- 1Marine Turtle Research, Ecology, and Conservation Group, Department of Earth, Ocean and Atmospheric Science, Florida State University, Tallahassee, FL, United States
- 2Conservation and Research Coordination Pipa/RN, Fundação Projeto TAMAR, Tibau do Sul, Brazil
- 3Department of Fisheries, Wildlife, and Conservation Sciences, Coastal Oregon Marine Experiment Station, Oregon State University, Newport, OR, United States
- 4Centro Nacional de Pesquisa e Conservação de Tartarugas Marinhas e da Biodiversidade Marinha do Leste do Instituto Chico Mendes de Conservação da Biodiversidade (TAMAR-ICMBio), Vitória, Brazil
- 5ENGEO – Soluções Integradas em Meio Ambiente, Vitória, Brazil
Introduction: An understanding of animal behavior is critical to determine their ecological role and to inform conservation efforts. However, observing hidden behaviors can be challenging, especially for animals that spend most of their time underwater. Animal-borne devices are valuable tools to estimate hidden behavioral states.
Methods: We investigated the fine-scale behavior of internesting hawksbill turtles using the mixed-membership method for movement (M4) which integrated dive variables with spatial components and estimated latent behavioral states.
Results: Five latent behavioral states were identified: 1) pre-nesting, 2) transit, 3) quiescence, and 4) area restricted search within and 5) near the residence of turtles. The last three states associated with a residency period, showed lower activity levels. Notably, when compared to other behaviors the pre-nesting exhibited shallower and remarkably long dives of up to 292 minutes. We noted high fidelity to residence core areas and nesting beaches, within and between nesting seasons, with residence areas decreasing within a season.
Discussion: The latent behaviors identified provide the most detailed breakdown of turtle movement behaviors during the internesting period to date, providing valuable insights into their ecology and behavior. This information can inform marine turtle conservation and management efforts since utilization distributions of individual behavioral states can be used to determine spatially-explicit susceptibility of turtles to various threats based on their behavior. The analyses of utilization distribution revealed a minimal overlap with existing marine protected areas (0.4%), and we show how a new proposal would expand protection to 30%. In short, this study provides valuable guidance for conservation and management of internesting marine turtles at a fine spatiotemporal resolution and can be used to enhance national action plans for endangered species, including the expansion of existing Marine Protected Areas. By flexibly incorporating biologically informative parameters, this approach can be used to study behavior outside of the hawksbill breeding season or even beyond this species.
1 Introduction
Animal behavior is a broad and multifaceted concept, encompassing not only observable behaviors (e.g., feeding, mating, or locomotion), but also subtle and nuanced behaviors, such as thermoregulation, that may be more difficult to detect (Okuyama et al., 2021). Understanding an animal’s behavior can provide important information about its ecological needs and its role within the ecosystem (Crain and Bertness, 2006; Hastings et al., 2007). For example, determining the utilization distribution (UD) of a species, which relates to the proportion of time an individual spends in specific areas can help identify critical habitats and resources, as well as guide conservation efforts (Wilcove et al., 1998; Berger-Tal et al., 2011; Hays et al., 2019; Ferreira et al., 2021). For purposes of this study, we refer to hidden behavioral states as latent behavioral states that are not directly observable but can be inferred through the analysis of the animal’s movements and other data (e.g., environmental data) (Morelle et al., 2017). Furthermore, mapping behavioral states spatially is essential for gaining insights into the ecology and behavior of animals, as well as for the conservation and management of species and their habitats.
Latent behavioral states are often determined from data obtained from animal-borne devices equipped with sensors to collect data on an animal’s movements (Jonsen et al., 2005; Patel et al., 2015; Nathan et al., 2022). However, the data collected by these devices are often acquired at irregular time intervals and have varying levels of positional accuracy, which can pose challenges to accurately assess the movement ecology of an individual (Kuhn et al., 2009; Costa et al., 2010; Patterson et al., 2010; Witt et al., 2010; Dujon et al., 2014). There are statistical approaches for modelling animal movement data acquired at irregular time, such as Continuous-Time Movement Models (CTMMs) (Johnson et al., 2008; Jonsen et al., 2020). However, statistical approaches (such as interpolation or resampling) have been used to regularize time intervals between observations (discrete-time), as well as for quality control of animal movement data that are susceptible to errors (Lopez et al., 2014; Jonsen et al., 2020). State-space models (SSMs) are statistical methods that has been commonly used to account for location error, regularize movement data at a single time interval, in addition to distinguishing between restricted movement and transit behavioral states (i.e., slow and tortuous vs fast and directed) of an animal (Jonsen et al., 2005). Although readily available software for implementing SSMs, such as the Bayesian SSM for animal movement (‘bsam’ R package; Jonsen, 2020), can make robust inferences about the behavioral states of an animal, it is limited to a single data stream (i.e., horizontal movements) that is typically interpreted with a binary output (Jonsen et al., 2005). Newer SSMs (e.g., ‘aniMotum’ R package; Jonsen, 2023) estimate a continuous behavioral state index from 0 to 1, representing a range of behaviors rather than a simple binary output (Jonsen et al., 2023).
Hidden Markov Models (HMMs) are another popular tools used to identify behavioral states, but estimate multiple finite states (unlike SSMs) and can integrate more than one data stream (Langrock et al., 2012; Patterson et al., 2017; Whoriskey et al., 2017). These estimated states can be used to infer a range of possible behaviors (e.g., foraging, mating, and migration) from a variety of taxa, including marine mammals, birds, and reptiles (Dean et al., 2013; DeRuiter et al., 2017). Changepoint analysis (CPA) detects changes in the mean and/or variance of time-series data, which can be associated with behavioral states, by repeatedly dividing the data into smaller segments until a changepoint is identified (Patel et al., 2015). Although CPA does not explicitly model the underlying states of a system like SSMs, it is a useful tool for detecting changes in animal behavior over time. While these approaches might provide a more nuanced picture of an animal’s behavior, they do not conceptually characterize animal trajectories as a set of track segments that each are comprised of multiple behaviors (Cullen et al., 2022). Translating an animal’s multi-faceted activity into distinct latent behavioral states is challenging, especially for free-ranging marine species due to their high mobility and wide range of behaviors in complex marine environments (Nathan et al., 2008; Hays et al., 2016).
As an especially complex case study, marine turtles remain submerged for long periods and may be difficult to observe (particularly at short time intervals throughout the course of a day), making it a challenge to accurately distinguish among multiple behavioral states (Hochscheid, 2014). Nesting female marine turtles are the most widely studied demographic group of marine turtles, as they can be easily observed while laying eggs (Mazaris et al., 2017). This accessibility has resulted in numerous studies exploring the behavior of post-nesting females, by using data from animal-borne devices deployed on females after they lay their eggs (Marcovaldi et al., 2012; Hays and Hawkes, 2018; Santos et al., 2021a). Although most studies have been focused on post-nesting migrations (e.g., Horrocks et al., 2001; Cuevas et al., 2008; Baudouin et al., 2015; Jim et al., 2022) some have focused on the internesting period, which refers to the interval between successive clutches within a nesting season (Sato et al., 1998; Hays et al., 2002; Hill et al., 2017; Santos et al., 2021b).
While information on the internesting behavior of marine turtles obtained from satellite tags provide spatially explicit data (Iverson et al., 2016; Hart et al., 2019; Santos et al., 2021a), the use of data-loggers such as time-depth recorders (TDRs) (Walcott et al., 2013) and cameras alone (Thomson and Heithaus, 2014) do not. The lack of integration of multiple data streams in such studies (i.e., spatial and dive profiles data) has hindered the understanding of marine turtle behavior during interesting intervals. However, with the increasing use of modern technology (e.g., GPS tags, acoustic sensors, accelerometers, satellite imagery) and the growing interest in the analysis of Big Data, it is now possible to collect and analyze large amounts of data to gain new insights into the behavior and ecology of marine turtles (Nathan et al., 2022). To better understand the internesting behavior of marine turtles, it is necessary to integrate multiple data streams, as well as to use clustering algorithms and machine learning to identify different behavior patterns (Cullen et al., 2022) based on the combination of horizontal and vertical movement patterns within the water column.
To address an existing knowledge gap, this study estimated the fine-scale behavioral states of internesting hawksbill turtles (Eretmochelys imbricata) via a non-parametric Bayesian mixed-membership method for movement (M4) (Cullen et al., 2022). We used internesting hawksbill turtles off the coast of northeastern Brazil as a complex case study to: 1) estimate behavioral states within the internesting interval, 2) determine the fine-scale spatial and temporal distribution of turtles, including site fidelity of turtles to nesting sites and residence areas, and 3) estimate activity budgets within the internesting interval. All objectives may be used to inform conservation and management efforts along one of the most important nesting areas for hawksbill turtles in the South Atlantic (Marcovaldi et al., 2007; Santos et al., 2013).
2 Materials and methods
2.1 Study site and species
This study was conducted on the southern coastline of Rio Grande do Norte, Brazil (Figure 1A), an important nesting area for hawksbill turtles in the South Atlantic (Santos et al., 2013). Nesting beaches in four municipalities (Figure 1C), Parnamirim (−5.91120° S, −35.15577° W), Senador Georgino Avelino (−6.15979° S, −35.09810° W), Tibau do Sul (−6.25713° S, −35.03790° W), and Baia Formosa (−6.32871° S, −35.03228° W) were patrolled by the non-profit organization Fundação Projeto Tamar nightly to tag nesting females. Patrols occurred throughout the nesting season, which extends from November to May, with most nests being laid between February and March (Santos et al., 2013), over four nesting seasons, specifically during the years 2014/2015, 2015/2016, 2017/2018, and 2018/2019. All turtles were checked for flipper tags (number 681 National Band and Tag Company). When tags were absent, they were applied to both front flippers as per Santos et al. (2013). The Brazilian Biodiversity authorization and information system (SISBio) granted a data collection license (42760) in compliance with regulations for the proper care of animals. In addition, we installed transmitters following the guidelines of the Brazilian Institute of Environment and Renewable Natural Resources (IBAMA), through the Authorizations for Capture, Collection and Transport of Biological Material (Abio) numbers 557/2014 and 900/2018.
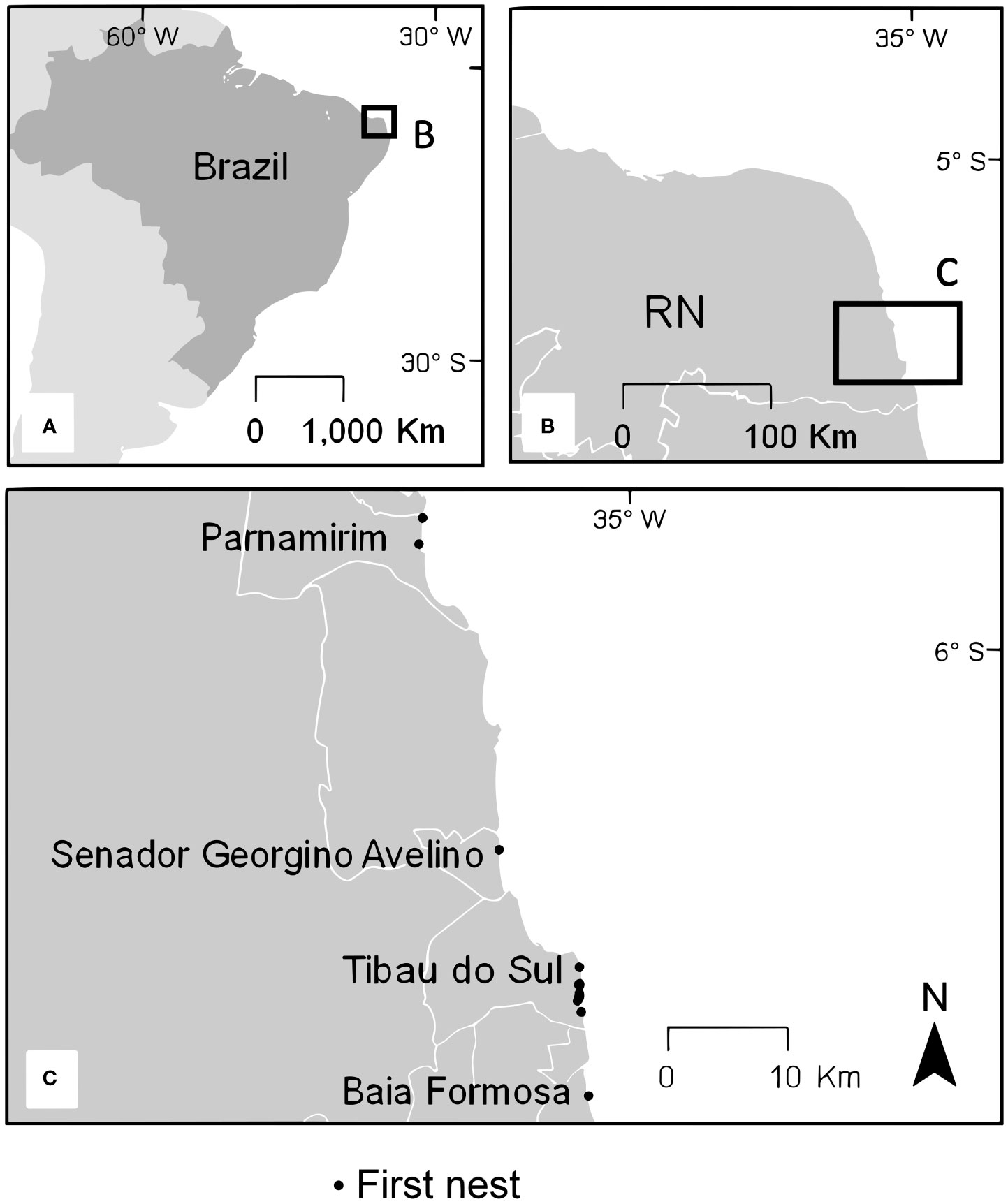
Figure 1 (A) Map of study area in Brazil. Inset boxes show maps zoomed in at: (B) the coastline of the state of Rio Grande do Norte (RN); (C) the four municipalities at the southern coastline of RN with black points showing the locations of the first nest event from each nesting season of the 10 tracked hawksbill turtles, including two turtles tracked in consecutive nesting seasons.
