- 1Bioversity International, Rome, Italy
- 2Department of Environmental Systems Science, ETH Zurich, Zurich, Switzerland
The genetic diversity, quality and suitability of seeds and planting stock is crucial for the short and long-term resilience of restored forest landscapes. However, these genetic aspects are widely neglected during both planning and implementation of restoration. Decisions on seed sourcing during implementation of forest landscape restoration (FLR) initiatives often prioritize short-term cost savings over long-term benefits. Such considerations result in strategies that favor rapid and cheap mass production of homogeneous plants and, thus, quantity over quality, with no regard for genetic diversity. This paper explores in detail the economic cost of improved integration of genetic diversity into restoration projects and tests the assumption that the benefits accruing from better integration of diversity exceed the costs. Using a bottom-up cost model, based on peer reviewed scientific literature, we analyse different FLR cost drivers, integrating genetic quality, in relation to the total costs of a range of tree-based restoration interventions, with a focus on seed sourcing, and tree species selection. The results indicate that the integration of genetic diversity into the management and planning of landscape restoration projects increased the costs incurred at the beginning of FLR interventions, specifically during seed sourcing, and species selection. These additional costs were largely due to the increased effort for the collection of genetically diverse and suitably adapted seed lots. However, despite this initial increase in costs the overall costs of restoration decreased substantially, due to cost savings relating to replacement costs of replanting. Even without these savings, the inclusion of genetic diversity is advisable since the costs involved in the integration of diversity are negligible compared to other restoration costs, such as labor costs related to controlling vegetative competition. We conclude that the expected long-term benefits associated with high genetic diversity far outweigh the costs. It also highlights that investing in genetic diversity as part of FLR is the smart thing to do to ensure cost effective and resilient landscape restoration. Restoration policies need to incentivise consideration of genetic diversity.
Introduction
The importance of tree-based forest landscape restoration for meeting local needs and global targets for sustainable development has never been more important. There is widespread recognition of the potential for reforestation and other tree-based restoration interventions to benefit society (Brancalion et al., 2019). Ambitious targets have been set by the Bonn Challenge to restore up to 350 million hectares of degraded land by 2030 (The Bonn Challenge, 2019). Growing political commitments at the national and international levels now need to be translated into sound policies, backed by adequate financial resources, to achieve these ambitious restoration aims (Graudal and Lillesø, 2007; The Bonn Challenge, 2019).
Native tree diversity and the use of tree genetic resources (TGR) are at the foundation of maximizing societal and environmental benefits in forest landscape restoration (FLR) (Jalonen et al., 2018; Kettle et al., 2020). FLR seeks to restore diversity and function at landscape scales through a diversity of interventions, including tree planting, agroforestry, and natural regeneration of forest. FLR also seeks through effective provision of multiple ecosystem functions and services, and diversified tree-based production systems to increase resilience of the livelihoods of local communities (Dawson et al., 2014; Wymore et al., 2014; Jalonen et al., 2018). In particular, genetic diversity provides the basis for tree populations' resistance to pests and diseases, and adaptation to changing climatic conditions (Kettle et al., 2008; Charlesworth and Willis, 2009; Alfaro et al., 2014; Graudal et al., 2014; Jalonen et al., 2018). Moreover, genetic diversity can also affect future productivity, seed set, and natural regeneration (Tito de Morais et al., 2020). The suitability of a species' or seed's origin for any given restoration site is another crucial factor in the survival of planted trees and their ability to meet desired objectives. Using locally sourced seeds, or site matched seeds can help ensure locally adapted seedlings for soil type, local plant communities, or climatic hardiness (e.g., ability to withstand extreme degrees of heat/cold), as well as other ecological interactions (Mortlock, 1999). However, in some areas the conditions might have changed or will change considerably due to climate change or land use. In such cases, locally adapted plants may not always provide the best conditions for a successful project (Mulawarman et al., 2003).
Despite the scientific evidence to support these considerations, the importance of integrating genetic diversity into restoration planning and implementation often remains neglected (Graudal and Lillesø, 2007; Alfaro et al., 2014; Dawson et al., 2014; Neto et al., 2014; Thomas et al., 2014; Dedefo et al., 2017; Roshetko et al., 2018). Many past projects have used unsuitable species, due to a lack of knowledge or access to native species, or reliance on a few exotic species (Lamb et al., 2005; Kettle et al., 2020). They often collected seeds from only a few mother trees (Dedefo et al., 2017), resulting in the narrow genetic basis undermining the vitality and resilience of restoration (Kettle et al., 2008). Roshetko et al. (2018) showed that only about a third of the project plans examined in their study displayed indications that project designers had, at least to some extent, included site matching, genetic diversity, or local adaptation of planting material in their planning.
There are multiple reasons why TGR does not receive adequate attention in projects. This might be due to a general lack of awareness of the importance of genetic considerations and of know-how on how to integrate genetic diversity effectively into restoration (Graudal and Lillesø, 2007; Jalonen et al., 2014). Failure to consider genetic diversity could also be due to economic incentives encouraging actors to prioritize low-cost high-volume production over quality (Dedefo et al., 2017). Such incentives may, for example, lead to strategies that aim to collect as many seeds as possible with the lowest possible investment (time and labor), using methods that often do not support high seed quality (Schmidt, 2000, 2007).
We examine the economic costs of integrating genetic diversity into the planning and implementation of four tree-based restoration approaches: Planting stock raised in a nursery from seeds and cuttings, planting vegetative propagules (stakes), and direct seeding. We do not include natural regeneration, since its cost structure differs considerably from the above-mentioned approaches, although many of the issues, such as seed source origins could equally compromise the effectiveness of natural regeneration. We limited our analyses to FLR in the tropics, since the costs of restoration are more comparable across tropical regions in contrast to temperate ones. We explore the notion that focusing on the least cost and easiest approach to scale up production of large numbers of low quality planting material, compared to a careful selection of seed sources and seed collection based on best practices to ensure suitability and genetic diversity, is actually more costly in economic terms. We therefore test the assumption that investing in genetically diverse plant material rather than using genetically homogeneous plant material is more economically efficient.
