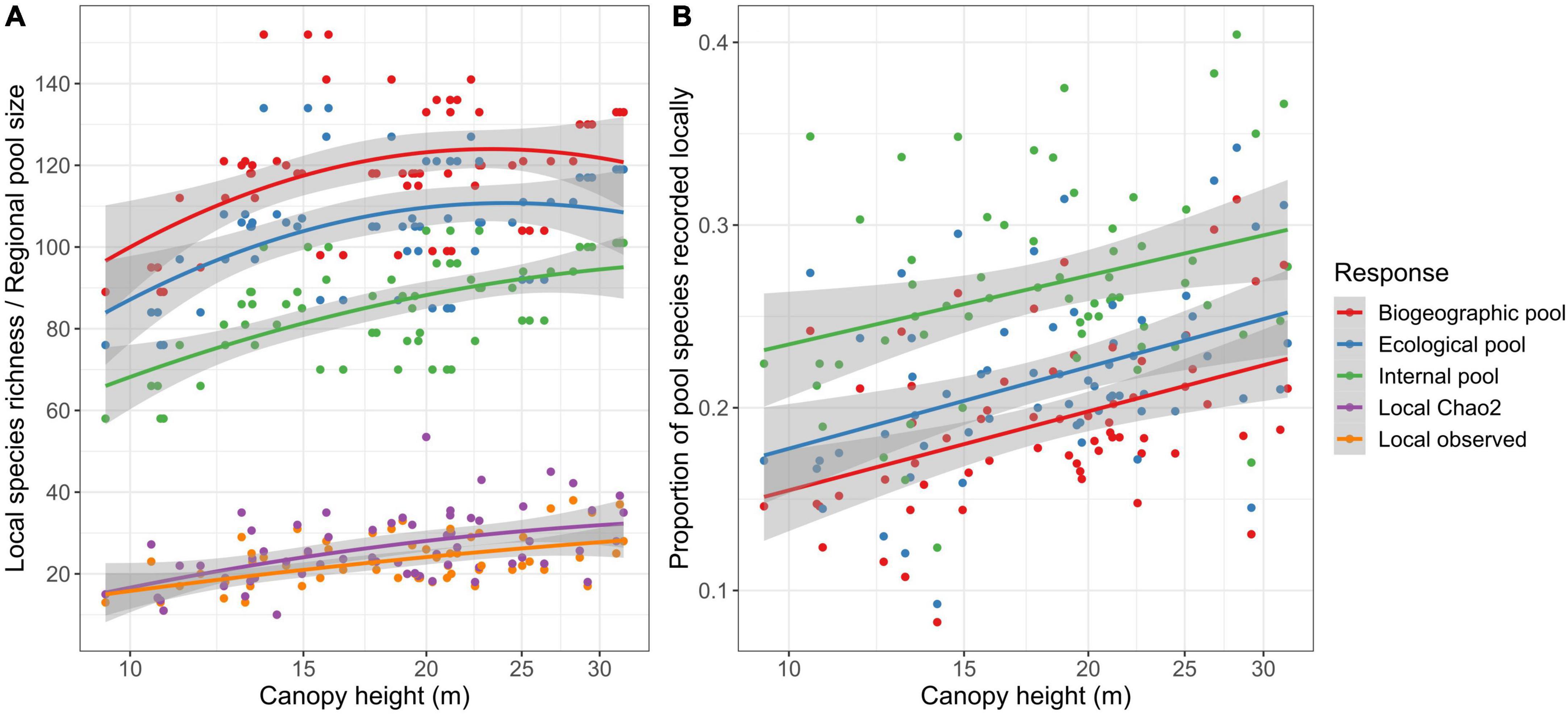
Figure 2. Species richness in relation to canopy height. (A) Both local species richness and the size of the regional species pool increased with canopy height. Quadratic regression fits are showed for illustration, although the quadratic term was not statistically significant. (B) The proportion of species from the species pool that was recorded on transects increased with canopy height. Here, we show observed species richness as a proportion of a given species pool, while the relationships for Chao2 richness were virtually the same (Supplementary Figure 5).