2.2 Transmitter deployment and tag configuration
Ten individual gravid hawksbill turtles were fitted with platform transmitter terminals (PTTs), hereafter referred to as “tags”, from the 2014/15 to 2018/19 nesting seasons. Two individuals were tracked in two different nesting seasons (Table 1). For simplicity, we use the number of the first tag to represent each individual, adding letters A for the first season and B for the second season. All turtles were satellite tagged with Fastloc GPS tags (SPLASH10-F-296A or SPLASH10-F-334; Wildlife Computers, Redmond, WA, USA). The data were obtained through direct download from the tags during turtle recaptures, which provided complete access to all Fastloc GPS and dive data stored by the device. This also minimized gaps that are common with data obtained from satellites, which is particularly problematic at low latitude locations (such as our study site) where Argos has lower coverage (CLS, 2016). Tags were attached as per Santos et al. (2021a; 2021b). In summary, turtles were restrained after nesting, the first vertebral scute of the carapace was sanded and cleaned with isopropyl alcohol, and the tag was attached with anchoring adhesive and painted with antifouling paint (see Santos et al., 2021a for the details). All turtles, except for one turtle (tag 146297), were restrained in situ where tag attachment took between 1.5 and 3 hours. Turtle 146297 was relocated from its capture site (Senador Georgino Avelino municipality), approximately 27 kilometers (km) northward to Barreira do Inferno (Parnamirim municipality; Figure 1B) where it was released. Relocation occurred in this particular case because the turtle was sighted after sunrise, and it was too hot to conduct installation on the beach. The Fastloc GPS sampling interval ranged from 15 to 60 minutes (min) and transmission days were set to daily. During the first nesting season (2014/2015), sampling interval was at an hourly interval. Subsequently, in the following seasons (2015/2016 to 2018/2019), the sampling interval was intensified to four fixes per hour, this finer scale resolution was used to allow a more detailed analysis. Dives were defined as submergences below −2 meters (m) and longer than 40 seconds (s), at 0.5 m of vertical resolution. Defining the dive threshold as −2 m was arbitrary (Hays et al., 2000), but reasonable considering that turtles in our study were adult females and the surface layer is commonly defined as between 1 and 2 m deep for adult hawksbill turtles (Gaos et al., 2012b; Walcott et al., 2013; Hill et al., 2017). A dry/wet sensor on the tags detected when turtles crawled up the beach, providing a binary output that reports “1” when the tag was hauled out on land and “0” if submerged underwater. Two different settings for haul out cycles were used: during the first nesting season (2014/2015) haul outs were classified when the sensor was dry for 20 min, whereas for the remaining seasons (2015/2016 to 2018/2019) a 5 min threshold was used. This change was made to detect nesting attempts more sensitively. Both settings exited haul out mode after the sensor was wet for 30 s. As long as hawksbill turtles took at least 40 min to successfully nest within the study site (AJBS personal observation), both settings were able to record nesting events, as well as false crawls (i.e., unsuccessful nesting attempt) in which the turtle spent at least 5–20 min on land.
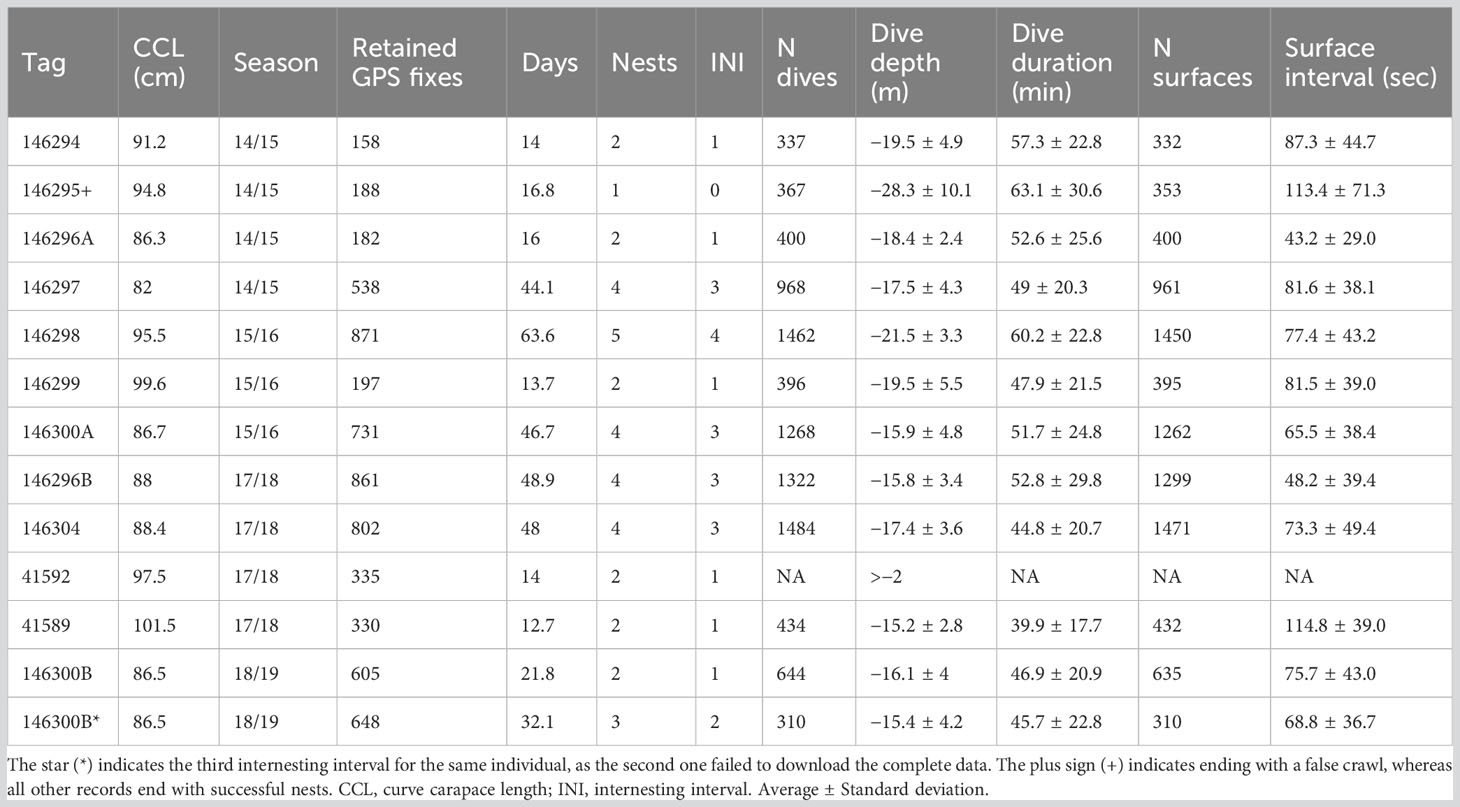
Table 1 Summary of the raw downloaded data from 12 tags deployed on ten nesting hawksbill turtles in Rio Grande do Norte, Brazil, including two tracked in two nesting seasons (146296A/B and 146300A/B).
2.3 Data filtering
The data downloaded directly from each tag were uploaded to the Wildlife Computers data portal for processing, resulting in 7,262 Fastloc GPS fixes. For comparative purposes, we also accessed the satellite transmitted Fastloc GPS fixes from the data portal (i.e., without the downloaded files) for the same period, resulting in 1,645 fixes. We used data with four satellites or more, removed duplicates, and deleted fixes in which latitude and longitude were blank, as well as those with residual error value greater than 30 (unitless) from the merged dataset (Witt et al., 2010; Shimada et al., 2012). We visually inspected each individual track and removed unlikely fixes such as those that were further inland or beyond the continental shelf. For each tag, the dataset started with the first nesting event (Figures 1B and 2) and ended with the last download made from the tag at the beach, which occurred after successful nesting. The timestamp of the combined dataset was transformed to local time (−3 UTC). Two turtles (146295 and 146303) had tags deployed after a false crawl, thus the interval between the first false crawl and the actual nest, respectively 2.1 and 1.1 days, for these turtles were not considered. We also removed fixes from the relocated turtle (tag 146297) which corresponded to its first internesting interval (17 days), to avoid including non-natural behavior. The second download for tag 41591 failed, resulting in incomplete data, with a gap of five days (from 15 to 20 February 2019); therefore, the whole second internesting interval for this turtle was removed (between Feb 5–20, 2019). The final number of fixes retained for our analysis was 6,858 (94.4% of downloaded data).
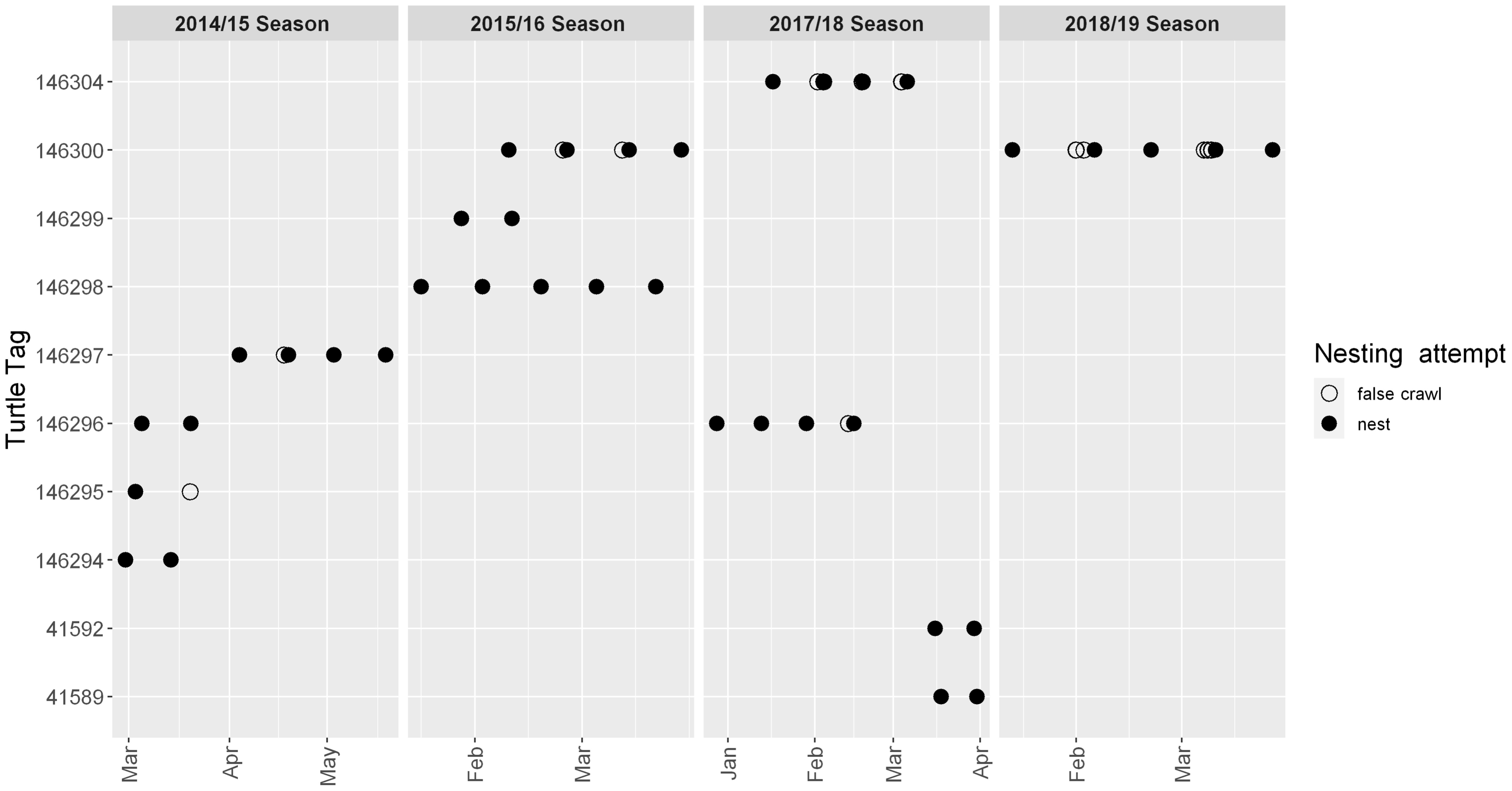
Figure 2 Nests and false crawls for ten hawksbill turtles tracked in Rio Grande do Norte, Brazil, including two turtles tracked in two nesting seasons (146300 and 146296). The second internesting interval for 146300 during the 2018/2019 season was not included in the analyses since the downloaded data were incomplete. (See * in Table 1).
2.4 Nesting attempts
Nesting attempts were classified based on the emergences indicated by the tag as hauled out or observed by beach patrols. For nesting attempts that were observed by beach patrols but not recorded by haul out sensor, the closest timestamp of the Fastloc GPS position on land was classified as a nest or false crawl. The subsequent emergences that occurred in the same night or within the five following nights were classified as a false crawl and the last emergence was classified as a nest (successful nesting attempt). This procedure was based on the internesting interval for hawksbill turtles in the region that ranges from 12 to 20 days (Santos et al., 2013), therefore intervals shorter than 12 days were assumed to represent unsuccessful nesting attempts.
2.5 Regularization of in-water tracks
To obtain uniform time steps and comparable in-water tracks for all individuals (i.e., given the variation in FastGPS sampling intervals among tags), we used a continuous-time SSM with a “move persistence” process from the ‘foieGras’ package (Jonsen et al., 2020) in R (R Core Team, 2022) to generate tracks at a regularized time interval. This model accounts for Fastloc GPS location error and was applied to estimate positions at 1-hour time intervals for in-water locations. We only included in-water tracks during this step and excluded nesting attempts as this might have resulted in nesting positions that fell in-water or that were too brief (i.e., false crawls) to correspond to 1-hour intervals.
2.6 Latent behavioral state estimation
To determine the fine-scale behavior of internesting hawksbill turtles, we used a non-parametric Bayesian framework (mixed-membership method for movement; M4) that first separates individual tracks into segments (via reversible-jump Markov chain Monte Carlo; RJMCMC) and further clusters the segments into latent behavioral states (via non-parametric Latent Dirichlet Allocation) (Cullen et al., 2022). The model requires that the chosen variables are first discretized into a set of bins since a categorical distribution is used. Six variables were considered to be informative for characterizing the fine-scale behavior of internesting hawksbill turtles and were selected for our model: 1) activity (i.e., nesting attempts or in-water), 2) horizontal spatial position within the internesting home range, 3) step length, 4) average surface interval, 5) average dive duration, and 6) average dive depth. Activity and home range position were categorical variables, whereas the other variables were continuous and needed to be discretized into bins. Such discretization was defined by plotting the density distribution of the variables and splitting them into classes that potentially characterize the shape of the distributions (Cullen et al., 2022) (see Supplementary Methods for specific bins).
To determine the type of activity that the turtle was engaged in, we classified the combined tracks into one of three classes: in-water data, false crawl, and successful nest (Supplementary Figure 1A; Table 2). We used Autocorrelated Kernel Density Estimation (AKDE) from the ctmmweb interactive Shiny application (Dong et al., 2018) to classify the GPS data into different classes of position within internesting home range: within the inner core (AKDE 25%), first ring around the AKDE, second ring, third ring, fourth ring, and within the nesting buffer (Supplementary Figure 1B; Table 2). For more details, please refer to the Supplementary Methods.
