- 1Department of Biology Western Washington University, Bellingham, WA, United States
- 2School of Marine Science and Policy, College of Earth, Ocean, and Environment, University of Delaware, Lewes, DE, United States
- 3Halmos College of Arts and Sciences, Nova Southeastern University, Dania Beach, FL, United States
- 4Delaware Sea Grant College of Earth, Ocean, and Environment, University of Delaware, Lewes, DE, United States
Using tags within a mark-recapture framework allows researchers to assess population size and connectivity. Such methods have been applied in coastal zone habitats to monitor salt marsh restoration success by comparing the movement patterns of Mummichogs (Fundulus heteroclitus) between restored and natural marshes. Visible Implant Elastomer (VIE) tags are commonly used to tag small fish like Mummichogs, though the retention and survival of small fish using this method varies between studies, producing uncertainty during mark-recapture-based approaches. To address this, we conducted a laboratory experiment to determine the rate of tag loss and mortality of VIE tags on Mummichogs of two size classes (greater or less than 61 mm) and across different taggers. Tag loss and mortality increased over time, and the latter significantly varied between taggers. We then developed a predictive model, R package ‘retmort’, to account for the effect of this increase on mark-recapture studies. When adapted to a series of published works, our model provided rational estimates of tagging error for multiple species and tagging methods. Of the case studies the model was applied to (n = 26), 15 resulted in a percent standard error greater than 5%, signaling a significant percent of error due to uncounted, tagged animals. By not accounting for these individuals, recapture studies, particularly those that assess restoration efforts and coastal resilience, could underestimate the effects of those projects, leading to superfluous restoration efforts and erroneous recapture data for species with low tag retention and high mortality rates.
1 Introduction
Since the 17th century, researchers have utilized tagging methods to estimate population size and understand animal spatial behavior for assessing fisheries, habitat restoration efforts, and land-use management (Lucas and Baras, 2000; Murray and Fuller, 2000; Walker et al., 2012; Lapointe et al., 2013). Tagging takes a variety of forms, from simple surface markings (e.g., freeze branding) to more complex, electronic tags used to track movements and habitat use across scales (e.g., acoustic tags, satellite tags; Lucas and Baras, 2000; Roday et al., 2024). In fisheries, conventional tagging, or the use of physical identification tags attached to the fish, is commonly used to answer questions of abundance, movement, survival, and growth in natural settings (Lucas and Baras, 2000; Hale et al., 2016; Sandford et al., 2020). Additionally, applying these techniques to modeling frameworks, such as mark-recapture methods (Otis et al., 1978), allows researchers to assess population size (e.g., Haines and Modde, 1996) and connectivity (e.g., Rogers et al., 2014). Within coastal zones, mark-recapture models have been used to study key species like Blue Crab (Callinectes sapidus) and Mummichogs (Fundulus heteroclitus) (e.g., Etherington et al., 2003; Teo and Able, 2003), which provide important commercial and ecological functions. Mummichogs, a forage fish found in salt marshes throughout the Mid-Atlantic coast, are crucial to ecosystem functioning in coastal estuaries, providing a trophic link between the subtidal and intertidal sections of salt marshes through their movement and foraging behaviors (Nixon and Oviatt, 1973; Valiela et al., 1977; Weisberg and Lotrich, 1982; Deegan et al., 2000; Kneib, 2000; Currin et al., 2003). The Mummichog is an important species to study when monitoring habitat quality following changes in coastal ecosystems due to their high abundance in salt marsh habitats (Lotrich, 1975), ecological importance (Teo and Able, 2003; McGowan et al., 2022), and their value as an indicator species for monitoring coastal communities (e.g., Teo and Able, 2003; Crum et al., 2018).
A challenge in tagging small forage fish, like the Mummichog, is ensuring the behavior or physiological functioning of the animal is not affected by the tagging procedure (Lucas and Baras, 2000). Ideally, the perfect tag would be inexpensive, small, have no impacts on animal health or behavior, and have 100% retention, yet no such tag has been developed (Lucas and Baras, 2000). In lieu of such a tag, researchers settle for choosing the most appropriate method while considering study species, the typical size of the fish, the duration of the project, and the objectives (Lucas and Baras, 2000; Sandford et al., 2020). Users must assume the retention, survivability, and readability of markers during tagging studies, potentially limiting predictive power by introducing sampling error. Not properly accounting for lost tags can be problematic for restoration efforts where misrepresentation of a local population can lead to a reduction in the effectiveness of a restoration project.
Visible Implant Elastomer (VIE) tags have become popular among researchers focusing on small fish while conducting relatively short studies (e.g., Griffiths, 2002; Leblanc and Noakes, 2012). VIE tagging procedure uses widely available insulin syringes to implant a liquid elastomer resin which quickly hardens to form a biocompatible, flexible, and colored (e.g., red, yellow, etc.) mark that can be seen through the skin (Frederick, 1997; Griffiths, 2002; Sandford et al., 2020). VIE tags are inexpensive, have little to no effect on growth, and do not require individuals to be sacrificed for tag retrieval (Griffiths, 2002; Josephson et al., 2008; Sandford et al., 2020).
Despite the relative utility and effectiveness of VIE tags, limitations persist. Two well-documented complications involved with VIE tagging, aside from not being a unique identifierand relying on a limited arrangement of colors and locations, are high variability in tag retention and tagging procedure-induced mortality. While many studies reported nearly full retention and insignificant mortality (FitzGerald et al., 2004; Skinner et al., 2006; Josephson et al., 2008; Leblanc and Noakes, 2012; Bangs et al., 2013; Neufeld et al., 2015; Eissenhauer et al., 2024), some saw intermediate levels of tag retention and mortality (Griffiths, 2002; Bolland et al., 2009; Close and Jones, 2002; Brannelly et al., 2013; Jungwirth et al., 2019; Moore and Brewer, 2021), and others demonstrated low retention rates and/or high levels of mortality (Reeves and Buckmeier, 2009; Fraiola and Carlson, 2016; Cabot et al., 2021).
There are many factors that impact tag retention and the survival of tagged organisms including species (e.g., Reeves and Buckmeier, 2009), size (e.g., Frederick, 1997), study duration (e.g., Haines et al., 1998), tag color (e.g., Haines et al., 1998; Jungwirth et al., 2019), the number of taggers (e.g., Eissenhauer et al., 2024), tag location (e.g., Olsen et al., 2004; Reeves and Buckmeier, 2009; Fraiola and Carlson, 2016), the usage of anesthesia (e.g., Moore and Brewer, 2021 in contrast to Frederick, 1997), predation on tagged fish (Catalano et al., 2001), and whether it is a field or lab study (e.g., varying light conditions as described in Josephson et al., 2008). It is crucial to carefully consider the effect of the above factors on a study’s results before its conception, as this will aid in deciding which tag is most appropriate (Neufeld et al., 2015; Sandford et al., 2020).
The limitations and species-specific variability in retention and survival are also commonly observed in other popular tagging methods, including passive integrated transponder (PIT) tags (i.e., Kimball and Mace, 2020; Moore and Brewer, 2021), acoustic tags (i.e. Bégout Anras et al., 2003; Wilder et al., 2016), and coded-wire tags (i.e. Ashton et al., 2014; Teo and Able, 2003). Hence, there is a need to account for species-specific and size-dependent variability in tagging effects to reduce sampling error in mark-recapture efforts. In addition, this information is also needed to meet the assumptions for closed-population models assessing abundance, where mortality is assumed to be zero (Otis et al., 1978).
To address the issues of mortality, tag retention, and tag misidentification of VIE and to improve the accuracy of field applications, we conducted a laboratory experiment to study the tag loss and mortality of Mummichogs of various sizes and across multiple people tagging (hereafter taggers). Using the results of this laboratory study, the retention-mortality model, ‘retentionmort’, was created to predict and account for error associated with tagging efforts over a five-week period. We then adapted and applied our model to a series of published tag retention studies to determine its ability to estimate tagging-related mortality and tag loss for several tagging methods and species of interest. The goal of the model is to identify the points at which the number of tagged individuals at large, recaptures, and tagging efforts result in the error in the observed number of recaptures exceeding a certain threshold (e.g., 5%, 10%, 15%, and 20%). We will then discuss the value of this product to facilitate method development in field-based mark-recapture studies and produce hindcast data adjustments to account for tagging-related errors in completed studies. An emphasis will be placed on tagging studies with low tag retention and survival post-tagging. With the ability to adequately account for tagged individuals that are missing because of tag loss or mortality, our model provides a method to improve the precision of monitoring coastal habitats, fisheries, and restoration efforts.
2 Methods
2.1 Laboratory evaluation of VIE tag loss and survival
To improve the assessment of fish recaptures using elastomer tags on small Mummichogs, the tagging-related mortality and tag loss rates across varying size classes of fish were assessed in a laboratory study. We gathered Mummichogs (n = 68) using eel traps in Canary Creek, DE (38.77899° N, 75.16461° W) during May of 2023. The portion of the creek we sampled is centered among a linear stretch with a tidally driven depth ranging from nearly 0–2 m and is approximately 3 m wide. Captured fish were held across 2 recirculating systems, each consisting of 4, 40L aerated tanks with PVC structures to serve as artificial habitat for enrichment, and a 120L head tank (280 L total per system) consisting of biomedia and particle filtration, where fish were acclimated for 1–2 weeks at a density of less than 10 fish per 40L tank (maximum 40 fish per system) prior to experimentation to acclimate to laboratory conditions. Fish were fed daily, ad-libitum with a variety of bait fish similar to the variety they would be exposed to in natural settings. Any uneaten food was siphoned from the tanks after an approximate 4-hour feeding window and any removed water was refilled into the head tank. Tanks were given partial water changes at least weekly, as needed. These fish were measured, then categorized into two size bins: small fish that were less than 61 mm (average = 51.87 mm, range = 45 - 60 mm, n = 31) and large fish that were greater than or equal to 61 mm (average = 68.56 mm, range = 61–80 mm, n = 37) and separated among the two recirculating tank systems. Our size bins were determined by the median length of all captured fish in our sampling location at Canary Creek, DE and have a similar range of ‘small’ and ‘large’ individuals as Kneib (1986, small is considered less than 50 mm) and Teo and Able (2003, large is considered above 60 mm). For each size bin, subsets of fish were tagged in the caudal peduncle (small = 12 tagged, 19 untagged; large = 24 tagged, 13 untagged) using a VIE tag (Northwest Marine Technology, Inc., Anacortes, Washington). Within each size class, and respective recirculating system, the total number of fish was kept even between the separate tanks ± 2 fish, and we randomized the number of tagged and untagged fish added to each tank. Four taggers, each using a unique tag color (red, blue, pink, green), were responsible for tagging to track variability in retention and survival among taggers. In doing this, we delineated the potential for misidentifying-colored tags in a field application with multiple taggers. The fish were then monitored weekly over three weeks for tag loss and survival. The use of live animals was carried out under the University of Delaware Institutional Animal Care and Use Committee (AUP #: 1394-2022-A). Temperature, salinity, dissolved oxygen, and pH were recorded for individual tanks during the experiment and at the location animals were obtained using a handheld YSI water quality meter (Xylem©, Yellow Springs, Ohio, USA). Temporal changes in water quality were assessed using a Mann-Kendall trend test and comparisons of water quality between tanks were made using non-parametric Kruskal-Wallis tests to determine potential confounding factors in tag loss and fish survival using an alpha value equal to 0.05 for statistical inference.