Structural equation modeling revealed that local species richness increased with both local canopy height and regional pool size; local canopy height was correlated with regional canopy height; and the size of the regional species pool increased with both regional canopy height and the moisture index (Figure 3). The weakest relationship was between pool size and the moisture index, and it was never statistically significant (although for the biogeographic pool only marginally so with p = 0.052; Figure 3 and Supplementary Figure 7). When different species richness metrics (observed vs. Chao2 richness) and regional pool definitions (biogeographic, ecological, and internal) were used, the results were mutually consistent. The only exception was that in case of internal species pool, the path from local canopy height to local species richness was not statistically significant and major part of local species richness was explained by the size of species pool (Supplementary Figure 7).
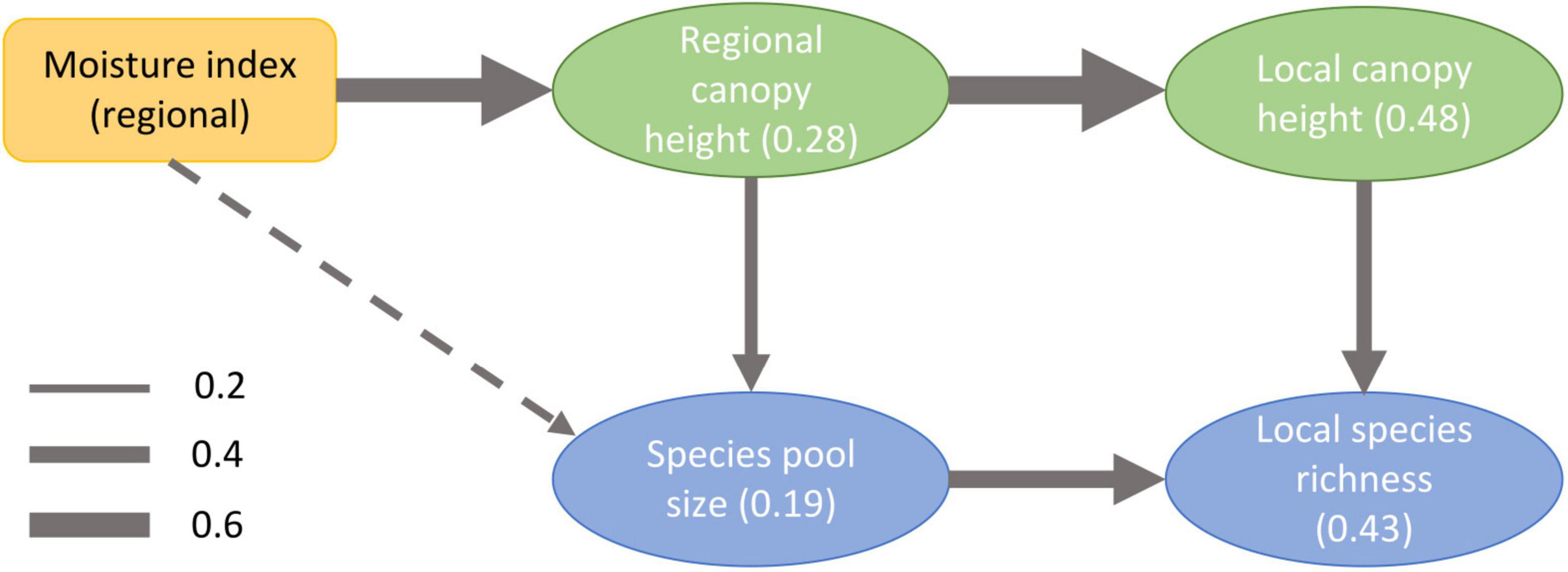
Figure 3. Results of the multiscale structural equation model (Harrison and Cornell, 2008) relating species richness, pool size, canopy height, and water availability across spatial scales. Local scale is at the level of our study transects (length of 2 km). Regional scale is at the level of circles with a radius of 50 km centered at each transect. This figure shows observed local species richness and ecological species pool. Other options gave mutually consistent results (see Supplementary Figure 7). Arrow width is proportional to the standardized effect size (see the legend for values). Solid lines depict statistically significant effects, while hatched lines show effects with p-value > 0.05. Numbers in parentheses depict the proportion of variability explained (R-squared values).