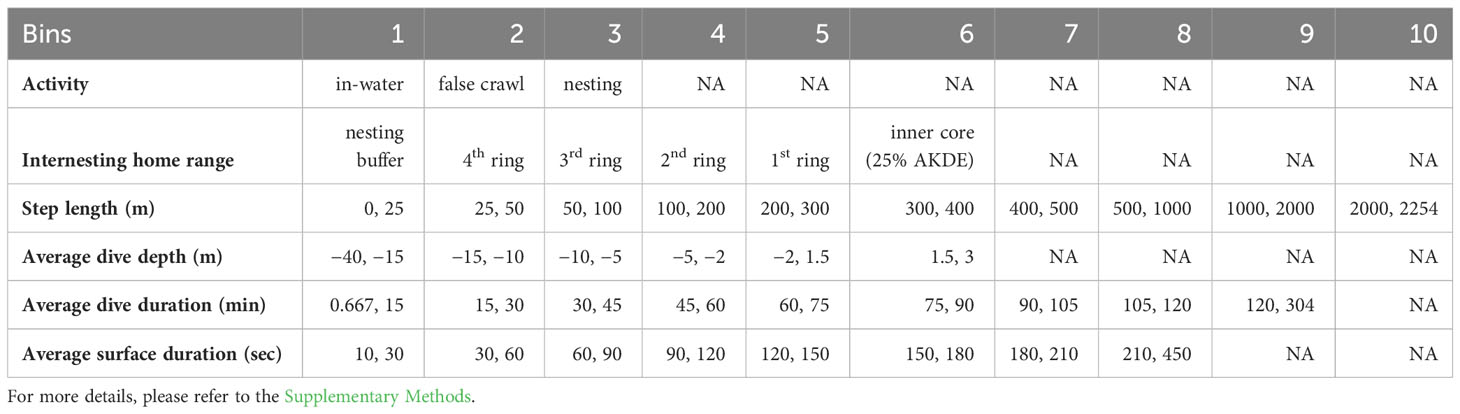
Table 2 Data stream discretization (i.e., binning) for the six variables considered for mixed-membership method for movement (M4).
Step lengths were calculated as the distance (in meters) between subsequent in-water regularized locations and were further discretized into ten bins (Supplementary Figure 1C; Table 2). Dive variables (average surface interval (s), dive duration (min) and dive depth (m)) were also extracted from the in-water dataset. Dive depth was discretized into six bins (Supplementary Figure 1D; Table 2). Average dive duration was discretized into nine bins (Supplementary Figure 1E; Table 2). Average surface duration was binned in eight bins (Supplementary Figure 1F; Table 2). For more details, please refer to the Supplementary Methods.
For each individual turtle, tracks (i.e., the whole set of data for a given nesting season) were split into sub-tracks (i.e., parts of a track) to represent internesting intervals, which started with the first in-water position immediately after a successful nest until the subsequent nesting attempt, regardless of whether it was successful or not. The next sub-track started with the first in-water position after the previous nesting attempt (false crawl) and continued until the next successful nesting event, including cases where multiple false crawls occurred in between. Additionally, there was a possibility of false crawl/false crawl sub-tracks, where the turtle had multiple false crawls consecutively without a successful nesting event. However, due to their brief nature and representation by a single point, these cases were considered within the false crawl/nest category for the purpose of the analysis. So, three types of sub-tracks were possible: nest/nest (a complete internesting interval in which false crawls were absent), nest/false crawl (a potential internesting interval, but for some reason the turtle did not successfully nest), and false crawl/nest (the female returned in the same night or following nights to successfully nest). The sum of the nest/false crawl with its related false crawl/nest sub-tracks were made for the complete internesting interval calculations. This split was conducted to help the model identify breakpoints within the tracks, as false crawls are often very brief and often represented by a single point.
Following data stream discretization (i.e., binning), the segmentation stage of the M4 model estimated breakpoints within each predefined internesting or false crawl period per sub-track to define relatively homogeneous segments based on the six data streams (Supplementary Figure 3) (Cullen et al., 2022). We used 800,000 RJMCMC iterations with a burn-in of 400,000 iterations and assessed model convergence by inspecting trace plots of the log marginal likelihood (Supplementary Figure 4B). Since different sets of breakpoints are estimated for each iteration of the model, the breakpoints that defined the maximum a posteriori (i.e., best-fitting model) of the samples after the burn-in period were used to define track segments within sub-tracks. To estimate the latent behavioral states from track segments, the mixed-membership model of M4 was fitted using 10,000 MCMC iterations, with 5,000 iterations as burn-in and a maximum number of nine potential clusters (i.e., behavioral states). The dominant latent behavioral states (i.e., those that corresponded to the majority of the data within clusters, up to 90% of the data) (Cullen et al., 2022) were used to determine the likely number of states and characterize the track segments. Since behavioral state estimates were derived from a mixed-membership model, the results were reported as proportions of each state per segment. We attributed each segment to its dominant behavior, depending on which one had the highest estimated proportion. We calculated the activity budget as the duration (in days) of each behavioral state across all tracks. Summary statistics are given as average ± standard deviation (range; sample size). Activity levels per behavioral state (i.e., low vs high) was evaluated based on the step length (the smaller, the less activity) and dive duration (the longest, the less activity) (Hochscheid, 2014).
2.7 State-specific utilization distributions
The utilization distribution (UD) for each identified behavioral state and individual turtle sub-tracks was calculated using ArcGIS Pro (ESRI, 2019). Consecutive GPS fixes were converted to line segments, where each segment was assigned with the behavioral state of the first/origin position of the pair. To complete the path, the last point was duplicated, and a final line segment was created with its behavior represented by the duplicated point. This procedure was done for paths represented in a grid, otherwise only spread points would be extracted to the grid. We assigned a weight attribute to each individual turtle to account for differences in sample size and number of internesting intervals. The weight attribute was calculated as the value 1 divided by the total number of fixes for each turtle. Turtles tracked in two seasons had their two tracks combined. The sum of the weight attribute was applied to 250 m × 250 m grid cells for all behaviors (Supplementary Figure 5) and a geometric classification with 30 classes was applied to allow comparison of the UDs for all behaviors at the same scale.
2.8 Site fidelity to nesting beach and residence
To determine nest site fidelity within and across two nesting seasons, we used the distance (in km) between the two furthest nesting attempts per individual for each season. For turtles with more than one internesting interval, we combined the behavioral state estimates for each interval related to residence period (i.e., low activity) to delineate each residence core as the 50% UD (Hays et al., 2021; Maurer et al., 2022) using AKDE from the ctmmweb interactive Shiny application (Dong et al., 2018). We then measured the residence areas (in km2) for each internesting interval and then calculated the overlap between successive residence areas using ArcGIS Pro (ESRI, 2019). Specifically, we calculated the overlap as the intersecting area between the two residence areas for each pair of subsequent internesting intervals and reported how much (percentage) the most recent residence overlapped with the previous one. Finally, we determined the distance between successive residences across internesting intervals by measuring the distance between centroids for each 50% UD.
3 Results
3.1 Data summary
Thirty-four sub-tracks corresponding to 24 complete internesting intervals (average 15.5 ± 1.8 days; Figure 2) were analyzed; 15 were complete nest to nest sub-tracks, 10 sub-tracks consisted of nest to false crawl, and 9 sub-tracks started with a false crawl and finished with a nest (Figure 2). Dive data were available for 11 tags and indicated that the turtles spent 97.7% of the recorded time submerged, with 9,392 dives (average dive duration of 51.3 ± 24.5 min and average dive depth −18.4 ± 3.7 m) and 9,300 surfaces (average surface interval of 73 ± 46 s) (Table 3). One individual (tag 41592) remained shallower than the −2 m threshold for the entire internesting interval and was only recorded diving when it began its post-nesting migration.
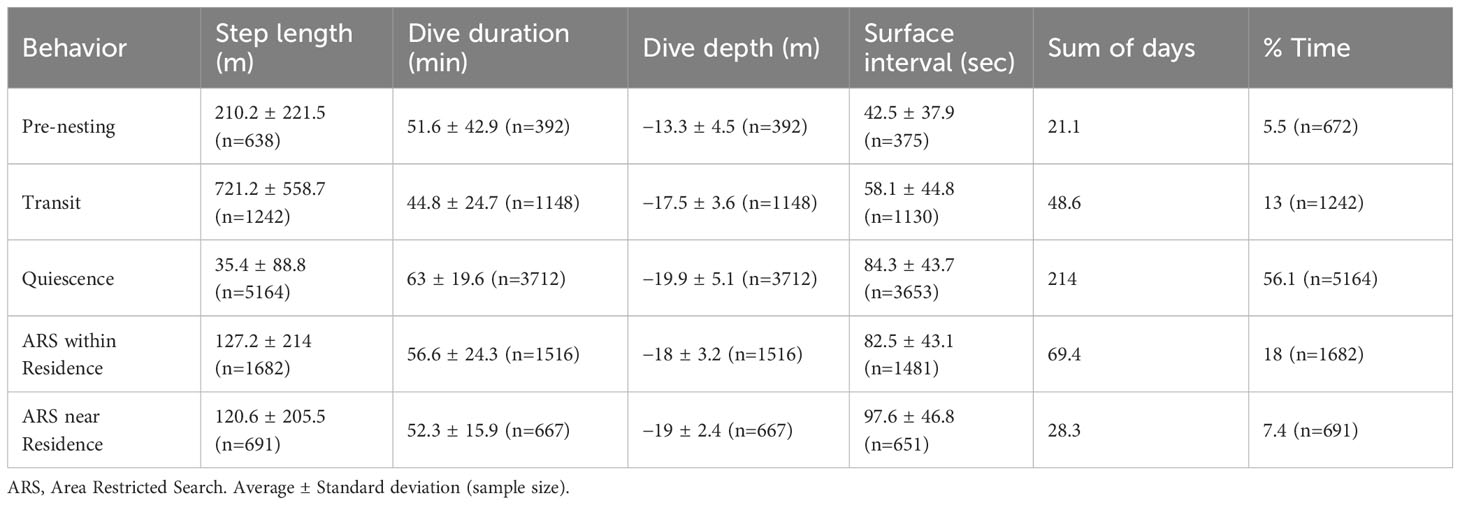
Table 3 Summary of the five dominant behaviors of interesting hawksbill turtles in Rio Grande do Norte, Brazil and their activity budgets aggregated for the 34 sub-tracks.
3.2 Latent behavioral states
One to eight breakpoints were estimated for each of the 34 sub-tracks by the segmentation model (Supplementary Figures 3, 4A). From these estimated segments within sub-tracks, the clustering model suggested that five behavioral states were likely, which comprised 94% of all observations on average (Supplementary Figure 6). The state-dependent distributions of the six variables used in the model were visualized to evaluate the biological meaning of the estimated states (Figure 3). Based on the state-dependent distributions, the five behaviors identified were labeled as “pre-nesting”, “transit”, “quiescence” (i.e., resting), “area restricted search (ARS) within the residence”, and “ARS near the residence” (i.e., within the first buffer ring around the residence (Figures 3–5; Table 3). The last three behaviors (quiescence and both ARS states) showed lower activity levels and represented a residency period (Figures 5C–E). The majority of the UD area from all five behaviors combined (Figure 5F) occurred shallower than the −20 m isobath.
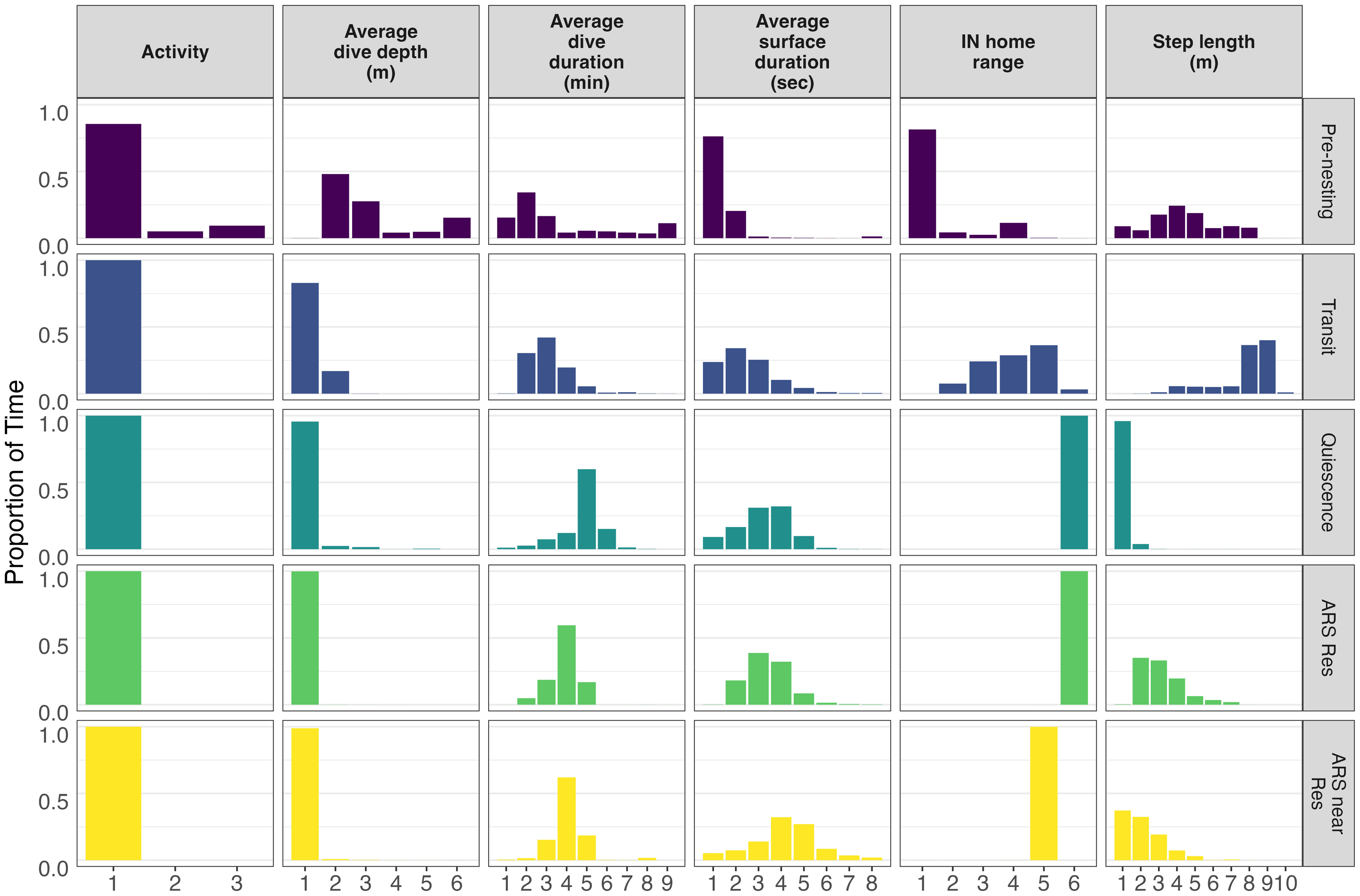
Figure 3 Five clusters of behavioral states that corresponded to 94% of the data showing the proportion distributions for each variable. The bins for activity are numbered as follows: 1 = in-water, 2 = false crawl, and 3 = nesting. The bins for average dive depth (m) are numbered as follows: 1 = [−40, −15), 2 = (−15, −10], 3 = (−10, −5], 4 = (−5, −2], 5 = (−2, 1.5], and 6 = (1.5, 3]. The bins for average dive duration (min) are numbered as follows: 1 = [0.667, 15), 2 = [15, 30), 3 = [30, 45), 4 = [45, 60), 5 = [60, 75), 6 = [75, 90), 7 = [90, 105), 8 = [105, 120), and 9 = [120, 304]. The bins for surface duration (sec) are numbered as follows: 1 = [10, 30), 2 = [30, 60), 3 = [60, 90), 4 = [90, 120), 5 = [120, 150), 6 = [150, 180), 7 = [180, 210), and 8 = [210, 450). The bins for internesting home range are numbered as follows: 1 = within nesting buffer, 2 = fourth ring around the in-water inner core (25% AKDE), 3 = third ring, 4 = second ring, 5 = first ring, and 6 = within the inner core (25% AKDE). The bins for step length (m) are numbered as follows: 1 = [0, 25), 2 = [25, 50), 3 = [50, 100), 4 = [100, 200), 5 = [200, 300), 6 = [300, 400), 7 = [400, 500), 8 = [500, 1000), 9 = [1000, 2000), and 10 = [2000, 2254]. Abbreviations used in the figure include: ARS Res, Area Restricted Search within residence; ARS near Res, Area Restricted Search within near residence.
