- Liaoning Provincial Key Laboratory of Hydrobiology, College of Fisheries and Life Science, Dalian Ocean University, Dalian, China
The emergence of metacommunity theory has significantly contributed to our understanding of the drivers of community assembly and biome variation. The Network Location Hypothesis (NPH) posits that tributary communities situated at the source regions of a river are disproportionately susceptible to environmental filtering due to their remote location and consequent reduced connectivity to downstream reaches of the river system. However, downstream communities located in central parts of the river network exhibit increased connectivity to other communities, thereby making them more susceptible to spatial effects. Nonetheless, empirical studies testing this theory have been relatively scarce to date. Additionally, it is widely acknowledged that integrating multiple dimensions of beta diversity can enhance our understanding of the mechanisms driving community assembly. Based on the above, we collected macroinvertebrate samples from a boreal river in China to verify these views. Specifically, we examined the significance of network location on metacommunity assembly (NPH hypothesis) by utilizing a distance-decay relationship and simultaneously assessing multiple dimensions of ecological drivers of beta diversity. Our results revealed that the predictions of the NPH hypothesis were not supported in the study area, with the impact of environmental filtering on community assembly being prevalent regardless of network location. Taxonomic beta diversity consists almost entirely of turnover, with turnover contributing more to functional beta diversity than nestedness, while phylogenetic beta diversity consists of a combination of turnover and nestedness. We observed that a uniform species composition across sites led to higher taxonomic beta diversity in the study area. However, functional redundancy and the presence of closely related species across sites resulted in lower functional and phylogenetic beta diversity compared to taxonomic beta diversity. Although we found some correlation between phylogenetic and functional beta diversity, their mechanisms of variation were not consistent, with phylogenetic beta diversity showing greater variability than functional beta diversity. This suggests that differences in functional traits may be primarily driven by more distantly related species. Therefore, our findings do not fully support the use of phylogenetic distance as a surrogate for functional distance. The present study emphasizes the significance of incorporating multiple dimensions of beta diversity in metacommunity research, as they offer unique insights into beta diversity. Specifically, we found that environmental factors play a crucial role in shaping macroinvertebrate community composition and functional traits, which is associated with the higher environmental heterogeneity within the study area. In contrast, spatial processes, such as dispersal limitations, lead to variations in the evolutionary history of organisms across different locations, which is associated with the larger geographical extent of the study area.
1. Introduction
The assembly of biomes and changes in biodiversity, along with their underlying mechanisms, have always been central to the research of community ecology (Willig et al., 2003; Sanders and Rahbek, 2012). In this regard, metacommunity theory has significantly contributed to our comprehension of community assemblages and biodiversity mechanisms. According to this theory, two primary ecological processes, namely ecological niche-based and spatial processes, play a crucial role in influencing the assembly and variation of biological communities (Fagan, 2002). Within this context, the impacts of these ecological processes on biological communities can be inferred from environmental and spatial factors (Leibold et al., 2004).
In recent years, an increasing amount of research has shifted its focus toward beta diversity, owing to its potential to provide complementary information for metacommunity studies (Tonkin et al., 2016; Rusanov et al., 2022). Beta diversity is characterized as the spatial variability in community membership, reflecting the biological response to environmental filtering and spatial processes (García-Girón et al., 2020). Beta diversity is comprised of two primary components, turnover and nestedness (Baselga, 2010). Turnover involves the substitution of species between different patches (Soininen et al., 2018), whereas nestedness refers to sites where the community composition or functional traits are a subset of more abundant sites, and where these abundant sites possess unique species composition or functional traits (Levesque-Beaudin and Wheeler, 2011). However, past studies have predominantly focused on taxonomic beta diversity (Leibold and Mikkelson, 2002), leading to a limited comprehension of beta diversity (Perez Rocha et al., 2018). Functional beta diversity, which explains ecosystem function and species ecological niche relationships primarily through differences in functional traits between organisms (Petsch et al., 2021), and phylogenetic diversity, which reflects the evolutionary processes of species in response to environmental and spatial effects, offer multiple perspectives on beta diversity and can aid in the understanding of community responses to ecological processes (Winter et al., 2013).
Numerous studies of rivers indicate that changes in beta diversity are mainly driven by ecological niche-based processes and spatial interactions (Frota et al., 2022; Rusanov et al., 2022). Environmental filtering, a phenomenon that selects species based on their adaptation to specific environmental conditions, generates unique community compositions across sites. This process ultimately results in the maintenance of species that can thrive in a given set of environmental conditions, thus producing variations in community structure among locations (Daniel et al., 2019). However, high dispersal rates (e.g., mass effects) can create homogeneous communities that obscure the effects of environmental filtering (Heino et al., 2015b). Conversely, low dispersal rates (e.g., dispersal limitation) may impede the response of organisms to environmental gradients, resulting in the absence of certain species in ecologically suitable locations (Heino et al., 2015b). Distance-decay relationships (DDRs) represent associations between organisms and ecological drivers (Morlon et al., 2008), and they suggest that beta diversity increases with geographical or environmental distance. DDRs can disclose the impact of both ecological niche-based and spatial processes on community assembly and change (Lentendu et al., 2018; Wang Y. et al., 2021).
The structure of a river’s dendritic network (Campbell Grant et al., 2007) provides new opportunities for studying metacommunities. For instance, the Network Position Hypothesis (NPH; Schmera et al., 2018) has recently emerged, which suggests that species sorting dominates the communities of tributaries due to their remote location and lack of connection with other communities (Doretto et al., 2020). In contrast, downstream mainstream communities are more influenced by spatial factors, specifically mass effects, because they are centrally located or connected to the river network (Brown and Swan, 2010). However, there is a scarcity of empirical studies that test NPH predictions, and several recent studies lack supporting evidence (Schmera et al., 2018; Tonkin et al., 2018b). Consequently, the immediate priority is to test the NPH hypothesis in more rivers.
In this research, we conducted an analysis of metacommunity dynamics based on beta diversity in a boreal river basin in China, examining three aspects: taxonomic, functional, and phylogenetic. Our study aimed to investigate the potential correlation between community differences and beta diversity measures with environmental and spatial gradients. We have the following hypotheses: (1) we first assume that communities at mainstream sites are assembled depending on niche-based and spatial processes (Schmera et al., 2018). In contrast, communities at tributary sites are primarily driven by environmental filtering (Brown and Swan, 2010). (2) There exists a strong correlation between functional traits and the environment, leading us to predict that environmental filtering, rather than spatial processes, is the primary driver of functional beta diversity (Wang J. et al., 2021). (3) We predict that both environmental filtering and dispersal limitation affect macroinvertebrate taxonomic beta diversity (Ning and Beiko, 2015; Jiang et al., 2021), as species composition should be affected by both environmental and dispersal limitation (Perez Rocha et al., 2018; Frota et al., 2022). (4) Furthermore, we predict that an increase in phylogenetic dispersion corresponds to an increase in the dispersion of traits (Yang et al., 2015). In other words, phylogenetic beta distance serves as a proxy for functional distance, and changes in phylogenetic beta diversity are therefore largely influenced by environmental filtering (Hardy et al., 2012). (5) Finally, based on previous studies (Kadmon, 1995; Condit et al., 2002; Graham and Fine, 2008; Hardy et al., 2012; Schneider et al., 2019; Hu et al., 2022), we predict that changes in the turnover components of different aspects of beta diversity can be influenced by both environmental filtering and dispersion limitation. However, changes in the nested components of the three aspects of beta diversity are likely to be primarily influenced by dispersion limitation.
2. Materials and methods
2.1. Location of study
The Taizi River (Figure 1) is situated in northeastern China and plays a crucial role in fostering economic and social progress in the region. Unfortunately, the watershed ecosystem has experienced severe degradation in recent years owing to the deteriorating environmental conditions in the Taizi River and a gradual transition toward farmland and urban land use (Kaishan et al., 2014; Zhang et al., 2015). Despite the growing concern over this issue (Kong et al., 2013), the conservation and restoration of biodiversity and ecosystem function remain uncertain since there is limited understanding of the interrelationships between biological variation and watershed drivers (Zhang et al., 2019).
2.2. Macroinvertebrate sampling
In May 2012 and April 2018, we conducted field sampling at 92 sites along the Taizi River. Specifically, we surveyed 42 sites in 2012 and 50 sites in 2018. Given that the surveys were conducted 6 years apart, we selected the 2018 sites to be as geographically close as possible to the 2012 sites and have similar environmental characteristics, in order to reduce errors in our data. At each site, representative habitats were identified within 100 m of the river using a Surber-net (0.09 m2), and the sampling was repeated five times. Subsequently, samples were collected by filtering out impurities using a 60 mesh (0.25 mm pore size) circular sieve. The organisms were then poured into white porcelain trays, and macroinvertebrate samples were manually picked on-site using pointed forceps and placed into 500 mL wide-mouth plastic bottles, which were subsequently preserved in a pre-prepared 70% ethanol solution. Macroinvertebrate samples were identified in the laboratory using relevant taxonomic tools to species or genus level (with 3.7% of individuals identified to the family level; Liu et al., 1979; Morse et al., 1994; Merritt and Cummins, 1996).
2.3. Functional traits of macroinvertebrates
A total of nine functional traits (including voltinism, dispersal, drift, respiration, rheophily, size, thermal tolerance, activity habits, and functional feeding group) from 32 categories were selected to describe the functional structure of macroinvertebrate communities in this study. The nine traits cover life history, resistance or resilience, and essential biological characteristics. In order to calculate the functional beta diversity of species, we classified the functional traits of each species using a binary “presence-absence” matrix, where 1 indicates the presence and 0 indicates the absence of the trait. We then obtained a binary “presence-absence” (1–0) data matrix of functional traits for macroinvertebrates. The classification of species traits was primarily accomplished through field observations, laboratory measurements, literature reviews, and reference books. When information on certain species traits was lacking, the traits of the genus level were utilized (Morse et al., 1994; Merritt and Cummins, 1996; Usseglio-Polatera et al., 2000; Wang, 2003; Tomanova and Usseglio-Polatera, 2007; Barnum et al., 2017).
2.4. Proxy for phylogenetic information
Due to the lack of true phylogenetic data for the species, here we calculated taxonomic distances for path lengths in the taxonomic tree as a proxy for the phylogenetic data (Winter et al., 2013). The levels of the taxonomic tree include species, genus, family, order, class, phylum. The species classification distances are calculated using the “taxa2dist” function in R’s vegan package (Oksanen et al., 2013).
2.5. Environmental variables
After collecting macroinvertebrate samples, we measured 18 environmental factors. The YSI-85 portable water quality analyzer was used to determine pH, water temperature, conductivity, and dissolved oxygen. Elevation, latitude, and longitude were recorded on-site using the MAGELLAN global positioning system (eXplorist 200). We measured water depth and velocity on-site using a stream gauge, and river width was measured with a rangefinder. To quantify the volumes of different substrate types, we used measuring cups. The substrate index (SI) was computed using the following formula (Allan et al., 2021): SI = 0.08%V (boulder) + 0.07%V (large cobble) + 0.06%V (small cobble) + 0.05%V (large pebble) + 0.04%V (small pebble) + 0.03%V (coarse gravel) + 0.02%V (fine gravel) + 0.01%V (sand), where V represents the volume (L) of each substrate type. Furthermore, water samples were obtained on-site from each sampling location and transported to the laboratory for subsequent analysis. According to relevant standards (Wei et al., 1989), we measured the permanganate index (CODMN), ammonia nitrogen (NH4), total nitrogen (TN), and total phosphorus (TP) in the laboratory. Subsequently, land use data were downloaded from GlobeLand301 which were pre-processed using ENVI 5.02 to correct for atmospheric and geometric distortions and other image data pre-processing procedures, followed by supervised classification using the support vector machine method (Pisner and Schnyer, 2020). The resulting processed data was used to extract land cover data from the catchment area upstream of each sampling point using ArcGis 10.83 extraction analysis. Six land use types, including forest land, farmland, grassland, urban land, and water areas, were identified and counted as environmental factors in subsequent analyses.
2.6. Data analysis
2.6.1. Mean differences between macroinvertebrate communities and environmental conditions
Prior to the statistical analysis, log (x + 1) transformations were first applied to the environmental factors (except pH). To quantify differences and heterogeneity in environmental variables and community composition between network locations. We ran a Permutational multivariate analysis of variance (PERMANOVA; Anderson and Walsh, 2013) and homogeneity of multivariate dispersion (PERMDISP; Anderson et al., 2006) on environmental data and species composition data to analyze their differences and heterogeneity. These analyses were based on environmental data based on Euclidean distances and species abundance data based on Bray-Curtis distances.