We developed a bottom-up cost model to test our assumptions. The model includes and is based partly upon a conceptual framework of interrelations among key components of currently applied seed sourcing strategies. The framework shows where different costs are incurred in the seed and seedling supply systems and which components and characteristics of the system substantially influenced overall restoration implementation costs. We then populated and parameterised the cost model with quantitative and qualitative data from a review of the relevant scientific and institutional literature.
Methods
Cost Model Structure
We assessed documented information on current tree seed and seedling sourcing strategies and their associated costs in two steps. Firstly, we conducted a review of academic peer reviewed literature. We used the search terms “forest,” “restoration,” “cost,” and “trop*” to search the Web of Science journal database for relevant literature. Secondly, we extended our search to institutional literature in order to gain a deeper understanding of the impact of genetic diversity on costs. To find relevant publications, we used the same search terms as for the scientific literature and searched Google Scholar and the websites of international organizations active in FLR.
Based on the literature review and information found on costs, we organized the cost model around four main activities, which are intrinsic to tree-based restoration and have distinct and independent costs associated with their implementation. We categorized these as “cost pools” according to the following project activities: seed collection, nursery propagation, site preparation and outplanting, and post-planting maintenance (Figure 1). Costs relating to awareness raising and capacity building were considered as a fifth potentially important cost pool, however, they were not integrated in the final cost model analysis since the actual costs were context-specific and therefore difficult to generalize.
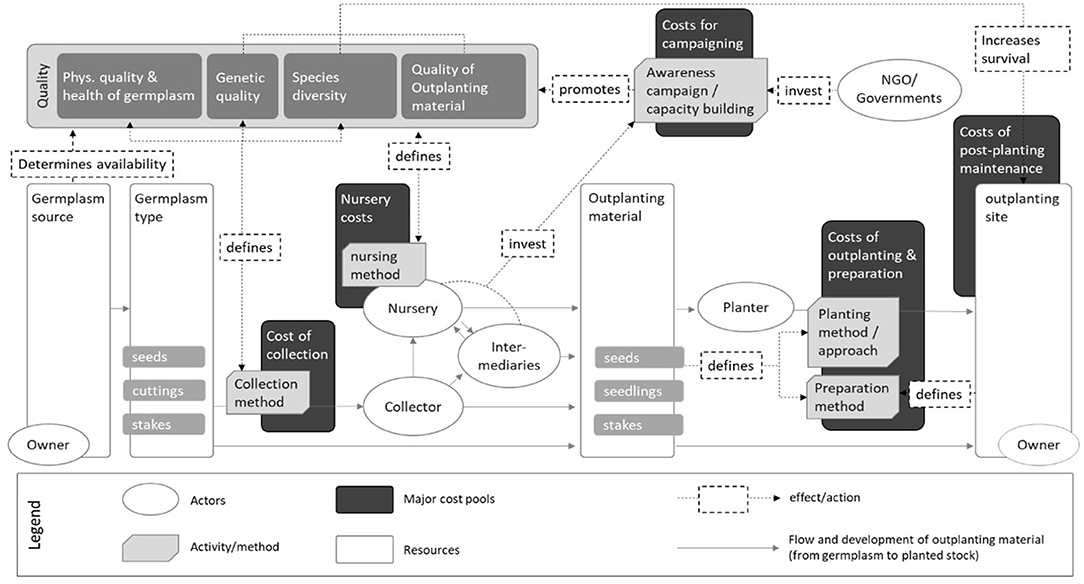
Figure 1. Cost framework for major activities and cost pools relating to tree seed and seedling supply systems for the implementation of tree-based restoration.
Each cost pool was composed of some or all of the following four cost components (CC): labor, transportation, equipment, and storage (e.g., seed storage). For example, the cost pool “post-planting maintenance” consisted of labor, transportation, and equipment costs; storage costs, however, were expected to be negligible in this phase. Each cost component consisted of basic costs (BC) and additional costs (AC) (Equation 1):
The basic costs (BC) comprised expenses that are always incurred in connection with the performance of restoration activity; for example, petrol for transportation, tools for land preparation, and salaries for nursery workers. The extent of these costs depends heavily on the chosen restoration approach. For example, equipment costs associated with the collection activity depend significantly on the type of germplasm, since this largely influences the choice of collection method and, thus, the required tools. Basic costs may also be influenced by regional factors that are not directly related to the chosen approach. For the sake of clarity and simplicity, we limited these region-specific factors to differences in local wage costs. We used an hourly rate of 1 USD as the default, since this was the rate that most of the studies we examined used for their calculations. Each deviation from this rate was considered in the calculation with the coefficient LWC (local wage costs).
The additional costs (AC) were caused by cost drivers (CD) associated with quality factors or the characteristics of the collection or outplanting site. The resulting additional costs were proportional to the basic costs (BC), since they also depended on the chosen restoration method (Equation 2).
Local wage costs (LWC) are only applicable if the cost driver (CD) or the basic costs (BC) are connected to the cost component “labor” (CClabor).
We used indicators to quantify the influence of a cost driver on cost components (Table 1). For example, collection costs significantly increase when collectors aim for a high genetic quality in their seed lot. In such a case, the collector would need to collect from a dispersed population comprising different combined provenances, instead of collecting seeds from a few individual trees. For this example, the cost driver would be “genetic quality” and the indicator, among others, “distance between mother trees.” The characteristics for the indicator would accordingly be “neighboring trees/isolated trees” for low genetic quality, “ <75 m” for medium genetic quality and “>75 m” for high genetic diversity. In the final step, we quantified the effect of a specific characteristic on the respective cost component by defining a coefficient (Supplementary Material 1). To stay with the above example: the decision to use a genetically diverse seed lot (CD) and, therefore, only seed from trees further than 75 m apart (characteristic), would increase the labor and transport costs (CC) of collection by 1.5 (coefficient), but would have no effect on equipment costs. Thus, the cost driver is a function of the characteristic of a specific indicator and of the resulting coefficient, the value of which differs according to the cost component (CC) affected (Supplementary Materials 1, 3).