Each week, all fish were temporarily removed from the tank, effectively performing a recapture event, and assessed for the presence or absence of a tag and the color of a tag then were placed back into their original tank. The same person checked for recaptures every week. If there was a sign of infection (noted as a discoloration, dermal film, or large sores), lethargic swimming behavior, or injury observed, we removed those animals from the experiment and considered the removed animals as a mortality, assuming their survival in a field application would be lower. Weekly, the survival, presence, absence, and tag color data were collected for each tank. These data were then used to estimate weekly mortality and tag loss (defined as tags shed + misidentified tags/total remaining live tagged fish). Shed and misidentified tags were manually counted at the end of the study by identifying missing tags or incorrect colors, respectively, between the original stocking and each weekly recapture event after accounting for mortality. Our definition of ‘tag loss’ loosely follows that of ‘daily retention’ where “only fish that were still alive were used” in the retention calculation,” from Archdeacon et al. (2009). We then summed the cumulative mortality and number of lost tags between size classes and tag color to develop an average tag loss rate and mortality rate over the length of the experiment. Mortality and tag loss rates were calculated by performing a linear regression of the summed tag loss and mortality per week, and a line of best fit was formed. The slope and intercept of the survival rate (mS, βS, respectively) and tag loss rate (mM, βM, respectively) formed by the line of best fit was used to model the reduction in tagged fish over time at large (t weeks; Equations 1a, b, 2a, b, Table 1). Most equations are duplicated across small (denoted by a lowercase ‘s’ subscript) and large (denoted by a lowercase ‘l’ subscript) fish before producing final summations in the succeeding equations. Using the variance in retention and survival across the four taggers, two standard deviations about the average tag loss and mortality were taken to derive a 95% confidence interval among tag loss and mortality rates. The resulting series of equations were then used as the basis for three predictive models (referred to as low-error, average, and high-error) that predict the range of error among field observations at a known time at large, t.
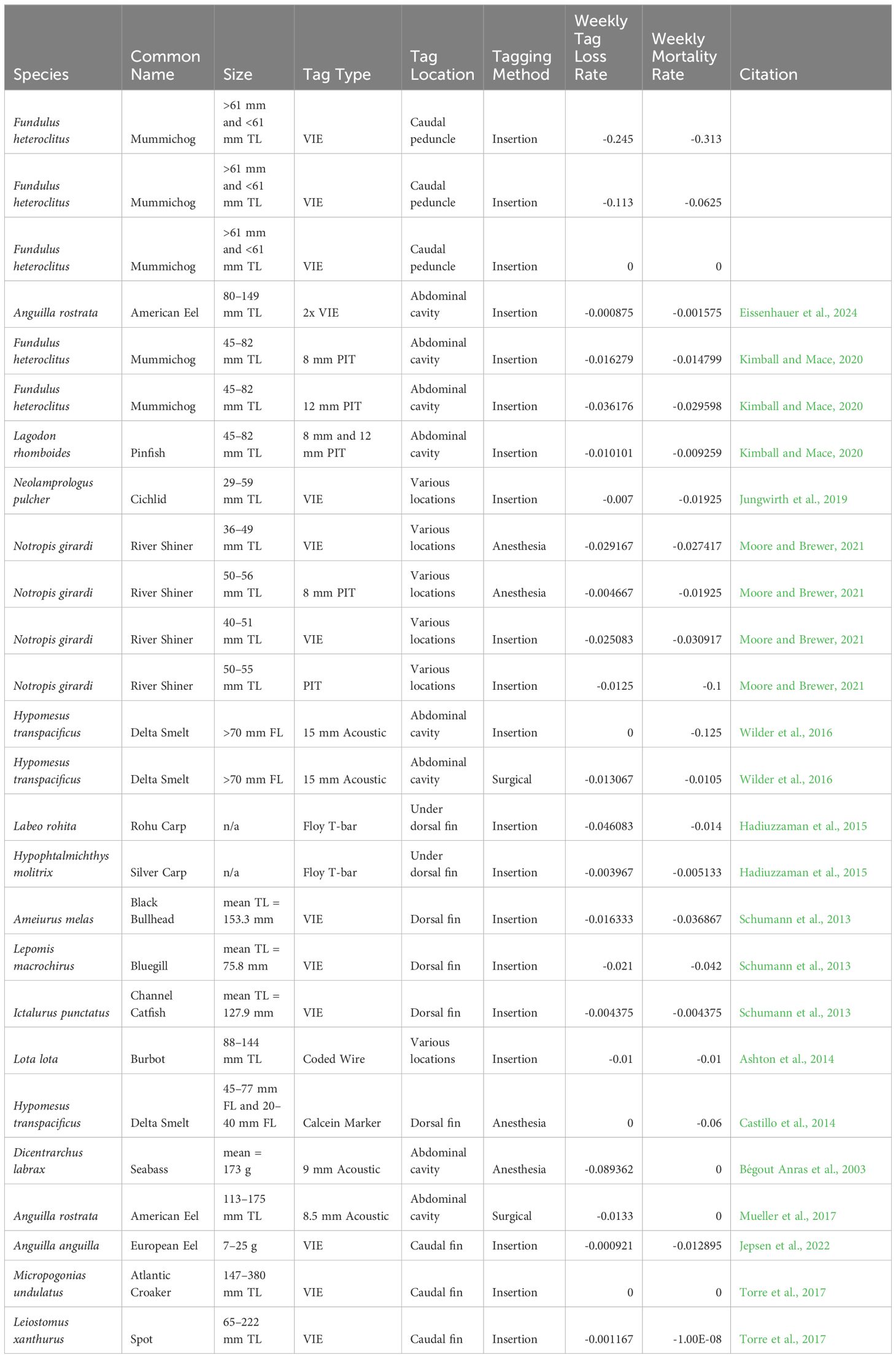
Table 1. Summary of data collected from our laboratory study and from other published case studies assessing tag retention and mortality in a laboratory setting that were used to validate the model.
2.2 Developing an ex-situ elastomer tag retention and mortality model for adjusting field recapture per unit effort
To apply observed changes in laboratory tag loss and survival of Mummichogs across two size classes to field observations, a predictive model was formed to adjust future field observations with respect to a weekly depreciation rate in potential recapturable fish. To calculate this loss in available animals to recapture, a series of equations were created to predict the total number of available fish at large to recapture with regards to fish TL and each size bin’s weekly changes in tag loss and survival. Using this, we estimated the number of recaptures that were missed due to tag loss or tag-related mortality. Table 2 lists all the associated variables used in the upcoming series of equations to calculate the tag depreciation factor (TDF).
To calculate the TDF and adjust raw recapture values, the proportion of tagged animals at large each week that were smaller and larger than 61 mm was determined (Equations 3, 4a, and 4b). The size designation we chose for this species was informed by the distribution of laboratory animals collected and recommendations for minimum tagging sizes by Kimball and Mace (2020). Using values of Tl and Ts and the coefficients derived from the slope and intercept of Equations 1a, 1b, 2a and 2b and known times at large, t, the reduction in available tagged animals at large due to mortality per time interval t was calculated for both small and large fish (Equations 5a and b, respectively). The second loss in tags at large due to misreads or shed tags per week was then calculated following mortality. This is done because only live animals can be captured in the field, and any missing tag due to a misread could only be observed from a living pool of animals. Hence why TAs and TAl were derived from survival-adjusted losses in fish, TSs and TSl (Equations 6a and b).
After accounting for mortality and tag loss, the total adjusted number of tags, TA, was calculated by summing the adjusted number of fish per size class from Equations 6a and b (Equation 7), and the sum across all sampling efforts was calculated by summing all values of TA (Equation 8). The TDF was then derived by dividing the adjusted sum of tagged animals at large by the total recorded fish at large, T (Equation 9). The TDF was used to estimate the total number of recaptured fish that would be present had there not been any loss in tagged animals at large (Equation 10). We then repeated this model using the variance in mortality and tag loss among taggers in the laboratory study to determine high error and low error scenarios. The high error scenario is based on increased mortality and tag loss (-95% confidence interval) while the low error scenario is based on low mortality and tag loss (+95% confidence interval) across the four taggers in our study. By testing the model against this range of scenarios, we can describe the potential error that might exist between different studies with overlapping objectives.