2.6.2. Calculation of beta diversity
To begin with, we generated three dissimilarity distance matrices based on species incidence data. These matrices included (i) Simpson pairwise dissimilarity, which represents turnover components, (ii) nestedness-resultant dissimilarity, which represents nested components, and (iii) Sorensen pairwise dissimilarity, which is the sum of the two components. The calculation was performed using the “beta.pair” function from the R package betapart, utilizing the “Sorensen dissimilarity index” (Baselga, 2010, 2012). We utilized presence-absence data for species traits and calculated trait distances based on Gower distances (Gower, 1971). The distance matrix was then used to perform Principal Coordinate Analysis (PCoA; Villéger et al., 2008), and reduce the dimensionality of the trait data to two dimensions. Subsequently, we employed the “functional.beta.pair” function from the betapart package to obtain three distance matrices based on the reduced dimensionality of trait data. These distance matrices were used to measure the spatial turnover and nestedness components of functional beta diversity, as well as the sum of the two values. We then calculated the taxonomic distance between species using the “taxa2dist” function (Clarke and Warwick, 1998). Using the interspecific taxonomic distance matrix, we computed the phylogenetic beta diversity of the organisms. The phylogenetic beta diversity matrix was obtained through the same approach as for functional beta diversity, and we also obtained a phylogenetic turnover component and a nested phylogenetic component. Finally, we used the “beta.multi” and “functional.beta.multi” functions from the betapart package (Baselga and Orme, 2012) to compute the beta diversity turnover and nestedness components of the taxonomic, functional, and phylogenetic beta diversity for three multiple-site dissimilarities, as well as the sum of these two values.
2.6.3. Calculation of spatial and environmental distances
As macroinvertebrate dispersal is mainly concentrated in river corridors, we used watercourse distances as a proxy to investigate the processes related to dispersal. To create the watercourse distance matrix, we employed network analysis in ArcGIS 10.84 to build a network. Next, we normalized the environmental variables and used the “Euclidean distance” approach to derive a matrix of environmental distances between pairs of locations (Cañedo-Argüelles et al., 2015).
2.6.4. The relationship between the decay of the dissimilarity, taxonomic, functional, and phylogenetic beta diversity of large invertebrate communities and their environmental and spatial distance
First, we used the “mantel.correlog” function (Shi et al., 2015) of the R package vegan to examine the spatial autocorrelation of different network locations (basin, mainstream, tributaries) of macroinvertebrate communities and different aspects (taxonomic, functional, phylogenetic) of the macroinvertebrate community beta diversity. To investigate the correlation between macroinvertebrate community differences, taxonomy, function, phylogenetic beta diversity and its components (total, turnover, and nested) with environmental and watercourse distances, respectively, we used Mantel tests with Moran Spectral Randomization (MSR; Crabot et al., 2019), which accounts for the spatial autocorrelation of the environment. We used the “partial.mantel” function from the vegan package to conduct a partial Mantel test to investigate the impact of each distance matrix on dissimilarity indices while disregarding the effect of the other distance matrix. The MSR permutation is computed using the “mantel.randtest” function from the ade4 package (Dray and Dufour, 2007) and the “msr” function from the adespatial package (Dray et al., 2018). Finally, we have identified significant environmental variables through the forward selection of db-RDA analysis (Legendre and Anderson, 1999) for macroinvertebrate communities at various network locations, aspects of beta diversity, and its components. Prior to the RDA analysis, we performed a Hellinger transformation on the abundance data and the beta diversity matrix. All data analyses in this study were conducted using R version 4.1.2 (Team RC, 2022).
3. Results
3.1. Biological data and environmental conditions
During our study, a total of 6,997 individuals belonging to 106 taxonomic groups, 40 families, 15 orders, 6 classes, and 3 phyla were identified collectively. The most abundant taxa comprised Ephemera orientalis (17.14% relative abundance), Hepyageniidae (5.85% relative abundance), and Baetis sp. (5.82% relative abundance). Our analysis revealed significant differences in the environmental conditions between the sampling sites, indicating a high environmental gradient in the research area (Table 1). Specifically, river width (RW), water depth (WD), ammonia (NH4), total nitrogen (TN), and percentage of water area were significantly (p < 0.05) higher in the mainstream communities than in the tributary communities. In contrast, elevation (ALT), substrate index (SI), and percentage of forest land were significantly (p < 0.05) higher in the tributary communities than in the mainstream communities (Table 1).
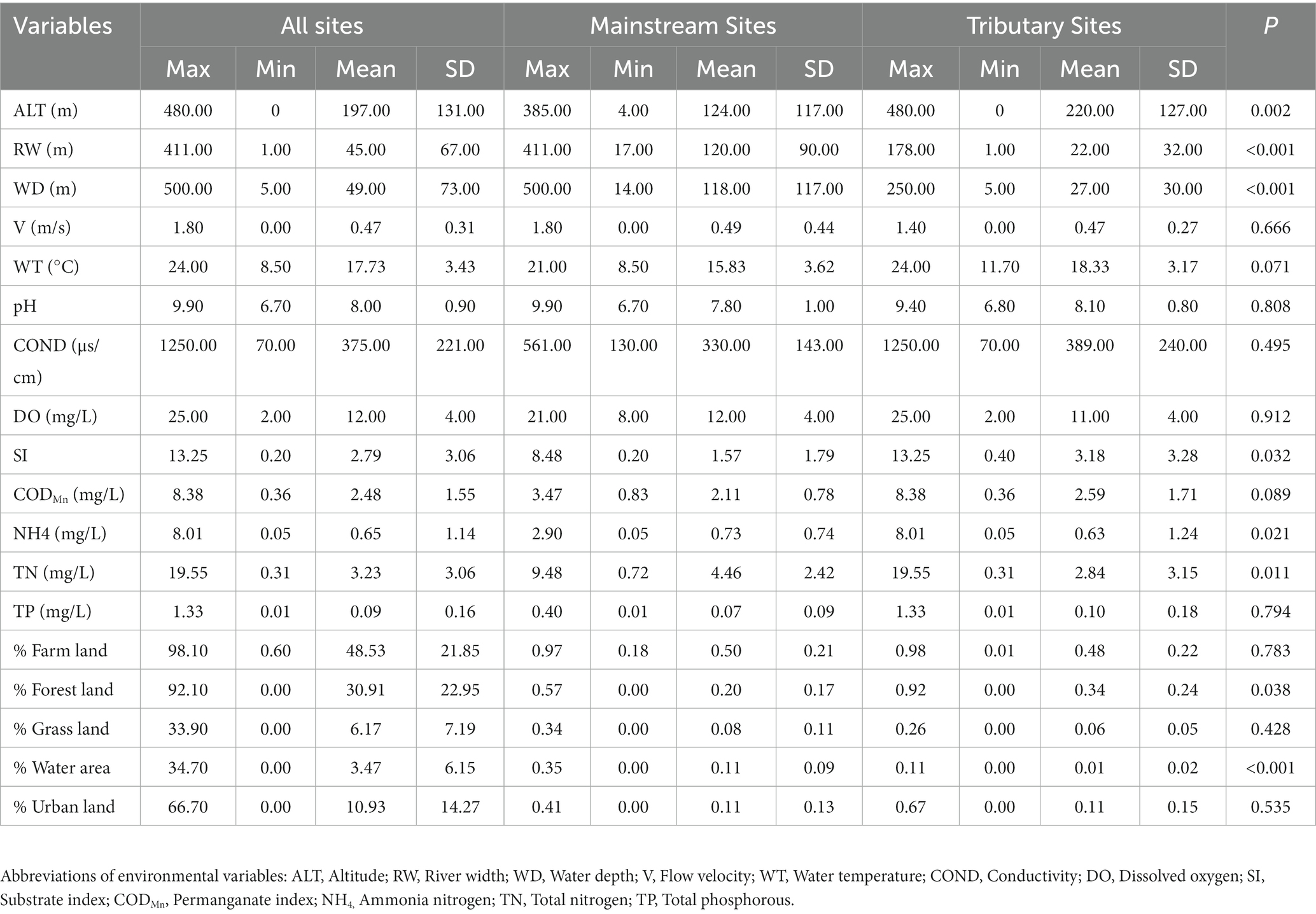
Table 1. Descriptive statistics of environmental variables and the results of non-parametric tests for environmental variables (p-values).
3.2. Mean differences in biological data and mean differences in environmental conditions
The results of the study indicate that there are significant differences in the community composition between the mainstream and tributaries of the network sites (R2 = 0.12915, p = 0.001), as demonstrated by the PERMANOVA analysis (Tables 2, 3). Additionally, network location (R2 = 0.12915, p = 0.001) had a notable impact on the variability of environmental conditions, as evidenced in the results presented in this study. Furthermore, the PERMDISP analysis (Figures 2, 3) revealed significant differences in macroinvertebrate community heterogeneity (p < 0.01) and environmental heterogeneity (p < 0.05) between network locations.
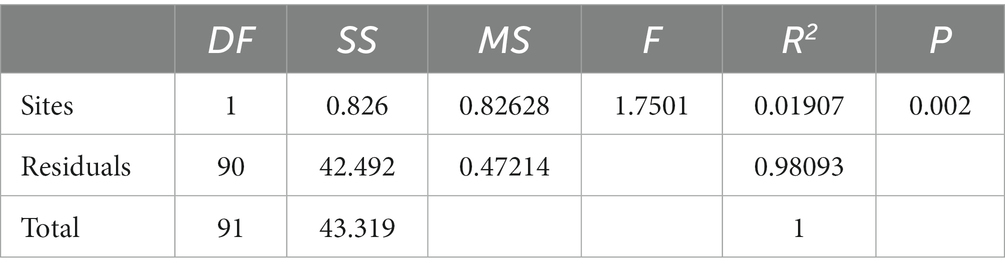
Table 2. Results of PERMANOVA for macroinvertebrate community composition at different network locations.
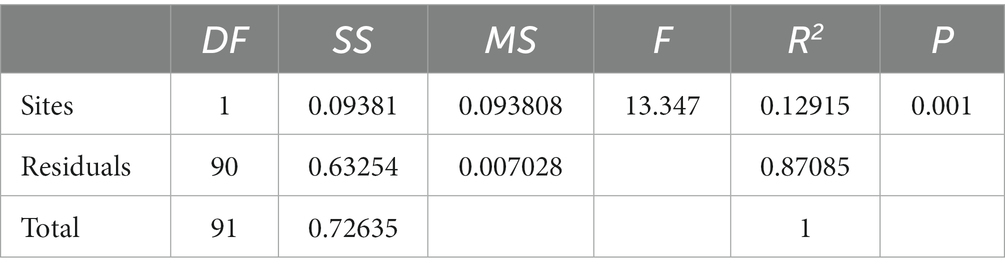
Table 3. Results of PERMANOVA for macroinvertebrate environmental conditions at different network locations.
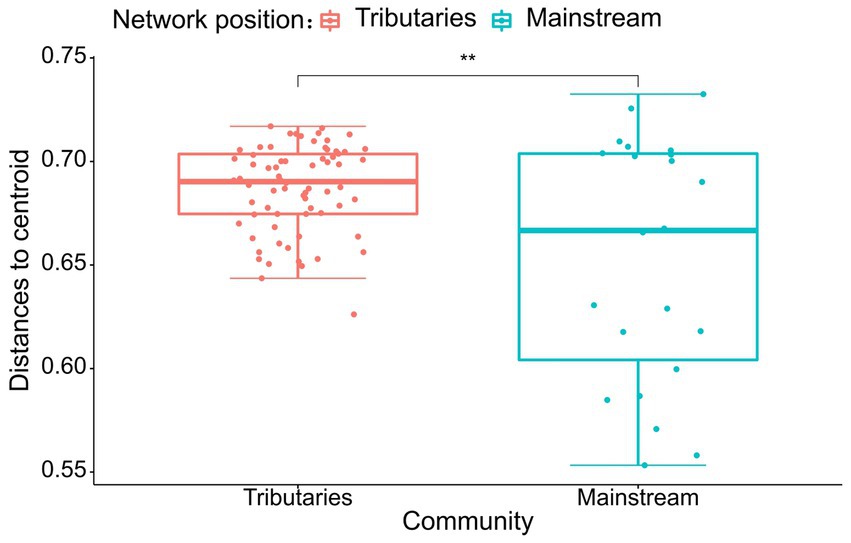
Figure 2. PERMDISP analysis of macroinvertebrate abundance (Bury-Curtis distance) based on the network location.
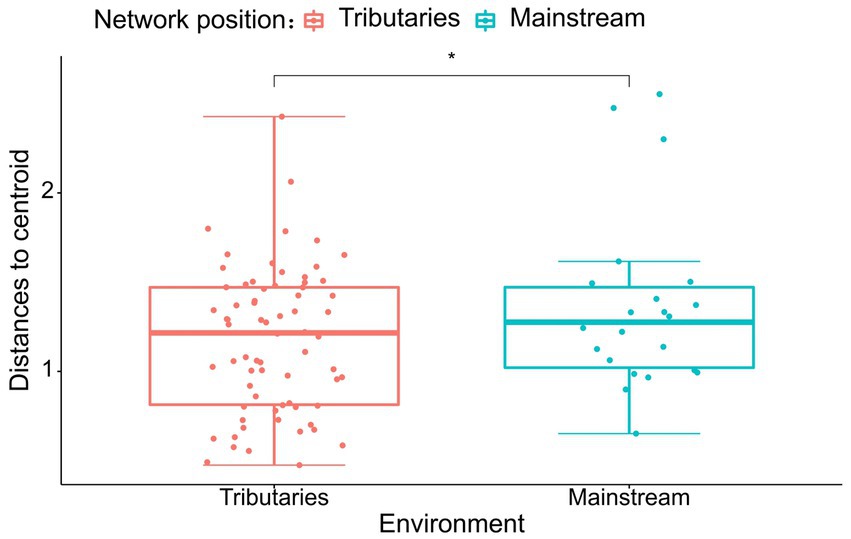
Figure 3. PERMDISP analysis of environmental data (Euclidean distance) based on the network location.
