- Institute of Bio- and Geosphere: Agrosphere (IBG-3), Forschungszentrum Jülich, Jülich, Germany
Droughts in recent years weaken the forest stands in Central Europe, where especially the spruce suffers from an increase in defoliation and mortality. Forest surveys monitor this trend based on sample trees at the local scale, whereas earth observation is able to provide area-wide information. With freely available cloud computing infrastructures such as Google Earth Engine, access to satellite data and high-performance computing resources has become straightforward. In this study, a simple approach for supporting the spruce monitoring by Sentinel-2 satellite data is developed. Based on forest statistics and the spruce NDVI cumulative distribution function of a reference year, a training data set is obtained to classify the satellite data of a target year. This provides insights into the changes in tree crown transparency levels. For the Northern Eifel region, Germany, the evaluation shows an increase in damaged trees from 2018 to 2020, which is in line with the forest inventory of North Rhine-Westphalia. An analysis of tree damages according to precipitation, land surface temperature, elevation, aspect, and slope provides insights into vulnerable spruce habitats of the region and enables to identify locations where the forest management may focus on a transformation from spruce monocultures to mixed forests with higher biodiversity and resilience to further changes in the climate system.
Introduction
Many parts of the world will suffer increasing tree mortality due to future projected climate change (Dai, 2013; Lausch et al., 2016). However, we are already within this process of higher background tree mortality rates, which have been documented on every vegetated continent and in most bioregions over the past two decades (Anderegg et al., 2013). A large portion of this forest die-off is triggered directly by drought and heat stress or indirectly through infestation-induced mortality, e.g., by the bark beetle (Sproull et al., 2016).
Steinkamp and Hickler (2015) were not able to identify a general drying trend or an increase in extreme drought events in forests globally, but they state that dry forests seem to be affected by decreasing water availability and increasing frequency of droughts. A later study by Senf et al. (2018) found that canopy mortality increased by 2.4% year–1 in Europe, resulting in a doubling of the forest area affected by this phenomenon between 1984 and 2016. Moreover, van Mantgem et al. (2009) reported widespread hydrologic changes for the western United States, such as a declining fraction of precipitation falling as snow, a declining snowpack water content, earlier spring snowmelt, and runoff, and a consequent lengthening of the summer drought. The physiological mechanisms through which drought drives tree death are a rapidly growing research area (Sala et al., 2010), but the impacts of forest die-off remain less well studied.
Even in environments that are not typically considered water-limited, this extensive tree mortality leads to a weakening of the terrestrial carbon sink and provides positive feedback to climate warming (Allen et al., 2010). Moreover, a decline in the sum of plant-level transpiration combined with a reduction of ecosystem photosynthesis leads to a decline in gross primary productivity (Anderegg et al., 2013). Tree mortality events are often “pulsed” due to their links to occasionally but in recent time more frequent drought stress. Two key characteristics determine the magnitude of these impacts, this is the magnitude of the initial “pulse” response and the recovery rate of the ecosystem (Anderegg et al., 2013). Several compensatory mechanisms (e.g., increased resource availability for the remaining trees, niche redundancy and complementarity) explain why substantial tree mortality may not necessarily translate into major changes in ecosystem fluxes (Anderegg et al., 2016).
Tree mortality is a natural ecological process. However, drought- and heat-induced mortality, including associated infestation-related forest die-off, is often a selective force that affects tree species in different ways and rapidly alters the size, age, and spatial structure of forests (Panayotov et al., 2016; Pretzsch et al., 2020). If this increase in mortality persists for a longer period, the average tree age within a forest will be reduced, resulting in a reduced average tree height, stem and crown diameter, and altered forest structure, composition, functioning, architecture, and, consequently, biodiversity and ecosystem service provision (e.g., carbon storage capacity) (van Mantgem et al., 2009; Anderegg et al., 2013). Monitoring and prediction of those tree species that might be most vulnerable are urgently needed to design mitigation strategies (O’Brien et al., 2017).
Spruce is a very fast-growing tree with 15.3 m3ha–1 a–1. The German National Forest Inventory identified an area of 2.7 Mio. ha covered with spruce in Germany (BMEL, 2015). This is about 25% of the forested area (BMEL, 2020). However, the tendency is decreasing, latest by -4% in the period from 2002 to 2012. The dramatic mortality of spruce is estimated to be about 10.5% in 2020 for North Rhine-Westphalia (NRW) (relation of dead trees to total number of evaluated trees), where other tree species show mortalities of 2.1% (MUNLV, 2020). Not only the severe damage but also the warning level for spruce crown transparency has recently been increased (BMEL, 2020). In addition to the general ecologically driven renunciation tendency in spruce monocultures, heat waves, storm impact, and bark beetle outbreaks are the main reasons. Mezei et al. (2017) call the composition of the latter three aspects the infernal trio for spruce stands. However, water limiting conditions may initialize the three aspects (Stadelmann et al., 2014; Netherer et al., 2015).
Earth observation (EO) data, i.e., satellite or ground-based observations and geospatial data, is an important source to monitor changes in forest ecosystems, especially to identify tree mortality (Lausch et al., 2016). Although costly and providing just single snapshots, typically airborne color infrared, multispectral or hyperspectral sensors are used to provide information to the authorities at the state level (Fassnacht et al., 2012; Nielsen et al., 2014; Stovall et al., 2019). For scientific research, more and more spaceborne sensor data is used. For instance, Rao et al. (2019) used passive microwave data and retrievals of vegetation optical depth as an indicator of drought-driven tree mortality at a scale of 0.25°. Latifi et al. (2018) combined coarse (250 m) Moderate Resolution Imaging Spectroradiometer (MODIS) and high resolution (5 m) RapidEye data to identify tree mortality, which they attributed to bark beetle outbreaks. It was possible to relate spectral vegetation indices to local beetle counts. Byer and Jin (2017) demonstrated that MODIS data and two-stage Random Forest models were capable of detecting the spatial patterns and severity of tree mortality with an overall producer’s accuracy of 96.3%. By feeding Gaofen-2 and Sentinel-2 data to different machine learning algorithms, Zhan et al. (2020) monitored tree mortality both at the single-tree and forest stand scale. Immitzer and Atzberger (2014) used multispectral WorldView-2 data to identify Norway Spruce mortality. For early detection, the multispectral sensor capabilities are rarely sufficient, but the identification of dead trees performed almost perfectly. Hansen et al. (2013) used the Google Earth Engine for a global assessment of forest loss by Landsat 30 m imagery with good accuracy also at the local scale where clear-cutting is common, and the fire is rare (Linke et al., 2017). Zimmermann and Hoffmann (2020) analyzed Sentinel-2 data to identify bark beetle infestations in German spruce forests, with good results over large areas where the reflectance properties have already been significantly changed. Fleming et al. (2015) used Landsat Thematic mapper data in tandem with forest inventory plot data to provide spatially and temporally explicit estimates of forest carbon stocks. These research examples show the readiness of EO data and related approaches for utilization in tree mortality detection and beyond, however, only little has found its way into the general management of practitioners or governmental monitoring programs in a straightforward operational way. The MODIS-based European forest condition monitor1 may be considered the first step to get an overview but does not provide the timeliness, and spatial resolution potential users need.
In the Northern Eifel we visually observed an increased mortality of spruce stands in the last years due to climate change. This region, therefore, may serve as an example to study the impact of severe droughts, heatwaves, and bark beetle infestation on spruce mortality using remote sensing. The intention of this study is to provide a method to quantify and monitor this process. In order to evaluate the severity of spruce damage in the Eifel region, and to support the governmental monitoring program (also to indirectly inform foresters, politicians, timber wood industry, and conservationists, etc.), up-to-date EO data can be used. However, governmental representatives may not be informed about technological innovations from other fields. The aim of this study is to introduce a cloud processing example to locate damaged spruce in the Northern Eifel region, and to evaluate potential environmental conditions for that damage. We focus here on the tree mortality and spruce health analysis after the 2018 summer drought. After an introduction to the area under investigation, it is necessary to explain the special situation of the spruce in this area to understand the environmental and artificial preconditions.
The Special Situation of Spruce in the Northern Eifel
The area of investigation has been limited to the Northern Eifel region (50.60°–50.80°N, 6.19°–6.53°E, and 532 km2, see Figure 1). More specific, this study focuses on the Rureifel, which encompasses the Eifel/Lower Rhine Valley hydrological observatory (Hasan et al., 2014; Ali et al., 2015; Bogena et al., 2018), a part of the TERrestrial ENvironmental Observatories (TERENO) (Zacharias et al., 2011; Bogena et al., 2018). The main land cover types are forests and grassland. Forest areas are covering the Venn anticline ranging from West to North and the Kermeter in the South East with forested slopes along the Rur and Kall rivers in the National Park Eifel. The elevation increases from the North East to the South West of the area of investigation. The geology mainly consists of silt- and mudstone sequences as well as sandstones and graywackes with small cavity and groundwater storage volumes (Montzka et al., 2013). The South East belongs to the so-called Mechernich Trias Triangle, where the Devonian basement is covered by variegated sandstone. The characteristic soils for the Eifel are shallow Cambisols and Leptosols.
Since the 15th century, the Northern Eifel was shaped by a pre-industrialized iron smelting economy, where the tree population provided the energy for the blast furnaces and the rivers the energy for the steel works. Extensive shifting cultivation and livestock farming put additional pressure on the forests. When the iron industry was disrupted in the region in 19th century due to the strong competition to the black coal in the Ruhr area, a large portion of the Eifel forests was cut and heathland dominated. At the Congress of Vienna in 1815, Prussia took over the former French Rhineland, including the Eifel region and started planting fast-growing trees on fallow areas to develop the economy of this poor region. Especially the Norway Spruce (Picea abies (L.) H. Karst.), since then known in the region as Prussian Tree, was established against initial refusal, which is native from Scandinavia to Northern Russia until the Ural, but also in the Alps and East European mountain ranges. Few generations later the cultivated spruce became the bread (i.e., high-yielding) tree of forestry, able to cover demands for (softwood) timber and to provide pulp for the developing paper industry. During the fierce battles of World War II in the Northern Eifel (Hurtgen Forest), tree stock was severely damaged so that, again, fast-growing spruce was planted in the 1950s to cover the bare hills. When in the 1960s many smallholders quit their business, the government of NRW supported the transfer of grassland and fields, even if not suited, to spruce monoculture. As a result of the major storm disasters of the early 1990s that laid waste to large areas of spruce forests, rethinking began. The latest disturbance to the spruce grown in the Eifel was frequent and severe droughts (see Figure 2). This is true especially for the 2018 drought, when a high-pressure system was established over central Europe and persisted nearly continuously from April to October (Buras et al., 2020). For further information about the European drought conditions we refer to the European Drought Observatory reports (Masante and Vogt, 2018).
