- Department of Life, Health & Environmental Sciences, University of L’Aquila, L’Aquila, Italy
Introduction: The European large carnivore community is recently showing positive trends. Beyond those, other species are expanding in Europe. In recent decades, the golden jackal (Canis aureus), a medium-sized canid, has rapidly expanded to western and northern Europe, after being historically confined to the Balkans. The Eurasian lynx (Lynx lynx), an apex predator once widely distributed throughout Europe, was nearly eradicated in the 19th century. Recent reintroductions, legal protection, and its natural expansion brought the lynx to recover, though some populations still face extinction. As the carnivores’ community across Europe is rearranging, ecological interactions are changing as well. Indeed, recent observations reported the golden jackal to kleptoparasitize the lynx. Our research aims to predict the potentially suitable habitats for the golden jackal and the Eurasian lynx, detailing the areas where those are expected to coexist soon.
Methods: We model their distribution in Europe for current and future scenarios, using both citizen-science and literature data, using the latter to buffer the possible biases of the former. For this aim, we included not only climatic variables, but also several biotic and abiotic predictors relevant to both species. Moreover, given his strong influence on the golden jackal, we compare our results with the predicted distribution of the grey wolf (Canis lupus).
Results: Our predictions show an increase in habitat suitability for the golden jackal, while an opposite trend is predicted for the Eurasian lynx. Also, we predict the target species to share a large portion of their range in the future, with most of these areas suitable for wolves, too.
Discussion: Our results may have several impacts in the context of large-scale management, helping to predict further expansion of the golden jackal, and to identify critical areas for lynx conservation, supporting the management of possible reintroductions, also shedding light on the large carnivores’ changing community
1 Introduction
Despite the increasingly severe effects of climate change, land use alterations, and habitat degradation, biodiversity is not spatially declining evenly: there are several cases of species that expanded their ranges (Nolet and Rosell, 1998; VerCauteren, 2003; Arnold et al., 2012; Deinet et al., 2013; Chapron et al., 2014; Halliez et al., 2015; Carvalho et al., 2018; Pacifici et al., 2020; Wehr, 2021) or managed to survive by adapting to new environmental conditions, which also involve the human presence (Basille et al., 2009; Chapron et al., 2014; Bouyer et al., 2015; Ražen et al., 2016; Fenton et al., 2021). Of course, these processes did not occur alone, but together with successful conservation actions which allowed many species to recover or survive (Hoffmann et al., 2010; Deinet et al., 2013).
This is the case of Europe, where the Living Planet Index, reports a slow decline rate linked both to conservation successes (Hoffmann et al., 2010; Chapron et al., 2014) and to the natural adaptability and recolonization capacity of some species (Ledger et al., 2022). Also, a meta-analysis noted increases in species richness and abundance in many bioregions of Northern and Eastern Europe (Pilotto et al., 2020).
Although Europe is one of the least wild continents, considering the human footprint which led (and continues to lead) to the loss of ecosystems (Williams et al., 2020) and the declining trend of some species (Inger et al., 2015; Warren et al., 2021), many species appear to be able to adapt, or in general, to persist. We are witnessing the comeback of various species of mammals, including the European bison (Bison bonasus), the elk (Alces alces), the Eurasian beaver (Castor fiber), the southern chamois (Rupicapra pyrenaica), and the alpine ibex (Capra ibex) (Deinet et al., 2013; Ledger et al., 2022). Also, the main European predators, such as the brown bear (Ursus arctos), the grey wolf (Canis lupus), and Eurasian lynx (Lynx lynx) are expanding their ranges (Chapron et al., 2014).
From a conservation perspective, the recovery of these species and their range expansion is particularly relevant, as predators require large spaces to survive and can easily come into conflict with local communities. It should not be forgotten that their return is linked to a series of factors such as an increase in forest cover, a decrease in human population densities, protective legislation, acceptance by public opinion, and the use of measures that allow coexistence with humans (Chapron et al., 2014; Cimatti et al., 2021). Preserving this delicate equilibrium between apex predators and human activities is thus important since not far as a hundred years ago these have been hunted and persecuted, and their decrease has facilitated the expansion of mesopredators (Prugh et al., 2009; Ripple et al., 2013; Newsome et al., 2017).
In Europe, the golden jackal (Canis aureus), represents an excellent example of this phenomenon; it is currently an expanding species, whose growth was initially favored by the absence of the wolf (Krofel et al., 2017). The European distribution of this medium-sized canid species was once limited to the Balkan area (Kryštufek et al., 1997), and saw a decline until 1950 caused by human persecution (Spassov, 1989) which reduced it to a few populations among Former Yugoslavia, Bulgaria, Turkey, and Greece (Spassov, 1989; Giannatos, 2004; Spassov, 2007). Then, two waves of expansion, the first occurred around the 1950s, and the second from the 1980s, also favored by the legal protection (in Bulgaria from 1962) led to a north- and westward expansion (Trouwborst et al., 2015; Krofel et al., 2017); in the following years, the jackal colonized most of the countries of central and southern Europe. More recently, a small population has established in the Baltic countries (Pyšková et al., 2016). Dispersing individuals have also been observed in northern Europe (Sørensen and Lindsø, 2021; Rykov et al., 2022), and central Europe (Hatlauf et al., 2016; Böcker et al., 2023), and its presence has been reported in 33 European countries, 10 of them in the last decade (Hatlauf et al., 2021).
The golden jackal is a generalist species with opportunistic feeding habits, with its prey spanning from ungulates to rodents (the most common prey), also comprising invertebrates, vegetables, and anthropogenic resources, including waste and carcasses (Markov and Lanszki, 2012; Aleksandra and Duško, 2015; Ćirović et al., 2016; Lange et al., 2021). The ecological plasticity of the golden jackal also emerges in the choice of habitat: the most used one is a mixture of shrub and herbaceous vegetation, including mesophilic woods or riparian habitats (Lapini et al., 2011; Šálek et al., 2014; Spassov and Acosta-Pankov, 2019), but sometimes it may also be attracted by human settlements (Lapini et al., 2011). However, the habitat choice of the golden jackal is highly influenced by the wolf presence (Krofel et al., 2017), and climatic-based models predict its change for the next future (Cunze & Klimpel, 2022).
The changing distribution of a species, such as in the case of the golden jackal which is currently expanding its range, can lead to new biotic communities, and new interactions with other species that find themselves coexisting for the first time (Gilman et al., 2010; Pecl et al., 2017). In the case of the golden jackal, it is exceptional the recent observation of kleptoparasitism towards the Eurasian lynx (Krofel et al., 2022), another predator expanding in Europe.
The Eurasian lynx is a European top predator that was intentionally eradicated from many parts of Europe between the 19th century and the beginning of the 20th century (Breitenmoser, 1998). Only four populations survived, in Scandinavia, the Carpathians, the Baltic area, and in the southwestern Balkans (von Arx et al., 2004). The species recolonized parts of its former range thanks to several factors, from legal protection and reintroductions to an increase in prey availability and greater public acceptance (Linnell et al., 2009), and is now slowly recovering (9–10,000 individuals estimated in Europe) (Chapron et al., 2014). This species is a predator specialized on roe deer (Capreolus capreolus) (Jędrzejewski et al., 1993), but the diet varies regionally and may include other species, from hare (Lepus europaeus) and chamois (Rupicapra spp.) to red deer (Cervus elaphus), also including livestock (Okarma et al., 1997; Stahl et al., 2001; Odden et al., 2006). The Eurasian lynx is associated with forest habitats with good environmental heterogeneity for hunting (Belotti et al., 2013) and many hiding places to rest (Podgórski et al., 2008). However, the species can select also more open habitats, especially at night (Filla et al., 2017) adapting to them by generations (Nagl et al., 2022). Also, it can adapt to habitats with moderate human activity if prey abundance is elevated and refuges are available, seeking a compromise between human avoidance and prey hunting (Basille et al., 2009; Bouyer et al., 2015; Oeser et al., 2023). The overlap between the golden jackal and Eurasian lynx ranges was scanty until the mid-20th. Indeed, the recent expansion of the jackal, especially since 2011, caused an increase in this overlap, currently estimated at 13% (Krofel et al., 2022). Considering the expansion of the golden jackal and the recovery of the Eurasian lynx, this overlap is likely to increase, even though no information is available to date. Moreover, considering the positive trends of the wolf populations, and their influence on the golden jackal (Krofel et al., 2017; Newsome et al., 2017), it could be also important to assess whether the range changes of both golden jackal and Eurasian lynx would increase in areas where the wolves are well-established, or where is predicted an expansion in the future.
To lay the foundation for the study of this ecological issue, we infer the areas of potential current and future overlap between the two target species, as they may indicate the zones where the interactions are more likely to occur. To achieve this result, we take advantage of the most up-to-date Ecological Niche Modeling techniques and post-modeling GIS spatial analyses. The use of Ecological Niche Models for the management of expanding species and the understanding of their dynamics has increased in recent years (De Marco et al., 2008; Jiménez-Valverde et al., 2011; Maiorano et al., 2011; Barbet-Massin et al., 2012a; Barbet-Massin et al., 2012b; Dawe and Boutin, 2016; Iannella et al., 2019; Rodríguez-Rey et al., 2019; Serva et al., 2023). Also, Ecological Niche Models can be refined through a post-modeling phase, converting them into more precise Species Distribution Models (SDMs), in a process which increases the predictive performances and allows the creation of more realistic models (Iannella et al., 2021).
Given the ongoing range changes observed for both the Eurasian lynx and the golden jackal, and the recently observed interactions between them, we build SDMs for these two species under current and future conditions. Then, we use those SDMs to analyze their possible range overlap under both current and future scenarios, using for these latter the areas predicted to remain stable and the ones predicted to be gained. We finally use these zones to highlight the areas where possible interaction may occur. Moreover, given the great influence of the wolf on the golden jackal, we build environmental niche models (ENMs) to estimate the current and the future predicted suitable areas for the wolf, to understand if the predicted overlap areas of the Eurasian lynx and golden jackal would overlay with this other large European carnivore.
2 Materials and methods
2.1 Target species and study area
The target species are represented by the Eurasian lynx, a top predator, and the golden jackal, a mesopredator. Secondly, we focused on the grey wolf, another European apex predator.
The Eurasian lynx is classified as Least Concern by the IUCN and is included both in the Bern Convention (Appendix III except for the Balkan lynx balcanicus, listed in Appendix II) and in the EU Habitats Directive (Annexes II and IV, except for some countries where it is included in Annex V and excluded from Annex II). The golden jackal is also classified as Least Concern by the IUCN, and it is included in Annex V of the Habitats Directive. However, the protection of the golden jackal in Europe has been under discussion, since some countries reported it as an alien species, and the Habitats Directive imposes distinct limitations on national policies and national management options (Trouwborst et al., 2015). Indeed, currently, both populations of the target species are increasing (Arnold et al., 2012; Deinet et al., 2013; Chapron et al., 2014; Spassov and Acosta-Pankov, 2019). Similarly, the grey wolf is increasing in Europe, and is classified as Least Concern by the IUCN. Like the Eurasian lynx, the grey wolf is protected both by the Bern Convention (Appendix II) and the EU Habitats Directive (Annex II and IV), even if there are some local derogations.
The area selected for our study is the western Palearctic, a region where the golden jackal is expanding and co-occurring with the lynx.
2.2 Environmental predictors
To encompass as much as possible the ecological needs and the factors influencing the two target species’ distribution, we used climatic, topographic, habitat, hydrographic, human disturbance and biotic predictors, each of which is described below.