3.3. Taxonomic, functional, phylogenetic beta diversity, and their components
The findings of this study reveal that the macroinvertebrates present in the investigated area exhibit a significant level of variation in taxonomic beta diversity (0.98), which is the highest among the three types of beta diversity. The next highest variation was observed in phylogenetic beta diversity (0.80), followed by functional beta diversity (0.76; Figure 4). Moreover, our research indicates that taxonomic beta diversity is primarily driven by turnover (0.97), whereas both turnover (0.46) and nestedness (0.34) contribute to phylogenetic beta diversity. On the contrary, nestedness (0.19) has a lower contribution to functional beta diversity, while turnover (0.57) plays a more prominent role in functional beta diversity (Figure 4).
3.4. Differences in the individual components of beta diversity
The Mantel test findings suggest that there exist significant differences in the correlations between the components of the three dimensions of beta diversity (Figure 5). Specifically, there is a high correlation (r > 0.45, p < 0.001) between the components of functional diversity and phylogenetic diversity. Moreover, the functional total beta diversity exhibits a strong correlation with the phylogenetic total beta diversity (r = 0.6437, p < 0.001), followed by the functional turnover component and the phylogenetic turnover component, which are also highly correlated (r = 0.5911, p < 0.001). However, the correlations between other components are relatively low (r < 0.40, p < 0.001; Figure 5).
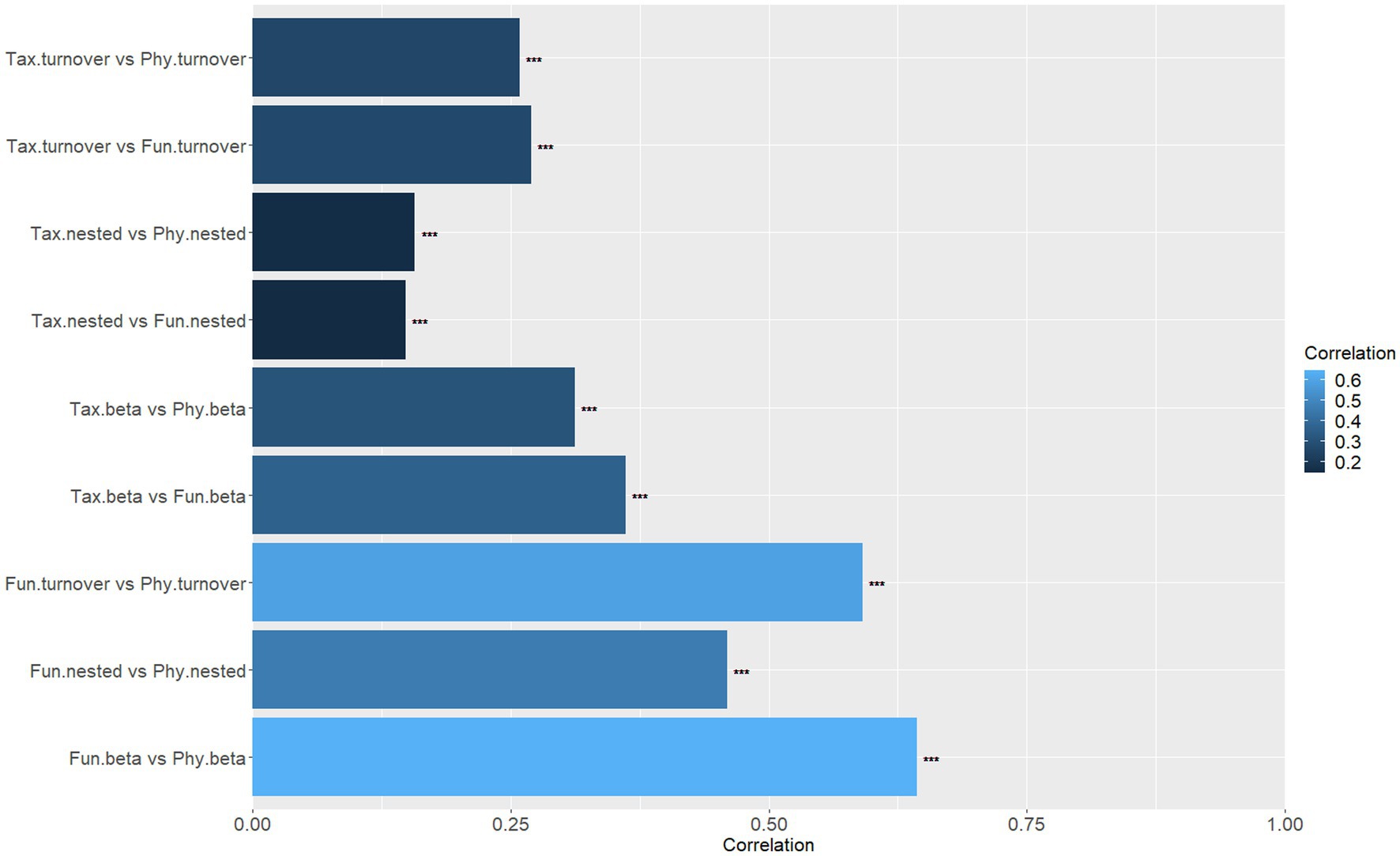
Figure 5. Mantel correlation coefficients between different components (beta diversity; turnover; nested) of different aspects of beta diversity (taxonomic, functional, phylogenetic; ***p < 0.001).
3.5. Correlation of environmental and spatial distances with macroinvertebrate communities and three aspects of beta diversity
In terms of macroinvertebrate communities with varying network locations, it was found that solely the mainstream community exhibited spatial autocorrelation at a distance class index of 30 km (Figure 6). Conversely, weak spatial autocorrelation was detected for all three similarity matrices of taxonomic beta diversity (Figure 6). Interestingly, the Sorensen similarity matrix for functional beta diversity demonstrated positive spatial autocorrelation at the first two distance class indices (Figure 6). Furthermore, our investigation revealed weak spatial autocorrelation for the three similarity matrices of phylogenetic beta diversity (Figure 6).
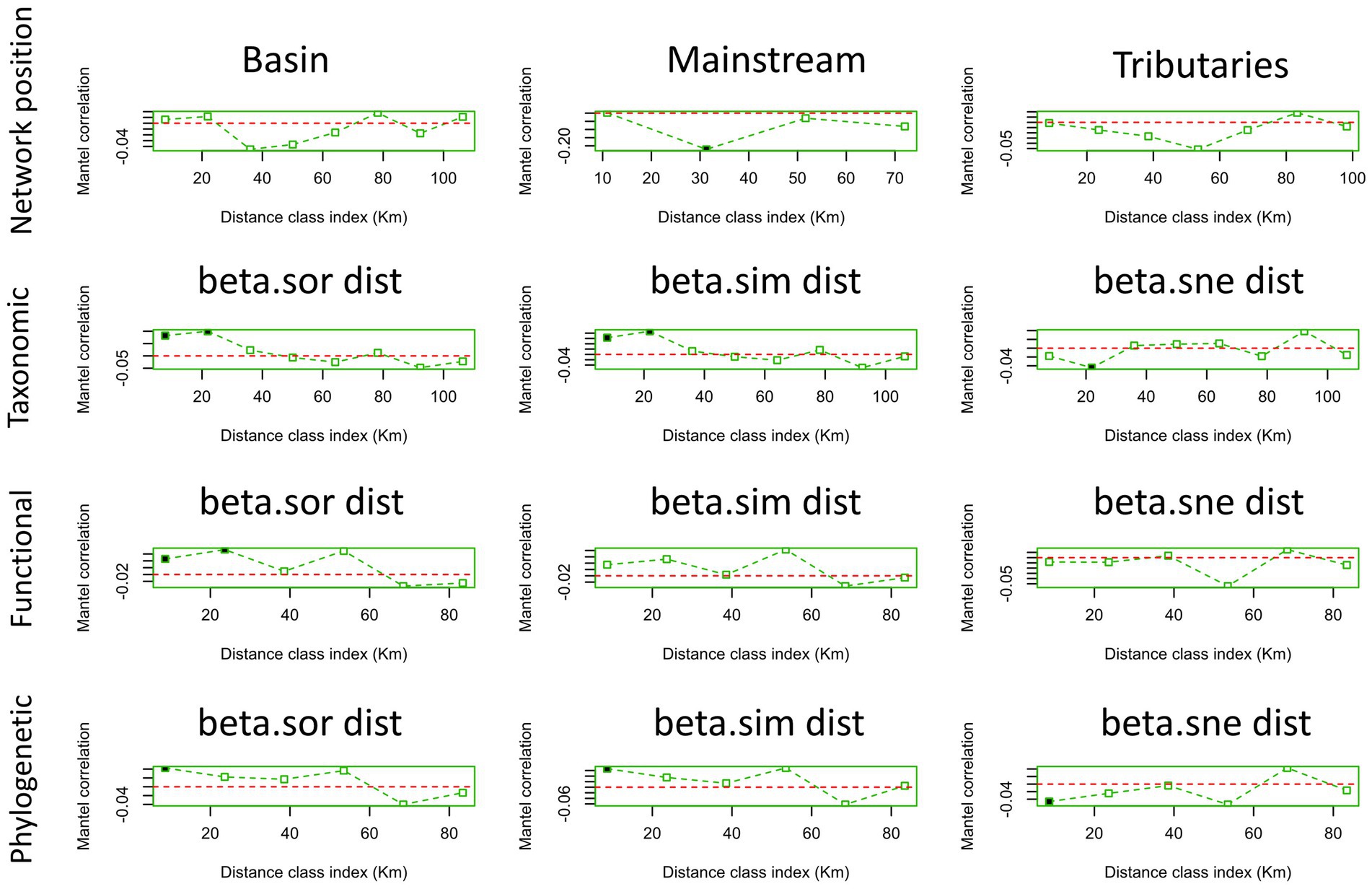
Figure 6. Mantel correlogram of macroinvertebrate communities at different network locations (basin, mainstream, tributaries) and multiple aspects of macroinvertebrate beta diversity (taxonomic, functional, phylogenetic). A solid point indicates the presence of spatial autocorrelation, and a hollow one the absence of it. Here, when we say “basin,” we refer to all points, including the mainstream and tributaries.
In general, the crucial environmental factors affecting macroinvertebrate communities and the diverse aspects affecting beta diversity exhibited significant variability among the sites (Tables 4–7). Specifically, elevation, water depth, water temperature, pH, conductivity, dissolved oxygen, substrate index, and permanganate index were the most critical environmental variables for macroinvertebrate communities throughout the watershed (Table 4). Among these variables, water temperature, substrate index, and permanganate index were particularly important for the mainstream community. In the tributaries, the macroinvertebrate community was primarily shaped by elevation, pH, water temperature, total nitrogen, and percentage of water area (Table 4). Notably, a variety of environmental variables, such as elevation, water depth, pH, conductivity, substrate index, percentage of water area, dissolved oxygen, percentage of forest land, percentage of urban land, river width, permanganate index, and total nitrogen, played a vital role in driving changes in taxonomic beta diversity (Table 5). Additionally, changes in functional beta diversity were significantly associated with elevation, water temperature, pH, and conductivity (Table 6). For phylogenetic beta diversity, the most critical environmental variables were water temperature, pH, water depth, and flow rate (Table 7). Finally, the extent to which spatial and environmental distance influenced macroinvertebrate communities and multifaceted beta diversity varied across network locations (Figures 7, 8; Table 8).