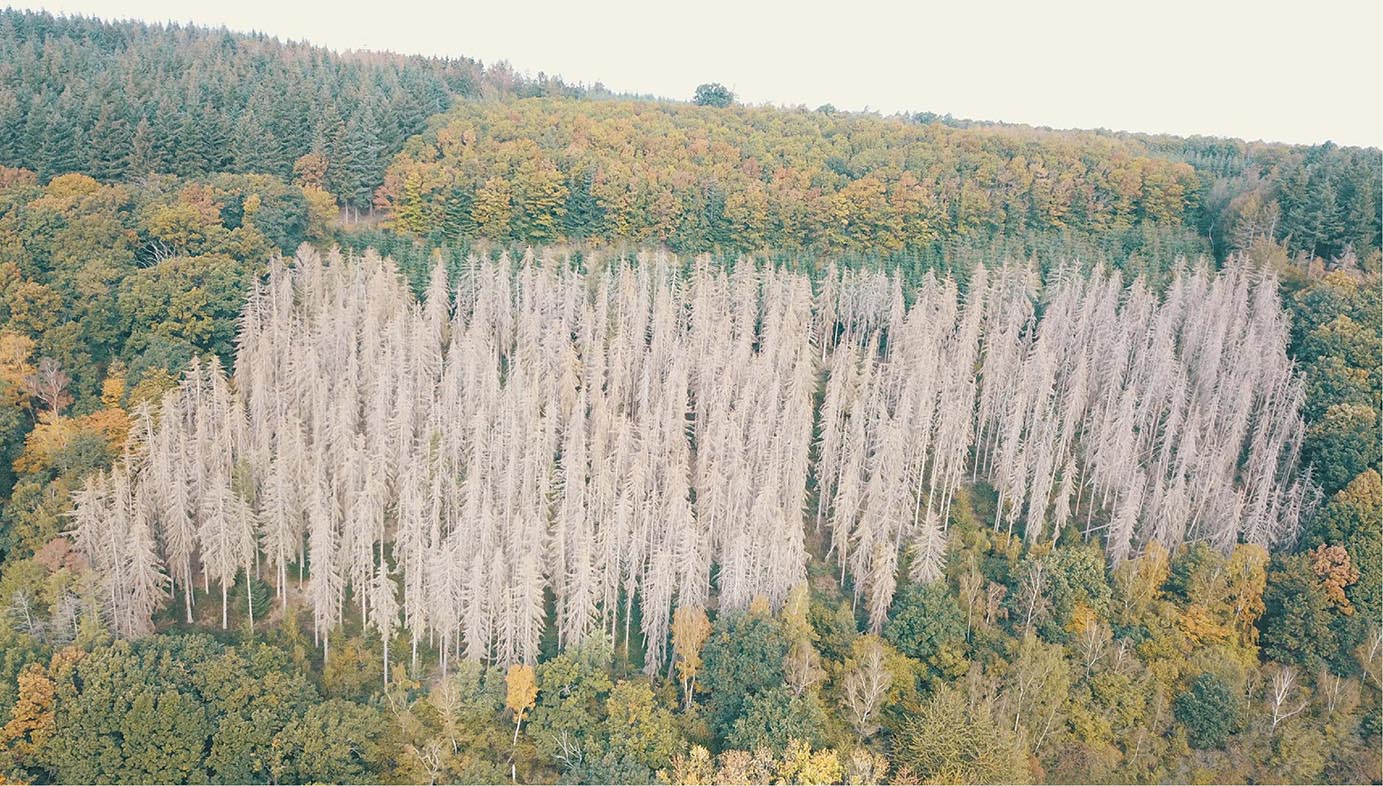
Figure 2. Typical situation of a spruce stand in the Northern Eifel in fall 2020. After the 2018 summer drought many spruce stands died and the forest management was not able to quickly remove and substitute the huge amount of affected trees.
The growing spruce forests suppressed the understory, and as a consequence, affected the biodiversity of the area. Moreover, the spruce with its shallow root system, is not well prepared for drought conditions, which become also in the Eifel more severe during recent decades. The weakened spruce stands are suffering from bark beetle attacks, the most significant natural mortality agent of mature spruce. That is why the current aim of forestry is to transform non-site-appropriate pure spruce stands into more stable and natural mixed stands or deciduous forest comprising native deciduous trees (Umweltbundesamt, 2019; Holzwarth et al., 2020). Therefore, the increased spruce mortality after the 2018 drought has, on the one hand, tragic impacts on forest management and timber wood prices, but on the other hand provides a chance for fastened forest structure transformation.
Google Earth Engine for Cloud Processing and Available Data Sets
An easy way to step into the field of EO application is to use the Google Earth Engine (GEE2). The user does not need to download and store large EO data sets, to work with unknown or complex file formats, to check the localization of different data sources, or to care about adequate information technology infrastructure such as high-performance computing facilities (supercomputers). All data is available and accessible to be processed on Google’s cloud infrastructure, from local to global geospatial scales. GEE is also designed to support the dissemination of results to other stakeholders or even the general public via Earth Engine Apps (Gorelick et al., 2017). Many solutions based on JavaScript are available, and the number is continuously increasing. Via Application Programming Interfaces (APIs) also Python and R code can be implemented. Small changes in location or time scale make published code easy to apply for own purposes. In this study, we use GEE also to analyze the data and for the preparation of figures.
Sentinel-2 Multispectral Data
The main analysis is based on the multispectral imaging mission Sentinel-2. With four bands at 10 m, six bands at 20 m, and three bands at 60 m spatial resolution Sentinel-2 covers the visible and near-infrared as well as the shortwave infrared regions of the electromagnetic spectrum (Drusch et al., 2012). The mission consists of two satellites launched in June 2015 (Sentinel-2A) and March 2017 (Sentinel-2B), resulting in a revisit time of 2–3 days at mid-latitudes. The data set available at the Earth Engine Data Catalog (S2_SR) is bottom-of-atmosphere surface reflectance (Level 2A) product already corrected for atmosphere, terrain and cirrus impact by sen2cor toolbox (Richter et al., 2012). The image collection was filtered by the cloudy pixel percentage property with values lower than 10% and the median was calculated based on available data in July and August for selected years. Bands B2 (Blue), B3 (Green), B4 (Red), B5 (Red Edge 1), B8 (Near Infra-Red), B11 (Shortwave Infrared 1), and B12 (Shortwave Infrared 2) are used for the analysis by calculating the band-wise temporal median, Bands B4 and B8 of the median were used to calculate the Normalized Difference Vegetation Index (NDVI) (Rouse et al., 1973).
SMAP Soil Moisture Data and Soil Moisture Anomalies
In order to visualize the drought conditions during the summer of 2018 and 2019 in relation to normal years, Soil Moisture Active and Passive (SMAP) satellite soil moisture retrievals were selected. The available data set at the Earth Engine Data Catalog has been developed by the Hydrological Science Laboratory (HSL) at NASA’s Goddard Space Flight Center in cooperation with the United Stated Department of Agriculture (USDA) Foreign Agricultural Services and USDA Hydrology and Remote Sensing Lab (Sazib et al., 2018). SMAP is a passive microwave sensor providing spatially enhanced soil moisture estimates at 9 km resolution with overpasses in mid-latitudes of 2–3 days. The product at hand is based on the SMAP Level 3 product which was transferred to a 10 km grid. This data is assimilated into the two-layer Palmer water balance model by a one-dimensional ensemble Kalman filter approach to reduce forcing and observation errors as well as to provide a root zone soil moisture product (Bolten et al., 2010). Here the depth of the effective root zone is defined according to the Food and Agriculture Organization of the United Nations (FAO) digital soil map of the world. The anomalies consider the deviation of the current conditions relative to the average of the available period standardized by the climatological standard deviation, where the climatology values are estimated based on the full data record of the satellite observation period over a 31-day moving window.
Statistical Forest Data of Crown Transparency
The evaluation of the forest conditions in Germany is performed yearly through the national forestry inventory (BMEL, 2020). The vitality of German forests is characterized by a visual inspection of treetops in the months July and August in a systematic 16 km∗ 16 km sampling grid. This is enhanced (to a 4 km∗ 4 km scheme) for the federal state of NRW (MUNLV, 2020). Sentinel-2 scenes have been selected during these inspection months.
As tree health cannot be easily characterized, and as multiple definitions exist, the forest inventory makes use of a single indicator, the tree crown transparency. Tree canopies may have been affected by damaging insect pests or fungal pathogens, so that the impact is often manifested through changes in tree crown conditions. In particular the attributes foliage discoloration and crown defoliation are found to provide good indicators of tree health leading to crown transparency (Metzger and Oren, 2001; Borianne et al., 2017).
During national forestry inventory, experts group trees by international standards into 5% steps of crown transparency. These are again grouped into five stages of tree damage, and these again into three groups indicating healthy trees (no increased crown transparency), a warning level (slightly increased crown transparency) and seriously damaged trees (considerably increased crown transparency) (Wellbrock et al., 2018). The standard error of the statistics is given with 2.3% (BMEL, 2020).
Auxiliary Data for Analysis of Mortality-Dependence
Land surface temperature (LST) at daytime has been selected from the Terra LST and Emissivity 8-Day Global 1km data set provided in the Earth Engine Data Catalog. Thermal infra-red bands 31 and 32 of MODIS were fed into a generalized split-window LST algorithm, where first-order topographic information (i.e., elevation) is considered in radiative transfer simulations. We analyzed LST data from June to September in 2018 and 2020 with a focus on summer droughts.
In addition, the dependence of crown transparency on precipitation is analyzed. The long-term monthly precipitation of the years 2007–2018 are provided at 1 km in the OpenLandMap data set (Hengl, 2018). The approach behind uses METOP Advanced Scatterometer (ASCAT) soil moisture information fed into the SM2RAIN processor to retrieve precipitation (Brocca et al., 2019). Via cubic splines it has been downscaled to 1 km and averaged between WorldClim, CHELSA, and IMERGE precipitation products. The long-term August precipitation is selected for spatial spruce health analysis here.
Elevation data is taken from the Shuttle Radar Topography Mission (SRTM) V3 C-band product at a resolution of 1 arc-second, i.e., ∼30 m at the equator (Farr et al., 2007). Gaps and voids have been filled by additional data such as the ASTER Global Digital Elevation Model 2 (Fujisada et al., 2012). Aspect and slope were calculated from the elevation data by GEE terrain functions.
Approach
The approach makes use of the forest health statistics applied to an analysis of the NDVI obtained by Sentinel-2. Two main assumptions provide the foundation of the analysis: The first assumption considers the spruce health statistics of NRW to be representative also for the spruce stands in the Northern Eifel. Noteworthy spruce stands can be found in the South of NRW only. Next to the Northern Eifel region, especially in the Sauerland and to a lower extent the Bergisches Land and the Weserbergland have spruce coverage. Those regions have comparable elevations (100–600 m), similar geology being German low mountain ranges developed in Devon and Trias, and with this comparable soil genesis. I.e., the environmental conditions are similar to the Northern Eifel. If more specific spruce crown transparency level data for the region of interest is available, this can be implemented similarly. Second, it is assumed that the tree health continuously increases with NDVI. With the previously mentioned crown transparency damage levels and their related foliage discoloration and crown defoliation, a reduction in chlorophyll content can be observed. Therefore, the assumption of reduced NDVI with increasing tree damage is in general valid and supported by many studies (Lausch et al., 2013; Misurec et al., 2016; Spruce et al., 2019; Gomez et al., 2020; Bryk et al., 2021).
Based on these preconditions, our simple approach evaluates the NDVI of the reference year and identifies NDVI thresholds for the groups damaged spruce and spruce at warning level. Applying the same thresholds to the target year instead of the reference one, data can be classified accordingly. In this study, we selected 2018 as the reference year and 2020 as the target year. All data is clipped to the area of interest to provide the regional statistics. Without this selection, the analysis can also be applied to larger scales, but the statistics calculation in GEE is limited to a certain number of pixels. A workaround is to reduce the spatial resolution of the analysis.