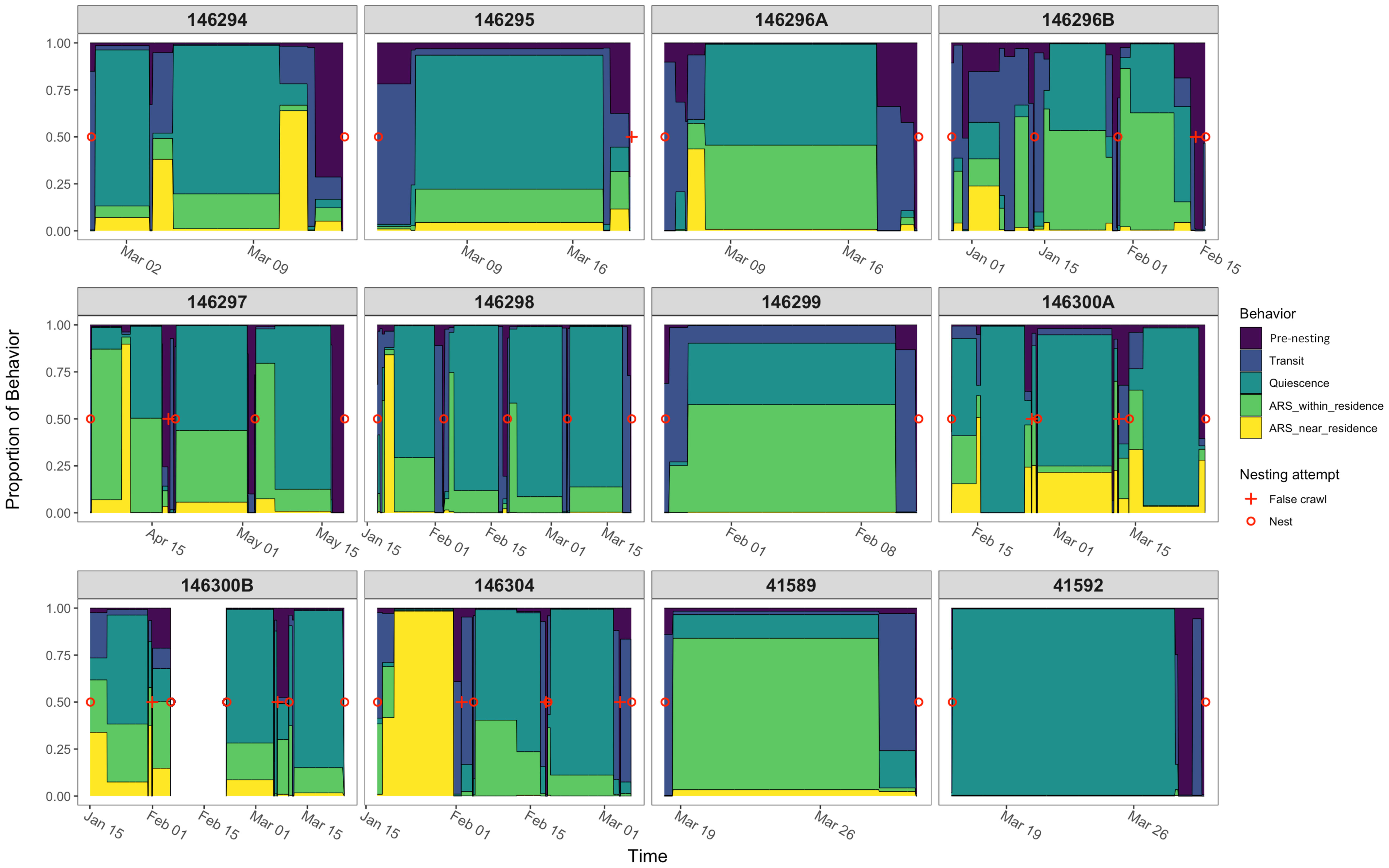
Figure 4 Behavioral proportions over time for ten internesting hawksbill turtles, including two individuals tracked in two nesting seasons (146296A/B and 146300A/B). Red plus signs indicate a false crawl and red open circles indicate a nest.
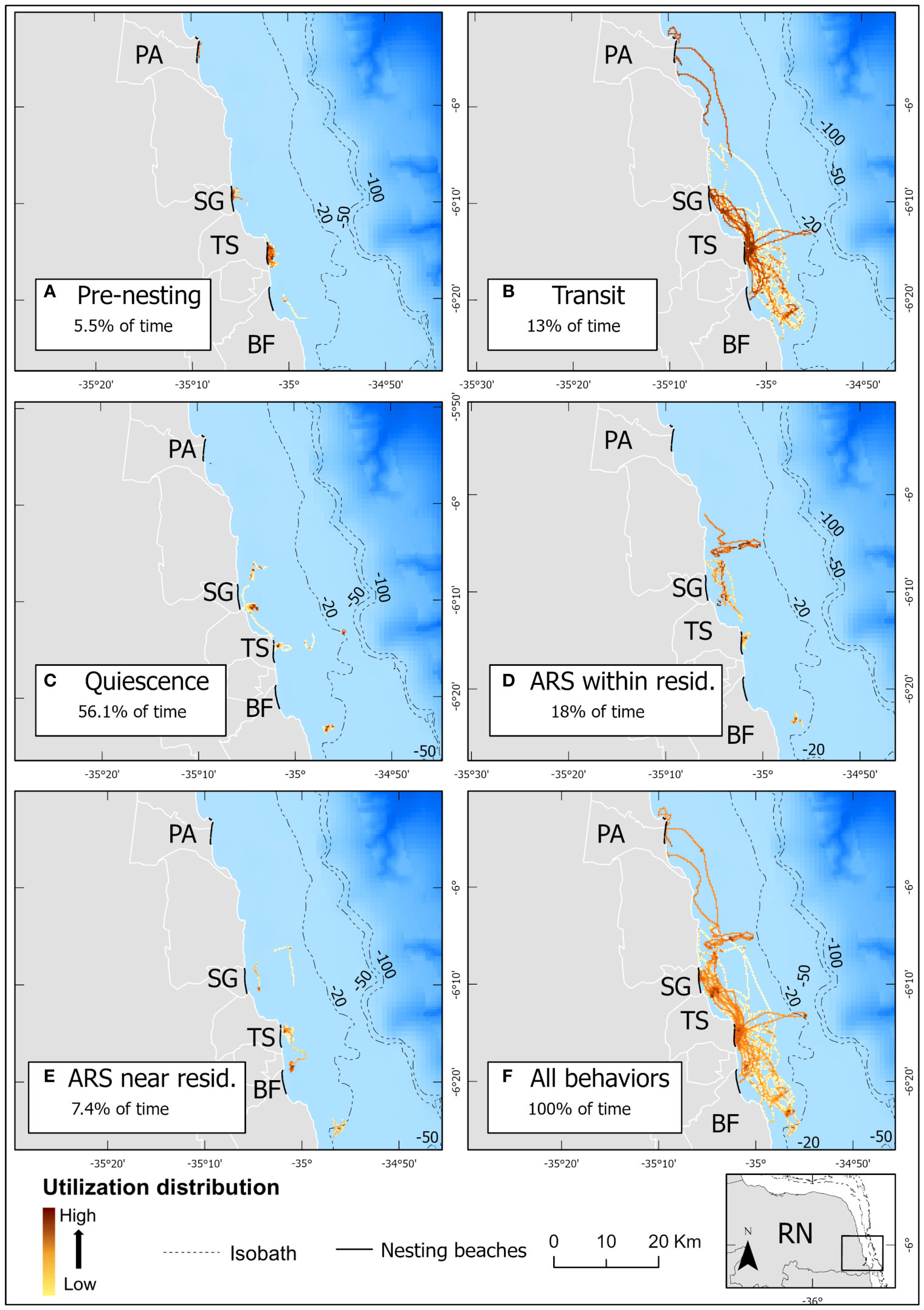
Figure 5 Utilization distribution within the internesting period of ten internesting hawksbill turtles in the south coast of Rio Grande do Norte, Brazil, calculated over 250 m x 250 m grid cells by behavior: (A) pre-nesting, (B) transit, (C) quiescence, (D) Area Restricted Search (ARS) within residence, (E) ARS near residence, and (F) all the five behaviors grouped. Acronyms for the relevant municipalities: PA, Parnamirim; SG, Senador Georgino Avelino; TS, Tibau do Sul; BF, Baia Formosa. Utilization distribution indicates the sum of the weight attribute across turtles.
3.2.1 Pre-nesting
The pre-nesting behavior (Figure 5A) was the only state that included on-land records, comprising 5.5% of the internesting intervals (Table 3) and lasting 22.7 hours on average (Table 4) per internesting interval. In-water records comprised most of the pre-nesting behavior estimates (87.3%), mainly concentrated within 1.5 km from the nesting beach (Figure 3). The remaining on-land records corresponded to successful nests (9.1%) and false crawls (3.6%). Despite pre-nesting being the shortest duration behavioral state, there were high levels of spatial overlap of turtles within nesting beach vicinity indicate a high probability of use during this behavioral state (Figure 5A). For some individuals, the pre-nesting behavior was very brief (1.3–4 hours; tags 41589, 146296A, and 146299) and most data were restricted to on-land records, so there were no dive data (Supplementary Figure 7). For those with dive data available, pre-nesting behavior had the shallowest average dive depths (Table 3). Despite having a short average dive duration of 51.6 min (Table 3), it also had the longest dives of all behaviors (Figure 3), reaching up to 292 minutes. Step lengths and dive durations were the most spread across bins, evidencing a large spectrum of activity, including low and high activities (Figure 3). Surface duration was the shortest among behavioral states (Table 3 and Figure 3). Turtles that exhibited a false crawl took an average of 2.1 ± 1.3 days (0.4–5 days: n = 9) with up to four nesting attempts before successfully nesting (Figure 2). During those false crawl/nest sub-tracks, turtles usually returned to pre-nesting behavior (45.3% of the time spent), but also exhibited other behaviors such as ARS within residence (26%), transit (24.8%), and quiescence (3.8%).
3.2.2 Transit
Transit behavior made up 13% of internesting intervals and averaged 2.3 days per interval (see Tables 3 and 4). High UD probability in this state occurred near nesting areas due to the convergence of individuals from various residence areas (Figure 5B). Transit behavior had the shortest average dive duration and largest average step length, evidencing the behavior with the highest activity level (Figure 3). The position in internesting home range distributed across four buffer rings, indicated the movement between residence and nesting sites (see Table 3 and Figure 4). One individual (146296B) exhibited inflated transit duration (11.8 days – see Table 4) in her first internesting interval (Figure 4; Supplementary Figure 8B). Transit behavior was further divided into “to residence” and “to nesting beach” for analysis (see Figure 6). Turtle 146300A/B was excluded due to lack of clear detection. On average, transit “to residence” took 20.8 hours (Table 4) with 84.2% of arrivals during daylight (Supplementary Table 2). Regarding transit “to nesting beach” (Table 4), turtles spent an average of 24.1 hours, with 73.7% of departures occurring after sundown (Supplementary Table 2).
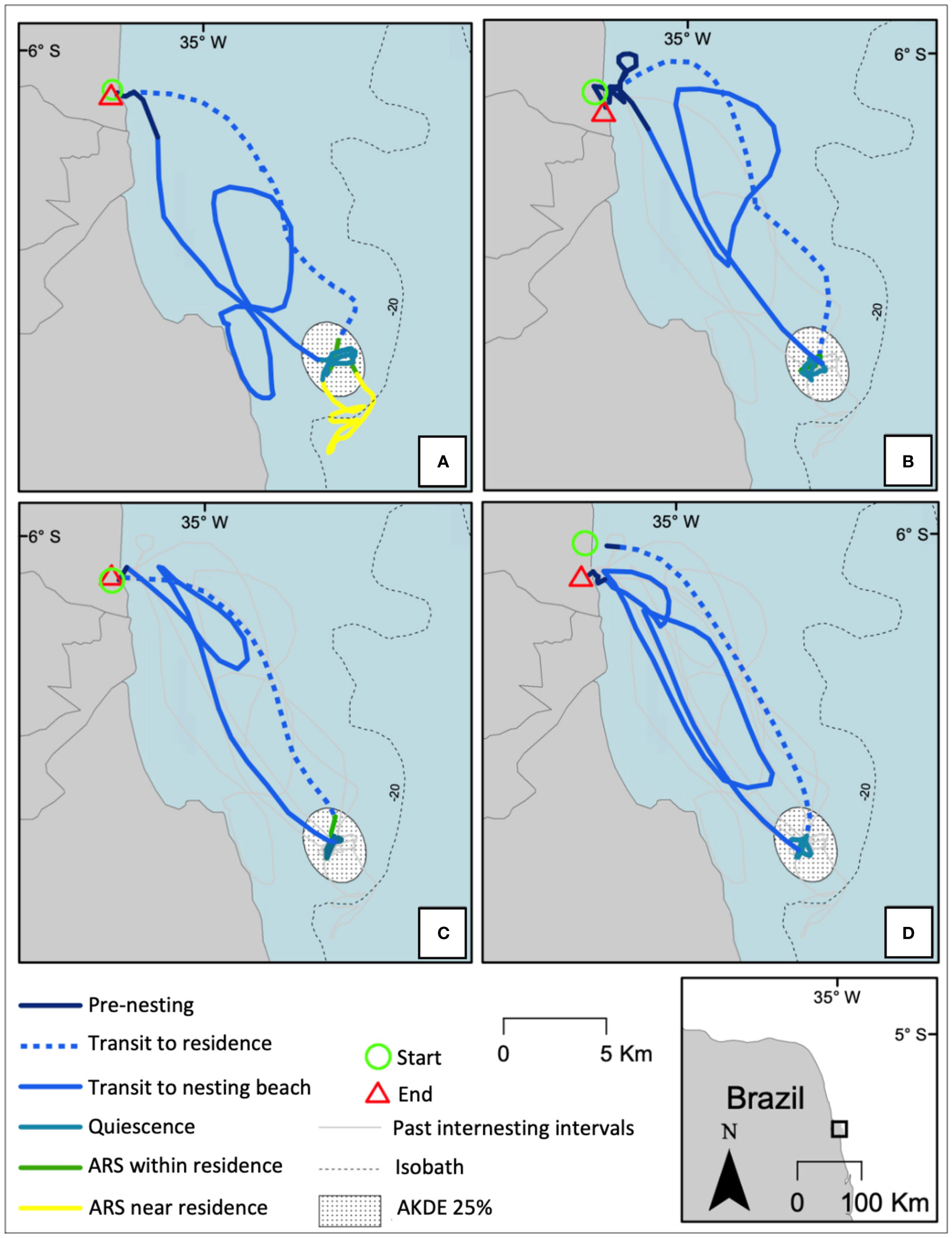
Figure 6 Tracks segmented by behavior for turtle 146298 for four internesting intervals. Panel (A): first internesting interval. Panel (B): second internesting interval. Panel (C): third internesting interval. Panel (D): fourth internesting interval. Line colors and types represent different behavioral states. After a successful nesting at the green circle “Start”, the track starts in-water in “transit to residence” (broken blue line), and further entered in residency phase, which encompass the three behaviors “Area Restricted Search (ARS) within residence” (green unbroken line), “ARS near residence” (unbroken yellow line), and “quiescence” (unbroken blue green line). After the residency phase, turtle returned towards the nesting vicinity in “transit to nesting beach” (unbroken blue line), and finally switches to “pre-nesting” (unbroken dark blue line), ending with a successful nesting at the red triangle “End”. The residency phase of first internesting included the three behavioral states “ARS near residence”, “ARS within residence” and “quiescence” (A). The residency phase during the second and third internesting intervals included “ARS within residence” and “quiescence” behaviors (B) and (C). The residency phase during last internesting interval was limited to “quiescence” (D).
