- 1Department of Meteorology and Climate Science, San José State University, San Jose, CA, United States
- 2Washington State Department of Ecology, Olympia, WA, United States
- 3Department of Atmospheric Sciences, University of Utah, Salt Lake City, UT, United States
- 4Department of Mathematical and Statistical Sciences, University of Colorado, Denver, Denver, CO, United States
- 5Department of Civil and Environmental Engineering, Washington State University, Pullman, WA, United States
The objective of this study was to assess feasibility of integrating a coupled fire-atmosphere model within an air-quality forecast system to create a multiscale air-quality modeling framework designed to simulate wildfire smoke. For this study, a coupled fire-atmosphere model, WRF-SFIRE, was integrated, one-way, with the AIRPACT air-quality modeling system. WRF-SFIRE resolved local meteorology, fire growth, the fire plume rise, and smoke dispersion, and provided AIRPACT with fire inputs. The WRF-SFIRE-forecasted fire area and the explicitly resolved vertical smoke distribution replaced the parameterized BlueSky fire inputs used by AIRPACT. The WRF-SFIRE/AIRPACT integrated framework was successfully tested for two separate wildfire events (2015 Cougar Creek and 2016 Pioneer fires). The execution time for the WRF-SFIRE simulations was <3 h for a 48 h-long forecast, suggesting that integrating coupled fire-atmosphere simulations within the daily AIRPACT cycle is feasible. While the WRF-SFIRE forecasts realistically captured fire growth 2 days in advance, the largest improvements in the air quality simulations were associated with the wildfire plume rise. WRF-SFIRE-estimated plume tops were within 300-m of satellite-estimated plume top heights for both case studies analyzed in this study. Air quality simulations produced by AIRPACT with and without WRF-SFIRE inputs were evaluated with nearby PM2.5 measurement sites to assess the performance of our multiscale smoke modeling framework. The largest improvements when coupling WRF-SFIRE with AIRPACT were observed for the Cougar Creek Fire where model errors were reduced by ∼50%. For the second case (Pioneer fire), the most notable change with WRF-SFIRE coupling was that the probability of detection increased from 16 to 52%.
Introduction
As the number and size of wildland fires increase (Westerling et al., 2006; Dennison et al., 2014), the potential for wildfire smoke to impact air quality (AQ) is a growing concern. Despite the reduction of industrial and vehicular emissions, AQ across the western US has been deteriorating, especially across the Pacific Northwest and the Intermountain West (McClure and Jaffe, 2018). Recent wildfire events are likely responsible for degrading AQ across the west, therefore exposing more Americans to unhealthy levels of particulate matter (PM2.5) and ozone (Jaffe et al., 2004; Jaffe and Wigder, 2012; Bytnerowicz et al., 2016; Mass and Ovens, 2019; Wilmot et al., 2021). With wildfire activity projected to increase in the coming decades due to climate change (Spracklen et al., 2009; Liu et al., 2016), forecasting AQ impacts from wildfires will be increasingly important. The ability for models to accurately forecast pollutants from wildfires is critical when issuing public warnings that enable AQ-sensitive individuals to limit their smoke exposure.
Over the past decades, researchers have sought to build and refine AQ modeling systems that can accurately forecast the impact of fires on AQ. For an overview of modeling approaches, readers are encouraged to read Jaffe et al. (2020), who outlined both the statistical and the dynamical approaches used for smoke modeling. Several AQ forecasting systems have been developed to assess the impacts of fire emissions on air pollution. Examples include the National Air Quality Forecast Capability (NAQFC), which runs on a 12-km grid, providing forecasts for the CONUS domain (Tang et al., 2015); the BlueSky system (Larkin et al., 2009) operated by the US Forest Service; and the AIRPACT system, operated by the Laboratory for Atmospheric Research at Washington State University (LAR at WSU) with support from the NW-AIRQUEST consortium of clean air agencies. These modeling systems estimate fire emissions using satellite hot spot detections and estimates of acres burned and fuel loading. Furthermore, the chemical transport model CMAQ (Byun and Schere, 2006) is driven by meteorological forecasts that are not coupled to the chemical transformations and smoke emissions. A different approach is used in the RAP/HRRR-smoke system (Ahmadov et al., 2017), which estimates PM2.5 emissions using the latest fire radiative power from satellite hot-spot detects. Smoke and meteorology within RAP/HRRR-smoke are simulated in-line within the dynamical core of the Weather Research and Forecast model (WRF; Skamarock et al., 2008) for a domain that covers the continental United States. To reduce computational costs, HRRR-SMOKE treats smoke as a passive tracer and ignores chemical transformations.
Regardless of the technical differences surrounding the modeling frameworks described above, there are two large sources of uncertainty that smoke forecasting systems need to address: (1) how to compute hourly fire emissions in a forecast mode and (2) how to distribute those emissions vertically (Val Martin et al., 2012; Herron-Thorpe et al., 2014; Walter et al., 2016; Mallia et al., 2018). Within the modeling systems described above, fire emission estimates are based on the most recent fire detections and are held constant into the future with a repeating diurnal pattern. While this approach is widely accepted by the modeling community, it has limitations for cases where fires exhibit significant day-to-day variations in the burned area or when the fire emissions do not conform to a typical diel pattern. Recent work by Graff et al. (2020) highlighted the limitations of assuming unchanged diurnal patterns and showed that regression models accounting for weather-driven changes in fire activity often perform better. For plume rises, most AQ models parameterize the smoldering fraction and calculate plume-top height using methods developed for point-source modeling (e.g., industrial facilities). Due to the reasons described above, most AQ forecast systems cannot account for the weather-driven fire behavior effects on plume dynamics.
An alternative smoke modeling approach was developed by Mandel et al. (2014), which simultaneously resolves the time evolution of fire emissions and fire-atmosphere interactions driving the plume evolution. This approach utilizes the Weather Research and Forecast model (WRF; Skamarock et al., 2008) to simulate meteorology, while a fire spread parameterization combined with a fuel moisture model (SFIRE) is dynamically coupled with local meteorology (WRF-SFIRE; Mandel et al., 2011). WRF-SFIRE has been successfully applied for selected wildfire events (Kochanski et al., 2016, 2019; Mallia et al., 2020b) where simulated plume heights compared favorably with plume heights derived from the multi-angle imaging spectroradiometer (MISR). In another study, WRF-SFIRE was used to forecast smoke from a large wildfire near Salt Lake City, UT during the fall of 2018 (Mallia et al., 2020a). Smoke simulations in this study were evaluated with a high-density AQ network with over 300 sensors. Mallia et al. (2020a) found that WRF-SFIRE was able to resolve inter-basin air exchanges and larger-scale canyon flows, while also capturing the smoke plume orientation and horizontal extent, along with the duration and timing of the smoke episode.
Despite the encouraging results described above, these studies also highlighted some of the limitations surrounding coupled fire-atmosphere models. One fundamental problem with coupled fire-atmosphere models is the computational resources needed to run full chemistry resolved at sub-kilometer grid spacing, absent of exceptional computing resources. The large computational costs associated with coupled fire-atmosphere models with full chemistry can make it difficult to complete an operational forecast within a reasonable amount of time. Another potential problem associated with existing coupled fire-atmosphere models is related to the simplified representation of heat fluxes and smoke emissions. For example, WRF-SFIRE heat fluxes and smoke emissions are computed based on the fuel consumed using approximations from Clark et al. (2004) of the standard fire behavior models and multiplicative emission factors (Anderson, 1982; Wiedinmyer et al., 2011).
To overcome some of the limitations described above, we developed a proof-of-concept multiscale smoke modeling framework that resolves fire-scale processes, plume rise dynamics, and regional-scale atmospheric chemistry. This framework also had to be fast enough to be deployed for forecasting applications. This smoke modeling framework utilized an optimized high-resolution coupled fire-atmosphere model (WRF-SFIRE) as a sub-grid-scale model linked to CMAQ via the Sparse Matrix Operator Kernel for Emissions (SMOKE)1 chemical preprocessor and the model platform used for AIRPACT.
The paper is organized as follows. We start from the description of the modeling components of the coupled fire-atmosphere model (WRF-SFIRE) and the AIRPACT AQ system (section “Materials and Methods”). Next, we discuss the coupling strategy and the model integration. For section “Results,” model results are evaluated with observations for two separate wildfire smoke episodes. These evaluations are used to highlight the differences and potential benefits of integrating a fully coupled fire-atmosphere model into a regional AQ model. Lastly, section “Discussion” concludes with a discussion of the results and recommendations for future work.
Materials and Methods
WRF-SFIRE was one-way coupled with the AIRPACT modeling system to assess the feasibility of a multiscale AQ forecasting system. This system was used to simulate smoke for two separate wildfire events. Two sets of simulations were performed during this study. The first set of simulations were from the operational version of AIRPACT with USFS BlueSky fire inputs, while the second set of simulations used the WRF-SFIRE model for fire inputs. WRF-SFIRE was used to produce vertically distributed smoke emissions, while dispersion within AIRPACT was driven by external 4-km WRF simulations from University of Washington available at https://a.atmos.washington.edu/wrfrt/. The primary objective of this study was to:
(1) Investigate the feasibility of using WRF-SFIRE output as a pre-processor for the AIRPACT modeling system.
(2) Determine how well the hybrid system resolved the plume rise and fire growth.
(3) Compare the hybrid system performance with the standard AIRPACT forecast.
WRF-SFIRE Description
WRF-SFIRE is a coupled fire-atmosphere model (Mandel et al., 2011, 2014, 2019), which simultaneously resolves atmospheric dynamics, fire progression, fuel moisture conditions, and fire-atmosphere interactions. This system integrates the WRF model with a fire spread model (SFIRE), where the fire-rate-of-spread is estimated based on the Rothermel model (Rothermel, 1972). Fire growth and fuel consumption computed in the fire model are used to estimate heat fluxes and smoke emissions, which are then fed back into the atmospheric model (Clark et al., 2004). As a result, WRF-SFIRE can explicitly resolve the wildfire plume dynamics when using a high-resolution (<1-km) atmospheric grid (Kochanski et al., 2019). WRF-SFIRE is coupled with a predictive fuel moisture model (Vejmelka et al., 2016) to account for diurnal variability in the fire behavior driven by fuel moisture fluctuations (Mandel et al., 2014). WRF-SFIRE operates on two separate meshes. The highest resolution grid is used to trace fire progression, compute emissions, and account for the impact of local topography on fire progression. The coarser atmospheric mesh is used to simulate local meteorology, the evolution of the 1, 10, and 100 h dead fuel moisture, plume rise, and smoke dispersion. For this study, we used WRF-SFIRE based on WRF version 3.4.1, available at https://github.com/openwfm/WRF-SFIRE/tree/wrf-fire-track/master.