First, for the reference year the median Sentinel-2 data was classified by a supervised support vector machine (SVM) method. Training polygons were provided for the classes spruce, other forest, water and others. The last class includes polygons for built-up areas, grassland and agriculture. The Radial Basis Function (RBF) kernel is used to solve non-linear problems with intermediate hyperplane (gamma of 0.5) and margin (cost of 10) parameters (Cortes and Vapnik, 1995). Our classification approach has been employed before, for instance, Wessel et al. (2018) successfully implemented a similar method for tree species classification using Sentinel-2 data. The area of the resulting spruce class for the reference year is then further analyzed, the other classes are neglected. All spruce class NDVI values are used to calculate the histogram and the related CDF. In correspondence with the forest statistics indicating the percentage of damaged (warning level) spruce, the NDVI threshold for the specific percentage of pixels is retrieved. The CDF in the physical NDVI domain provides the probability that the NDVI is below the threshold value for damaged spruce (NDVID). Here for the reference year 2018 the NRW forest statistics indicate that 37% of the spruce is damaged:
Similarly, the statistics indicate that 36% of the spruce forest in 2018 has reached the warning level. This level is used to retrieve the respective threshold NDVIW:
Due to the large number of pixels and histogram bins we omitted fitting a CDF function and used the original spruce NDVI CDF, i.e., the empirical CDF, instead with a negligible error.
Once NDVID and NDVIW have been retrieved based on the reference year, with a random selection of respective pixels (here 10% of each level) in the three levels, a supervised SVM was trained based on the reference year Sentinel-2 median composite bands. The same parameters for the classification into spruce transparency levels were used as in the previous SVM application for the land cover classification. In addition to the classes provided in the forest statistics, we wanted to separately identify removed spruce stands. The SVM was trained on representative areas of the 2020 Sentinel-2 data. This information is then applied to classify the 2020 target year Sentinel-2 median composite. However, intermediate results showed significant differences in the reflectance values of the median composites of the reference and target years. The calculation of the median was not able to fully compensate for that. Therefore a CDF matching approach was applied for each median composite band to correct for biases and to map a target image pixel value to a corresponding value in the reference CDF that has the equivalent cumulative probability value. This approach ensures full comparability of Sentinel-2 data from different years, where atmospheric conditions were not fully corrected, or where observation angles or illumination conditions were different. Note that this CDF matching was applied to the full median image and not for the spruce class only.
The area fractions of the three classes of spruce health conditions are calculated for the target year. In addition, the classes are evaluated regarding their frequency in specific levels of LST, elevation, slope, and aspect. The processing of the Sentinel-2 data is performed at 20 m scale for performance reasons. The histogram analysis related to the mentioned environmental preconditions is performed at 50 m resolution.
Results and Discussion
Drought Conditions
The root zone soil moisture anomalies (dating back to 2015) spatially averaged for the Northern Eifel region are presented in Figure 3. They indicate the relative differences between the years to rate the relative dryness during the seasons. Whereas 2016 had a very wet summer and 2017 was a normal year, the recent years exhibit serious drought conditions. In late May 2018 the significant drought began and lasted until the end of the year. During the winter 2018/2019 rainfall was not sufficient to restore the soil water storage capacity. Already in March 2019 soil moisture conditions were slightly lower than the average, but here the specific preconditions from 2018 and the long duration of 7 months was a challenge for some ecosystems. The year 2020 was characterized by a very dry spring separated by few precipitation events from a dry summer.
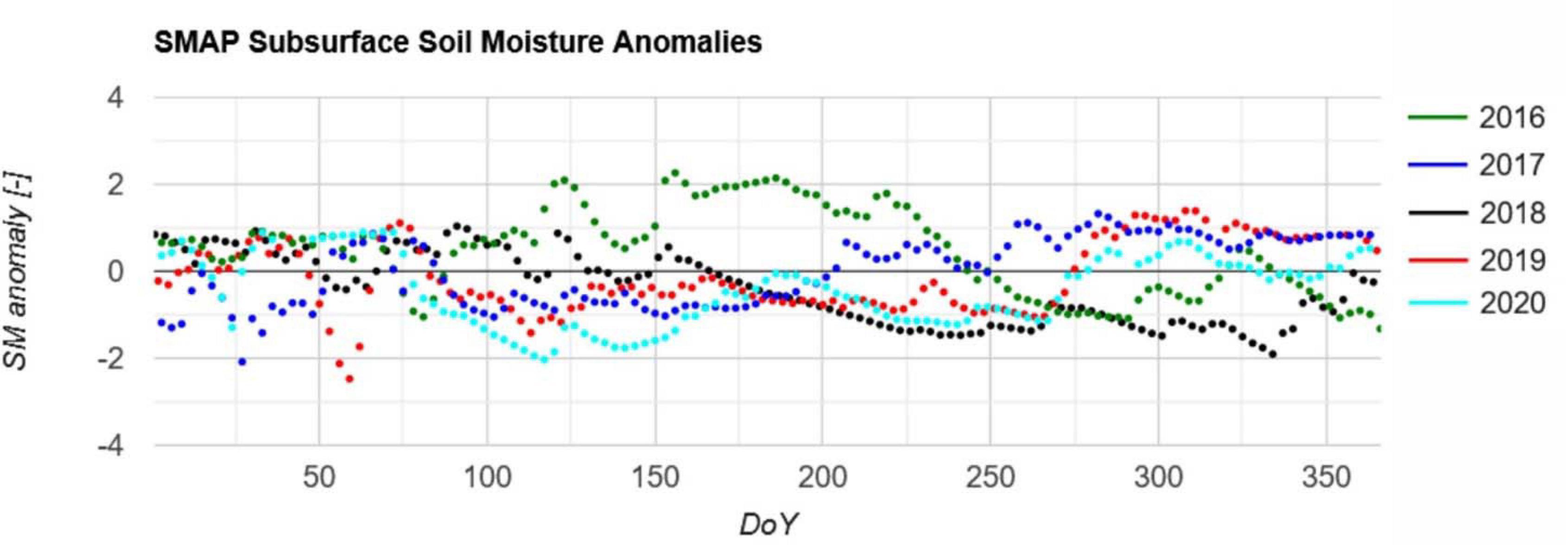
Figure 3. SMAP subsurface soil moisture anomalies for the years 2016–2020 averaged over the area of investigation. Anomalies were calculated with respect to the reference period 2015–2020. Due to the non-continuous 2–3 day acquisitions of SMAP it is shown as a point diagram.
This illustrates that in recent years 2018–2020 several ecosystems affected by dry weather conditions. In addition, the spruce stands were placed on the barren Eifel regions with shallow soils (Montzka et al., 2008a,b), which were even less productive for agriculture. The shallow soils over the bedrock have a very low total water storage capacity so that longer dry periods cannot be bridged. These two corresponding factors lead to the increased spruce mortality in the Northern Eifel. Another driver is bark beetle infestation, e.g., Netherer et al. (2019) report for Austrian forests that dry and shallow soil conditions are typically less affected, but acute drought proved to raise the probability of bark beetle attacks. As the low soil depth is not adequately reflected by the higher resolution soil information provided by the Earth Engine Data Catalog, e.g., the OpenLandMap soil texture map, a more detailed analysis may provide misleading results within GEE. Interested analysts may implement the NRW soil map 1:50.000 for this purpose.
Identification of the Area Covered by Spruce
The SVM classification results of the reference year 2018 were evaluated with independent validation samples. The kappa coefficient (Hudson and Ramm, 1987) for the classification is given with 0.965. The confusion matrix indicates that only the class other has misclassifications, the class spruce was perfectly classified. The separability of spruce is remarkable, especially when using the full potential of the Sentinel-2 bands. Visual inspection of the classification results indicate minor spruce misclassifications at the coastlines of the lakes, where the spectral signature of mixed pixels and the re-greening of fallen dry areas after the summer period is closest to the spruce class. The classification is shown in Figure 4, in line with the multispectral Sentinel-2 data of 2018 and 2020 (Figure 5).
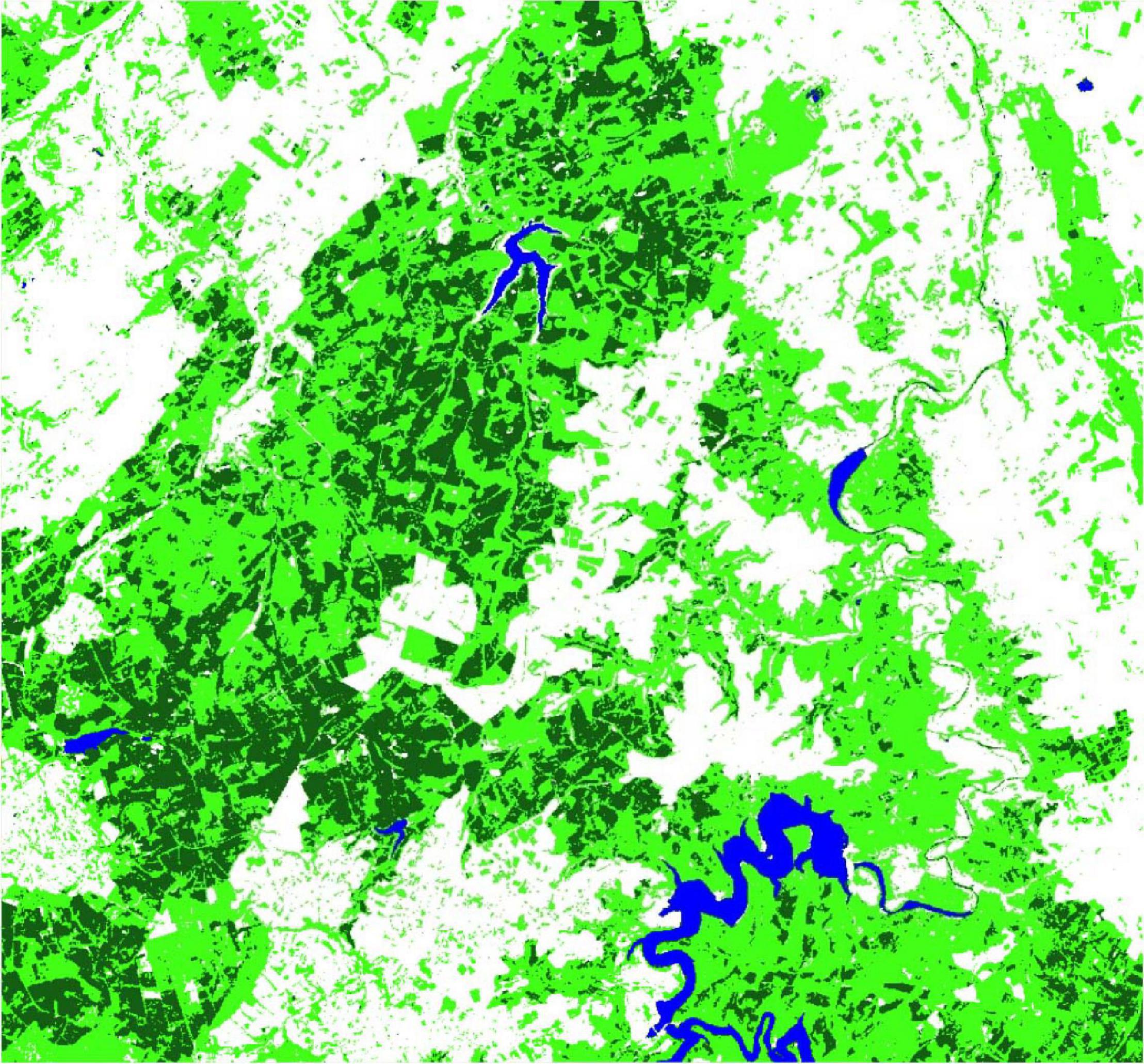
Figure 4. Support Vector Machine (SVM) classification result of the Sentinel-2 2018 median composite of the Northern Eifel (dark green, spruce; light green, other forest; blue, water; and white, other).