3.2.3 Quiescence
Quiescence was the most frequent behavior recorded, composing 56.1% of internesting intervals (Table 3), with an average duration of 11.3 days per internesting period (Table 4). This behavior had limited movement, longer dives, and occurred in the inner core area (25% UD), being the behavior with the lowest activity level (Figure 3). With the shortest step length (Table 3) and the longest overall duration (Tables 3 and 4), this behavior presented the highest UD probability within single grid cells and scattered distribution across the study area (Figure 5C). Its average dive duration was the longest of all states (Table 3). Quiescence did not appear as a dominant behavior in some internesting intervals (Figure 4) but appeared subsequently in multiple internesting intervals, suggesting a trend of reduced movement over time. Except for turtle tag 146296B, which had higher movement as the internesting interval progressed, tracked turtles typically increased their proportion of time in quiescence over time (Figure 4).
3.2.4 Area Restricted Search within the residence
The ARS within the residence behavior comprised 18% of internesting intervals (Table 3) and lasted an average of 5.8 days per interval (Table 4). This behavior was exhibited within the inner core area (25% UD), but with relatively larger step lengths than quiescence, but still considered a low activity level when compared with other behaviors (Figure 3). High use cells were more spread out compared to quiescence, including paths with less intense UD (Figure 5D). While some turtles presented ARS within residence as dominant behavior during their residency phase (Figure 4, see tags 41589 and 146299), this behavior appeared as a small proportion associated to the dominant quiescence for other turtles (Figure 4, see tags 146294, 146298 and 146304).
3.2.5 Area Restricted Search near the residence
ARS near residence behavior accounted for 7.4% of internesting intervals (Table 3) and had an average duration of 3.6 days per interval (Table 4). This behavior had step lengths and dive duration proportions similar to quiescence and ARS within residence combined, but outside of the inner core area (25% UD) and was considered a low activity level (Figure 3). The UD was similar to both quiescence and ARS within residence, with high use within single cells, but also spread out in less intense use paths (Figure 5E). Only one turtle (tag 146304) showed this behavior for most of the residency period within an internesting interval, but only during the first internesting interval (Figure 4). This behavior had short step lengths similar to ARS within residence and a high proportion of the slowest step lengths, similar to quiescence (Figure 3).
3.3 Site fidelity to nesting beach and residence
The average distance between the two most extreme nesting attempts for the same turtle within a nesting season was 3.1 ± 6 km (range 0.3–22 km; n = 12). Notably, both turtles tracked over two nesting seasons returned to the same 3 km beach stretch in Tibau do Sul municipality, indicating strong site fidelity. All the turtles tracked for more than one internesting interval (n = 5) showed overlap in their residence core areas (50% UD) within and between nesting seasons (Figure 7), with an average residence area of 20.9 km2, but 75% of the residence areas were < 0.4 km2 (Supplementary Table 3). The average overlap of residence areas was 60.2% (Supplementary Table 3). However, turtle 146304 only showed overlap in its last two internesting intervals (Figure 7). The use of a larger area during the first internesting interval was observed in turtles 146304, 146300B, 146297, and 146298 (Supplementary Table 3; Figure 6; Supplementary Figure 9). In contrast, turtle 146296A/B was observed to have high movement during residency period in all internesting intervals, which inflated the 50% UDs. If we exclude this atypical turtle 146296A/B for residence area measurement, the average drops to 7.4 km2. The average distance between consecutive residence centroids was 4.1 ± 8.2 km (range 0.009–23.6 km, n = 15).
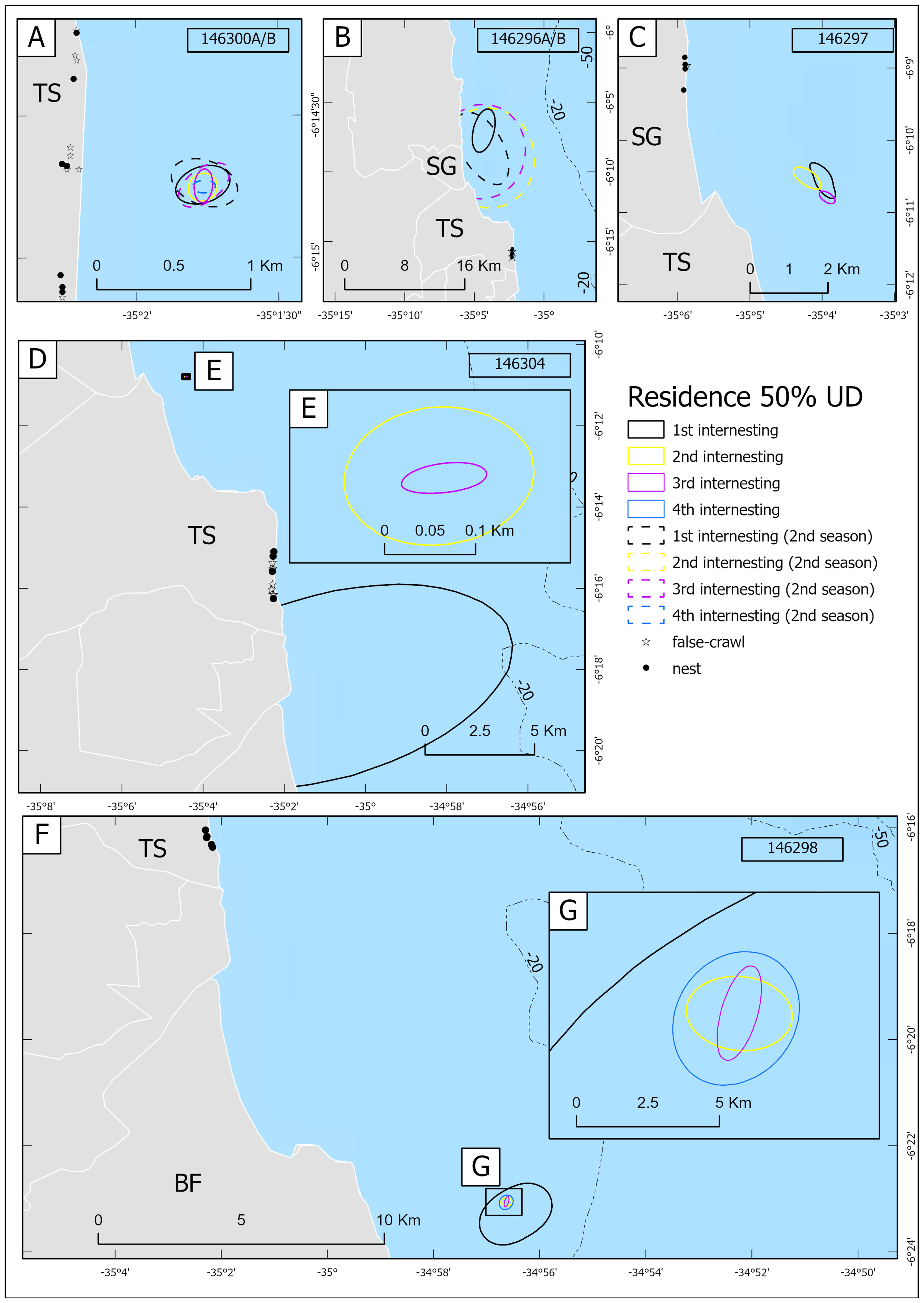
Figure 7 Residence 50% UD contours and locations of nesting attempts for five gravid hawksbill turtles in the south coast of Rio Grande do Norte, Brazil, tracked for more than one internesting interval: (A) 146300A/B, (B) 146296A/B, (C) 146297, (D) 146304, (F) 146298. Turtles 146300A/B and 146296A/B were tracked for two different nesting seasons. Insert boxes show zoom-in at: (E) 146304, and (G) 146298. Continental layer was overlayed to AKDE contours to emphasize that UDs were restricted to in-water. Different colors indicate different internesting intervals, with unbroken lines indicating internesting intervals in the first season and broken lines indicating internesting intervals in the second season.
4 Discussion
Our study identified five distinct latent behavioral states of internesting hawksbill turtles off the coastline of Rio Grande do Norte, Brazil using a novel approach that combines dive variables and spatial components obtained through animal-borne devices. Specifically, we assessed turtle activities using three dive variables: dive duration, dive depth, and surface interval. Shorter dive durations indicated higher activity levels, while longer dives suggested resting behavior (Hochscheid, 2014), with changes in average bottom depths indicating changes in activity levels (Hill et al., 2017). These dive variables were combined with spatial components such as the turtle’s position within the internesting home range, their activity (e.g., on-land nesting or remaining in-water), and speed of movement (e.g., step lengths) to determine latent behaviors. Most studies on internesting hawksbill turtles did not distinguish behaviors within this critical period (Bell and Parmenter, 2008; Gaos et al., 2012a; Gaos et al., 2012b; Marcovaldi et al., 2012; Pilcher et al., 2014; Esteban et al., 2015; Revuelta et al., 2015; Nivière et al., 2018; Hart et al., 2019; Hamilton et al., 2021; Santos et al., 2021a; Santos et al., 2021b; Maurer et al., 2022; Robinson et al., 2022). Indeed, few studies have differentiated behaviors within the internesting period of hawksbill turtles by visual inspection of displacement (Walcott et al., 2012; Walcott et al., 2013) or using changepoint analysis applied to dive variables (Hill et al., 2017). Such studies have divided internesting behavior of hawksbill turtles into three phases: 1) transit from nesting beach towards residence, 2) residency, 3) transit back to the nesting beach (Walcott et al., 2012; Walcott et al., 2013; Hill et al., 2017). Our study confirms the previously identified internesting phases of hawksbill turtles, but it expands previous work by describing the complex behaviors within each phase, particularly in the residency period and pre-nesting behavior. We also found that activity levels changed over time, where turtles exhibited decreasing activity levels and smaller residence areas over the nesting season.
Examining the total activity budgets for each identified behavior during the internesting periods, we found that hawksbill turtles remained in low activity most of the time (81.5%), primarily in quiescent behavior, but also in both ARS behaviors (Table 3). These three behaviors combined were categorized as the “residency period,” which lasted an average of 12.3 days or 79.1% of a single internesting period (Table 4). Despite differences in methodology, internesting hawksbill turtles in the US Virgin Islands and Barbados showed shorter residency periods of 10.3 days (or 68.7% of the internesting interval) and 9.8 days (66.2% of the internesting interval), respectively (Walcott et al., 2012; Hill et al., 2017). However, the average internesting length was slightly longer in our study (15.5 days), while in the US Virgin Islands it was 15 days (Hill et al., 2017) and in Barbados it was 14.8 days (Walcott et al., 2012). Average dive duration during the residency period in our study ranged from 52.3 to 63 min, similar to the 60 min found in Barbados (Walcott et al., 2013), but longer than the 39 min observed in St. Croix, US Virgin Islands (Hill et al., 2017). Notably, all studies consistently found that the residency period had the longest average dive durations. In our study, surface intervals were longer for behavioral states associated with residency period (Table 3). In contrast, surface intervals during residency in St. Croix, US Virgin Islands was the smallest amongst internesting phases (Hill et al., 2017), however still longer (2.3 min) in comparison with our average surface interval (1.3 min; Table 3). While longer surface intervals may aid in recovery after prolonged dives, the underlying factors influencing surface intervals (such as boat traffic, sea surface temperature, dive duration and depth) warrant further investigation in future studies.
Residency period in our study occurred within 14 km from the coast (average distance of 3.6 km), with average dive depths mainly between −18 m and −20 m (Table 3). Similar dive depths were reported for internesting hawksbill in the Northwest Atlantic, where turtles use near-shore habitats (Walcott et al., 2013; Nivière et al., 2018; Hart et al., 2019). In contrast, internesting hawksbill turtles from the Pacific use in-shore habitats such as estuaries in addition to near-shore areas, resulting in shallower dive depths (Bell and Parmenter, 2008; Gaos et al., 2012b). The bathymetry and complexity of the sea bottom affects dive depths during the internesting residency period, with more complex sea bottom topography and increased rugosity offering better quality habitat that is generally occupied earlier in the nesting season (Walcott et al., 2014). Moreover, sea surface temperature can also play a role in influencing dive depths during the internesting residency period, as turtles may choose shallower depths where temperatures are warmer to reduce gestation time between bouts of nesting (Sato et al., 1998; Hays et al., 2002; Robinson et al., 2022).
During the residency period, individuals showed fidelity to their residence areas within and between nesting seasons, as indicated by the average 60.2% of overlap of 50% UD for individuals tracked across multiple internesting intervals (Figure 7; Supplementary Table 3). Initial residence areas tended to be larger than subsequent ones within a season (Figure 7; Supplementary Table 3), which aligns with the increased proportion of quiescent behavior over time. Similar findings were reported for hawksbill turtles in Barbados (Walcott et al., 2012) and green turtles in Guinea-Bissau (Raposo et al., 2023), which also exhibited fidelity to internesting residence areas and a reduction in occupied area over time was observed. This is of importance for future study design since tracking marine turtles later in the nesting season will result in smaller estimates of occurrence distributions and these areas are important for conservation.