WRF-SFIRE Numerical Setup for the Cougar Creek Fire
The WRF-SFIRE simulations for the 2015 Cougar Creek Fire in southern Washington consisted of four nested domains at 12-, 4-, 1. 33-, and 0.444-km grid spacing (Figure 1A), which progressively downscaled meteorological fields provided by the North American Regional Reanalysis (NARR; Mesinger et al., 2006). A 22-m fire mesh was embedded within the innermost WRF domain (d04) to simulate fire progression. Fuel and elevation data from LANDFIRE at 30-m grid spacing was interpolated to WRF-SFIRE’s fire mesh grid. More details behind the WRF-SFIRE simulations such as domain size and parameterizations used can be seen in Table 1, while the spatial extent of the domains is shown in Figure 1A. The first Cougar Creek Fire simulation was initialized on August 11th, 2015 at 0000 UTC and simulated through the next 5 days until August 16th 0000 UTC. This initial run served to represent a case where a 120 h-long forecast was generated, without fire reinitialization. The first simulation was started from a point ignition at –121.374°W and 46.134°N, with a radius of 400 m. This fire was ignited on the 11th of August.
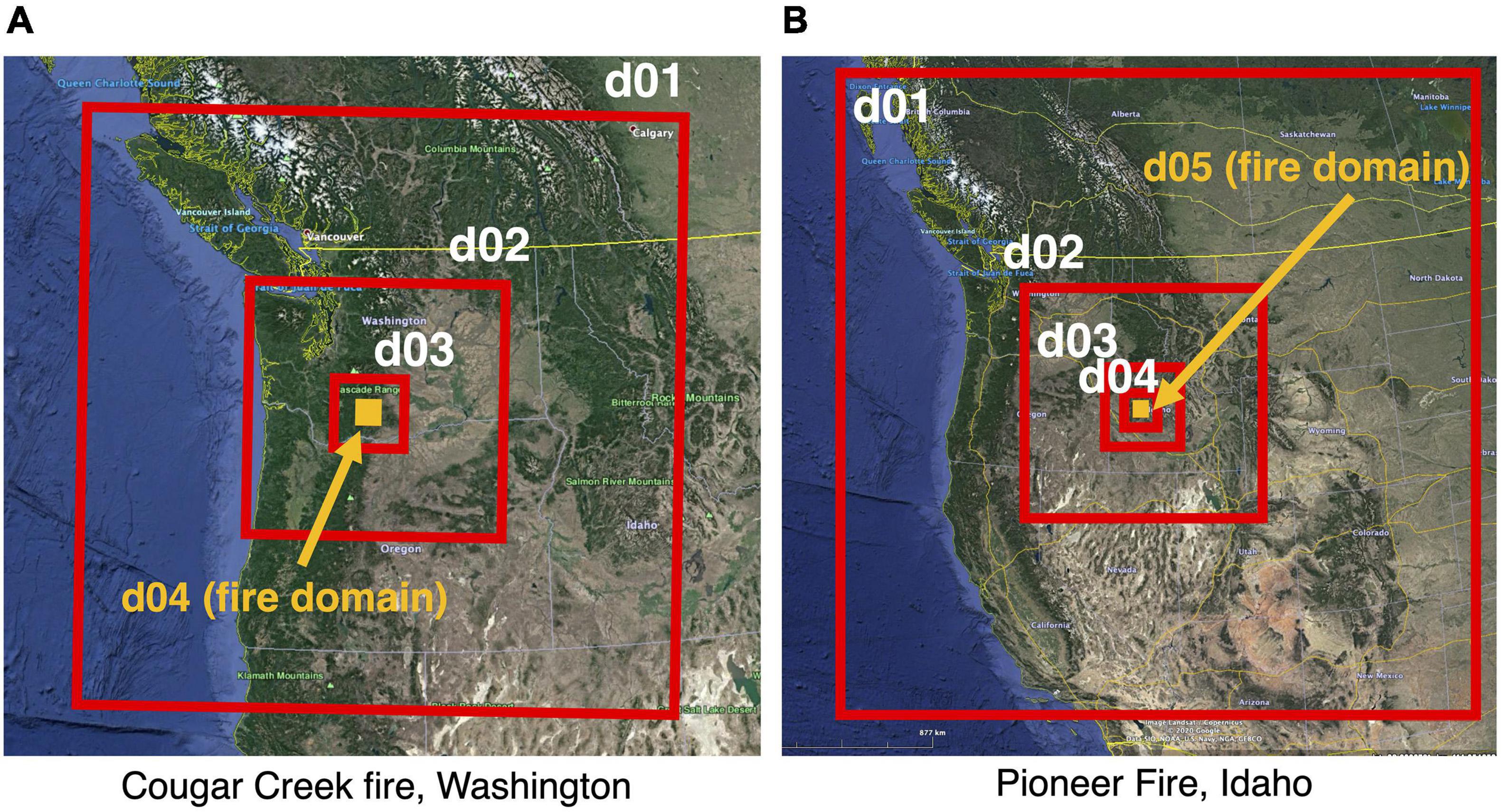
Figure 1. Domain configuration for the (A) Cougar Creek Fire, (B) Pioneer Fire. Orange box indicated the innermost domain used for fire spread computations.
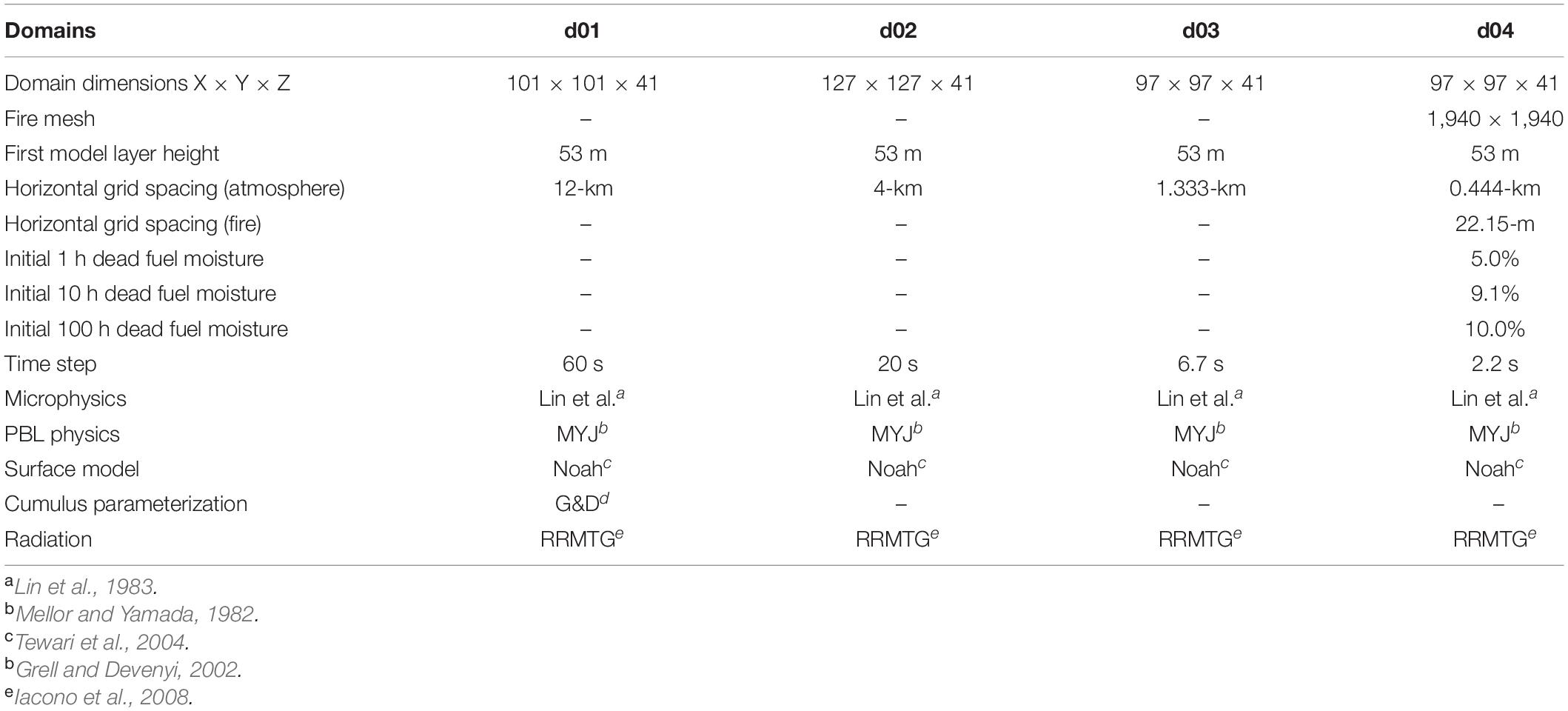
Table 1. Detailed Weather Research and Forecast model (WRF) configurations for the WRF-SFIRE simulations of the Cougar Creek Fire.
WRF-SFIRE Numerical Setup for the Pioneer Fire
The 2016 Pioneer Fire in central Idaho was simulated using a 5-domain setup with the domain grid spacing gradually decreasing from 27 to 0.333 km. Analyses from the Climate Forecast System Reanalysis (CFSR) was used to initialize meteorology within WRF-SFIRE, while fuel and topographic information was provided by LANDIFIRE. WRF-SFIRE simulations covered the period of August 14–31st 2016, with reinitializations occurring every 48 h. More details behind the WRF-SFIRE simulations such as domain size and parameterizations used can be seen in Table 2, while the spatial extent of the domains is shown in Figure 1B.