Equation (3) shows an example of the calculation for the cost component “labor,” for the cost pool “collection costs,” when using the restoration approach “direct seeding”:
Cost drivers relevant for this cost component are: r = reachability of collection site, sd = species diversity, gq = genetic quality, pq = physiological quality and seed health.
Cost Model Parametrisation
To populate the model, we first approximated the costs for each cost pool in each restoration approach, based on the average amounts of the reported costs (Table 2). We then searched for potential cost drivers that could explain some of the significant differences between the reported costs. Studies that explicitly stated costs gave very few indications of possible cost drivers; only the type of vegetation at the outplanting site and the restoration approach itself were mentioned as having an influence on costs [e.g., Cole et al. (2011) and Zahawi and Holl (2009)]. In order to identify further possible cost drivers, we expanded our review to articles that discussed costs in a qualitative context. In total, we identified ten cost drivers that we believed to have a significant influence on restoration costs (Table 1). We distinguished between context-dependent cost drivers, which were inherent to the specific context (e.g., the characteristics of the landscape) and cost drivers that arose as a result of people's decisions (e.g., the high genetic quality of the seed lot).
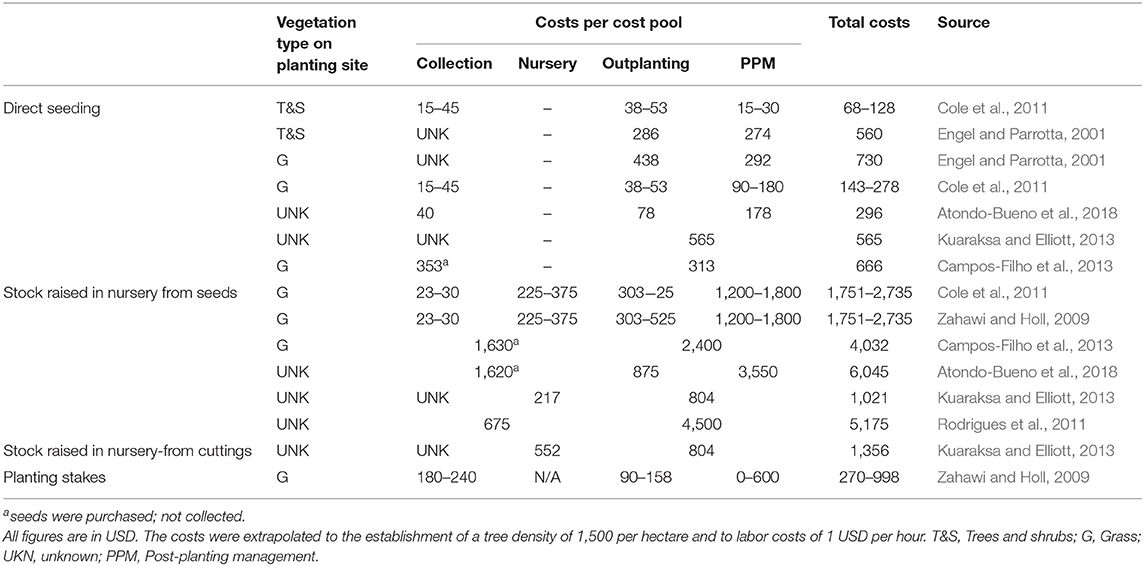
Table 2. List of all publications, with explicitly-stated cost estimates that were considered for the parametrisation of the model.
In the next step, we determined the share of the four cost components in the total costs of individual cost pools (Table 3). This was essential, because the identified cost drivers could influence the cost components to varying degrees; for example, the cost driver “vegetation at the planting site” significantly influenced labor costs, but not transportation costs. In some cases, the reported costs were sufficiently detailed to enable us to approximate the amount of each cost component and thus determine its share in a cost pool (e.g., costs associated with outplanting); in other cases, this was not possible. Instead, we derived the individual cost components based on qualitative considerations. For example, we approximated the share of labor and equipment in costs associated with post-planting maintenance based on the quantitative information provided by Engel and Parrotta (2001) in their evaluation of direct seeding. Further literature, especially practical manuals on restoration approaches, suggested that the necessary work steps in this phase differed only slightly for other restoration approaches in comparable contexts. We therefore expected the shares of each cost component to be of a similar magnitude to those of other restoration approaches.
We used a Monte Carlo (MC) simulation to explicitly account for the uncertainty associated with the coefficients that quantify the impact of various indicators. The simulation is based on the assumption that the coefficients follow a normal distribution with a specified mean given by the value of the dimensionless coefficient (between 0.7 and 1.9) and standard deviation dependent on the level of certainty of the underlying sources (see Supplementary Material for details). We defined three different standard deviations (Supplementary Material 1): 0.6 for coefficients based solely on qualitative data (highest uncertainty), 0.2 for coefficients based solely on quantitative data (lowest uncertainty), and 0.4 for coefficients based on both qualitative and quantitative data (medium uncertainty). We used Python 3.7 software to run the model multiple times for several scenarios, each with 10,000 iterations (Python Programming Language, Python 3.7, RRID:SCR_008394). The resulting cost distributions are presented in this article with the approximate mean values and the associated standard deviations. In order to show the greatest possible variance in terms of overall costs, we selected scenarios that differed as much as possible in their cost implications. The scenarios are determined by the two main factors that influence the overall restoration implementation costs more than any other factor: the restoration method and the predominant vegetation type at the planting site [e.g., Cole et al. (2011), Engel and Parrotta (2001), and Grossnickle and Ivetić (2017)]. We created a scenario matrix (restoration approach X vegetation type) and assessed the impact of genetic diversity for each resulting scenario separately.