The climatic aspect was assessed through the 19 bioclimatic variables available on WorldClim (version 2.1, 2.5 arc-min, Supplementary Document 1) (Fick and Hijmans, 2017) downloaded for current and future (2050 and 2070) conditions. For each future scenario, we selected the shared socio-economic pathways (SSP) 2.45, 3.70, and 5.85 to involve all the different possible trajectories except the most optimistic one (SSP 1.26) (Riahi et al., 2017). Since the use of a single General Circulation Model (GCM) in future projections is shown to produce variable predictions (Stralberg et al., 2015), we selected and managed three different GCMs, namely the BCC-CSM2-MR (Wu et al., 2019), the IPSL-CM6A-LR (Boucher et al., 2020) and the MIROC6 (Tatebe et al., 2019) for all future models’ forecasts.
We collected elevation data from the ASTER NASA database (https://asterweb.jpl.nasa.gov/gdem.asp), deriving the slope raster map at the same spatial resolution (2.5 arc-min) (‘Slope’ function, Spatial Analyst in ArcGIS Pro), because the slope is considered as a relevant variable for the ecology of both the Eurasian lynx (acting as a proxy for resting sites) and the golden jackal (Filla et al., 2017; Spassov and Acosta-Pankov, 2019; Hočevar et al., 2021).
About the habitat predictors, we took advantage of the 100-m resolution Global Corine Land Cover map of the Copernicus repository (https://land.copernicus.eu/global/products/lc), containing a discrete classification with 23 classes according to the UN-FAO Land Cover Classification System (Buchhorn et al., 2020).
To incorporate the human influence over the two target species, we considered the influence of roads, since they represent a major limit to the dispersal of lynx and jackal, and are capable to alter movement behavior, increase direct mortality and lower patches’ connectivity (Kramer-Schadt et al., 2004; Lapini et al., 2011; Heurich et al., 2018). For these reasons, we downloaded the roads data from the “World Roads” Layer Package managed by ESRI (ESRI Inc, 2022) and divided them into major roads and highways. To use this information as a modeling predictor, we calculated the distances from those, classifying them into four intervals (0–500 m, 500–1,000 m, 1,000–5,000 m, 5,000–20,000 m). To further account for human disturbance, especially important for the Eurasian lynx (Ripari et al., 2022), we also downloaded the GHSL SMOD data (Global Human Settlement Layer, available at https://ghsl.jrc.ec.europa.eu/) at ~1 km resolution: this map takes into account both population densities and built-up area densities, in a unique spatial data (Pesaresi et al., 2019).
Also, we used three other predictors for golden jackal modeling only: the average snow cover map (since snow cover was used in previous studies and could partially limit its suitable habitat) (Ranc et al., 2015; Spassov and Acosta-Pankov, 2019; Männil and Ranc, 2022), the river network (as riverine habitats are known to be important dispersal corridors; Arnold et al., 2012; Spassov and Acosta-Pankov, 2019) and the grey wolf current range (since this species is known to be a major limiting factor for the jackal, especially through intraguild predation; Krofel et al., 2017; Ranc et al., 2018; Spassov and Acosta-Pankov, 2019).
Specifically, for the snow cover information we used the MODIS data (Terra Snow Cover Monthly L3 Global, ~5 km resolution, available at https://nsidc.org/data/MOD10CM; Hall and Riggs, 2021) averaging each month from 2010 to 2020.
The rivers’ network information were first obtained from the HydroRIVERS dataset (https://www.hydrosheds.org/products/hydrorivers; Lehner and Grill, 2013) and then processed through the ‘Euclidean distance’ tool in ArcGIS Pro 10.3 (ESRI Inc, 2022), to obtain distance intervals form each watercourse, with the following intervals: 0–1 km, 1–2 km, 2–3 km, 3–10 km, > 10 km. Finally, the grey wolf current range was obtained at a resolution of 10 km from the work of Kaczensky et al. (2021), giving a different weight to the cells in which the presence of the wolf is permanent, compared to the cells in which the presence of the wolf is sporadic.
2.3 Ecological niche modeling
The occurrence datasets were obtained from the GBIF repositories for both golden jackal (GBIF, 2022a) and Eurasian lynx (GBIF, 2022b). Two filters were applied, namely a temporal (selecting the occurrences 1970–current only) and a spatial (coordinate uncertainty < 5 km) one. Moreover, we added occurrence data from recent reports in literature of both the golden jackal and the Eurasian lynx (Supplementary Tables), comparing the final datasets with the known distributions of the species. For the purposes of the modeling process, we processed the GBIF-filtered records so as to obtain a comparable spatial resolution between the occurrences and environmental predictors (Sillero and Barbosa, 2021), moreover reducing the spatial autocorrelation. In order to do that, we used the ‘Spatial rarefy’ tool of SDMtoolbox (Brown et al., 2017).
To better explore the potential expansion of the golden jackal in the future, we decided to keep the records from dispersing individuals (~19% of the total occurrences), given that these individuals are likely to form stable population, considering his ongoing expansion pattern. The records belonging to dispersing individuals can be noted in Supplementary Figure 1, together with the core areas for each population. However, to assess possible biases caused by the inclusion of dispersing individuals in the species distribution models or related to the nature of the dataset (mainly composed of citizen-science-derived data), we repeated the analysis (building ENMs) using only records from the dataset of Ranc et al. (2022) selecting only the cells where golden jackal is reported as “permanent” or “high confidence presence” (expert-based ENMs).
To model the grey wolf predicted distribution for the current and future projections, we relied on to the dataset of Kaczensky et al. (2021), considering only the cells where the species is reported as “permanent”. Then, we assessed the multicollinearity of the 19 bioclimatic variables by measuring the Variance Inflation Factor (‘vifstep’ algorithm of the ‘usdm’ R package (Naimi, 2015), threshold = 10); we included the ones both not exceeding this threshold and being important for the species’ ecology (Brandt et al., 2017). To minimize the variation caused by the use of individual General Circulation Models (GCMs) in future projections (Stralberg et al., 2015), we used during the modeling process the three different GCMs, namely the BCC-CSM2-MR (Wu et al., 2019), the IPSL-CM6A-LR (Boucher et al., 2020) and the MIROC6 (Tatebe et al., 2019), and the three SSPs mentioned before for future projections (2.45, 3.70, and 5.85).
To build the ENMs we used the ‘biomod2’ package (Thuiller et al., 2016) in R (R Core Team, 2016). The advantage of this package is that allows to create ensemble models, which are the result of the combination of individual algorithms’ outcomes, corresponding to different modeling techniques. We generated five sets of 1,000 pseudo-absences each (for the wolf, five sets of 10,000 pseudo-absences) using the Surface Range Envelope algorithm (quantile = 0.05) (Barbet-Massin et al., 2012a). We parametrized the single models through the ‘BIOMOD_ModelingOptions’ as follows: GLM: type = “quadratic”, interaction.level = 3; GBM: n.trees = 10,000, interaction.depth = 3, cv.folds = 10; GAM: type=“s_smoother”, interaction.level=3; RF: n.trees=10,000, bag.fraction = 0.75, shrinkage = 0.001. Then, the ‘BIOMOD_Modeling’ function was used to calibrate the single models, and the ‘BIOMOD_EnsembleModeling’ algorithm was finally run to obtain the Ensemble models, using as input only single models with high performances (AUC >0.8 and TSS >0.7). To merge the single models, we use the ‘wmean’ algorithm, that averages models into the Ensemble one based on a weighted mean function, in which weights are the discrimination scores (Thuiller et al., 2016). Finally, we projected to future scenarios with the ‘BIOMOD_EnsembleForecasting’ function.
For each projection of the same future scenario (i.e., keeping as fixed the year/SSP combination, for instance the 2050 SSP 3.70), we reduced the uncertainties deriving from the calibration performed on each GCM as follows. We first checked for model extrapolation (i.e., the dissimilarity from the calibration conditions) by assessing the Multivariate Environmental Surface Similarity (MESS) (Elith et al., 2010), computed through the function ‘mess’ of the ‘dismo’ package (Hijmans et al., 2016). The MESS maps so obtained were then included in the Multivariate Environmental Dissimilarity Index (MEDI) algorithm (Iannella et al., 2017), a form of weighted raster average which down-weights extrapolation based on projections and MESS, thus reducing the uncertainties in future projections. We finally used those MEDI-corrected future projections as baseline models for all the following analyses.
2.4 Weighted overlay process and post-modeling analyses
To obtain a more informative and robust models, we applied the ‘Weighted overlay’ framework of Iannella et al. (2021) to both target species. This procedure permits to refine Ecological Niche Models into more precise Species Distribution Models, incorporating, in a post-modeling GIS environment, the information not included during the Ecological Niche Modeling process, also increasing predictive performances (Iannella et al., 2021). Thus, we included in this process all the predictors mentioned above except the climatic ones, which were included in the ENM calibration and projection. The information about slope, habitat, population density, snow cover, and wolf range was extracted in occurrence localities for both species through the ‘Extract multi values to points’ tool in ArcGIS. Then, relative frequencies were calculated for each predictor/occurrence localities and converted into absolute frequencies, on a 1-to-10 scale, to make them comparable with the ENMs. The only exception we made is the conversion into the 1-to-10 scale of the roads and rivers distance categories, deduced from literature (roads information for Eurasian lynx model referring to Dobbert et al. (2021); rivers data for the golden jackal modeling referring to Spassov and Acosta-Pankov (2019) and thus not obtained through the occurrences’ frequencies).
Specifically, we used as input into the weighted process on current conditions the ENMs, habitat, population/built areas density, slope, distance from roads and rivers, snow cover, and wolf range predictors to model the distribution of the golden jackal; about the Eurasian lynx, we used the ENMs, habitat, population/built areas density, slope, and distance from roads ones.
For the projections to future scenarios, we kept all the predictors as fixed, except the ENMs; when needed, weighted models were binarized using as a threshold the 10th percentile value (Iannella et al., 2021). Subsequently, the ‘BIOMOD_RangeSize’ function of the ‘biomod2’ package (Thuiller et al., 2016) was applied to calculate the areas predicted to be lost, remaining stable, or gained from the two target species in the future scenarios. Considering the presence-only nature of the data, we assess the predictive performance of the weighted models (as well as for the ENMs presented above) through the Boyce index (Hirzel et al., 2006; Leroy et al., 2018).
To evaluate areas where sympatry between the two species may occur, we assessed the overlap of their respective “gain + stable” areas for both the present and the future (considering all the SSPs for this latter case).
Wolf ENMs were binarized through the 10th percentile; we overlap the predicted suitable areas with the zones where future sympatry between golden jackal and Eurasian lynx is predicted to occur.
3 Results
3.1 Ecological Niche Models
After the presence localities filtering processes, we obtained 2,164 occurrences for the Eurasian lynx, and 564 for the golden jackal within the study area (Figures 1A, B). These datasets are roughly comparable to current distributions of the target species, even if they show some gaps, especially for the golden jackal in the core distribution areas.