2.3 Applicability of model to previously published works, the ‘retmort’ package
The model was formatted into a series of functions within the ‘retmort’ package in R (https://github.com/Campbellb13-UD/retentionmort_R/tree/main; R Core Team, 2021) to predict tag loss and mortality for any mark-recapture-based study. This package includes several functions to provide estimates on existing datasets (i.e., retentionmort) or to predict the potential error associated with an upcoming mark-recapture study (i.e., retentionmort_generation) to guide method development. The resulting data frames from these functions can then be input into the ‘retentionmort_figure’ function to provide an Rmarkdown file of preliminary analyses describing the parameters that produce certain levels of error between expected and observed recaptures (example in the Supplementary Material). By adding custom m and β coefficients from applicable laboratory studies (e.g. Table 1) and a relevant dataset, the model will generate a percent standard error (PSE) between the observed number of recaptured individuals and the expected number of recaptures in the absence of tagging-based mortality or tag loss (Equation 11). With enough model runs (e.g., 1000), critical points of a chosen error threshold can be found for each model run to determine the necessity for a predictive model to generate an adjusted number of individuals tagged at large and recaptured after accounting for expected tag loss and mortality in a field application.
To assess the application of this model derivation to existing tag retention and mortality case studies, we sourced tag retention and mortality data from 14 published works to generate 26 unique cases of weekly tag loss and survival across various fish taxa (Table 1). We selected case studies with a wide range in weekly mortality (0 - 31.3%/week) and tag loss rate (0 – 24.5%/week) to assess potential limitations to model applicability. From each case study, we derived values of mS, βS, mM, and βM to inform model parameters, then used the retentionmort_generation function in retmort to create 1,000 simulated mark-recaptured datasets (parameters used for model: n = 1000 model iterations, min_weeks = 6, max_weeks = 100, max_tags = 500, prop_class1 = 1, max_recap = 0.2). From the data added, the retentionmort function was used to derive 5%, 10%, 15%, and 20% PSE critical thresholds (± 0.5%) between observed and estimated recaptured individuals from all the generated datasets per case study to determine conditions where field efforts may be affected by apparent tag retention or tagging mortality. Considerably large values were used in the dataset generation for each case study to encompass the full range of potential experimental scenarios and produce asymptotes yielding the maximum PSE for each case study.
3 Results
3.1 Laboratory evaluation of VIE tag loss and survival
During the laboratory experiment, water quality parameters were consistent over time (average ± SD: temperature = 23.61 ± 1.18 °C, salinity = 22.88 ± 3.95 ppt, pH = 7.64 ± 0.15; dissolved oxygen = 5.28 ± 1.05 mg/L). There were no significant changes in any water quality parameters during the time animals were held (p > 0.05, Mann-Kendall test). However, the salinity in the tanks holding smaller fish was on average 6.62 ppt greater than tanks holding large fish (p = 0.001, Kruskal-Wallis Test). There were no significant differences in the salinities of the separate tanks holding small fish or amongst the tanks with large fish (p = 1, p = 0.979, respectively). All other water quality parameters were not statistically different between tanks (all interactions have p > 0.05, Kruskal-Wallis Test). Water quality parameters in the laboratory were comparable to those simultaneously observed in Canary Creek, where animals were collected (average ± SD: temperature = 25.39 ± 3.21 °C, salinity = 18.20 ± 9.91 ppt; dissolved oxygen = 3.81 ± 2.61 mg/L).
After the third week of the study, there were visual signs of an infection in several tank treatments, resulting in a premature end to the experimental trial. During that time, average fish survival rate and the proportion of accurately recorded VIE tags decreased linearly (all data, r = -0.982, p < 0.001; r = -0.970, p < 0.001, Pearson Correlation, respectively) for both small (r = -1, p < 0.001; r = -0.866, p < 0.001, respectively) and large fish (r = -0.866, p < 0.001; r = -1, p < 0.001, respectively; Figure 1). The survival rate of small fish after three weeks was 78.9% for untagged fish and 83.3% for tagged fish, which was lower than larger fish with a survivorship of 100% for untagged and 95.8% for tagged fish (Table 3). Of the tagged fish, there was a significant difference in survival rate between taggers but not by size class (p = 0.043, p = 0.374, respectively; interaction, p = 0.124, Two-Way ANOVA with arcsine square root transformation) and no differences in tag loss (p = 0.634, p = 0.702, respectively; interaction, p = 0.197). Small fish had a significantly lower tag retention rate with a greater variation in retention between taggers after three weeks (average = 79.4%, SD = 23.6%) compared to larger fish (average = 88.1%, SD = 8.3%).
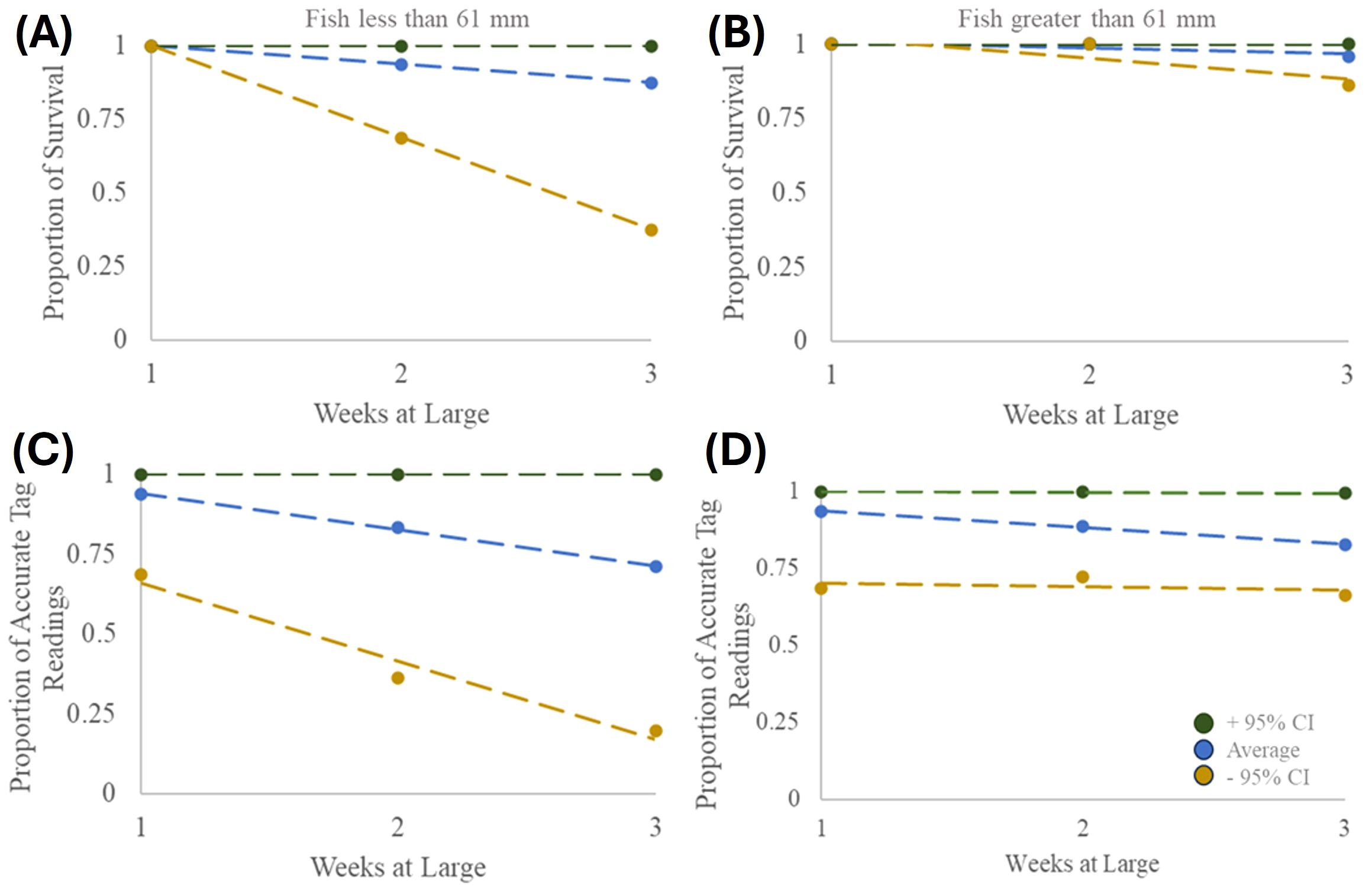
Figure 1. Strong linear decreases in survival rate (A, B, n = 12 small fish, n = 24 large fish) and retention rate (C, D, N = 34 tag observations for small fish, N = 67 tag observations for large fish) exist between small (A, C) and large (B, D) tagged Mummichogs held in laboratory settings for three weeks. Blue line indicates the average trend across all taggers while yellow indicates the minus 95% confidence and green indicated plus 95% confidence interval across all taggers. Coefficients of the resulting linear regression are listed in Table 1.
