- 1College of Resources and Environmental Sciences, Tibet Agricultural and Animal Husbandry University, Nyingchi, China
- 2Key Laboratory of Forest Ecology in Tibet Plateau, Tibet Agricultural and Animal Husbandry University, Nyingchi, China
- 3Lhasa Plateau Ecosystem Research Station, Key Laboratory of Ecosystem Network Observation and Modeling, Institute of Geographic Sciences and Natural Resources Research, Chinese Academy of Sciences, Beijing, China
- 4State Key Laboratory of Desert and Oasis Ecology, Xinjiang Institute of Ecology and Geography, Chinese Academy of Sciences, Urumqi, China
- 5Cele National Station of Observation and Research for Desert-Grassland Ecosystems, Cele, China
- 6University of Chinese Academy of Sciences, Beijing, China
- 7College of Chemistry, Chemical Engineering and Resource Utilization, Northeast Forestry University, Harbin, China
Soil quality evaluation is the foundation for understanding the current situation of soil quality and promoting the sustainable utilization of land resources. However, the evaluation of the impacts of the different long-term afforestation timescales on the soil quality in a typical watershed valley area is still insufficient. Here, based on the soil samples collected from different afforestation years of 2, 5, 11, 15, and 31 years and natural sample plots with similar site conditions in the same region, the gray target model and criteria importance through intercriteria correlation (CRITIC) method are used in this study to evaluate the soil quality of the sample plots with different afforestation years and the corresponding natural sample plots in the Tibet Plateau. Results show that: (1) The most positive soil physical and chemical indexes increase with the increase in afforestation years based on the analysis of the characteristics, variation, and fertility grade of 13 soil physical and chemical indexes in different areas. The content of most soil physical and chemical indexes is greater than that in the corresponding natural sample plots in 31 years of afforestation. (2) The bullseye degree of the soil quality in the study area is divided into five grades according to the principle of equal division and integration. The soil quality grade of the corresponding sample plot after 15 years of afforestation is grade 4. The other plots are grade 5. (3) The soil quality index (SQI) generally increases with the increase of in forestation years. The different afforestation years indicate SQI (0.709) of 15 years > SQI (0.662) of 31 years > SQI (0.426) of 11 years > SQI (0.348) of 2 years > SQI (0.309) of 5 years. (4) The full data set method verifies the effectiveness of the gray target model. The two soil quality evaluation methodologies are consistent and can verify each other’s accuracy. The correlation coefficient of the two results reaches 0.957. This study finds that the long-term afforestation timescales have improved the soil quality of watershed valley. Available phosphorus, silt, soil water content are the main factors impacting the soil quality in the afforestation timescales.
Introduction
Soil is the fundamental element that connects the atmosphere, hydrosphere, biosphere, and lithosphere (Lehmann et al., 2020; Varol et al., 2020). This component plays an important role in maintaining the natural ecosystem services and human society health (Li X. et al., 2021; Cetin and Jawed, 2022), ensuring environmental and energy security, and protecting biodiversity (Zhu et al., 2015; Pekkan et al., 2021). A series of soil degradation problems, such as soil erosion, fertility decline, salinization, and soil pollutions, has emerged because of the intensified human activities and climate change. Recently, the soil degradation issues have posed major threats to the ecological environmental security at the local, regional, and national levels (Lal, 2015; Smith et al., 2016), especially on the Qinghai–Tibet Plateau (Chen et al., 2013; Zhang et al., 2022).
The Qinghai–Tibet Plateau is the highest plateau on Earth with an average elevation of 4,000 m (Sun et al., 2019; Zhang et al., 2022), having an important ecological security barrier (Mu et al., 2021). The ecosystems on the Plateau have generally shown degradation over the decades. The restoration and reconstruction of the degraded ecosystems have become a key target for the sustainable Qinghai–Tibet Plateau (Qin et al., 2017). The Yarlung Zangbo River Basin is located at the hinterland of the Qinghai–Tibet Plateau. It is the largest outflow river in Southwest China. Moreover, this basin is an important geographical barrier and water vapor channel in China. However, the vegetation and ecological environment are extremely fragile in this area. The desertification is highly serious. The effects of global warming, human activities, and climate change have seriously degraded the soil quality of the Yarlung Zangbo River Basin. Some studies have pointed out the different degrees of desertification, grassland degradation, soil erosion, salinization, freeze–thaw erosion, and other different land degradation phenomena in the middle reaches of the Yarlung Zangbo River (Chen et al., 2022). Grassland degradation is more serious, having showed a moderate degradation level in the study of comprehensive land degradation in the Qinghai–Tibet Plateau (Wang et al., 2009). The study of soil quality in the middle reaches of the Yarlung Zangbo River is of great significance for protecting land resources and ecological environment and slowing down land degradation on the Tibetan Plateau.
Since the concept of soil quality was proposed in 1971 (Mausel, 1971), it has been continuously improved and developed for more than 50 years. The development of soil quality connotation mainly experienced three stages: soil productivity, soil quality, and soil health (Doran et al., 1994). Soil quality and soil health have different connotations. Soil health is a “dynamic” and “potential” soil property. This aspect pays more attention to the natural resource, environmental, and ecological attributes, including resistance and resilience of soil as an ecosystem (Brady and Weil, 2007). By contrast, soil quality focuses on the “internal” and “static” status of soil on a long-term scale. Soil quality is measured by soil physical, chemical, and biological properties at a temporal or spatial scale (Larson and Pierce, 1991; Doran et al., 1996; Bastida et al., 2008; Idowu et al., 2008). Soil quality or health is evaluated mainly from the ecosystem service functions (biological production, soil conservation, environmental purification, biodiversity conservation, water conservation, climate regulation, nutrient and carbon cycle, and cultural inheritance) (Lal, 2016).
Soil quality evaluation is the basis for mastering the soil quality status and promoting sustainable utilization of land resources. The common methods of soil quality evaluation are divided into qualitative and quantitative ways. The researchers mainly used multivariate index Kriging method (Smith et al., 1993; Liu et al., 2006), soil quality dynamics method (Doran et al., 1994), soil quality comprehensive scoring method (Larson and Pierce, 1994), and soil relative quality method (Fu et al., 2004) in the early stage of soil quality evaluation before the 1990s. At the beginning of the 21st century, the fuzzy mathematics method has been preliminarily applied in soil quality evaluation by using the soil quality index (SQI) method (Chen et al., 2021; Zhu et al., 2021), gray correlation analysis (He et al., 2022), matter–element method (Yu et al., 2012), artificial neural network method (Yang et al., 2008), and TOPSIS method (Xie et al., 2019). Many scholars have used the SQI to quantitatively evaluate the comprehensive soil quality. The SQI method can be widely used for the qualitative and quantitative evaluation of the soil quality of different land use types because of its simple operation and flexible quantization (Rairsi, 2017). Despite the full data set method is a tool for evaluating the SQI through all the physical and chemical indicators comprehensively and accurately in the process of soil quality assessment, the determination of many evaluation indicators is often time-consuming and laborious (Huang and Xu, 2010). In recent years, to analyze the soil quality using a relatively small amount of data, the criteria importance through intercriteria correlation (CRITIC) method and gray target model is used to comprehensively measure the SQI, and shows the advantage of methods. How to select the such effective approaches containing important soil information has become challenge in the soil quality assessment.