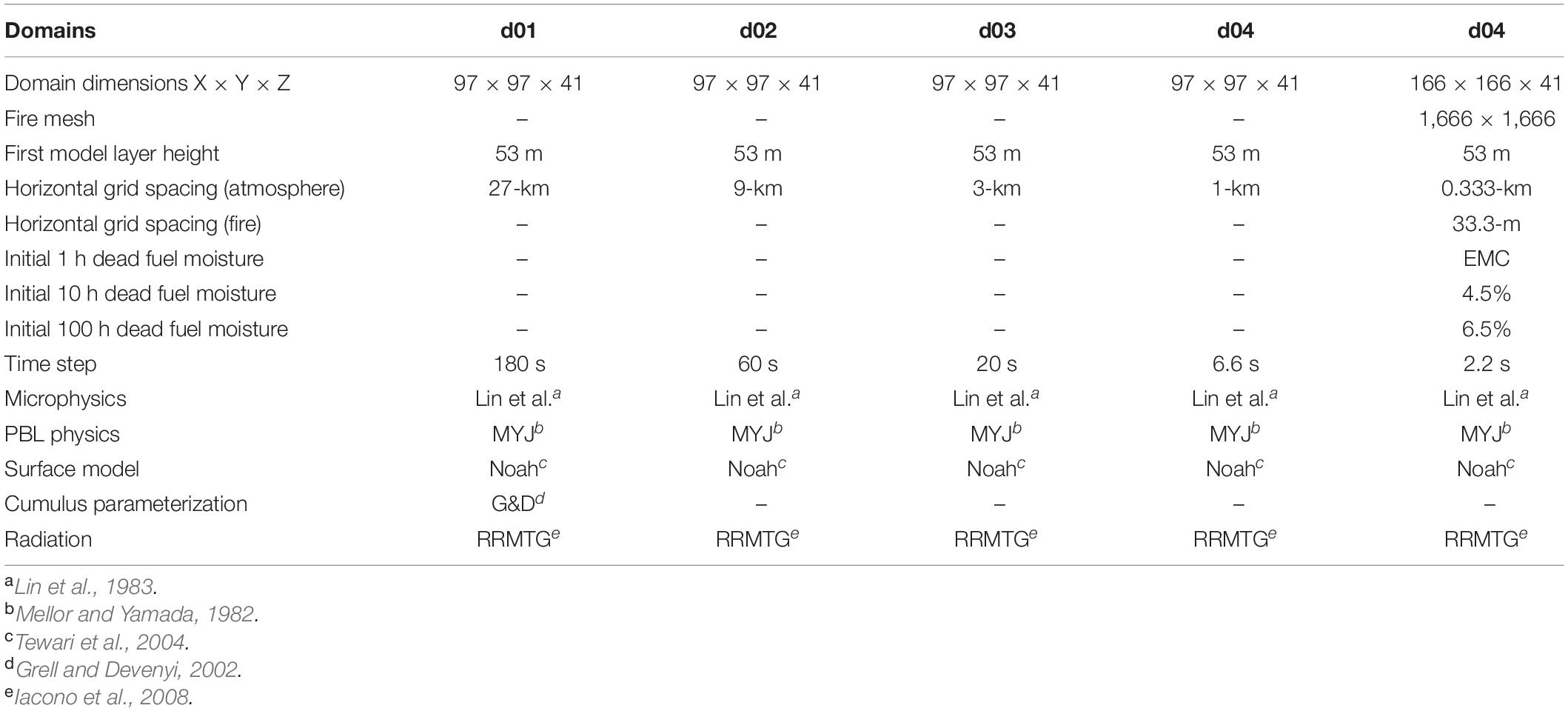
Table 2. Detailed Weather Research and Forecast model (WRF) configurations for the WRF-SFIRE simulations of the Pioneer Fire.
Since the Pioneer Fire ignition time was almost a month before the intended simulation start date, the model was initialized using infrared fire perimeters provided by GeoMac.2 Each re-initialization carried over the simulated fuel moisture from the previous simulation. For each WRF-SFIRE simulation, WRF-SFIRE was re-initialized with GeoMac infrared fire perimeters to (1) locate active fire perimeters and to (2) mask out previously burned fuels. Infrared fire perimeters were then used to generate a fire arrival matrix by linearly interpolating between two consecutive perimeters bracketing the ignition time, like the method described in Kochanski et al. (2019). Fuel within the first perimeter was removed with an extra margin of 60 m to prevent fire ignition along inactive sections of the fire perimeter. The fire arrival time was then used to prescribe the fire growth within WRF-SFIRE for the first 2 h of each forecast. This spin-up time was used to ensure that the fire-induced circulation was established before the start of each run, which was necessary to limit model instability. Once the simulation reached t = 2 h, fire growth within WRF-SFIRE was estimated by the predictive fire model driven by modeled winds, terrain slope, fuel moisture, and fuel types.
AIRPACT Description
Operational AIRPACT provides daily 48-h forecasts for the Pacific Northwest, using a suite of models (WRF/SMOKE/CMAQ). AIRPACT’s 48-hr forecast uses initial conditions for chemical and aerosol species from the previous AIRPACT run, along with vertical boundary conditions derived from MOPITT CO-Assimilated MOZART-4 forecast extractions (Emmons et al., 2010). AIRPACT uses Global Forecast System-initialized WRF runs from the University of Washington.3 SMOKE is the Sparse Matrix Operator Kernel for Emissions, an emissions preprocessor for CMAQ. CMAQ is the Community Multi-scale Model for Air Quality (Byun and Schere, 2006).
Smoke from wildland fire is predicted using BlueSky (Larkin et al., 2009), which has detailed fuel loading estimates from Fuel Characteristic Classification System (FCCS) but does not consider dynamic fire behavior. BlueSky forecasts use recent satellite-derived fire locations to compute daily smoke emissions and heat fluxes. For forecasting purposes, once fires are detected by the model, they are assumed to persist unchanged from day to day, while fire emissions are assumed to follow an average diel profile where emissions increase during the day and decrease overnight. SMOKE uses heat fluxes estimated by BlueSky to calculate vertical smoke plume distributions. AIRPACT forecasts used daily fire emissions derived from BlueSky 3.5.1 to model wildfire smoke over the past decade. BlueSky emissions were parameterized using a fuel-specific sensitivity analysis, which greatly reduced computational time for fire location emissions, especially for simulations with many fire locations. AIRPACT originally relied on SMARTFIREv2 (Raffuse et al., 2009) to provide the fire size and location. However, in 2020, AIRPACT was updated with the Fire Information System with BlueSky Pipeline. The evolution of the operational AIRPACT forecast methodology is documented in the following papers (Vaughan et al., 2004; Chen et al., 2008; Herron-Thorpe et al., 2014; Ravi et al., 2018, 2019) and at http://www.lar.wsu.edu/airpact.
The Pouliot-Godowitch plume rise algorithm (Pouliot et al., 2005) in SMOKE converts the fire heat flux to a buoyancy flux using a plume rise calculation based on the Briggs equation (Briggs, 1982). Plume rise calculations in AIRPACT were modified in 2018 to use a method developed by the Idaho Department of Environmental Quality, which improved fire plume characterization relative to the default method used within SMOKE. SMOKE determines plume top height and plume bottom height from the heat flux input while also separating the smoldering fraction from the area burned input. Despite these improvements, the Pouliot algorithm can sometimes produce unrealistic results. Therefore, the Idaho DEQ method using virtual area and virtual heat values instead provides inputs for SMOKE. The virtual area is based on the flaming consumption from BlueSky while virtual heat is based on a method from Air Sciences’ Deterministic & Empirical Assessment of Smoke’s Contribution to Ozone project (Mavko and Morris, 2013), which accounts for multiple flame fronts as are typically associated with large fires.
CMAQ results are written out on an hourly basis while post-processing produces derived results such as PM2.5, which accounts for contribution from CMAQ-resolved aerosols, and time averaged variations of PM2.5.
Offline Coupling
The WRF-SFIRE with AIRPACT simulations were generated in 24-h segments, rather than the typical 48-h simulations used for AIRPACT operational forecasting. The methodology used for offline coupling between WRF-SFIRE to AIRPACT went through three iterations as described below, with varying reliance on WRF-SFIRE and the SMOKE preprocessor for calculating the wildfire plume rise. In each test case the fire size and location from WRF-SFIRE were fed to the SMOKE processor (see the gray line in Figure 2). The emissions were then parameterized within the AIRPACT emission processor. The key difference between the methodologies presented below was how the fire heat flux and resolved plume rise were ingested by AIRPACT.
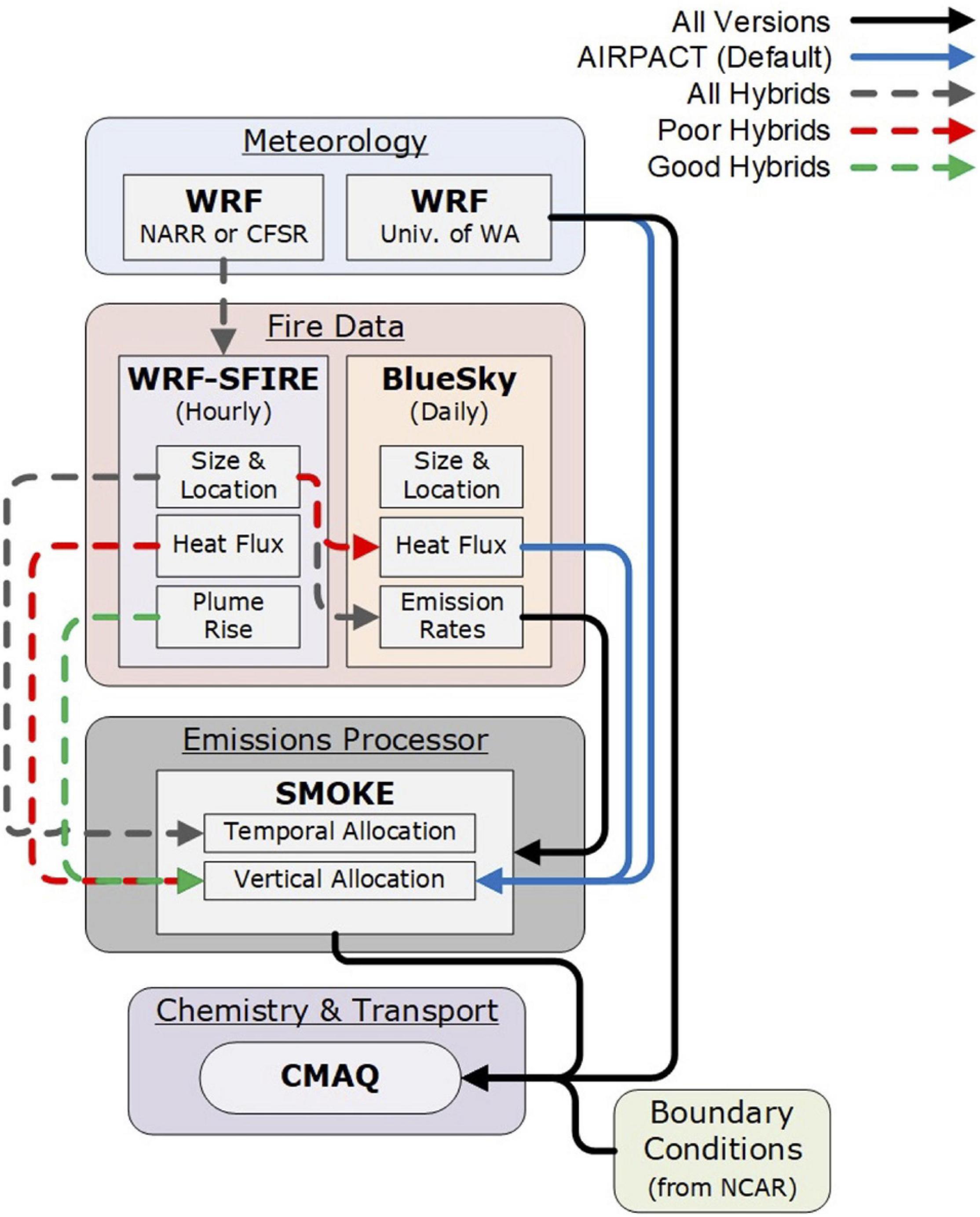
Figure 2. Data flow and coupling between the fire model WRF-SFIRE and AIRPACT air quality (AQ) system.