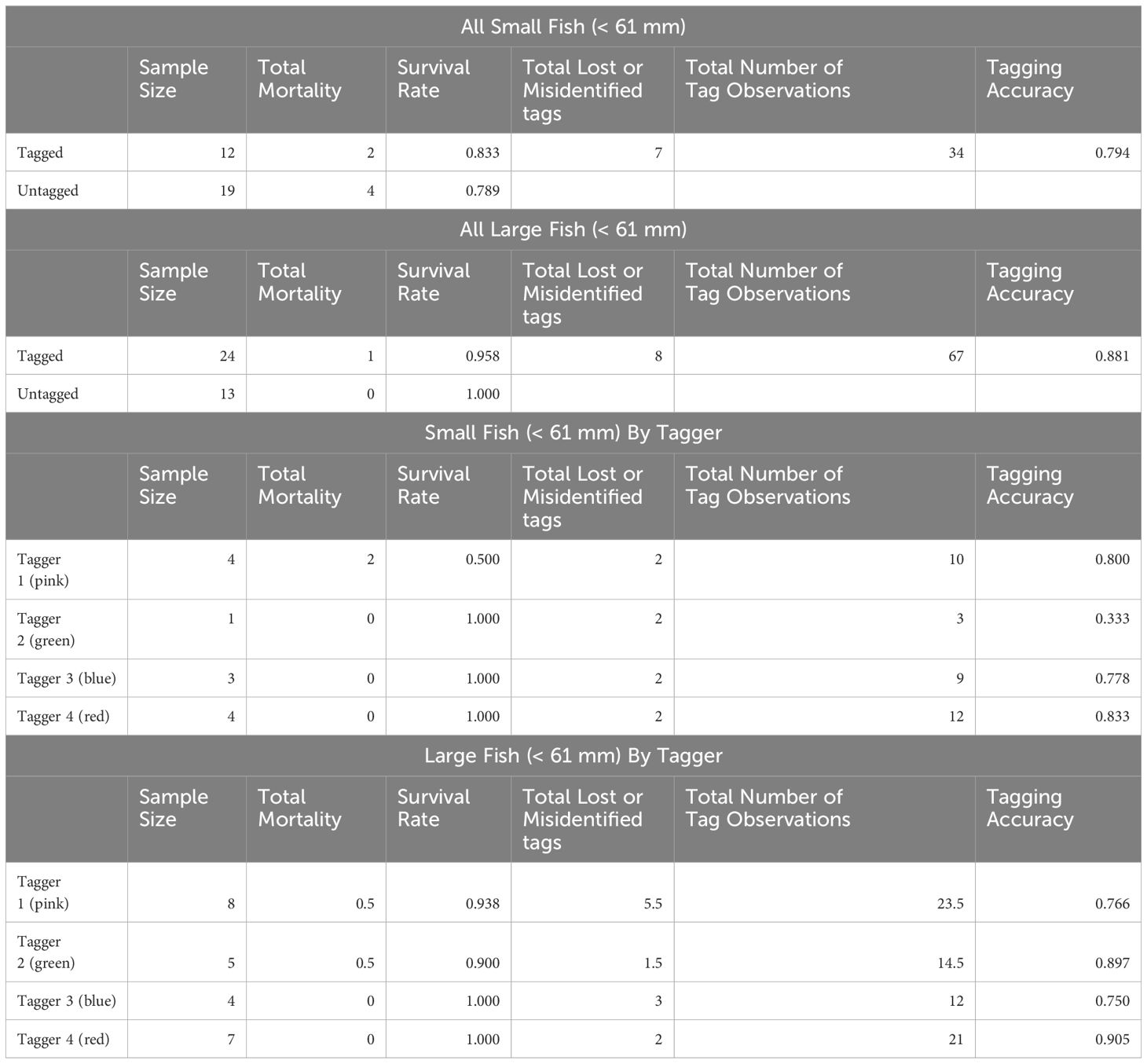
Table 3. Summary of survival and tag accuracy across all tags and separated by individual taggers compared to untagged individuals from the laboratory study; a mortality of 0.5 indicates one case where an individual died and lost a tag, preventing a fully confident identification of the individual’s tag color.
3.2 Application of model to published case studies to determine critical PSE thresholds
All 26 case studies generated logical estimates of expected recaptures using the functions within the retmort package. Across all case studies, the average (± standard deviation) PSE was 7.56 ± 2.12% (N = 26, Table 4). The four cases that reported no mortality and tag loss had 0% PSE, and our case study on Mummichogs, after adding the 95% confidence interval, generated the highest PSE in recaptures at 49.13%. When determining critical points associated with each model run against different error thresholds, we observed that 11.5% of the case studies exceeded the 20% PSE threshold, consisting of an average weekly mortality rate of 12.52% ± 16.56% and tag loss rate of 14.91% ± 8.39% (n = 3). Five of the case studies (19.2%) exceeded the 15% PSE threshold, consisting of an average weekly mortality rate of 8.38% ± 13.02%, and a weekly tag loss rate of 14.12% ± 8.39%. The 10% PSE threshold was exceeded in 26.9% of the case studies, with an average weekly mortality rate of 6.82% ± 10.96% and a weekly tag loss rate of 8.34% ± 7.85% (n = 7). Lastly, 57.7% of cases exceeded the 5% PSE threshold with an average weekly mortality rate of 4.67% ± 7.82% and an average weekly tag loss rate of 4.64% ± 6.27% (n = 15). Both mortality rate and tag loss rate contributed to the average PSE of each model (p = 0.001, multiple linear regression; n = 26; Figure 2).
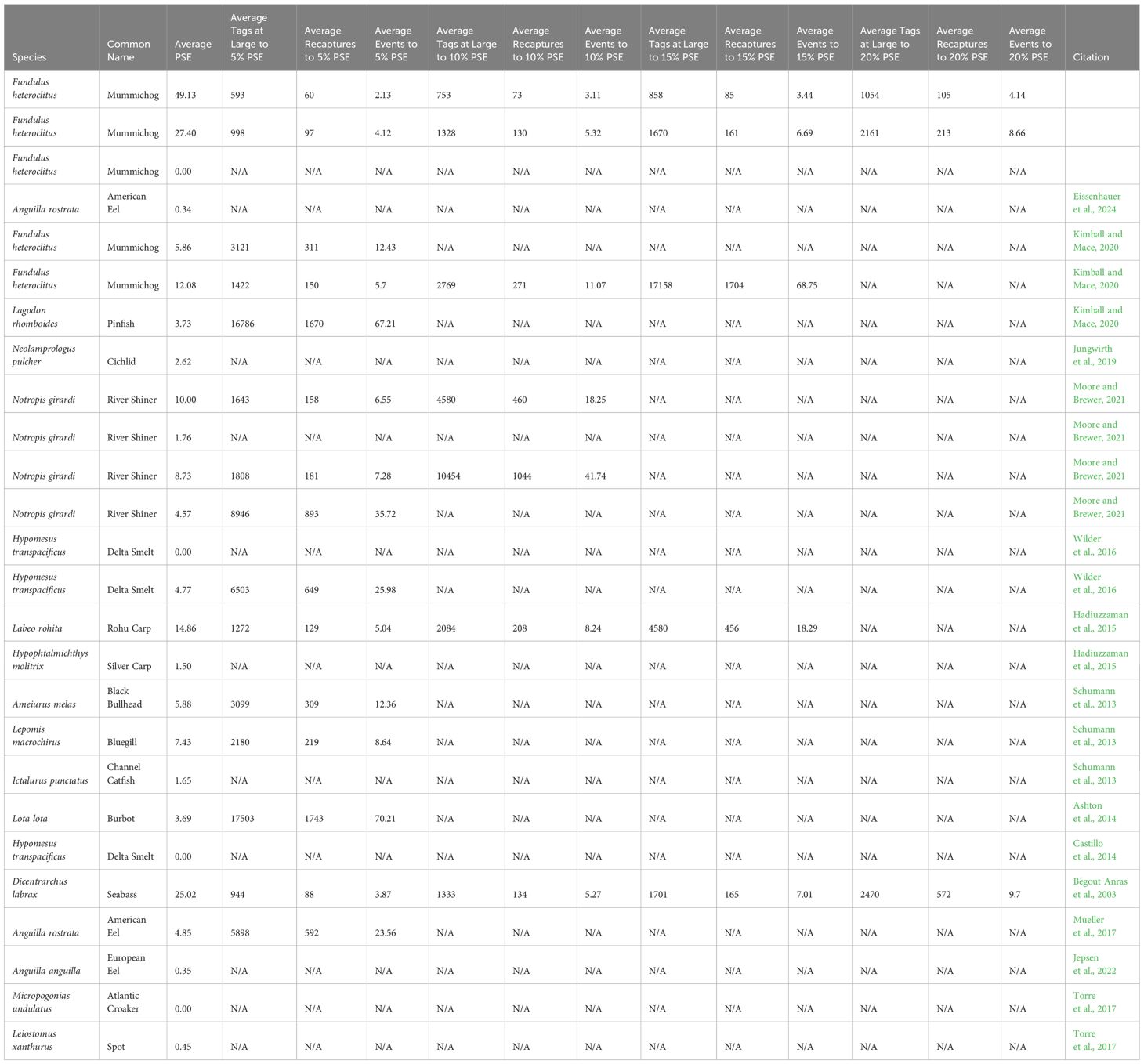
Table 4. Model response from each case study after 1,000 model iterations of randomized mark-recapture datasets, and the resulting average number of tags at large and recaptures that occurred at the critical point for each error threshold – N/As signify that this error threshold was not reached.
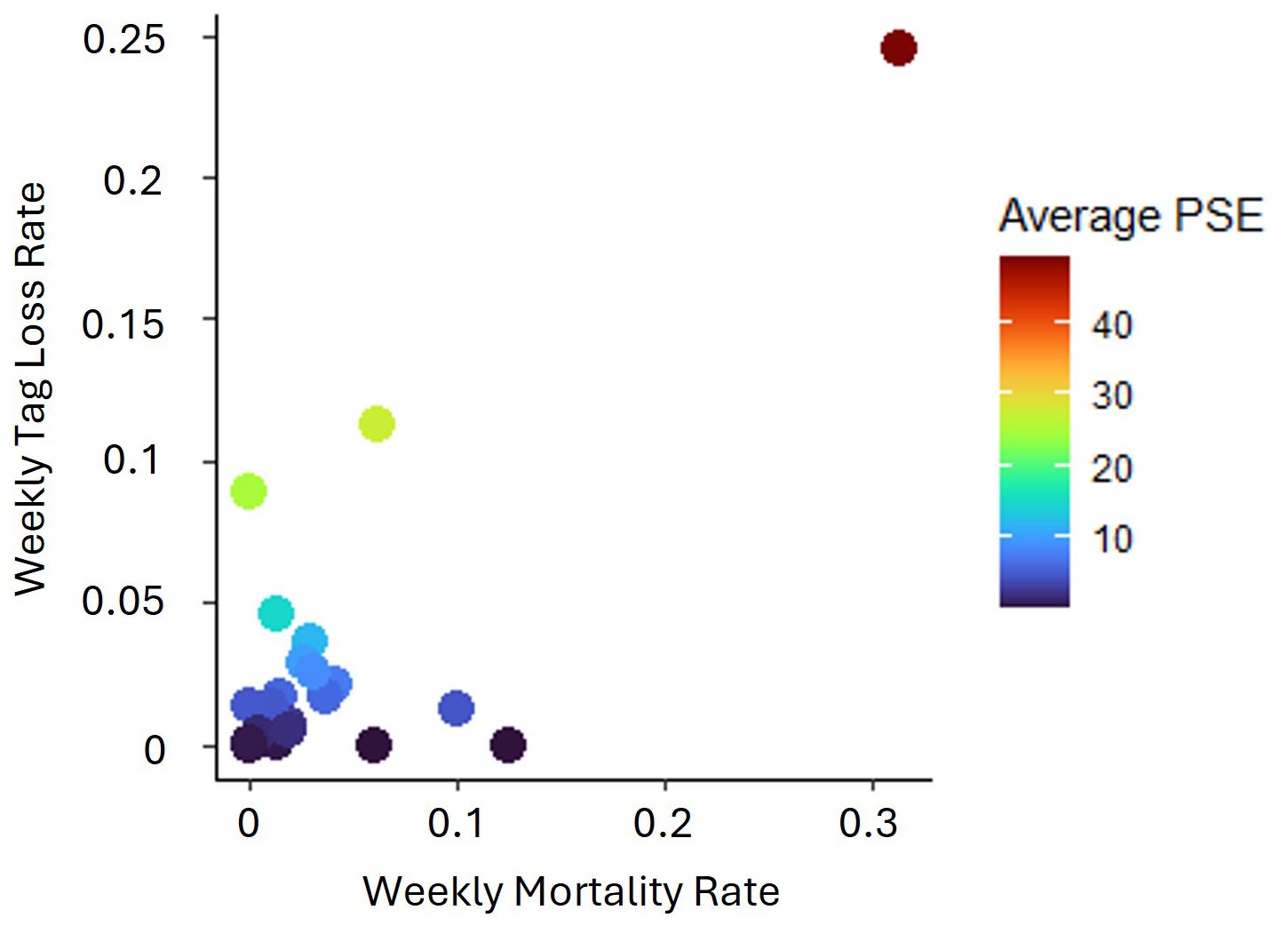
Figure 2. The average percent standard error from each case study increases as a function of both weekly tag loss rate and weekly mortality rate.