Results
Cost Pools and Overall Costs of FLR
We calculated the costs for each restoration approach for two scenarios. One scenario assumed that the restoration site was formerly pasture and therefore mainly covered with grass; the other scenario assumed that the vegetation at the site consisted mainly of trees and shrubs. The importance of this cost driver (vegetation at the restoration site) stemmed from the fact that the type of vegetation influences, not only the scope of activities relating to outplanting and associated site preparation, but also the activities relating to post-planting maintenance and therefore result in increased labor and material costs. Our cost model showed that labor costs were about 1.7 times higher in the planting phase, and 1.9 times higher for the 2-year maintenance phase, when former pastures and corresponding grass-covered areas were restored compared to when the dominant vegetation of a restoration site consisted of trees and shrubs. Moreover, both the literature (Table 2) and our cost model showed that the preparation of the planting site, the planting activity itself, and the 2-year post-planting maintenance accounted for the largest percentage of total costs for all four planting approaches except planting stakes (Figure 2). In the case of planting stock raised in a nursery from seeds, for example, post-planting maintenance accounted for almost two-thirds (60%) of the total costs when the vegetation at the restoration site consisted mainly of grass. If the vegetation consisted of trees and shrubs, the respective share was slightly lower (52%). Thus, cost drivers that particularly influenced the costs associated with planting and post-planting maintenance had a disproportionate effect on the total costs.
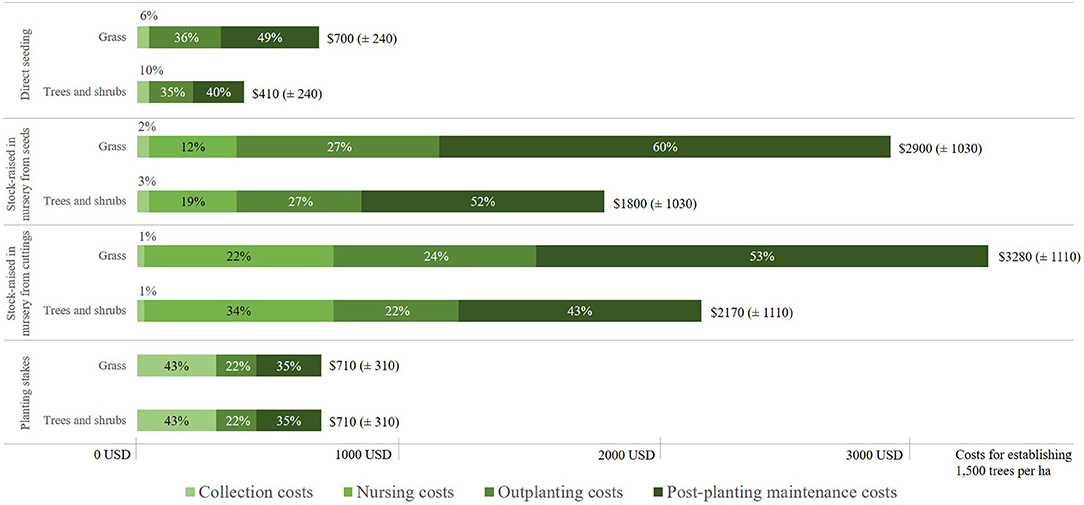
Figure 2. Share of the different cost pools in the total costs (approximate mean values and the associated standard deviations) of different restoration approaches on sites with a vegetation consisting predominantly of grass (former pasture sites) or trees and shrubs. All other cost drivers where assumed to have a medium effect on costs (e.g., medium genetic diversity).
The costs presented in Figure 2 are average costs, since all other cost drivers were set to have a medium influence on costs. The full range of costs only became visible when extreme positions of other cost drivers (e.g., high herbivory pressure or high species diversity in planted trees) were also taken into account. Costs for the restoration of pasture sites1 using stock raised in a nursery from cuttings ranged between 2,820 USD (±SD 1,020) ha−1 and 3,720 USD (±SD 940) ha−1, and for stock raised from seeds between 2,400 USD (±SD 950) ha−1 and 3,400 USD (±SD 860) ha−1. This made these two restoration approaches the most expensive ones in our study. By comparison, direct seeding and planting stakes on the same land type were more cost-efficient, with costs of between 570 USD (±SD 270) ha−1 and 830 USD (±SD 210) ha−1, and between 530 USD (±SD 270) ha−1 and 880 USD (±SD 260) ha−1, respectively. The difference in ranges for different approaches was due to the different specifications of different cost drivers.
Compared to the effort required to restore land covered by grass, the effort required for land covered by shrubs and trees was significantly lower. According to our calculations, planting stock raised in a nursery from seeds on this land cost between 1,300 USD (±SD 943) ha−1 and 2,290 USD (±SD 860) ha−1. If seeds were directly sown, the costs were between 280 USD (±SD 220) ha−1 and 550 USD (±SD 210) ha−1, but if the restoration approach involved the planting of stakes, vegetation cover had no effect on costs whatsoever.
Cost of Considering Genetic Quality
Unless otherwise stated, the examples used to illustrate the effects of considering genetic quality on costs refer to the scenario “restoration of pasture sites.”