(1) Total fire size from WRF-SFIRE used to compute emissions based on BlueSky method.
This method used the total hourly fire size from the entire WRF-SFIRE domain as input to BlueSky as a single location. The total emissions resulting from each input were inserted into SMOKE using an hour-specific source classification code (SCC), which bypassed the diurnal profile typically used in wildfire temporal processing. This method was tested using heat fluxes from both WRF-SFIRE and BlueSky as input to SMOKE using the Pouliot-Godowitch plume rise algorithm. This method showed no improvement over the default AIRPACT forecast (results not shown).
(2) Discrete fire locations from WRF-SFIRE used to compute emissions based on BlueSky method.
This method used hourly fire size from each WRF-SFIRE grid-cell (444 m spacing) as input to BlueSky. The total emissions resulting from each input were inserted into SMOKE using an hour-specific SCC, which bypassed the diurnal profile typically used in wildfire temporal processing. This method was tested using heat fluxes from both WRF-SFIRE and BlueSky as inputs to SMOKE using the Pouliot-Godowitch plume rise algorithm. This method resulted in many distinct point locations with low heat fluxes, and subsequently poor performance for smoke transport. Therefore, this method was modified further. In the second iteration, the total hourly heat flux from WRF-SFIRE across all fire locations was integrated and included as input for SMOKE and the Pouliot-Godowitch plume rise algorithm. This method greatly increased the fire heat flux and resulted in a single aggregated smoke plume. Performance for this method was improved but large differences in smoke transport still existed between the WRF-SFIRE and original AIRPACT results. Both variants of this methods are labeled as “Poor Hybrids” in Figure 2.
(3) Discrete fire locations from WRF-SFIRE used to compute emissions based on BlueSky method, with plume height information taken directly from WRF-SFIRE.
This method used hourly fire area from each WRF-SFIRE grid-cell (444 m spacing) as input to BlueSky. The hourly emissions for each location were inserted into SMOKE using a legacy input format that specifies hourly plume top, plume bottom, and smoldering fraction from WRF-SFIRE. This method does not use the Pouliot-Godowitch plume rise algorithm. The plume heights downwind from the active fire front were averaged, which ignored those plumes directly above fire pixels where the smoke was emitted to. This method provided the best results amongst all the attempted methods. This coupling method is indicated as “Good Hybrid” in Figure 2. All hybrid simulations presented in this paper were performed using this coupling method.
Results
The wildfire events chosen for our analysis were based on the following criteria. First, we sought relatively recent wildfire events that occurred within the AIRPACT domain. Secondly, well-defined smoke plumes that remained distinct from other plumes were preferred. The selected case studies also needed to have a reasonable coverage of AQ monitoring stations. Lastly, fires that were responsible for generating smoke plumes needed to be well-documented with infrared fire perimeters to initialize the WRF-SFIRE simulations and to evaluate the modeled fire progression. The two wildfires that met our criteria were the 2015 Cougar Creek and 2016 Pioneer fires, which burned in southern Washington, and central Idaho, respectively.
Coupled Fire-Atmosphere Simulations
Cougar Creek Fire, 2015
WRF-SFIRE meteorological simulations were evaluated at several surface stations located throughout southern Washington State. Despite the complex topography across the domain of interest, WRF-SFIRE model results for temperature (T), wind speed (WS), and relative humidity (RH) were in relatively good agreement with nearby weather stations (Table 3). Biases for all three variables were relatively small (T = +0.8°C, WS = +0.5 m s–1, RH = –4.6%) while there were slightly larger Root Mean Square Error (RMSE) for T and RH (T = +3.2°C, RH = +17.1%). The overall burned area during the simulation period was in line with estimates from the infrared perimeters. The final fire area simulated by the model was within 5% of the observations. The fire size at the end of the first day was also well-forecasted (Figure 3). However, between August 12th and 15th, the simulated fire area was underestimated. The fire after the first day tended to diverge from the observations due to underestimated growth rates relative to observations. After August 14th, the observed fire growth deaccelerated, and the simulated burned area started to converge with the observed fire area. It should be noted that this simulation was started from an ignition point, and the fire propagation advanced during the whole simulation period without any fire model reinitialization. There are many possible explanations for the observed discrepancies in fire area. First, the model slightly overestimated near-surface winds (mean bias = 0.5 m s–1) and underestimated the RH (4.6%; see Table 3), which together could have resulted in an overpredicted rate of fire spread. In addition, it is suspected that overestimated initial values of the dead fuel moisture could have also resulted in underpredicted fire growth near the start of simulation, when the fuel moisture model was equilibrating with the atmospheric model.
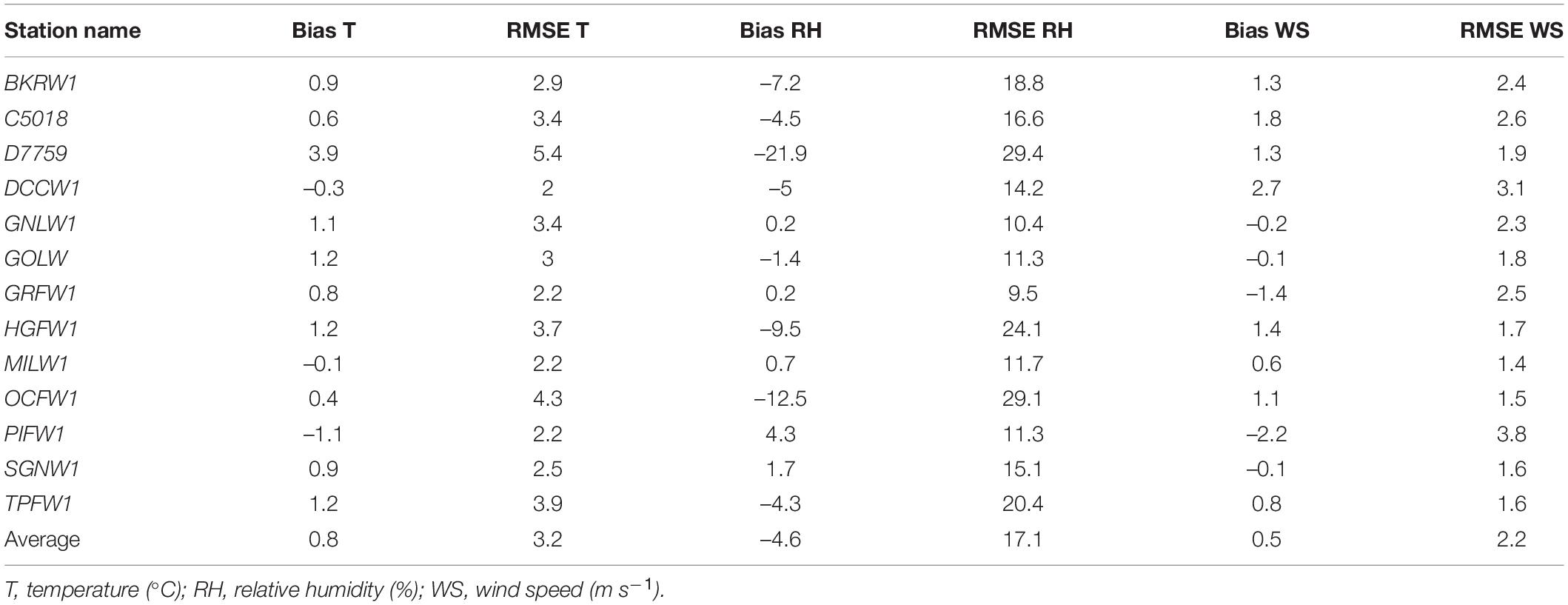
Table 3. WRF-SFIRE meteorological error statistics for weather stations across southern Washington during the 2015 Cougar Creek Fire.
To assess WRF-SFIRE’s ability to resolve the vertical plume extent, the simulated smoke plume tops were compared to MISR plume top retrievals during the early afternoon (18–1900 UTC) of August 18th, 2015. Overall, the plume rise simulation was largely in agreement with satellite observations (Figures 4A,B). MISR detections indicated that the plume tops averaged for the Cougar Creek Fire had an average height of 1990 mASL. Within the WRF-SFIRE forecast model simulations, plume tops had slightly higher average plume height of 2,214 mASL. However, some regions corresponding to the highest plume tops located near Mount Adams were missing in the MISR plume detections. These locations near Mount Adams corresponded to the bright glaciated areas near the peak of the mountain, which resulted in the donut-like hole around this mountain’s summit (Figure 4). This could have skewed the MISR statistics toward lower plume top heights by omitting some of the tallest sections of the plume. AIRPACT-CMAQ estimated plume top heights were around 2,700-m, which was ∼700-m greater than MISR-estimated plume top heights and 500-m higher than WRF-SFIRE plume top heights (not shown).
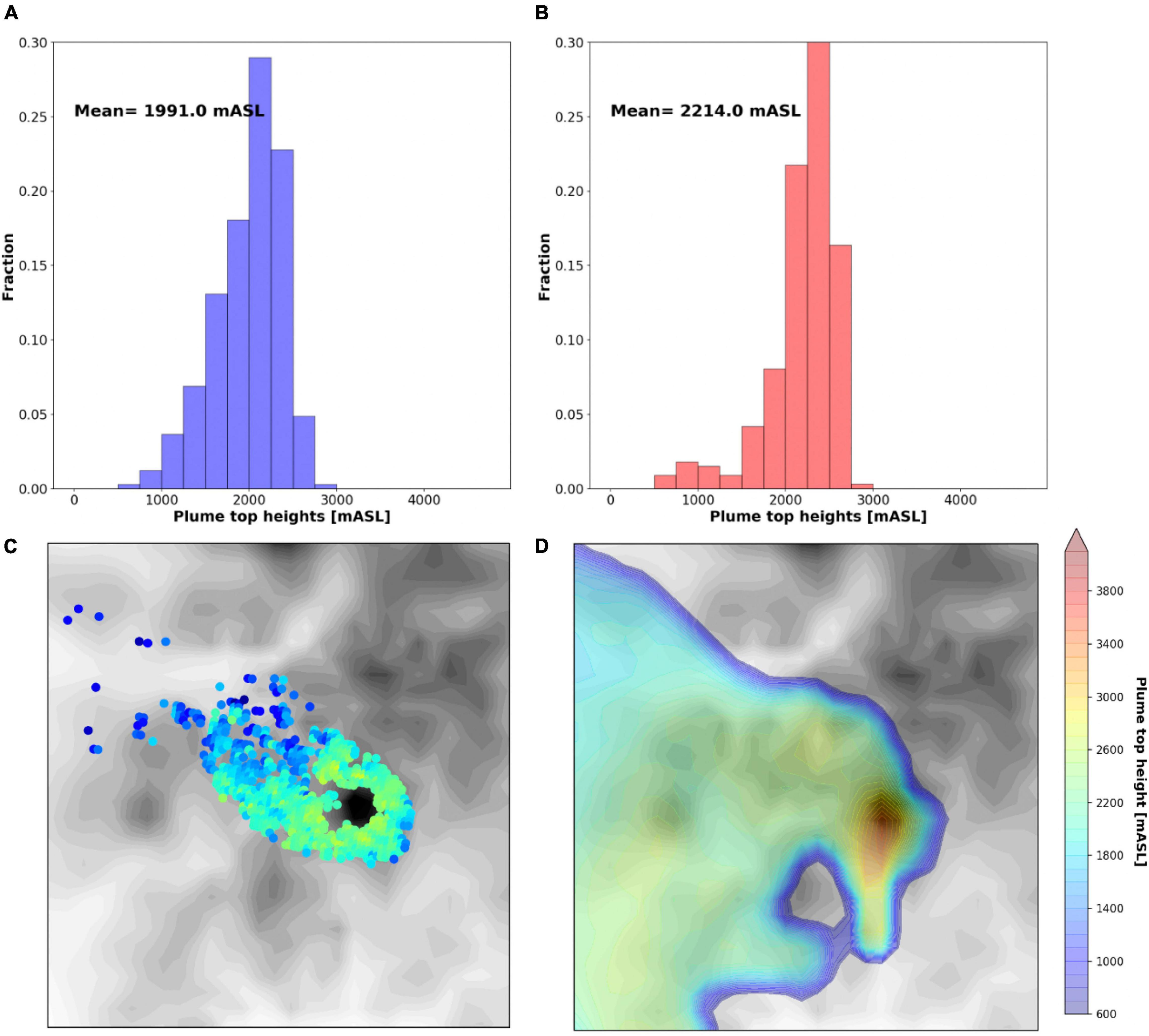
Figure 4. Multi-angle imaging spectroradiometer (MISR)-derived (A,C) vs. modeled (B,D) plume top heights for the Cougar Creek Fire on August 18th, 2015 at 19:14 UTC. Panels (A,B) show histograms of plume top heights near the center of the fire plume while panels (C,D) show spatial distribution of the same plume top heights. The gray shaded contours represent terrain heights where black corresponds to higher terrain heights while the lighter gray shading represents lower terrain heights.