The desertification trend in the Yarlung Zangbo River Basin has been significantly improved in recent years (Smith et al., 1993), with the development of key regional ecological public welfare forest construction project, ecological security barrier construction project, sand prevention, and control demonstration area project in the middle reaches of the Yarlung Zangbo River. Soil quality has been effectively improved. The main studies on the Yarlung Zangbo River Basin are sandy soil physical and chemical properties (Wang et al., 2020), soil erosion (Tan et al., 2019), soil moisture (Li et al., 2013), soil bacterial community structure (Chen et al., 2022). Relevant studies on soil quality evaluation of alpine grassland on the Qinghai–Tibet Plateau are also available. However, there are relatively few studies on soil quality evaluation resulting from the specific ecological engineering afforestation measures using effective approaches.
The aim of this study adopts the CRITIC method and the improved gray target model to evaluate the soil quality change and its responses to different afforestation years in Renbu, Gongga, Zhanang, and Sangri counties in the middle reaches of the Yarlung Zangbo River Basin: (1) the changes in the soil’s physical and chemical properties between different afforestation years and control plots are examined; (2) the SQI of different afforestation years and the contribution of different soil quality evaluation indexes to soil quality are assessed; and (3) the consistency and accuracy of the improved gray target model is verified by comparing the correlation indexes with full data set methods in evaluating the soil quality in this region.
Study area
The study area is located at the middle reaches of Yarlung Zangbo River in Tibet Autonomous Region. This area is located between 29°00′–29°40′N and 89°30′–92°30′E (Figure 1). The administrative units include Sangri, Zhanang, and Gongga counties in Shannan City and Renbu County in Xigazê City. The middle reaches of the Yajiang River is a temperate semi-humid and semi-arid plateau monsoon climate zone, with intense radiation, long sunshine duration, low average temperature, and low total accumulated temperature (Du and Yang, 2013). The mean annual temperature in the Yarlung Zangbo River Basin decreases with the increase in altitude and latitude. The average annual precipitation gradually decreases from east to west.
The precipitation in the basin is unevenly distributed throughout the year. In particular, the precipitation is mainly concentrated from June to September, accounting for 86.6% of the annual precipitation. Among the four counties sampled, the terrain of Sangri County is high from north to south and valley in the middle, with an average altitude of 3,800 m, annual precipitation of 418.0 mm, and annual average temperature of 8.2°C. The terrain of Zhanang County is high from north to south and valley in the middle, with an average altitude of 3,620 m, precipitation of 402.7.0 mm, and annual average temperature of 8.1°C. Gongga County is located at the west and east directions, with an average altitude of 3,750 m, annual precipitation of 392.1 mm, and annual average temperature of 8.6°C. The terrain of Renbu County is high in the northeast and low in the southwest, with an average altitude of 3,950 m, annual precipitation of 385.0 mm, and annual average temperature of 6.5°C.
The study area is mainly composed of a tall mountain system and intermountain valley. The terrain is complex and varied. The relatively flat valley terraces and alluvial plains provide favorable topographic conditions for agricultural cultivation in the region. Furthermore, valley winds are frequent due to the impact of topography. The land along the river is prone to desertification. The soil is mainly sandy soil. Therefore, the people begin to carry out many ecological and environmental projects in the 1980s in this area, such as afforestation, fencing, and grazing prohibition, to protect the environment.
Data sources and methods
Sample collection and analysis
At the beginning of August 2021, a field survey was conducted on the artificial afforestation area in the middle reaches of the Yarlung Zangbo River Basin. The data and soil samples were collected from five forest areas. The areas include Mongolian pine and Hebei poplar in 2019 (2 years of afforestation), Xinjiang poplar in 2016 (5 years of afforestation), silver white poplar in 2010 (11 years of afforestation), silver white poplar in 2006 (15 years of afforestation), and dry willow forest in 1990 (31 years of afforestation). All afforestation areas are located at relatively flat valley terraces and alluvial plains. Control plots are collected under similar topographic conditions around the afforestation area.
Three 20 m × 20 m standard plots were selected in a uniform environment for sampling plantations in different years. A 20 m × 20 m standard plot was selected as a control in the non-afforestation area for comparison. Real-time kinematic was used to measure the elevation and slope direction of the various fields. The slope is measured using a level. Approximately 5–10 photographs were taken down and up at the four corners and center of the quadrat with the camera 3 m above the ground. It is expressed by extracting the proportion of the vertical projection of vegetation in the total area of the photograph. The five-point sampling methods were adopted for soil sampling in each sample plot. The ring knife soil, aluminum box soil, and topsoil at each sample point were sampled at three soil depths of 0–10, 10–20, and 20–30 cm. The aluminum box soil was sampled in layers through a soil drill. Part of each layer of the soil sample was evenly taken from top to bottom and packed into three aluminum boxes. The samples were immediately sealed with sealant to prevent moisture evaporation. Then, the samples were taken back to the laboratory for weighing and measuring soil moisture content (SMC). Finally, the average water content, bulk density, and porosity of the three layers of each sample point are taken as the values of the sample point.
The 1.0 kg of soil was collected from the topsoil of each layer by soil drilling. Three soil samples of 0–30 cm of different afforestation years were taken, each of which was numbered and weighed 1 kg. In addition, three repeated soil samples of 0–30 cm in the natural control plots were also taken. All the soil samples were taken back to the laboratory for determination of soil mechanical composition and soil chemical properties. The vegetation of all plots was also investigated. The basic distribution of the sample plot is shown in Figure 2 and Table 1.