According to our model, the costs of integrating genetic diversity into seed collection strategies significantly increased associated costs. For example, the effort of effectively capturing greater genetic diversity in seed lots increased collection costs2 from 27 USD (±SD 13) ha−1 to 36 USD (±SD 15) ha−1, representing a 33% increase in costs (Figure 3); however, relative to the total costs, these additional costs were small. For restoring pasture sites, the additional cost of 9 USD ha−1 did not represent even half a per cent of the total cost of 2,652 USD (±SD 946) ha−1. This stemmed from the fact that costs for collecting seeds and cuttings accounted for only a very small proportion of total costs (Figure 2). For restoring pasture sites with seedlings, they only accounted for 2% of total costs. By contrast, the costs for collecting stakes constituted a considerably higher proportion of the total cost for this approach; accordingly, an increase in collection costs associated with high genetic quality, from 192 USD (±SD 108) ha−1 to 277 USD (±SD 123) ha−1, accounted for 14% of the total cost.
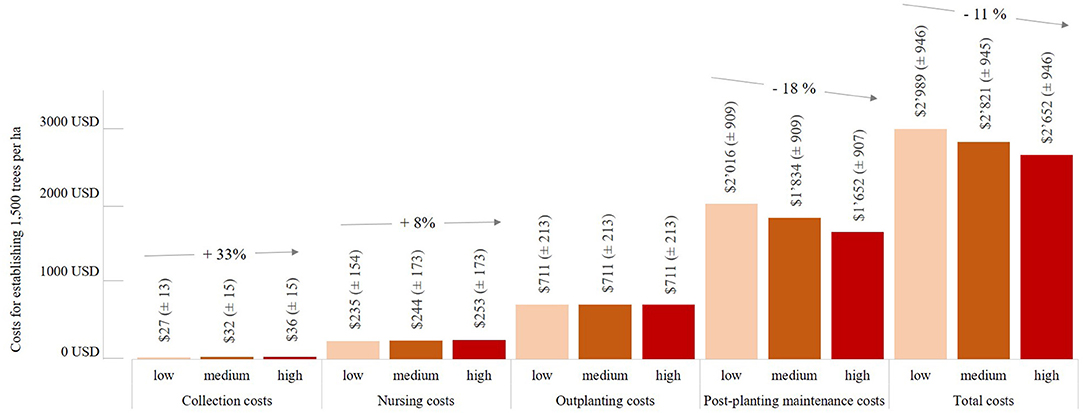
Figure 3. Effects of different categories of genetic quality (low, medium, and high) on costs (approximate mean values and the associated standard deviations) of planting stock raised in a nursery from seed on sites with a vegetation consisting predominantly of grass (former pasture sites). In this scenario, the level of impact of all other cost drivers was low.
For all other restoration approaches, the impact of high genetic quality on total costs only became significant after the seeds or seedlings had been planted on the planting site. According to the assumptions made in our model, high genetic quality reduced the costs of post-planting maintenance for planted seedlings from 2'016 USD (±SD 909) ha−1 to 1'652 USD (±SD 907) ha−1, which corresponded to an 18% decrease compared to the costs incurred when planting seedlings with low genetic quality. In total, this would result in an 11% reduction of total costs (Figure 3). The effects would be even greater if, in addition to genetic quality, physiological quality aspects and species diversity were also taken into account; in this scenario, the costs of collecting seeds would increase by 115% and the costs of nursing them by 86%. Again, the benefits would outweigh the higher costs, because the consideration of quality would ultimately reduce the total costs by 17%, mainly due to considerable reductions in maintenance costs of −36% being expected for this scenario (because fewer trees would need to be replaced).
Discussion
In this article, we have presented a bottom-up model for better understanding the broad costs of FLR in general and, specifically, estimating the costs relating to the integration of genetic diversity. We believe this to be important since, even though there is broad consensus regarding the pertinence of the resources invested in FLR (Chazdon et al., 2017), there are at least some doubts about their efficiency (Summers et al., 2015). We do not consider efficiency in this context to be the planting of as many trees as possible per invested capital, but rather the long-term establishment of a tree population that serves the aim of restoration and thus ensures the sustainability of the invested capital. A substantial body of evidence shows that genetic diversity is a key determinant for performance and survival of numerous tree species seedlings and thus contributes considerably to efficiency of restoration by increasing the viability and resilience of tree population (Kettle et al., 2008; Graudal et al., 2014; Jalonen et al., 2018). What this study provides is some evidence that investments in genetic diversity are also profitable from an economic perspective. Our model is a way to evaluate this based on evidence.
The simulated overall costs of FLR were in line with expectations, since other authors reported similar magnitudes of costs [e.g., Campos-Filho et al. (2013), Cole et al. (2011), and Kuaraksa and Elliott (2013)]. In addition to the restoration approach, and associated to that different work processes and required equipment, the characteristics of the restoration site played a decisive role in terms of costs. For these characteristics influenced not only the scope of activities related to planting and the associated preparation of the site, but also the activities related to post-planting maintenance.
The focus of this article was the simulation of the costs associated with the integration of genetic diversity into FLR. The results showed that including genetic diversity significantly increased the cost of collection, regardless of which type of germplasm was collected. The additional costs were caused by increased search and collection efforts, because a genetically diverse seed lot requires the collection of seeds from at least 10 to 100 unrelated mother trees (Sedgley et al., 1989; Jaenicke, 1999; Mortlock, 1999; Mulawarman et al., 2003; Schmidt, 2007). Since the risk of kinship decreases with increasing distance between mother trees, the distance between these mother trees should be greater than the distance over which seed dispersal is possible (Kitzmiller, 1990; Mortlock, 1999; Kindt et al., 2006; Schmidt, 2007). Although this causes a significant increase in collection costs, these additional costs are negligible relative to the total costs. The costs for collecting seeds account for only a very small proportion of total costs. The impact of high genetic quality on total costs only becomes apparent after the seedlings or seeds have been planted on the planting site. The underlying consideration is that TGR is critical for the adaptability of the seedlings to the site and, therefore, substantially affects their successful establishment and survival. Although there is broad consensus regarding the relationship between maladaptation and mortality rates (Squillace and Silen, 1962; Rogers and Montalvo, 2004; Loha et al., 2006), no studies have provided proven examples of how this could affect post-planting maintenance costs or cause the failure of projects (Rogers and Montalvo, 2004). Nevertheless, even with the most pessimistic calculations, in which collection and nursing costs (e.g., for planting seedlings raised in nurseries from seeds) would increase due to improving genetic quality by 33 and 8%, respectively, without any positive effects on the maintenance costs, the total cost would only increase by around 1%.