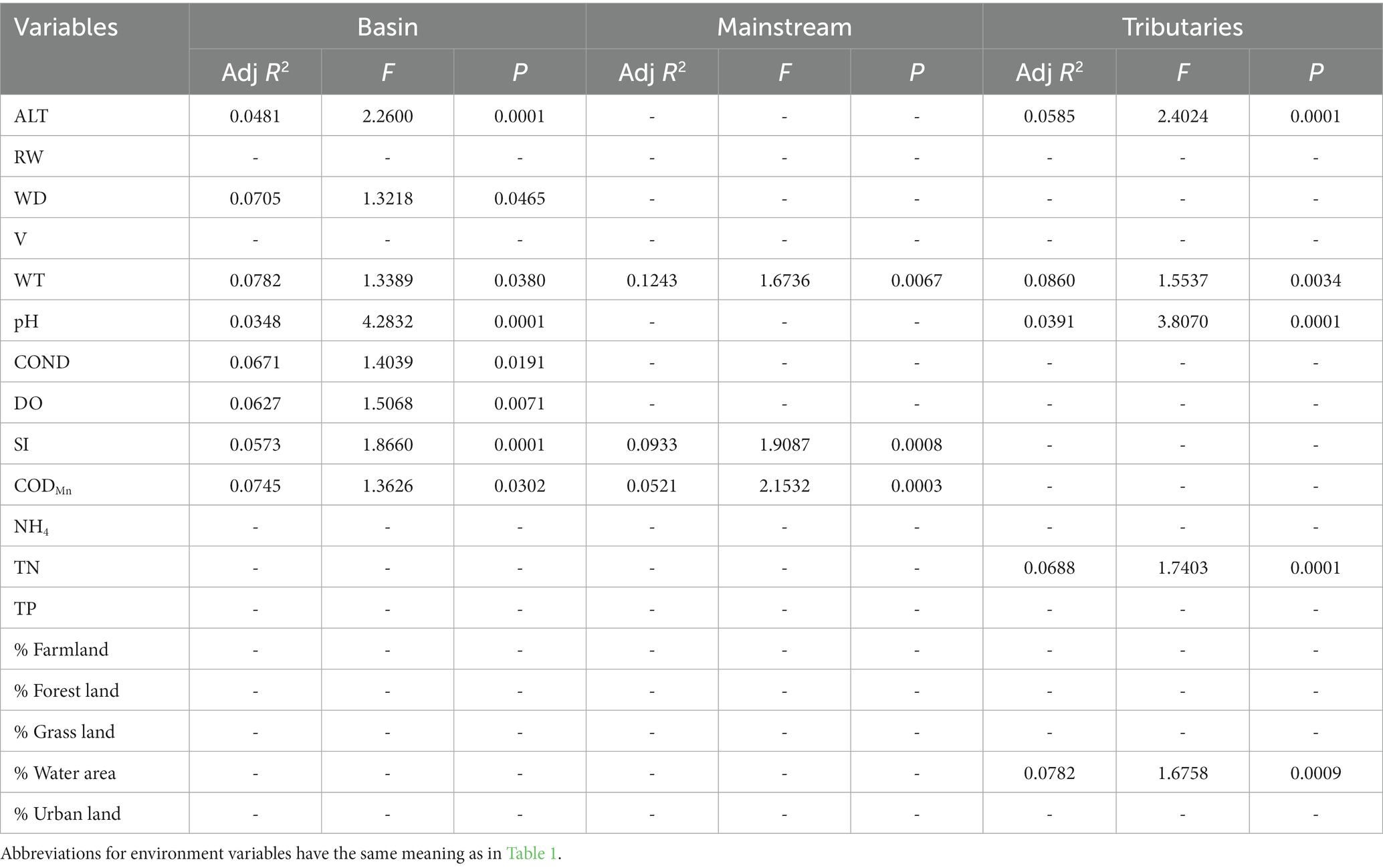
Table 4. The results of forward selection of significant environmental variables for macroinvertebrate communities at different network locations using db-RDA are presented, with a “-” indicating environmental variables that were not selected.
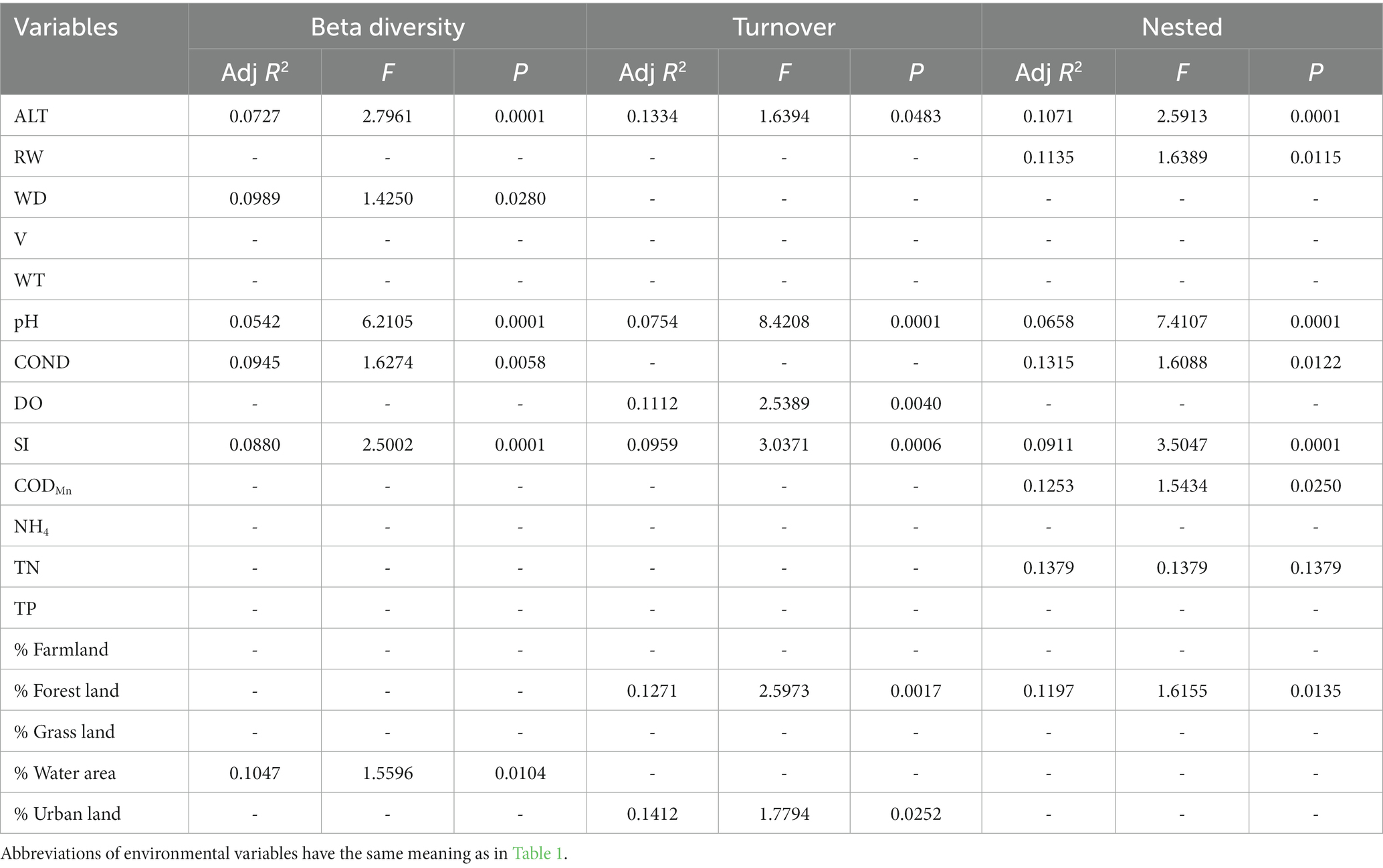
Table 5. The results of forward selection for significant environmental variables based on db-RDA for taxonomic beta diversity are presented, with a “-” indicating environmental variables that were not selected.
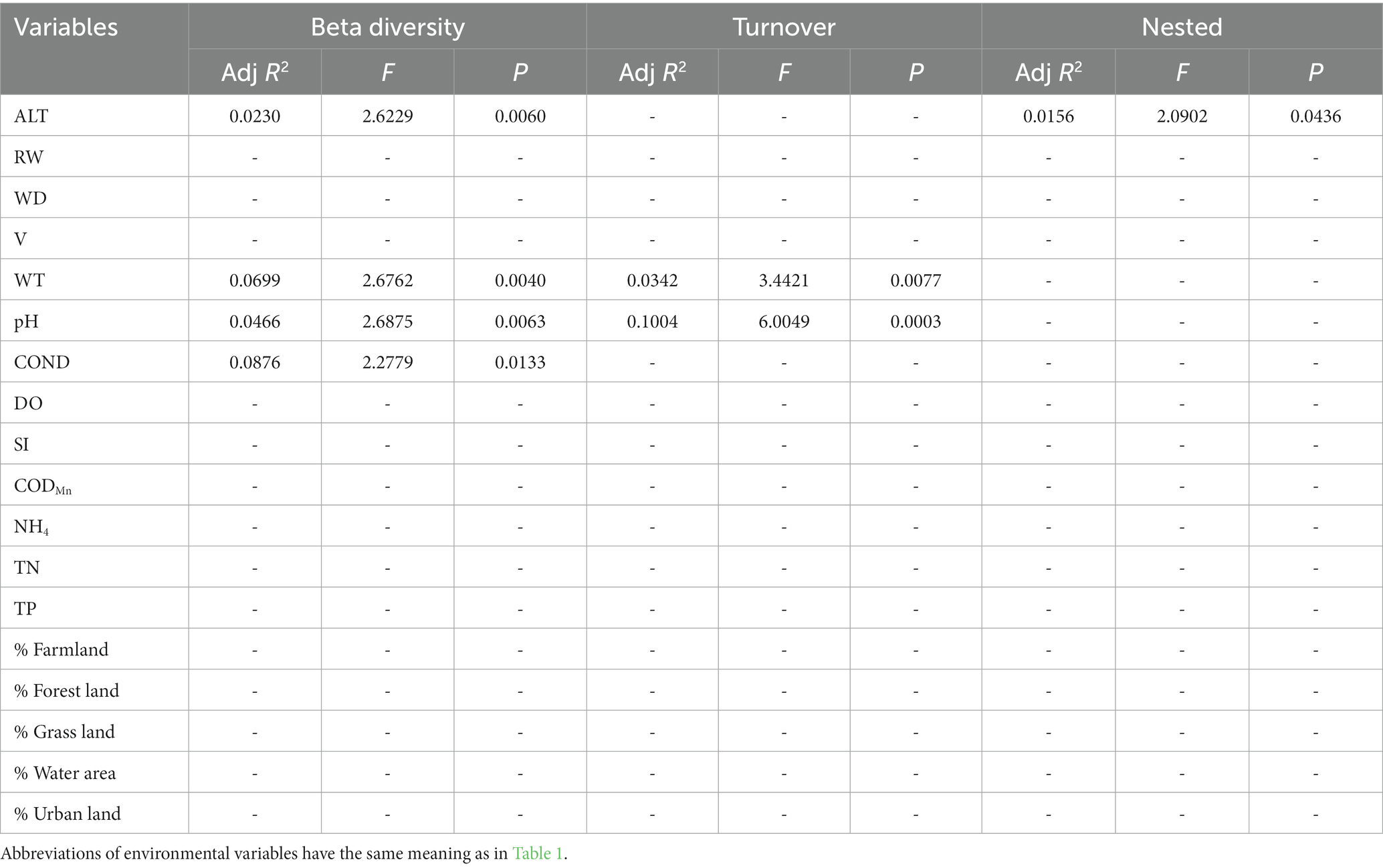
Table 6. The results of forward selection for significant environmental variables based on db-RDA for functional beta diversity are presented, with a “-” indicating environmental variables that were not selected.
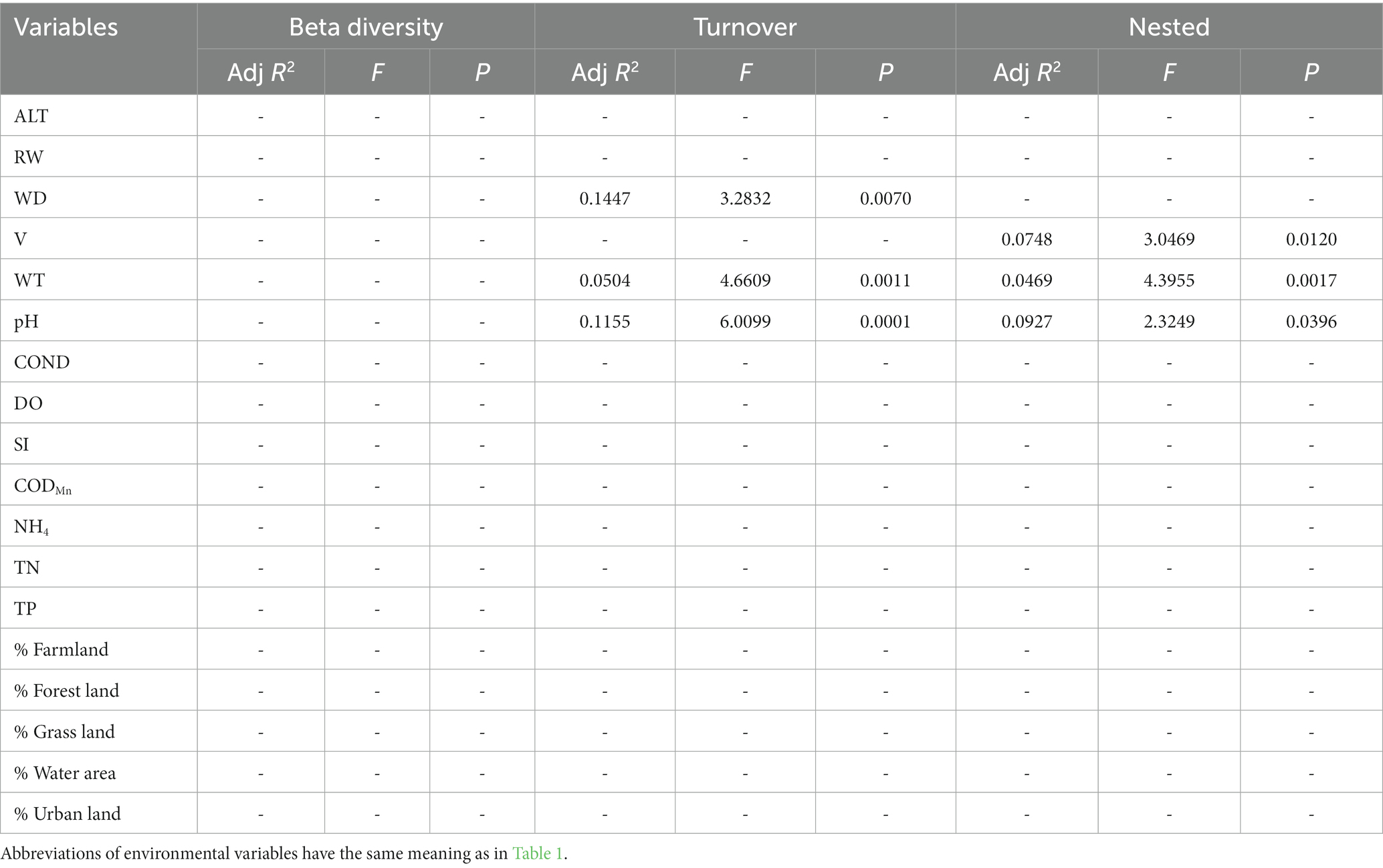
Table 7. The results of forward selection for significant environmental variables based on db-RDA for phylogenetic beta diversity are presented, with a “-” indicating environmental variables that were not selected.