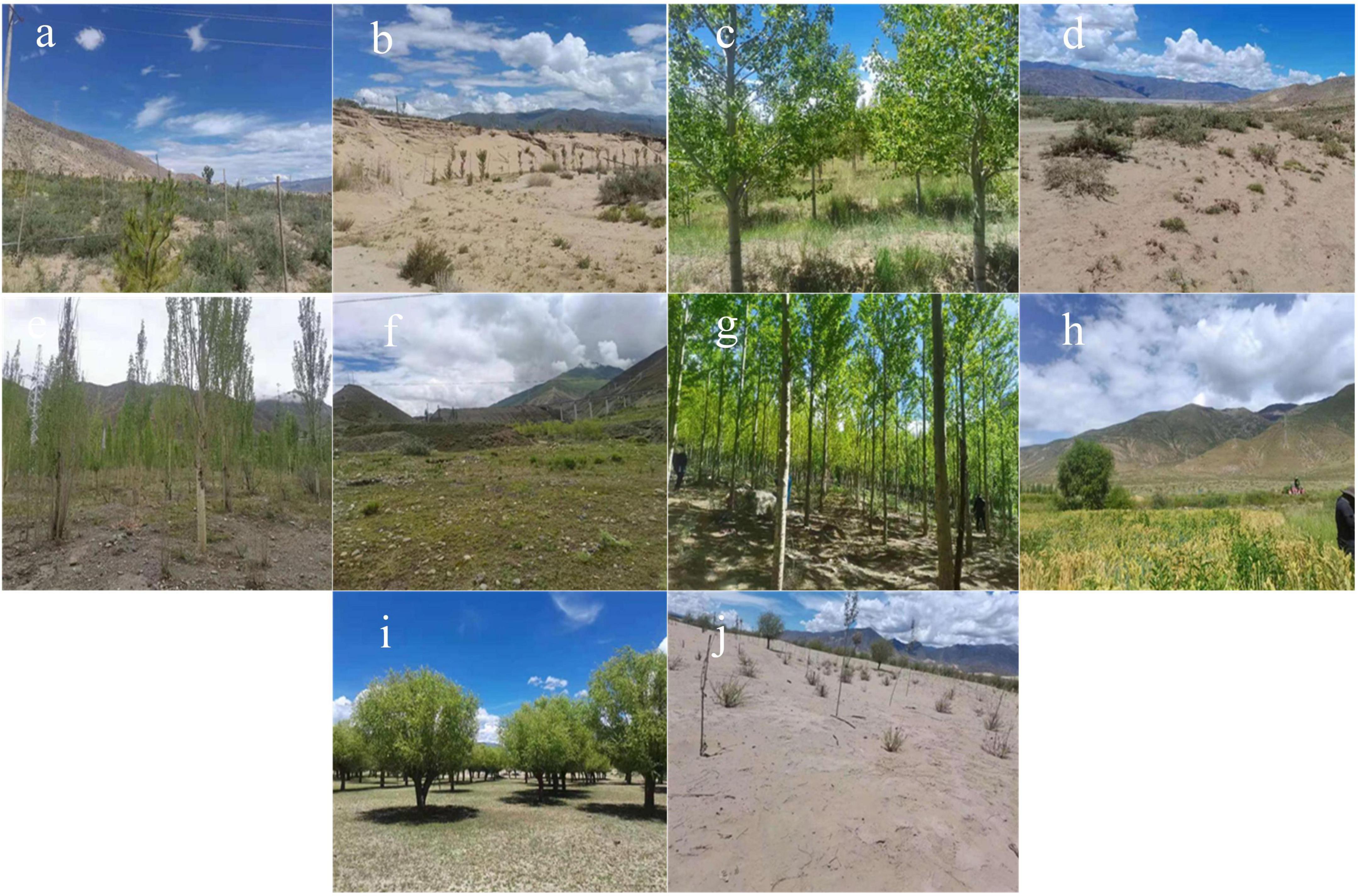
Figure 2. Sample plot landscape of artificial afforestation at different times. Panels (a) and (b), (c) and (d), (e) and (f), (g) and (h), (i) and (j) are artificial afforestation and control plot for 2, 5, 11, 15, and 31 years, respectively.
Vegetation in 2019 (2 years of afforestation) includes Pinus sylvestris var. mongolica Litv., Populus × hopeiensis Hu and Chow, Sophora moorcroftiana (Benth.) Baker, Moq. Dysphania schraderiana (Roemer and Schultes) Mosyakin and Clemants, and Orinus thoroldii (Stapf ex Hemsl.) Bor. Meanwhile, the vegetation of the control plot includes Agriophyllum squarrosum (L.) and Moq. D. schraderiana (Roemer and Schultes) Mosyakin and Clemants. The vegetation in 2016 (afforestation for 5 years) includes Populus alba var. pyramidalis, Poa annua L., and Pennisetum flaccidum Grisebach. The vegetation of the control plot includes P. flaccidum Grisebach. The vegetation in 2010 (11 years of afforestation) includes P. alba, P. annua L., Astragalus licentianus Hand.-Mazz, P. flaccidum Grisebach, Artemisia hedinii Ostenf. et Pauls, and Taraxacum mongolicum Hand.-Mazz. The vegetation of the control plot includes Artemisia stechmanniana Bess., P. annua L, A. licentianus Hand.-Mazz, and Tribulus terrestris Linnaeus. The vegetation in 2006 (15 years of afforestation) includes P. alba var. pyramidalis and S. moorcroftiana (Benth.) Baker. The vegetation of the control plot includes Hordeum vulgare var. coeleste Linnaeus Pisum sativum L. The vegetation in 1990 (31 years of afforestation) includes Salix matsudana Koidz, Eragrostis pilosa (L.) Beauv., Kobresialittledalei C. B. Clarke, and Potentilla chinensis Ser. Lancea tibetica Hook. f. et Thoms. The vegetation of the control plot includes A. squarrosum (L.), Moq. D. schraderiana (Roemer and Schultes) Mosyakin and Clemants, P. flaccidum Grisebach, and A. stechmanniana Bess.
The determination of the physical and chemical properties of the soil samples includes as follows: the soil organic matter (OM) is determined via potassium dichromate oxidation and external heating method. The total nitrogen (TN) is determined via semi-trace Kjeldahl method. The total phosphorus (TP) is determined via molybdenum-antimony resistance colorimetry. The total potassium (TK) is determined with an atomic absorption-flame photometer. The available phosphorus (AP) is determined via sodium bicarbonate extraction-colorimetric method. The alkali-hydrolyzed nitrogen (AN) is determined via alkali-hydrolyzed diffusion method. The soil particle size (clay, silt, and sand) is measured with a particle size analyzer. The SMC is determined via drying method. The soil bulk density (SBD), soil total porosity (TSP), and soil aeration (SA) are determined via ring knife method.
Methods
Gray target model
Gray target theory is a gray correlation analysis theory used to deal with a pattern sequence. The principle is to determine the standard mode, namely, bullseye degree according to the polarity of each index (Li X. et al., 2021). This mechanism combines each mode with the standard mode to form a gray target. When no standard reference mode is available, this mechanism compares the mode to be evaluated with the standard mode by setting a gray target and finding the bullseye degree. This mechanism also compares the mode to be evaluated with the standard mode and identifies the degree to which the mode to be evaluated close to the bullseye degree. Pattern recognition, classification, and optimization are carried out based on the bullseye degree to determine the evaluation grade (Zhang et al., 2021).
First, a standard model was established according to the various soil physical and chemical indexes selected in this study to evaluate the soil quality. In the standard mode, the forward index took the maximum value. Meanwhile, the backward index took the minimum value. Then, the gray target transformation was carried out to obtain the matrix after the gray target transformation. Thereafter, the difference information of the gray correlation was determined to form the difference information matrix of the mode. Finally, we obtained the bullseye coefficient of each index of the model to be evaluated by calculating the bullseye coefficient. The matrix composed of the bullseye coefficients is obtained. The obtained bullseye coefficient and the corresponding weight of each index are substituted into the corresponding formula to calculate the comprehensive bullseye degree of every model to be evaluated.
Building standard patterns
In this study, the soil quality of the different soil samples in various regions is the model to be evaluated. The positive SQIs include organic matter, TN, TP, TK, alkali-hydrolyzable nitrogen, AP, clay particles, soil water content, soil porosity, and SA. The soil quality has a maximum eigenvalue. The SBD of silt and sand is an inverse index, and it has minimal eigenvalues. The standard pattern sequence was determined according to the effect types of different indexes on the soil quality. Specifically, the standard model consists of extreme values of each indicator (Deng, 2002):
where Y0 is the standard mode sequence; and Y0(Xj) is the standard value of the jth index, j = 1, 2, 3, …, n, where n is the number of indicators.