Discussion
We evaluated effects of canopy height on species richness within and across spatial scales, with the following major results. First, the size of the regional species pool increased with canopy height quantified on a regional scale, while the direct effect of water availability on pool size was negligible. However, water availability promoted canopy height on both regional and local scales; at the local scale, it promoted canopy height indirectly through its correlation with canopy height at the regional scale. Second, local species richness increased additively with both local canopy height and regional pool size. The only exception was a statistically non-significant effect of canopy height when fitted with the internal species pool. The reason might be that the internal pool contained only species that occurred at least once on at least one of the transects, and thus its relation to species richness was tight, probably decreasing the explanatory power of canopy height. Third, local communities in high canopy forests contained not only more species, but also sampled higher proportion of species from the regional species pool. Thus, vegetation complexity had both direct and indirect (via regional pool size) positive effects on local avian species richness.
Species pool size increased with canopy height, confirming previous studies conducted on regional and continental scales (Coops et al., 2018; Remeš and Harmáčková, 2018; Feng et al., 2020). The reason of increasing pool size with canopy height regionally might be niche conservatism, whereby moist forests covered most of Australia for millions of years (Byrne et al., 2011) and consequently many species originated that are adapted to this biome (Miller et al., 2013). Subsequently, as Australia dried up with the development of the Antarctic ice shield, only a limited number of clades produced arid-adapted species of birds (Byrne et al., 2008). Large pool size would thus be a legacy of integrated area-age effect identified as promoting high biome-specific diversity in general (Jetz and Fine, 2012; Belmaker and Jetz, 2015). However, once moist forests have shrunk, the many species associated with these forests must have survived in much smaller biome area. This could have been facilitated by a high number of bird individuals present per area of woodlands and forests, as compared to more arid regions, which should be beneficial for species persistence due to high numbers of individuals preventing species extinction in a long run (Storch et al., 2018). Indeed, many forest species in tropical Australia have inverse range-abundance relationships, being range-restricted and locally abundant at the same time (Williams et al., 2009), which might promote their persistence. In support of this conjecture, we found an increasing total density of passerines with canopy height (see Data processing Section in Materials and Methods), showing that tall, more productive forests with more vegetation layers contained more individuals of birds. Additionally, larger regional species pools in tall forests might be facilitated by their higher floristic diversity (Rice and Westoby, 1983; Andrew et al., 2021), an effect suggested to explain pool size in coral-dwelling fishes (Belmaker, 2009). Indeed, several studies suggested that floristic diversity might be as important as, or even more important than, vegetation structural diversity in promoting bird species richness (e.g., Adams and Matthews, 2019).
Local species richness increased with canopy height and regional pool size (the only exception being a statistically non-significant effect of canopy height when fitted with the internal pool size). Although this has been observed previously in various taxa (Terborgh and Faaborg, 1980; Karlson et al., 2004; Harrison et al., 2006; Belmaker, 2009; White and Hurlbert, 2010; Belmaker and Jetz, 2012), we bring two new aspects. First, we used a multiscale structural equation modeling, which links drivers of species richness across spatial scales (Harrison and Cornell, 2008). More specifically, we showed that canopy height increased with the moisture index on the regional scale, and this carried over to local avian richness via both local canopy height and pool size. Thus, water availability affected local avian species richness indirectly by two different pathways. On the other hand, a pathway from the moisture index to local species richness directly via pool size was very weak and statistically not significant. This suggests that water availability affects the size of avian species pool in Australia mainly due to its effect on vegetation complexity. Second, we showed that local communities in high canopy forests contained not only more species (Recher, 1971; Moss, 1978; Mills et al., 1991; Santillán et al., 2020; Remeš et al., 2021a), but also sampled a higher proportion of species from the regional species pool (this was true even when the internal pool was used). Thus, high canopy had a doubly positive effect on local species richness: due to promoting a large regional species pool and, independently, due to facilitating the coexistence of disproportionately many species locally.