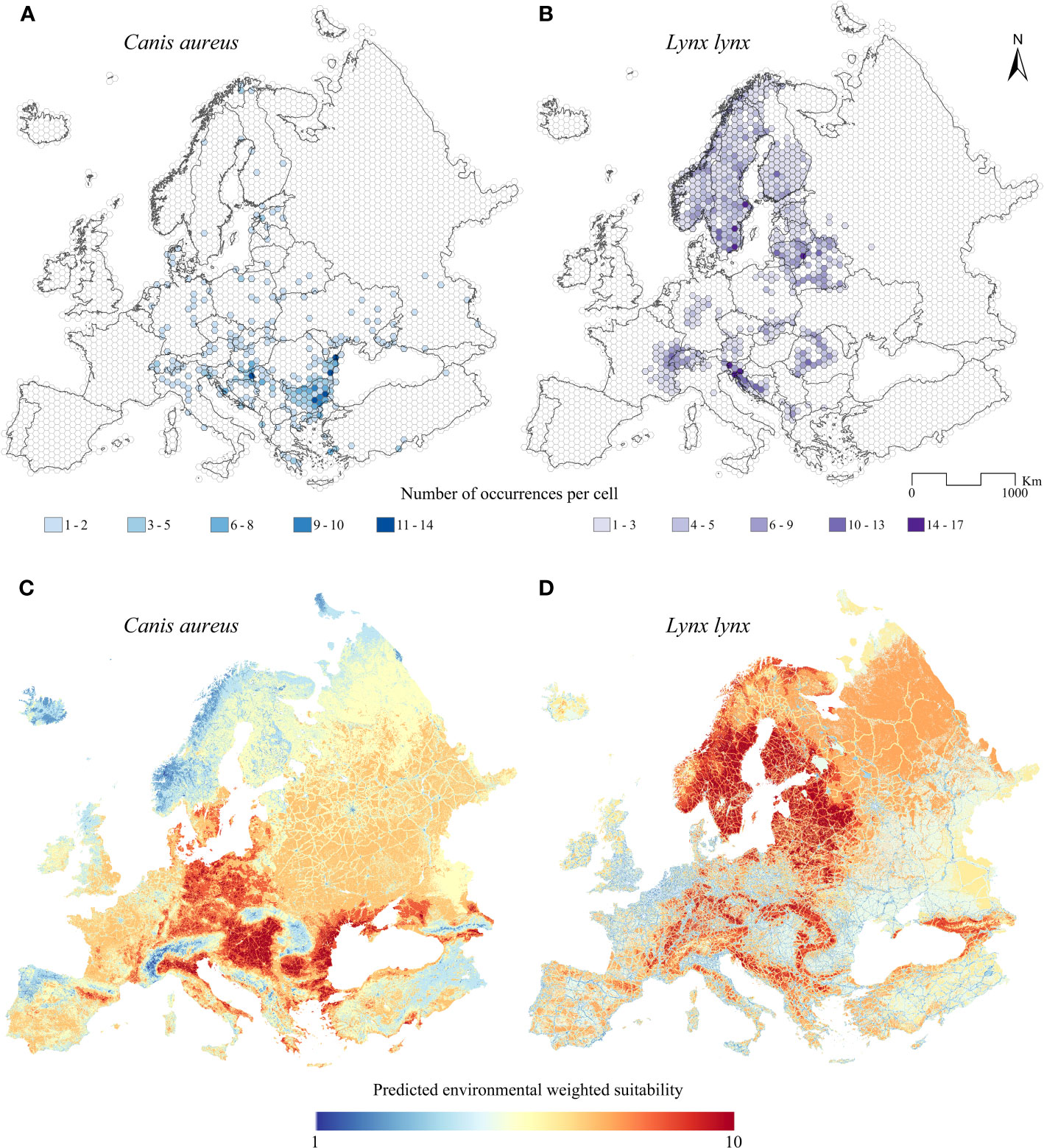
Figure 1 Current and predicted distribution. Current European distribution of (A) the golden jackal (Canis aureus) and (B) the Eurasian lynx (Lynx lynx). Predicted species distribution model for (C) the golden jackal and (D) the Eurasian lynx, ranging from low (blue) to high (red) suitability.
Considering the results of the VIF analysis and the ecological characteristics of the species, we kept ten bioclimatic variables for the modeling of the golden jackal: BIO1 (Annual Mean Temperature), BIO2 (Annual Mean Diurnal Range), BIO4 (Temperature Seasonality), BIO6 (Min Temperature of Coldest Month), BIO8 (Mean Temperature of Wettest Quarter), BIO9 (Mean Temperature of Driest Quarter), BIO14 (Precipitation of Driest Month), BIO15 (Precipitation Seasonality), BIO18 (Precipitation of Warmest Quarter), BIO19 (Precipitation of Coldest Quarter), and ten for the modeling of the Eurasian lynx: BIO1 (Annual Mean Temperature), BIO2 (Annual Mean Diurnal Range), BIO4 (Temperature Seasonality), BIO8 (Mean Temperature of Wettest Quarter), BIO9 (Mean Temperature of Driest Quarter), BIO11 (Mean Temperature of Coldest Quarter), BIO14 (Precipitation of Driest Month), BIO15 (Precipitation Seasonality), BIO18 (Precipitation of Warmest Quarter), BIO19 (Precipitation of Coldest Quarter). About the wolf, after the VIF analysis, we kept eight bioclimatic variables: BIO1 (Annual Mean Temperature), BIO2 (Annual Mean Diurnal Range), BIO4 (Temperature Seasonality), BIO8 (Mean Temperature of Wettest Quarter), BIO9 (Mean Temperature of Driest Quarter), BIO15 (Precipitation Seasonality), BIO18 (Precipitation of Warmest Quarter), BIO19 (Precipitation of Coldest Quarter).
The ENMs for the Eurasian lynx, the golden jackal, and the wolf resulted in high predictive performances, scoring a Boyce index value of B = 0.897, B = 0.869, and B = 0.921 respectively. The models calibrated on current climatic conditions reveal a wide, highly suitable territory in central Europe, between Germany and Poland, in the Pannonian basin, and the Po valley in Italy, for the golden jackal (Supplementary Figure 2A). Also, some minor and highly suitable patches appear in southern France and eastern Iberian peninsula, in the Northwestern coasts of the Black Sea, and Caucasus (Supplementary Figure 2A). Regarding the future predictions, a great expansion of the climatically-suitable areas is inferred (Supplementary Figures 3A–F). In general, a northeastern suitability gain is observed, as well as an increase in Germany and France, for all the future scenarios (Supplementary Figures 3A–F). The expert-based ENM for the current condition concerning the golden jackal shows similar habitat suitability compared with the prediction obtained with the first ENMs, exception made for a minor suitability between Poland and Germany, and southern Sweden (Supplementary Figure 4). The future habitat suitability maps obtained from expert-based ENMs indicate an analogous pattern of increase in habitat suitability towards the northernmost part of the study area (Supplementary Figures 5A–F). However, a slight difference is observed in Finland and among Ukraine and Russia (Supplementary Figures 3G–L, 5A–F). Regarding the Eurasian lynx, two highly suitable areas emerge, namely the main European mountain systems (Pyrenees, Alps, Apennines, Dinarides, Carpathians, and Caucasus) and the central and northern European territories, with the latter being vaster than the former (Supplementary Figure 2B). In future scenarios, the climatic suitability is generally predicted to massively decrease from all the aforementioned territories, especially in the central and northern European ones (but excluding the Scandinavian peninsula) (Supplementary Figures 3G–L).
3.2 Species’ frequencies in non-climatic predictors
The extraction of the information about slope, habitat, population density, snow cover, and wolf range performed in occurrence localities for both species highlighted different trends between the species.
About the habitat information (Supplementary Figure 6A), 40% of the occurrences of the golden jackal fall into the “Cropland” habitats, with the lynx reaching only 10% of its occurrences in this category. On the other side, the “Closed forests” resulted to be the most preferred habitat for the Eurasian lynx (~52%), while the golden jackal occurred in 27% of its total occurrences. The “Open forest” category had similar percentages for the two species: 11.4% for the Eurasian lynx and 11% for the golden jackal. Finally, the “Herbaceous vegetation” category is more used by the lynx (21.4%) compared with the golden jackal (6.4%).
Regarding slope, the golden jackal highly prefers low values of it (53.9% of occurrences in the 2% slope category, followed by 17.8% in the 3% slope one), while the Eurasian lynx uses territories both with low (32.5% for the 2% category, 16.6% for the 3%) and medium (10% of slope used in the ~5% of the occurrences) slopes (Supplementary Figure 6B).
Another result from the frequency of occurrence emerges from the ones falling into territories where human presence occurs. The golden jackal is mainly found in human settlements classified as low-density rural or rural clusters (78% and 13%, respectively), while the Eurasian lynx, when occurring in those settlements, is mainly found in low-density rural patches (89%) (Supplementary Figure 6C).
3.3 Weighted suitability models and overlap analyses
The weighted models obtained starting from the ENMs and from the frequency of occurrence of the two target species into the non-climatic predictors are reported for current (Figures 1C, D) and future (Figures 2A–L) conditions. The predictive performance resulted in B = 0.988 for the Eurasian lynx and B = 0.943 for the golden jackal.
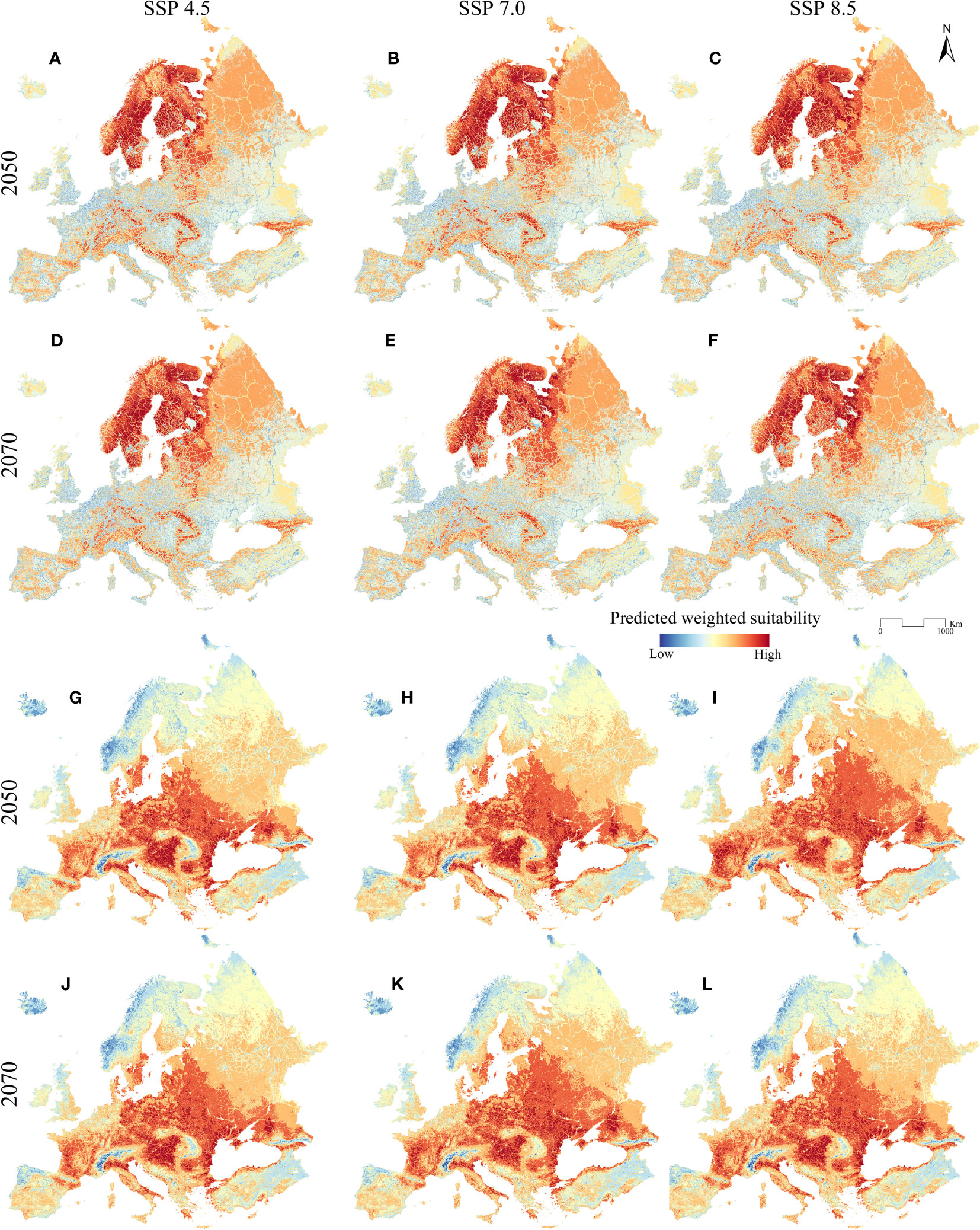
Figure 2 Future suitability predictions (low to high on a blue-to-red scale) for (A–F) the Eurasian lynx and (G–L) the golden jackal, for each different Shared Socio-economic Pathway and projection year considered.