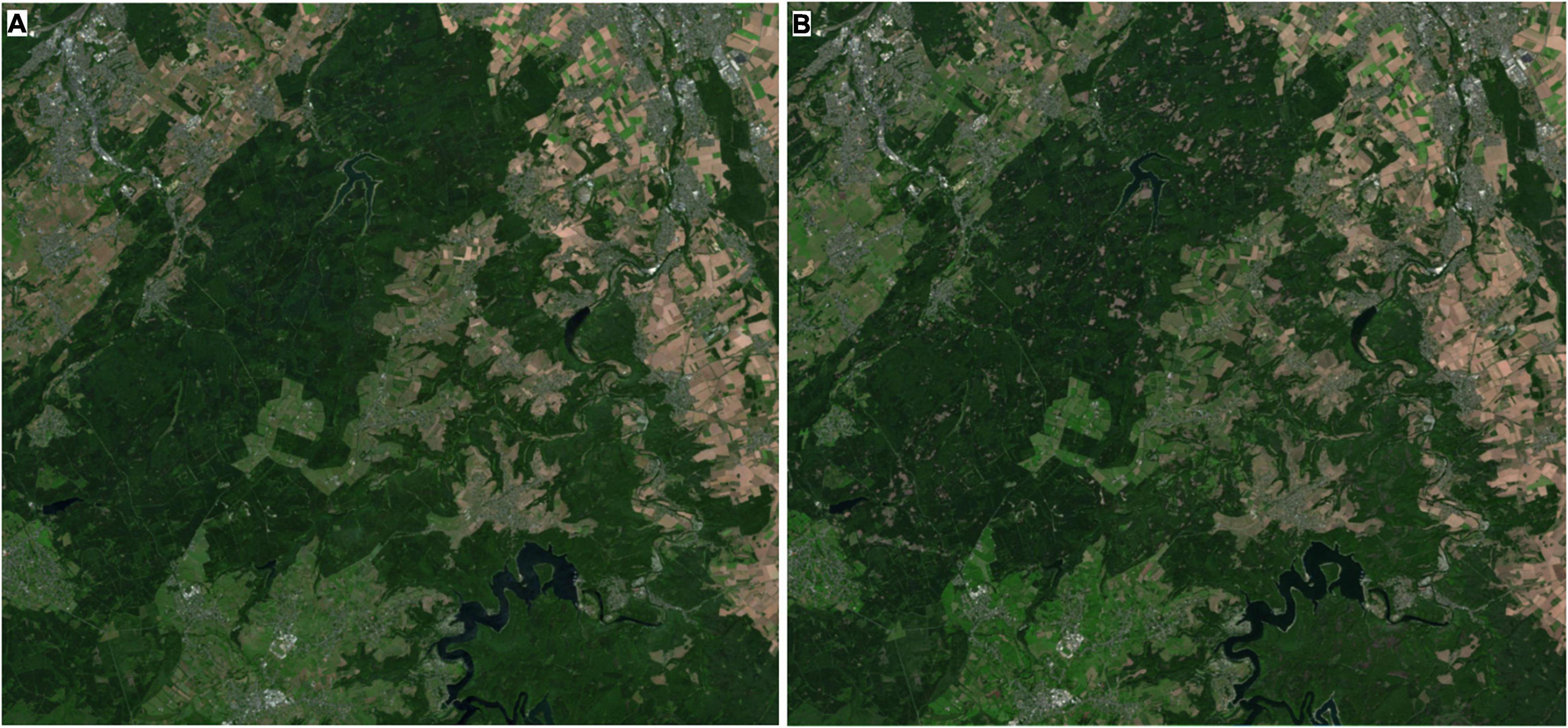
Figure 5. Sentinel-2 July/August median composite (RGB = B4, B3, and B2) (A) for the year 2018, and (B) for the year 2020 (CDF-matched).
Quantifying Tree Health Classes
From the 2018 forest statistics, the damaged and warning level percentages for spruce (i.e., 37% and 36%, respectively) were applied to the NDVI CDF to obtain the threshold values to differentiate between the three treetop transparency levels. The corresponding 2018 histogram and CDF are presented in Figure 6. The spruce NDVI histogram for 2018 is close to Gaussian with a mean of 0.78, but slightly skewed with a tail to lower NDVI. The CDF provides the resulting thresholds NDVID and NDVIW with 0.774 and 0.808, respectively. This is in line with other studies evaluating spruce health or bark beetle outbreaks (Lastovicka et al., 2020; Zimmermann and Hoffmann, 2020).
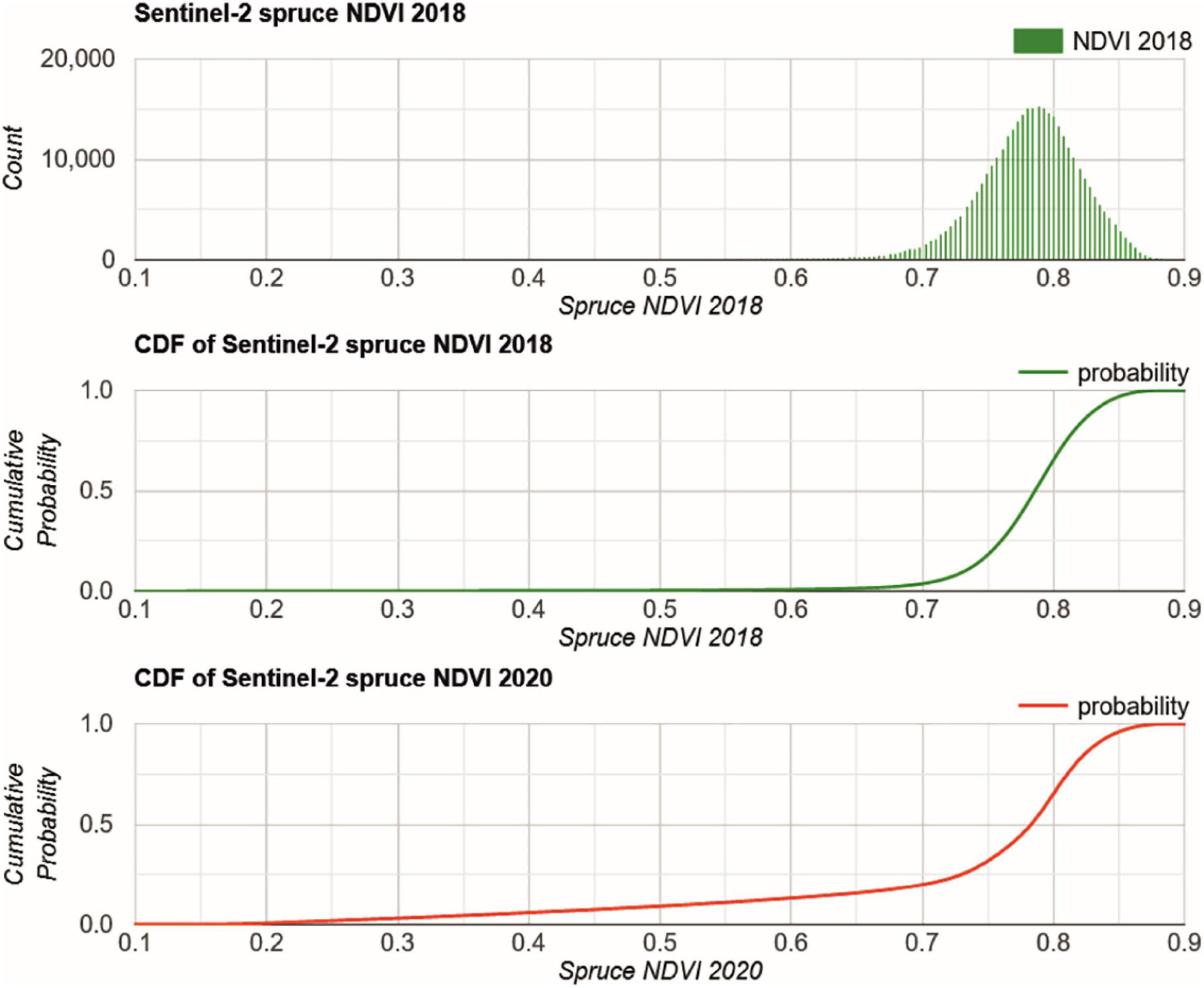
Figure 6. GEE histogram (top) and CDF (center) of spruce NDVI 2018 and CDF (bottom) of spruce 2020. The CDF 2018 is used to identify the NDVI threshold for damaged and warning level spruce according to the forest statistics.
In 2020, the CDF delivered a different picture (Figure 6). Here, the CDF is continuously increasing from 0.15, where removed spruce stands are characterized with low NDVI. After the 2018 drought and later tree removal the NDVI is increasing in some locations according to the occasional re-greening of the understory.
After identification of the spruce health levels based on the CDF matched Sentinel-2 median composite for 2020, their fractions were calculated (Table 1). The estimation of the area fraction falling into the healthy class was close to that of the forest statistics, i.e., 28.4% and 26%, respectively. The class warning level is overestimated and the class damaged is underrepresented in the remote sensing estimation, i.e., the high amount of 45% of damaged spruce is not fully captured with an area fraction of 38.3%. In this class, the damaged and removed spruce stands are combined. 13.8% of the spruce can be considered damaged as they have been already removed.
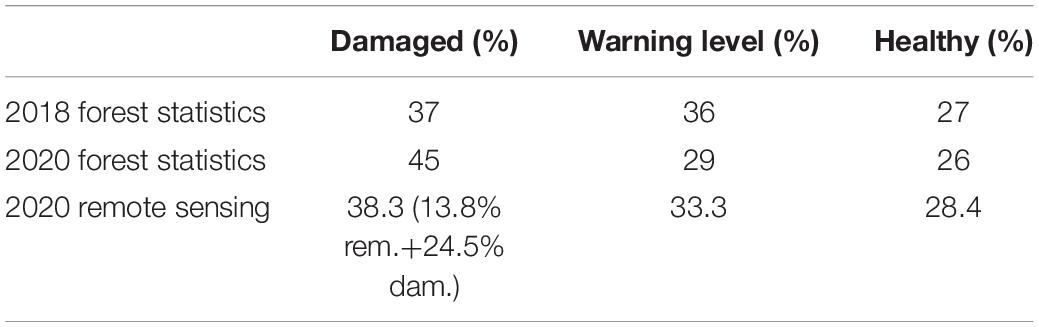
Table 1. Fractions of classified spruce health conditions by the forest statistics and by the proposed remote sensing approach.
The band-wise CDF matching of the target year Sentinel-2 data to the reference year is very important. Otherwise, not adequately compensated atmospheric conditions may impact the threshold application and, therefore the relative area fractions of the three main classes. Here, advanced atmospheric correction methods, e.g., using pseudo-invariant features (Schott et al., 1988) or the iteratively re-weighted multivariate alteration detection (Canty and Nielsen, 2008), may improve the accuracy of the method applied.
Figure 7 shows the spatial extent of the spruce health levels in the Northern Eifel. The removed and damaged classes appear more compact than the warning level and healthy classes. For removed this is clearly related to the forest management activities typically applied at larger patches, whereas for the damaged class this might be an indication for the environmental preconditions on spruce vitality. Figure 7 and the visual inspection of the landscape, such as in Figure 2 suggest that no single trees are affected, but large patches of spruce monocultures. In addition to the genotype of the spruce stand in a parcel, which may show a specific resilience to droughts, also common factors such as soil water storage capacity or precipitation levels may affect a parcel of spruce as a whole. Further analysis of these environmental preconditions is provided in section “The Dependency of Spruce Health on Environmental Conditions.” The division between the warning level and healthy classes is not that clearly localized, and gradually draws through the parcels. Here, more research is needed if the NDVI is the adequate vegetation index to identify transparency levels of still dense spruce canopies.