The three case studies that exceeded the 20% PSE threshold did so after an average of 7.50 ± 2.96 sampling events (range = 3 – 15), with an average of 1895 ± 745 tags at large (range = 308 – 3983), and an average of 191 ± 77 recaptures (range = 2 – 723). The five case studies that exceeded the 15% PSE threshold occurred after an average of 20.83 ± 27.37 sampling events (range = 3 – 100), with an average of 5193 ± 6836 tags at large (range = 224 - 27636) and an average of 514 ± 680 recaptures (range = 1 - 5365). The seven case studies that exceeded the 10% PSE threshold did so after an average of 13.29 ± 13.51 sampling events (range = 3 - 98), an average of 3329 ± 3387 tags at large (range = 227 - 25919), and 331 ± 339 recaptures (range = 1 - 4660). The fifteen case studies that exceeded the 5% PSE threshold did so after an average of 19.39 ± 22.18 sampling events (range = 2 - 100), an average of 4848 ± 5534 tags at large (range = 12 - 27636), and an average of 483 ± 551 recaptures (range = 1 - 5365) (Table 4). In all cases, the PSE observed in our model increased linearly before reaching an asymptote across the number of simulated tagging effortss per model run (Figure 3), the number of tags at large (Supplementary Figure S1), and the error between expected and observed recaptures which increased linearly (Figure 4).
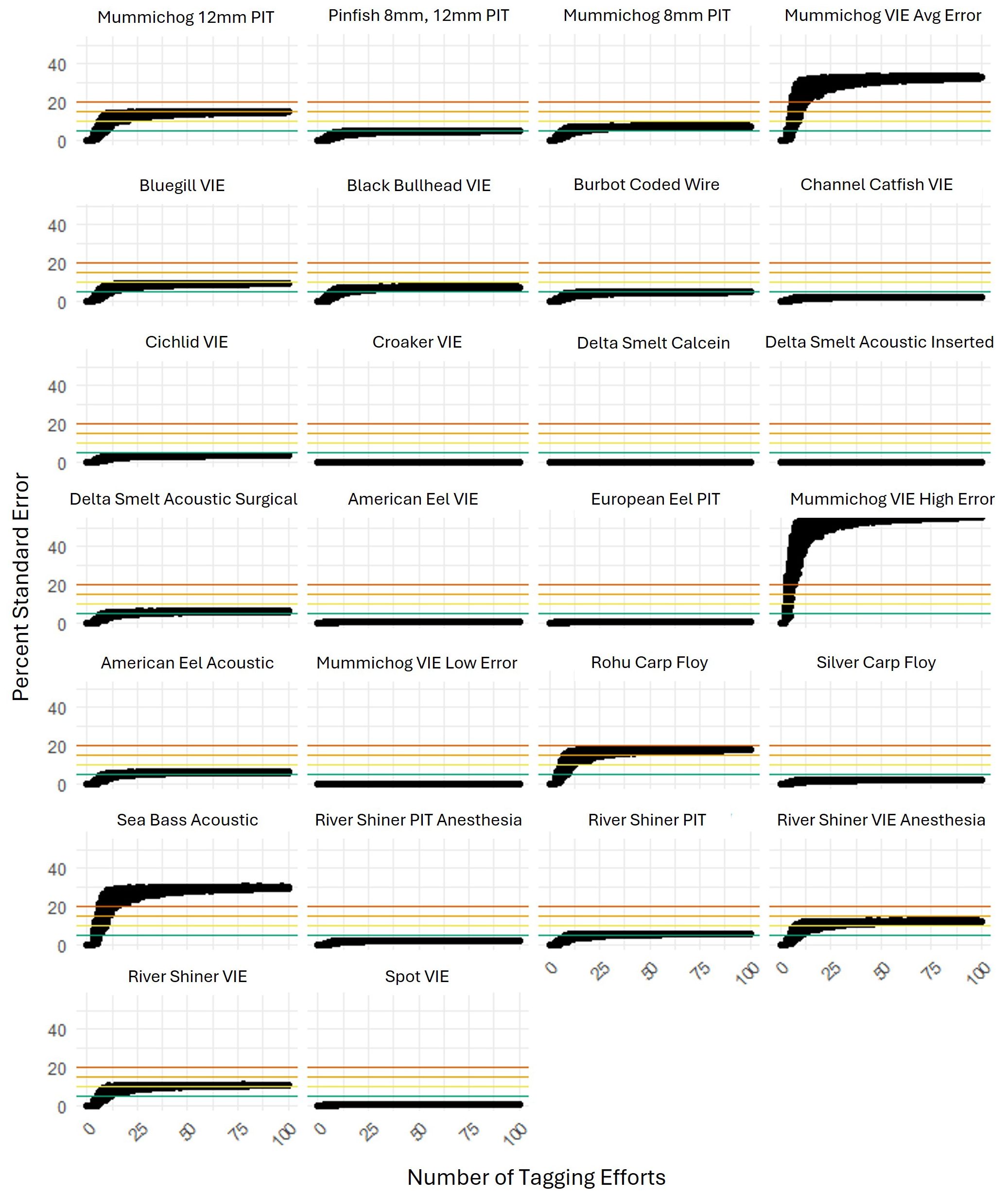
Figure 3. The percent error for each mark-recapture tagging event across 1,000 model iterations for each case study. PSE thresholds are drawn at 5% (green), 10% (yellow), 15% (orange), and 20% (red).
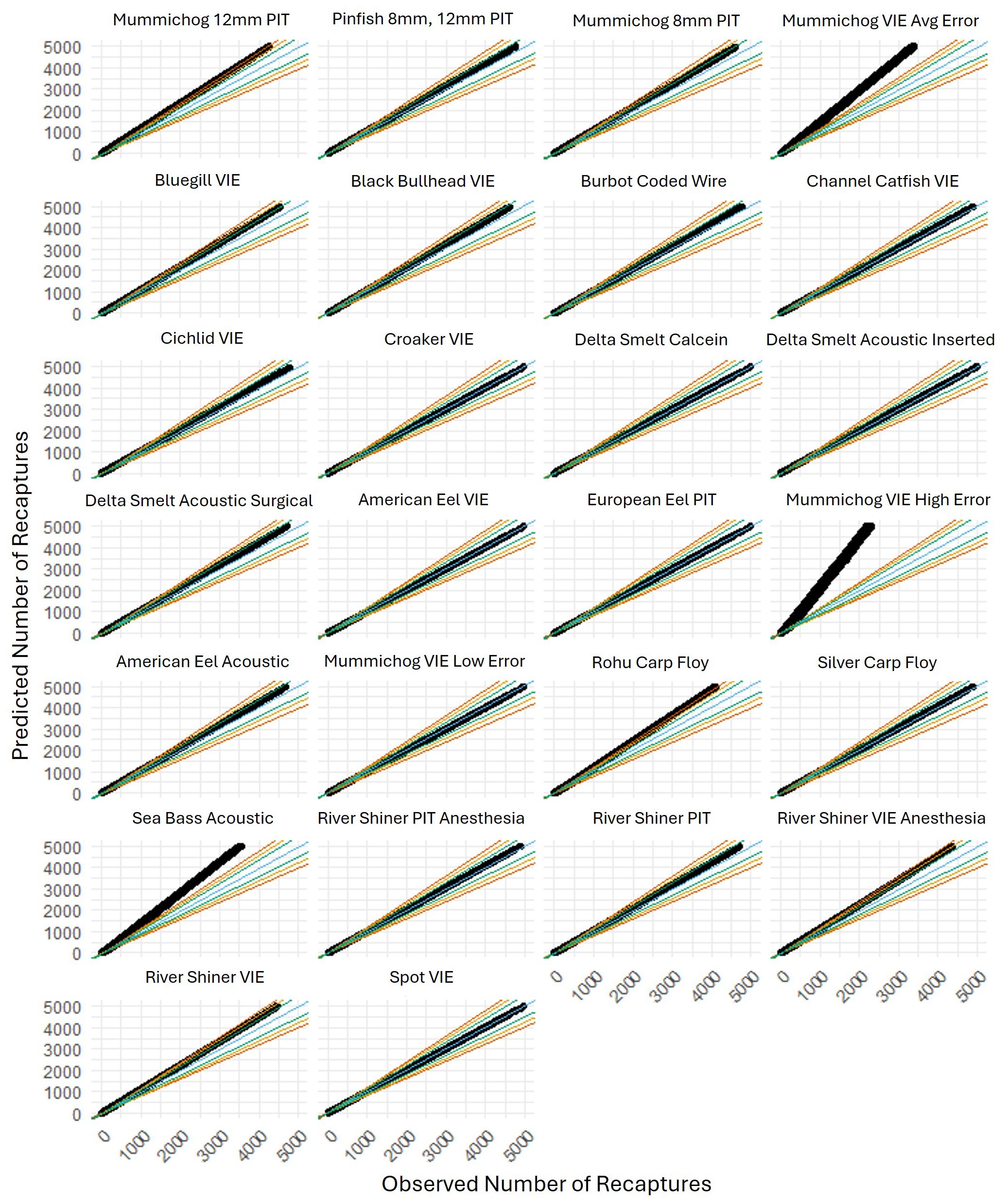
Figure 4. The error between the predicted and observed number of recaptures increases linearly with the total number of recaptures when applying 1,000 model iterations of artificial mark-recapture data. PSE critical points are highlighted by the green (5% PSE), yellow (10% PSE), orange (15% PSE), and red (20% PSE). The blue line signals a perfect 1:1 agreement between expected and observed values.