Pioneer Fire, 2016
The simulated weather conditions during the Pioneer Fire were evaluated with observations from weather stations located within the innermost WRF domain. The summary of the meteorological statistics for the WRF-SFIRE simulation is presented in Table 4. Since the Pioneer Fire burned in a relatively remote location in Idaho, weather station coverage was limited. Overall, WS errors throughout the simulation were minimal, with an average bias and RMSE that were <1.3 m s–1. RH, which can impact fuel moisture, and subsequently, fire growth, had an RMSE and bias of +14.9 and 12.7%, respectively. Larger differences existed between the modeled and observed 2-m temperature, particularly at the LLFI1 site, where temperature was consistently underpredicted by 8°C. Given that this station was located on the wall of a very narrow mountain valley, we hypothesize that mismatches in the modeled vs. actual station height could have resulted in the difference seen in Table 4. The temperature discrepancy observed at the LLFI1 site appeared to be an outlier relative to RMSE and biases reported at the two other stations, so it is not unreasonable to assume that there was some sort of instrument malfunction at this site.

Table 4. WRF-SFIRE meteorological error statistics for weather stations across Idaho during the 2016 Pioneer Fire.
As discussed in section “Offline Coupling,” the Pioneer Fire in Idaho was simulated using WRF-SFIRE between August 14th and 30th, 2016. This coincided with periods of significant fire growth (Figure 5), including the August 28–31st event which saw the fire growth from 110,000 to over 160,000 acres in the span of 2 days. Overall, the WRF-SFIRE simulated fire areas were in agreement with observed fire perimeters from daily infrared measurements. On average, daily growth rates were within 30% of the observed rates. As shown in Figure 5 the simulated fire growth tended to slowly diverge from the observations. Beyond 36 h, WRF-SFIRE began to overestimate the total burned area, however, the simulations were still in agreement with infrared fire perimeters on August 28–30th, when the Pioneer Fire was undergoing significant growth. To reduce the accumulation of the error in the simulated fire progression, the Pioneer Fire simulations were reinitialized every 2 days using observed infrared perimeters. The strategy employed here provided the model with better and more frequent initializations, which reduced simulated fire growth rate errors.
Plume top heights from WRF-SFIRE on August 24th, 2016 were in agreement with MISR-estimated plume top heights with the model underestimating plume tops by ∼320 m (Figure 6). Overall, these results were expected given that the modeled fire progression matched observed fire growth rates. It should be noted that the MISR-detected plume extent was smaller than the plume extent seen in the WRF-SFIRE runs (Figure 6). We suspect that MISR was unable to capture parts of the smoke plume that were especially diffuse relative to the plume centerline. From these results, it appears that WRF-SFIRE can skillfully capture the wildfire plume rise when fire growth rates are accurately forecasted.
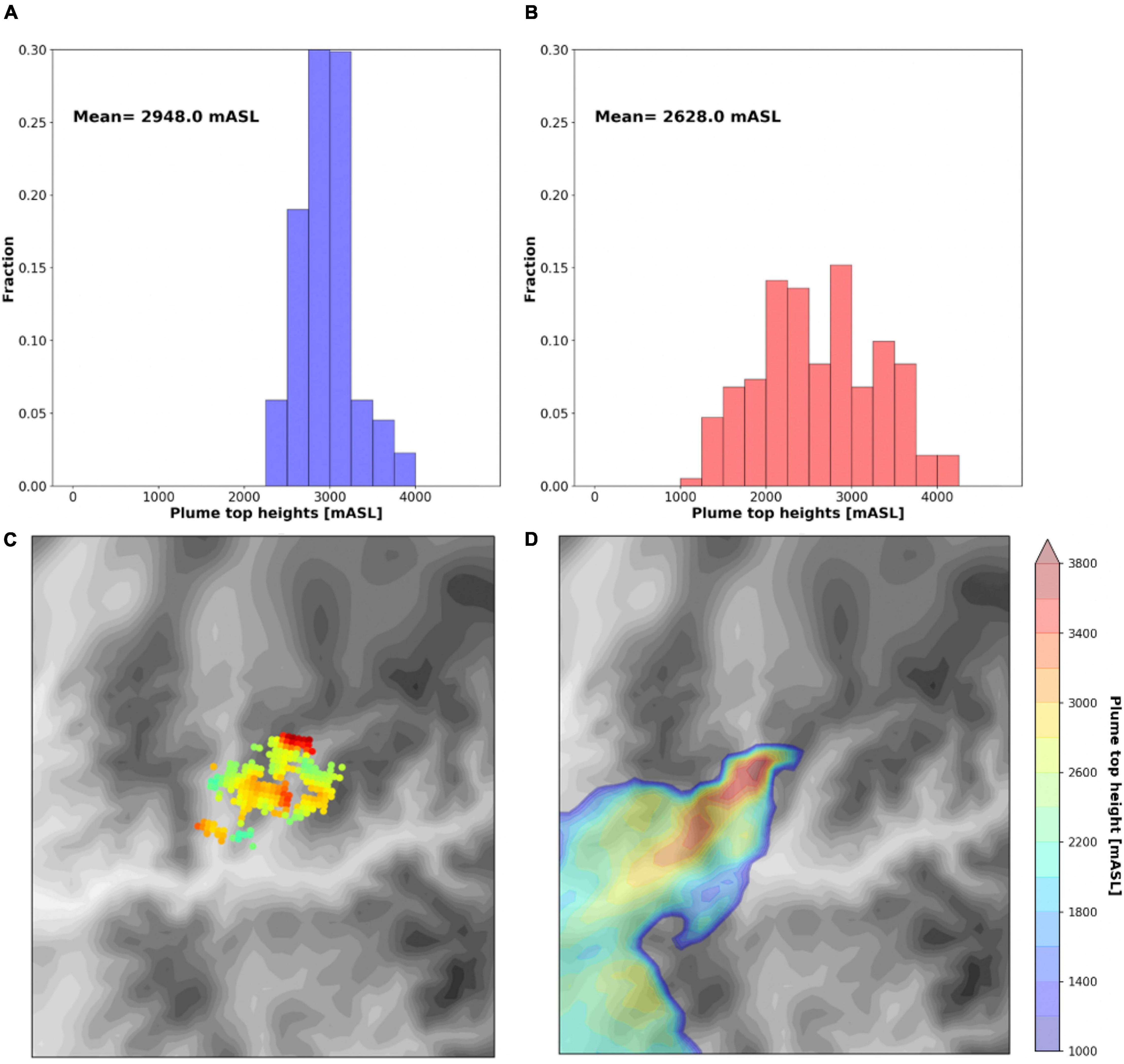
Figure 6. MISR-derived (A,C) vs. modeled (B,D) plume top heights for the Pioneer fire on August 24, 2016 at 18:50 UTC. (A,B) Histograms of plume top heights near the center of the fire plume. (C,D) Spatial maps of the plume top heights. The gray shaded contours represent terrain heights where black corresponds to higher terrain heights, while the lighter gray shading represents lower terrain heights.
Air Quality Simulations Using AIRPACT
Cougar Creek Fire, 2015
CMAQ simulation results for PM2.5 for the 2015 Cougar Creek Fire, both original AIRPACT simulations and WRF-SFIRE-informed simulations, are presented in Tables 5, 6. These simulations were evaluated with AQ sites from the AirNow network (Figure 7A).
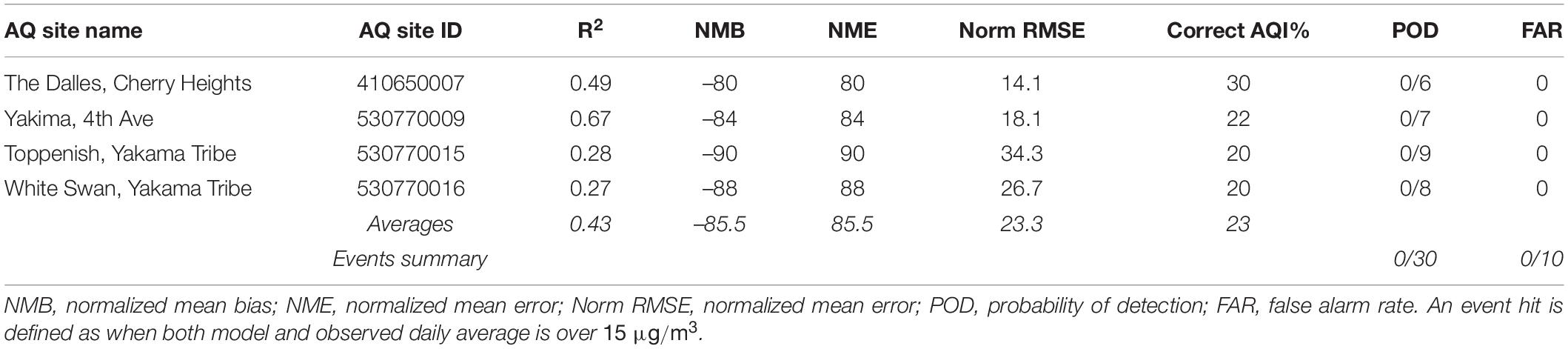
Table 5. Performance statistics for AIRPACT5 CMAQ simulation 24-h PM2.5 at AQ-monitoring sites for the 2015 Cougar Creek Fire.