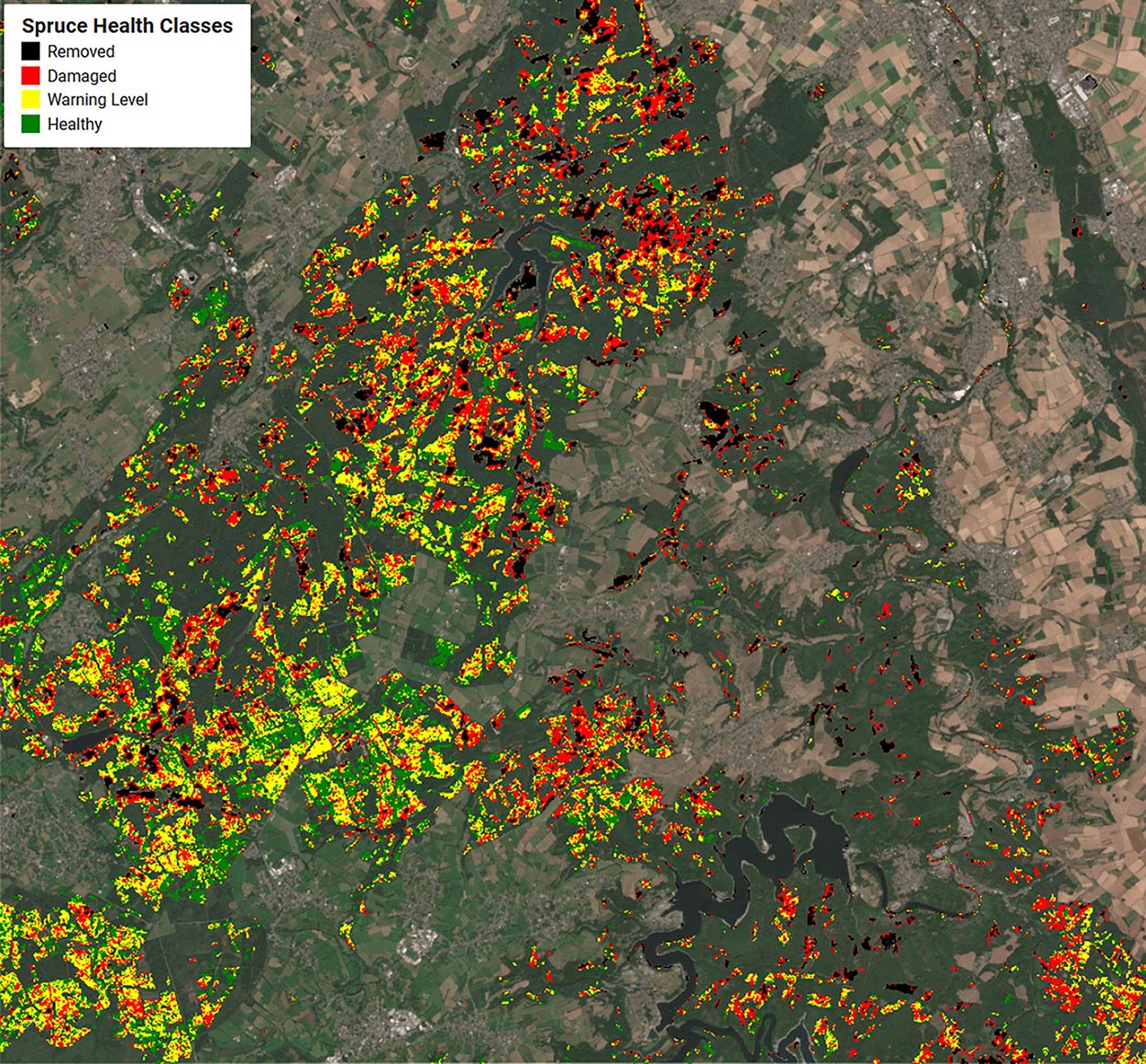
Figure 7. 2020 spruce health classes removed (black), damaged (red), warning level (yellow), and healthy (green) estimated by this study in respect to the CDF matched Sentinel-2 median composite of 2020. The region of interest covers an area of 533 km2.
In Figure 8 we show a detailed example for the period July to August in 2018 and 2020. The upper panel depicts Sentinel-2 median composite for the reference year 2018 and the changes after two years for the same period of the year (middle and lower panel). First, the two RGB images appear very similar as a result of the CDF matching. This is not only the case for the bands B2–B4 used to construct the RGB, but also for the other bands including B8 which has been selected for calculating the NDVI (B4 and B8). Second, the cleared patches in 2020 are very prominent, and by comparing with the classification at the bottom, it is indeed the spruce that has been removed only. Those locations are very well captured by the procedure presented before. Moreover, the severely damaged spruce sites are dominating in the Eastern part of this section, originating from both the lower elevated area with generally less precipitation and the lee side to the prevailing West winds, with relatively dryer downward oriented air masses. The relationships of the spruce vitality levels toward environmental conditions is discussed in section “The Dependency of Spruce Health on Environmental Conditions”.
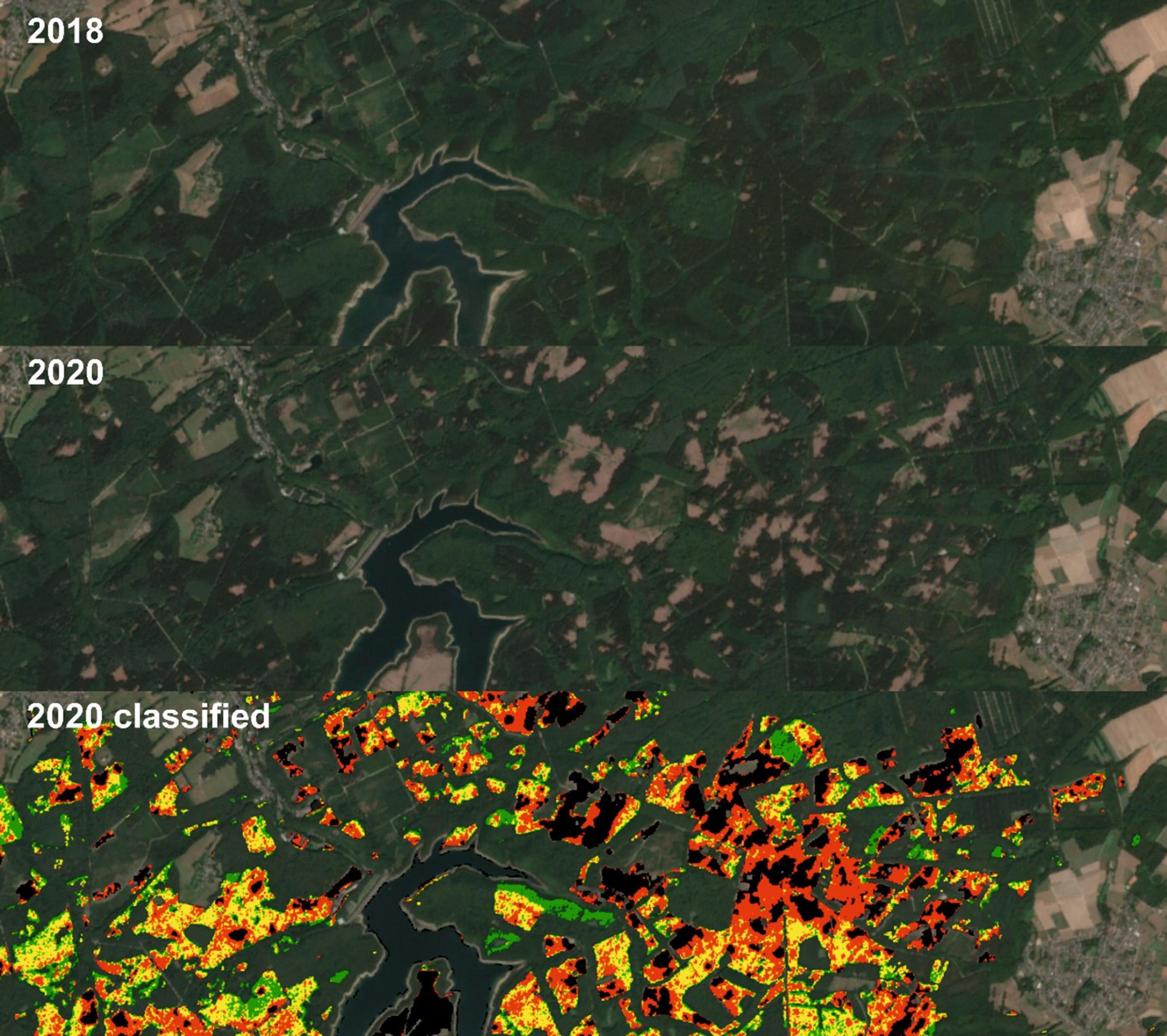
Figure 8. Sentinel-2 data (RGB = B4, B3, and B2) of the Wehe dam area in the Venn anticline for 2018 (top), 2020 (middle, CDF matched), and estimated spruce health classes in 2020 (bottom, removed = black, damaged = red, warning level = yellow, and healthy = green).
Foresters confirm that the main reason for the spruce mortality in the Northern Eifel is the drought conditions in recent years. Earlier studies in German spruce forests already reported the impact at dryer sites, where crown transparency was higher in years following hot summers (Seidling et al., 2012). However, small fractions are also affected by windfall, which may not be directly considered by the forest statistics applied here. A special incidence may support this interpretation: On March 13, 2019 a tornado devastated houses and forests close to the village of Roetgen in the South West of the investigated area. Figure 9 shows the conditions before and after the tornado and the corresponding spruce health classification for 2020. The damages within the tornado track is clearly visible in the 2020 Sentinel-2 scene, but a 4 km sampling scheme of the forest statistics would not be able to identify the full dimension.