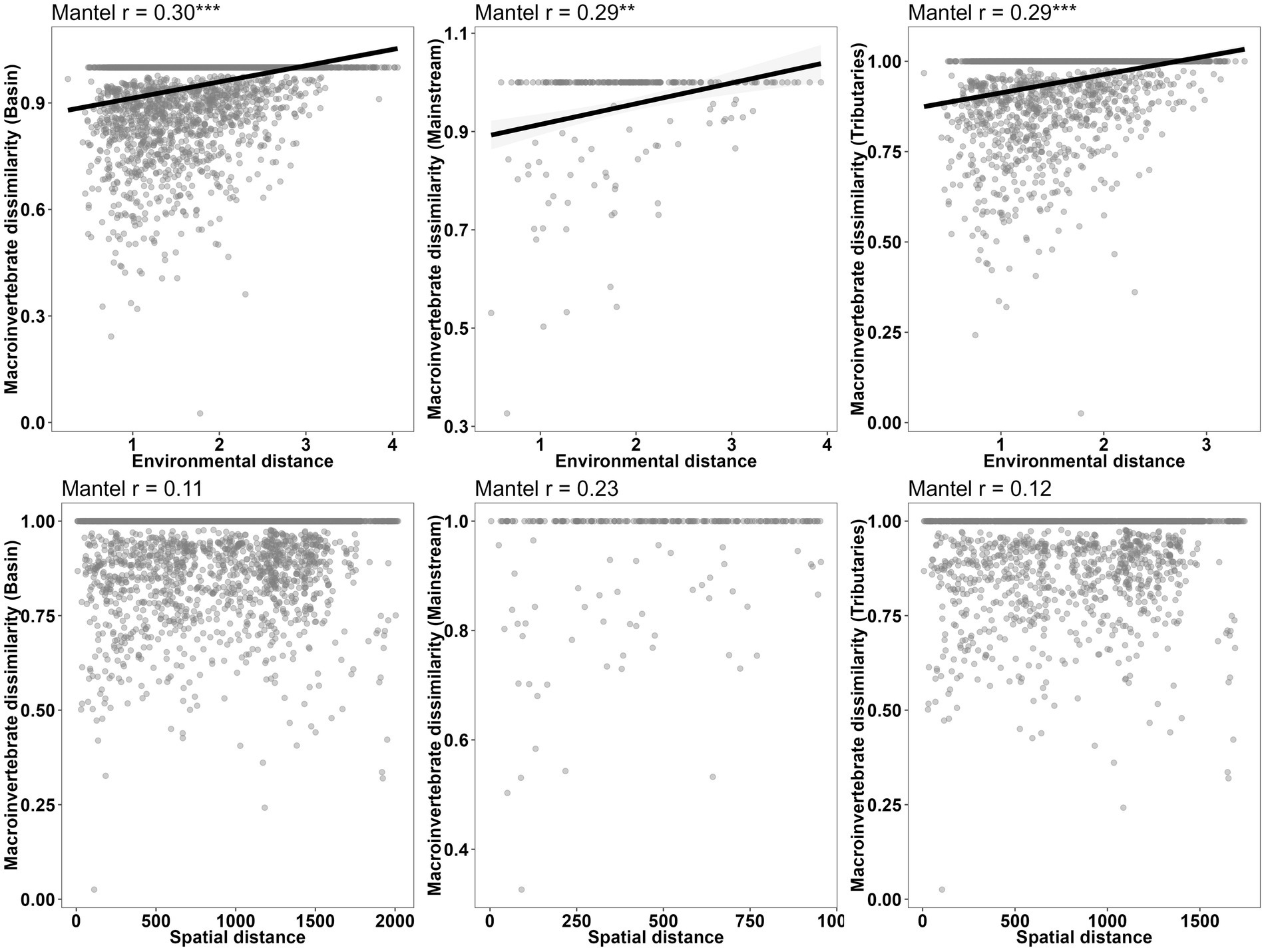
Figure 7. Relationship between abundance dissimilarity and environmental or spatial distances of macroinvertebrate communities in different network locations (basin, mainstream, and tributaries).
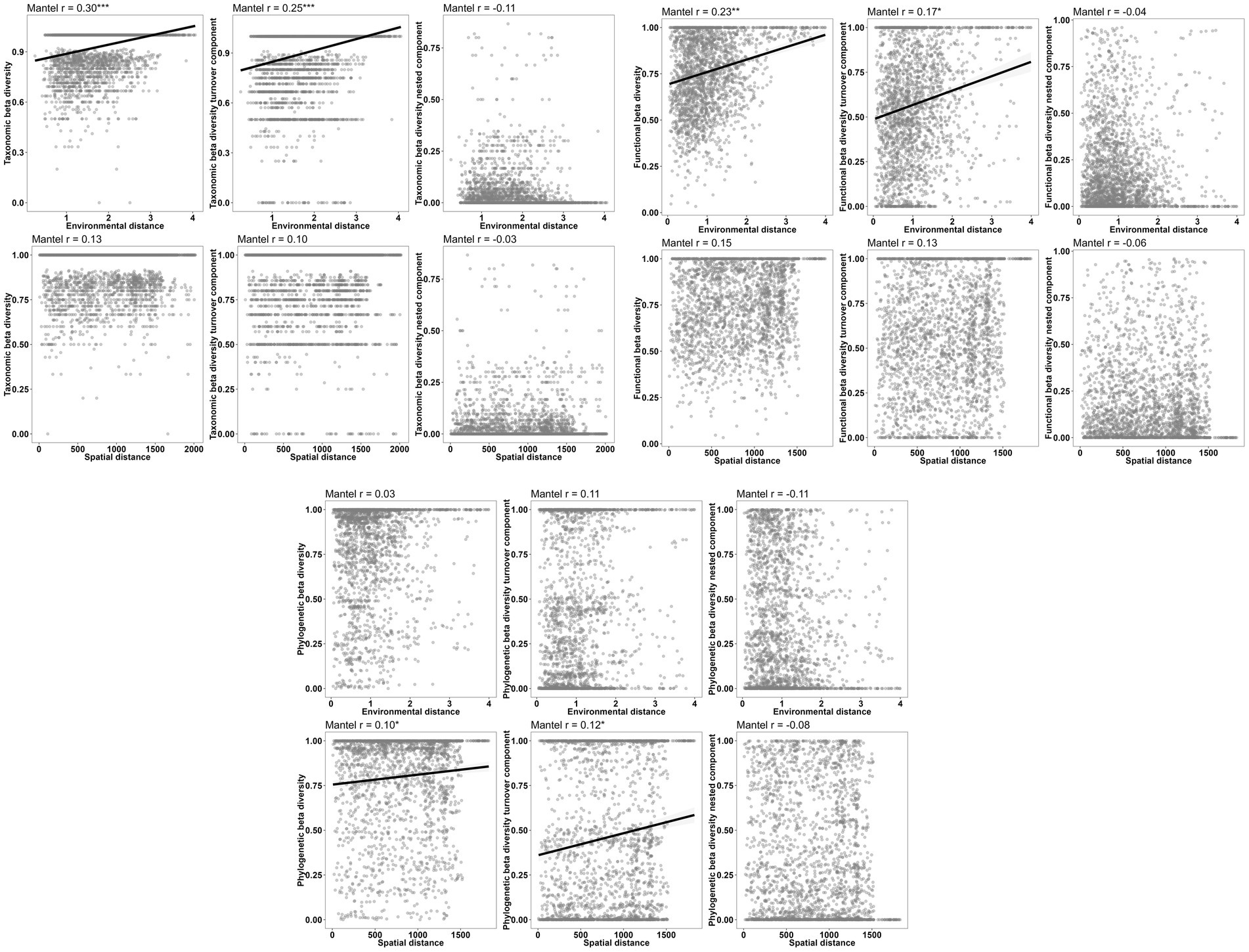
Figure 8. Relationships between individual components of the three aspects of beta diversity and environmental and spatial distances.
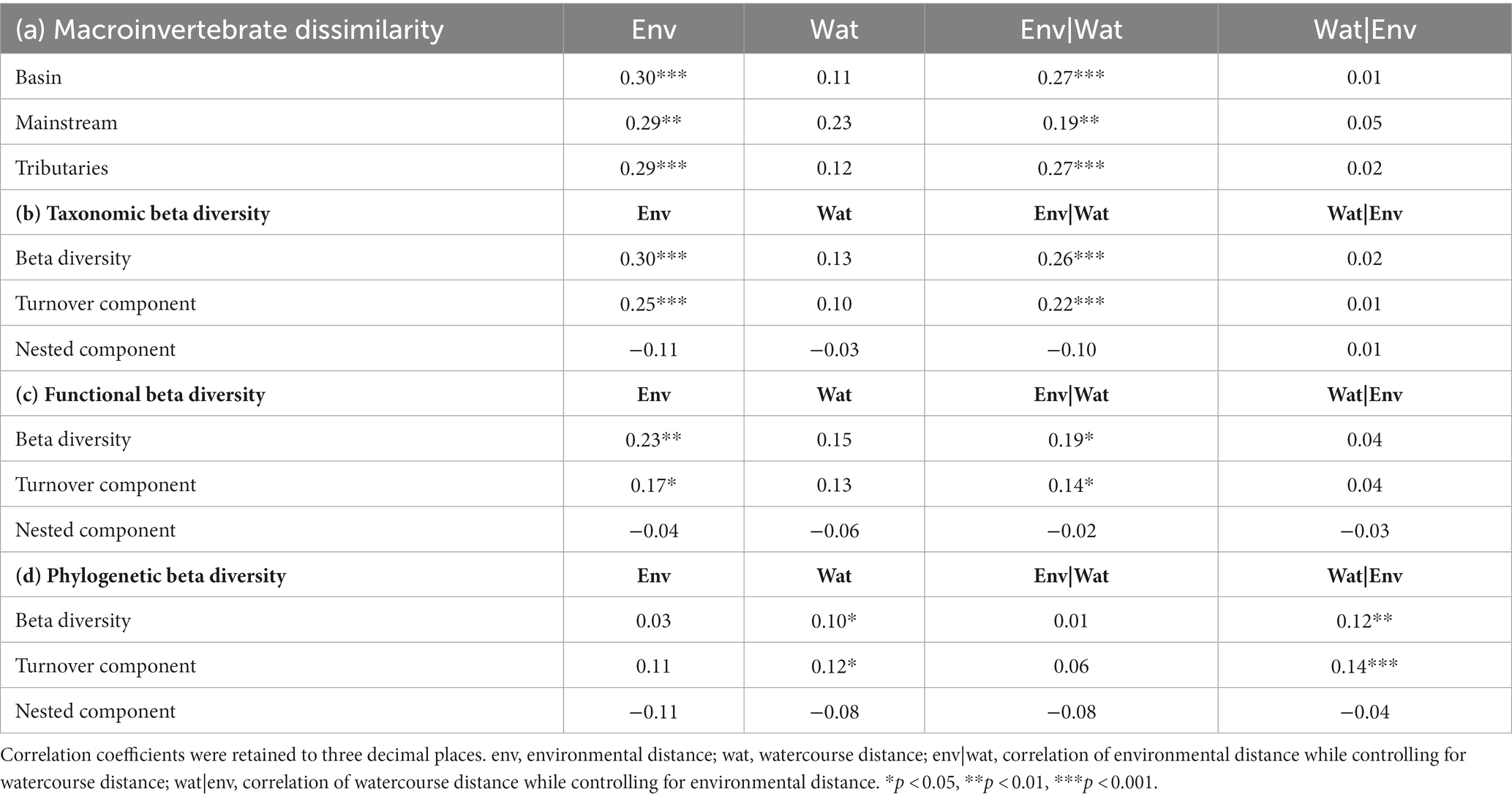
Table 8. The results of the Mantel test and partial Mantel test for Moran spectral randomization of macroinvertebrate dissimilarity, including basin, mainstream, and tributaries, and three aspects of macroinvertebrate beta diversity, taxonomic, functional, and phylogenetic beta diversity, were analyzed in relation to environmental and watercourse distances.