High local species richness in tall forests might be a consequence of (i) a higher number of individuals (Storch et al., 2018) present due to higher productivity and more abundant resources (Sam et al., 2019), (ii) more available niches due to structurally complex vegetation (MacArthur and MacArthur, 1961; Remeš et al., 2021b), or (iii) a combination of these two mechanisms (Hurlbert, 2004; Mönkkönen et al., 2006). Indeed, the total number of individuals recorded during standardized censuses increased with canopy height. Thus, better sampling of tall forests might have biased our estimates of local richness upward. However, using a rarefaction on sample coverage and a non-parametric estimator of species richness adjusted for unobserved species (Chao2), we showed that this was not likely [see Section (Materials and Methods) for details]. Apart from sampling biases, communities with more individuals might include more species in a long run because ecological drift eliminating species is less effective in large samples of individuals (Vellend, 2016). Although definitely true, this is probably not the whole explanation due to at least two reasons. First, the range of resource types is often more important than the total amount of available energy in predicting avian species richness (Hurlbert and Jetz, 2010). This has certainly been a classical explanation for higher avian richness of tropical rain forests as compared to temperate forests, with tropical forests providing more diverse resources (Terborgh, 1980; Bell, 1983; Rosenberg, 1997). Second, in accordance with these previous observations, larger plants offer more types of resources (e.g., more species of insects; Lawton, 1983), more complex vegetation offers more resources to birds (Ferger et al., 2014), and more functional types of birds are present in high canopy forests than expected simply based on total densities increasing with canopy height (Remeš et al., 2021a,b). We thus conclude that at least part of high avian species richness in tall, more productive forests with more vegetation layers is due to non-neutral, niche-based processes (Hurlbert, 2004).
Data availability statement
The dataset used in this study can be accessed at Zenodo, https://doi.org/10.5281/zenodo.6622877.
Author contributions
VR conceived the ideas for this study, analyzed the data, and wrote the manuscript. LH and ER compiled the data. VR, LH, BM, LR, and ER collected the data, contributed to the article, and approved the submitted version.
Funding
This work was supported by the Czech Science Foundation (GAČR project. no. 21-26376S).
Acknowledgments
We appreciate the Atlas of Living Australia for making very useful online resources available free of charge.
Conflict of interest
The authors declare that the research was conducted in the absence of any commercial or financial relationships that could be construed as a potential conflict of interest.
Publisher’s note
All claims expressed in this article are solely those of the authors and do not necessarily represent those of their affiliated organizations, or those of the publisher, the editors and the reviewers. Any product that may be evaluated in this article, or claim that may be made by its manufacturer, is not guaranteed or endorsed by the publisher.
Supplementary material
The Supplementary Material for this article can be found online at: https://www.frontiersin.org/articles/10.3389/fevo.2022.964180/full#supplementary-material
Footnotes
References
Adams, B. T., and Matthews, S. N. (2019). Diverse temperate forest bird assemblages demonstrate closer correspondence to plant species composition than vegetation structure. Ecography 42, 1752–1764. doi: 10.1111/ecog.04487
Andrew, S. C., Mokany, K., Falster, D. S., Wenk, E., Wright, I. J., Merow, C., et al. (2021). Functional diversity of the Australian flora: strong links to species richness and climate. J. Veg. Sci. 32:e13018. doi: 10.1111/jvs.13018
Bell, H. L. (1983). A bird community of lowland rainforest in New Guinea. 6 Foraging ecology and community structure of the avifauna. Emu 84, 142–158.
Belmaker, J. (2009). Species richness of resident and transient coral-dwelling fish responds differentially to regional diversity. Glob. Ecol. Biogeogr. 18, 426–436. doi: 10.1111/j.1466-8238.2009.00456.x
Belmaker, J., and Jetz, W. (2012). Regional pools and environmental controls of vertebrate richness. Am. Nat. 179, 512–523. doi: 10.1086/664610
Belmaker, J., and Jetz, W. (2015). Relative roles of ecological and energetic constraints, diversification rates and region history on global species richness gradients. Ecol. Lett. 18, 563–571. doi: 10.1111/ele.12438
BirdLife-International and NatureServe (2020). Bird Species Distribution Maps of the World. Cambridge, MA: BirdLife-International.