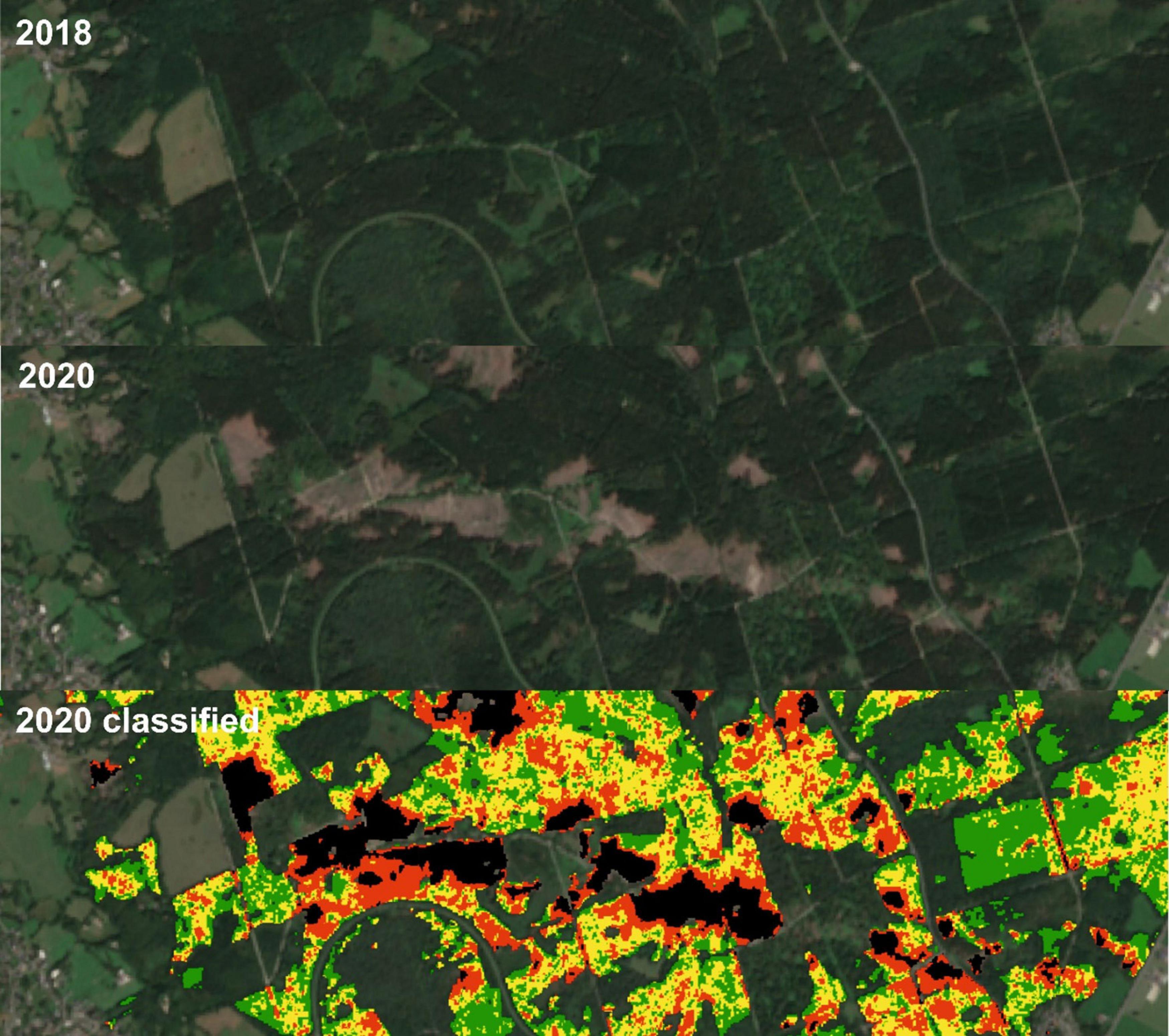
Figure 9. Sentinel-2 median composite (RGB = B4, B3, and B2) for the year 2018 before the tornado (top), 2020 after the tornado (middle, CDF matched), and estimated spruce health classes in 2020 (bottom, removed = black, damaged = red, warning level = yellow, and healthy = green).
The Dependency of Spruce Health on Environmental Conditions
The different levels of Norway Spruce vitality in the Northern Eifel were analyzed with respect to their dependency on precipitation, LST, elevation, slope, and aspect. Figure 10 shows both the absolute pixel counts at 50 m resolution in the function of these factors as well as the relative fractions normalized by total spruce.
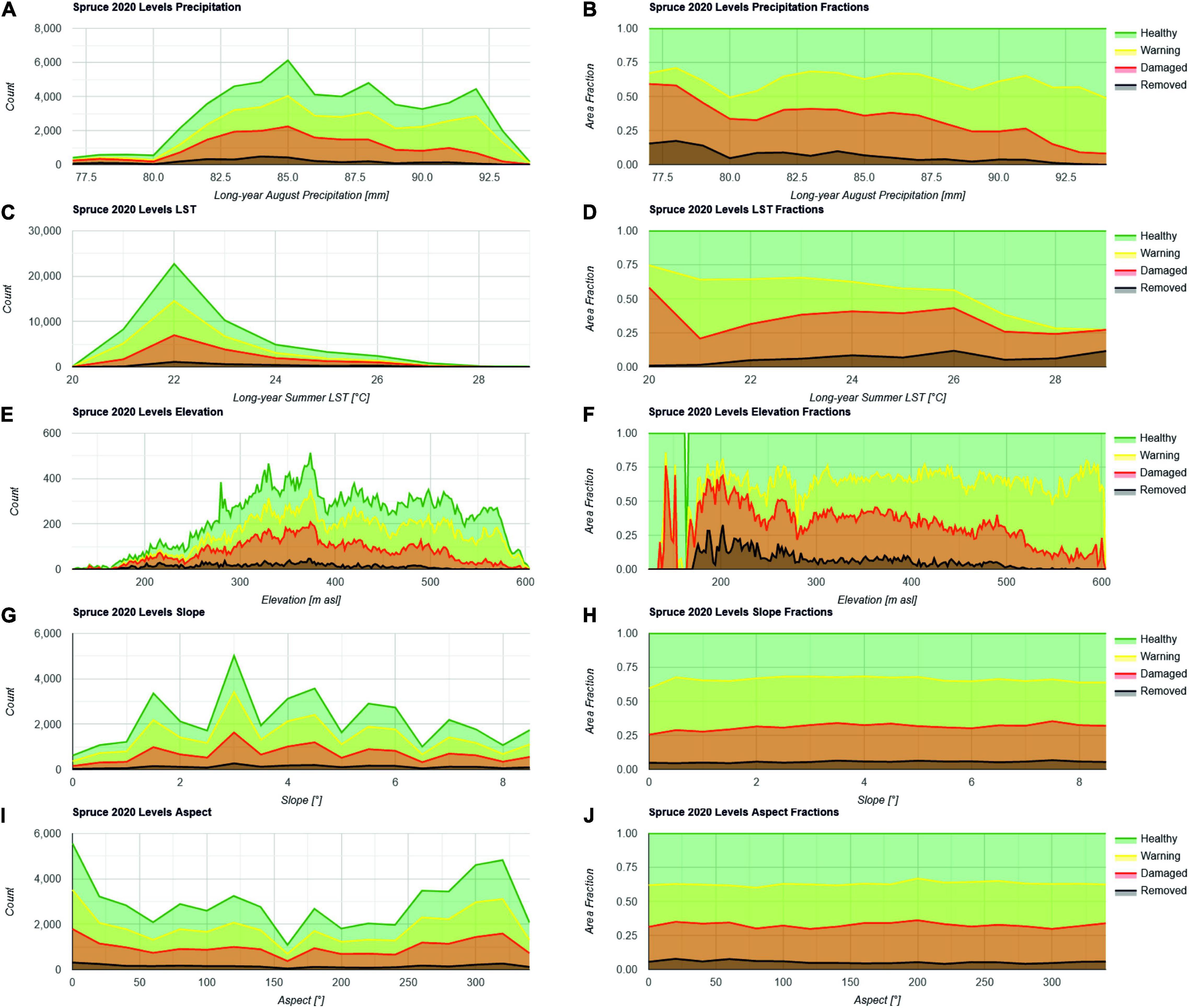
Figure 10. Histograms of spruce health levels expressed in number of pixels showing a specific health level for 2020 in relation to the environmental conditions such as precipitation in August (A,B) summer land surface temperature from June to September 2018–2020 (C,D) elevation (E,F) slope (G,H) and aspect (I,J). Both absolute pixel counts (left) and relative spruce area fractions (right) are shown.
Figure 10A shows the typical August precipitation (X-axis) ranging between 77 and 93 mm (panels A and B). The importance of precipitation with respect to spruce vitality was already demonstrated with its related soil moisture in Figure 3. The Y-axis shows the absolute pixel counts classified as spruce. Most spruce trees receive precipitation between 82 and 92 mm. Figure 10B shows that there is a clear trend in increasing fractions of removed and damaged spruce with lower precipitation. This indicates that precipitation (deficit) might be considered as one of the main drivers of spruce mortality, and that dryer areas require higher attention for transforming a monoculture spruce forest into a resilient mixed broadleaved forest. However, wetter areas are classified in the warning level already predicting further impacts of anticipating changes in the climate system.
The analysis of the summer LST distribution over the spruce locations shown in Figures 10C,D has been performed in order to clarify the impact of relative energy portions through insolation and a loss in transpirable water for plant cooling. This yields that most spruce forests receive a LST of 22°C. When ignoring the few stands with summer LST < 21°C and >26°C, also a trend becomes visible. Increasing mortality is documented with increasing summer LST. Similar to precipitation, the spruce at warning level is evenly distributed over the area, predicting that also lower summer LST may not help to reduce mortality in the future.
Figures 10E,F supports the hypothesis that removed and damaged trees can be increasingly found with lower elevations from 600 m to 200 m asl. The general perception in Figure 7 is that the negative impact increases from South West to North East, which may be in line with a general decrease in elevation. This hypothesis is supported by Figure 10. It is obvious that the same patterns arise from elevation and from summer precipitation and LST, which coincide in the area of investigation.
The distribution of spruce vitality levels according to the steepness of slopes shown in Figures 10G,H has its origin in the assumption, that soils at steeper hangs are shallower than soils in flat areas due to gravity forces and erosion. Those shallow soils above bedrock may have lower water holding capacity indicating a lower resilience of the cultivated trees to drought conditions. With slopes up to 8° in the Northern Eifel according to the 1 arc-second resolution SRTM elevation model, no trend is visible. Damaged spruce might be slightly increasing with slope, but this is not significant.
Figures 10I,J shows the relation between the orientation of the hillslope toward the sun expressed in degrees counting clock-wise with 0° and 360° being North and the various health levels. Examining this relationship foots on the assumption that the direction toward direct solar radiation relatively modifies evapotranspiration and, therefore, the soil water storage. Here, no significant trend is visible, but there is a small tendency of damaged spruce showing higher fractions at 160–200°, i.e., the orientation toward South.
In summary, there is evidence for trends in the spatial distribution of impact on spruce stands, where precipitation and the corresponding LST and elevation dependencies are most significant. A smaller trend is visible for the aspect according to the insolation, but the slope does not seem to have an effect.
Conclusion and Outlook
In this study, we presented an approach that allows to transfer forest monitoring statistics of a reference year (2018) to Sentinel-2 data to identify different spruce crown transparency levels. We made use of the spruce vegetation index CDF to identify parcels with severe crown transparency and a warning level where the tree health was already affected. In addition, their area fraction was estimated for a target year (2020) as well to support the statistical evaluation and reporting. Within two years, in the Northern Eifel region 14% of the spruce had to be removed, as they fell victim to the extreme drought conditions in recent years. The fraction of trees with severe crown transparency has increased to 38.3% (by including those of removed ones), which shows the dramatic situation in the typical Norway Spruce monocultures in the area. Only 28.3% remains in good condition. The area fractions are supported by the recent forest statistics of 2020. Against the background that the Norway Spruce is not endemic in the Eifel, current and future forest management needs to move toward alternative species such as Chestnut and American Red Oak.
An extension or transfer of the study to Federal State level or further Central European spruce areas such as the Thuringian Forest, the Erzgebirge, Hunsrück, Taunus, Westerwald, Rothaargebirge, Harz or the Southern regions of the Eifel is possible by selecting few adequate training data. Conducting the statistical evaluation of the results on GEE, however, is a limiting factor for selecting the region size. Besides that, also national-scale evaluations of the spruce health are feasible to identify spruce locations under pressure. The utilization of the so-called Level II environmental monitoring sites for forest vitality for that purpose could lead to a standardized spruce monitoring tool (Seidling, 2004). Similarly, machine learning approaches may be trained by data obtained at the 4×4 km sampling grid to predict actual spruce vitality levels.