The current weighted map resulted for the Eurasian lynx (Figure 1D) emphasized the high-suitability areas which are compliant with the current distribution of the species (Figure 1B), except for the suitable territories in the Apennines. In contrast, the golden jackal current weighted suitability map (Figure 1C) shows high values in areas from which the jackal is expanding, such as southern and eastern Europe. On the other side, areas where the jackal is currently extending its range (e.g., Baltic countries, Finland, and Norway) show lower environmental suitability.
About future weighted scenarios, the Eurasian lynx is predicted to face a reduction in its environmental suitability. The greatest reduction is observed for the 2070_SSP8.5 scenario, where only some clusters in central and southern Europe persist with medium-high values of environmental suitability (Figures 2A-F). Similarly, all the other projections in other year scenarios report similar trends, indicating that all the southern populations of the Eurasian lynx (the ones occurring in the Alps, Dinarides, Balkans, and Carpathians) could face a decrease in habitat suitability in the near future. Specifically, a clear decrease in suitability is observable in all future projections, and it is highlighted the fragmentation effect of the road network, especially in the Alps and the Balkans, resulting in a patchy mosaic of suitable areas separated by these infrastructures. By contrast, Scandinavia, the Baltic area, and northern Russia’s suitability remains stable at high values, even though the road network heavily influences those areas.
The future scenarios for the golden jackal, on the other hand, report an increase in its environmental suitability in the entire study area, except for the Atlantic coast of Scandinavia and northern Russia (Figures 2G–L). This can be observed across central Europe and in the Baltic States. Also, Italy, France, Balkan countries, and southern Sweden are predicted to be more suitable, with the greater increment represented by scenario 2070_8.5, where also Finland appears to be environmentally suitable for the golden jackal.
When binarizing the weighted predictions (10th percentile threshold = 7.6 for the Eurasian lynx, Figure 3A, and threshold = 6.4 for the golden jackal, Figure 3B) and subsequently assessing the current potential overlapping territories for the two species, we find that this is mainly limited to areas where mountain systems are not present (Figure 3C).
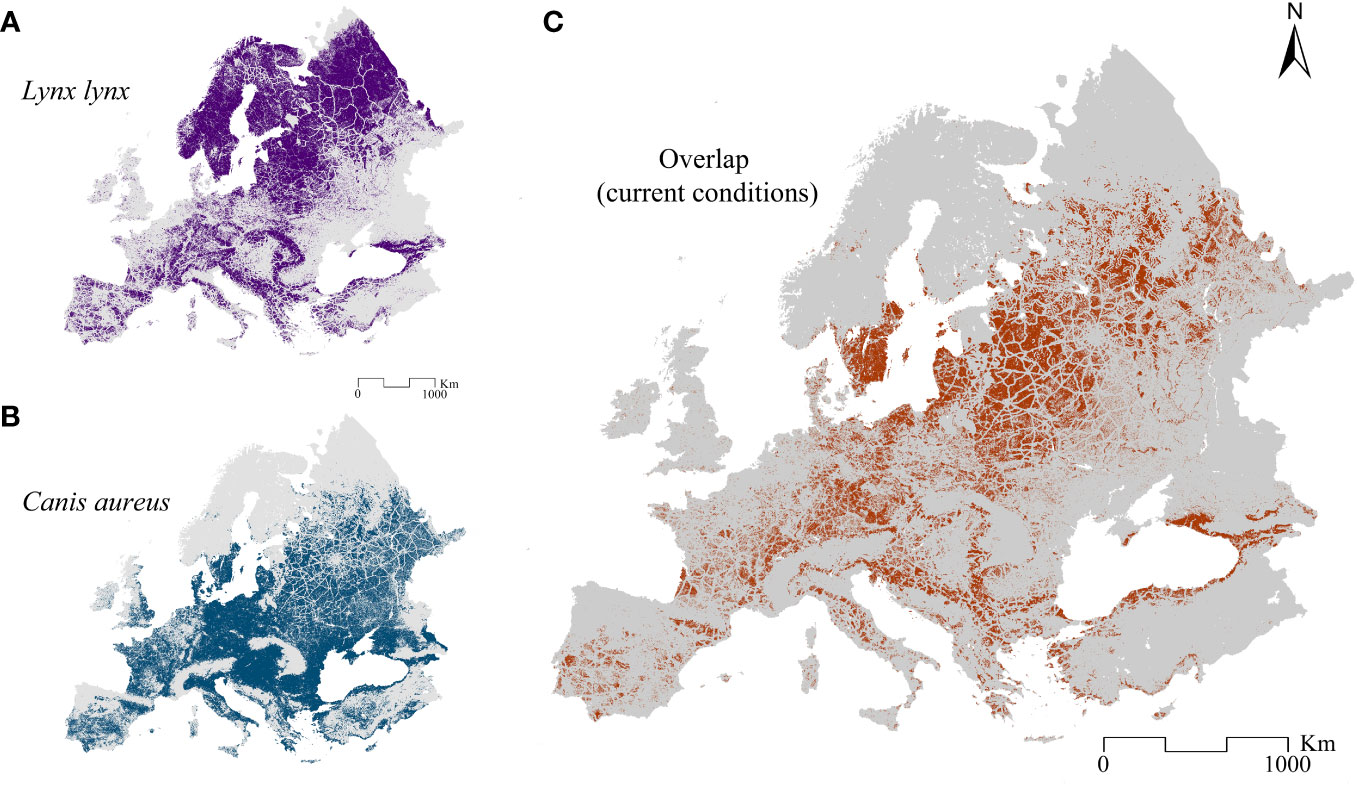
Figure 3 Binarized predictions and corresponding overlap. The binarized weighted suitability model for (A) the Eurasian lynx, (B) the golden jackal, and (C) the shared areas between the two models, obtained by overlapping them.
3.4 Future sympatry areas and overlap with grey wolf distribution
In the future, an evident trend emerges: the overlap between the two species gradually shifts towards northeastern Europe, concurrently decreasing in southern Europe (Supplementary Figure 7). When comparing these trends with the range shifts analyses (Supplementary Figure 7), we find that this pattern is driven both by the expansion of the environmental suitability of the golden jackal towards the north-east, and by the reduction of the environmental suitability of the Eurasian lynx in the southwest.
In particular, sympatric areas gradually increase in Finland, southern and eastern Sweden, and Russia, especially in the scenario 2070_8.5.
A slight increase in overlap arises on the slopes of the Carpathians Mountains, in the Balkan area, and in the Dinaric range, where both species occur. Also, along the Apennines, it emerges an increase in the overlapping ranges, where however the Eurasian lynx is not present and the golden jackal sporadically occurs.
Comparing these results with the current wolf-predicted suitable areas, most of the current sympatric areas appear to be overlapped (Figure 4). These mainly occur in the Carpathians Mountains, the Dinaric range, and various zones between Bulgaria, Albania, Macedonia, and Greece (Figure 4). Moreover, the current overlapping areas from the Baltic area to those in central Europe are also suitable for the grey wolf (Figure 4), which occur in some of these countries with stable populations. Similarly, between Italy and France, the predicted sympatric areas for the golden jackal and the Eurasian lynx are eligible for the wolf, which is recently spreading in these countries. Thus, considering the current wolf suitable areas, the potential sympatric areas for the golden jackal and Eurasian lynx (lacking wolf suitable areas) are only a few, located in the Pannonian Basin, Germany, Slovenia, and Russia (Figure 4).
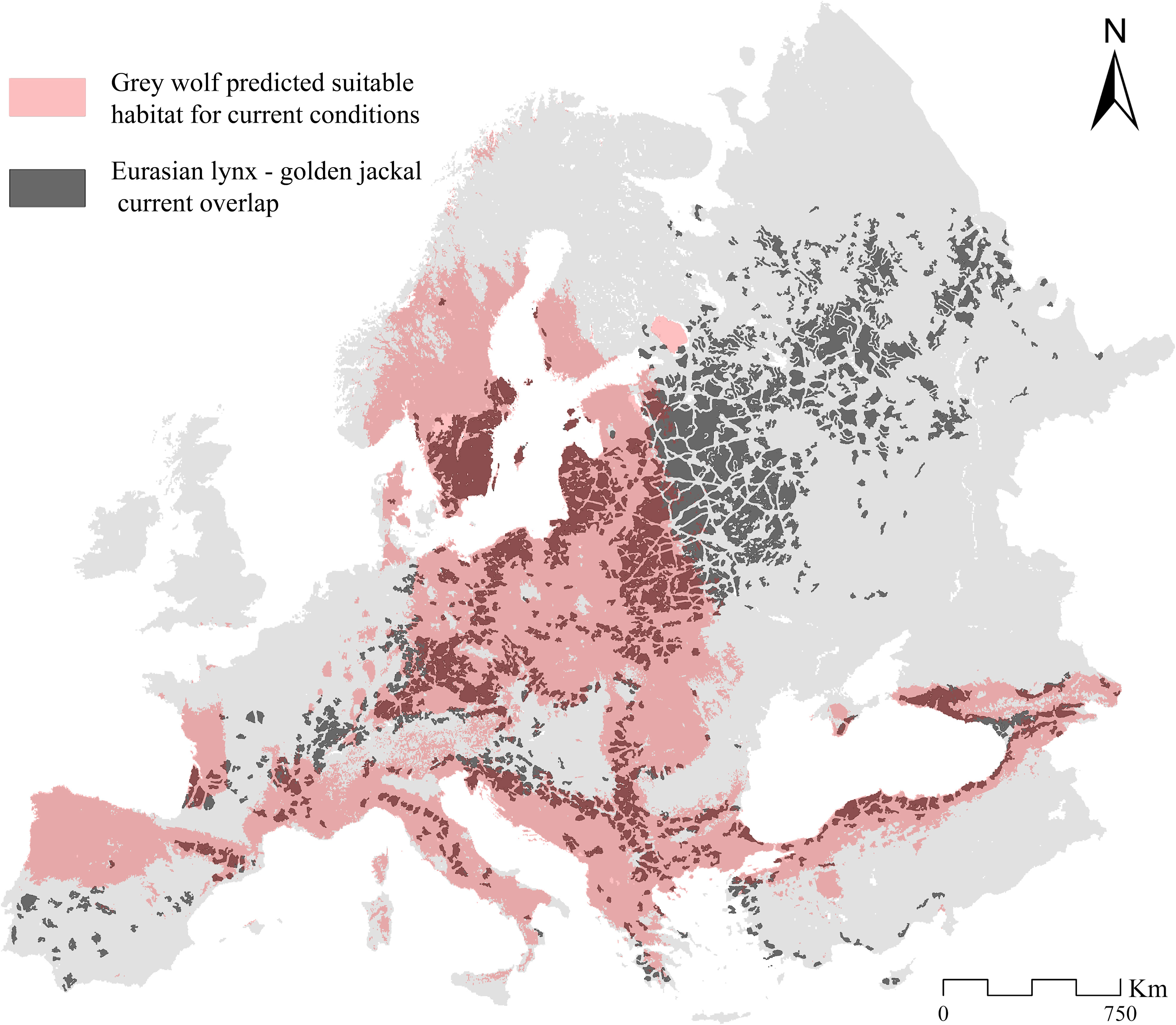
Figure 4 Predicted current overlap between the Eurasian lynx and the golden jackal (dark grey areas) and the grey wolf predicted suitable areas as inferred from the ENMs built on current climatic conditions.
