Number of lifetime menses increases breast cancer occurrence in postmenopausal women at high familial risk
- 1CREEC/CANECEV (CREES), MIVEGEC, Unité Mixte de Recherches, IRD 224–CNRS 5290, Université de Montpellier, Montpellier, France
- 2UMR 7206 Eco-Anthropologie (EA), Muséum National d'Histoire Naturelle, CNRS, Musée de l'Homme, Université Paris Cité, Paris, France
- 3Service Génétique Médicale, Maladies Rares et Médecine Personnalisée, Centre Hospitalier Universitaire de Montpellier, Montpellier, France
- 4Centre for Integrative Ecology, School of Life and Environmental Sciences, Deakin University, Waurn Ponds, VIC, Australia
It is increasingly thought that part of human susceptibility to cancer is the result of evolutionary mismatches: our ancestors evolved cancer suppression mechanisms in a world largely different from our modern environments. In that context, it has been shown in cohorts from general Western populations that reproductive traits modulate breast cancer risk. Overall, the more menses women experience, the more at risk they are to develop postmenopausal breast cancer. This points towards an evolutionary mismatch but brings the question whether the reproductive pattern also modulates the breast cancer risk in menopausal women at high familial risk. We thus studied the influence of menses on breast cancer risk in a case–control study of 90 postmenopausal women (including BRCA1/2 and non BRCA1/2) nested within a cohort at high familial risk. We tested the association of the lifetime number of menses and the number of menses before first full-term pregnancy with postmenopausal breast cancer risk using Cox survival models. We showed that the total lifetime number of menses was significantly associated with postmenopausal breast cancer risk and associated with a quicker onset of breast cancer after menopause. Those results align with similar studies lead in general cohorts and suggest that the reproductive pattern modulates the familial risk of developing breast cancer after menopause. Altogether, those results impact how we envision breast cancer prevention and call for more research on how ecological and genetic factors shape breast cancer risk.
Introduction
Because natural selection usually takes multiple generations to shape fitness-related traits in organisms, individuals living in rapidly changing environments may display, at least temporarily, maladapted features. In humans, such evolutionary mismatches are now known to cause several “modern” diseases (Nesse, 2011) such as obesity or allergies. In addition, most of the major environmental changes are recent in the history of humankind. For instance, investigations on Non-Communicable Diseases (NCDs), as opposed to infectious diseases (Allender et al., 2008) pointed out the role of sedentary lifestyle due to urbanization. In return, sedentarity is known to be a risk factor for obesity (Lopez and Hynes, 2006). Those evolutionary considerations are important: understanding our vulnerability to NCDs helps to shape public health policies (Nesse and Stearns, 2008).
Although cancer is at least as old as 1.7 millions years (Odes et al., 2016) and has presumably affected humans for a long time (Prates et al., 2011), it is increasingly assumed that part of our susceptibility to it results from evolutionary mismatches between the environment that our cancer suppression systems evolved in and our modern world [see, for instance, Aktipis and Nesse (2013) and Hochberg and Noble (2017)]: in such cases, defense mechanisms are overwhelmed by novel exposures to carcinogens. For instance, regular red meat consumption has been associated with an increased risk of developing prostate (You and Henneberg, 2018) and colorectal (Aykan, 2015) cancers.
In that context, increased breast cancer incidence in controlled-fertility populations could be the result of such evolutionary mismatch. Indeed, a compelling range of reproductive features have been associated with breast cancer risk. For instance, having few offspring and having them late in life is associated with an increased risk of developing breast cancer (MacMahon et al., 1970). You et al. (2018) showed that birth rate variation explains breast cancer incidence variations between countries. In this study, epidemiological indicators such as gross domestic product (GDP), urbanization or overweight, were no longer significantly associated with breast cancer risk once incidence was normalized on birth rate (You et al., 2018).
A lower parity actually results in very high exposure to reproductive hormones: Eaton et al. (1994) calculated that modern American women are, on average, experiencing 450 menstrual cycles in their lifetime (Eaton et al., 1994) whereas Strassmann (1997) showed that Malian Dogon women only experience about 100 menses due to their “natural fertility” (i.e., no contraceptive). Concomitantly, American women are 12 times more at risk to develop breast cancer compared to women from West Africa [who have a similar reproductive pattern according to Strassmann (1999)]. As reviewed by Persson (2000), other traits of the modern reproductive pattern have been associated with breast cancer risk such as ages at menarche and menopause, breastfeeding and exogenous estrogen such as oral contraceptive (hereafter, “OC”) and postmenopausal hormone replacement therapy (“HRT”). Hormonal mechanisms partly explain why reproductive traits are tied to breast cancer risk. For instance, the Collaborative Group on Hormonal Factors in Breast Cancer (2012) found that women with mutated estrogen-receptors were more likely to develop tumors during menopause. Additionally, it has been shown that estrogens have a proliferative effect and trigger mammary cell differentiations (Coe and Steadman, 1995). In that same study, Coe and Steadman described the ancestral reproductive pattern as a continuing series of pregnancies and lactation with ancestral women spending most of their lifetime in amenorrhea (implying the absence of ovulation). They hypothesized that departure from this ancestral pattern increased breast cancer risk. Building on observations on the natural fertility population of Dogons in Mali, Strassmann (1999) came to the same conclusion and suggested that allocating more energy to sustain more ovulations could in return decrease the investment in maintenance and protection against proliferative problems. In other words, part of our susceptibility to hormone positive breast cancer might result from an evolutionary mismatch: our exposure to cycling hormones may be higher than our cancer suppression systems have evolved to handle.