Performing gray target transformation
Let T be the gray target transformation. The target center Tw0 = x0 = (1, 1,…, 1), and the gray target transformation formula of the different indexes is:
where i is the number of samples; and j is the standard value of the evaluation index, and its value range is the same as above.
Determining the gray correlation difference information
The formula represents the difference information between the mode to be evaluated. The standard mode and the meaning of each parameter in the formula are consistent with the previous text.
Calculating the bullseye coefficient
where ρ ∈ (0,∞) is the resolution coefficient. The smaller the value is, the greater the resolution will be. The general value range is (0,1). When the ρ-value ≤ 0.5463, the value is usually taken ρ = 0.5.
Calculating the bullseye degree
where the obtained bullseye coefficient and the corresponding weight of each index are brought into the formula to calculate the comprehensive bullseye degree of each mode; wj is the weight of the jth index; and γ[y0(Xj), yi(Xj)] represents the bullseye coefficient.
Criteria importance through intercriteria correlation method
The CRITIC method is a mechanism used to comprehensively measure the objective weight of indicators by using the contrast strength and conflict between indicators. This method considers the variability of indicators and their correlation. The CRITIC method is a more objective weighting method. The contrast intensity between indicators is reflected by the standard deviation. The larger the standard deviation, the greater the value difference between schemes, and the higher the weight. The conflict is expressed in the form of correlation coefficient. The stronger the correlation, the smaller the conflict, and the lower the weight. The greater the comprehensive weight formed by the combination of standard deviation and correlation coefficient, the higher the weight of the index.
The expression formula of the CRITIC method is as follows:
where Pj is the information contained by the jth indicator; Sj is the standard deviation of the jth indicator and represents the comparative intensity of the jth index; γij is the correlation coefficient between the ith and the jth evaluation indicators, and it is used to quantify the conflict between indicators; and Wj represents the weight of the jth indicator.
Calculation of the soil quality index
Soil quality index is a comprehensive reflection of soil function. The higher the SQI, the higher the soil quality. The SQI is calculated by using the equations determined by the weights and scores of the soil evaluation indexes. The specific steps are as follows: (1) we determine the weight of each index through principal component analysis. The variance of the common factors obtained by principal component analysis can reflect the contribution rate of an index to the overall variance. The larger the value is, the greater the contribution rate to the overall variance will be. The weight of each indicator is calculated by the ratio of the variance of the common factor of each indicator to the sum of the variance of all common factors (Rahmanipour et al., 2014); (2) The dimensionless value of each indicator is determined; and (3) the SQI is calculated according to the calculation formula of the index weight and membership value:
where n is the number of indexes, Si is the membership value of soil indexes, and Wi is the weight of indexes. The SQI ranges from zero to one.
The membership functions of the different soil indexes varies because the different soil physical and chemical property indexes have varying effects on soil quality. The membership function can be divided into ascending and descending types. In this study, the indicators of descending membership function are soil bulk density, silt content, and sand content, while those of ascending membership function include soil organic matter, total nitrogen, total phosphorus, total potassium, available phosphorus, alkali hydrolyzed nitrogen, clay content, soil water content, total soil porosity, and soil aeration.
The ascending membership function formula is as follows:
The descending membership function formula is as follows:
where f(x) is the membership function and x is the measured value of the evaluation index. a, b indicate the minimum and maximum values of the measured values, respectively.
Result
Characteristic statistics of the soil physical and chemical indexes in different afforestation times
The characteristics of the soil physical and chemical indexes are shown in Figure 3. The content of soil organic matter in different plots shows 31 years of afforestation > 15 years of afforestation > 5 years of afforestation > 11 years of afforestation > 2 years of afforestation. Moreover, the content of the soil organic matter typically increases with the increase in afforestation time. Figure 3 shows that the increment of the soil organic matter content is large in the sample plot of 31 and 15 years of afforestation and the sample plot of natural state. The increment in the soil organic matter content is small in the sample plots of 2 and 11 years of afforestation and the corresponding natural sample plots. This finding is related to the afforestation time of the index and the background of the sample plot. The order of the soil TN content from high to low is 31, 15, 5, 11, and 2 years of afforestation. The content of soil TN increases with the increase in afforestation time. The soil TN content of the sample plot of 31 and 15 years of afforestation is quite different from that of the sample plot of the natural state (Figure 3). A little can be observed difference between 2 years of afforestation and the corresponding natural plots. The TN content slightly decreases in the sample plot and the corresponding sample plot with 11 years of afforestation. The content of the TP is high in the sample plots of 15, 31, and 5 years of afforestation. The soil TP content of the afforestation sample plots in these 3 years increases more than that of the sample plots in the same natural state. The content of TK is 2 years > 15 years > 31 years > 11 years > 5 years of afforestation. No obvious law exists between the content of soil TK and the afforestation time of the sample plot. The content of nitrogen was the highest in 31 and 15 years of afforestation. The content is low in the remaining years. The 31, 15, and 5 years of afforestation increase compared with the corresponding natural plots. Afforestation decreases after 11 and 2 years. The content of phosphorus is the highest in the sample plot of 15 years of afforestation. This content greatly increases in the afforestation time and the corresponding natural plot. The content and variation of phosphorus are low in other sample plots.