Concerning the future predicted sympatric areas between the golden jackal and the Eurasian lynx, it is shown a gradual increase towards the northernmost part of the study area (Figure 5). Sympatric areas expand across the Baltic area, Russia, and Scandinavia. On the contrary, sympatric areas remain stable or decrease elsewhere in future scenarios (Figure 5). However, considering the future predictions for the wolf distribution, the great majoring of the predicted sympatric areas in Scandinavia and the Baltic area are covered by suitable areas (Figure 5). Thus, considering the predicted expansion of the wolf in these countries, it is unlikely that the golden jackal could spread significantly. A different situation could occur in central and southern Europe. In fact, the future predictions for the wolf show that its suitable areas will increase between France and Italy, and between France and Germany, but reduce in eastern Europe, with suitable areas kept only in the Carpathians, Balkans, and Dinaric range (Figure 5). Then, sympatric areas between the golden jackal and the Eurasian lynx in these areas could expand, such as in some zones in central Europe, between Germany and Poland (Figure 5).
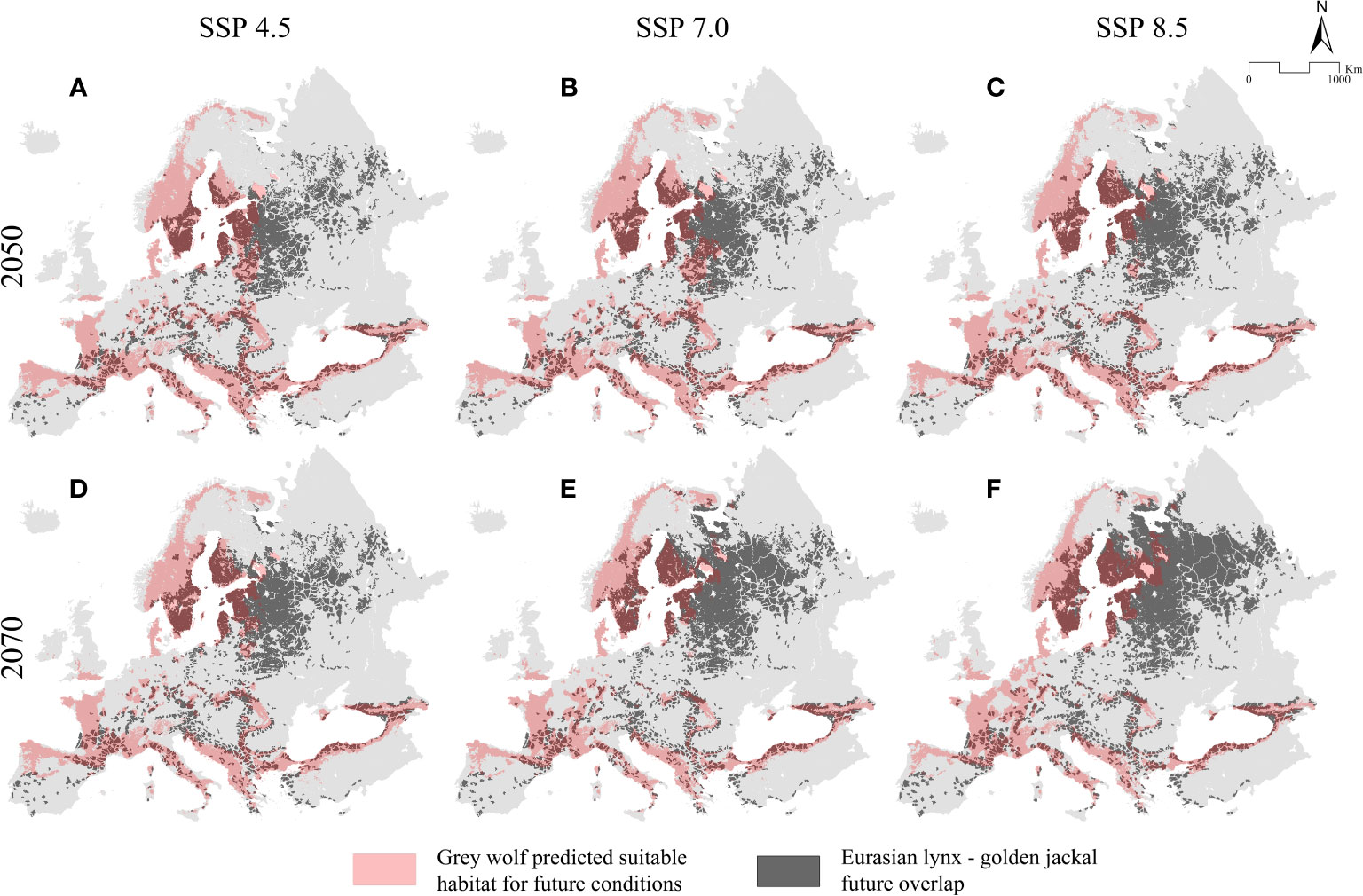
Figure 5 Predicted future overlap (A–F) between the Eurasian lynx and the golden jackal (dark grey) and the binarized predicted suitable range, inferred for future climatic scenarios, of the grey wolf.
4 Discussion
Europe is seeing a great return of some wildlife species in recent years (Deinet et al., 2013; Ledger et al., 2022), despite the effect of climate and land use changes. While these may favor the expansion of some species, for others they represent a threat that makes their future uncertain (Pacifici et al., 2020; Ledger et al., 2022), as also our results suggest (Figures 2A–L).
Indeed, the expansion of the jackal is favored by several factors: deforestation and land use changes (Šálek et al., 2014), additional anthropogenic trophic resources, the absence of competitors (such as the wolf) (Pyšková et al., 2016; Krofel et al., 2017; Spassov and Acosta-Pankov, 2019). In addition to these elements, climate change may also favor the expansion of the jackal. While in the past it was believed that deep snow could act as a limiting factor for the golden jackal, recent findings proved that the species is able to adapt even in areas with stable snow cover, such as in the boreal ecoregion. Although, in the Baltic states, it was reported that the golden jackal tends to avoid zones with permanent snow cover, preferring coastal areas without snow (Männil and Ranc, 2022). In our results, the greatest expansions are observed in the scenarios with the greatest impact of climate change, especially in the northern part of the study area (Figure 2G–L). The same expansion process affects not only higher latitudes but altitudes as well. In fact, the Range Shift analysis shows a growing rise of “gains” in the main mountain ranges of the study area. The strength of our results is highlighted by the fact that they are in line with those recently obtained (Cunze and Klimpel, 2022) for a similar area, with the important difference that in our analysis we used not only climatic predictors but also non-climatic parameters.
The redistribution of a species can lead to the appearance of new interactions with the fauna already present (Pecl et al., 2017). Changes in the dynamics of predation, herbivory, competition, and mutualism can all have substantial impacts at the community level (Gilman et al., 2010). The expansion of the golden jackal may change these dynamics, particularly interspecies competition, as observed from interactions with the red fox (Vulpes vulpes), where the golden jackal is dominant (Giannatos, 2004; Stoyanov, 2012). On the contrary, with the grey wolf, the cases of competition are few, and the golden jackal is usually defeated (Krofel et al., 2017). Competition is also possible with the European badger (Meles meles), pine marten (Martes martes), and stone marten (Martes foina) (Stratford, 2015). Data about the interactions between golden jackals and other large European predators are scant, but recently it has been observed a case of kleptoparasitism toward the Eurasian lynx (Krofel et al., 2022).
The recovery of the Eurasian lynx has been linked to a series of factors: various measures of legal protection, greater acceptance of this species by the population, reintroductions and recent population reinforcements in many European countries, the return of ungulate species that have expanded the availability of prey (Breitenmoser, 1998; Linnell et al., 2009). The modern expansion of this species is also associated with reduced human population density and increased forest cover (Cimatti et al., 2021). The current suitability map shows that there are large suitable zones in the study area where the species does not occur even if it could survive (e.g., in the Alpine range). The current environmental suitability is comparable with that obtained in a previous study (Dobbert et al., 2021). Our spatially explicit results are in line with those obtained from previous studies which explore lynx habitat selection at European scale (Ripari et al., 2022; Oeser et al., 2023). In fact, our maps of environmental suitability show that at the landscape scale, lynx tend to avoid human-modified areas and human infrastructures network, with habitat suitability remaining clustered in forested areas and/or zones associated with moderate slopes. But the future of this iconic predator is likely to be worse: our results of environmental suitability for the future show clear reductions, particularly around the Alps, in the Carpathian Mountains, and the Balkan area, with the suitability remaining high only in Scandinavia and northwestern Russia (Figures 2A–F; Supplementary Figure 4). Despite these reductions, the Eurasian lynx is a plastic species, especially regarding climatic conditions, and is able to survive even in warmer and drier habitats (Fležar et al., 2023).
In addition to these reductions, there are also some areas with increases in habitat suitability. In particular, beyond the Scandinavia and the Baltic area, good environmental suitability will be maintained in the Alps. Considering these predictions, it is essential to encourage conservation measures for the populations of Eastern Europe (such as the Balkan population and the Dinaric population), but it is also important to improve connectivity along the Alpine range to favor the expansion of the Alpine population.
Our overlap analysis shows that in the future the two species could have much more than the current 13% overlap between the ranges, as currently found by Krofel et al. (2022): this increase is mainly linked to the predicted expansion of the golden jackal throughout Europe, especially in the area between Russia, Baltics States and Scandinavia (Figures 2G–L; Supplementary Figure 4). In the same countries, Eurasian lynx suitability is expected to increase, so it could become an area of sympatry in the future. However, considering that these areas host stable populations of wolves, and that habitat suitability is predicted to increase in the future (Figure 5), it is unlikely that the golden jackal could expand significantly in the future, or at least his expansion will be restrained only in areas where the wolf is less abundant.
From a conservation point of view are interesting the overlap dynamics in the Carpathians, the Balkan area, and the Alpine area. Although our maps show future losses for the Eurasian lynx, some suitable patches will be maintained, and for the Alps, some increases are predicted. However, “gains” are also expected in the same areas for the golden jackal, therefore it is probable that in the future the slopes of these mountain ranges may also become areas of sympatry between the two species. But in the same mountain ranges the wolf is also expanding, and habitat suitability is predicted to increase (Figure 5). Thus, even in this case, the predicted sympatric areas between the golden jackal and the Eurasian lynx could be limited by the wolf’s presence. However, even considering the wolf’s presence, interactions between these species can’t be excluded a priori. And the consequences of this coexistence, not only between the golden jackal and Eurasian lynx but involving the grey wolf and the brown bear as well, must be deepened, especially considering that the lynx populations of Alpine, Balkan, and Dinaric areas are the focus of several conservation efforts, such as translocations and reintroductions (Topličanec et al., 2022).
A previous sympatric occurrence between the golden jackal, grey wolf, Eurasian lynx, and brown bear, was reported in a dense forest in Slovakia (Kysucké Beskydy Mountains), demonstrating the capacity of the golden jackal to coexist even in the presence of other large carnivores (Guimãraes et al., 2021). Thus, the adaptability of the golden jackal could led to different interactions with these large carnivores, beyond the ones reported.
Observing future scenarios, the greatest overlap among the ranges is observed in the 2070 scenario with SSP 8.5, a projection where is observed the greatest expansion for the golden jackal, especially in the northernmost areas of the study area. Despite the reduction in habitat suitability for the Eurasian lynx in future scenarios (especially 2070), the overlap between the ranges remains high because the “losses” of the lynx are balanced by the higher “gains” of the golden jackal (Supplementary Figure 4).