4 Discussion
4.1 Laboratory evaluation of VIE tag loss and survival
Understanding sources of bias that impact the analysis of mark-recapture data is critical for improving the inferencing strength of tagging studies. To assist future research efforts, we conducted a laboratory experiment to determine how variation in tagging personnel and fish size influence rates of tag loss and mortality when using VIE tagging methods. We were able to derive a linear decrease in the proportion of accurately read tags and Mummichog survival over the three-week period, which was consistent with results from similar studies conducted over larger temporal scales (Bolland et al., 2009). We also found that survival varied significantly between taggers while tag loss was not significantly affected by fish size or tagger. Our results emphasize the potential sources of error in VIE-based methodologies and fell within the range of values observed in past studies (see Bangs et al., 2013; Jungwirth et al., 2019; Cabot et al., 2021). However, our mortality and tag loss percentages were higher than most other studies on Mummichogs in the average and high error scenarios and lower in the low error scenario (other studies consistently observe above 95% retention and survival, see Skinner et al., 2005, 2006).
A potential source of the increased mortality rate we observed may be due to our premature definition of mortality (removing animals, tagged and untagged, when they exhibited negative behavioral or physical impacts from the tag such as large sores or skin discolorations, lethargic swimming behaviors, and injury), which would inflate mortality rates, though we expect that this definition best represents latent mortality observed in situ. The cause of the poor body condition observed in some of the fish might be attributed to an infection that was observed in some of the individuals, producing added stress to the animals (see Kimball and Mace, 2020). While we did not use anesthesia in this study, doing so may have helped to enhance the observed survival and retention, as shown in other studies (Skinner et al., 2005, 2006) by limiting stress on the animals and potentially reducing handling time (Myszkowski et al., 2003; Cooke et al., 2004; Neiffer and Stamper, 2009). Also, the colors used (red, pink, blue, green) and combining tagged and untagged individuals within tanks (unlike Skinner et al., 2006 and Fraiola and Carlson, 2016) could have led to additional misread tags compared to prior studies, by creating the opportunity to misidentify a tagged individual as one not tagged, further inflating the observed tag loss rate compared to these studies. Pink and red tags were particularly difficult to distinguish (also noted by Jungwirth et al., 2019), and blue was hard to see on the caudal peduncle of the Mummichogs as it blended in with the dark coloration of the fish. We recommend that future VIE tagging efforts use contrasting colors, relative to each other and the study species pigmentation. Despite observing different salinities between size treatments, we do not expect that those differences affected survival since the full range of salinity did not approach the physiological thresholds for Mummichogs (Griffith, 1974; Weisberg, 1986; Marshall et al., 2016) and the variability in salinity falls within the typical tidal range for Canary Creek, DE. We believe our experimental treatments still resemble natural conditions and are unlikely to have resulted in the added mortality observed in smaller fish, though further research is required to confidently draw that conclusion.
While all the taggers in our study were sufficiently trained, their innate skill levels varied. Hence, our study captures the potential variability in past studies that used similar methods carried out by several taggers. Having multiple taggers within a study can introduce variance between and among each tagger, as tagging success will likely vary across taggers and potentially for each tagger over time. However, having multiple taggers is an inherent requirement in large-scale field studies that require several thousand tags to be administered in a reasonable timeframe. While multiple taggers produce a source of variance in tag retention and mortality, the variation between taggers can be calculated by using similar tag retention experiments to our study. Further, applying modeled projections using retmort functions can describe the magnitude of this variation to determine its impact on completed and anticipated works.
4.2 Application of model to published case studies to determine critical PSE thresholds
The results of our laboratory study led us to develop a predictive model that can provide a logical adjustment to observed RPUE in field studies to account for tagging-related mortality and tag loss. Given the disparity in tag loss rates between the two size classes of fish we sampled, we were also interested in accounting for the effect of such size classes. Our model can improve the accuracy of future recapture studies by estimating the critical points where a correction would be required to account for tagging-induced mortality and retention. The model readily provides this corrected value as the total number of recaptured fish that would be present had there not been any loss in tagged animals at large due to tag-related mortality, tag loss, and/or misread tags. The application of this model will improve the robustness of future recapture studies.
After applying our retention-mortality model to 26 case studies, we concluded that this tool can effectively predict the combined influence of mortality and tag loss across a wide range of species. Most of the case studies exhibited a PSE lower than 20%, indicating a small deviance between the expected and observed numbers of recaptured individuals estimated using artificial mark-recapture datasets. The deviance is attributable to the low mortality and tag loss most of the case studies reported. That said, many tested studies elicited errors greater than 5%, indicating a need for this application in future mark-recapture efforts to improve the accuracy and precision of forecasting success in restoration projects.
Our model requires a series of assumptions. First, we assumed that the mortality and tag loss rates were linear and occurred only over a 5-week period, then halted. The 5-week estimate we applied extends past our 3-week case study to better represent the period of tag loss and mortality from other studies. Many of the studies we tested using our model had similar trends at the beginning of the experiment, where mortality and tag loss were initially high. Longer studies observed stable retention following the initial period of loss, sometimes extending greater than one year, with several exceptions (see Sandford et al., 2020). In an attempt to relate broadly to various studies, account for the limited data presented in many studies (commonly consisting of one time length value and a resulting proportion of mortality or tag loss), and utilize relationships seen in our case study, we applied linear relationships to project tag loss and mortality, rather than a more complex, logarithmic-based relationship, for example. As a result, our model likely overestimates tag loss and mortality during the first five weeks after an individual is tagged, then underestimates those values following that time point. Refining the model to provide options to account for the various relationships observed across tag retention studies would further improve accuracy and applicability and should be considered in future studies. Second, when the laboratory study was performed, we assumed that all mortality and tag loss events had been caused by the user and not due to any husbandry-related effects, as water quality parameters were similar to those encountered in a natural marsh. This assumption may underestimate tag loss and mortality rates in the field, where predation and more extreme environmental conditions exist. However, for smaller fish, where the untagged group had lower survivability than the tagged group, their mortality could have been more associated with animal containment rather than tag-based mortality, possibly providing an over-estimate of tag loss and survival rates. It is also important to note that when applying the model to each case study, we intentionally selected a large range of values for each model parameter that might not be reasonably applicable in all cases. This was done to force an asymptotic result of tag loss and mortality over time, regardless of the study subject or field methods employed, so that all potential outcomes were properly reflected from one set of model runs and to standardize the results across all case studies used. Future applications of this model should be specified based on the anticipated number of tagged animals and expected recapture rates to provide more critical insight into expected error.
Based on the composition of species trialed in this model’s evaluation, there is an emphasis on the application of this model to coastal and freshwater fish species. The coastal species represented in our study (i.e. Mummichog, American Eel, European Eel, Pinfish, Sea Bass, Atlantic Croaker, Spot) reflect abundant species that have considerable ecological and commercial value which require monitoring efforts to assess ecological functioning, determine restoration needs, and monitor the progress of restoration efforts (Bégout Anras et al., 2003; Mueller et al., 2017; Torre et al., 2017; Kimball and Mace, 2020; Jepsen et al., 2022; Eissenhauer et al., 2024). It is crucial to ensure the effective management of coastal zone habitats given their ecological and economic importance (Seitz et al., 2014). Such coastal zone work, like restoration projects, often involve tagging methods (e.g., Teo and Able, 2003; Crum et al., 2018) to assess population changes in response to these efforts and track progress. Unfortunately, retaining 100% of deployed tags is unlikely, and some species report significant tag-related mortality or shedding, especially smaller fish, violating closed-population assumptions for population estimation models (Pine et al., 2012; Spurgeon et al., 2020; Dettloff, 2023). By not accounting for errors, studies that monitor coastal resilience and restoration efforts (e.g., Teo and Able, 2003) could underestimate the effects of coastal zone restoration projects, potentially leading to additional, and possibly redundant restorative effort. By accounting for unavoidable errors in recapture studies (i.e., the loss of tagged fish), our model improves the precision of tag-based mortality and has the capacity to enhance the evaluation of coastal restoration efforts on indicator species.
Data availability statement
The datasets presented in this study can be found in online repositories. The names of the repository/repositories and accession number(s) can be found in the article/Supplementary Material. Further inquiries can be directed to the corresponding author/s.
Ethics statement
The animal study was approved by University of Delaware Institutional Animal Care and Use Committee. The study was conducted in accordance with the local legislation and institutional requirements.