The correlation between macroinvertebrate metacommunities and environmental factors (r = 0.27, p < 0.001) was found to be stronger when considering the entire watershed (Figure 7; Table 8). Conversely, although correlations between mainstream communities and both environmental factors and watercourse distance were high, the correlation with watercourse distance was not significant. Additionally, the correlations between communities and watercourse distance were low and insignificant when the effects of environmental variables were not considered (r = 0.05, p > 0.05). Furthermore, a partial Mantel test indicated that the relative importance of environmental filtering was greater for tributary community assemblages compared to spatial processes (Figure 7; Table 8).
The results of the Partial Mantel tests reveal significant correlations between macroinvertebrate taxonomic beta diversity and the turnover component of taxonomic beta diversity with environmental distance. Conversely, not any correlation was found between nestedness and environmental distance. Although functional beta diversity also exhibited a significant correlation with environmental distance, the strength of this relationship was comparatively weaker than that observed for taxonomic beta diversity. Notably, the Sorensen dissimilarity matrix and Simpson dissimilarity matrix for phylogenetic beta diversity displayed significant correlations with spatial distance rather than environmental distance (Figure 8; Table 8).
4. Discussion
This study tested the NPH hypothesis for macroinvertebrate metacommunities in a boreal river basin in China. The NPH hypothesis posits that changes in tributary communities are driven by environmental filtering, while changes in mainstream communities are driven by both environmental filtering and spatial processes. We also investigated three aspects of beta diversity in response to environmental and spatial distance. Our findings suggest that environmental filtering almost entirely drives the metacommunities of macroinvertebrates in the watershed, mainstream, and tributaries. Thus, the mechanisms of assembly of regional macroinvertebrate metacommunities may not depend on network position. Additionally, we found that taxonomic beta diversity variation within the basin was more significant than functional and phylogenetic beta diversity variation. The turnover component was found to drive most of the variation in beta diversity, with species beta diversity almost entirely contributed by the turnover component. Furthermore, functional beta diversity showed a greater contribution from the turnover component than the nested component, while phylogenetic beta diversity was primarily interpreted by both turnover and nested components. Our findings suggest that the ecological factors driving various aspects of beta diversity exhibit distinct patterns, with deterministic processes predominantly influencing turnover in species and trait composition. Conversely, phylogenetic turnover is primarily driven by dispersal constraints. We observed significant effects of environmental filtering and dispersal limitation on the turnover components of different aspects of beta diversity. Nonetheless, nested components of beta diversity may have drivers other than environmental filtering and dispersal limitation, such as biotic interactions and ecological drift.
In line with recent research (Schmera et al., 2018; He et al., 2020), we have found that the network location hypothesis lacks general support in predicting the macroinvertebrate community assembly in the Taizi River basin, tributaries, and the mainstream. Our study indicates that community assembly is related to environmental filtering, rather than spatial processes. Moreover, the effects of ecological niche-based processes and spatial processes on community assembly may be independent of network location. Several studies have shown (Datry et al., 2016; Tonkin et al., 2018a) that the influence of ecological niches and spatial processes in shaping community assemblages is specific to the system under investigation, rather than the location of the network. River ecosystems exhibit significant variability, as reflected by the variation in environmental conditions such as water temperature, flow rate, ammonia, total nitrogen, total phosphorus, and conductivity. Additionally, river network connectivity varies with the season, and the connectivity of the river network is likely to increase during the wet season (Jiang et al., 2014; Zhao et al., 2017). Moreover, environmental heterogeneity differs from one river system to another. These factors may explain why the NPH hypothesis has not been widely supported. On the other hand, limitations to the study of the NPH hypothesis exist, as dispersal limitations may be negligible for aquatic insects with adult flight capabilities, even if they are in geographically remote locations, as they can easily fly several hundred kilometers (Schmera et al., 2018). Nevertheless, related studies have demonstrated that the NPH hypothesis is context-dependent, even for taxa that only disperse exclusively within streams (Henriques-Silva et al., 2019).
Our study has demonstrated that macroinvertebrate communities exhibit a strong distance-decay relationship with environmental distance. However, the influence of spatial distance on macroinvertebrate communities was not statistically significant. This suggests that environmental filtering is the primary cause of variation observed in macroinvertebrate communities in the Taizi River. Macroinvertebrates have moderate dispersal rates, which enables them to monitor changes in environmental gradients and locate suitable habitats. In line with previous studies (Forio et al., 2017; Nguyen et al., 2018) investigating the key environmental variables affecting river macroinvertebrates, we have identified geographic variables, such as elevation, physicochemical water quality variables (conductivity, flow rate, substrate, permanganate index, total nitrogen, and pH), and land use factors (percentage of urban land, water area, and forest land) as crucial environmental variables that impact macroinvertebrate community assembly and beta diversity in the Taizi River. Our research has revealed a relatively large range of variability in these environmental variables (Table 1), contributing to the substantial environmental gradient observed in the study area, which drives macroinvertebrate variation.
The correlations (Figure 5) among individual components of different aspects of beta diversity were found to be low. Therefore, we suggest that three aspects of beta diversity offer distinct insights into beta diversity (Heino and Tolonen, 2017). Our investigation revealed that taxonomic and functional beta diversity were predominantly composed of turnover. This suggests that differences in species and functional beta diversity within watersheds result from the interchange of species and functional traits across sites, largely driven by deterministic processes (Nunes et al., 2016). Furthermore, our findings indicate that taxonomic beta diversity is primarily influenced by environmental filtering and is subject to weaker spatial effects, which is consistent with recent studies (Liu et al., 2022; Wu et al., 2022). Less consistent with our third hypothesis, we did not find a significant effect of dispersal limitation on macroinvertebrate taxonomic beta diversity. In general, differences in species dispersal ability may interact with geographic isolation to form nested patterns of species composition (Kadmon, 1995). However, the taxonomic beta diversity in this study is mainly composed of turnover, with a minor nested component, which could explain the lack of a detectable effect of dispersal limitation on taxonomic beta diversity. The correlation between local environmental variables and taxonomic beta diversity underscores the importance of environmental filtering as an ecological process affecting riverine macroinvertebrate communities (Cottenie and De Meester, 2004; Viana et al., 2016). The role of environmental variables in community assembly primarily depends on the environmental heterogeneity of the study area (Li et al., 2021). In our study area, environmental characteristics such as water temperature, substrate index, permanganate index, total nitrogen, and others vary widely due to human disturbance (e.g., domestic sewage discharge, urbanization) and natural geographical factors (e.g., climate change, seasonal variation). As a result, environmental conditions act as a filter that selects species suitable for survival while excluding the sensitive ones (Cottenie and De Meester, 2004).
Surprisingly, we found that the taxonomic beta diversity of macroinvertebrates was exceptionally high (0.98), indicating that the composition of communities between different locations was almost entirely dissimilar. This could be due to the fact that, despite the high total abundance of 6,997 species in this study, the species composition at each location was relatively homogeneous (with an average of 75 individuals per location, but only 5 species per location). However, we also discovered that functional beta diversity (0.75) was lower than the taxonomic beta diversity. Additionally, functional beta diversity was not entirely driven by turnover, implying that species turnover between habitats does not cause a complete turnover of functional traits. This indicates that there is some degree of functional redundancy among different habitats. In fact, our investigation found that 76% of the study area is composed of aquatic insects belonging to 27 families. Moreover, we found that some functional traits of species within the same family are similar.
Consistent with previous research (Bispo et al., 2021; Liu et al., 2022), our findings indicate that functional beta diversity is associated with environmental distance, rather than spatial distance, supporting our second hypothesis. It is widely acknowledged in the field of ecology that environmental conditions play a crucial role in filtering species from the regional species pool based on their functional traits, such as their activity and feeding habits, dispersal patterns, and environmental tolerance. This process ultimately drives changes in functional beta diversity, strongly correlated with environmental filtering (McGill et al., 2006). Furthermore, species with similar functional traits tend to occupy similar ecological niches, while dissimilar ecological niches provide opportunities for greater functional trait diversity, leading to increased differences in functional beta diversity as environmental dissimilarity (i.e., distance) increases (Rusanov et al., 2022). However, our study results did not find a significant spatial effect, although the impact of spatial processes cannot be entirely disregarded. Our findings suggest that functional redundancy likely occurs across different locations, meaning that different species may share similar functional traits.
Our fourth hypothesis suggests that phylogenetic beta diversity should be highly correlated with functional beta diversity and be largely influenced by environmental filtering. Nevertheless, our Mantel test and partial Mantel test for the phylogenetic component of beta diversity did not reveal a significant role for environmental variables. Instead, we found that spatial distance had a significant effect. The observed spatial signal may be attributed to environmental variables whose spatial structure was not measured, resulting in a spatial structure on the community known as induced spatial dependence (Peres-Neto and Legendre, 2010). Despite the potential for confounding variables to influence our results, we assert that such an outcome is highly unlikely in our study. This confidence stems from our comprehensive assessment of a diverse array of environmental variables, including, but not limited to, hydrological parameters such as river width, depth, flow rate, and substrate index, and a suite of water quality physicochemical parameters encompassing water temperature, conductivity, dissolved oxygen, pH, ammonia nitrogen, total phosphorus, and permanganate index. Additionally, we considered key land use parameters within the catchment, such as the percentage of farmland and urban land (Mykrä et al., 2007), further bolstering our confidence in the validity of our findings. Considering the vast geographical extent of the study area, where the maximum river network distance between sampling sites spanned 406 km and encompassed an elevation gradient ranging from 0 to 480 m, it is highly likely that dispersal limitations are a key factor in shaping the patterns of biodiversity observed in the Taizi River. Specifically, the constraints imposed by dispersal limitations may affect the ability of organisms to respond to environmental conditions, leading to the occurrence of species in only a subset of environmentally suitable locations (Gianuca et al., 2017). Thus, dispersal limitation may promote higher levels of phylogenetic beta diversity, potentially reducing the amount of evolutionary history shared by species from different parts of the study area (Devictor et al., 2010; Hardy et al., 2012). Furthermore, we found that nestedness had a greater impact on functional and phylogenetic beta diversity in macroinvertebrates than taxonomic beta diversity. Despite its significance, we did not observe any significant correlation between nestedness and environmental or spatial factors, which contradicts our fifth hypothesis that nestedness and diffusion limitation are linked. Our results suggest that historical factors, biotic interactions, and stochastic processes may also play a significant role in driving high functional and phylogenetic beta diversity in the Taizi River. These factors may have contributed to the partial loss of functional traits and phylogeny observed in our study area. Notably, while we found some correlation between phylogenetic beta diversity and functional beta diversity, their mechanisms of change were inconsistent, and phylogenetic beta diversity was more variable than functional beta diversity. This suggests that changes in functional traits may be mainly caused by more distantly related species. Therefore, our results do not fully support the notion that phylogenetic distance can be used as a proxy for functional distance.
Our findings support the view that dividing beta diversity into turnover and nested components can provide complementary insights. We found that species are replaced at both spatial and temporal levels in terms of species composition, regardless of the role of ecological factors. This promotes species diversity by adding complexity to the ecosystem and providing more opportunities for new species to occupy vacant ecological niches, thereby increasing their use of ecological resources. From a functional and phylogenetic composition perspective, some species may share similar functional traits and affinities, and their ecological niches may overlap. They may use these resources at different times or spaces. Nested ecological niches can improve ecosystem stability and provide a suitable habitat for many different species. Therefore, it is essential to focus on multiple perspectives of beta diversity simultaneously and divide them into turnover and nested components. This approach provides different but complementary perspectives, leading to a better understanding of biodiversity in ecosystems and a more comprehensive picture of how biodiversity responds to ecological drivers.
However, it is worth noting that in certain cases, environmental and spatial distances may not be correlated with communities, suggesting that other unconsidered factors may influence community assembly and variation, such as interactions between organisms and the effects of climate. Furthermore, spatial distance may not fully account for dispersal-related processes, as it is merely a proxy for the actual dispersal distance of species. Additionally, both communities and riverine ecosystems exhibit high levels of unpredictability and variability, and unexplained community variation may be attributed to the effects of stochastic processes in the metacommunity assembly of freshwater macroinvertebrates (Heino et al., 2015a). Changes in environmental and physiographic conditions may result in species extinction or stochastic colonization and drift, further contributing to the observed variability in macroinvertebrate communities (Devictor et al., 2010). Meanwhile, the data used in this study spanned 6 years (2012 to 2018). Although we selected locations that were geographically close and had similar environments, the influence of time on river communities cannot be ignored. Therefore, we used PERMANOVA (permutational multivariate analysis of variance) to test the interaction between sampling year and network position on the impact of environmental factors (Appendix Table 1). The results showed that the interaction between sampling year and network position did not have a significant effect (R2 = 0.01102, p = 0.216) on the changes in environmental conditions. Hence, we believe that the results of this study are reliable. In general, our study underscores the predominant function of environmental filtering in molding macroinvertebrate communities in the Taizi River basin. Thus, conservation endeavors should prioritize the preservation of high levels of environmental heterogeneity, such as environmental gradients, in the local watershed to encourage the conservation of local macroinvertebrate communities. Additionally, we suggest that local environmental monitoring concentrates on water depth, temperature, pH, conductivity, dissolved oxygen, substrate index, permanganate index, total nitrogen, and flow velocity as crucial environmental variables. The present study highlights the significant impacts of environmental filtering and dispersal limitation on the taxonomic, functional, and phylogenetic beta diversity of macroinvertebrates in the Taizi River. However, future studies on macroinvertebrates in the region should also incorporate more information on the effects of biotic interactions and stochastic, to better understand their impact on different aspects of beta diversity.