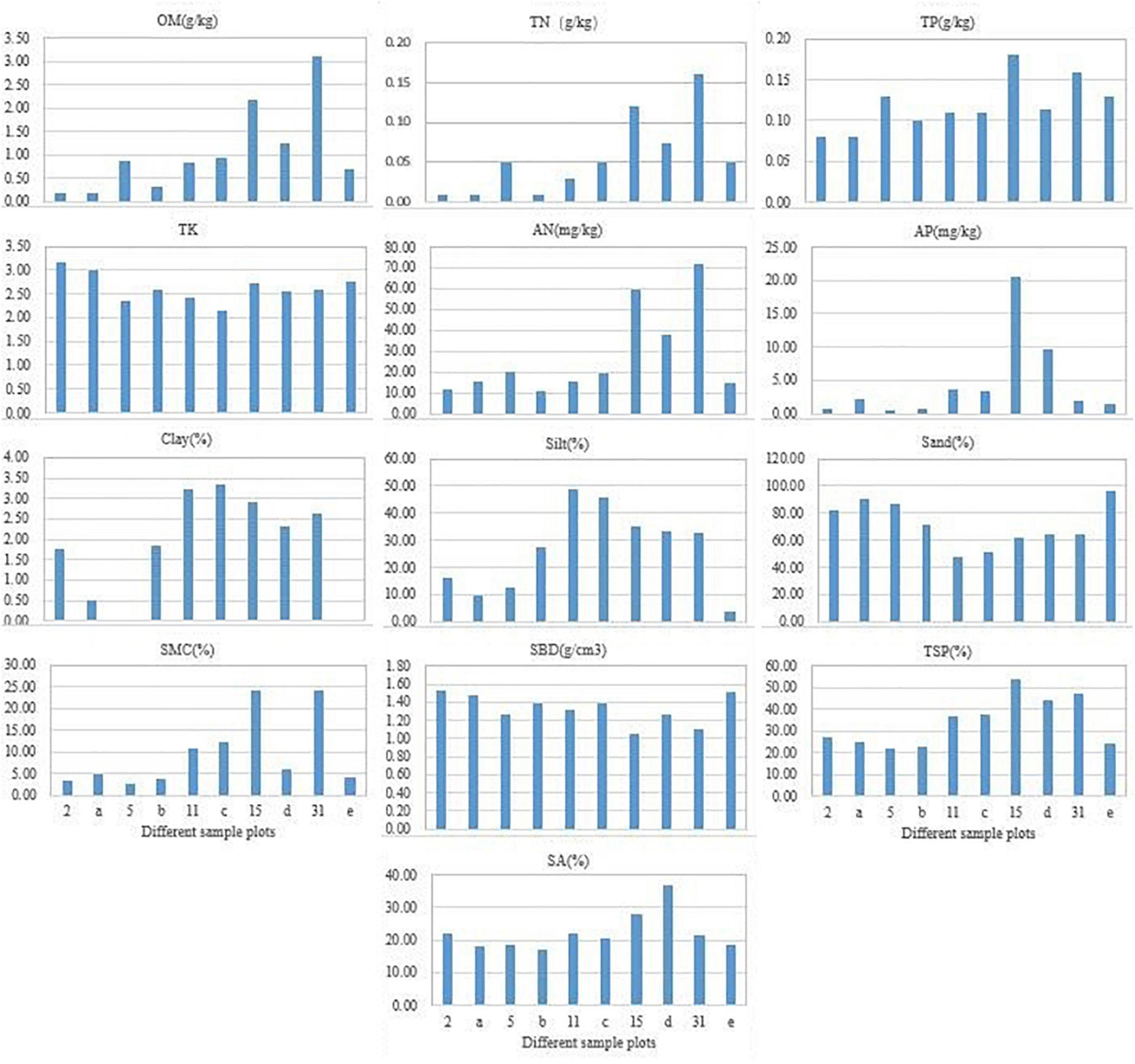
Figure 3. Statistical chart of the soil physical and chemical index characteristics of the different afforestation years. OM, soil organic matter (g/kg); TN, total nitrogen (g/kg); TP, total phosphorus (g/kg); TK, total potassium (g/kg); AP, available phosphorus (mg/kg); AN, alkaline hydrolyzed nitrogen (mg/kg); clay is clay (%); silt powder (%); sand is sand grains (%); SMC, soil water content (%); SBD, soil bulk density (g/cm3); TSP, soil total porosity (%); SA, soil aeration (%).
The clay content is 11 years > 15 years > 31 years > 2 years > 5 years. The clay content in the sample plots of 31, 2, and 15 years of afforestation is higher than that in the corresponding natural sample plots in the same region. The 5 and 11 years of afforestation plots are lower than the corresponding natural plots. Given that the clay content of the 5 years of afforestation sample plot is zero, it has a large decrease compared with the natural sample plot in the same region. The silt content from low to high was 5, 2, 31, 15, and 11 years. Except for the 5 years of the afforestation sample plot, the silt content of the rest of the afforestation sample plot is increased compared with the corresponding natural sample plot. With regard to the index of sand grain, the comparison between the afforestation sample plot and the corresponding natural sample plot is just opposite to that of silt grain. The sand content from low to high is afforestation for 11, 15, 31, 2, and 5 years. Meanwhile, the soil water content from high to low is afforestation for 31, 15, 11, 2, and 5 years. The water content basically increases with the increase in afforestation years. In comparison with the corresponding natural sample plots, a significant increase was observed in 15 and 31 years. The remaining afforestation years are reduced. The SBD from low to high is 15, 31, 5, 11, and 2 years. The SBD of the sample plots planted for 15, 31, 5, and 11 years is significantly reduced compared with the corresponding natural sample plots. The sample plot of afforestation for 2 years is slightly higher than the corresponding natural sample plot. Soil porosity from high to low is 15, 31, 11, 2, and 5 years. Afforestation in 31 and 15 years has significantly increased compared with the corresponding natural sample plots. The remaining plots slightly changed. SA from high to low is 15, 11, 2, 31, and 5 years. The SA significantly decreased compared with the corresponding natural sample plots after 15 years of afforestation. The remaining years are increased, but it is not significant.
The differences of the soil physical and chemical indexes among sample plots with different afforestation years are analyzed. The sample plots with different years demonstrated significant differences in organic matter (P < 0.05). Significant differences in soil organic matter are also observed between 31 and 15 year sample plots, between 15 year sample plots and 11 and 5 year sample plots, and between 2 year sample plots and 5 and 11 year sample plots. The mean value of the soil organic matter varies from the minimum value (0.18 ± 0.02) to the maximum value (3.10 ± 0.09). Significant differences in TN content in different years are also noticeable. No significant difference was evident between 11 and 5 years of afforestation. However, significant differences can be observed among the other different afforestation years. The mean value of TN varies from the minimum value (0.01 ± 0.00) to the maximum value (0.16 ± 0.02). Significant differences in TP can be observed among different sites. The mean value of the TP varied from the minimum value (0.08 ± 0.01) to the maximum value (0.16 ± 0.00).
In comparison with the difference of TK among different plots, significant differences can be observed in the soil TK content between 2 and 11 year sample plot. The mean value of the TP varied from the minimum value (2.13 ± 0.13) to the maximum value (3.16 ± 0.12). Varying degrees of significant differences can be observed between the sample plots of different years compared with the nitrogen in the sample plots of different years. The mean value varied from the minimum value (11.14 ± 1.21) to the maximum value (72.09 ± 9.38). Varying degrees of significant differences between the sample plots of different years can be observed compared with the soil AP in the sample plots of different years. The mean value of AP varies from the minimum value (0.6 ± 0.14) to the maximum value (20.52 ± 1.20).
Different degrees of significant differences can be observed between the sample plots of different years compared with the soil clay content in the sample plots of different years. The mean value of clay varies from the minimum value (0.00 ± 0.00) to the maximum value (3.35 ± 0.24). Different degrees of significant differences can be observed between the sample plots of different years compared with the soil silt content in the sample plots of different years. The mean value of powder varies from the minimum value (3.86 ± 0.93) to the maximum value (48.99 ± 12.14). Different degrees of significant differences can be observed between the sample plots of different years compared with the soil sand content in the sample plots of different years. The mean value of sand varies from the minimum value (47.77 ± 13.16) to the maximum value (96.13 ± 20.97).
Different degrees of significant differences can be observed between the sample plots of different years compared with the soil water content in the sample plots of different years. Significant difference in soil water content can be observed between 11 year sample plots and 5, and 2, year sample plots. The mean value of the soil water content varies from the minimum value (2.55 ± 1.09) to the maximum value (24.3 ± 1.09). Different degrees of significant differences can be observed between the sample plots of different years compared with the SBD in the sample plots of different years. Significant differences can be observed in SBD between 2 year sample plots and 11, year sample plots. The mean value of SBD varies from the minimum value (1.1 ± 0.05) to the maximum value (1.53 ± 0.06). Different degrees of significant differences can be observed between the sample plots of different years compared with the soil porosity in the sample plots of different years. Significant differences in soil porosity are evident between 5 and 2 year sample plots. The mean value of soil porosity varies from the minimum value (21.9 ± 3.05) to the maximum value (53.88 ± 1.57). Significant differences in different degrees can be observed among the sample plots with different years compared with the SA in the sample plots with different years. The mean value of SA varied from the minimum value (17.39 ± 0.64) to the maximum value (37.04 ± 4.37).