Table 6. Same as Table 5, but for the WRF-SFIRE-informed simulations.
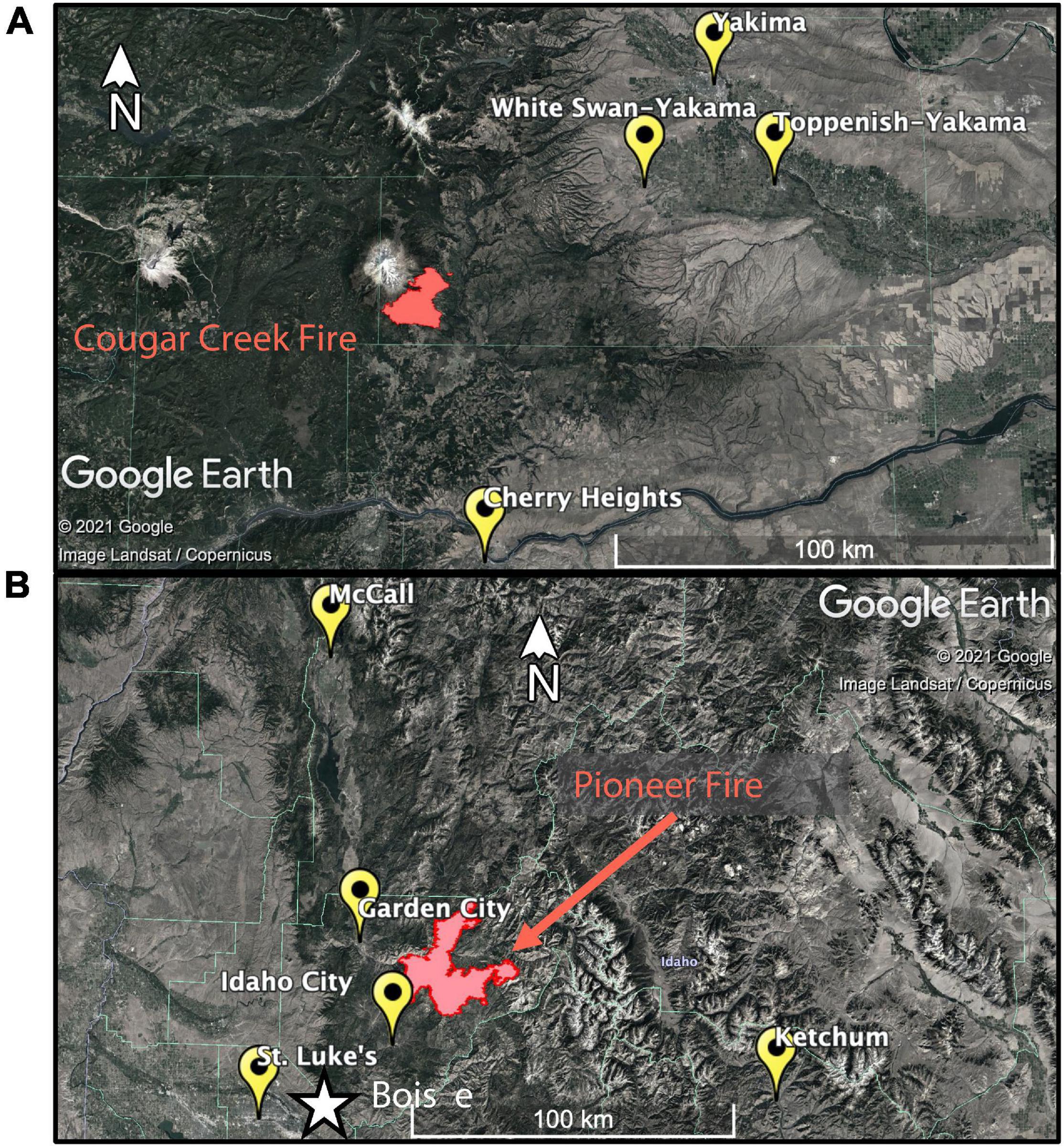
Figure 7. Map of air quality station (yellow pins) and infrared fire perimeters (red polygons) for the (A) Cougar Creek and (B) the Pioneer fires.
Comparisons between the performance statistics for the 2015 Cougar Creek Fire are presented in Table 5 for AIRPACT5, and in Table 6 for WRF-SFIRE with CMAQ. Performance statistics for R2 decreased slightly from 0.43 to 0.31, while the normalized mean bias (NMB) and normalized mean error (NME) also saw small reductions of –85 to –72 and 85 to 72, respectively. The normalized RMSE decreased from ∼23 to ∼11μgm− 3 while the percentage of correct Air Quality Index (AQI) category attributions increased from 23 to 30%. To assess the skill of the model in capturing smoke episodes, several other performance metrics were investigated. Here, we analyzed the number of successfully captured smoke days when the average daily PM2.5 concentration exceeded 15 μgm−3 (—i.e., probability of detection or POD). The number of false alarms were defined as the number of days when station data did not confirm elevated daily PM2.5 concentrations predicted by the model (—i.e., the false alarm rate or FAR). For the Cougar Creek Fire, the WRF-SFIRE-driven run also exhibited a noticeable improvement for event detections (3 for the WRF-SFIRE driven run vs. 0 for the original AIRPACT). It should be noted that the values in the bottommost row of Tables 5–8 for the POD represent cumulative values, summed across all the stations. For the FAR, the summary values (Tables 5, 6) correspond to the number of false alarms across the stations with respect to the number of simulated days (e.g., 10 for the Cougar Creek Fire).
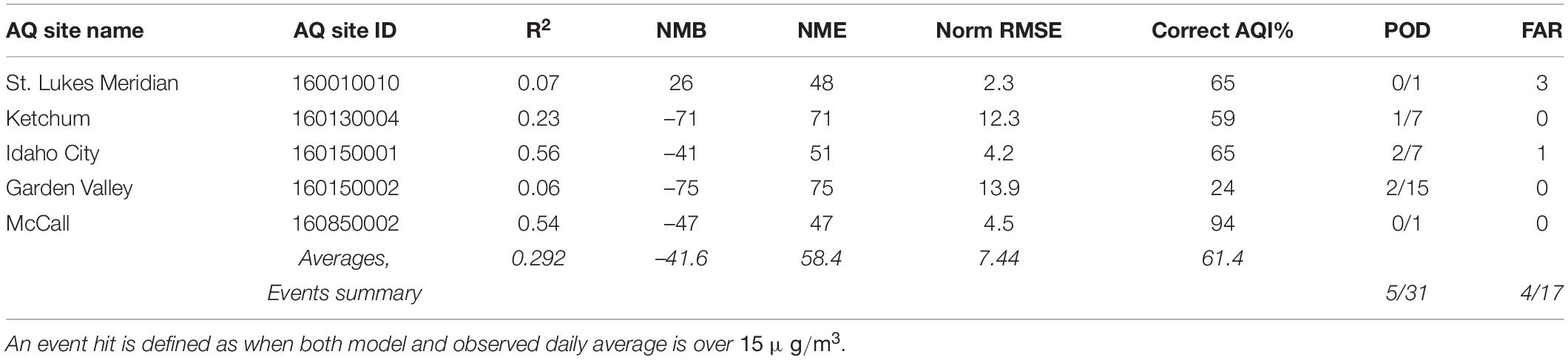
Table 7. Performance statistics for AIRPACT5 CMAQ simulation 24-h PM2.5 at AQ-monitoring sites for the 2016 Pioneer Fire.
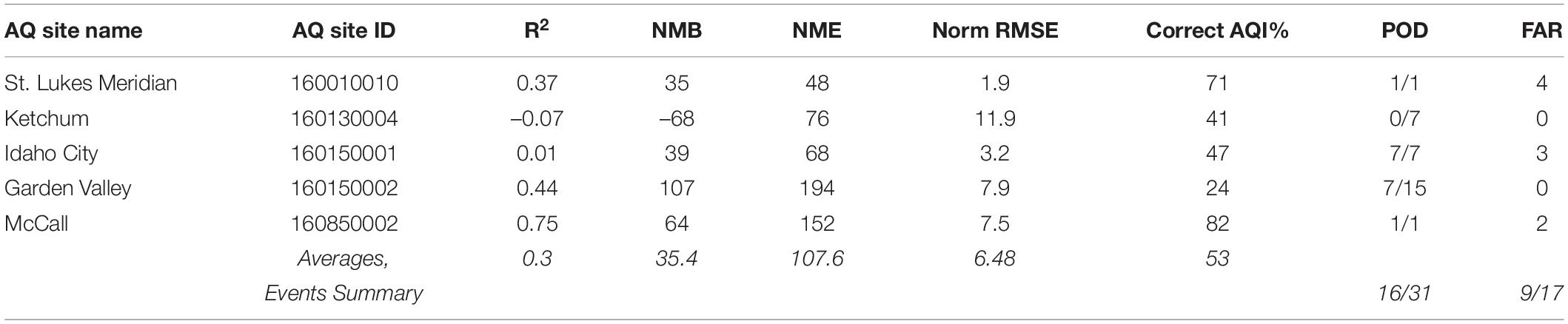
Table 8. Same as Table 5, but for the WRF-SFIRE-informed simulations.
Pioneer Fire, 2016
Model evaluations for the original AIRPACT and WRF-SFIRE-informed simulations for the Pioneer Fire can be seen in Tables 7, 8, respectively. The location of the AirNow sites relative to the Pioneer Fire can be seen in Figure 7B.
Comparison of the performance statistics for the 2016 Pioneer Fire are presented in Tables 7, 8 for AIRPACT5 and WRF-SFIRE with CMAQ, respectively. Overall, R2 remained about the same at ∼0.3, The NMB improved from ∼42 to ∼35, while the NME worsened from ∼58 to ∼108, the Normalized RMSE improved slightly, and the Correct Category AQI% worsened by ∼8%. The FAR nearly doubled, however the POD also increased for the WRF-SFIRE informed CMAQ simulations. The original run captured 5 out of 31 events while the WRF-SFIRE driven AIRPACT run captured 16 of 31 events. In summary, the simulations utilizing the coupled fire-atmosphere model had more false alarms, showing mixed performance against AIRPACT in terms of NMB, NME and RMSE, but showed a large improvement in the POD.