There are different opinions on the conservation of beta diversity. Initially, the turnover component primarily contributes to discrepancies in the taxonomic and functional beta diversity of macroinvertebrates in the basin. Hence, safeguarding macroinvertebrate communities across the basin can assist in maintaining high taxonomic and functional beta diversity. If the objective is to conserve high local phylogenetic beta diversity, it may be necessary to protect regions with diverse species of varying evolutionary relationships. Concurrently, the spatial impacts identified in this study propose that upholding the habitat integrity and connectivity of the river network is also critical for community assembly.
5. Conclusion
Our results illustrate that ecological niche-based processes are the main drivers of macroinvertebrate communities, regardless of the location of the river network. Taxonomic beta diversity consists almost entirely of turnover, which drives functional beta diversity, and phylogenetic beta diversity is driven by a combination of turnover and nestedness. A highly variable species composition across sites results in very high taxonomic beta diversity in the basin, but some functional redundancy of species within the basin, leading to lower functional beta diversity compared to taxonomic beta diversity. Species turnover and loss together result in high phylogenetic beta diversity in the Taizi River basin. Ecological niche-based processes are the key agents of taxonomic beta diversity and functional beta diversity, while changes in phylogenetic beta diversity are largely influenced by spatial processes. Our results, therefore, emphasize that NPH predictions may not apply to all river systems. Uncovering the ecological drivers of different aspects of beta diversity adds useful insights for metacommunity studies and biodiversity conservation.
Data availability statement
The raw data supporting the conclusions of this article will be made available by the authors, without undue reservation.
Author contributions
BZ: conceptualization, methodology, data-analyses, writing—original draft, and review version. XY: writing—original draft and review version. All authors contributed to the article and approved the submitted version.
Funding
This study was supported by the National Natural Science Foundation of China (41977193) and the National Science and Technology Basic Resources Survey Program of China (2019FY101700).
Acknowledgments
We would like to express our gratitude to the editors at Frontiers and the two reviewers for their valuable feedback, it is through your comments that we were able to improve the quality of the manuscript.
Conflict of interest
The authors declare that the research was conducted in the absence of any commercial or financial relationships that could be construed as a potential conflict of interest.
Publisher’s note
All claims expressed in this article are solely those of the authors and do not necessarily represent those of their affiliated organizations, or those of the publisher, the editors and the reviewers. Any product that may be evaluated in this article, or claim that may be made by its manufacturer, is not guaranteed or endorsed by the publisher.
Supplementary material
The Supplementary material for this article can be found online at: https://www.frontiersin.org/articles/10.3389/fevo.2023.1131403/full#supplementary-material
Footnotes
1. ^http://www.globallandcover.com/
2. ^https://www.l3harrisgeospatial.com/Software-Technology/ENVI
3. ^https://www.esri.com/zh-cn/arcgis/products/arcgis-desktop
4. ^https://www.esri.com/zh-cn/arcgis/products/arcgis-desktop
References
Allan, J. D., Castillo, M. M., and Capps, K. A. (2021). Stream ecology: Structure and function of running waters. Cham, Switzerland: Springer Nature.
Anderson, M. J., Ellingsen, K. E., and McArdle, B. H. (2006). Multivariate dispersion as a measure of beta diversity. Ecol. Lett. 9, 683–693. doi: 10.1111/j.1461-0248.2006.00926.x
Anderson, M. J., and Walsh, D. C. I. (2013). PERMANOVA, ANOSIM, and the mantel test in the face of heterogeneous dispersions: what null hypothesis are you testing? Ecol. Monogr. 83, 557–574. doi: 10.1890/12-2010.1
Barnum, T. R., Weller, D. E., and Williams, M. (2017). Urbanization reduces and homogenizes trait diversity in stream macroinvertebrate communities. Ecol. Appl. 27, 2428–2442. doi: 10.1002/eap.1619
Baselga, A. (2010). Partitioning the turnover and nestedness components of beta diversity. Glob. Ecol. Biogeogr. 19, 134–143. doi: 10.1111/j.1466-8238.2009.00490.x
Baselga, A. (2012). The relationship between species replacement, dissimilarity derived from nestedness, and nestedness. Glob. Ecol. Biogeogr. 21, 1223–1232. doi: 10.1111/j.1466-8238.2011.00756.x
Baselga, A., and Orme, C. D. L. (2012). Betapart: an R package for the study of beta diversity. Methods Ecol. Evol. 3, 808–812. doi: 10.1111/j.2041-210X.2012.00224.x
Bispo, P. C., Branco, C. C. Z., Novaes, M. C., Costa, L. S. M., Yokoyama, E., Barfield, M., et al. (2021). Partitioning and drivers of aquatic insect beta diversity in mountain streams. Freshwater Sci 40, 644–658. doi: 10.1086/717485
Brown, B. L., and Swan, C. M. (2010). Dendritic network structure constrains metacommunity properties in riverine ecosystems. J. Anim. Ecol. 79, 571–580. doi: 10.1111/j.1365-2656.2010.01668.x
Campbell Grant, E. H., Lowe, W. H., and Fagan, W. F. (2007). Living in the branches: population dynamics and ecological processes in dendritic networks. Ecol. Lett. 10, 165–175. doi: 10.1111/j.1461-0248.2006.01007.x
Cañedo-Argüelles, M., Boersma, K. S., Bogan, M. T., Olden, J. D., Phillipsen, I., Schriever, T. A., et al. (2015). Dispersal strength determines meta-community structure in a dendritic riverine network. J. Biogeogr. 42, 778–790. doi: 10.1111/jbi.12457
Clarke, K. R., and Warwick, R. M. (1998). A taxonomic distinctness index and its statistical properties. J. Appl. Ecol. 35, 523–531. doi: 10.1046/j.1365-2664.1998.3540523.x
Condit, R., Pitman, N., Leigh, E. G., Chave, J., Terborgh, J., Foster, R. B., et al. (2002). Beta-diversity in tropical Forest trees. Science 295, 666–669. doi: 10.1126/science.1066854
Cottenie, K., and De Meester, L. (2004). Metacommunity Structure: Synergy of Biotic Interactions As Selective Agents and Dispersal as Fuel. Ecology 85, 114–119. doi: 10.1890/03-3004
Crabot, J., Clappe, S., Dray, S., and Datry, T. (2019). Testing the mantel statistic with a spatially-constrained permutation procedure. Methods Ecol. Evol. 10, 532–540. doi: 10.1111/2041-210X.13141
Daniel, J., Gleason, J. E., Cottenie, K., and Rooney, R. C. (2019). Stochastic and deterministic processes drive wetland community assembly across a gradient of environmental filtering. Oikos 128, 1158–1169. doi: 10.1111/oik.05987
Datry, T., Melo, A. S., Moya, N., Zubieta, J., De la Barra, E., and Oberdorff, T. (2016). Metacommunity patterns across three Neotropical catchments with varying environmental harshness. Freshw. Biol. 61, 277–292. doi: 10.1111/fwb.12702
Devictor, V., Mouillot, D., Meynard, C., Jiguet, F., Thuiller, W., and Mouquet, N. (2010). Spatial mismatch and congruence between taxonomic, phylogenetic and functional diversity: the need for integrative conservation strategies in a changing world. Ecol. Lett. 13, 1030–1040. doi: 10.1111/j.1461-0248.2010.01493.x
Doretto, A., Piano, E., and Larson, C. E. (2020). The river continuum concept: lessons from the past and perspectives for the future. Can. J. Fish. Aquat. Sci. 77, 1853–1864. doi: 10.1139/cjfas-2020-0039
Dray, S., Blanchet, G., Borcard, D., Guenard, G., Jombart, T., Larocque, G., et al. (2018). Package ‘adespatial’. R Package 2018, 3–8.
Dray, S., and Dufour, A.-B. (2007). The ade4 package: implementing the duality diagram for ecologists. J. Stat. Softw. 22, 1–20. doi: 10.18637/jss.v022.i04
Fagan, W. F. (2002). Connectivity, fragmentation, and extinction risk in dendritic metapopulations. Ecology 83, 3243–3249. doi: 10.1890/0012-9658(2002)083[3243:CFAERI]2.0.CO;2
Forio, M. A. E., Lock, K., Radam, E. D., Bande, M., Asio, V., and Goethals, P. L. M. (2017). Assessment and analysis of ecological quality, macroinvertebrate communities and diversity in rivers of a multifunctional tropical island. Ecol. Indic. 77, 228–238. doi: 10.1016/j.ecolind.2017.02.013
Frota, A., Ganassin, M. J. M., Pacifico, R., Gomes, L. C., and da Graça, W. J. (2022). Spatial distribution patterns and predictors of fish beta-diversity in a large dam-free tributary from a Neotropical floodplain. Ecohydrology 15:e2376. doi: 10.1002/eco.2376
García-Girón, J., Heino, J., García-Criado, F., Fernández-Aláez, C., and Alahuhta, J. (2020). Biotic interactions hold the key to understanding metacommunity organisation. Ecography 43, 1180–1190. doi: 10.1111/ecog.05032
Gianuca, A. T., Declerck, S. A. J., Lemmens, P., and De Meester, L. (2017). Effects of dispersal and environmental heterogeneity on the replacement and nestedness components of β-diversity. Ecology 98, 525–533. doi: 10.1002/ecy.1666
Gower, J. C. (1971). A general coefficient of similarity and some of its properties. Biometrics 27, 857–871. doi: 10.2307/2528823
Graham, C. H., and Fine, P. V. A. (2008). Phylogenetic beta diversity: linking ecological and evolutionary processes across space in time. Ecol. Lett. 11, 1265–1277. doi: 10.1111/j.1461-0248.2008.01256.x
Hardy, O. J., Couteron, P., Munoz, F., Ramesh, B. R., and Pélissier, R. (2012). Phylogenetic turnover in tropical tree communities: impact of environmental filtering, biogeography and mesoclimatic niche conservatism. Glob. Ecol. Biogeogr. 21, 1007–1016. doi: 10.1111/j.1466-8238.2011.00742.x
He, S., Soininen, J., Chen, K., and Wang, B. (2020). Environmental factors override dispersal-related factors in shaping diatom and macroinvertebrate communities within stream networks in China. Front. Ecol. Evol. 8:141. doi: 10.3389/fevo.2020.00141
Heino, J., Alahuhta, J., and Fattorini, S. (2015a). Phylogenetic diversity of regional beetle faunas at high latitudes: patterns, drivers and chance along ecological gradients. Biodivers. Conserv. 24, 2751–2767. doi: 10.1007/s10531-015-0963-z
Heino, J., Melo, A. S., Siqueira, T., Soininen, J., Valanko, S., and Bini, L. M. (2015b). Metacommunity organisation, spatial extent and dispersal in aquatic systems: patterns, processes and prospects. Freshw. Biol. 60, 845–869. doi: 10.1111/fwb.12533
Heino, J., and Tolonen, K. T. (2017). Ecological drivers of multiple facets of beta diversity in a lentic macroinvertebrate metacommunity. Limnol. Oceanogr. 62, 2431–2444. doi: 10.1002/lno.10577
Henriques-Silva, R., Logez, M., Reynaud, N., Tedesco, P. A., Brosse, S., Januchowski-Hartley, S. R., et al. (2019). A comprehensive examination of the network position hypothesis across multiple river metacommunities. Ecography 42, 284–294. doi: 10.1111/ecog.03908
Hu, D., Jiang, L., Hou, Z., Zhang, J., Wang, H., and Lv, G. (2022). Environmental filtration and dispersal limitation explain different aspects of beta diversity in desert plant communities. Global Ecol Conserv 33:e01956. doi: 10.1016/j.gecco.2021.e01956
Jiang, Y.-J., He, W., Liu, W.-X., Qin, N., Ouyang, H.-L., Wang, Q.-M., et al. (2014). The seasonal and spatial variations of phytoplankton community and their correlation with environmental factors in a large eutrophic Chinese lake (Lake Chaohu). Ecol. Indic. 40, 58–67. doi: 10.1016/j.ecolind.2014.01.006
Jiang, X., Pan, B., Jiang, W., Hou, Y., Yang, H., Zhu, P., et al. (2021). The role of environmental conditions, climatic factors and spatial processes in driving multiple facets of stream macroinvertebrate beta diversity in a climatically heterogeneous mountain region. Ecol. Indic. 124:107407. doi: 10.1016/j.ecolind.2021.107407
Kadmon, R. (1995). Nested species subsets and geographic isolation: a case study. Ecology 76, 458–465. doi: 10.2307/1941204
Kaishan, S., Chunyang, T., Yongshan, W., and Jinhong, W. (2014). Study on land use and landscape shifting in Huntai River basin from 2000 to 2010. For Resourc Manage 98–103. doi: 10.13466/j.cnki.lyzygl.2014.01.019
Kong, W., Meng, W., Zhang, Y., Gippel, C., and Qu, X. (2013). A freshwater ecoregion delineation approach based on freshwater macroinvertebrate community features and spatial environmental data in Taizi River basin, northeastern China. Ecol. Res. 28, 581–592. doi: 10.1007/s11284-013-1048-7
Legendre, P., and Anderson, M. J. (1999). Distance-Based Redundancy Analysis: Testing Multispecies Responses In Multifactorial Ecological Experiments. Ecol. Monogr. 69, 1–24. doi: 10.1890/0012-9615(1999)069[0001:DBRATM]2.0.CO;2
Leibold, M. A., Holyoak, M., Mouquet, N., Amarasekare, P., Chase, J. M., Hoopes, M. F., et al. (2004). The metacommunity concept: a framework for multi-scale community ecology. Ecol. Lett. 7, 601–613. doi: 10.1111/j.1461-0248.2004.00608.x
Leibold, M. A., and Mikkelson, G. M. (2002). Coherence, species turnover, and boundary clumping: elements of meta-community structure. Oikos 97, 237–250. doi: 10.1034/j.1600-0706.2002.970210.x
Lentendu, G., Mahé, F., Bass, D., Rueckert, S., Stoeck, T., and Dunthorn, M. (2018). Consistent patterns of high alpha and low beta diversity in tropical parasitic and free-living protists. Mol. Ecol. 27, 2846–2857. doi: 10.1111/mec.14731
Levesque-Beaudin, V., and Wheeler, T. A. (2011). Spatial scale and nested patterns of beta-diversity in temperate forest Diptera. Insect Conserv Diversity 4, 284–296. doi: 10.1111/j.1752-4598.2010.00127.x
Li, Z., Chen, X., Jiang, X., Tonkin, J. D., Xie, Z., and Heino, J. (2021). Distance decay of benthic macroinvertebrate communities in a mountain river network: do dispersal routes and dispersal ability matter? Sci. Total Environ. 758:143630. doi: 10.1016/j.scitotenv.2020.143630
Liu, Y., Zhang, W., Wang, Y., and Wang, E., (1979). Freshwater molluscs of Chinese economic fauna. Science Press, Beijing.