The results of the Point Sampling tool underline the known differences in the habitat preference between the Eurasian lynx and the golden jackal: while the jackal tends to prefer suburban rural environments, such as cropland, the lynx tends to select preferentially dense forests. Although, this does not exclude interactions, as both species may select the other’s preferred habitat. In fact, the golden jackal can select woodland habitats to search for burrows and reproduce, and its ecological plasticity allows it to use even more closed forests, especially when any apical predators are absent (Krofel et al., 2017). Still, Eurasian lynx select more open habitats (Belotti et al., 2013), even if close to humans, if they have high prey densities (Basille et al., 2009; Filla et al., 2017). Ungulates often are abundant near human infrastructures because they find protection from predators due to the proximity of man, a process known as the “human shield effect” (Berger, 2007). However, given that the golden jackal habitat selection is strongly influenced by the presence of wolves (Krofel et al., 2017), where sympatry may occur between the species (Figures 4, 5), the golden jackal habitat selection would probably change significantly.
After being the most widely distributed large terrestrial predator in the northern hemisphere, wolves went to a severe decline mainly due to human persecution (Ripple et al., 2014). Recently the species is recolonizing Europe: expanding both from its permanent range in Italy and Spain, and from the Dinaric-Balkan, Carpathian, and Polish lowland populations, recolonizing central Europe and Scandinavia, and expanding in eastern Europe (Boitani and Linnell, 2015; Hindrikson et al., 2017).
Several studies prove the wolf’s strong influence on the golden jackal through intraguild predation (Mohammadi et al., 2017), and the wolf’s absence is considered one of the main causes of golden jackal expansion (Krofel et al., 2017).
Our predicted sympatric areas for the golden jackal and the Eurasian lynx show that most of them overlap with current wolf-predicted suitable areas. In these areas it is more probable that the golden jackal could exploit different resources, like anthropogenic food sources, using more human-modified habitats and more open habitats. Consequently, the interactions with the Eurasian lynx would be significantly reduced.
Moreover, it should not be forgotten that Europe hosts other populations of a large predator (i.e., brown bear) and mesopredators, like the red fox. For example, the Eurasian lynx suffers kleptoparasitism from the red fox, which feeds on lynx prey (Krofel et al., 2019), and similarly, there are also cases of brown bears feeding on the prey hunted by the lynx (Krofel et al., 2012).
Future studies will be needed to fully understand the outcomes of the interaction between the golden jackal and the Eurasian lynx, an interaction already real, but which our study shows would be much more frequent in the future. Furthermore, future research will also have to better consider possible interactions, apart from kleptoparasitism, with other species of predators.
A possible limitation of our study could be linked to the dataset used, especially for the golden jackal, because we retained the records from dispersing individuals (i.e., out of the current core areas), and the main part of the dataset is obtained from the GBIF repository, known to be possibly spatially biased due to differences in data-sharing between countries and the consequent unequal effort of sampling (Beck et al., 2014). This could have led to an inflated effect on the environmental suitability in the northernmost part of the study area, even though the robust design of the modeling methods we used, together with the limited number of the dispersing individuals’ occurrences, buffer this issue. To check possible biases in the models’ output, we repeated the ecological niche modeling process by using only records reported as “permanent” or “high-confidence presence” from an expert-based dataset. Comparing these modeling outputs (Supplementary Figures 2–5), only some subtle differences are noted, resulting in similar habitat suitability values, both for current and future conditions. Thus, this interesting finding proves that for some specific contexts (i.e., modeling habitat suitability of a generalist species at the landscape scale), the use of citizen-science data could be as useful as data from expert-based datasets. However, considering the small differences in the northernmost part of the study area between the two different ENMs (the ones built with our dataset and the one obtained from the expert-based dataset), we recommend considering this aspect when exploring our maps. However, the future availability of more robust and expert-based datasets would surely improve the quality of ecological modeling studies, and for species in rapid expansion, these datasets are also urgently needed.
In this complex context of large carnivores expansion through the European landscapes, is important to improve measures that could bring the conservation of the entire biocenosis: for example, regulating hunting activities, especially of wolves and ungulates, in order to reduce possible competition between large predators. Other measures could include the coverage increase of protected areas, which have favored the expansion of large carnivores in the past, together with an increasing public acceptance (Dressel et al., 2015), especially in areas where these species are making a comeback after some time. The use of economic compensation for any damage by predators can also help improve the public acceptance of these species (Dickman et al., 2011).
In conclusion, our results can support conservationists by identifying which areas these two carnivores could coexist in the future. Both in areas already occupied by both species and in those where sympatry is expected in the future, further studies will be needed to better understand the effects of this coexistence and any interactions.
Data availability statement
The raw data supporting the conclusions of this article will be made available by the authors, without undue reservation.
Author contributions
DS and VC collected the data; DS and MI analysed the data; MI and MB provided the scope and guidance, DS authored the first draft of the manuscript; all authors wrote the article and gave final approval for submission.
Conflict of interest
The authors declare that the research was conducted in the absence of any commercial or financial relationships that could be construed as a potential conflict of interest.
Publisher’s note
All claims expressed in this article are solely those of the authors and do not necessarily represent those of their affiliated organizations, or those of the publisher, the editors and the reviewers. Any product that may be evaluated in this article, or claim that may be made by its manufacturer, is not guaranteed or endorsed by the publisher.
Supplementary material
The Supplementary Material for this article can be found online at: https://www.frontiersin.org/articles/10.3389/fevo.2023.1165968/full#supplementary-material
References
Aleksandra P., Duško Ć. (2015). Seasonal variation in diet of the golden jackal (Canis aureus) in Serbia. Mamm Res. 60, 309–317. doi: 10.1007/s13364-015-0241-1
Arnold J., Humer A., Heltai M., Murariu D., Spassov N., Hackländer K. (2012). Current status and distribution of golden jackals Canis aureus in Europe. Mammal Rev. 42, 1–11. doi: 10.1111/j.1365-2907.2011.00185.x
Barbet-Massin M., Jiguet F., Albert C. H., Thuiller W. (2012a). Selecting pseudo-absences for species distribution models: how, where and how many? Methods Ecol. Evol. 3, 327–338. doi: 10.1111/j.2041-210X.2011.00172.x
Barbet-Massin M., Thuiller W., Jiguet F. (2012b). The fate of European breeding birds under climate, land-use and dispersal scenarios. Global Change Biol. 18, 881–890. doi: 10.1111/j.1365-2486.2011.02552.x
Basille M., Herfindal I., Santin-Janin H., Linnell J. D., Odden J., Andersen R., et al. (2009). What shapes Eurasian lynx distribution in human dominated landscapes: selecting prey or avoiding people? Ecography 32, 683–691. doi: 10.1111/j.1600-0587.2009.05712.x
Beck J., Böller M., Erhardt A., Schwanghart W. (2014). Spatial bias in the GBIF database and its effect on modeling species’ geographic distributions. Ecol. Inf. 19, 10–15. doi: 10.1016/j.ecoinf.2013.11.002
Belotti E., Červený J., Šustr P., Kreisinger J., Gaibani G., Bufka L. (2013). Foraging sites of Eurasian lynx Lynx lynx: relative importance of microhabitat and prey occurrence. Wildlife Biol. 19, 188–201. doi: 10.2981/12-077
Berger J. (2007). Fear, human shields and the redistribution of prey and predators in protected areas. Biol. Lett. 3, 620–623. doi: 10.1098/rsbl.2007.0415
Böcker F., Weber H., Collet S. (2023). First documentation of golden jackal (Canis aureus) reproduction in Germany. Mamm Res. 68, 249–252. doi: 10.1007/s13364-022-00666-y
Boitani L., Linnell J. D. C. (2015). “Bringing Large Mammals Back: Large Carnivores in Europe,” in Rewilding European Landscapes. Eds. Pereira H. M., Navarro L. M. (Cham: Springer International Publishing), 67–84. doi: 10.1007/978-3-319-12039-3_4
Boucher O., Servonnat J., Albright A. L., Aumont O., Balkanski Y., Bastrikov V., et al. (2020). Presentation and evaluation of the IPSL-CM6A-LR climate model. J. Adv. Modeling Earth Syst. 12, e2019MS002010. doi: 10.1029/2019MS002010
Bouyer Y., San Martin G., Poncin P., Beudels-Jamar R. C., Odden J., Linnell J. D. (2015). Eurasian lynx habitat selection in human-modified landscape in Norway: effects of different human habitat modifications and behavioral states. Biol. Conserv. 191, 291–299. doi: 10.1016/j.biocon.2015.07.007
Brandt L. A., Benscoter A. M., Harvey R., Speroterra C., Bucklin D., Romañach S. S., et al. (2017). Comparison of climate envelope models developed using expert-selected variables versus statistical selection. Ecol. Model. 345, 10–20. doi: 10.1016/j.ecolmodel.2016.11.016
Breitenmoser U. (1998). Large predators in the Alps: the fall and rise of man’s competitors. Biol. Conserv. 83, 279–289. doi: 10.1016/S0006-3207(97)00084-0
Brown J. L., Bennett J. R., French C. M. (2017). SDMtoolbox 2.0: the next generation Python-based GIS toolkit for landscape genetic, biogeographic and species distribution model analyses. PeerJ 5, e4095. doi: 10.7717/peerj.4095
Buchhorn M., Smets B., Bertels L., De Roo B., Lesiv M., Tsendbazar N.-E., et al (2020). Copernicus global land service: Land cover 100m: collection 3: epoch 2019: Globe. Version V3. 0.1. doi: 10.5281/zenodo.3939050
Carvalho J., Torres R. T., Acevedo P., Santos J. P., Barros T., Serrano E., et al. (2018). Propagule pressure and land cover changes as main drivers of red and roe deer expansion in mainland Portugal. Diversity Distributions 24, 551–564. doi: 10.1111/ddi.12703
Chapron G., Kaczensky P., Linnell J. D. C., von Arx M., Huber D., Andrén H., et al. (2014). Recovery of large carnivores in Europe’s modern human-dominated landscapes. Science 346, 1517–1519. doi: 10.1126/science.1257553
Cimatti M., Ranc N., Benítez-López A., Maiorano L., Boitani L., Cagnacci F., et al. (2021). Large carnivore expansion in Europe is associated with human population density and land cover changes. Diversity Distributions 27, 602–617. doi: 10.1111/ddi.13219
Ćirović D., Penezić A., Krofel M. (2016). Jackals as cleaners: Ecosystem services provided by a mesocarnivore in human-dominated landscapes. Biol. Conserv. 199, 51–55. doi: 10.1016/j.biocon.2016.04.027
Cunze S., Klimpel S. (2022). From the Balkan towards Western Europe: Range expansion of the golden jackal (Canis aureus) – A climatic niche modeling approach. Ecol. Evol. 12, e9141. doi: 10.1002/ece3.9141
Dawe K. L., Boutin S. (2016). Climate change is the primary driver of white-tailed deer (Odocoileus virginianus) range expansion at the northern extent of its range; land use is secondary. Ecol. Evol. 6, 6435–6451. doi: 10.1002/ece3.2316
Deinet S., Ieronymidou C., McRae L., Burfield I. J., Foppen R. P., Collen B., et al. (2013). Wildlife comeback in Europe. The recovery of selected mammal and bird species (UK: Zoological Society of London).
De Marco P. Jr., Diniz-Filho J. A. F., Bini L. M. (2008). Spatial analysis improves species distribution modelling during range expansion. Biol. Lett. 4, 577–580. doi: 10.1098/rsbl.2008.0210
Dickman A. J., Macdonald E. A., Macdonald D. W. (2011). A review of financial instruments to pay for predator conservation and encourage human–carnivore coexistence. Proc. Natl. Acad. Sci. 108, 13937–13944. doi: 10.1073/pnas.1012972108
Dobbert S., Pape R., Löffler J. (2021). Continent-wide modelling of potential habitats and connectivity for the eurasian lynx (Lynx lynx) in Europe. Erdkunde, (H. 2), 69–86. doi: 10.3112/erdkunde.2021.02.01
Dressel S., Sandström C., Ericsson G.. (2015). A meta-analysis of studies on attitudes toward bears and wolves across Europe 1976–2012. Conservation Biology 29(2), 565–574.