We encountered two main limitations during the process of developing the cost model. First, our literature review revealed a large variability for costs reported for the different phases of FLR initiatives in each study. This was to be expected, as other authors had already encountered such variability [e.g., Summers et al. (2015)]. It was not possible for us to identify the relevant underlying factors that would explain this variability, as most costs were presented in an aggregated form. This variability might be a consequence of the complexity and diversity of individual contexts in which restoration activities took place. Second, statements about costs were often based on a qualitative argumentation. On only very few occasions have projects explicitly stated costs, especially with regard to the impact of genetic diversity on costs. In order to increase the accuracy of the results, more quantitative data is needed for the parameterization of the model. It will also be valuable to develop cost-benefit models in more detail that enable long-term benefits accrued through better integration of genetic resources to be evaluated. This will however require systematic gathering of data on the benefits of specific interventions of long time periods, data which to our knowledge is currently not available.
The interdependencies in the system and their relative magnitudes, on which our conclusions are essentially based, allow a holistic understanding of cause and effect relationships in respect to costs in FLR. This is important to counteract short-sighted economic perspective. Our model helps to establish missing knowledge that illustrates appropriate incentives, such as diversity depended seed prices, for better informed decision making.
Conclusion
The model presented here is the first cost model to analyse the impact of integrating genetic diversity into FLR on costs incurred along the seed and seedling supply chain. We demonstrate the potential of genetic quality in increasing sustainability and economic efficiency. Even without any positive effects on the post-maintenance costs, the inclusion of genetic diversity would increase total costs only marginally. Moreover, there is great consensus regarding the potential positive effects of genetic diversity on the sustainability of FLR initiatives in the long term. Against this background, we believe that the costs of genetic quality are too low not to ensure its formal integration into management practices. We are convinced that a solid cost model provides an important basis for far-sighted decision-making. We share the opinion of Jalonen et al. (2014) that awareness of the benefits of genetic diversity should be developed and strengthened among all involved stakeholders, hand in hand with appropriate capacity building and economic investment in infrastructure. Investing in genetic diversity as part of FLR is the smart thing to do to ensure cost effective and resilient landscape restoration. This is an urgent call for restoration policies to recognize the importance of integrating genetic diversity in restoration planning and management.
Data Availability Statement
The original contributions presented in the study are included in the article/Supplementary Materials, further inquiries can be directed to the corresponding author/s.
Author Contributions
DN, EG, and CK designed the research. EG secured funding. DN led the analysis and CK, EG, and MZ provided advice on analysis. DN, EG, CK, and GW co-wrote the initial manuscript and DN led writing of final version. All authors contributed to further writing and editing.
Funding
This research leading to this publication was supported by the CGIAR Research Program on Forests, Trees, and Agroforestry (FTA) and its donors who supported this research through their contributions to the CGIAR Fund.
Conflict of Interest
The authors declare that the research was conducted in the absence of any commercial or financial relationships that could be construed as a potential conflict of interest.
Acknowledgments
We thank Marta Kozicka and Evert Thomas from Bioversity International, and Jarkko Koskela from FAO for helpful discussions and comments on a previous version of the manuscript.
Supplementary Material
The Supplementary Material for this article can be found online at: https://www.frontiersin.org/articles/10.3389/ffgc.2020.615682/full#supplementary-material
Footnotes
1. ^With a tree density of 1,500 ha−1 and covering all activities over the total period of the entire seed and seedling supply lifecycle, including 2 years post-planting maintenance.
2. ^Per hectare of restored land. The level of impact of all other cost drivers was assumed to be low.
References
Alfaro, R. I., Fady, B., Vendramin, G. G., Dawson, I. K., Fleming, R. A., Sáenz-Romero, C., et al. (2014). The role of forest genetic resources in responding to biotic and abiotic factors in the context of anthropogenic climate change. For. Ecol. Manag. 333, 76–87. doi: 10.1016/j.foreco.2014.04.006
Atondo-Bueno, E. J., Bonilla-Moheno, M., and Lopez-Barrera, F. (2018). Cost-efficiency analysis of seedling introduction vs. direct seeding of Oreomunnea mexicana for secondary forest enrichment. For. Ecol. Manag. 409, 399–406. doi: 10.1016/j.foreco.2017.11.028
Bonner, F. T., and Karrfalt, R. P. (2008). The Woody Plant Seed Manual, Agriculture Handbook 727. Washington, DC: US Department of Agriculture, Forest Service, 1223.