Byrne, M., Steane, D. A., Joseph, L., Yeates, D. K., Jordan, G. J., Crayn, D., et al. (2011). Decline of a biome: evolution, contraction, fragmentation, extinction and invasion of the Australian mesic zone biota. J. Biogeogr. 38, 1635–1656. doi: 10.1111/j.1365-2699.2011.02535.x
Byrne, M., Yeates, D. K., Joseph, L., Kearney, M., Bowler, J., Williams, M. A. J., et al. (2008). Birth of a biome: insights into the assembly and maintenance of the Australian arid zone biota. Mol. Ecol. 17, 4398–4417. doi: 10.1111/j.1365-294X.2008.03899.x
Cadotte, M. W., and Davies, T. J. (2016). Phylogenies in Ecology: A Guide to Concepts and Methods. Princeton: Princeton University Press.
Caley, M. J., and Schluter, D. (1997). The relationship between local and regional diversity. Ecology 78, 70–80.
Carstensen, D. W., Lessard, J. P., Holt, B. G., Krabbe Borregaard, M., and Rahbek, C. (2013). Introducing the biogeographic species pool. Ecography 36, 1310–1318. doi: 10.1111/j.1600-0587.2013.00329.x
Chao, A., and Jost, L. (2012). Coverage-based rarefaction and extrapolation: standardizing samples by completeness rather than size. Ecology 93, 2533–2547. doi: 10.1890/11-1952.1
Coops, N. C., Rickbeil, G. J. M., Bolton, D. K., Andrew, M. E., and Brouwers, N. C. (2018). Disentangling vegetation and climate as drivers of Australian vertebrate richness. Ecography 41, 1147–1160. doi: 10.1111/ecog.02813
Cornell, H. V., and Harrison, S. P. (2014). What are species pools and when are they important? Annu. Rev. Ecol. Evol. Syst. 45, 45–67. doi: 10.1146/annurev-ecolsys-120213-091759
Cornell, H. V., and Lawton, J. H. (1992). Species interactions, local and regional processes, and limits to the richness of ecological communities: a theoretical perspective. J. Anim. Ecol. 61, 1–12. doi: 10.2307/5503
Diamond, J. M. (1975). “Assembly of species communities,” in Ecology and Evolution of Communities, eds M. L. Cody and J. M. Diamond (Cambridge, MA: Harvard University Press), 342–443.
Feng, G., Zhang, J., Girardello, M., Pellissier, V., and Svenning, J. C. (2020). Forest canopy height co-determines taxonomic and functional richness, but not functional dispersion of mammals and birds globally. Glob. Ecol. Biogeogr. 29, 1350–1359. doi: 10.1111/geb.13110
Ferger, S. W., Schleuning, M., Hemp, A., Howell, K. M., and Böhning-Gaese, K. (2014). Food resources and vegetation structure mediate climatic effects on species richness of birds. Glob. Ecol. Biogeogr. 23, 541–549. doi: 10.1111/geb.12151
Fox, J. W., and Srivastava, D. (2006). Predicting local-regional richness relationships using island biogeography models. Oikos 113, 376–382. doi: 10.1111/j.2006.0030-1299.14768.x
Gotelli, N. J., and Ellison, A. M. (2012). A Primer of Ecological Statistics, 2nd Edn. Sunderland: Sinauer Associates.