Indeed, a second fundamental aspect differs between the ancestral and modern reproductive patterns: exposure to exogenous estrogen and progestogen, OC and HRT. The influence of those exogenous hormones on breast cancer risk is not straightforward. For instance, OCs are supposed to mimic pregnancy and, therefore, could lower the breast cancer risk National Cancer Institute (2018) fact sheet. Conversely, OCs have been associated with an increased risk of developing breast cancer in different studies (see references in the fact sheet). Studies and meta-analyses shedding light on the increased risk due to OC first find a moderate rate increase (Collaborative group on hormonal factors, 2012) and, second, find that the magnitude of the increase depends on both the duration and the type of OC (for instance, progestogen-only OCs are not associated with an increased risk; Mørch et al., 2017). Lastly, levels of exposure must also be compared to those experienced in natural cycles (Lovett et al., 2017), hence differences between progestogens must be asserted. Regarding HRT, the increase in breast cancer risk depends on whether estrogens are administered alone or combined with progestogens and influence marginally decreases after the treatment is stopped (Manson et al., 2013). Overall, OCs seem to moderately increase the risk under certain situations and HRT effects are even more unclear.
To explore the influence of all those reproductive traits, the total number of menses women experience in their lifetime has been used as a metric. Two studies investigated the postmenopausal breast cancer risk associated with the cumulative number of menses and the number of menses before First Full-Term Pregnancy (hereafter “FFTP”) in cohorts from the general population (Clavel-Chapelon and E3N Group, 2002; Chavez-MacGregor et al., 2005). These two studies, albeit very similar, slightly differed in their results: Clavel-Chapelon and E3N Group (2002) found a linear risk increase whereas Chavez-MacGregor et al. (2005) suggested that the risk was increasing after a threshold.
Both studies computed the lifetime number of menses by subtracting the time spent in amenorrhea to the reproductive span (between menarche and menopause) and then dividing the result by the average length cycle. It is interesting to note that the lifetime number of menses they compute is a proxy for the “true” number of ovulatory cycles as they consider irregularity to only lead to longer cycles (shorter ones cannot be ovulatory) and as amenorrhea is due to reproductive events known to stop ovulation (such as pregnancy or breastfeeding). However, on the other hand, both studies consider that OCs, while blocking ovulation, create cycles equivalent to ovulatory ones. This is puzzling as (i) more hormones are involved in menstrual cycles than in OCs and (ii) it has been shown that hormonal exposure is different with OCs compared to menstrual cycles (Lovett et al., 2017).
Although paving the way, those two studies did not address the question of how the reproductive pattern influences the risk in women at high risk due to familial history. This is crucial as some genetic loci have been associated with an increased risk to develop cancer but have not been otherwise associated with the phenotypic reproductive traits mentioned above (Warren Andersen et al., 2014). Moreover, known genes are responsible for familial cases of breast cancer (Kuchenbaecker et al., 2017) and can also be associated with reproductive traits. For instance, carriers of mutation in the BReast CAncer (BRCA) gene exhibit differential breast cancer risk according to not only their parity but also the timing of the different pregnancies (Terry et al., 2018).
Here we investigate whether the cumulative lifetime number of menses and the number of menses before FFTP modulates the postmenopausal risk of breast cancer in women at high familial risk. We use this metric as (i) it encompasses the reproductive traits known to modulate breast cancer risk and (ii) it is a proxy for the total number of ovulations that is otherwise inaccessible. We consider that OCs block ovulation and therefore that OCs participate in amenorrhea. Most importantly, we consider the timespan between menopause and either diagnosis or age at recruitment (in 2017) to address whether women experiencing more menses accelerates the breast cancer onset. We find that the cumulative number of menses is significantly associated with a shorter timespan between menopause and cancer diagnosis whereas the association with FFTP is not significant.
Materials and methods
Cohort: Recruitment, data collection, and ethics
Our study sample comes from a cohort of 522 women recruited at the Centre Hospitalier Université de Montpellier, France in 2017. The inclusion criteria was family medical history: women were recruited because at least one of their first-degree relatives (n = 427) – in majority mothers and sisters – or higher-degree relatives (second degree, n = 358 and up to the third degree, n = 191) developed breast cancer (216 mothers and 186 sisters) or other reproductive tumors (e.g., ovaries: 55 mothers, 31 sisters). Median age at inclusion was 54 years old. Out of the 522 women, 356 of them had developed breast cancer (n = 355 confirmed with medical record). The median age at first breast cancer diagnosis was 40 years old (n = 337). Patients lacking age at diagnosis (n = 19) were subsequently removed from analysis. Among those 522 patients, 144 carry BRCA1 pathogenic variants and 84 BRCA2 pathogenic variants (44% of our cohort in total).
Patients were asked to answer a survey by phone. Briefly, this survey was made to allow the calculation of the number of menses for each patient so information on the reproductive pattern was collected. Because breast cancer risk may also include a genetic component, patients were asked about their BRCA1 and BRCA2 mutational status, if known. Lastly, family cancer records were assembled according to the relatedness degree and the tumor type.
In this study, we restrict the analysis to a sample of this cohort focusing on postmenopausal breast cancer risk. Therefore, the retrospective case–control analyses were done on postmenopausal women who declared their menopause as “natural” (as hysterectomy without oophorectomy can mimic menopause without blocking the ovarian function and treatments required further assumptions). Patients not reporting ages at menarche and menopause (n = 7) or lacking information on their menopausal status (n = 8) were removed from the analysis. Eight additional patients were removed when their cancer status (Yes/No) was missing or uncertain. The final sample size is n = 90 patients with 49 postmenopausal breast cancer cases and 41 postmenopausal cancer-free controls. Out of the 90 women in the sample, 5 patients were nulliparous and 1 patient had missing FFTP data. Therefore, they were not included in the analysis that focuses on the number of menses before FFTP.
Reproductive and menstrual variables, mutational status
As explained below, computing the number of menses each patient experienced required to compute the total length they spent in amenorrhea. Each patient’s reproductive history was divided into several “blocks” before reviewing the literature on whether those variables are associated with amenorrhea:
(i) “Pregnancy” we considered pregnancy to be 41 weeks long. Puerperium was considered to be between 6 and 8 weeks long as in Wang et al. (2015). We also considered that miscarriages and abortions were followed by a puerperium of the same length (n = 29 patients declared at least one abortion and n = 30 patients declared at least one miscarriage with two patients reporting a maximum of four events). Patients reported the breastfeeding time for each child. For patients that did not report any length, breastfeeding was considered to induce amenorrhea during a maximum of 6 months per breastfed child and a minimum of 0, assuming the breastfeeding was not exclusive (i.e., use of baby formula) therefore not inducing amenorrhea.