Although it was the interest to provide a robust tool to evaluate the spruce health conditions in German low mountain ranges, several improvements are still possible. For example, while the NDVI is a robust metric, it may have problems with saturation and non-linearity with forest canopies (Stone and Mohammed, 2017). Alternative vegetation indices may improve the results at hand and may better differentiate affected and non-affected spruce stands by overcoming NDVI saturation effects. Red-edge dependent indices, water-related indices involving a SWIR band, or a combination of multiple indices may improve the method’s performance (see e.g., Hawrylo et al., 2018; Abdullah et al., 2019; Lastovicka et al., 2020). Our approach was to support the statistical evaluation of tree health, so that the timing of the observations was aligned to the in situ survey. However, Solberg (2004) discussed the causal mechanisms of Norway spruce crown conditions and droughts and observed that the defoliation resulted from increased needle-fall in the autumn after dry summers. Therefore, postponing the in situ and remote observations may identify the most recent effects of droughts on spruce stands. Moreover, technical improvements such as the implementation of the Python API instead of JavaScript application would increase the flexibility of the tool in terms of data processing as well as the secondary analysis of the environmental preconditions.
In this study a significant dependency of cut and damaged spruce was reported to precipitation and elevation, a clear sign for the limited resilience of the Norway spruce to increasing drought conditions and a climate change. The spruce mortality stands as well as their environmental conditions identified here can be used to develop a variety of transformation scenarios (Hilmers et al., 2020) and to predict potential secondary infestation (Lausch et al., 2013). The proposed method may support the in situ forest evaluation as well as governmental monitoring programs in order to prioritize management practices or to identify highly vulnerable spruce stands. The approach implemented for the Northern Eifel case study may serve as a blueprint for similar analyses in spruce forests affected by climate change.
Code Availability
The GEE tool can be accessed and the JavaScript code can be tested and implemented for own purposes via the following link: https://code.earthengine.google.com/cde6a13b3c35a72a5d6bf0c3e5a3ea7a.
Data Availability Statement
The original contributions presented in the study are included in the article/supplementary material, further inquiries can be directed to the corresponding author.
Author Contributions
CM: conceptualization, methodology, implementation, visualization, and initial draft. BB, AT, DM, and HV: review and editing the manuscript. All authors contributed to the article and approved the submitted version.
Funding
We acknowledge support from the German Federal Ministry of Economics and Technology for the AssimEO project under the grant 50EE1914A, the German Federal Ministry of Education and Research for the Agricultural Systems of the Future project DAKIS (grant 031BO729F), and from the Helmholtz research infrastructure Modular Observation Solutions for Earth Systems (MOSES).
Conflict of Interest
The authors declare that the research was conducted in the absence of any commercial or financial relationships that could be construed as a potential conflict of interest.
Footnotes
References
Abdullah, H., Skidmore, A. K., Darvishzadeh, R., and Heurich, M. (2019). Sentinel-2 accurately maps green-attack stage of European spruce bark beetle (Ips typographus, L.) compared with Landsat-8. Remote Sens. Ecol. Con. 5, 87–106. doi: 10.1002/rse2.93
Ali, M., Montzka, C., Stadler, A., Menz, G., Thonfeld, F., and Vereecken, H. (2015). Estimation and validation of rapideye-based time-series of leaf area index for winter wheat in the rur catchment (Germany). Remote Sens. Basel. 7, 2808–2831. doi: 10.3390/Rs70302808
Allen, C. D., Macalady, A. K., Chenchouni, H., Bachelet, D., McDowell, N., Vennetier, M., et al. (2010). A global overview of drought and heat-induced tree mortality reveals emerging climate change risks for forests. For. Ecol. Manag. 259, 660–684. doi: 10.1016/j.foreco.2009.09.001
Anderegg, W. R. L., Kane, J. M., and Anderegg, L. D. L. (2013). Consequences of widespread tree mortality triggered by drought and temperature stress. Nat. Clim. Change 3, 30–36. doi: 10.1038/nclimate1635
Anderegg, W. R. L., Martinez-Vilalta, J., Cailleret, M., Camarero, J. J., Ewers, B. E., Galbraith, D., et al. (2016). When a tree dies in the forest: scaling climate-driven tree mortality to ecosystem water and carbon fluxes. Ecosystems 19, 1133–1147. doi: 10.1007/s10021-016-9982-1
BMEL (2020). Ergebnisse der Waldzustanderhebung 2019. Bonn: Federal Ministry of Food and Agriculture (BMEL).
Bogena, H. R., Montzka, C., Huisman, J. A., Graf, A., Schmidt, M., Stockinger, M., et al. (2018). The TERENO-rur hydrological observatory: a multiscale multi-compartment research platform for the advancement of hydrological science. Vadose Zone J. 17:55. doi: 10.2136/vzj2018.03.0055
Bolten, J. D., Crow, W. T., Zhan, X. W., Jackson, T. J., and Reynolds, C. A. (2010). Evaluating the utility of remotely sensed soil moisture retrievals for operational agricultural drought monitoring. IEEE J. Select. Top. Appl. Earth Observ. Rem. Sens. 3, 57–66. doi: 10.1109/Jstars.2009.2037163
Borianne, P., Subsol, G., and Caraglio, Y. (2017). Automated efficient computation of crown transparency from tree silhouette images. Comput. Electron. Agr. 133, 108–118. doi: 10.1016/j.compag.2016.12.011
Brocca, L., Filippucci, P., Hahn, S., Ciabatta, L., Massari, C., Camici, S., et al. (2019). SM2RAIN-ASCAT (2007-2018): global daily satellite rainfall data from ASCAT soil moisture observations. Earth Syst. Sci. Data 11, 1583–1601. doi: 10.5194/essd-11-1583-2019
Bryk, M., Kolodziej, B., and Pliszka, R. (2021). Changes of norway spruce health in the bialowie(z) over dota forest (CE Europe) in 2013-2019 during a bark beetle infestation, studied with landsat imagery. Forests 12:10034. doi: 10.3390/f12010034
Buras, A., Rammig, A., and Zang, C. S. (2020). Quantifying impacts of the 2018 drought on European ecosystems in comparison to 2003. Biogeosciences 17, 1655–1672. doi: 10.5194/bg-17-1655-2020
Byer, S., and Jin, Y. F. (2017). Detecting drought-induced tree mortality in sierra nevada forests with time series of satellite data. Remote Sens. Basel. 9:ARTN929. doi: 10.3390/rs9090929
Canty, M. J., and Nielsen, A. A. (2008). Automatic radiometric normalization of multitemporal satellite imagery with the iteratively re-weighted MAD transformation. Remote Sens. Environ. 112, 1025–1036. doi: 10.1016/j.rse.2007.07.013
Cortes, C., and Vapnik, V. (1995). Support-vector networks. Mach. Learn. 20, 273–297. doi: 10.1023/A:1022627411411
Dai, A. G. (2013). Increasing drought under global warming in observations and models (vol 3, pg 52, 2013). Nat. Clim. Change 3, 171–171. doi: 10.1038/Nclimate1811
Drusch, M., Del Bello, U., Carlier, S., Colin, O., Fernandez, V., Gascon, F., et al. (2012). Sentinel-2: ESA’s optical high-resolution mission for GMES operational services. Remote Sens. Environ. 120, 25–36. doi: 10.1016/j.rse.2011.11.026
Farr, T. G., Rosen, P. A., Caro, E., Crippen, R., Duren, R., Hensley, S., et al. (2007). The shuttle radar topography mission. Rev. Geophys. 45:RG2004. doi: 10.1029/2005rg000183
Fassnacht, F. E., Latifi, H., and Koch, B. (2012). An angular vegetation index for imaging spectroscopy data-preliminary results on forest damage detection in the Bavarian National Park, Germany. Int. J. Appl. Earth Obs. 19, 308–321. doi: 10.1016/j.jag.2012.05.018
Fleming, A. L., Wang, G. X., and McRoberts, R. E. (2015). Comparison of methods toward multi-scale forest carbon mapping and spatial uncertainty analysis: combining national forest inventory plot data and landsat TM images. Eur. J. For. Res. 134, 125–137. doi: 10.1007/s10342-014-0838-y
Fujisada, H., Urai, M., and Iwasaki, A. (2012). Technical Methodology for ASTER Global DEM. IEEE Transact. Geosci. Remote Sens. 50, 3725–3736. doi: 10.1109/Tgrs.2012.2187300
Gomez, D. F., Ritger, H. M. W., Pearce, C., Eickwort, J., and Hulcr, J. (2020). Ability of remote sensing systems to detect bark beetle spots in the southeastern US. Forests 11:1167.
Gorelick, N., Hancher, M., Dixon, M., Ilyushchenko, S., Thau, D., and Moore, R. (2017). Google earth engine: planetary-scale geospatial analysis for everyone. Remote Sens. Environ. 202, 18–27. doi: 10.1016/j.rse.2017.06.031
Hansen, M. C., Potapov, P. V., Moore, R., Hancher, M., Turubanova, S. A., Tyukavina, A., et al. (2013). High-resolution global maps of 21st-century forest cover change. Science 342, 850–853. doi: 10.1126/science.1244693
Hasan, S., Montzka, C., Rüdiger, C., Ali, M., Bogena, H., and Vereecken, H. (2014). Soil moisture retrieval from airborne L-band passive microwave using high resolution multispectral data. ISPRS J. Photogram. Remote Sens. 91, 59–71.
Hawrylo, P., Bednarz, B., Wezyk, P., and Szostak, M. (2018). Estimating defoliation of scots pine stands using machine-learning methods and vegetation indices of Sentinel-2. Eur. J. Remote Sens. 51:194. doi: 10.1080/22797254.2018.1469337
Hengl T. (ed.). (2018). Monthly Precipitation in mm at 1 km Resolution Based on SM2RAIN-ASCAT 2007-2018, IMERGE, CHELSA Climate and WorldClim. Castellon: OpenLandMap.
Hilmers, T., Biber, P., Knoke, T., and Pretzsch, H. (2020). Assessing transformation scenarios from pure Norway spruce to mixed uneven-aged forests in mountain areas. Eur. J. For. Res. 139, 567–584. doi: 10.1007/s10342-020-01270-y
Holzwarth, S., Thonfeld, F., Abdullahi, S., Asam, S., Da Ponte Canova, E., Gessner, U., et al. (2020). Earth observation based monitoring of forests in Germany: a review. Remote Sens. Basel 12:3570.
Hudson, W., and Ramm, C. W. (1987). Correct formation of the kappa coefficient of agreement. Photogram. Eng. Remote Sens. 53, 421–422.
Immitzer, M., and Atzberger, C. (2014). Early detection of bark beetle infestation in norway spruce (Picea abies, L.) using WorldView-2 data. Photogram. Fernerk. 14, 351–367. doi: 10.1127/1432-8364/2014/0229
Lastovicka, J., Svec, P., Paluba, D., Kobliuk, N., Svoboda, J., Hladky, R., et al. (2020). Sentinel-2 data in an evaluation of the impact of the disturbances on forest vegetation. Remote Sens. Basel. 12:1914. doi: 10.3390/rs12121914
Latifi, H., Dahms, T., Beudert, B., Heurich, M., Kubert, C., and Dech, S. (2018). Synthetic RapidEye data used for the detection of area-based spruce tree mortality induced by bark beetles. Gisci. Remote Sens. 55, 839–859. doi: 10.1080/15481603.2018.1458463
Lausch, A., Erasmi, S., King, D. J., Magdon, P., and Heurich, M. (2016). Understanding forest health with remote sensing -part IA review of spectral traits, processes and remote-sensing characteristics. Remote Sens. Basel. 8:1029. doi: 10.3390/rs8121029
Lausch, A., Heurich, M., Gordalla, D., Dobner, H. J., Gwillym-Margianto, S., and Salbach, C. (2013). Forecasting potential bark beetle outbreaks based on spruce forest vitality using hyperspectral remote-sensing techniques at different scales. For. Ecol. Manag. 308, 76–89. doi: 10.1016/j.foreco.2013.07.043
Linke, J., Fortin, M. J., Courtenay, S., and Cormier, R. (2017). High-resolution global maps of 21st-century annual forest loss: independent accuracy assessment and application in a temperate forest region of Atlantic Canada. Remote Sens. Environ. 188, 164–176. doi: 10.1016/j.rse.2016.10.040
Masante, D., and Vogt, J. (2018). Drought in Central-Northern Europe – August 2018. JRC European Drought Observatory (EDO), ed. EDO Analytical Report. Available online at: http://edo.jrc.ec.europa.eu/documents/news/EDODroughtNews201808_Central_North_Europe.pdf (accessed June 14, 2019).