We determined the soil nutrient level of the soil chemical property indexes in the sample plots with different afforestation years according to the soil nutrient classification standard of the second National Soil Fertility Survey. Organic matter, TN, TP, and TK are all grade 6 among different afforestation sample plots. The content of alkali-hydrolyzable nitrogen was the highest in the sample plot with 31 years of afforestation, and the nutrient grade was grade 4. The alkali-hydrolyzable nitrogen grade with 15 years of afforestation is grade 5, and the nutrient grade in the other sample plots is grade 6. The AP was the highest in the sample plot with 15 years of afforestation, and the nutrient grade was grade II. The AP in the sample plots with 11 years of afforestation is grade 5, and the nutrient grade in the sample plots of other years is grade 6. The soil nutrient grade of the experimental plot in the study area is low, and the soil is poor.
Figure 4 shows the changes of the soil physical and chemical indexes of the sample plots with different afforestation years and their control plots. The change between the sample plot of 31 years of afforestation and the corresponding natural sample plot is the largest. The indicators with obvious reduction are sand particle and SBD, which are inverse indexes. The reduction of these two indicators has a positive effect on improving soil quality. In the positive index, except for the index of TK, the amount of change increased. Sand content, SBD, and SA decreased in the 15 year afforestation plots compared with the corresponding natural plots, while the mean values of other indices increased. The changes of the soil physical and chemical indexes are small in 11 years of afforestation plots and the corresponding natural plots. TP, TK, AP, silt, and SA increased in different degrees. The main chemical properties of the plots are increased compared with the corresponding natural plots, except for TK. The changes of some physical property indexes have a negative effect on the improvement of soil quality. For example, the specific gravity of clay and silt decreases, the specific gravity of sand increases, and the soil water content decreases. The decrease in SBD and the increase in SA play a positive role in the improvement of soil quality. The AP and nitrogen in the soil chemical indexes significantly decreased compared with the corresponding natural sample plots. TP slightly decreased. TK slightly increased. The remaining indicators remained basically unchanged. The particle size composition changes in a positive direction, in which the specific gravity of clay and powder increases, and that of sand decreases. Among the soil physical indexes, SA and soil porosity are increased. The decrease in soil water content and the increase in SBD are not conducive to the soil quality improvement.
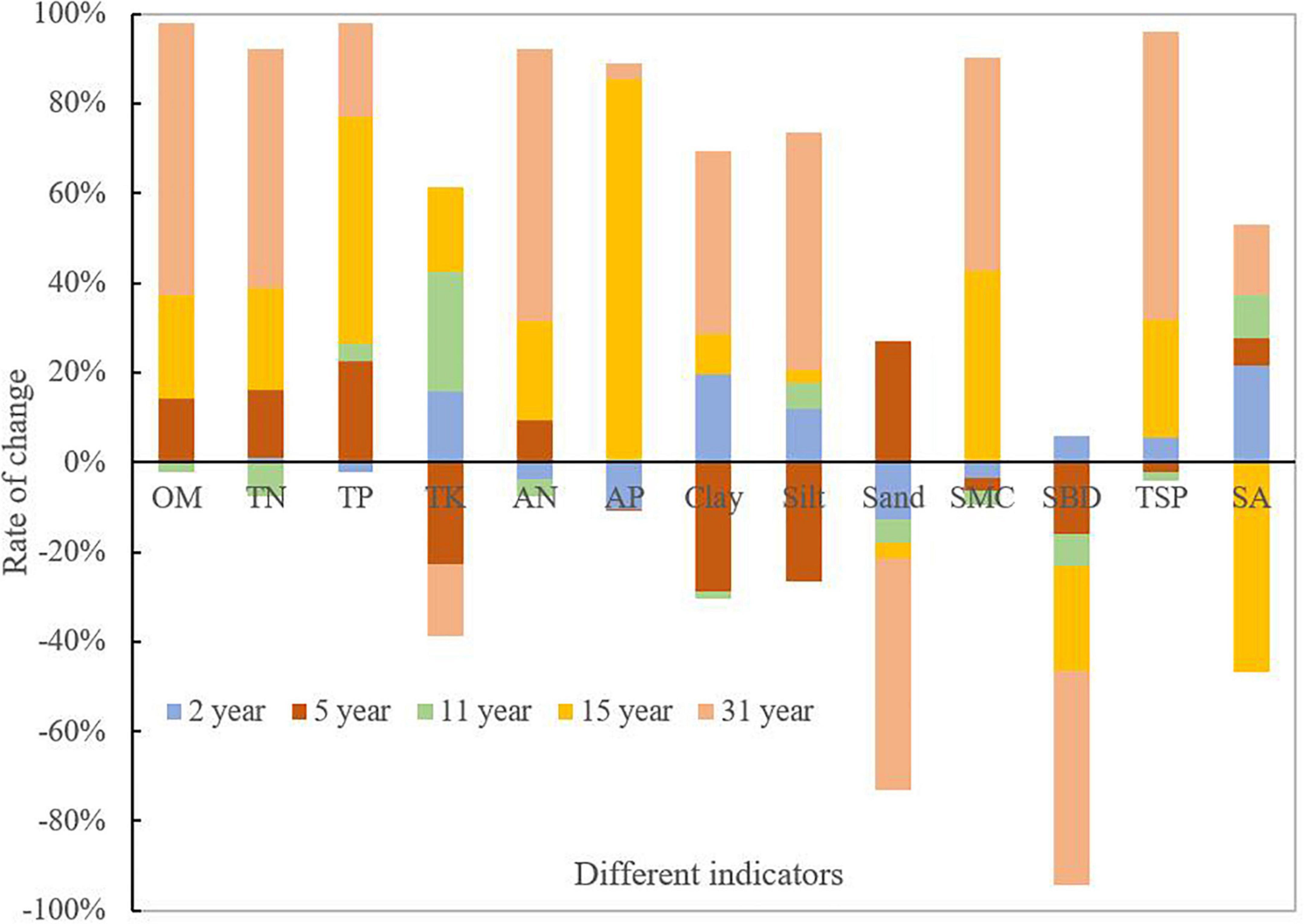
Figure 4. Changes of the soil physical and chemical properties in different years of afforestation and natural state plots in the same region.
Bullseye degree of soil quality by gray target model
The objective weight of each evaluation index is calculated by critical method. The objective weight of each index and the ratio of each index weight to the sum of all weights are shown in Figure 5. The top five indicators with high weight are AP, silt, soil water content, nitrogen, and TN. The five indexes with lower weight are TP, TK, soil porosity, SBD, and clay. The weight of the chemical property index is slightly higher than that of the physical property index. The contrast intensity and conflict between different indexes are expressed by standard deviation and correlation coefficient according to the calculation principle of the critical method, respectively. If the correlation between different indicators is stronger, and the conflict is smaller, then the weight is lower. If the standard deviation of an index is larger, then the weight is higher. This study uses the mode matrix after gray target transformation to calculate the standard deviation, considering the various measurement units of different physical and chemical properties. According to the correlation analysis table between different indicators, organic matter, TN, TP, and SBD show a strong correlation with other indicators. Based on the analysis of the standard deviation of different indexes, the standard deviation of soil water content, TN, nitrogen, AP, and organic matter is large.