Most of the turtles (9 out of 10) stayed in a restricted area during their residency period, but one individual (146296B) used a larger residence area (Supplementary Figure 8), further investigation is needed to determine if this turtle followed the coastal current. One individual (41592) stayed in a single 250 × 250 m grid cell for the entire residency period, never diving below −2 m during its tracked internesting interval, and displaying quiescent behavior as shown in Figure 5C (the cell with high intensity of UD near Parnamirim municipality). This inter-individual variability indicates plasticity in hawksbill turtles’ behavior during their internesting residency period.
In between periods of residency, turtles actively moved back and forth between the nesting beach and residence area, with 13% of their internesting intervals spent in transit (2.3 days per interval). During transit, turtles moved at an average of 0.7 km h−1 (step lengths >700; Table 3), similar to transit speeds for internesting hawksbill turtles in Barbados (0.63 and 0.69 km h−1; Walcott et al., 2012) and also for Tortuguero, Costa Rica (0.8 km h−1; Troëng et al., 2005). As turtles converged around the nesting beaches, UD estimates gradually increased towards the nesting vicinity (Figure 5B), which highlight the importance of those areas for hawksbill turtles. Interestingly, when moving parallel to the coast towards the residence area, turtles swam farther from the coast compared to when they were returning towards the nesting beach (Figure 6 and Supplementary Figures 8, 9). It is possible that swimming farther from the coast towards the residence helps turtles avoid the stronger longshore current or being closer to the coastline while moving towards the nesting beach helps identify the best access point to crawl up the beach to nest. Most transit behaviors (73.7%) from residence towards nesting beach started after sundown, similar to hawksbill turtles in Barbados, in which 76.9% of turtles also initiated their return to the nesting vicinity at night (Walcott et al., 2012). At both sites, hawksbill turtles nest at night, and perhaps the decreasing light is used as a cue to start movement towards the nesting vicinity. It would be interesting to examine whether the opposite occurs with hawksbill turtles in the Indian Ocean, where they nest predominantly during the day (Diamond, 1976; Hirth, 1980).
While transit behavior was marked by fast and directed movement, turtles switched to pre-nesting behavior upon arriving in the shallower nesting vicinity, slowing down but still actively moving parallel to the coast. This suggests they were potentially seeking specific areas to nest. Despite pre-nesting behavior being the briefest state (average of 22.7 hours per internesting interval) of those estimated, a high spatial overlap between turtles in this state was observed within 1.5 km of the nesting beach. It is interesting to note that pre-nesting behavior showed the briefest average surface intervals (Table 3), in accordance with highest surfacing frequency of pre-emergence internesting hawksbill turtles in Barbados (Walcott et al., 2012).
Fine-scale spatial data of internesting turtle behavior can be used to inform targeted management and conservation efforts, particularly when considering the diverse impacts that various threats may have on turtles based on their behavior and overlap with a given threat. For example, turtles that spend more time at the surface may be at higher risk of vessel strikes (Shimada et al., 2017; Fuentes et al., 2021), of inhaling airborne pollutants (Jernelv, 2010) or encountering floating debris (Gregory, 2009; Duncan et al., 2017; Abreo et al., 2023). In contrast, when turtles are at the bottom of the ocean, they may be more susceptible as bycatch to trawling fishing vessels (Brewer et al., 2006; Kumar and Deepthi, 2006) and various bottom-set fishing gear (Wallace et al., 2010b), as well as pollution that accumulates on the seafloor (Putman et al., 2015). Although the spatial distribution of threats in the Rio Grande do Norte region are poorly known, artisanal fisheries and marine traffic are likely to pose the greatest in-water threats (Santos et al., 2021a), so identifying behaviors that increase the vulnerability to such threats (e.g., long surface times) is of importance. For example, the slower pre-nesting behavior is highly sensitive to threats such as coastal fishing and beach development, which brings with it a myriad of threats such as light pollution, introduced predators, vehicle traffic, and beach armoring (van de Me et al., 2012; Kamrowski et al., 2013; Aguilera et al., 2019; Nelson Sella and Fuentes, 2019; Colman et al., 2020; Lira et al., 2023; Sella et al., 2023). Moreover, the coastline of Northeastern Brazil, being in the corner of the South American continent, is particularly susceptible to beach erosion (Siqueira et al., 2021; Queiroz et al., 2022), which could be aggravated by climate change (Fuentes et al., 2010; Fuentes et al., 2012; Patrício et al., 2021). Our study site has a narrow band of beach surrounded by cliffs, and suitable habitat for nesting is being squeezed with sea level rise (see Mazaris et al., 2009). Considering these various and increasing threats, and the status of this regional management unit (RMU) (Wallace et al., 2010a), hawksbill turtles in this region are considered a conservation-dependent species that requires long-term management and monitoring activities (Casale et al., 2018; Omeyer et al., 2021).
Currently, less than 1% of the UD for internesting hawksbill turtles identified in this study overlaps with a Marine Protected Area (MPA) (Supplementary Figures 10A, B). In this case, the MPA was created and is managed by the Tibau do Sul municipality, is classified as multiple use, and was designed to protect the Guiana dolphin (Sotalia guianensis) (Lunardi et al., 2017). This is of concern since the rookeries considered in this region have the highest hawksbill nesting density within the South Atlantic (Santos et al., 2013) and belong to the data-deficient Southwest Atlantic RMU (Wallace et al., 2010a). In addition, hawksbill turtles are classified as endangered in Brazil (Ministerio do Meio Ambiente (MMA), 2022) and critically endangered internationally (Meylan and Donnelly, 1999). Finally, it is widely recognized that gravid female marine turtles have a high reproductive value in relation to other marine turtle life-stages, and are especially important as they contribute the most to population growth; thus protecting areas where they are found is of importance (Crouse et al., 1987; Bolten et al., 2011). The current MPAs in Brazil lack representativeness of ecosystems (Magris et al., 2013; Mills et al., 2020), explicit conservation goals, and targets, which highlights the need for urgent action by policymakers to establish new MPAs that prioritize the protection of critical habitats for threatened species (Santos et al., 2021a). Delineating areas with high UDs for hawksbill turtles can inform national action plans for conserving endangered species (ICMBio, 2011) and guide the expansion of MPAs in Brazil. This would help Brazil meet the Aichi Biodiversity Targets (Vercillo et al., 2023) and safeguard biodiversity. For instance, based on our results, 30.2% of internesting hawksbill turtle UDs would be protected if a multiple use MPA was implemented to protect the reef formation in Parrachos de Pirangi, compared to the current protection of 0.4% (Supplementary Figures 10A, B). Such protection should expand southward to include high UD areas within the −20 m isobath further south, which would add protection to internesting hawksbill turtles near Tibau do Sul and Baia Formosa municipalities as well.
As we continue to gain knowledge on the importance of specific in-water habitats to internesting hawksbill turtles, protecting these environments should become a priority. Spatiotemporal data can inform dynamic ocean management, such as mitigating threats that are likely to impact marine turtles. The estimation of high-use UDs could serve as a basis for defining protective buffer zones, extending the protection concept currently adopted in Brazil exclusively for major nesting beaches, to the sea (Santos et al., 2021a). Australia is recognized for having advanced environmental protection policies, which includes the mapping of Biological Important Areas (BIAs) for all six species of marine turtles found in Australian waters, available in the Conservation Values Atlas (Commonwealth of Australia, 2017; Tucker et al., 2020). The buffer zone from marine turtle nesting areas suggested for protection varies according to species, with 20 km suggested for five marine turtle species and 60 km for the flatback turtle (Natator depressus) (Commonwealth of Australia, 2017; Tucker et al., 2020). Protection of areas near nesting beaches could be seasonal to align with the period that turtles are using in those areas (i.e., could be scaled back to protect 1–3 peak months, or include the whole nesting season). In four regions of Brazil between Rio de Janeiro and Espirito Santo, Espirito Santo and Bahia, Bahia and Alagoas and Rio Grande do Norte, periodic restrictions of oil and gas exploration and production activities were instituted by the federal government during marine turtle nesting season for leatherback, loggerhead, olive ridley and hawksbill turtles (IBAMA, 2011). Such restrictions, which cover up to 27 km from the coast, encompass the whole UD estimates for internesting hawksbill turtles identified by this study. Other potentially impactful activities, including offshore wind turbines that are increasingly being planned for environments as shallow as −5 m along the northeastern coast of Brazil, may threaten marine turtles in the region. As a precaution to protect internesting hawksbill turtles, however, these turbines should be placed in areas deeper than the −20 m isobath since we found that turtles in these regions are utilizing areas shallower than −20 m isobath. This aspect should be considered during the licensing process for any potential development. Further, information on important areas to protect could be identified by satellite tagging additional turtles from Baia Formosa, which has the highest density of nesting hawksbill turtles in Brazil (Santos et al., 2013); only one female was tracked in that municipality in this study. Based on this disparity in tracking individuals across nesting beaches, the higher UD for all behaviors combined found in the southern part of Tibau do Sul municipality should be interpreted with caution (see Figure 5F) as a larger number of tracked turtles was due to logistical convenience and does not necessarily indicate a larger density of turtles in that region. To expand the estimation of important biological areas for hawksbill turtles and other marine species, especially in developing countries where satellite telemetry studies are costly, habitat suitability models could be a cost-effective alternative (Hacohen-Domené et al., 2015; Wright et al., 2022).
In this study, we used a mixed-membership model to estimate the proportion of different behavioral states per track segment and reported the dominant behavior within each segment. Thus, one limitation of our approach is that some segments had similar proportions of different behaviors and the model could not identify which specific positions within a segment corresponded to each behavioral state. Thus, despite the model being able to capture the complexity of behaviors within a segment, it did not offer insights into the specific positions within a segment that were associated with each behavioral state. We also acknowledge that marine turtles are long-lived animals, and in this study, we investigated the behavior of internesting hawksbill turtles within a single nesting season and for two turtles over two seasons; future work should explore behavioral patterns across more nesting seasons for individual turtles to determine the extent of variability exhibited by this important life history event. Nevertheless, our study of specific behavioral states using biologging technology has provided valuable insights into the ecology and conservation of threatened hawksbill turtles. This study demonstrated the complexity associated with the critical internesting period and highlighted inter-individual variation as well as fidelity to residence areas and nesting beaches, which can be used to potentially assess susceptibility to various threats. The mixed-membership model for movement (M4) effectively discriminated among multiple behavioral states from six variables and can be applied beyond the breeding season for this species by incorporating other data streams. The flexibility of the model allows for customized application to a wide range of animal behavior studies. As such, information from this method may be used to inform the management and conservation of different species. In our study, for example, we found that the establishment of buffer zones within the −20 m isobath and within 14 km from the coast of main nesting beaches would be impactful to the protection of internesting hawksbill turtles in Brazil. These findings highlight the need to continue to invest in innovative and flexible approaches to advance our understanding of marine animal behavior to guide conservation efforts and ensure their long-term survival.
Data availability statement
The raw data supporting the conclusions of this article will be made available by the authors, without undue reservation.
Ethics statement
The animal study was approved by Brazilian Biodiversity authorization and information system (SISBio) granted a data collection license (42760). The study was conducted in accordance with the local legislation and institutional requirements.
Author contributions
AS: Idea, design, field work, analyses and writing. JC: design, analyses, comments, and edits to the manuscript. DV: field work. EL: institutional support. VQ: dive variables extraction, comments, and edits to the manuscript. ES: regulatory proposal, GIS support, comments and edits to the manuscript. CB: regulatory proposal and field work. RR: Data curation. MF: design, supervision, comments, and edits to the manuscript. All co-authors approved the manuscript for publication.
Funding
National Science Foundation (Award 1904818). This work was done with a subset of data of a satellite telemetry project as part of licensing requirement by IBAMA for seismic surveys in Brazil, under supervision of Centro TAMAR/ICMBio. The funding for this work was provided as part of licensing requirement for seismic surveys carried out by the companies PGS (Petroleum Geo-Services) and Spectrum Geo.
Acknowledgments
We are thankful to the Santuario Ecologico de Pipa and Centro de Lançamento da Barreira do Inferno for the support on conducting this work. We also thank Kevin Lay and engineers from Wildlife Computers for the help with recovering data from retrieved satellite tags and Gustavo Stahelin for comments on the manuscript. We are also thankful for the support from the National Science Foundation (Award 1904818). This work was done with a subset of data of a satellite telemetry project as part of licensing requirement by IBAMA for seismic surveys in Brazil, under supervision of Centro TAMAR/ICMBio.
Conflict of interest
The authors declare that this study received funding from PGS (Petroleum Geo-Services) and Spectrum Geo. The funders were not involved in the study design, collection, analysis, interpretation of data, the writing of this article, or the decision to submit it for publication.
Publisher’s note
All claims expressed in this article are solely those of the authors and do not necessarily represent those of their affiliated organizations, or those of the publisher, the editors and the reviewers. Any product that may be evaluated in this article, or claim that may be made by its manufacturer, is not guaranteed or endorsed by the publisher.
Supplementary material
The Supplementary Material for this article can be found online at: https://www.frontiersin.org/articles/10.3389/fevo.2023.1229144/full#supplementary-material
References
Abreo N. A. S., Aurelio R. M., Kobayashi V. B., Thompson K. F. (2023). ‘Eye in the sky’: Off-the-shelf unmanned aerial vehicle (UAV) highlights exposure of marine turtles to floating litter (FML) in nearshore waters of Mayo Bay, Philippines. Mar. Pollut. Bull. 186, 114489. doi: 10.1016/j.marpolbul.2022.114489
Aguilera M., Medina-Suárez M., Pinós J., Liria A., López-Jurado L. F., Benejam L. (2019). Assessing the effects of multiple off-road vehicle (ORVs) tyre ruts on seaward orientation of hatchling sea turtles: implications for conservation. J. Coast. Conserv. 23, 111–119. doi: 10.1007/s11852-018-0641-x
Baudouin M., de Thoisy B., Chambault P., Berzins R., Entraygues M., Kelle L., et al. (2015). Identification of key marine areas for conservation based on satellite tracking of post-nesting migrating green turtles (Chelonia mydas). Biol. Conserv. 184, 36–41. doi: 10.1016/j.biocon.2014.12.021
Bell I. P., Parmenter C. J. (2008). The diving behavior of inter-nesting hawksbill turtles, Eretmochelys imbricata (Linnaeus 1766), on Milman Island Reef, Queensland, Australia. Herpetol Conserv Biol 3, 254–263.