Gouveia, S. F., Villalobos, F., Dobrovolski, R., Beltrão-Mendes, R., and Ferrari, S. F. (2014). Forest structure drives global diversity of primates. J. Anim. Ecol. 83, 1523–1530. doi: 10.1111/1365-2656.12241
Harmáčková, L., Remešová, E., and Remeš, V. (2019). Specialization and niche overlap across spatial scales: revealing ecological factors shaping species richness and coexistence in Australian songbirds. J. Anim. Ecol. 88, 1766–1776. doi: 10.1111/1365-2656.13073
Harrison, S., and Cornell, H. (2008). Toward a better understanding of the regional causes of local community richness. Ecol. Lett. 11, 969–979. doi: 10.1111/j.1461-0248.2008.01210.x
Harrison, S., Safford, H. D., Grace, J. B., Viers, J. H., and Davies, K. F. (2006). Regional and local species richness in an insular environment: serpentine plants in California. Ecol. Monogr. 76, 41–56. doi: 10.1890/05-0910
Hawkins, B. A., Field, R., Cornell, H. V., Currie, D. J., Guégan, J. F., Kaufman, D. M., et al. (2003). Energy, water, and broad-scale geographic patterns of species richness. Ecology 84, 3105–3117. doi: 10.1890/03-8006
He, F., Gaston, K. J., Connor, E. F., and Srivastava, D. S. (2005). The local-regional relationship: immigration, extinction, and scale. Ecology 86, 360–365. doi: 10.1890/04-1449
HilleRisLambers, J., Adler, P. B., Harpole, W. S., Levine, J. M., and Mayfield, M. M. (2012). Rethinking Community Assembly through the Lens of Coexistence Theory. Annu. Rev. Ecol. Evol. Syst. 43, 227–248. doi: 10.1146/annurev-ecolsys-110411-160411
Hsieh, T. C., Ma, K. H., and Chao, A. (2016). iNEXT: an R package for rarefaction and extrapolation of species diversity (Hill numbers). Methods Ecol. Evol. 7, 1451–1456. doi: 10.1111/2041-210X.12613
Hurlbert, A. H. (2004). Species-energy relationships and habitat complexity in bird communities. Ecol. Lett. 7, 714–721. doi: 10.1111/j.1461-0248.2004.00630.x
Hurlbert, A. H., and Jetz, W. (2010). More than “more individuals”: the nonequivalence of area and energy in the scaling of species richness. Am. Nat. 176, E50–E65. doi: 10.1086/650723
Hutchinson, G. E. (1959). Homage to Santa Rosalia or Why are there so many kinds of animals? Am. Nat. 93, 145–159. doi: 10.1086/282070
Jaeger, B. (2017). r2glmm: Computes R Squared for Mixed (Multilevel) Models. R package version 0.1.2.
Jetz, W., and Fine, P. V. A. (2012). Global gradients in vertebrate diversity predicted by historical area-productivity dynamics and contemporary environment. PLoS Biol. 10:e1001292. doi: 10.1371/journal.pbio.1001292
Karlson, R. H., Cornell, H. V., and Hughes, T. P. (2004). Coral communities are regionally enriched along an oceanic biodiversity gradient. Nature 429, 867–870. doi: 10.1038/nature02685
Lawton, J. H. (1983). Plant architecture and the diversity of phytophagous insects. Annu. Rev. Entomol. 28, 23–39. doi: 10.1146/annurev.en.28.010183.000323
Lefcheck, J. S. (2016). piecewiseSEM: piecewise structural equation modelling in r for ecology, evolution, and systematics. Methods Ecol. Evol. 7, 573–579. doi: 10.1111/2041-210X.12512
Lessard, J. P., Belmaker, J., Myers, J. A., Chase, J. M., and Rahbek, C. (2012). Inferring local ecological processes amid species pool influences. Trends Ecol. Evol. 27, 600–607. doi: 10.1016/j.tree.2012.07.006
McGill, B. (2003). Strong and weak tests of macroecological theory. Oikos 102, 679–685. doi: 10.1034/j.1600-0706.2003.12617.x
Miller, E. T., Zanne, A. E., and Ricklefs, R. E. (2013). Niche conservatism constrains Australian honeyeater assemblages in stressful environments. Ecol. Lett. 16, 1186–1194. doi: 10.1111/ele.12156
Mills, G. S., Dunning, J. B. J., and Bates, J. M. (1991). The relationship between breeding bird density and vegetation volume. WIlson Bull. 103, 468–479. doi: 10.1002/ece3.8162
Mittelbach, G. G., and McGill, B. J. (2019). Community Ecology, 2nd Edn. Oxford: Oxford University Press.