Elith J., Kearney M., Phillips S. (2010). The art of modelling range-shifting species. Methods Ecol. Evol. 1, 330–342. doi: 10.1111/j.2041-210X.2010.00036.x
Fenton S., Moorcroft P. R., Ćirović D., Lanszki J., Heltai M., Cagnacci F., et al. (2021). Movement, space-use and resource preferences of European golden jackals in human-dominated landscapes: insights from a telemetry study. Mamm. Biol. 101, 619–630. doi: 10.1007/s42991-021-00109-2
Fick S. E., Hijmans R. J. (2017). WorldClim 2: new 1-km spatial resolution climate surfaces for global land areas. Int. J. climatol. 37, 4302–4315. doi: 10.1002/joc.5086
Filla M., Premier J., Magg N., Dupke C., Khorozyan I., Waltert M., et al. (2017). Habitat selection by Eurasian lynx (Lynx lynx) is primarily driven by avoidance of human activity during day and prey availability during night. Ecol. Evol. 7, 6367–6381. doi: 10.1002/ece3.3204
Fležar U., Hočevar L., Sindičić M., Gomerčić T., Konec M., Slijepčević V., et al. (2023). Surveillance of the reinforcement process of the Dinaric -SE Alpine lynx population in the lynx-monitoring year 2021-2022. University of Ljubljana.
Giannatos G. (2004). Conservation action plan for the golden jackal Canis aureus L. Greece Vol. 47 (WWF, Greece).
Gilman S. E., Urban M. C., Tewksbury J., Gilchrist G. W., Holt R. D. (2010). A framework for community interactions under climate change. Trends Ecol. Evol. 25, 325–331. doi: 10.1016/j.tree.2010.03.002
Guimãraes N., Bučko J., Slamka M. (2021). At the table with the big three carnivores-a sympatric occurrence of the golden jackal with bear, wolf and lynx captured on a camera trap in Slovakia. Eur. J. Ecol. 7 (1), 126–132. doi: 10.17161/eurojecol.v7i1.14655
Hall D. K., Riggs G. A. (2021). MODIS/Terra Snow Cover Daily L3 Global 0.05Deg CMG, Version 61. NASA National snow and ice data center distributed active archive center. doi: 10.5067/MODIS/MOD10C1.061
Halliez G., Renault F., Vannard E., Farny G., Lavorel S., Giraudoux P. (2015). Historical agricultural changes and the expansion of a water vole population in an Alpine valley. Agriculture Ecosyst. Environ. 212, 198–206. doi: 10.1016/j.agee.2015.07.006
Hatlauf J., Banea O. C., Lapini L. (2016). Assessment of golden jackal species (Canis aureus, L. 1758) records in natural areas out of their known historic range. OJAGE Golden Jackal Informal Study Group Europe. GOJAGE eBulletin 12, 1–7. doi: 10.13140/RG.2.1.1429.2243
Hatlauf J., Bayer K., Trouwborst A., Hackländer K. (2021). New rules or old concepts? The golden jackal (Canis aureus) and its legal status in Central Europe. Eur. J. Wildl Res. 67, 25. doi: 10.1007/s10344-020-01454-2
Heurich M., Schultze-Naumburg J., Piacenza N., Magg N., Červený J., Engleder T., et al. (2018). Illegal hunting as a major driver of the source-sink dynamics of a reintroduced lynx population in Central Europe. Biol. Conserv. 224, 355–365. doi: 10.1016/j.biocon.2018.05.011
Hijmans R., Phillips S., Leathwick J., Elith J. (2016). dismo: species distribution modeling (R package ver. 1), 1–4.
Hindrikson M., Remm J., Pilot M., Godinho R., Stronen A. V., Baltrūnaité L., et al. (2017). Wolf population genetics in Europe: a systematic review, meta-analysis and suggestions for conservation and management. Biol. Rev. 92, 1601–1629. doi: 10.1111/brv.12298
Hirzel A. H., Le Lay G., Helfer V., Randin C., Guisan A. (2006). Evaluating the ability of habitat suitability models to predict species presences. Ecol. Model. 199, 142–152. doi: 10.1016/j.ecolmodel.2006.05.017
Hočevar L., Oliveira T., Krofel M. (2021). Felid bedrooms with a panoramic view: selection of resting sites by Eurasian lynx (Lynx lynx) in a karstic landscape. Behav. Ecol. Sociobiol. 75, 1–11. doi: 10.1007/s00265-021-02977-7
Hoffmann M., Hilton-Taylor C., Angulo A., Böhm M., Brooks T. M., Butchart S. H., et al. (2010). The impact of conservation on the status of the world’s vertebrates. Science 330, 1503–1509. doi: 10.1126/science.1194442
Iannella M., Cerasoli F., Biondi M. (2017). Unraveling climate influences on the distribution of the parapatric newts Lissotriton vulgaris meridionalis and L. italicus. Front. zoology 14, 1–14. doi: 10.1186/s12983-017-0239-4
Iannella M., Console G., Cerasoli F., De Simone W., D’Alessandro P., Biondi M. (2021). A step towards SDMs: A “couple-and-weigh” framework based on accessible data for biodiversity conservation and landscape planning. Diversity Distributions 27, 2412–2427. doi: 10.1111/ddi.13410
Iannella M., D’Alessandro P., Longo S., Biondi M. (2019). New records and potential distribution by Ecological Niche Modeling of Monoxia obesula in the Mediterranean area. Bull. Insectol. 72, 135–142.
Inger R., Gregory R., Duffy J. P., Stott I., Voříšek P., Gaston K. J. (2015). Common European birds are declining rapidly while less abundant species’ numbers are rising. Ecol. Lett. 18, 28–36. doi: 10.1111/ele.12387
Jędrzejewski W., Schmidt K., Miłkowski L., Jędrzejewska B., Okarma H. (1993). Foraging by lynx and its role in ungulate mortality: the local (Białowieża Forest) and the Palaearctic viewpoints. Acta theriologica 38, 385–403. doi: 10.4098/AT.arch.93-30
Jiménez-Valverde A., Peterson A. T., Soberón J., Overton J., Aragón P., Lobo J. M. (2011). Use of niche models in invasive species risk assessments. Biol. invasions 13, 2785–2797. doi: 10.1007/s10530-011-9963-4
Kaczensky P., Linnell J., Huber D., Von Arx M., Andren H., Breitenmoser U., et al. (2021). Distribution of Large Carnivores in Europe 2012–2016: Distribution maps for Brown bear, Eurasian lynx, Grey wolf, and Wolverine (Dryad Dataset).
Kramer-Schadt S., Revilla E., Wiegand T., Breitenmoser U. (2004). Fragmented landscapes, road mortality and patch connectivity: modelling influences on the dispersal of Eurasian lynx. J. Appl. Ecol. 41, 711–723. doi: 10.1111/j.0021-8901.2004.00933.x
Krofel M., Giannatos G., Ćirovič D., Stoyanov S., Newsome T. M. (2017). Golden jackal expansion in Europe: a case of mesopredator release triggered by continent-wide wolf persecution? Hystrix: Ital. J. mammal. 28, 9–15. doi: 10.4404/hystrix-28.1-11819
Krofel M., Hočevar L., Fležar U., Topličanec I., Oliveira T. (2022). Golden jackal as a new kleptoparasite for Eurasian lynx in Europe. Global Ecol. Conserv. 36, e02116. doi: 10.1016/j.gecco.2022.e02116
Krofel M., Kos I., Jerina K. (2012). The noble cats and the big bad scavengers: effects of dominant scavengers on solitary predators. Behav. Ecol. Sociobiol. 66, 1297–1304. doi: 10.1007/s00265-012-1384-6
Krofel M., Skrbinšek T., Mohorović M. (2019). Using video surveillance to monitor feeding behaviour and kleptoparasitism at Eurasian lynx kill sites. Folia Zoologica 68, 274–284. doi: 10.25225/fozo.037.2019
Kryštufek B., Murariu D., Kurtonur C. (1997). Present distribution of the Golden Jackal Canis aureus in the Balkans and adjacent regions. Mammal Rev. 27, 109–114. doi: 10.1111/j.1365-2907.1997.tb00375.x
Lange P. N., Lelieveld G., De Knegt H. J. (2021). Diet composition of the golden jackal Canis aureus in south-east Europe–a review. Mammal Rev. 51, 207–213. doi: 10.1111/mam.12235
Lapini L., Conte D., Zupan M., Kozlan L. (2011). Italian jackals 1984-2011: an updated review (Canis aureus: Carnivora, Canidae). Bollettino del Museo Civico di Storia Naturale di Venezia 62, 219–232.
Ledger S. E., Rutherford C. A., Benham C., Burfield I. J., Deinet S., Eaton M., et al. (2022). Wildlife Comeback in Europe: Opportunities and challenges for species recovery. Final report to rewilding Europe by the Zoological society of London, Birdlife international and the European bird census council., (ZSL, London, UK) doi: 10.13140/RG.2.2.24283.44324
Lehner B., Grill G. (2013). Global river hydrography and network routing: baseline data and new approaches to study the world’s large river systems. Hydrological Processes 27, 2171–2186. doi: 10.1002/hyp.9740
Leroy B., Delsol R., Hugueny B., Meynard C. N., Barhoumi C., Barbet-Massin M., et al. (2018). Without quality presence–absence data, discrimination metrics such as TSS can be misleading measures of model performance. J. Biogeogr. 45, 1994–2002. doi: 10.1111/jbi.13402
Linnell J. D., Breitenmoser U., Breitenmoser-Würsten C., Odden J., von Arx M. (2009). Recovery of Eurasian lynx in Europe: what part has reintroduction played. Reintroduction top-order predators 4, 72–91. doi: 10.1002/9781444312034.ch4
Maiorano L., Falcucci A., Zimmermann N. E., Psomas A., Pottier J., Baisero D., et al. (2011). The future of terrestrial mammals in the Mediterranean basin under climate change. Philos. Trans. R. Soc. B: Biol. Sci. 366, 2681–2692. doi: 10.1098/rstb.2011.0121
Männil P., Ranc N. (2022). Golden jackal (Canis aureus) in Estonia: development of a thriving population in the boreal ecoregion. Mamm Res. 67, 245–250. doi: 10.1007/s13364-021-00615-1
Markov G., Lanszki J. (2012). Diet composition of the golden jackal, Canis aureus in an agricultural environment. Folia Zoologica 61, 44–48. doi: 10.25225/fozo.v61.i1.a7.2012
Mohammadi A., Kaboli M., López-Bao J. V. (2017). Interspecific killing between wolves and golden jackals in Iran. European journal of wildlife research 63, 1–5.
Nagl D., Breitenmoser U., Hackländer K., Ryser A., Zimmermann F., Signer S., et al. (2022). Long-term changes in habitat selection and prey spectrum in a reintroduced Eurasian lynx (Lynx lynx) population in Switzerland. Ecol. Evol. 12, e8614. doi: 10.1002/ece3.8614
Naimi B. (2015). USDM: Uncertainty analysis for species distribution models. R package version 1.1–15. R Documentation.