(ii) “Contraception” only hormonal means of contraception were considered. In our cohort, patients used either OCs or implants. If a patient started using hormonal contraception before her FFTP, we considered they used it continuously until this FFTP. This assumption of the model is, on the one hand, supported by the fact that OCs remain the majority contraceptive method in France and are usually used before the first pregnancy and, on the other hand, challenged by the fact that a substantial proportion of women stop taking OCs after 1 year (Vigoureux and Le Guen, 2018)
(iii) “Cycle” including age at menarche, age at menopause, overall regularity and average length of the cycle.
Genetic factors increasing breast cancer risk, such as BRCA pathogenic variants, were considered through a binary variable “mutational status” (Y/N). As shown by You et al. (2018), association of non-reproductive confounders (such as BMI or the living environment) with breast cancer incidence is no longer significant after normalizing by the birth rate. Moreover, BMI were shown to be non-significantly different between cases and controls (t = 1.2421, value of p = 0.22) and all patients live in the Montpellier area, in an environment that can be assumed to be uniform. Consequently, we chose to only conduct analyses taking into account the reproductive traits, excluding other life variables such as life environment, alcohol consumption or smoking.
Handling missing answers
In our reduced sample, some patients did not report their age at menarche (n = 1), their ages at menopause (n = 4), their average cycle length (n = 8), how long into the pregnancy before choosing an abortion (n = 28) and for how long they took OCs (n = 8). Reducing our analysis only to the complete cases would result in too much of a power loss. We therefore chose to fill in missing values using two different methods.
When either the age of menarche or the age of menopause was missing, sample mean values were used as a replacement (respectively, 12.8 and 45.8 yo). Using central measures to replace missing values of a variable relies on two assumptions: the variable sample distribution is symmetric and missing values are randomly scattered in the cohort. To test the first assumption, probability density functions were visually examined before replacement. Additionally, mean values were compared to values in the literature. On the one hand, mean age at menarche (12.8 in our sample vs. 12.6 in the literature) was consistent with literature (Rochebrochard De La, 1999). On the other hand, mean age at menopause was lower than estimates found in the literature (see, e.g., Soules et al., 2001; Weinstein et al., 2003). Lastly, this method was used in similar studies that also used central metrics as values for single-imputation (Clavel-Chapelon and E3N Group, 2002; Hartz and He, 2013; Khalis et al., 2018).
When a timespan (cycle length, abortion or OC) was missing, a range of plausible values was defined, assuming the distributions were normal. For the cycle average length and the OC, we choose the limit values so that 95% of the sample values belong to the range. For the time to abortion, the lower limit is also the 95% range lower limit but the upper limit is 12 weeks as current French regulation does not allow abortions later than that. This allowed to (i) limit result distortion (Houari et al., 2014) and (ii) to avoid making further biological assumptions.
General formula for the number of menses
Number of menstrual episodes were computed as follows:
For the number of menses before FFTP, the reproductive span is the length between menarche and FFTP and amenorrhea can only be due to OC. For the cumulative number of menses, the reproductive span is the time between menopause and menarche and amenorrhea can be due to pregnancy, breastfeeding or OC.
Statistical analyses
We first report the average numbers of menses and their standard deviations before testing whether the distributions of the lifetime number of menses and the distributions of the number of menses before FFTP are significantly different between breast cancer patients and controls using the Kolmogorov–Smirnov test and without adjusting for any confounders.
Because it cannot be assumed that controls will never develop breast cancer, survival analyses were conducted to allow for right-censoring. Here we focus on the influence of the cumulative number of menses on the occurrence of postmenopausal breast cancer. Therefore, we use the timespan between menopause and either age at diagnostic (cases) or age at the time of the study (controls) as a time scale to test for the influence of the lifetime number of menses and the number before FFTP. We first compare the Kaplan–Meier curves along the timescale and we then test for potential confounding effects using Cox models. The lifetime number of menses influence is tested along with the mutational status, the parity (Y/N), OC (Y/N), breastfeeding and age at recruitment. All the confounding variables are tested on the same baseline (p > 0.005). The number of menses before FFTP influence was tested along with the mutational status and two models were run, adjusting or not for the lifetime number of menses.
Continuous variables have been discretized before being tested in the models: as prescribed by Clark et al. (2003), cutoffs were non-informative (use of quantiles) and more than two categories were created. Two quantiles were used (pooled distribution and distribution in cases) so that comparative results give a first insight on the robustness of the results. All analyses were done in R 3.6.1 (R Core Team, 2020) with the package Survival (Therneau, 2020). Testing on the proportionality assumption using the cox.zph() function showed no violation of the assumption in either model.
Results
Description of the sample
Table 1 below shows how patient and control samples compare.
Lifetime menses and menses before first full-term pregnancy: Distribution comparison (midpoint of plausible ranges)
Considering OC use periods as part of time spent in amenorrhea, midpoint means (and standard deviations) for the number of cycles before FFTP were 16.68 (±6.92), 15.59 (±5.32), and 17.94 (±8.29) for pooled distribution, distributions in women with and without cancer, respectively. As seen on Figure 1, the distributions of the number of menses before FFTP are similar between patients with cancer and controls. The Kolmogorov–Smirnov test was not significant (value of p = 0.27). Regarding the lifetime number of menses, looking at the distribution of the midpoint values, means were 389.56 (±113.34), 419.94 (±108.12), 353.25 (±109.87) in the pooled sample, in cancer cases and in controls. The Kolmogorov–Smirnov test was significant (value of p<0.05).