Mittelbach, G. G., and Schemske, D. W. (2015). Ecological and evolutionary perspectives on community assembly. Trends Ecol. Evol. 30, 241–247. doi: 10.1016/j.tree.2015.02.008
Mönkkönen, M., Forsman, J. T., and Bokma, F. (2006). Energy availability, abundance, energy-use and species richness in forest bird communities: a test of the species-energy theory. Glob. Ecol. Biogeogr. 15, 290–320. doi: 10.1111/j.1466-822X.2006.00224.x
Moss, D. (1978). Diversity of woodland song-bird populations. J. Anim. Ecol. 47, 521–527. doi: 10.2307/3798
Nakagawa, S., and Schielzeth, H. (2013). A general and simple method for obtaining R2 from generalized linear mixed-effects models. Methods Ecol. Evol. 4, 133–142. doi: 10.1111/j.2041-210x.2012.00261.x
Olivares, I., Karger, D. N., and Kessler, M. (2018). Assessing species saturation: conceptual and methodological challenges. Biol. Rev. 93, 1874–1890. doi: 10.1111/brv.12424
Pinheiro, J., Bates, D., DebRoy, S., and Sarkar, D. (2021). nlme: Linear and Nonlinear Mixed Effects Models. R Package Version 3.1-152.
Ptacnik, R., Andersen, T., Brettum, P., Lepistö, L., and Willén, E. (2010). Regional species pools control community saturation in lake phytoplankton. Proc. R. Soc. B Biol. Sci. 277, 3755–3764. doi: 10.1098/rspb.2010.1158
Recher, H. F. (1971). Bird species diversity: a review of the relation between species number and environment. Proc. Ecol. Soc. Aust. 6, 135–152.
Remeš, V., and Harmáčková, L. (2018). Disentangling direct and indirect effects of water availability, vegetation, and topography on avian diversity. Sci. Rep. 8:15475. doi: 10.1038/s41598-018-33671-w
Remeš, V., Harmáčková, L., Matysioková, B., Rubáčová, L., and Remešová, E. (2022). Data from “Vegetation complexity and pool size predict species richness of forest birds”. Available online at: https://doi.org/10.5281/zenodo.6622877 (accessed June 8, 2022).
Remeš, V., Remešová, E., Friedman, N. R., Matysioková, B., and Rubáčová, L. (2021a). “Foraging behavior, guild structure, and species diversity in Australian passerines,” in Advances in Animal Science and Zoology 18, ed. O. P. Jenkins (New York, NY: Nova Science Publishers), 117–150.
Remeš, V., Remešová, E., Friedman, N. R., Matysioková, B., and Rubáčová, L. (2021b). Functional diversity of avian communities increases with canopy height: from individual behavior to continental-scale patterns. Ecol. Evol. 11, 11839–11851. doi: 10.1002/ece3.7952
Remešová, E., Matysioková, B., Turčoková Rubáčová, L., and Remeš, V. (2020). Foraging behaviour of songbirds in woodlands and forests in eastern Australia: resource partitioning and guild structure. Emu 120, 22–32. doi: 10.1080/01584197.2019.1644183
Rice, B., and Westoby, M. (1983). Plant species richness at the 0.1 hectare scale in Australian vegetation compared to other continents. Vegetatio 52, 129–140. doi: 10.1007/BF00044988
Ricklefs, R. E., and Schluter, D. (1993). Species Diversity in Ecological Communities. Chicago, IL: University of Chicago Press.