Brancalion, P. H., Meli, P., Tymus, J. R., Lenti, F. E., Benini, R. M., Silva, A. P. M., et al. (2019). What makes ecosystem restoration expensive? A systematic cost assessment of projects in Brazil. Biol. Conserv. 240:108274. doi: 10.1016/j.biocon.2019.108274
Campos-Filho, E. M., Da Costa, J. N., de Sousa, O. L., and Junqueira, R. G. (2013). Mechanized direct-seeding of native forests in Xingu, Central Brazil. J. Sustain. For. 32, 702–727. doi: 10.1080/10549811.2013.817341
Charlesworth, D., and Willis, J. H. (2009). The genetics of inbreeding depression. Nat. Rev. Genet. 10, 783–796. doi: 10.1038/nrg2664
Chazdon, R. L., Brancalion, P. H. S., Lamb, D., Laestadius, L., Calmon, M., and Kumar, C. (2017). A policy-driven knowledge agenda for global forest and landscape restoration. Conserv. Lett. 10, 125–132. doi: 10.1111/conl.12220
Cole, R. J., Holl, K. D., Keene, C. L., and Zahawi, R. A. (2011). Direct seeding of late-successional trees to restore tropical montane forest. For. Ecol. Manag. 261, 1590–1597. doi: 10.1016/j.foreco.2010.06.038
Dawson, I. K., Leakey, R., Clement, C. R., Weber, J. C., Cornelius, J. P., Roshetko, J. M., et al. (2014). The management of tree genetic resources and the livelihoods of rural communities in the tropics: Non-timber forest products, smallholder agroforestry practices and tree commodity crops. For. Ecol. Manag. 333, 9–21. doi: 10.1016/j.foreco.2014.01.021
Dedefo, K., Derero, A., Tesfaye, Y., and Muriuki, J. (2017). Tree nursery and seed procurement characteristics influence on seedling quality in Oromia, Ethiopia. For. Trees Livelihoods 26, 96–110. doi: 10.1080/14728028.2016.1221365
Engel, V. L., and Parrotta, J. A. (2001). An evaluation of direct seeding for reforestation of degraded lands in central São Paulo state, Brazil. For. Ecol. Manag. 152, 169–181. doi: 10.1016/S0378-1127(00)00600-9
Graudal, L., Aravanopoulos, F., Bennadji, Z., Changtragoon, S., Fady, B., Kjaer, E. D., et al. (2014). Global to local genetic diversity indicators of evolutionary potential in tree species within and outside forests. Forest Ecol. Manag. 333, 35–51. doi: 10.1016/j.foreco.2014.05.002
Graudal, L., and Lillesø, J. P. B. (2007). Experiences and Future Prospects for Tree Seed Supply in Agricultural Development Support – Based on Lessons Learnt in Danida Supported Programmes, 1965–2005. Copenhagen: The Ministry of Foreign Affairs of Denmark.
Greenwood, M. S., Foster, G. S., and Amerson, H. V. (1991). “Vegetative propagation of southern pines,” in Forest Regeneration Manual, eds M. L. Duryea and P. M. Dougherty (Dordrecht: Springer Netherlands), 75–86. doi: 10.1007/978-94-011-3800-0_5
Grossnickle, S. C., and Ivetić, V. (2017). Direct seeding in reforestation – a field performance review. Reforesta 94–142. doi: 10.21750/REFOR.4.07.46
Haase, D. L., Landis, T. D., and Dumroese, R. K. (2014). “Outplanting,” in Tropical Nursery Manual: A Guide to Starting and Operating a Nursery for Native and Traditional Plants, Vol. 732, eds K. M. Wilkinson, T. D. Landis, D. L. Haase, and B. F., Daley (Washington, DC: US Department of Agriculture, Forest Service), 376.
Jaenicke, H. (1999). Good tree nursery practices: practical guidelines for research nurseries. Nairobi: International Centre for Research in Agroforestry, 93.
Jalonen, R., Thomas, E., Cavers, S., Bozzano, M., Boshier, D., Bordàcs, S., et al. (2014). “Analysis of genetic considerations in restoration methods,” in Genetic Considerations in Ecosystem Restoration using Native Tree Species. State of the World's Forest Genetic Resources—Thematic Study, ed M. Bozzano et al. (Rome: FAO and Bioversity International), 249–253.
Jalonen, R., Valette, M., Boshier, D., Duminil, J., and Thomas, E. (2018). Forest and landscape restoration severely constrained by a lack of attention to the quantity and quality of tree seed: insights from a global survey. Conserv. Lett. 11:e12424. doi: 10.1111/conl.12424
Kamnerdratana, S., and Mittelman, A. (1993). Collection, Storage and Treatment of Tree Seeds, A Handbook for Small Farm Tree Planters. Bangkok: FAO Regional Wood Energy Development Programme in Asia.
Kettle, C. J., Atkinson, R., Boshier, D., Ducci, F., Dawson, I., Ekué, M., et al. (2020). Priorities, Challenges and Opportunities for Supplying Tree Genetic Resources FAO 2020. Restoring the Earth - The Next Decade, Vol. 71. Rome, 51–61.
Kettle, C. J., Ennos, R. A., Jaffre, T., Gardner, M., and Hollingsworth, P. M. (2008). Cryptic genetic bottlenecks during restoration of an endangered tropical conifer. Biol. Conserv. 141, 1953–1961. doi: 10.1016/j.biocon.2008.05.008
Kindt, R., Lilles,ø, J. P. B, Mbora, A., Muriuki, J., Wambugu, C., Frost, W., et al. (2006). Tree Seeds for Farmers: a Toolkit and Reference Source. Nairobi: World Agroforestry Centre.
Kitzmiller, J. H. (1990). Managing genetic diversity in a tree improvement program. For. Ecol. Manag. 35, 131–149. doi: 10.1016/0378-1127(90)90237-6
Kuaraksa, C., and Elliott, S. (2013). The use of Asian ficus species for restoring tropical forest ecosystems. Restor. Ecol. 21, 86–95. doi: 10.1111/j.1526-100X.2011.00853.x
Lamb, D., Erskine, P. D., and Parrotta, J. A. (2005). Restoration of degraded tropical forest landscapes. Science 310, 1628–1632. doi: 10.1126/science.1111773
Loha, A., Tigabu, M., Teketay, D., Lundkvist, K., and Fries, A. (2006). Provenance variation in seed morphometric traits, germination, and seedling growth of Cordia africana Lam. New For. 32, 71–86. doi: 10.1007/s11056-005-3872-2
Mortlock, W. (1999). FloraBank Guidelines 5: Seed Collection from Woody Plants for Local Revegetation. Canberra: FloraBank.
Mulawarman, R. J. M., Sasongko, S. M., and Iriantono, D. (2003). Tree Seed Management Seed Sources, Seed Collection and Seed Handling: A Field Manual for Field Workers and Farmers. Indonesia: Bogor, 54.