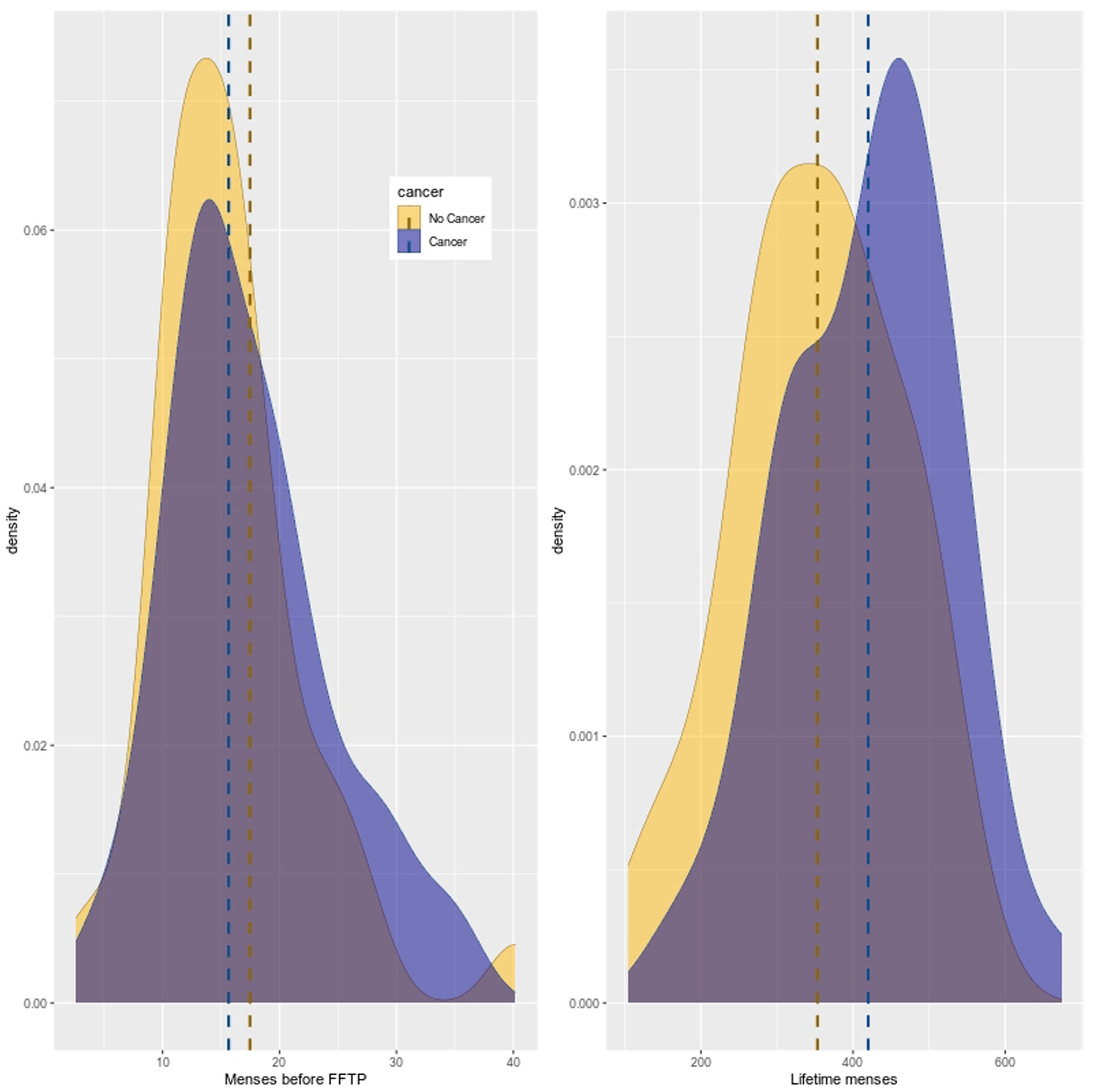
Figure 1. Distributions of the number of menses before FFTP and the lifetime number of menses. The distribution of menses before FFTP is similar between cases and controls but distribution of lifetime number of menses is not.
Latency in onset of postmenopausal breast cancer: Cox models (median and limit values in ranges)
To test whether more lifetime menses and more menses before FFTP are associated with an early onset of cancer after menopause compared to controls, we first compared the lifetime number of menses between patients with cancer and controls. As seen on Figure 2, the Kaplan–Meier curves are different: the purple curve is skewed on the right for cancer patients as they experience more menses during their reproductive lifespans (x axis represents the lifetime number of menses). Then, we performed Cox model analyses with the timespan between menopause and either age at diagnosis or age at recruitment (right-censoring) as the timescale. To account for uncertainties in the number of menses, we ran the models with different values (lower and upper limits of plausible range and midpoint values).