Computational Considerations
Both WRF-SFIRE simulations were executed on six 36-core nodes (216 CPUs total) and had a very similar computation cost. Each 48-h segment of the Pioneer Fire simulation took about 2.7 h wall clock time, while the full 6 day run required ∼ 8.2 h to run. Similarly, the Pioneer Fire 48-h simulations needed ∼2.7 h wall time. The coupled fire-atmosphere simulation as expected carried a relatively high computational burden. However, it should be emphasized that this cost was still much lower than what would be required to run a fully integrated plume-resolving simulation that includes chemistry. For example, the same Pioneer Fire forecasts run with WRF-SFIRE-CHEM would require ∼5 times the original runtime even using a relatively simple aerosol scheme with no chemistry (GOCART scheme). Coupling with the large-scale AQ system allows modeling frameworks to avoid the high computation cost of the fine-resolution chemical simulations, thus significantly reducing the lead time of fully coupled fire-atmosphere-chemistry forecasts. Since the typical AIRPACT daily runs only provide 48-h forecasts, there is no benefit of extending WRF-SFIRE fire simulations beyond this forecast period.
Discussion
Smoke transport and dispersion is dependent on many processes over a range of spatial and temporal scales, which can be difficult to resolve using a single modeling tool. For this study, we described a proof-of-concept modeling framework that embeds an offline coupled fire-atmosphere model (WRF-SFIRE) within a regional AQ model (AIRPACT). WRF-SFIRE is primarily used to forecast fire growth, local-scale smoke transport, and fire-atmosphere interactions. Since WRF-SFIRE operates on a high-resolution atmospheric grid (grid spacing = 400–500-m), this model can explicitly resolve wildfire-related phenomena such as the wildfire plume rise (Kochanski et al., 2016, 2019). However, running fine-scale AQ simulations comes at the expense of computational resources, which limits WRF-SFIRE from being deployed at the scales needed to simulate regional AQ with full chemistry for forecasting applications.
Out of three different coupling methods investigated as a part of this study, only one method provided satisfactory results. The method that used the plume bottom and height data derived from the fire resolving model WRF-SFIRE, combined with the fuel moisture and fire area, provided the most promising results. In contrast, using the default SMOKE method to compute plume heights from heat flux and fire area did not provide realistic results, despite several configuration attempts. Using heat and area from discrete locations in WRF-SFIRE to recalculate plume height resulted in inconsistent plume dynamics, often characterized by a low vertical plume extent, extreme surface concentrations and limited long-range smoke transport. Aggregating WRF-SFIRE heat fluxes to drive the Brigg’s plume rise parameterization marginally improved smoke simulations, but did not provide the consistent improvements seen when plume height was taken directly from WRF-SFIRE.
The fire progression resolved by WRF-SFIRE generally matched the observed fire area as measured by infrared fire perimeters. Daily burned area differences were <30%, on average. However, the WRF-SFIRE burned area data were not enough to improve the benchmark AIRPACT simulations that use the default BlueSky method. The real benefit of coupling AIRPACT with a high-resolution coupled fire-atmosphere model came from resolving the vertical plume extent. WRF-SFIRE provided realistic plume top heights that compared well with MISR observations. Both WRF-SFIRE simulations had plume rises that were within 300-m of each other. The AIRPACT-CMAQ estimated plume top heights for the Cougar Creek Fire were higher than the MISR observed plume tops by ∼700-m. The WRF-SFIRE plume rise results were encouraging given that previous work has shown plume rise parameterizations often have a difficult time predicting plume top heights (Val Martin et al., 2012). The WRF-SFIRE plume rise evaluations presented here are consistent with results from Kochanski et al. (2016) and Kochanski et al. (2019), and Mallia et al. (2020b).
Noticeable improvements in WRF-SFIRE-informed AIRPACT simulations were observed at AQ stations near the Cougar Creek Fire. Here, the normalized RMSE was reduced by 50% while the model was able to identify the correct AQ category and elevated PM2.5 episodes more frequently when using the WRF-SFIRE forecasted burned area and vertical smoke distribution. Model improvements for the Pioneer Fire were less clear, with little to no value added in terms of the model bias. However, there was some improvement in the correlation coefficient (0.3 vs. 0.22). The WRF-SFIRE driven AIRPACT run was also able to detect more PM2.5 events, but also exhibited more false alarms relative to the standard AIRPACT configuration. Despite each fire being located near several AQ stations, we suspect that the relative scarcity of AQ observations limited our ability to definitively evaluate the model performance for the Pioneer Fire. This issue is discussed more in-depth in Mallia et al. (2020a). Another issue with this analysis is that due to many concurrent wildfire events, it is difficult to ascertain whether smoke from other regional wildfires were contributing to model errors observed here. Based on satellite imagery for each of the fires, the smoke plumes were relatively isolated from other smoke plumes. Nonetheless, both the Cougar Creek and Pioneer fires occurred during active wildfire seasons where some background smoke was likely present across the Pacific Northwest.
The promising results of this study suggest that using a coupled fire-atmosphere model as a “super-parameterization” within a regional AQ model is feasible. Explicitly resolving the wildfire plume with a coupled fire-atmosphere model represents the largest improvement for the host AQ model. While limited computational resources may prevent a coupled fire-atmosphere model from simulating all concurrent wildland fires (—i.e., the entire Western United States), WRF-SFIRE could be used to forecast fire progression and plume rises for select events in the vicinity of densely populated areas.
It is worth noting that this study only focused on the two aspects smoke modeling, namely the burnt area and the vertical plume structure. The overall meteorology driving smoke dispersion in AIRPACT was identical in both experiments. Therefore, the errors in simulated smoke dispersion associated with the biases in the input meteorology could not be addressed through the coupling strategy deployed here. While it is plausible to embed WRF-SFIRE directly within CMAQ/AIRPACT, this method was not tested. Given that wildfires and smoke transport are multiscale phenomena (Goodrick et al., 2012), multiscale solutions, such as the modeling framework presented here, are likely be needed to improve smoke forecasting. Advancing computing capabilities and cloud computing will hopefully make integrations between fire-atmosphere models and regional AQ systems easier in the future.
Data Availability Statement
The WRF-SFIRE model used in this study can be found in online repositories. The names of the repository/repositories and accession number(s) can be found below: https://github.com/openwfm/WRF-SFIRE.
Author Contributions
AK, JV, and FH-T were responsible for designing the experiment. DM, FH-T, and JV ran the analyses presented in this study. AK ran the coupled fire atmosphere simulations, while FH-T and JV ran AIRPACT. AK, JM, and FH-T modified the model code necessary for offline coupling between AIRPACT and WRF-SFIRE. AK, DM, FH-T, and JV wrote the manuscript. JV and AK were responsible for acquiring the funding necessary to carry out the work presented here.
Funding
This work was supported by the Utah Department of Environmental Quality Division of Air Quality, by Joint Fire Science Program grant L15AC00170, by NW-AIRQUEST’s ongoing support for AIRPACT, by NASA grant 80NSSC19K1091, and by NSF grants DEB-2039552 and ICER-1664175.
Conflict of Interest
The authors declare that the research was conducted in the absence of any commercial or financial relationships that could be construed as a potential conflict of interest.
Publisher’s Note
All claims expressed in this article are solely those of the authors and do not necessarily represent those of their affiliated organizations, or those of the publisher, the editors and the reviewers. Any product that may be evaluated in this article, or claim that may be made by its manufacturer, is not guaranteed or endorsed by the publisher.
Acknowledgments
We would like to acknowledge high-performance computing support from both Cheyenne (doi: 10.5065/D6RX99HX, provided by NCAR’s Computational and Information Systems Laboratory, sponsored by the National Science Foundation), and the high-performance computing system at Washington State University, Voiland College of Engineering and Architecture. We are also grateful for University of Utah’s Center for High Performance Computing (CHPC) for providing the computational resources needed to carry out model analyses shown here.
Footnotes
- ^ https://www.cmascenter.org/smoke/
- ^ https://www.geomac.gov
- ^ https://a.atmos.washington.edu/wrfrt/
References
Ahmadov, R., Grell, G., James, E., Freitas, S., Pereira, G., Csiszar, I., et al. (2017). A high-resolution coupled meteorology-smoke modeling system HRRR-Smoke to simulate air quality over the CONUS domain in real time. Geophys. Res. Abstracts 19:10841.
Anderson, H. E. (1982). Aids to Determining Fuel Models for Estimating Fire Behavior. Sidney: USDA Forest Service.
Briggs, G. A. (1982). “Plume rise predictions,” in Lectures on Air Pollution and Environmental Impact Analysis, ed. D. Haugen (Massachusetts: American Meteorological Society), 59–111. doi: 10.1007/978-1-935704-23-2_3
Bytnerowicz, A., Hsu, Y.-M., Percy, K., Legge, A., Fenn, M. E., Schilling, S., et al. (2016). Ground-level air pollution changes during a boreal wildland mega-fire. Sci. Total Environ. 572, 755–769. doi: 10.1016/j.scitotenv.2016.07.052
Byun, D., and Schere, K. L. (2006). Review of the governing equations, computational algorithms, and other components of the Models-3 Community Multiscale Air Quality (CMAQ) Modeling System. Appl. Mechanics Rev. 59, 51–77. doi: 10.1115/1.2128636
Chen, J., Vaughan, J., Avise, J., O’Neill, S., and Lamb, B. (2008). Enhancement and evaluation of the AIRPACT ozone and PM2.5 forecast system for the Pacific Northwest. J. Geophys. Res. 113:D14305.
Clark, T. L., Coen, J., and Latham, D. (2004). Description of a coupled atmosphere-fire model. Int. J. Wildland Fire 13, 49–64. doi: 10.1071/wf03043
Dennison, P. E., Brewer, S. C., Arnold, J. D., and Moritz, M. A. (2014). Large wildfiretrends in the western United States,1984–2011. Geophys. Res. Lett. 41, 2928–2933.
Emmons, L. K., Walters, S., Hess, P. G., Lamarque, J.-F., Pfister, G. G., Fillmore, D., et al. (2010). Description and evaluation of the Model for Ozone and Related chemical Tracers, version 4 (MOZART-4). Geosci. Model Dev. 3, 43–67. doi: 10.5194/gmd-3-43-2010
Goodrick, S. L., Achtemeier, G. L., Larkin, N. K., Narasimhan, Y., and Strand, T. (2012). Modelling smoke transport from wildland fires: a review. Int. J. Wildland Fire 22, 83–94. doi: 10.1071/WF11116
Graff, C. A., Coffield, S. R., Chen, Y., Foufoula-Georgiou, E., Randerson, J. T., and Smyth, P. (2020). Forecasting daily wildfire activity using poisson regression. IEEE Trans. Geosci. Remote Sens. 58, 7, 4837–4851. doi: 10.1109/TGRS.2020.2968029
Grell, G. A., and Devenyi, D. (2002). A generalized approach to parameterizing convection combining ensemble and data assimilation techniques. Geophys.Res. Lett. 29:1693.