Newsome T. M., Greenville A. C., Ćirović D., Dickman C. R., Johnson C. N., Krofel M., et al. (2017). Top predators constrain mesopredator distributions. Nat. Commun. 8, 1–7. doi: 10.1038/ncomms15469
Nolet B. A., Rosell F. (1998). Comeback of the beaver Castor fiber: an overview of old and new conservation problems. Biol. Conserv. 83, 165–173. doi: 10.1016/S0006-3207(97)00066-9
Odden J., Linnell J. D. C., Andersen R. (2006). Diet of Eurasian lynx, Lynx lynx, in the boreal forest of southeastern Norway: the relative importance of livestock and hares at low roe deer density. Eur. J. Wildl Res. 52, 237–244. doi: 10.1007/s10344-006-0052-4
Oeser J., Heurich M., Kramer-SChadt S., Andrén H., Bagrade G., Belotti E., et al. (2023). Prerequisites for coexistence: human pressure and refuge habitat availability shape continental-scale habitat use patterns of a large carnivore. Landsc Ecol 38(7), 1713–1728. doi: 10.1007/s10980-023-01645-7
Okarma H., Jedrzejewski W., Schmidt K., KowALCZYK R., Jedrzejewska B. (1997). Predation of Eurasian lynx on roe deer and red deer in Bialowieza Primeral Forest, Poland. Acta Theriologica 42, 203–224. doi: 10.4098/AT.arch.97-22
Pacifici M., Rondinini C., Rhodes J. R., Burbidge A. A., Cristiano A., Watson J. E. M., et al. (2020). Global correlates of range contractions and expansions in terrestrial mammals. Nat. Commun. 11, 2840. doi: 10.1038/s41467-020-16684-w
Pecl G. T., Araújo M. B., Bell J. D., Blanchard J., Bonebrake T. C., Chen I.-C., et al. (2017). Biodiversity redistribution under climate change: Impacts on ecosystems and human well-being. Science 355, eaai9214. doi: 10.1126/science.aai9214
Pesaresi M., Florczyk A., Schiavina M., Melchiorri M., Maffenini L. (2019). GHS settlement grid, updated and refined regio model 2014 in application to ghs-built r2018a and ghs-pop r2019a, multitemporal, (1975-1990-2000-2015) r2019a. Eur. Commission Joint Res. Centre (JRC) 10. doi: 10.2905/42E8BE89-54FF-464E-BE7B-BF9E64DA5218
Pilotto F., Kühn I., Adrian R., Alber R., Alignier A., Andrews C., et al. (2020). Meta-analysis of multidecadal biodiversity trends in Europe. Nat. Commun. 11, 3486. doi: 10.1038/s41467-020-17171-y
Podgórski T., Schmidt K., Kowalczyk R., Gulczyńska A. (2008). Microhabitat selection by Eurasian lynx and its implications for species conservation. Acta Theriologica 53, 97–110. doi: 10.1007/BF03194243
Prugh L. R., Stoner C. J., Epps C. W., Bean W. T., Ripple W. J., Laliberte A. S., et al. (2009). The rise of the mesopredator. Bioscience 59, 779–791. doi: 10.1525/bio.2009.59.9.9
Pyšková K., Storch D., Horáček I., Kauzál O., Pyšek P. (2016). Golden jackal (Canis aureus) in the Czech Republic: the first record of a live animal and its long-term persistence in the colonized habitat. Zookeys 151–163. doi: 10.3897/zookeys.641.10946
Ranc N., Acosta-Pankov I., Balys V., Bučko J., Cirovic D., Fabijanić N., et al. (2022). Distribution of large carnivores in Europe 2012 - 2016: Distribution map for Golden Jackal (Canis aureus). doi: 10.5281/zenodo.6382216
Ranc N., Álvares F., Banea O., Berce T., Caganacci F., Červinka J., et al. (2018). The golden jackal in Europe: Where to go next? Proceedings of the 2nd International Jackal Symposium. Marathon Bay, Attiki, Hell Zool Arch.
Ranc N., Cagnacci F., Banea O., Berce T., Ćirović D., Csànyi S., et al. (2015). Where to go next? Predicting habitat suitability of an expanding mesocarnivore: the golden jackal (Canis aureus) in Europe. ICCB : 27th International Congress for Conservation Biology, 4th European Congress for Conservation Biology, Montpellier, France.
Ražen N., Brugnoli A., Castagna C., Groff C., Kaczensky P., Kljun F., et al. (2016). Long-distance dispersal connects Dinaric-Balkan and Alpine grey wolf (Canis lupus) populations. Eur. J. wildlife Res. 62, 137–142. doi: 10.1007/s10344-015-0971-z
R Core Team (2016). R: A language and environment for statistical computing (Vienna, Austria: R Foundation for Statistical Computing). Available at: http://www.R-project.org/.
Riahi K., Van Vuuren D. P., Kriegler E., Edmonds J., O’neill B. C., Fujimori S., et al. (2017). The shared socioeconomic pathways and their energy, land use, and greenhouse gas emissions implications: an overview. Global Environ. Change 42, 153–168. doi: 10.1016/j.gloenvcha.2016.05.009
Ripari L., Premier J., Belotti E., Bluhm H., Breitenmoser-Würsten C., Bufka L., et al. (2022). Human disturbance is the most limiting factor driving habitat selection of a large carnivore throughout Continental Europe. Biol. Conserv. 266, 109446. doi: 10.1016/j.biocon.2021.109446
Ripple W. J., Estes J. A., Beschta R. L., Wilmers C. C., Ritchie E. G., Hebblewhite M., et al. (2014). Status and ecological effects of the world’s largest carnivores. Science 343, 1241484. doi: 10.1126/science.1241484ù
Ripple W. J., Wirsing A. J., Wilmers C. C., Letnic M. (2013). Widespread mesopredator effects after wolf extirpation. Biol. Conserv. 160, 70–79. doi: 10.1016/j.biocon.2012.12.033
Rodríguez-Rey M., Consuegra S., Börger L., Garcia de Leaniz C. (2019). Improving Species Distribution Modelling of freshwater invasive species for management applications. PloS One 14, e0217896. doi: 10.1371/journal.pone.0217896
Rykov A. M., Kuznetsova A. S., Tirronen K. F. (2022). The first record of the golden jackal (Canis aureus Linnaeus 1758) in the Russian subarctic. Polar Biol. 45, 965–970. doi: 10.1007/s00300-022-03037-0
Šálek M., Červinka J., Banea O. C., Krofel M., Ćirović D., Selanec I., et al. (2014). Population densities and habitat use of the golden jackal (Canis aureus) in farmlands across the Balkan Peninsula. Eur. J. Wildl Res. 60, 193–200. doi: 10.1007/s10344-013-0765-0
Serva D., Biondi M., Iannella M. (2023). The Eurasian beaver range expansion reveals uneven future trends and possible conservation issues: an European assessment. Biodiversity Conserv., 1–18. doi: 10.1007/s10531-023-02587-x
Sillero N., Barbosa A. M. (2021). Common mistakes in ecological niche models. Int. J. Geographical Inf. Sci. 35, 213–226. doi: 10.1080/13658816.2020.1798968
Sørensen O., Lindsø L. (2021). The golden jackal Canis aureus detected in Norway – management challenges with naturally dispersed species new to the country. Fauna 74, 74–87.
Spassov N. (1989). The position of jackais in the Canis genus and iife-history of the goiden jackai (Canis aureus l.) in Buigaria and the baikans. Hist. Naturalis Bulgarica 1, 44–56.
Spassov N. (2007). “Order Carnivora,” in The mammals, important for conservation in Bulgaria. Eds. Miteva S., Mihova B., Georgiev K., Petrov B., Vandino D. (Arnhem, Netherlands: Dutch Mammal Society VZZ), 217–290.
Spassov N., Acosta-Pankov I. (2019). Dispersal history of the golden jackal (Canis aureus moreoticus Geoffroy 1835) in Europe and possible causes of its recent population explosion. Biodivers Data J. 7, e34825. doi: 10.3897/BDJ.7.e34825
Stahl P., Vandel J., Herrenschmidt V., Migot P. (2001). Predation on livestock by an expanding reintroduced lynx population: long-term trend and spatial variability. J. Appl. Ecol. 38, 674–687. doi: 10.1046/j.1365-2664.2001.00625.x
Stoyanov S. (2012). Golden jackal (Canis aureus) in Bulgaria. Current status, distribution, demography and diet. In Proceedings from the 2nd international symposium on hunting ‘modern aspects of sustainable management of game population’, Zemun-Belgrade, Serbia. 22–24.
Stralberg D., Matsuoka S., Hamann A., Bayne E., Sólymos P., Schmiegelow F., et al. (2015). Projecting boreal bird responses to climate change: the signal exceeds the noise. Ecol. Appl. 25, 52–69. doi: 10.1890/13-2289.1
Stratford J. (2015). Golden jackal in Lithuania, a consideration of its arrival, impact and status. Zool. Ecol. 25, 277–287. doi: 10.1080/21658005.2015.1073894
Tatebe H., Ogura T., Nitta T., Komuro Y., Ogochi K., Takemura T., et al. (2019). Description and basic evaluation of simulated mean state, internal variability, and climate sensitivity in MIROC6. Geoscientific Model. Dev. 12, 2727–2765. doi: 10.5194/gmd-12-2727-2019
Thuiller W., Georges D., Engler R., Breiner F., Georges M. D., Thuiller C. W. (2016). Package ‘biomod2.’ Species Distrib. Model. within an ensemble Forecast. Framew. R package version 3.3-7. Available at: http://CRAN.R-project.org/package=biomod2.
Topličanec I., Gomerčić T., Černe R., Krofel M., Pop I.-M., Kubala J., et al. (2022). Early post-release behaviour of Eurasian lynx translocated to the transboundary region of the Dinaric Mountains. fozo.1 71, 22064.1–16. doi: 10.25225/jvb.22064
Trouwborst A., Krofel M., Linnell J. D. C. (2015). Legal implications of range expansions in a terrestrial carnivore: the case of the golden jackal (Canis aureus) in Europe. Biodivers Conserv. 24, 2593–2610. doi: 10.1007/s10531-015-0948-y
VerCauteren K. C. (2003). The deer boom: discussins on population growth and range expansion of the white-tailed deer. Hisey G., Hisey K., editors. Bowhunting records of North American white-tailed deer, Second edition. The Pope and Young Club, Chatfield, Minnesota, USA. 15–20.
von Arx M., Breitenmoser C., Zimmermann F., Breitenmoser U. (2004). Status and conservation of the eurasian lynx. Kora 19, 1–319.
Warren M. S., Maes D., van Swaay C. A. M., Goffart P., Van Dyck H., Bourn N. A. D., et al. (2021). The decline of butterflies in Europe: Problems, significance, and possible solutions. Proc. Natl. Acad. Sci. 118, e2002551117. doi: 10.1073/pnas.2002551117
Wehr N. H. (2021). Historical range expansion and biological changes of Sus scrofa corresponding to domestication and feralization. Mamm Res. 66, 1–12. doi: 10.1007/s13364-020-00534-7
Williams B. A., Venter O., Allan J. R., Atkinson S. C., Rehbein J. A., Ward M., et al. (2020). Change in terrestrial human footprint drives continued loss of intact ecosystems. One Earth 3, 371–382. doi: 10.1016/j.oneear.2020.08.009
Keywords: Canis aureus, Lynx lynx, species distribution modeling, Europe, Canis lupus, large carnivores, sympatry, range expansion
Citation: Serva D, Iannella M, Cittadino V and Biondi M (2023) A shifting carnivore’s community: habitat modeling suggests increased overlap between the golden jackal and the Eurasian lynx in Europe. Front. Ecol. Evol. 11:1165968. doi: 10.3389/fevo.2023.1165968
Received: 14 February 2023; Accepted: 27 September 2023;
Published: 12 October 2023.
Edited by:
Bryan Lyle Brown, Virginia Tech, United StatesReviewed by:
Miha Krofel, University of Ljubljana, SloveniaAleksandra Uzelac, University of Belgrade, Serbia
Copyright © 2023 Serva, Iannella, Cittadino and Biondi. This is an open-access article distributed under the terms of the Creative Commons Attribution License (CC BY). The use, distribution or reproduction in other forums is permitted, provided the original author(s) and the copyright owner(s) are credited and that the original publication in this journal is cited, in accordance with accepted academic practice. No use, distribution or reproduction is permitted which does not comply with these terms.
*Correspondence: Mattia Iannella, bWF0dGlhLmlhbm5lbGxhQHVuaXZhcS5pdA==