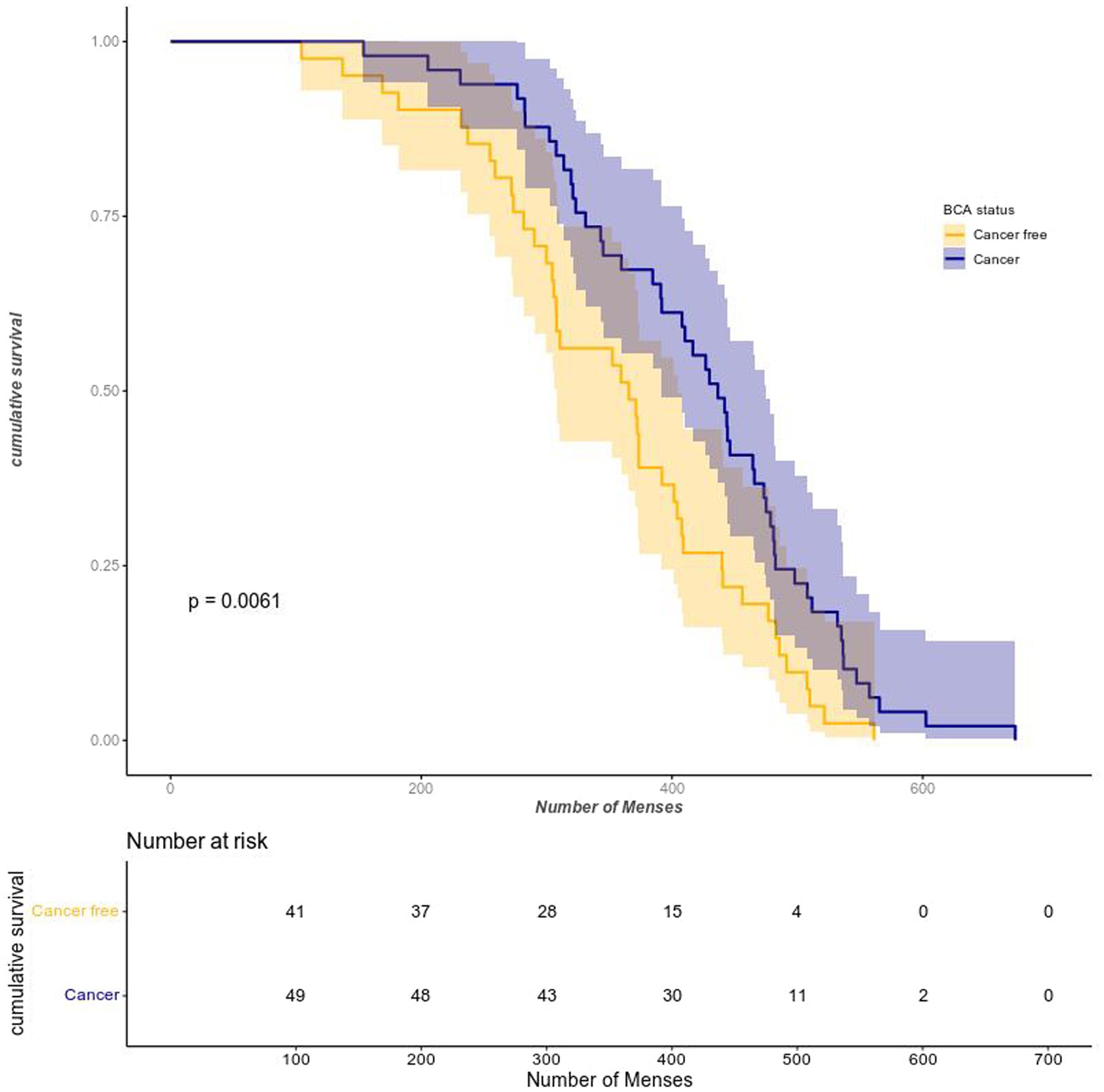
Figure 2. Survival as a function of the lifetime number of episodes. The Kaplan–Meier shows that without correcting for any cofactors, cancer patients experienced more lifetime menses than controls. Please note that the horizontal axis represents the lifetime number of menses, not chronological time. BCA status = Breast CAncer.
Breast cancer risk according to three levels of the lifetime number of menses (“low” [104;381], “middle” [381;475] and “high” [475;674], computed as the three thirds of the midpoint number distribution in cases. Note that the range notation here shows that patients with the upper limit number of menses for a given range are included in the following range, hence the open bracket) was first tested. Having experienced more menses is positively associated with risk of postmenopausal breast cancer (HR = 1.66 CI95%[0.83;3.33] and HR = 2.24 CI95%[1.13;4.45] for “middle” and “high” levels, respectively) but only the association with the higher category was significant (p < 0.05). Additionally, mutational status was added as a confounder and did not yield different results (see Supplementary material S1). When adjusted for OC (Y/N), parity (Y/N), breastfeeding (Y/N) and cycle regularity (Y/N), the model yielded the same results (see Supplementary material S2A).
When the plausible range limit values are used, results are less straightforward. The upper limit values yield that only the “middle” category of lifetime number of menses (Supplementary material S2B) is significantly associated and the lower limit values do not significantly associate any confounders with the latency between menopause and breast cancer diagnosis (Supplementary material S2C). In the same manner, models using the pooled distribution of the number of menses yielded contrasted results (Supplementary material S4) depending on whether the midpoint or the range limit values were assayed. This shows that our results are i) sensitive to the cutoffs and ii) sensitive to the hypotheses made to compute the ranges.
Regarding the number of menses before FFTP, regardless of whether the lifetime of menses was included, both models yielded the same trend: although the category “high” was positively associated, the value of p was not significant. Those results are similar to both Clavel-Chapelon and E3N Group (2002) and Chavez-MacGregor et al. (2005): a positive trend exists but is not significant.
Discussion
The work presented here investigated the existence of a reproductive mismatch: in postmenopausal women, the risk of breast cancer is increased by greater past exposure to cyclic hormones. We investigated this question using data from a cohort of 90 women drawn from an at-risk population with heavy cancer family history. For each subject, a range of plausible values for the number of menses was computed by considering information on menstrual and reproductive histories.
It has been hypothesized that before the first full-term pregnancy (FFTP), breast cells are highly susceptible to proliferative hormones (i.e., estrogens). Since the question of whether more numerous menses before FFTP is associated with an increase in breast cancer risk is still debated [compare, e.g., Eaton et al. (1994) approach with results reviewed by Persson (2000)], we also investigated how significantly those menses were associated with cancer risk in our cohort. All subjects lived in the area around Montpellier, France, were recruited in the same hospital, surveyed by the same person and had family history of cancer. Therefore, only reproductive confounders have been used in the survival models presented here.
Overall, all the models testing the cumulative number of menses pointed towards the same results: only the lifetime number of menses was positively and significantly associated with a shorter timespan between menopause and cancer diagnosis. This suggests that a greater lifetime exposure to cyclic hormones reduces the latency of breast cancer onset after menopause. On the other hand, the models testing the number of menses before the FFTP yielded a positive but non-significant trend. This results for the number of menses before FFTP should be considered taking into account at least two potential source of bias: i) cycles in the few years after the menarche can be anovulatory (hence, we cannot conclude on the “true” exposure to cyclic hormones) and ii) we assumed that, if patients started taking OCs before the FFTP, they continuously took them. It reduces the number of menses before FFTP and leads to very large ranges of plausible numbers.