The improved gray target model was used to estimate the soil quality results of different sites, as shown in Figure 6. The basic idea of the gray target model is to compare the evaluation item with the standard model (target center) and identify the degree of the evaluation item close to the target center. According to the analysis results of the gray target model, the value range of target degree γ of soil quality in different forestation years in this study is 0.42–0.75. The soil quality in the study area was divided into five levels according to the principle of equal division and integration. The classification here is only for the grading of the soil quality target degree of the sample plots involved in this study and represents the grading of soil quality relative to the sample plots in this study. The value of target degree of soil quality at all levels and the proportion of sample land are shown in Table 2.
Table 2 shows that about 63.33% of the sample plots in this study have a grade V bullseye degree. The soil fertility is low, and the bullseye degree of about 16.67% of the sample plots is grade IV. The soil quality slightly improved, and the bullseye degree of about 20% of the sample plots is grade I in the sample plots of 15 and 31 years of afforestation. Figure 5 shows that the soil quality bullseye degree of the sample plot after 15 years of afforestation is the largest (0.7153 ± 0.0244), followed by the bullseye degree after 31 years of afforestation (0.6923 ± 0.0020). The bullseye degree (0.4400 ± 0.0029) of soil quality in the sample plot of afforestation for 2 years is the lowest. The soil quality bullseye degree (0.4738 ± 0.0188) of 11 years of afforestation sample plot is slightly higher than that of 5 years of afforestation sample plot (0.4431 ± 0.0012). The bullseye degree of soil quality increases with the increase in afforestation years. Based on the variation coefficient of soil quality bullseye degree in different afforestation years, afforestation for 5 years (0.26%) < afforestation for 31 years (0.28%) < afforestation for 2 years (0.65%) < afforestation for 15 years (3.41%) < afforestation for 11 years (3.96%). The coefficient of variation of SQI of different afforestation years are all less than 10%, which belonged to the low variation level. However, different soil quality targets are related to the various site and background conditions of the sample plots. The variation of the target degree of soil quality between the 31 years of afforestation plots and the natural plots in the same region was about 0.208, which was the largest, compared with the corresponding natural state plots in the same region. The change of the soil quality target degree is about 0.184, followed by the change of the soil quality target degree. The minimum change of the soil quality target degree between 5 years of afforestation plots and the corresponding plots was about 0.019. The change of the soil quality target degree between 11 years of afforestation sample plots and the corresponding natural plots is the smallest, approximately 0.006. The change of the soil quality target degree between 2 years of afforestation sample plots and the corresponding plots was negative, which is related to the background condition and afforestation years of the plots. The soil quality shows a rising trend with the increase in afforestation years.
Evaluation of soil quality based on the full data set
Principal component analysis is used to determine the weights of the soil physical and chemical indexes in this study (Table 3). The test value of KMO in this study is 0.757, which conforms to the principal component analysis. Principal component analysis is used to determine the weight of each index. The SQI of the different forestation years and the corresponding natural state sample plots is calculated according to the determination method of membership value of different soil indexes introduced above. The SQI of the plots with different forestation years and the corresponding plots with natural state is shown in Figure 7.
The SQI can reflect the quality of soil in different afforestation areas. The SQI of the different afforestation years and the corresponding natural state was analyzed. The order of SQI was SQI15 (0.709) > SQI31 (0.662) > SQI11 (0.426) > SQI2 (0.348) > SQI5 (0.309). The SQI increased with the increase in forestation years. However, the SQI of the 15 years of afforestation plot was higher than that of the 31 years of afforestation plot. The SQI of the 2 years of afforestation plot is greater than 5 years. In comparison with the natural plots in the same region, the SQIs of the plots with different forestation years were as follows: afforestation for 31 years (0.31) > afforestation for 15 years (0.19) > afforestation for 2 years (0.03) > afforestation for 5 years (0.00) > afforestation for 11 years (−0.01). The variation coefficient of the SQI in different forestation years was 1.68% (2 years) < 11 years (1.89%) < 31 years (2.88%) < 15 years (3.62%) < 5 years (7.36%). The coefficient of variation of the SQI of different afforestation years were all less than 10%, which belonged to low variation level.
The classification of SQI was represented by a box diagram (Figure 8). “Box graph” is a statistical graph drawn by “quartile” method, which can be used to display data dispersion and compare the distribution characteristics of multiple groups of data. The characteristics of the SQI of the different afforestation years and the corresponding natural plots were analyzed. According to the box plot of SQI, the SQI of the different afforestation years and natural plots in the study area can be divided into four grades (grades 1, 2, 3, and 4). The range of the SQI of grade 1 is 0.29–0.34, grade 2 is 0.34–0.43, grade 3 is 0.43–0.67, and grade 4 is 0.67–0.74. The SQI ranges from 0.30 to 0.32 in grade 1, 0.32 to 0.35 in grade 2, 0.35 to 0.44 in grade 3, and 0.44 to 0.53 in grade 4. The soil quality of the sample plots with different afforestation years and natural sample plots in the study area are classified according to this classification method. The SQI grade of the sample plot for 15 years of afforestation is the highest grade 4, and the soil quality is good.
The soil fertility grade of the 15 years of afforestation sample plot and the natural sample plot in the same area is also the highest grade 4. However, the SQI of the natural sample plot is lower than that of the afforestation sample plot. The SQI grade of the sample plot for 31 years of afforestation is grade 3, and the soil quality is good. The soil fertility grade of the 31 years of afforestation sample plot and the natural sample plot in the same area is also grade 3. The SQI of the natural sample plot is lower than that of afforestation sample plot. The SQI grade of the sample plot for 11 years of afforestation is grade 2, and the soil quality is good. The soil fertility grade of the natural sample plots in the same area is also grade 3. The SQI of the afforestation sample plot and natural sample plot in this area is equal. The soil fertility of the 5 year sample system is in the corresponding low level. The soil quality of the sample plots with two afforestation years has little improvement compared with the corresponding natural sample plots. The classification method of the SQI here is different from that of soil quality bullseye degree in Table 2. The “quartile” method is adopted here, and the grading of bullseye degree adopts the division method of equal division and rounding. The value range of each level and the relative level of soil quality expressed are different.
Comparison between gray target model and full data set
The full data set of SQIs can generally make a comprehensive and accurate evaluation of the soil quality. The gray target model combines all models with the standard model to form gray targets and compares the models to be evaluated with the standard model. The aim is to identify the degree to which the model is close to the center of the target, that is, the degree of the target. Pattern recognition, classification, and optimization are carried out based on the bullseye degree. The results of the soil quality evaluation based on the improved gray target model showed that the range of soil quality target degree varied from 0.4211 to 0.7496 in the study area. The mean value was 0.5113 ± 0.1014, and the coefficient of variation was 19.83%. The range of soil quality index SQI-TDS based on the full dataset ranged from 0.2850 to 0.7402. The mean value was 0.4384 ± 0.1403, and the coefficient of variation was 32.03%, both of which belong to moderate variation level (Zhang, 2005; Guan et al., 2012). The coefficient of variation of soil index in the whole dataset is greater than that based on the gray target model. This finding indicates that the spatial variability of soil quality in the study area is small. Except for 11 years of afforestation, the coefficient of variation of SQI calculated by the full dataset method was greater than the gray target degree calculated by the gray target model method. In Figure 9, the changes of the soil quality curves in the study area under the two methods are consistent. A comparative analysis of the two soil quality evaluation methods shows that the correlation coefficient of the two evaluation results was 0.957, showing a significant positive correlation. The results show that the two methods of soil quality evaluation had good consistency and verified their accuracy.