Neto, O., Aguiar, A. V., Twyford, A. D., Neaves, L. E., Pennington, R. T., and Lopes, A. V. (2014). Genetic and ecological outcomes of inga vera subsp affinis (Leguminosae) tree plantations in a fragmented tropical landscape. PLoS ONE 9:e0099903. doi: 10.1371/journal.pone.0099903
Rodrigues, R. R., Gandolfi, S., Nave, A. G., Aronson, J., Barreto, T. E., Vidal, C. Y., et al. (2011). Large-scale ecological restoration of high-diversity tropical forests in SE Brazil. For. Ecol. Manag. 261, 1605–1613. doi: 10.1016/j.foreco.2010.07.005
Rogers, D. L., and Montalvo, A. M. (2004). Genetically Appropriate Choices for Plant Materials to Maintain Biological Diversity. Report to the USDA Forest Service, Rocky Mountain Region, Lakewood, CA: University of California. Available online at: https://www.fs.USDa.gov/Internet/FSE_DOCUMENTS/fsbdev3_039080.pdf (accessed September 15, 2020).
Roshetko, J. M., Dawson, I. K., Urquiola, J., Lasco, R. D., Leimona, B., Weber, J. C., et al. (2018). To what extent are genetic resources considered in environmental service provision? A case study based on trees and carbon sequestration. Clim. Dev. 10, 755–768. doi: 10.1080/17565529.2017.1334620
Schmidt, I. B., Urzedo, D. I., Piña-Rodrigues, F. C. M., Vieira, D. L. M., Rezende, G. M., Sampaio, A. B., et al. (2018). Community-based native seed production for restoration in Brazil – the role of science and policy. Plant Biol. 21, 389–397. doi: 10.1111/plb.12842
Schmidt, L. H. (2000). Guide to Handling of Tropical and Subtropical Forest Seed. Danida Humlebaek: Forest Seed Centre.
Schmidt, L. H. (2007). Tropical Forest Seed. Berlin; Heidelberg: Springer Science & Business Media. doi: 10.1007/978-3-540-68864-8
Sedgley, M., Hand, F., Smith, R., and Griffin, A. (1989). Pollen tube growth and early seed development in Eucalyptus regnans F. Muell.(Myrtaceae) in relation to ovule structure and preferential outcrossing. Austr. J. Bot. 37, 397–411. doi: 10.1071/BT9890397
Squillace, A. E., and Silen, R. R. (1962). Racial variation in ponderosa pine. For. Sci. Monogr. 2, a0001–a0027.
Summers, D. M., Bryan, B. A., Nolan, M., and Hobbs, T. J. (2015). The costs of reforestation: a spatial model of the costs of establishing environmental and carbon plantings. Land Use Policy 44, 110–121. doi: 10.1016/j.landusepol.2014.12.002
The Bonn Challenge (2019). The Challenge: A Global Effort. Retrieved from http://www.bonnchallenge.org/content/challenge (accessed September 10, 2020).
Thomas, E., Jalonen, R., Loo, J., Boshier, D., Gallo, L., Cavers, S., et al. (2014). Genetic considerations in ecosystem restoration using native tree species. For. Ecol. Manag. 333, 66–75. doi: 10.1016/j.foreco.2014.07.015
Tito de Morais, C., Kettle, C. J., Philipson, C. D., Maycock, C. R., Burslem, D. F., Khoo, E., et al. (2020). Exploring the role of genetic diversity and relatedness in tree seedling growth and mortality: a multispecies study in a Bornean rainforest. J. Ecol. 108, 1174–1185. doi: 10.1111/1365-2745.13331
Wilkinson, K. M., Landis, T. D., Haase, D. L., Daley, B. F., and Dumroese, R. K. (2014). Tropical Nursery Manual: a Guide to Starting and Operating a Nursery for Native and Traditional Plants, Vol. 732. Washington, DC: US Department of Agriculture; Forest Service, 376.
Wymore, A. S., Bothwell, H. M., Compson, Z. G., Lamit, L. J., Walker, F. M., Woolbright, S. A., et al. (2014). “Community genetics applications for forest biodiversity and policy: planning for the future,” in Challenges and Opportunities for the World's Forests in the 21st Century, ed T. Fenning (Dordrecht: Springer), 707–725. doi: 10.1007/978-94-007-7076-8_31
Xingu Seed Network (2013). Seeds. Retrieved from http://sementesdoxingu.org.br/site/sementes/ (accessed September 10, 2020).
Keywords: genetic diversity, tree genetic resources, forest landscape restoration, costs of forest landscape restoration, tree seed and seedling supply systems, costs of genetic diversity
Citation: Nef DP, Gotor E, Wiederkehr Guerra G, Zumwald M and Kettle CJ (2021) Initial Investment in Diversity Is the Efficient Thing to Do for Resilient Forest Landscape Restoration. Front. For. Glob. Change 3:615682. doi: 10.3389/ffgc.2020.615682
Received: 09 October 2020; Accepted: 10 December 2020;
Published: 13 January 2021.
Edited by:
Peter Spathelf, Eberswalde University for Sustainable Development, GermanyReviewed by:
Stephanie Mansourian, Université de Genève, SwitzerlandChristian Temperli, Swiss Federal Institute for Forest, Snow and Landscape Research (WSL), Switzerland
Copyright © 2021 Nef, Gotor, Wiederkehr Guerra, Zumwald and Kettle. This is an open-access article distributed under the terms of the Creative Commons Attribution License (CC BY). The use, distribution or reproduction in other forums is permitted, provided the original author(s) and the copyright owner(s) are credited and that the original publication in this journal is cited, in accordance with accepted academic practice. No use, distribution or reproduction is permitted which does not comply with these terms.
*Correspondence: Danny P. Nef, ZGFubnkubmVmQHVzeXMuZXRoei5jaA==