Liu, Z., Zhou, T., Heino, J., Castro, D. M. P., Cui, Y., Li, Z., et al. (2022). Land conversion induced by urbanization leads to taxonomic and functional homogenization of a river macroinvertebrate metacommunity. Sci. Total Environ. 825:153940. doi: 10.1016/j.scitotenv.2022.153940
McGill, B. J., Enquist, B. J., Weiher, E., and Westoby, M. (2006). Rebuilding community ecology from functional traits. Trends Ecol. Evol. 21, 178–185. doi: 10.1016/j.tree.2006.02.002
Merritt, R. W., and Cummins, K. W. (1996). An introduction to the aquatic insects of North America. Univ. of California, Berkeley: Kendall/Hunt Publishing Company.
Morlon, H., Chuyong, G., Condit, R., Hubbell, S., Kenfack, D., Thomas, D., et al. (2008). A general framework for the distance–decay of similarity in ecological communities. Ecol. Lett. 11, 904–917. doi: 10.1111/j.1461-0248.2008.01202.x
Morse, J. C., Yang, L., and Tian, L. (1994). Aquatic insects of China useful for monitoring water quality. Nanjing: Hohai University Press.
Mykrä, H., Heino, J., and Muotka, T. (2007). Scale-related patterns in the spatial and environmental components of stream macroinvertebrate assemblage variation. Glob. Ecol. Biogeogr. 16, 149–159. doi: 10.1111/j.1466-8238.2006.00272.x
Nguyen, T. H. T., Forio, M. A. E., Boets, P., Lock, K., Damanik Ambarita, M. N., Suhareva, N., et al. (2018). Threshold responses of macroinvertebrate communities to stream velocity in relation to hydropower dam: a case study from the Guayas River basin (Ecuador). Water 10:1195. doi: 10.3390/w10091195
Ning, J., and Beiko, R. G. (2015). Phylogenetic approaches to microbial community classification. Microbiome 3:47. doi: 10.1186/s40168-015-0114-5
Nunes, C. A., Braga, R. F., Figueira, J. E. C., Neves, F. D. S., and Fernandes, G. W. (2016). Dung beetles along a tropical altitudinal gradient: environmental filtering on taxonomic and functional diversity. PLoS One 11:e0157442. doi: 10.1371/journal.pone.0157442
Oksanen, J., Blanchet, F. G., Kindt, R., Legendre, P., Minchin, P. R., O’Hara, R., et al. (2013). Package ‘vegan’. Community ecology package, version 2, 1–295.
Peres-Neto, P. R., and Legendre, P. (2010). Estimating and controlling for spatial structure in the study of ecological communities. Glob. Ecol. Biogeogr. 19, 174–184. doi: 10.1111/j.1466-8238.2009.00506.x
Perez Rocha, M., Bini, L. M., Domisch, S., Tolonen, K. T., Jyrkänkallio-Mikkola, J., Soininen, J., et al. (2018). Local environment and space drive multiple facets of stream macroinvertebrate beta diversity. J. Biogeogr. 45, 2744–2754. doi: 10.1111/jbi.13457
Petsch, D. K., Saito, V. S., Landeiro, V. L., Silva, T. S. F., Bini, L. M., Heino, J., et al. (2021). Beta diversity of stream insects differs between boreal and subtropical regions, but land use does not generally cause biotic homogenization. Freshwater Sci 40, 53–64. doi: 10.1086/712565
Pisner, D. A., and Schnyer, D. M. (2020). “Chapter 6-support vector machine” in Machine learning. eds. A. Mechelli and S. Vieira (Austin, TX, United States: Academic Press), 101–121.
Rusanov, A. G., Bíró, T., Kiss, K. T., Buczkó, K., Grigorszky, I., Hidas, A., et al. (2022). Relative importance of climate and spatial processes in shaping species composition, functional structure and beta diversity of phytoplankton in a large river. Sci. Total Environ. 807:150891. doi: 10.1016/j.scitotenv.2021.150891
Sanders, N. J., and Rahbek, C. (2012). The patterns and causes of elevational diversity gradients. Ecography 35, 1–3. doi: 10.1111/j.1600-0587.2011.07338.x
Schmera, D., Árva, D., Boda, P., Bódis, E., Bolgovics, Á., Borics, G., et al. (2018). Does isolation influence the relative role of environmental and dispersal-related processes in stream networks? An empirical test of the network position hypothesis using multiple taxa. Freshw. Biol. 63, 74–85. doi: 10.1111/fwb.12973
Schneider, B., Cunha, E. R., Espínola, L. A., Marchese, M., and Thomaz, S. M. (2019). The importance of local environmental, hydrogeomorphological and spatial variables for beta diversity of macrophyte assemblages in a Neotropical floodplain. J. Veg. Sci. 30, 269–280. doi: 10.1111/jvs.12707
Shi, Y., Grogan, P., Sun, H., Xiong, J., Yang, Y., Zhou, J., et al. (2015). Multi-scale variability analysis reveals the importance of spatial distance in shaping Arctic soil microbial functional communities. Soil Biol. Biochem. 86, 126–134. doi: 10.1016/j.soilbio.2015.03.028
Soininen, J., Heino, J., and Wang, J. (2018). A meta-analysis of nestedness and turnover components of beta diversity across organisms and ecosystems. Glob. Ecol. Biogeogr. 27, 96–109. doi: 10.1111/geb.12660
Team RC, (2022). R: A language and environment for statistical computing. R Foundation for Statistical Computing, Vienna, Austria.
Tomanova, S., and Usseglio-Polatera, P. (2007). Patterns of benthic community traits in neotropical streams: relationship to mesoscale spatial variability. Fundamental Appl Limnol Archiv fur Hydrobiologie 170, 243–255. doi: 10.1127/1863-9135/2007/0170-0243
Tonkin, J. D., Altermatt, F., Finn, D. S., Heino, J., Olden, J. D., Pauls, S. U., et al. (2018a). The role of dispersal in river network metacommunities: patterns, processes, and pathways. Freshw. Biol. 63, 141–163. doi: 10.1111/fwb.13037
Tonkin, J. D., Heino, J., and Altermatt, F. (2018b). Metacommunities in river networks: the importance of network structure and connectivity on patterns and processes. Freshw. Biol. 63, 1–5. doi: 10.1111/fwb.13045
Tonkin, J. D., Stoll, S., Jähnig, S. C., and Haase, P. (2016). Contrasting metacommunity structure and beta diversity in an aquatic-floodplain system. Oikos 125, 686–697. doi: 10.1111/oik.02717
Usseglio-Polatera, P., Bournaud, M., Richoux, P., and Tachet, H. (2000). Biological and ecological traits of benthic freshwater macroinvertebrates: relationships and definition of groups with similar traits. Freshw. Biol. 43, 175–205. doi: 10.1046/j.1365-2427.2000.00535.x
Viana, D. S., Figuerola, J., Schwenk, K., Manca, M., Hobæk, A., Mjelde, M., et al. (2016). Assembly mechanisms determining high species turnover in aquatic communities over regional and continental scales. Ecography 39, 281–288. doi: 10.1111/ecog.01231
Villéger, S., Mason, N. W. H., and Mouillot, D. (2008). New multidimensional functional diversity indices for a multifaceted framework in functional ecology. Ecology 89, 2290–2301. doi: 10.1890/07-1206.1
Wang, J. (2003). Tolerance values of benthic macroinvertebrates and bioassessment of water quality in the Lushan nature reserve. Chin. J. App. Environ. Biol. 9, 279–284. doi: 10.3321/j.issn:1006-687X.2003.03.015
Wang, J., Ding, C., Tao, J., Jiang, X., Heino, J., Ding, L., et al. (2021). Damming affects riverine macroinvertebrate metacommunity dynamics: insights from taxonomic and functional beta diversity. Sci. Total Environ. 763:142945. doi: 10.1016/j.scitotenv.2020.142945
Wang, Y., Lu, G., Yu, H., Du, X., He, Q., Yao, S., et al. (2021). Meadow degradation increases spatial turnover rates of the fungal community through both niche selection and dispersal limitation. Sci. Total Environ. 798:149362. doi: 10.1016/j.scitotenv.2021.149362
Wei, F., Kou, H., and Hong, S., (1989). Methods for the examination of water and wastewater. China Environmental Science Press, Beijing (in Chinese).
Willig, M. R., Kaufman, D. M., and Stevens, R. D. (2003). Latitudinal gradients of biodiversity: pattern, process, scale, and synthesis. Annu. Rev. Ecol. Evol. Syst. 34, 273–309. doi: 10.1146/annurev.ecolsys.34.012103.144032
Winter, M., Devictor, V., and Schweiger, O. (2013). Phylogenetic diversity and nature conservation: where are we? Trends Ecol. Evol. 28, 199–204. doi: 10.1016/j.tree.2012.10.015
Wu, N., Wang, Y., Wang, Y., Sun, X., Faber, C., and Fohrer, N. (2022). Environment regimes play an important role in structuring trait- and taxonomy-based temporal beta diversity of riverine diatoms. J. Ecol. 110, 1442–1454. doi: 10.1111/1365-2745.13859
Yang, J., Swenson, N. G., Zhang, G., Ci, X., Cao, M., Sha, L., et al. (2015). Local-scale partitioning of functional and phylogenetic Beta diversity in a tropical tree assemblage. Sci. Rep. 5:12731. doi: 10.1038/srep12731
Zhang, Y., Ding, S., Bentsen, C. N., Ma, S., Jia, X., and Meng, W. (2015). Differences in stream fish assemblages subjected to different levels of anthropogenic pressure in the Taizi River catchment, China. Ichthyol. Res. 62, 450–462. doi: 10.1007/s10228-015-0459-6
Zhang, Y., Wang, X.-N., Ding, H.-Y., Dai, Y., Ding, S., and Gao, X. (2019). Threshold responses in the taxonomic and functional structure of fish assemblages to land use and water quality: a case study from the Taizi River. Water 11:661. doi: 10.3390/w11040661
Zhao, K., Song, K., Pan, Y., Wang, L., Da, L., and Wang, Q. (2017). Metacommunity structure of zooplankton in river networks: roles of environmental and spatial factors. Ecol. Indic. 73, 96–104. doi: 10.1016/j.ecolind.2016.07.026
Appendix
Table 1: Effects of sampling year, network location, and their interaction on environmental conditions. Network location × sampling year represents the interaction between network location and sampling year.
Keywords: metacommunity, network position, river, beta diversity, ecological drivers
Citation: Zheng B and Yin X (2023) Assembly mechanism of macroinvertebrate metacommunities and ecological factors of multiple aspects of beta diversity in a boreal river basin, China. Front. Ecol. Evol. 11:1131403. doi: 10.3389/fevo.2023.1131403
Edited by:
Francois Munoz, Université Grenoble Alpes, FranceReviewed by:
Julie Crabot, Délégation Rhône Auvergne (CNRS), FranceJinlong Zhang, Kadoorie Farm and Botanic Garden, Hong Kong SAR, China
Copyright © 2023 Zheng and Yin. This is an open-access article distributed under the terms of the Creative Commons Attribution License (CC BY). The use, distribution or reproduction in other forums is permitted, provided the original author(s) and the copyright owner(s) are credited and that the original publication in this journal is cited, in accordance with accepted academic practice. No use, distribution or reproduction is permitted which does not comply with these terms.
*Correspondence: Xuwang Yin, eWlueHV3YW5nQGRsb3UuZWR1LmNu