Berger-Tal O., Polak T., Oron A., Lubin Y., Kotler B. P., Saltz D. (2011). Integrating animal behavior and conservation biology: a conceptual framework. Behav. Ecol. 22, 236–239. doi: 10.1093/beheco/arq224
Bolten A. B., Crowder L. B., Dodd M. G., Macpherson S. L., Musick J. A., Schroeder B. A., et al. (2011). Quantifying multiple threats to endangered species: An example from Loggerhead Sea turtles. Front. Ecol. Environ. 9, 295–301. doi: 10.1890/090126
Brewer D., Heales D., Milton D., Dell Q., Fry G., Venables B., et al. (2006). The impact of turtle excluder devices and bycatch reduction devices on diverse tropical marine communities in Australia’s northern prawn trawl fishery. Fish Res. 81, 176–188. doi: 10.1016/j.fishres.2006.07.009
Casale P., Broderick A. C., Camiñas J. A., Cardona L., Carreras C., Demetropoulos A., et al. (2018). Mediterranean sea turtles: Current knowledge and priorities for conservation and research. Endanger Species Res. 36, 229–267. doi: 10.3354/esr00901
CLS (2016). Argos User’s Manual 2007–2016. Collecte localisation satellites. Available at: https://www.argos-system.org/wp-content/uploads/2023/01/CLS-Argos-System-User-Manual.pdf. (Accessed 22 July 2022).
Colman L. P., Lara P. H., Bennie J., Broderick A. C., de Freitas J. R., Marcondes A., et al. (2020). Assessing coastal artificial light and potential exposure of wildlife at a national scale: the case of marine turtles in Brazil. Biodivers Conserv. 29, 1135–1152. doi: 10.1007/s10531-019-01928-z
Commonwealth of Australia (2017). Recovery plan for marine turtles in Australia. (Australian Government, Brisbane, Queensland: Australian Government, Department of the Environment and Energy). 154 pp.
Costa D. P., Robinson P. W., Arnould J. P. Y., Harrison A. L., Simmons S. E., Hassrick J. L., et al. (2010). Accuracy of ARGOS locations of pinnipeds at-sea estimated using fastloc GPS. PloS One 5, e8677. doi: 10.1371/journal.pone.0008677
Crain C. M., Bertness M. D. (2006). Ecosystem engineering across environmental gradients: implications for conservation and management. Bioscience 56 (3), 211–218.
Crouse D. T., Crowder L. B., Caswell H. (1987). A stage-based population model for loggerhead sea turtles and implications for conservation. Ecology 68, 1412–1423. doi: 10.2307/1939225
Cuevas E., Abreu-Grobois F. A., Guzmán-Hernández V., Liceaga-Correa M. A., van Dam R. P. (2008). Post-nesting migratory movements of hawksbill turtles Eretmochelys imbricata in waters adjacent to the Yucatan Peninsula, Mexico. Endanger Species Res. 10, 123–133. doi: 10.3354/esr00128
Cullen J. A., Poli C. L., Fletcher R. J., Valle D. (2022). Identifying latent behavioural states in animal movement with M4, a nonparametric Bayesian method. Methods Ecol. Evol. 13, 432–446. doi: 10.1111/2041-210X.13745
Dean B., Freeman R., Kirk H., Leonard K., Phillips R. A., Guilford T. (2013). Behavioural mapping of a pelagic seabird: combining multiple sensors and a hidden Markov model reveals the distribution of at-sea behaviour. J R Soc Interface 10, 20120570. doi: 10.1098/rsif.2012.0570
DeRuiter S. L., Langrock R., Skirbutas T., Goldbogen J. A., Calambokidis J., Friedlaender A. S., et al. (2017). A multivariate mixed hidden markov model for blue whale behaviour and responses to sound exposure. Ann. Appl. Stat 11, 362–392. doi: 10.1214/16-AOAS1008
Diamond A. W. (1976). Breeding biology and conservation of hawksbill turtles, ERETMOCHELYS IMBRICATA L., on Cousin Island, Seychelles. Biol. Conserv. 9, 199–215.
Dong X., Fleming C. H., Noonan M. J., Calabrese J. M. (2018). ctmmweb: A Shiny web app for the ctmm movement analysis package. Available at: https://github.com/ctmm-initiative/ctmmweb. (Accessed 22 July 2022).
Dujon A. M., Lindstrom R. T., Hays G. C. (2014). The accuracy of Fastloc-GPS locations and implications for animal tracking. Methods Ecol. Evol. 5, 1162–1169. doi: 10.1111/2041-210X.12286
Duncan E. M., Botterell Z. L. R., Broderick A. C., Galloway T. S., Lindeque P. K., Nuno A., et al. (2017). A global review of marine turtle entanglement in anthropogenic debris: A baseline for further action. Endanger Species Res. 34, 431–448. doi: 10.3354/esr00865
Esteban N., van Dam R. P., Harrison E., Herrera A., Berkel J. (2015). Green and hawksbill turtles in the Lesser Antilles demonstrate behavioural plasticity in inter-nesting behaviour and post-nesting migration. Mar. Biol. 162, 1153–1163. doi: 10.1007/s00227-015-2656-2
Ferreira L. C., Thums M., Fossette S., Wilson P., Shimada T., Tucker A. D., et al. (2021). Multiple satellite tracking datasets inform green turtle conservation at a regional scale. Divers. Distrib 27, 249–266. doi: 10.1111/ddi.13197
Fuentes M. M. P. B., Fish M. R., Maynard J. A. (2012). Management strategies to mitigate the impacts of climate change on sea turtle’s terrestrial reproductive phase. Mitig Adapt Strateg Glob Chang 17, 51–63. doi: 10.1007/s11027-011-9308-8
Fuentes M. M. P. B., Limpus C. J., Hamann M., Dawson J. (2010). Potential impacts of projected sea-level rise on sea turtle rookeries. Aquat Conserv. 20, 132–139. doi: 10.1002/aqc.1088
Fuentes M. M. P. B., Meletis Z. A., Wildermann N. E., Ware M. (2021). Conservation interventions to reduce vessel strikes on sea turtles: A case study in Florida. Mar. Policy 128, 104471. doi: 10.1016/j.marpol.2021.104471
Gaos A. R., Lewison R. R., Wallace B. P., Yañez I. L., Liles M. J., Baquero A., et al. (2012b). Dive behaviour of adult hawksbills (Eretmochelys imbricata, Linnaeus 1766) in the eastern Pacific Ocean highlights shallow depth use by the species. J. Exp. Mar. Biol. Ecol. 432–433, 171–178. doi: 10.1016/j.jembe.2012.07.006
Gaos A. R., Lewison R. L., Wallace B. P., Yañez I. L., Liles M. J., Nichols W. J., et al. (2012a). Spatial ecology of critically endangered hawksbill turtles Eretmochelys imbricata: Implications for management and conservation. Mar. Ecol. Prog. Ser. 450, 181–194. doi: 10.3354/meps09591
Gregory M. R. (2009). Environmental implications of plastic debris in marine settings-entanglement, ingestion, smothering, hangers-on, hitch-hiking and alien invasions. Philos. Trans. R. Soc. B 364, 2013–2025. doi: 10.1098/rstb.2008.0265
Hacohen-Domené A., Martínez-Rincón R. O., Galván-Magaña F., Cárdenas-Palomo N., de la Parra-Venegas R., Galván-Pastoriza B., et al. (2015). Habitat suitability and environmental factors affecting whale shark (Rhincodon typus) aggregations in the Mexican Caribbean. Environ. Biol. Fishes 98, 1953–1964. doi: 10.1007/s10641-015-0413-5
Hamilton R. J., Desbiens A., Pita J., Brown C. J., Vuto S., Atu W., et al. (2021). Satellite tracking improves conservation outcomes for nesting hawksbill turtles in Solomon Islands. Biol. Conserv. 261, 109240. doi: 10.1016/j.biocon.2021.109240
Hart K. M., Iverson A. R., Benscoter A. M., Fujisaki I., Cherkiss M. S., Pollock C., et al. (2019). Satellite tracking of hawksbill turtles nesting at Buck Island Reef National Monument, US Virgin Islands: Inter-nesting and foraging period movements and migrations. Biol. Conserv. 229, 1–13. doi: 10.1016/j.biocon.2018.11.011
Hastings A., Byers J. E., Crooks J. A., Jones C. G., Lambrinos J. G., Talley T. S., et al. (2007). Ecosystem engineering in space and time. Ecol. Lett. 10, 153–164. doi: 10.1111/j.1461-0248.2006.00997.x
Hays G. C., Adams C. R., Broderick A. C., Godley B. J., Lucas D. J., Metcalfe J. D., et al. (2000). The diving behaviour of green turtles at Ascension Island. Anim. Behav. 59, 577–586. doi: 10.1006/anbe.1999.1326
Hays G. C., Bailey H., Bograd S. J., Bowen W. D., Campagna C., Carmichael R. H., et al. (2019). Translating marine animal tracking data into conservation policy and management. Trends Ecol. Evol. 34, 459–473. doi: 10.1016/j.tree.2019.01.009
Hays G. C., Broderick A. C., Glen F., Godley B. J., Houghton J. D. R., Metcalfe J. D. (2002). Water temperature and internesting intervals for loggerhead (Caretta caretta) and green (Chelonia mydas) sea turtles. J. Therm Biol. 27, 429–432. doi: 10.1016/S0306-4565(02)00012-8
Hays G. C., Ferreira L. C., Sequeira A. M. M., Meekan M. G., Duarte C. M., Bailey H., et al. (2016). Key questions in marine megafauna movement ecology. Trends Ecol. Evol. 31, 463–475. doi: 10.1016/j.tree.2016.02.015
Hays G. C., Hawkes L. A. (2018). Satellite tracking sea turtles: Opportunities and challenges to address key questions. Front. Mar. Sci. 5. doi: 10.3389/fmars.2018.00432
Hays G. C., Mortimer J. A., Rattray A., Shimada T., Esteban N. (2021). High accuracy tracking reveals how small conservation areas can protect marine megafauna. Ecol. Appl. 31, e02418. doi: 10.1002/eap.2418
Hill J. E., Robinson N. J., King C. M., Paladino F. V. (2017). Diving behavior and thermal habitats of gravid hawksbill turtles at St. Croix, USA. Mar. Biol. 164, 1–9. doi: 10.1007/s00227-016-3050-4
Hirth H. F. (1980). Some aspects of the nesting behavior and reproductive biology of sea turtles. Amer. Zool. 20, 507–523.
Hochscheid S. (2014). Why we mind sea turtles’ underwater business: A review on the study of diving behavior. J. Exp. Mar. Biol. Ecol. 450, 118–136. doi: 10.1016/j.jembe.2013.10.016
Horrocks J. A., Vermeer L. A., Krueger B. H., Coyne M. S., Schroeder B., Balazs G. H. (2001). Migration routes and destination characteristics of post-nesting hawksbill turtles satellite-tracked from Barbados, West Indies. Chelonian Conserv. Biol. 4, 107–114.
IBAMA. (2011). Instituto Brasileiro do Meio Ambiente e dos Recursos Naturais Renováveis (IBAMA) e Instituto Chico Mendes de Conservação da Biodiversidade (ICMBio). Instrução Normativa IBAMA/ICMBIO N° 1, de 27 de maio de 2011. Diário Oficial da União, Brasília, n° 102, 30 de maio de 2011, Seção 1, p. 122. ISSN 1677-7042
ICMBio (2011). Plano de Ação Nacional para Conservação das Tartarugas Marinhas. Série Espécies Ameaçadas 25. Eds. Marcovaldi M. A. G., Santos A. S., Sales G. (Brasilia: ICMBio). Available at: www.icmbio.gov.br.
Iverson A., Hart K., Fujisaki I. (2016). Hawksbill satellite-tracking case study: Implications for remigration interval and population estimates. Mar. Turtle Newslett. 148, 2–7.
Jernelv A. (2010). The threats from oil spills: Now, then, and in the future. Ambio 39, 353–366. doi: 10.1007/s13280-010-0085-5
Jim L. M. R., Rice M. R., Hickey F. R., Balazs G. H. (2022). Destination revealed: post-nesting migrations of hawksbill turtles (Eretmochelys imbricata) from Moso Island, Republic of Vanuatu 1. Micronesica 1, 1–16.
Johnson D. S., London J. M., Lea M.-A., Durban J. W. (2008) Continuous-time correlated random walk model for animal telemetry data. Available at: https://www.argos-system.org/manual/i.
Jonsen I. (2020). bsam: bayesian state-space models for animal movement. (Version 1.1.2) [R package].
Jonsen I. (2023). aniMotum: fit continuous-time state-space and latent variable models for quality control of argos satellite (and other) telemetry data and for estimating changes in animal movement. (v1.1-04) [R package].
Jonsen I. D., Flemming J. M., Myers R. A. (2005). Robust state-space modeling of animal movement data. Ecology 86, 2874–2880. doi: 10.1890/04-1852
Jonsen I. D., Grecian W. J., Phillips L., Carroll G., McMahon C., Harcourt R. G., et al. (2023). aniMotum, an R package for animal movement data: Rapid quality control, behavioural estimation and simulation. Methods Ecol. Evol. 14, 806–816. doi: 10.1111/2041-210X.14060
Jonsen I. D., Patterson T. A., Costa D. P., Doherty P. D., Godley B. J., Grecian W. J., et al. (2020). A continuous-time state-space model for rapid quality control of argos locations from animal-borne tags. Mov Ecol. 8, 1–13. doi: 10.1186/s40462-020-00217-7
Kamrowski R. L., Limpus C., Moloney J., Hamann M. (2013). Coastal light pollution and marine turtles: Assessing the magnitude of the problem. Endanger Species Res. 19, 85–98. doi: 10.3354/esr00462
Kuhn C. E., Johnson D. S., Ream R. R., Gelatt T. S. (2009). Advances in the tracking of marine species: Using GPS locations to evaluate satellite track data and a continuous-time movement model. Mar. Ecol. Prog. Ser. 393, 97–109. doi: 10.3354/meps08229
Kumar A. B., Deepthi G. R. (2006). Trawling and by-catch: Implications on marine ecosystem. Curr. Sci. 90, 922–930.
Langrock R., King R., Matthiopoulos J., Thomas L., Fortin D., Morales J. M. (2012). Flexible and practical modeling of animal telemetry data: Hidden Markov models and extensions. Ecology 93, 2336–2342. doi: 10.1890/11-2241.1
Lira L. N., Cavalcante I. C. O. G., da S., Ferreira L. V. F. (2023). Buggy rides as a tourist attraction in Rio Grande Do Norte, Brazil. Appl. Tourism 7, 83–94. doi: 10.14210/at.v7i3.19324
Lopez R., Malardé J. P., Royer F., Gaspar P. (2014). Improving argos doppler location using multiple-model kalman filtering. IEEE Trans. Geosci. Remote Sens. 52, 4744–4755. doi: 10.1109/TGRS.2013.2284293
Lunardi D. G., Azevedo Dos Santos J. E., Sousa Do Nascimento L. L., De Freitas D. C., De Oliveira Lunardi V. (2017). An assessment of the guiana dolphin watching tourism activities at the coastal wildlife reserve of tibau do sul (Refauts), Rio Grande do Norte State, Brazil. Sustentabilidade em Debate 8, 40–53. doi: 10.18472/SustDeb.v8n1.2017.20213
Magris R. A., Mills M., Fuentes M. M. P. B., Pressey R. L. (2013) Analysis of progress towards a comprehensive system of marine protected areas in Brazil. Available at: http://www.mma.gov.br/areas-protegidas/.