This work builds up on the work conducted on large prospective cohorts by Clavel-Chapelon and E3N Group (2002) and Chavez-MacGregor et al. (2005). It has the novelty of investigating how the risk pattern linked to menstrual and reproductive histories will be affected in the context of a population selected based on heavy familial history. It also answers another question: do women who experienced more menses also get breast cancer more quickly after menopause?
Regarding the total number of menses, again, we obtained comparable results to both studies. We found that only the highest range of menses had a significant association. This supports the hypothesis of Chavez-MacGregor et al. (2005) that the risk is likely shaped as a threshold function rather than linear. The similarity in risk pattern between a cohort from the general population and our sample with patients at high familial risk suggests that a high family history burden is not the major risk factor, at least not compared to menstrual and reproductive histories. This is, at first sight, supported by the non-significant positive association of BRCA pathogenic variants with breast cancer risk in our cohort. However, that non-significance can also be the result of a lack of statistical power, so further study on larger sample sizes is required. It also fits well with Genome-Wide Association Studies (GWAS) showing that loci associated with an increased breast cancer risk are not associated with reproductive traits (Warren Andersen et al., 2014). Additionally, the number of menses before FFTP is not significantly associated with the risk despite a positive trend: this advocates even more in favor of this idea that elements of menstrual and reproductive histories influence breast cancer risk in synergy.
Interestingly, the risk pattern was similar in our work compared to the literature despite some differing assumptions. In previous work, whether researchers were counting ovulatory or anovulatory was uncertain. For instance, counting menses during OC treatment suggests a focus on all exposures (not only exposure due to complete ovulatory cycles) but considering puerperium in amenorrhea is considering the cessation of ovarian function. Here, we tried to consider only ovulatory cycles. Because the “true” number of ovulatory cycles was not accessible, we computed a range. It has the advantage of avoiding the need for some biological assumptions, for instance the fraction of ovulatory cycles in the few years before menopause. On the other hand, it also shifted the focus towards endogenous hormones and did not allow us to answer whether OCs protective (and whether, as suggested by Britt and Short (2012), it should be given to nuns as a preventive treatment) or associated to a slight increase in breast cancer risk (Mørch et al., 2017).
First of all, the data required missing value replacement due to the low sample size not allowing for removal. Two strategies have been adopted: mean and interval substitutions. Both strategies assume the same thing: data are missing at random. As explained by Acock (2005), missing data arising from individuals refusing or failing to complete surveys is not entirely random. In our dataset, some of the surveys have been completed by family members of the sampled women. Therefore, we cannot ensure that all missing data are at random. Mean replacement is the simplest way of dealing with missing data but assumes a symmetrical distribution. Additionally, it adds information by increasing the sample size and distorts the distribution by reducing the variance. Assumptions were met for the age at menarche. Regarding age at menopause, it was less straightforward. Interval replacement assumes that data are normally distributed. Once again, this would need to be more thoroughly assessed.
Second, this work presented results obtained on a relatively small sample size. Because Cox models require at least 10 cases per group to yield a decent statistical power (Bradburn et al., 2003), the number of covariates that could be adjusted for in the same model was limited. Lastly, the different models were not as robust as the ones found in literature: p-values were sensitive to covariate discretization (using quantiles from either the pooled or the cases distributions).
Third, the work presented here is an application of a prospective model to retrospective data. We computed the number of menses the same way as others did but all subjects who had cancer had it beforehand. Consequently, we cannot ensure the absence of recall bias: medical history has been shown to influence the way individuals remember events. Additionally, women answered about distant past events (Skegg, 1988). The Collaborative Group on Hormonal Factors in Breast Cancer (2012) found evidence that women’s answers tend to regress to the mean with further time from the event. Hence, we cannot ensure the absence of recall errors either.
Ultimately, our analysis considered molecular subtypes of cancer altogether whereas reproductive and menstrual traits have been shown to be positively and significantly associated with breast cancer risk for specific molecular subtypes (Aktipis et al., 2014).
This work suggests that the lifetime number of menses is associated with an increased risk of developing breast cancer more quickly after menopause in women at high familial risk. Therefore, high familial risk could be modulated by the reproductive history and the interplay between genetic and reproductive factors should be further studied.
Data availability statement
The datasets presented in this article are not publicly available as they contain private and medical information. Requests to access the datasets should be directed to margaux.bieuville@cri-paris.org; p-pujol@chu-montpellier.fr; v-galibert@chu-montpellier.fr.
Ethics statement
The studies involving human participants were reviewed and approved by the Ethical Committee of the Centre Hospitalier Universitaire de Montpellier. The patients/participants provided their written informed consent to participate in this study.
Author contributions
MB conducted the statistical analyses. PP, VG, MH, and DF collected the data. BR and FT designed the question and supervised the project. All authors participated in the manuscript writing and approved the final version of the manuscript.
Funding
This work was supported by an ANR TRANSCAN (ANR-18-CE35-0009), the MAVA Foundation, the Rotary Club Les Sables d’Olonne, an ARC Linkage (LP170101105), Deakin SEBE_RGS_2019 and a CNRS International Associated Laboratory Grant.
Conflict of interest
The authors declare that the research was conducted in the absence of any commercial or financial relationships that could be construed as a potential conflict of interest.
Publisher’s note
All claims expressed in this article are solely those of the authors and do not necessarily represent those of their affiliated organizations, or those of the publisher, the editors and the reviewers. Any product that may be evaluated in this article, or claim that may be made by its manufacturer, is not guaranteed or endorsed by the publisher.