Author contributions
JM: Conceptualization, Data curation, Formal analysis, Investigation, Methodology, Validation, Visualization, Writing – original draft, Writing – review & editing, Project administration. BC: Conceptualization, Data curation, Formal analysis, Investigation, Methodology, Project administration, Supervision, Validation, Visualization, Writing – original draft, Writing – review & editing. RH: Conceptualization, Investigation, Methodology, Supervision, Visualization, Writing – review & editing, Data curation, Formal analysis, Project administration. NM: Formal analysis, Methodology, Writing – review & editing, Data curation, Investigation, Validation, Visualization. MW: Conceptualization, Investigation, Methodology, Writing – review & editing. AC: Funding acquisition, Resources, Writing – review & editing, Project administration, Supervision. EH: Conceptualization, Funding acquisition, Methodology, Project administration, Resources, Software, Supervision, Writing – review & editing.
Funding
The author(s) declare that financial support was received for the research and/or publication of this article. This material is based upon work supported by the US Army Corps of Engineers, ERDC Contracting Office under Contract No. W912HZ-22-2–0015 and student support was provided by the National Science Foundation (NSF-OCE) Research Experiences for Undergraduates Award (#2051069).
Acknowledgments
We would like to acknowledge the Department of Defense and the NSF REU program for supporting this project. We would also like to acknowledge the members of our laboratory who volunteered their time and expertise in the field battling both the elements and the plague of biting flies we endured along the way, including Rachel Roday, Alyssa Campbell, and Dan Millea.
Conflict of interest
The authors declare that the research was conducted in the absence of any commercial or financial relationships that could be construed as a potential conflict of interest.
Generative AI statement
The author(s) declare that no Generative AI was used in the creation of this manuscript.
Publisher’s note
All claims expressed in this article are solely those of the authors and do not necessarily represent those of their affiliated organizations, or those of the publisher, the editors and the reviewers. Any product that may be evaluated in this article, or claim that may be made by its manufacturer, is not guaranteed or endorsed by the publisher.
Author disclaimer
Any opinions, findings and conclusions or recommendations expressed in this material are those of the author(s) and do not necessarily reflect the views of the US Army Corps of Engineers, ERDC Contracting Office.
Supplementary material
The Supplementary Material for this article can be found online at: https://www.frontiersin.org/articles/10.3389/fevo.2025.1572994/full#supplementary-material
Supplementary Figure 1 | The percent error for each mark-recapture against the number of tags at large for each tagging event across 1,000 model iterations for each case study. PSE thresholds are drawn at 5% (green), 10% (yellow), 15% (orange), and 20% (red).
References
Archdeacon T. P., Remshardt W. J., Knecht T. L. (2009). Comparison of two methods for implanting Passive Integrated Transponders in Rio Grande silvery minnow. N. Am. J. Fish. Manage. 29, 346–351. doi: 10.1577/M08-130.1
Ashton N. K., Anders P. J., Young S. P., Cain K. D. (2014). Coded wire tag and Passive Integrated Transponder tag implantations in juvenile burbot. N. Am. J. Fish. Manage. 34, 391–400. doi: 10.1080/02755947.2014.882458
Bangs B. L., Falcy M. R., Scheerer P. D., Clements S. (2013). Comparison of three methods for marking a small floodplain minnow. Anim. Biotelem. 1, 18. doi: 10.1186/2050-3385-1-18
Bégout Anras M. L., Covés D., Dutto G., Laffargue P., Lagardère F. (2003). Tagging juvenile seabass and sole with telemetry transmitters: medium-term effects on growth. ICES J. Mar. Sci. 60, 1328–1334. doi: 10.1016/S1054-3139(03)00135-8
Bolland J. D., Cowx I. G., Lucas M. C. (2009). Evaluation of VIE and PIT tagging methods for juvenile cyprinid fishes. J. Appl. Ichthyol. 25, 381–386. doi: 10.1111/j.1439-0426.2009.01261.x
Brannelly L. A., Chatfield M. W., Richards-Zawacki C. (2013). Visual Implant Elastomer (VIE) tags are an unreliable method of identification in adult anurans. Herpetol. J. 23, 125–129.
Cabot M. L., Troan B. V., Ange-van Heugten K., Schnellbacher R. W., Smith D., Ridgley F., et al. (2021). Migration and histologic effects of Visible Implant Elastomer (VIE) and Passive Integrated Transponder (PIT) tags in the marine toad (Rhinella marina). Animals 11, 3255. doi: 10.3390/ani11113255
Castillo G., Morinaka J., Fujimura R., DuBois J., Baskerville-Bridges B., Lindberg J., et al. (2014). Evaluation of calcein and photonic marking for Cultured Delta Smelt. N. Am. J. Fish. Manage. 34, 30–38. doi: 10.1080/02755947.2013.839970
Catalano M. J., Chipps S. R., Bouchard M. A., Wahl D. H. (2001). Evaluation of injectable fluorescent tags for marking Centrarchid fishes: retention rate and effects on vulnerability to predation. N. Am. J. Fish. Manage. 21, 911–917. doi: 10.1577/1548-8675(2001)021<0911:EOIFTF>2.0.CO;2
Close T. L., Jones T. S. (2002). Detection of Visible Implant Elastomer in fingerling and yearling rainbow trout. N. Am. J. Fish. Manage. 22, 961–964.
Cooke S. J., Suski C. D., Ostrand K. G., Tufts B. L., Wahl D. H. (2004). Behavioral and physiological assessment of low concentrations of clove oil anaesthetic for handling and transporting largemouth bass (Micropterus salmoides). Aquaculture 239, 509–529. doi: 10.1016/j.aquaculture.2004.06.028
Crum K. P., Balouskus R. G., Targett T. E. (2018). Growth and movements of mummichogs (Fundulus heteroclitus) along armored and vegetated estuarine shorelines. Estuar. Coast. 41, 131–143. doi: 10.1007/s12237-017-0299-x
Currin C. A., Wainright S. C., Able K. W., Weinstein M. P., Fuller C. M. (2003). Determination of food web support and trophic position of the mummichog, Fundulus heteroclitus, in New Jersey smooth cordgrass (Spartina alterniflora), common reed (Phragmites australis), and restored salt marshes. Estuaries 26, 495–510. doi: 10.1007/BF02823726
Deegan L. A., Hughes J. E., Rountree R. A. (2000). “Salt marsh ecosystem support of marine transient species,” in Concepts and Controversies in Tidal Marsh Ecology. Eds. Weinstein M. P., Kreeger D. A. (Kluwer Academic Publishers, Dordrecht), 333–365. doi: 10.1007/0-306-47534-0_16
Dettloff K. (2023). Assessment of bias and precision among simple closed population mark-recapture estimators. Fish. Res. 265, 106756. doi: 10.1016/j.fishres.2023.106756
Eissenhauer F., Martin M., Adesola J., Curry R. A., Linnansaari T., Harrison P. M. (2024). Short-term survival and growth of American eel elvers marked with Visible Implant Elastomer tags. N. Am. J. Fish. Manage. 44, 826–834. doi: 10.1002/nafm.11016
Etherington L. L., Eggleston D. B., Stockhausen W. T. (2003). Partitioning loss rates of early juvenile blue crabs from seagrass habitats into mortality and emigration. Bull. Mar. Sci. 72, 371–391.
FitzGerald J. L., Sheehan T. F., Kocik J. F. (2004). Visibility of Visual Implant Elastomer tags in Atlantic salmon reared for two years in marine net-pens. N. Am. J. Fish. Manage. 24, 222–227. doi: 10.1577/M02-138
Fraiola K. M. S., Carlson S. M. (2016). Feasibility of using Passive Integrated Transponder technology for studying the ecology of juvenile striped mullet (Mugil cephalus) in streams. Pac. Sci. 70, 349–360. doi: 10.2984/70.3.6
Frederick J. L. (1997). Evaluation of fluorescent elastomer injection as a method for marking small fish. Bull. Mar. Sci. 61, 399–408.
Griffith R. W. (1974). Environment and salinity tolerance in the genus Fundulus. Copeia 1974, 319–331. doi: 10.2307/1442526
Griffiths S. (2002). Retention of visible implant tags in small rockpool fishes. Mar. Ecol. Prog. Ser. 236, 307–309. doi: 10.3354/meps236307
Hadiuzzaman M., Hasan M. R., Ali M. F., Habib M. A. B. (2015). Feasibility study using Floy Tag and Visible Implant Fluorescent Elastomer marker in major carps. Int. J. Res. Fish. Aquacult. 5, 151–155.