Marcovaldi M., Lopez G., Soares L., López-Mendilaharsu M. (2012). Satellite tracking of hawksbill turtles Eretmochelys imbricata nesting in northern Bahia, Brazil: turtle movements and foraging destinations. Endanger Species Res. 17, 123–132. doi: 10.3354/esr00421
Marcovaldi M. A., Lopez G. G., Soares L. S., Santos A. J. B., Bellini C., Barata P. C. R. (2007). Fifteen years of hawksbill sea turtle (Eretmochelys imbricata) nesting in northern Brazil. Chelonian Conserv. Biol. 6, 223. doi: 10.2744/1071-8443(2007)6[223:fyohst]2.0.co;2
Maurer A. S., Dawson C., Bjorkland R., Donaldson A., Stapleton S. P., Richardson J. I., et al. (2022). Satellite telemetry elucidates migratory pathways and foraging areas for hawksbill sea turtles, eretmochelys imbricata, in the caribbean. Caribb J. Sci. 52 (1), 126–141. doi: 10.18475/cjos.v52i1.a10
Mazaris A. D., Matsinos G., Pantis J. D. (2009). Evaluating the impacts of coastal squeeze on sea turtle nesting. Ocean Coast. Manag 52, 139–145. doi: 10.1016/j.ocecoaman.2008.10.005
Mazaris A. D., Schofield G., Gkazinou C., Almpanidou V., Hays G. C. (2017). Global sea turtle conservation successes. Sci. Adv. 3, e1600730. doi: 10.1126/SCIADV.1600730
Meylan A. B., Donnelly M. (1999). Status justification for listing the hawksbill turtle (Eretmochelys imbricata) as critically endangered on the 1996 IUCN Red List of Threatened Animals. Chelonian conservation Biol. 3 (2), 200–224.
Mills M., Magris R. A., Fuentes M. M. P. B., Bonaldo R., Herbst D. F., Lima M. C. S., et al. (2020). Opportunities to close the gap between science and practice for Marine Protected Areas in Brazil. Perspect. Ecol. Conserv. 18, 161–168. doi: 10.1016/j.pecon.2020.05.002
Ministério do Meio Ambiente. (2020). Portaria no 148, de 7 de junho de 2022. Diário Oficial da União, Brasília, Edição: 108, Seção: 1, p. 74.
Morelle K., Bunnefeld N., Lejeune P., Oswald S. A. (2017). From animal tracks to fine-scale movement modes: a straightforward approach for identifying multiple spatial movement patterns. Methods Ecol. Evol. 8, 1488–1498. doi: 10.1111/2041-210X.12787
Nathan R., Getz W. M., Revilla E., Holyoak M., Kadmon R., Saltz D., et al. (2008). A movement ecology paradigm for unifying organismal movement research. PNAS 105, 19052–19059. doi: 10.1073/pnas.0800375105
Nathan R., Monk C. T., Arlinghaus R., Adam T., Alós J., Assaf M., et al. (2022). Big-data approaches lead to an increased understanding of the ecology of animal movement. Sci. (1979) 375, 375. doi: 10.1126/science.abg1780
Nelson Sella K. A., Fuentes M. M. P. B. (2019). Exposure of marine turtle nesting grounds to coastal modifications: Implications for management. Ocean Coast. Manag 169, 182–190. doi: 10.1016/j.ocecoaman.2018.12.011
Nivière M., Chambault P., Pérez T., Etienne D., Bonola M., Martin J., et al. (2018). Identification of marine key areas across the Caribbean to ensure the conservation of the critically endangered hawksbill turtle. Biol. Conserv. 223, 170–180. doi: 10.1016/j.biocon.2018.05.002
Okuyama J., Benson S. R., Dutton P. H., Seminoff J. A. (2021). Changes in dive patterns of leatherback turtles with sea surface temperature and potential foraging habitats. Ecosphere 12 (2), e03365. doi: 10.1002/ecs2.3365
Omeyer L. C. M., Stokes K. L., Beton D., Çiçek B. A., Davey S., Fuller W. J., et al. (2021). Investigating differences in population recovery rates of two sympatrically nesting sea turtle species. Anim. Conserv. 24, 832–846. doi: 10.1111/acv.12689
Patel S. H., Morreale S. J., Panagopoulou A., Bailey H., Robinson N. J., Paladino F., et al. (2015). Changepoint analysis: A new approach for revealing animal movements and behaviors from satellite telemetry data. Ecosphere 6, 1–13. doi: 10.1890/ES15-00358.1
Patrício A. R., Hawkes L. A., Monsinjon J. R., Godley B. J., Fuentes M. M. P. B. (2021). Climate change and marine turtles: Recent advances and future directions. Endanger Species Res. 44, 363–395. doi: 10.3354/esr01110
Patterson T. A., McConnell B. J., Fedak M. A., Bravington M. V., Hindell M. A. (2010). Using GPS data to evaluate the accuracy of state-space methods for correction of Argos satellite telemetry error. Ecology 91, 273–285. doi: 10.1890/08-1480.1
Patterson T. A., Parton A., Langrock R., Blackwell P. G., Thomas L., King R. (2017). Statistical modelling of individual animal movement: an overview of key methods and a discussion of practical challenges. AStA Adv. Stat. Anal. 101, 399–438. doi: 10.1007/s10182-017-0302-7
Pilcher N. J., Antonopoulou M., Perry L., Abdel-Moati M. A., Al Abdessalaam T. Z., Albeldawi M., et al. (2014). Identification of important sea turtle areas (ITAs) for hawksbill turtles in the Arabian region. J. Exp. Mar. Biol. Ecol. 460, 89–99. doi: 10.1016/j.jembe.2014.06.009
Putman N. F., Abreu-Grobois F. A., Iturbe-Darkistade I., Putman E. M., Richards P. M., Verley P. (2015). Deepwater Horizon oil spill impacts on sea turtles could span the Atlantic. Biol. Lett. 11 (12), 20150596. doi: 10.1098/rsbl.2015.0596
Queiroz H. A., de A., Gonçalves R. M., Mishra M. (2022). Characterizing global satellite-based indicators for coastal vulnerability to erosion management as exemplified by a regional level analysis from Northeast Brazil. Sci. Total Environ. 817, 152849. doi: 10.1016/j.scitotenv.2021.152849
Raposo C., Mestre J., Rebelo R., Regalla A., Davies A., Barbosa C., et al. (2023). Spatial distribution of inter-nesting green turtles from the largest Eastern Atlantic rookery and overlap with a marine protected area. Mar. Ecol. Prog. Ser. 703, 161–175. doi: 10.3354/meps14225
R Core Team (2022) R: A language and environment for statistical computing. Available at: https://www.r-project.org/.
Revuelta O., Hawkes L., León Y. M., Godley B. J., Raga J. A., Tomás J. (2015). Evaluating the importance of Marine Protected Areas for the conservation of hawksbill turtles Eretmochelys imbricata nesting in the Dominican Republic. Endanger Species Res. 27, 169–180. doi: 10.3354/esr00660
Robinson N. J., Sanfèlix M. M., Blanco G. S., Clyde-Brockway C., Hill J. E., Paladino F. V., et al. (2022). Effect of water temperature on the duration of the internesting interval across sea turtle species. J. Therm Biol. 110, 103342. doi: 10.1016/j.jtherbio.2022.103342
Santos A. J. B., Bellini C., Sales G., Ramos R., Gillis A. J., Wildermann N. E., et al. (2021a). Effectiveness and design of marine protected areas for migratory species of conservation concern: a case study of post-nesting hawksbill turtles in Brazil. Biol. Conserv. 261, 109229. doi: 10.1016/j.biocon.2021.109229
Santos A. J. B., Bellini C., Vieira D., Neto L., Corso G. (2013). Northeast Brazil shows highest hawksbill turtle nesting density in the South Atlantic. Endanger Species Res. 21, 25–32. doi: 10.3354/esr00505
Santos A. J. B., Vieira D. H. G., Bellini C., Corso G., Ceriani S. A., Fuentes M. M. P. B. (2021b). Using data from nesting beach monitoring and satellite telemetry to improve estimates of marine turtle clutch frequency and population abundance. Mar. Biol. 168, 1–16. doi: 10.1007/s00227-021-03983-z
Sato K., Matsuzawa Y., Tanaka H., Bando T., Minamikawa S., Sakamoto W., et al. (1998). Internesting intervals for loggerhead turtles, Caretta caretta, and green turtles, Chelonia mydas, are affected by temperature. Can. J. Zool 76, 1651–1662. doi: 10.1139/z98-107
Sella K. A. N., Ware M., Ceriani S. A., Desjardin N., Eastman S., Addison D., et al. (2023). Urban pocket beaches as nesting habitat for marine turtles: Their importance and risk from inundation. Glob Ecol. Conserv. 41, e02366. doi: 10.1016/j.gecco.2023.e02366
Shimada T., Jones R., Limpus C., Hamann M. (2012). Improving data retention and home range estimates by data-driven screening. Mar. Ecol. Prog. Ser. 457, 171–180. doi: 10.3354/meps09747
Shimada T., Limpus C., Jones R., Hamann M. (2017). Aligning habitat use with management zoning to reduce vessel strike of sea turtles. Ocean Coast. Manag 142, 163–172. doi: 10.1016/j.ocecoaman.2017.03.028
Siqueira S. C. W., Gonçalves R. M., Queiroz H. A. A., Pereira P. S., Silva A. C., Costa M. B. (2021). Understanding the coastal erosion vulnerability influence over sea turtle (Eretmochelys imbricata) nesting in NE of Brazil. Reg. Stud. Mar. Sci. 47, 101965. doi: 10.1016/j.rsma.2021.101965
Thomson J. A., Heithaus M. R. (2014). Animal-borne video reveals seasonal activity patterns of green sea turtles and the importance of accounting for capture stress in short-term biologging. J. Exp. Mar. Biol. Ecol. 450, 15–20. doi: 10.1016/j.jembe.2013.10.020
Troëng S., Dutton P. H., Evans D. (2005). Migration of hawksbill turtles Eretmochelys imbricata from Tortuguero, Costa Rica. Ecography 28, 394–402. doi: 10.1111/j.0906-7590.2005.04110.x
Tucker T., Whiting S., Fossette S., Rob D., Barnes P. (2020). Inter-nesting and migrations by marine turtles of the Muiron Islands and Ningaloo Coast: Final Report. Prepared for Woodside Energy Limited. Department of Biodiversity, Conservation and Attractions, Perth. pp. 1–93
van de Me M., West E., Ibrahim K. (2012). Effects of off-road vehicle tyre ruts on the beach dispersal of green sea turtle Chelonia mydas hatchlings. Endanger Species Res. 18, 27–34. doi: 10.3354/esr00436
Vercillo U. E., Morato R. G., de Almeida Cunha A., de Marco P., Strier K. B., Mittermeier R. A., et al. (2023). Action plans for species conservation are an important tool to meet global and national biodiversity targets – A study case in Brazil. J. Nat. Conserv. 71, 126324l. doi: 10.1016/j.jnc.2022.126324
Walcott J., Eckert S., Horrocks J. A. (2012). Tracking hawksbill sea turtles (Eretmochelys imbricata) during inter-nesting intervals around Barbados. Mar. Biol. 159, 927–938. doi: 10.1007/s00227-011-1870-9
Walcott J., Eckert S., Horrocks J. A. (2013). Diving behaviour of hawksbill turtles during the inter-nesting interval: Strategies to conserve energy. J. Exp. Mar. Biol. Ecol. 448, 171–178. doi: 10.1016/j.jembe.2013.07.007
Walcott J., Eckert S., Oxenford H. A., Horrocks J. A. (2014). Use of a towed camera system to investigate benthic habitat use by inter-nesting female hawksbill sea turtles. Endanger Species Res. 24, 159–170. doi: 10.3354/esr00597
Wallace B. P., DiMatteo A. D., Hurley B. J., Finkbeiner E. M., Bolten A. B., Chaloupka M. Y., et al. (2010a). Regional management units for marine turtles: a novel framework for prioritizing conservation and research across multiple scales. PloS One 5, e15465. doi: 10.1371/journal.pone.0015465
Wallace B. P., Lewison R. L., Mcdonald S. L., Mcdonald R. K., Kot C. Y., Kelez S., et al. (2010b). Global patterns of marine turtle bycatch. Conserv. Lett. 3, 131–142. doi: 10.1111/j.1755-263X.2010.00105.x
Whoriskey K., Auger-Méthé M., Albertsen C. M., Whoriskey F. G., Binder T. R., Krueger C. C., et al. (2017). A hidden Markov movement model for rapidly identifying behavioral states from animal tracks. Ecol. Evol. 7, 2112–2121. doi: 10.1002/ECE3.2795
Wilcove D. S., Rothstein D., Dubow J., Phillips A., Losos E. (1998). Quantifying threats to imperiled species in the United States. BioScience 48 (8), 607–615. doi: 10.2307/1313420
Witt M. J., Åkesson S., Broderick A. C., Coyne M. S., Ellick J., Formia A., et al. (2010). Assessing accuracy and utility of satellite-tracking data using Argos-linked Fastloc-GPS. Anim. Behav. 80, 571–581. doi: 10.1016/j.anbehav.2010.05.022
Keywords: sea turtle, hawksbill turtle, dive behavior, latent behavior, spatial ecology, conservation management, utilization distribution, satellite telemetry
Citation: Santos AJB, Cullen J, Vieira DHG, Lima EHSM, Quennessen V, Santos EAPd, Bellini C, Ramos R and Fuentes MMPB (2023) Decoding the internesting movements of marine turtles using a fine-scale behavioral state approach. Front. Ecol. Evol. 11:1229144. doi: 10.3389/fevo.2023.1229144
Received: 26 May 2023; Accepted: 10 October 2023;
Published: 10 November 2023.
Edited by:
Cristiano Bertolucci, University of Ferrara, ItalyReviewed by:
Jacques-Olivier Laloe, Deakin University, AustraliaAlexander Richard Gaos, National Oceanic and Atmospheric Administration, United States
Copyright © 2023 Santos, Cullen, Vieira, Lima, Quennessen, Santos, Bellini, Ramos and Fuentes. This is an open-access article distributed under the terms of the Creative Commons Attribution License (CC BY). The use, distribution or reproduction in other forums is permitted, provided the original author(s) and the copyright owner(s) are credited and that the original publication in this journal is cited, in accordance with accepted academic practice. No use, distribution or reproduction is permitted which does not comply with these terms.
*Correspondence: Armando J. B. Santos, armando_barsante@msn.com