Supplementary material
The Supplementary material for this article can be found online at: https://www.frontiersin.org/articles/10.3389/fevo.2023.912083/full#supplementary-material
References
Acock, A. C. (2005). Working with missing values. J. Marriage Fam. 67, 1012–1028. doi: 10.1111/j.1741-3737.2005.00191.x
Aktipis, C. A., Ellis, B. J., Nishimura, K. K., and Hiatt, R. A. (2014). Modern reproductive patterns associated with estrogen receptor positive but not negative breast cancer susceptibility. Evol. Med. Public Health 2015, 52–74. doi: 10.1093/emph/eou028
Aktipis, C. A., and Nesse, R. M. (2013). Evolutionary foundations for cancer biology. Evol. Appl. 6, 144–159. doi: 10.1111/eva.12034
Allender, S., Foster, C., Hutchinson, L., and Arambepola, C. (2008). Quantification of urbanization in relation to chronic diseases in developing countries: a systematic review. J. Urban Health 85, 938–951. doi: 10.1007/s11524-008-9325-4
Bradburn, M. J., Clark, T. G., Love, S. B., and Altman, D. G. (2003). Survival analysis part III: multivariate data analysis – choosing a model and assessing its adequacy and fit. Br. J. Cancer 89, 605–611. doi: 10.1038/sj.bjc.6601120
Britt, K., and Short, R. (2012). The plight of nuns: hazards of nulliparity. Lancet 379, 2322–2323. doi: 10.1016/S0140-6736(11)61746-7
Chavez-MacGregor, M., Elias, S. G., Onland-Moret, N. C., van der Schouw, Y. T., Van Gils, C. H., Monninkhof, E., et al. (2005). Postmenopausal breast cancer risk and cumulative number of menstrual cycles. Cancer Epidemiol. Biomark. Prev. 14, 799–804. doi: 10.1158/1055-9965.EPI-04-0465
Clark, T. G., Bradburn, M. J., Love, S. B., and Altman, D. G. (2003). Survival analysis part IV: further concepts and methods in survival analysis. Br. J. Cancer 89, 781–786. doi: 10.1038/sj.bjc.6601117
Clavel-Chapelon, F., E3N Group (2002). Cumulative number of menstrual cycles and breast cancer risk: results from the E3N cohort study of French women. CCC 13, 831–838. doi: 10.1023/a:1020684821837
Coe, K., and Steadman, L. B. (1995). The human breast and the ancestral reproductive cycle: a preliminary inquiry into breast cancer etiology. Hum. Nat. 6, 197–220. doi: 10.1007/BF02734139
Collaborative Group on Hormonal Factors in Breast Cancer (2012). Menarche, menopause, and breast cancer risk: individual participant meta-analysis, including 1,18,964 women with breast cancer from 117 epidemiological studies. Lancet Oncol. 13, 1141–1151. doi: 10.1016/S1470-2045(12)70425-4
Eaton, S. B., Pike, M. C., Short, R. V., Lee, N. C., Trussell, J., Hatcher, R. A., et al. (1994). Women's reproductive cancers in evolutionary context. Q. Rev. Biol. 69, 353–367. doi: 10.1086/418650
Hartz, A. J., and He, T. (2013). Cohort study of risk factors for breast cancer in post menopausal women. Epidemiol. Health 35:3003. doi: 10.4178/epih/e2013003
Hochberg, M. E., and Noble, R. J. (2017). A framework for how environment contributes to cancer risk. Ecol. Lett. 20, 117–134. doi: 10.1111/ele.12726
Houari, R., Bounceur, A., Tari, A. K., and Kecha, M. T. (2014). Handling missing data problems with sampling methods. Int. Conf. Adv. Netw. Distrib. Syst. Applic. 2014, 99–104. doi: 10.1109/INDS.2014.25
Khalis, M., Charbotel, B., Chajès, V., Rinaldi, S., Moskal, A., Biessy, C., et al. (2018). Menstrual and reproductive factors and risk of breast cancer: a case-control study in the fez region, Morocco. PLoS One 13:e0191333. doi: 10.1371/journal.pone.0191333
Kuchenbaecker, K. B., Hopper, J. L., Barnes, D. R., Phillips, K. A., Mooij, T. M., Roos-Blom, M. J., et al. (2017). Risks of breast, ovarian, and contralateral breast cancer for BRCA1 and BRCA2 mutation carriers. JAMA 317, 2402–2416. doi: 10.1001/jama.2017.7112
Lopez, R. P., and Hynes, H. P. (2006). Obesity, physical activity, and the urban environment: public health research needs. Environ. Health 5:25. doi: 10.1186/1476-069X-5-25
Lovett, J. L., Chima, M. A., Wexler, J. K., Arslanian, K. J., Friedman, A. B., Yousif, C. B., et al. (2017). Oral contraceptives cause evolutionarily novel increases in hormone exposure: a risk factor for breast cancer. Evol. Med. Public Health 2017, 97–108. doi: 10.1093/emph/eox0009
MacMahon, B., Cole, P., Lin, T. M., Lowe, C. R., Mirra, A. P., Ravnihar, B., et al. (1970). Age at first birth and breast cancer risk*. Bull. World Health Organ. 43, 209–221. Available at: https://apps.who.int/iris/handle/10665/262427.
Manson, J. E., Chlebowski, R. T., Stefanick, M. L., Aragaki, A. K., Rossouw, J. E., Prentice, R. L., et al. (2013). Menopausal hormone therapy and health outcomes during the intervention and extended poststopping phases of the Women's Health Initiative randomized trials. JAMA 310, 1353–1368. doi: 10.1001/jama.2013.278040
Mørch, L., Skovlund, C., Hannaford, P., Iversen, L., Fielding, S., and Lidegaard, Ø. (2017). Contemporary hormonal contraception and the risk of breast cancer. N. Engl. J. Med. 377, 2228–2239. doi: 10.1056/nejmoa1700732
National Cancer Institute. (2018). Oral contraceptives (birth control pills) and cancer risk. National Cancer Institute. United States government. Available at: https://www.cancer.gov/about-cancer/causes-prevention/risk/hormones/oral-contraceptives-fact-sheet (Accessed. February, 2019).