Herron-Thorpe, F. L., Mount, G. H., Emmons, L. K., Lamb, B. K., Jaffe, D. A., Wigder, N. L., et al. (2014). Air quality simulations of wildfires in the Pacific Northwest evaluated with surface and satellite observations during the summers of 2007 and 2008. Atmos. Chem. Phys. 14, 12533–12551. doi: 10.5194/acp-14-12533-2014
Iacono, M. J., Delamere, J. S., Mlawer, E. J., Shephard, M. W., Clough, S. A., and Collins, W. D. (2008). Radiative forcing by long-lived greenhouse gases: calculations with the AER radiative transfer models. J. Geophys. Res. 113:D13103.
Jaffe, D., Bertschi, I., Jaegle, L., Novelli, P., Reid, J. S., Tanimoto, H., et al. (2004). Long-range transport of Siberian biomass burning emissions and impact on surface ozone in western North America. Geophys. Res. Lett. 31:L16106.
Jaffe, D. A., O’Neill, S. M., Larkin, N. K., Holder, A. L., Peterson, D. L., Halofsky, J. E., et al. (2020). Wildfire and prescribed burning impacts on air quality in the United States. J. Air Waste Manage. Assoc. 70, 583–615.
Jaffe, D. A., and Wigder, N. L. (2012). Ozone production from wildfires: a critical review. Atmos. Environ. 51, 1–10. doi: 10.1016/j.atmosenv.2011.11.063
Kochanski, A., Mallia, D. V., Fearon, M., Brown, T., and Mandel, J. (2019). Modeling wildfire smoke feedback mechanisms using a coupled fire-atmosphere model with a radiatively active aerosol scheme. J. Geophys. Res. 124, 9099–9116. doi: 10.1029/2019jd030558
Kochanski, A. K., Jenkins, M. A., Yedinak, K., Mandel, J., Beezley, J., and Lamb, B. (2016). Toward an integrated system for fire, smoke, and air quality simulations. Int. J. Wildland Fire 25, 558–568.
Larkin, N. K., O’Neill, S. M., Solomon, R., Raffuse, S., Strand, T., Sullivan, D. C., et al. (2009). The bluesky smoke modeling framework. Int. J. Wildland Fire 18, 906–920.
Lin, Y.-L., Farley, R., and Orville, H. D. (1983). Bulk parameterization of the snowfield in a cloud model. J. Climate Appl. Meteor. 22, 1065–1092. doi: 10.1175/1520-0450(1983)022<1065:bpotsf>2.0.co;2
Liu, J. C., Mickley, L. J., Sulprizio, M. P., Dominici, F., Yue, X., Ebisu, K., et al. (2016). Particulate air pollution from wildfires in the Western U.S. under climate change. Clim. Change 138, 655–666. doi: 10.1007/s10584-016-1762-6
Mallia, D. V., Kochanski, A., Urbanski, S., and Lin, J. C. (2018). Optimizing smoke and plume rise modeling approaches at local scales. Atmosphere 9:116.
Mallia, D. V., Kochanski, A., Urbanski, S., Mandel, J., Farguell, A., and Krueger, S. (2020b). Incorporating a canopy parameterization within a coupled fire-atmosphere model to improve a smoke simulation for a prescribed burn. Atmosphere 11:832.
Mallia, D. V., Kochanski, A., Kelly, K. E., Whitaker, R., Xing, W., Mitchell, L., et al. (2020a). Evaluating wildfire smoke transport within a coupled fire-atmosphere model using a high-density observation network for an episodic smoke event along Utah’s Wasatch Front. J. Geophys. Res. 125:e2020JD032712.
Mandel, J., Amram, S., Beezley, J. D., Kelman, G., Kochanski, A. K., Kondratenko, V. Y., et al. (2014). Recent advances and applications of WRF-SFIRE. Nat. Hazards Earth Syst. Sci. 14, 2829–2845. doi: 10.5194/nhess-14-2829-2014
Mandel, J., Beezley, J. D., and Kochanski, A. K. (2011). Coupled atmosphere-wildland fire modeling with WRF 3.3 and SFIRE 2011. Geosci. Model Dev. 4, 591–610. doi: 10.5194/gmd-4-591-2011
Mandel, J., Vejmelka, M., Kochanski, A. K., Farguell, A., Haley, J. D., Mallia, D. V., et al. (2019). “An interactive data-driven HPC system for forecasting weather, wildland fire, and smoke,” in 2019 IEEE/ACM HPC for Urgent Decision Making (UrgentHPC), (Manhattan: IEEE), 35–44.
Mass, C. F., and Ovens, D. (2019). The Northern California Wildfires of 8–9 October 2017: the role of a major downslope wind event. Bull. Am. Meteorol. Soc. 100, 235–256. doi: 10.1175/bams-d-18-0037.1
Mavko, M., and Morris, R. (2013). DEASCO3 Project Updates to the Fire Plume Rise Methodology to Model Smoke Dispersion. Denver: Air Sciences, Inc.
McClure, C. D., and Jaffe, D. A. (2018). US particulate matter air quality improves except in wildfire-prone areas. Proc. Natl. Acad. Sci. U. S. A. 115, 7901–7906. doi: 10.1073/pnas.1804353115
Mellor, G. L., and Yamada, T. (1982). Development of a turbulence closure model for geophysical fluid problems. Rev. Geophys. Space Phys. 20, 851–875. doi: 10.1029/rg020i004p00851
Mesinger, F., DiMeg, G., Kalnay, E., Mitchell, K., Shafran, P. C., Ebisuzaki, W., et al. (2006). North American regional reanalysis. Bull. Am. Meteorol. Soc. 87, 343–360.
Pouliot, G., Pierce, T., Benjey, W., O’Neill, S. M., and Ferguson, S. A. (2005). “Wildfire emission modeling: integrating BlueSky and SMOKE,” in 14th International Emission Inventory Conference, (Las Vegas: National Exposure Research Laboratory), 11–14.
Raffuse, S. M., Pryden, D. A., Sullivan, D. C., Larkin, N. K., Strand, T., and Solomon, R. (2009). SMARTFIRE Algorithm Description. Seattle: Pacific Northwest Research Laboratory
Ravi, V., Gao, A. H., Martinkus, N. B., Wolcott, M. P., and Lamb, B. K. (2018). Air quality and health impacts of an aviation biofuel supply chain using forest residue in the northwestern United States. Environ. Sci. Technol. 52, 4154–4162. doi: 10.1021/acs.est.7b04860
Ravi, V., Vaughan, J. K., Wolcott, M. P., and Lamb, B. K. (2019). Impacts of prescribed fires and benefits from their reduction for air quality, health, and visibility in the Pacific Northwest of the United States. J. Air Waste Manage. Assoc. 69, 289–304. doi: 10.1080/10962247.2018.1526721
Rothermel, R. C. (1972). A Mathematical Model for Predicting Fire Spread in Wildland Fires. Sidney: USDA Forest Service.
Skamarock, W., Klemp, J. B., Dudhia, J., Gill, D. O., Barker, D. M., Duda, M. G., et al. (2008). A Description of the Advanced Research WRF Version 3. Boulder: NCAR.
Spracklen, D. V., Mickley, L. J., Logan, J. A., Hudman, R. C., Yevich, R., Flannigan, M. D., et al. (2009). Impacts of climate change from 2000 to 2050 on wildfire activity and carbonaceous aerosol concentrations in the western United States. J. Geophys. Res. 114:D20301.
Tang, Y., Zhong, S. Y., Luo, L. F., Bian, X., Heilman, W. E., and Winkler, J. (2015). The potential impact of regional climate change on fire weather in the United States. Ann. Am. Assoc. Geogr. 105, 1–21. doi: 10.1080/00045608.2014.968892
Tewari, M. F., Chen, W., Wang, J., Dudhia, M. A., LeMone, K., Mitchell, M. E., et al. (2004). “Implementation and verification of the unified NOAH land surface model in the WRF model,” in 20th conference on weather analysis and forecasting.16th Conference on Numerical Weather Prediction, (Seattle: American Meteorological Society), 11–15.
Val Martin, N., Kahn, R., Logan, J. A., Paugnam, R., Wooster, M., and Ichoku, C. (2012). Space-based observational constraints for 1-D fire smoke plume-rise models. J. Geophys. Res. 117:D22204.
Vaughan, J., Lamb, B., Frei, C., Wilson, R., Bowman, C., Figueroa-Kaminsky, C., et al. (2004). A numerical daily air-quality forecast system for the Pacific Northwest. Bull. Am. Meteorol. Soc. 85, 549–561. doi: 10.1175/bams-85-4-549
Vejmelka, M., Kochanski, A. K., and Mandel, J. (2016). Data assimilation of dead fuel moisture observations from remote automatic weather stations. Int. J. Wildland Fire 25, 558–568. doi: 10.1071/wf14085
Walter, C., Freitas, S. R., Kottmeier, C., Kraut, I., Rieger, D., Vogel, H., et al. (2016). The importance of plume rise on the concentrations and atmospheric impacts of biomass burning aerosol. Atmos. Chem. Phys. 16, 9201–9219. doi: 10.5194/acp-16-9201-2016
Westerling, A. L., Hidalgo, H. G., Cayan, D. R., and Swetnam, T. W. (2006). Warming and earlier spring increases western U.S. forest fire activity. Science 313, 940–943. doi: 10.1126/science.1128834
Wiedinmyer, C., Akagi, S. K., Yokelson, R. J., Emmons, L. K., Al-Saadi, J. A., Orlando, J. J., et al. (2011). The Fire INventory from NCAR (FINN): a high resolution global model to estimate the emissions from open burning. Geosci. Model Dev. 4, 625–641.
Keywords: air quality modeling, smoke modeling, fire modeling, coupled fire-atmosphere model, WRF-SFIRE, AIRPACT, Cougar Creek Fire
Citation: Kochanski AK, Herron-Thorpe F, Mallia DV, Mandel J and Vaughan JK (2021) Integration of a Coupled Fire-Atmosphere Model Into a Regional Air Quality Forecasting System for Wildfire Events. Front. For. Glob. Change 4:728726. doi: 10.3389/ffgc.2021.728726
Received: 21 June 2021; Accepted: 08 October 2021;
Published: 10 November 2021.
Edited by:
Chad M. Hoffman, Colorado State University, United StatesReviewed by:
Gianni Pagnini, Basque Center for Applied Mathematics, SpainScott Goodrick, Southern Research Station, Forest Service, United States Forest Service, United States Department of Agriculture (USDA), United States
Copyright © 2021 Kochanski, Herron-Thorpe, Mallia, Mandel and Vaughan. This is an open-access article distributed under the terms of the Creative Commons Attribution License (CC BY). The use, distribution or reproduction in other forums is permitted, provided the original author(s) and the copyright owner(s) are credited and that the original publication in this journal is cited, in accordance with accepted academic practice. No use, distribution or reproduction is permitted which does not comply with these terms.
*Correspondence: Adam K. Kochanski, YWRhbS5rb2NoYW5za2lAc2pzdS5lZHU=