Rosenberg, K. V. (1997). Ecology of dead-leaf foraging specialists and their contribution to amazonian bird diversity. Ornithol. Monogr. 48, 673–700. doi: 10.2307/40157560
Sam, K., Koane, B., Bardos, D. C., Jeppy, S., and Novotny, V. (2019). Species richness of birds along a complete rain forest elevational gradient in the tropics: habitat complexity and food resources matter. J. Biogeogr. 46, 279–290. doi: 10.1111/jbi.13482
Santillán, V., Quitián, M., Tinoco, B. A., Zárate, E., Schleuning, M., Böhning-Gaese, K., et al. (2020). Direct and indirect effects of elevation, climate and vegetation structure on bird communities on a tropical mountain. Acta Oecol. 102:103500. doi: 10.1016/j.actao.2019.103500
Simard, M., Pinto, N., Fisher, J. B., and Baccini, A. (2011). Mapping forest canopy height globally with spaceborne lidar. J. Geophys. Res. Biogeosciences 116, 1–12. doi: 10.1029/2011JG001708
Song, C., Von Ahn, S., Rohr, R. P., and Saavedra, S. (2020). Towards a probabilistic understanding about the context-dependency of species interactions. Trends Ecol. Evol. 35, 384–396. doi: 10.1016/j.tree.2019.12.011
Srivastava, D. S. (1999). Using local-regional richness plots to test for species saturation: pitfalls and potentials. J. Anim. Ecol. 68, 1–16. doi: 10.1046/j.1365-2656.1999.00266.x
Storch, D., Bohdalková, E., and Okie, J. (2018). The more-individuals hypothesis revisited: the role of community abundance in species richness regulation and the productivity–diversity relationship. Ecol. Lett. 21, 920–937. doi: 10.1111/ele.12941
Szava-Kovats, R. C., Ronk, A., and Partel, M. (2013). Pattern without bias: local-regional richness relationship revisited. Ecology 94, 1986–1992. doi: 10.1890/13-0244.1
Terborgh, J. (1980). “Causes of tropical species diversity,” in Acta XII Congressus Internationalis Ornithologici, ed. R. Nohring (Berlin: Springer), 955–961.
Terborgh, J. W., and Faaborg, J. (1980). Saturation of bird communities in the West Indies. Am. Nat. 116, 178–195. doi: 10.1086/283621
Vellend, M. (2016). The Theory of Ecological Communities. Princeton, NJ: Princeton University Press.
Weiher, E., and Keddy, P. A. (1999). Ecological Assembly Rules: Perspectives, Advances, Retreats. Cambridge, MA: Cambridge University Press.
White, E. P., and Hurlbert, A. H. (2010). The combined influence of the local environment and regional enrichment on bird species richness. Am. Nat. 175, E35–E43. doi: 10.1086/649578
Williams, S. E., Williams, Y. M., VanDerWal, J., Isaac, J. L., Shoo, L. P., and Johnson, C. N. (2009). Ecological specialization and population size in a biodiversity hotspot: how rare species avoid extinction. Proc. Natl. Acad. Sci. U.S.A. 106, 19737–19741. doi: 10.1073/pnas.0901640106
Zhang, J., Nielsen, S. E., Mao, L., Chen, S., and Svenning, J. C. (2016). Regional and historical factors supplement current climate in shaping global forest canopy height. J. Ecol. 104, 469–478. doi: 10.1111/1365-2745.12510
Keywords: coexistence, niches, spatial scales, species diversity, vegetation structure
Citation: Remeš V, Harmáčková L, Matysioková B, Rubáčová L and Remešová E (2022) Vegetation complexity and pool size predict species richness of forest birds. Front. Ecol. Evol. 10:964180. doi: 10.3389/fevo.2022.964180
Received: 08 June 2022; Accepted: 08 September 2022;
Published: 30 September 2022.
Edited by:
Manuel B. Morales, Autonomous University of Madrid, SpainReviewed by:
Robert M. McElderry, Research Corporation of the University of Guam (RCUOG), GuamJulia Gómez Catasús, Novia University of Applied Sciences, Finland
Copyright © 2022 Remeš, Harmáčková, Matysioková, Rubáčová and Remešová. This is an open-access article distributed under the terms of the Creative Commons Attribution License (CC BY). The use, distribution or reproduction in other forums is permitted, provided the original author(s) and the copyright owner(s) are credited and that the original publication in this journal is cited, in accordance with accepted academic practice. No use, distribution or reproduction is permitted which does not comply with these terms.
*Correspondence: Vladimír Remeš, dmxhZC5yZW1lc0BnbWFpbC5jb20=