Nesse, R. M. (2011). Ten questions for evolutionary studies of disease vulnerability. Evol. Appl. 4, 264–277. doi: 10.1111/j.1752-4571.2010.00181.x
Nesse, R. M., and Stearns, S. C. (2008). The great opportunity: evolutionary applications to medicine and public health. Evol. Appl. 1, 28–48. doi: 10.1111/j.1752-4571.2007.00006.x
Odes, E. J., Randolph-Quinney, P. S., Steyn, M., Throckmorton, Z., Smilg, J. S., Zipfel, B., et al. (2016). Earliest hominin cancer: 1.7-million-year-old osteosarcoma from Swartkrans cave, South Africa. S. Afr. J. Sci. 112:471. doi: 10.17159/sajs.2016/20150471
Persson, I. (2000). Estrogens in the causation of breast, endometrial and ovarian cancers – evidence and hypotheses from epidemiological findings. J. Steroid Biochem. Mol. Biol. 74, 357–364. doi: 10.1016/s0960-0760(00)00113-8
Prates, C., Sousa, S., Oliveira, C., and Ikram, S. (2011). Prostate metastatic bone cancer in an Egyptian Ptolemaic mummy, a proposed radiological diagnosis. Int. J. Paleopathol. 1, 98–103. doi: 10.1016/j.ijpp.2011.09.002
R Core Team (2020). R: A language and environment for statistical computing. R Foundation for Statistical Computing, Vienna, Austria. Available at: https://www.R-project.org/
Rochebrochard De La, E. (1999). La puberté des filles et des garçons en France, (in French:) INED – French Institute for Demographic Studies (in English). Population 54, 933–962. https://hal.archives-ouvertes.fr/hal-02270381
Skegg, D. C. (1988). Potential for bias in case-control studies of oral contraceptives and breast cancer. Am. J. Epidemiol. 127, 205–212. doi: 10.1093/oxfordjournals.aje.a114796
Soules, M. R., Sherman, S., Parrott, E., Rebar, R., Santoro, N., Utian, W., et al. (2001). Executive summary: stages of reproductive aging workshop (STRAW). Fertil. Steril. 76, 874–878. doi: 10.1016/s0015-0282(01)02909-0
Strassmann, B. I. (1997). The biology of menstruation in homo sapiens: Total lifetime menses, fecundity, and non-synchrony in a natural-fertility population. Curr. Anthropol. 38, 123–129. Available at: http://www.jstor.org/stable/2744446. doi: 10.1086/204592
Strassmann, B. I. (1999). Menstrual cycling and breast cancer: an evolutionary perspective. J. Women's Health 8, 193–202. doi: 10.1089/jwh.1999.8.193
Terry, M. B., Liao, Y., Kast, K., Antoniou, A. C., and McDonald, J. A., Mooij, [...] EMBRACE, GENEPSO, BCFR, HEBON, kConFab and IBCCS (2018). The influence of number and timing of pregnancies on breast cancer risk for women with BRCA1 or BRCA2 mutations. JNCI Cancer Spect. 2:78. doi: 10.1093/jncics/pky078
Therneau, T. (2020). A package for survival analysis in R package version 3.1-12. Available at: https://CRAN.R-project.org/package=survival
Vigoureux, S., and Le Guen, M. (2018). Contexte de la contraception en France. RPC Contraception CNGOF. Gynécologie Obstétrique Fertilité Sénologie 46, 777–785. doi: 10.1016/j.gofs.2018.10.005
Wang, Z., Risch, H., Lu, L., Irwin, M. L., Mayne, S., Schwartz, P., et al. (2015). Joint effect of genotypic and phenotypic features of reproductive factors on endometrial cancer risk. Sci. Rep. 5:15582. doi: 10.1038/srep15582
Warren Andersen, S., Trentham-Dietz, A., Gangnon, R. E., Hampton, J. M., Skinner, H. G., Engelman, C. D., et al. (2014). Breast cancer susceptibility loci in association with age at menarche, age at natural menopause and the reproductive lifespan. Cancer Epidemiol. 38, 62–65. doi: 10.1016/j.canep.2013.12.001
Weinstein, M., Gorrindo, T., Riley, A., Mormino, J., Niedfeldt, J., Singer, B., et al. (2003). Timing of menopause and patterns of menstrual bleeding. Am. J. Epidemiol. 158, 782–791. doi: 10.1093/aje/kwg223
You, W., and Henneberg, M. (2018). Prostate cancer incidence is correlated to Total meat intake– a cross-National Ecologic Analysis of 172 countries. Asian Pac. J. Cancer Prev. 19, 2229–2239. doi: 10.22034/APJCP.2018.19.8.2229
Keywords: evolutionary mismatch, breast cancer, reproductive pattern, survival models, menopause
Citation: Bieuville M, Faugère D, Galibert V, Henard M, Dujon AM, Ujvari B, Pujol P, Roche B and Thomas F (2023) Number of lifetime menses increases breast cancer occurrence in postmenopausal women at high familial risk. Front. Ecol. Evol. 11:912083. doi: 10.3389/fevo.2023.912083
Edited by:
Elise Huchard, UMR5554 Institut des Sciences de l'Evolution de Montpellier (ISEM), FranceReviewed by:
Wenjuan Kang, Tianjin Medical University Cancer Institute and Hospital, ChinaFrank Stanczyk, University of Southern California, United States
Edson Zangiacomi Martinez, University of São Paulo, Brazil
Copyright © 2023 Bieuville, Faugère, Galibert, Henard, Dujon, Ujvari, Pujol, Roche and Thomas. This is an open-access article distributed under the terms of the Creative Commons Attribution License (CC BY). The use, distribution or reproduction in other forums is permitted, provided the original author(s) and the copyright owner(s) are credited and that the original publication in this journal is cited, in accordance with accepted academic practice. No use, distribution or reproduction is permitted which does not comply with these terms.
*Correspondence: Frédéric Thomas, ✉ frederic.thomas2@ird.fr; Benjamin Roche, ✉ benjamin.roche@ird.fr