Haines G. B., Modde T. (1996). Evaluation of marking techniques to estimate population size and first-year survival of Colorado squawfish. N. Am. J. Fish. Manage. 16, 905–912. doi: 10.1577/1548-8675(1996)016<0905:EOMTTE>2.3.CO;2
Haines G. B., Severson S. H., Modde T. (1998). Evaluation of razorback sucker and Colorado squawfish batch marking techniques. Prog. Fish Cult. 60, 272–275. doi: 10.1577/1548-8640(1998)060<0272:EORSAC>2.0.CO;2
Hale E. A., Park I. A., Fisher M. T., Wong R. A., Stangl M. J., Clark J. H. (2016). Abundance estimate for and habitat use by early juvenile Atlantic sturgeon within the Delaware River Estuary. Trans. Am. Fisheries Soc. 145, 1193–1201. doi: 10.1080/00028487.2016.1214177
Jepsen N., Richter L., Pedersen M. I., Deng Z. (2022). Survival, growth and tag retention of juvenile European eel (Anguilla Anguilla L.) with implanted 12 mm Passive Integrated Transponder tags and acoustic tags. J. Fish Biol. 101, 1375–1380. doi: 10.1111/jfb.15183
Josephson D. C., Robinson J. M., Weidel B. C., Kraft C. E. (2008). Long-term retention and visibility of Visible Implant Elastomer tags in brook trout. N. Am. J. Fish. Manage. 28, 1758–1761. doi: 10.1577/M08-019.1
Jungwirth A., Balzarini V., Zöttl M., Salzmann A., Taborsky M., Frommen J. G. (2019). Long-term individual marking of small freshwater fish: the utility of Visual Implant Elastomer tags. Behav. Ecol. Sociobiol. 73, 49. doi: 10.1007/s00265-019-2659-y
Kimball M. E., Mace M. M. III. (2020). Survival, growth, and tag retention in estuarine fishes implanted with Passive Integrated Transponder (PIT) tags. Estuar. Coast. 43, 151–160. doi: 10.1007/s12237-019-00657-4
Kneib R. T. (1986). The role of fundulus heteroclitus in salt marsh trophic dynamics. Am. Zool. 26, 259–269. doi: 10.1093/icb/26.1.259
Kneib R. T. (2000). “Salt marsh ecoscapes and production transfers by estuarine nekton in the Southeastern United States,” in Concepts and Controversies in Tidal Marsh Ecology. Eds. Weinstein M. P., Kreeger D. A. (Kluwer Academic Publishers, Dordrecht), 267–291. doi: 10.1007/0-306-47534-0_13
Lapointe N. W. R., Thiem J. D., Doka S. E., Cooke S. J. (2013). Opportunities for improving aquatic restoration science and monitoring through the use of animal electronic-tagging technology. BioScience 63, 390–396. doi: 10.1525/bio.2013.63.5.12
Leblanc C. A., Noakes D. L. (2012). Visible Implant Elastomer (VIE) tags for marking small rainbow trout. N. Am. J. Fish. Manage. 32, 716–719. doi: 10.1080/02755947.2012.683234
Lotrich V. A. (1975). Summer home range and movements of Fundulus heteroclitus (Pisces: Cyprinodontidae) in a tidal creek. Ecology 56, 191–198. doi: 10.2307/1935311
Lucas M. C., Baras E. (2000). Methods for studying spatial behaviour of freshwater fishes in the natural environment. Fish. Fish. 1, 283–316. doi: 10.1046/j.1467-2979.2000.00028.x
Marshall W. S., Tait J. C., Mercer E. W. (2016). Salinity preference in the estuarine teleost fish mummichog (Fundulus heteroclitus): halocline behavior. Physiol. Biochem. Zool. 89, 225–232. doi: 10.1086/686037
McGowan A. T., Hale E. A., Bartow D. H., Greco M. (2022). Population dynamics of common nearshore forage fishes in the Delaware inland bays, USA. Estuar. Coasts 45, 2181–2203. doi: 10.1007/s12237-022-01066-w
Moore D. M., Brewer S. K. (2021). Evaluation of Visual Implant Elastomer, PIT, and p-Chip tagging methods in a small-bodied minnow species. N. Am. J. Fish. Manage. 41, 1066–1078. doi: 10.1002/nafm.10607
Mueller R. P., Janak J., Liss S. A., Brown R. S., Deng Z., Harnish R. A. (2017). Retention and effects of miniature transmitters in juvenile American eels. Fish. Res. 195, 52–58. doi: 10.1016/j.fishres.2017.06.017
Murray D. L., Fuller M. R. (2000). “A critical review of the effects of marking on the biology of vertebrates,” in Research Techniques in Animal Ecology. Eds. Boitani L., Fuller T. K. (Columbia University Press, New York), 15–64.
Myszkowski L., Kamiński R., Wolnicki J. (2003). Response of juvenile tench Tinca tinca (L.) to the anaesthetic 2-phenoxyethanol: response of juvenile tench to 2-phenoxyethanol. J. Appl. Ichthyol. 19, 142–145. doi: 10.1046/j.1439-0426.2003.00460.x
Neiffer D. L., Stamper M. A. (2009). Fish sedation, anesthesia, analgesia, and euthanasia: considerations, methods, and types of drugs. ILAR J. 50, 343–360. doi: 10.1093/ilar.50.4.343
Neufeld K., Blair S., Poesch M. (2015). Retention and stress effects of visible implant tags when marking western silvery minnow and its application to other Cyprinids (family Cyprinidae). N. Am. J. Fish. Manage. 35, 1070–1076. doi: 10.1080/02755947.2015.1079576
Nixon S. W., Oviatt C. A. (1973). Ecology of a New England salt marsh. Ecol. Monogr. 43, 463–498. doi: 10.2307/1942303
Olsen E. M., Gjøsæter J., Stenseth N. C. (2004). Evaluation of the use of visible implant tags in age-0 Atlantic cod. N. Am. J. Fish. Manage. 24, 282–286. doi: 10.1577/M02-190
Otis D. L., Burnham K. P., White G. C., Anderson D. R. (1978). Statistical inference from capture data on closed animal populations. Wildlife Monogr. 62, 3–135.
Pine W. E., Hightower J. E., Coggins L. G., Lauretta M. V., Pollock K. H. (2012). “Design and analysis of tagging studies,” in Fisheries Techniques. Eds. Zale A. V., Parrish D. L., Sutton T. M. (American Fisheries Society, Bethesda, Maryland), 521–572.
R Core Team (2021). R: a language and environment for statistical computing (Vienna, Austria: R Foundation for Statistical Computing). Available at: https://www.R-project.org/ (Accessed June 1, 2023).
Reeves K. S., Buckmeier D. L. (2009). Mortality, predation, and tag visibility of fish marked with Visible Implant Elastomer tags. N. Am. J. Fish. Manage. 29, 323–329. doi: 10.1577/M08-086.1
Roday R. E., Park I. A., Moore J. E., Feris Serrano R. J., Fox D. A., Hale E. A. (2024). Acoustic-tagged American Shad utilize historic habitat following dam removal in northern Delaware. Marine Coastal Fisheries 16, e10323. doi: 10.1002/mcf2.10323
Rogers L., Olsen E., Knutsen H., Stenseth N. (2014). Habitat effects on population connectivity in a coastal seascape. Mar. Ecol. Prog. Ser. 511, 153–163. doi: 10.3354/meps10944
Sandford M., Castillo G., Hung T. (2020). A review of fish identification methods applied on small fish. Rev. Aquac. 12, 542–554. doi: 10.1111/raq.12339
Schumann D. A., Koupal K. D., Hoback W. W., Schoenebeck C. W. (2013). Evaluation of sprayed fluorescent pigment as a method to mass-mark fish species. Open Fish Sci. J. 6, 41–47. doi: 10.2174/1874401X01306010041
Seitz R. D., Wennhage H., Bergström U., Lipcius R. N., Ysebaert T. (2014). Ecological value of coastal habitats for commercially and ecologically important species. ICES J. Mar. Sci. 71, 648–665. doi: 10.1093/icesjms/fst152
Skinner M. A., Courtenay S. C., Parker W. R., Curry R. A. (2005). Site fidelity of mummichogs (Fundulus heteroclitus) in an Atlantic Canadian estuary. Water Qual. Res. J. 40, 288–298. doi: 10.2166/wqrj.2005.034
Skinner M. A., Courtenay S. C., Parker W. R., Curry R. A. (2006). Evaluation of techniques for the marking of mummichogs with emphasis on Visible Implant Elastomer. N. Am. J. Fish. Manage. 26, 1003–1010. doi: 10.1577/M05-106.1
Spurgeon J. J., Pegg M. A., Siddons S. F., Hansen H. H. (2020). Retention of T-Bar Anchor tags for channel catfish in the Red River of the North. N. Am. J. Fish. Manage. 40, 330–334. doi: 10.1002/nafm.10410
Teo S. L. H., Able K. W. (2003). Habitat use and movement of the mummichog (Fundulus heteroclitus) in a restored salt marsh. Estuaries 26, 720–730. doi: 10.1007/BF02711983
Torre M. P., Lifavi D. M., Kaestner W. P., Targett T. E. (2017). No evidence of site fidelity in Atlantic croakers or spot in the shore zone of Lower Delaware Bay. Trans. Am. Fish. Soc 146, 1093–1100. doi: 10.1080/00028487.2017.1360391
Valiela I., Wright J. E., Teal J. M., Volkmann S. B. (1977). Growth, production and energy transformations in the salt-marsh killifish Fundulus heteroclitus. Mar. Biol. 40, 135–144. doi: 10.1007/BF00396259
Walker K. A., Trites A. W., Haulena M., Weary D. M. (2012). A review of the effects of different marking and tagging techniques on marine mammals. Wildl. Res. 39, 15. doi: 10.1071/WR10177
Weisberg S. B. (1986). Competition and coexistence among four estuarine species of Fundulus. Am. Zool. 26, 249–257. doi: 10.1093/icb/26.1.249
Weisberg S. B., Lotrich V. A. (1982). The importance of an infrequently flooded intertidal marsh surface as an energy source for the mummichog Fundulus heteroclitus: an experimental approach. Mar. Biol. 66, 307–310. doi: 10.1007/BF00397037
Keywords: tag retention, mark-recapture, visual implant elastomer, restoration, mortality, Mummichog, retmort, tagging
Citation: McCutcheon J, Campbell B, Hudock RE, Motz N, Windsor M, Carlisle A and Hale E (2025) An approach to predicting linear trends in tagging-related mortality and tag loss during mark-recapture studies. Front. Ecol. Evol. 13:1572994. doi: 10.3389/fevo.2025.1572994
Received: 08 February 2025; Accepted: 03 April 2025;
Published: 01 May 2025.
Edited by:
Amanda Tritinger, Engineer Research and Development Center (ERDC), United StatesReviewed by:
Martin Posey, University of North Carolina Wilmington, United StatesDouglas Peterson, Abernathy Fish Technology Center, United States
Copyright © 2025 McCutcheon, Campbell, Hudock, Motz, Windsor, Carlisle and Hale. This is an open-access article distributed under the terms of the Creative Commons Attribution License (CC BY). The use, distribution or reproduction in other forums is permitted, provided the original author(s) and the copyright owner(s) are credited and that the original publication in this journal is cited, in accordance with accepted academic practice. No use, distribution or reproduction is permitted which does not comply with these terms.
*Correspondence: Edward Hale, ZWhhbGVAdWRlbC5lZHU=
†These authors have contributed equally to this work and share first authorship
‡ORCID: Jasper McCutcheon, orcid.org/0009-0004-4138-532X
Brendan Campbell, orcid.org/0000-0001-5604-7658
Rileigh E. Hudock, orcid.org/0009-0009-2769-2880
Noah Motz, orcid.org/0009-0002-2480-9712
Madison Windsor, orcid.org/0009-0000-4517-0677
Aarown Carlisle, orcid.org/0000-0003-0796-6564
Edward Hale, orcid.org/0000-0002-7176-6420