Metzger, J. M., and Oren, R. (2001). The effect of crown dimensions on transparency and the assessment of tree health. Ecol. Appl. 11, 1634–1640.
Mezei, P., Jakus, R., Pennerstorfer, J., Havasova, M., Skvarenina, J., Ferencik, J., et al. (2017). Storms, temperature maxima and the Eurasian spruce bark beetle Ips typographus-An infernal trio in Norway spruce forests of the Central European High Tatra Mountains. Agr. For. Meteorol. 242, 85–95. doi: 10.1016/j.agrformet.2017.04.004
Misurec, J., Kopackova, V., Lhotakova, Z., Campbell, P., and Albrechtova, J. (2016). Detection of Spatio-temporal changes of norway spruce forest stands in ore mountains using landsat time series and airborne hyperspectral imagery. Remote Sens. Basel. 8:92. doi: 10.3390/rs8020092
Montzka, C., Bogena, H. R., Weihermüller, L., Jonard, F., Bouzinac, C., Kainulainen, J., et al. (2013). Brightness temperature and soil moisture validation at different scales during the SMOS validation campaign in the Rur and Erft catchments, Germany. IEEE Transact. Geosci. Remote Sens. 51, 1728–1743. doi: 10.1109/TGRS.2012.2206031
Montzka, C., Canty, M., Kreins, P., Kunkel, R., Menz, G., Vereecken, H., et al. (2008a). Multispectral remotely sensed data in modelling the annual variability of nitrate concentrations in the leachate. Environ. Model. Softw. 23, 1070–1081. doi: 10.1016/j.envsoft.2007.11.010
Montzka, C., Canty, M., Kunkel, R., Menz, G., Vereecken, H., and Wendland, F. (2008b). Modelling the water balance of a mesoscale catchment basin using remotely sensed land cover data. J. Hydrol. 353, 322–334. doi: 10.1016/j.jhydrol.2008.02.018
MUNLV (2020). Waldzustandsbericht 2020. Ministerium für Umwelt, Landwirtschaft, Natur- und Verbraucherschutzdes Landes Nordrhein-Westfalen, Düsseldorf. Düsseldorf: MUNLV.
Netherer, S., Matthews, B., Katzensteiner, K., Blackwell, E., Henschke, P., Hietz, P., et al. (2015). Do water-limiting conditions predispose Norway spruce to bark beetle attack? New Phytol. 205, 1128–1141. doi: 10.1111/nph.13166
Netherer, S., Panassiti, B., Pennerstorfer, J., and Matthews, B. (2019). Acute drought is an important driver of bark beetle infestation in austrian norway spruce stands. Front. Glob. Chang. 2:39. doi: 10.3389/ffgc.2019.00039
Nielsen, M. M., Heurich, M., Malmberg, B., and Brun, A. (2014). Automatic mapping of standing dead trees after an insect outbreak using the window independent context segmentation method. J. For. 112, 564–571. doi: 10.5849/jof.13-050
O’Brien, M. J., Engelbrecht, B. M. J., Joswig, J., Pereyra, G., Schuldt, B., Jansen, S., et al. (2017). A synthesis of tree functional traits related to drought-induced mortality in forests across climatic zones. J. Appl. Ecol. 54, 1669–1686. doi: 10.1111/1365-2664.12874
Panayotov, M., Kulakowski, D., Tsvetanov, N., Krumm, F., Barbeito, I., and Bebi, P. (2016). Climate extremes during high competition contribute to mortality in unmanaged self-thinning Norway spruce stands in Bulgaria. For. Ecol. Manag. 369, 74–88. doi: 10.1016/j.foreco.2016.02.033
Pretzsch, H., Grams, T., Haberle, K. H., Pritsch, K., Bauerle, T., and Rotzer, T. (2020). Growth and mortality of Norway spruce and European beech in monospecific and mixed-species stands under natural episodic and experimentally extended drought. Results of the KROOF throughfall exclusion experiment. Trees Struct. Funct. 34, 957–970. doi: 10.1007/s00468-020-01973-0
Rao, K., Anderegg, W. R. L., Sala, A., Martinez-Vilalta, J., and Konings, A. G. (2019). Satellite-based vegetation optical depth as an indicator of drought-driven tree mortality. Remote Sens. Environ. 227, 125–136. doi: 10.1016/j.rse.2019.03.026
Richter, R., Louis, J., and üller-Wilm, U. M. (2012). Sentinel-2 MSI—Level 2A Products Algorithm Theoretical Basis Document. Darmstadt: Telespazio VEGA Deutschland GmbH.
Rouse, J. W., Haas, R. H., Schell, J. A., and Deering, D. W. (1973). Monitoring the Vernal Advancement and Retrogradation (Green Wave Effect) of Natural Vegetation. College Station, TX: Remote Sensing Centre, Texas A&M University.
Sala, A., Piper, F., and Hoch, G. (2010). Physiological mechanisms of drought-induced tree mortality are far from being resolved. New Phytol. 186, 274–281. doi: 10.1111/j.1469-8137.2009.03167.x
Sazib, N., Mladenova, I., and Bolten, J. (2018). Leveraging the google earth engine for drought assessment using global soil moisture data. Remote Sens. Basel. 10:1265. doi: 10.3390/rs10081265
Schott, J. R., Salvaggio, C., and Volchok, W. J. (1988). Radiometric scene normalization using pseudoinvariant features. Remote Sens. Environ. 26, 1–16. doi: 10.1016/0034-4257(88)90116-2
Seidling, W. (2004). Crown condition within integrated evaluations of level II monitoring data at the German level. Eur. J. For. Res. 123, 63–74. doi: 10.1007/s10342-004-0014-x
Seidling, W., Ziche, D., and Beck, W. (2012). Climate responses and interrelations of stem increment and crown transparency in Norway spruce, Scots pine, and common beech. For. Ecol. Manag. 284, 196–204. doi: 10.1016/j.foreco.2012.07.015
Senf, C., Pflugmacher, D., Zhiqiang, Y., Sebald, J., Knorn, J., Neumann, M., et al. (2018). Canopy mortality has doubled in Europe’s temperate forests over the last three decades. Nat. Commun. 9:4978. doi: 10.1038/s41467-018-07539-6
Solberg, S. (2004). Summer drought: a driver for crown condition and mortality of Norway spruce in Norway. For. Pathol. 34, 93–104. doi: 10.1111/j.1439-0329.2004.00351.x
Sproull, G. J., Adamus, M., Szewczyk, J., Kersten, G., and Szwagrzyk, J. (2016). Fine-scale spruce mortality dynamics driven by bark beetledisturbance in Babia Gora National Park, Poland. Eur. J. For. Res. 135, 507–517. doi: 10.1007/s10342-016-0949-8
Spruce, J. P., Hicke, J. A., Hargrove, W. W., Grulke, N. E., and Meddens, A. J. H. (2019). Use of MODIS NDVI products to map tree mortality levels in forests affected by mountain pine beetle outbreaks. Forests 10:811. doi: 10.3390/f10090811
Stadelmann, G., Bugmann, H., Wermelinger, B., and Bigler, C. (2014). Spatial interactions between storm damage and subsequent infestations by the European spruce bark beetle. For. Ecol. Manag. 318, 167–174. doi: 10.1016/j.foreco.2014.01.022
Steinkamp, J., and Hickler, T. (2015). Is drought-induced forest dieback globally increasing? J. Ecol. 103, 31–43. doi: 10.1111/1365-2745.12335
Stone, C., and Mohammed, C. (2017). Application of remote sensing technologies for assessing planted forests damaged by insect pests and fungal pathogens: a review. Curr. Rep. 3, 75–92. doi: 10.1007/s40725-017-0056-1
Stovall, A. E. L., Shugart, H., and Yang, X. (2019). Tree height explains mortality risk during an intense drought. Nat. Commun. 10:4385. doi: 10.1038/s41467-019-12380-6
Umweltbundesamt (2019). Monitoringbericht 2019 zur Deutschen Anpassungsstrategie an den Klimawandel. Bonn: Umweltbundesamt.
van Mantgem, P. J., Stephenson, N. L., Byrne, J. C., Daniels, L. D., Franklin, J. F., Fule, P. Z., et al. (2009). Widespread increase of tree mortality rates in the western United States. Science 323, 521–524. doi: 10.1126/science.1165000
Wellbrock, N., Eickenscheidt, N., Hilbrig, L., Dühnelt, P.-E., Holzhausen, M., Bauer, A., et al. (2018). Leitfaden und Dokumentation zur Waldzustandserhebung in Deutschland. Thünen Working Paper. Braunschweig: Johann Heinrich von Thünen-Institut, 97.
Wessel, M., Brandmeier, M., and Tiede, D. (2018). Evaluation of different machine learning algorithms for scalable classification of tree types and tree species based on sentinel-2 data. Remote Sens. Basel. 10:1419. doi: 10.3390/rs10091419
Zacharias, S., Bogena, H., Samaniego, L., Mauder, M., Fuss, R., Putz, T., et al. (2011). A network of terrestrial environmental observatories in Germany. Vadose Zone J. 10, 955–973. doi: 10.2136/Vzj2010.0139
Zhan, Z. Y., Yu, L. F., Li, Z., Ren, L. L., Gao, B. T., Wang, L. X., et al. (2020). Combining GF-2 and sentinel-2 images to detect tree mortality caused by red turpentine beetle during the early outbreak stage in North China. Forests 11:172. doi: 10.3390/f11020172
Keywords: Norway spruce, tree crown transparency, Sentinel-2, Eifel, Google Earth Engine
Citation: Montzka C, Bayat B, Tewes A, Mengen D and Vereecken H (2021) Sentinel-2 Analysis of Spruce Crown Transparency Levels and Their Environmental Drivers After Summer Drought in the Northern Eifel (Germany). Front. For. Glob. Change 4:667151. doi: 10.3389/ffgc.2021.667151
Received: 12 February 2021; Accepted: 11 June 2021;
Published: 22 July 2021.
Edited by:
Francesco Ripullone, University of Basilicata, ItalyReviewed by:
Martina Pollastrini, University of Florence, ItalyAhmed Kenawy, Mansoura University, Egypt
Copyright © 2021 Montzka, Bayat, Tewes, Mengen and Vereecken. This is an open-access article distributed under the terms of the Creative Commons Attribution License (CC BY). The use, distribution or reproduction in other forums is permitted, provided the original author(s) and the copyright owner(s) are credited and that the original publication in this journal is cited, in accordance with accepted academic practice. No use, distribution or reproduction is permitted which does not comply with these terms.
*Correspondence: Carsten Montzka, Yy5tb250emthQGZ6LWp1ZWxpY2guZGU=
†ORCID: Carsten Montzka, orcid.org/0000-0003-0812-8570; Bagher Bayat, orcid.org/0000-0002-7761-9544; Andreas Tewes, orcid.org/0000-0002-8899-0285; David Mengen, orcid.org/0000-0003-4041-6599; Harry Vereecken, orcid.org/0000-0002-8051-8517