Discussion
Effectiveness of criteria importance through intercriteria correlation method and the gray target model
The CRITIC method is mainly used to determine the weight of indexes based on the comparative strength of the evaluation index and the conflict between the index to measure the objective weight of the index (Diakoulaki et al., 1995; Abdel-Basset and Mohamed, 2019). The CRITIC is applied when the indexes are correlated and is an effective method to assess the convergent validity of indexes that are related (Diakoulaki et al., 1995). In the CRITIC method, the smaller the conflict between indexes is, the smaller the weight is. The qualitative indexes can be transformed into quantitative indexes without the independence of attributes (Alinezhad and Khalili, 2019). This approach showed a better objective weight assignment method than entropy weight and standard deviation methods (Kumaraswamy and Ramaswamy, 2016).
The gray target model is one of the more acceptable methods for soil quality assessment based on gray system theory (Zhu and Hipel, 2012). According to the similarity or dissimilarity of the development trend among factors, the method of gray correlation degree analysis for each subsystem can explore the numerical relationship between subsystems in the system through a certain method (Du et al., 2021). Based on gray relational analysis, pattern recognition, classification, and optimization of the system to be evaluated are carried out to determine the evaluation level (Shi and Li, 2009). In the evaluation of soil quality, the relative levels of soil quality in different regions are compared in the study area, but the traditional gray target model is difficult to solve the weight assignment issue (Deng, 2002). In this study, CRITIC method is introduced into the traditional gray target model. The improved gray target model based on CRITIC weight is established to add the weight of each index to the model, which improves the accuracy and objectivity of the evaluation results. The soil quality results obtained by the improved gray target model and the full data set method have good consistency and can verify their accuracy. This result is consistent with other research findings (Liu et al., 2018).
In this study, The AP weight value calculated by the CRITIC weight method greatly reduced, while the weight of the clay particles, sand, SBD, and TSP greatly increased. Meanwhile, the weight of soil water content and SA greatly decreased. This implies that the soil nutrient level of the afforestation plots in the study area is low and the soil is relatively poor (Rahmanipour et al., 2014). According to the bullseye degree of soil quality, about 63.33% of the sample plots in this study have a grade 5 bullseye degree, and about 16.67% of the sample plots is grade 4. The soil quality improved slightly improved, and the bullseye degree of about 20% of the sample plots is grade 1 in the 15 and 31 years of afforestation. This shows that the amount of soil change is relatively slow in different afforestation years. Therefore, the ecological environment in the study area is poor and the restoration period is relatively long once damaged (Gao et al., 2010; Wen et al., 2013; Zhang et al., 2019).
Main factors affecting the soil quality of different afforestation timescales
The SQI of the sample plots with different afforestation years increased with the increase in afforestation years. The sample plot of 15 years of afforestation belongs to the abandoned farmland before afforestation, and the soil quality is better than that of other afforestation sites. In comparison with the control farmland in the nearby area, the changes in the soil physical and chemical properties shows that the change of each index has greatly improved the soil quality (Li X. et al., 2021). The sample plot of 11 years of afforestation is an area with gravel coverage and no vegetation before afforestation. The opposite land is the grassland with good vegetation coverage nearby. Accordingly, the change of soil indexes in 11 years of afforestation is small. Thus, afforestation will change the physical and chemical properties of soil better.
According to the comparison and analysis of the bullseye degree of soil quality with different physical and chemical properties, the bullseye degree of the soil chemical properties is greater than that of physical properties in most of the samples in this study area. The proportion of such plots is greater than 90%. A total of 13 indexes are selected in this study, including six chemical indexes and seven physical indexes. This finding indicates that the chemical properties play a major role in the evaluation of soil quality (Li X. et al., 2021). The soil quality improvement is mainly caused by chemical properties. This notion provides a basis for the selection of soil quality evaluation indexes in future soil quality evaluation research. Bünemann et al. (2018) made a statistical analysis of 62 published papers on soil quality evaluation and showed that the physical indexes with the highest frequency were all related to soil water holding capacity, such as soil capillary porosity and non-capillary porosity. Total soil porosity was selected in this study. This index reflects the soil structure and affects the stability of soil aggregates, soil water holding capacity, soil moisture, plant growth, and the ecological function of soil (Zha and Tang, 2003).
According to statistics, more than 90% of soil quality research papers are related to soil organic matter. Soil organic matter was also selected as the evaluation index of soil quality in this study. Soil organic matter controls soil gas exchange and water relationship by affecting the soil aggregate stability and soil porosity. Moreover, soil organic matter plays an important role in soil carbon cycling and nutrient utilization (Schoenholtz et al., 2000). In this study, among the 13 soil physical and chemical indicators in soil quality evaluation, CRITIC weight shows the AP, Silt, SMC, AN, TN, and OM are the main factors affecting soil quality. This result also supports the preliminary research finding (Schoenholtz et al., 2000; Zha and Tang, 2003).
The sample plot of afforestation for 5 years is afforestation in the Piedmont conglomerate desert area. A little difference in site conditions of the natural sample plots in the same region can be observed. However, the contents of soil organic matter, TN, TP, and nitrogen are increasing. The soil physical properties are also getting better. This improvement leads to the variation of the soil physical and chemical properties. In the area where afforestation has been carried out for 2 years, the chemical properties of the soil are basically unchanged even though sprinkler irrigation measures are taken. The soil physical properties did not return to the level of soil physical properties before afforestation. Consequently, the physical and chemical properties of soil after afforestation take 2–5 years to restore.
Due to the particularity of the geographical location and the fragility of the natural environment and the short period of soil development, the soil degradation of the Qinghai-Tibet Plateau is serious. Some studies have pointed out that there are different degrees of permafrost degradation, vegetation degradation, soil degradation, desertification, grassland degradation, soil erosion, salinization, freeze-thaw erosion and other land degradation phenomena on the Qinghai-Tibet Plateau (Chen et al., 2013; Wu et al., 2015). Some studies have found that land degradation will affect the physical and chemical properties of soil, and then affect the hydraulic characteristics and water transport status of soil (He et al., 2003; Wang et al., 2007, Graves et al., 2015). Most existing soil quality evaluation studies chose soil physical and chemical indicators. The biological indicators were not considered in this study due to low impact of soil microorganisms in the study area (Li S. et al., 2021; Li X. et al., 2021). In spite of this, the role of biological indicators in the assessment of soil quality can’t be ignored. The application of the soil biological index to evaluate the soil quality is the development direction in the future. In addition, soil quality assessment is mainly focused on the specific points and regions, and there are few researches on large-scale soil quality. Meanwhile, the inadequate soil samples also introduce uncertainty in soil quality assessment. It is still an important problem to be solved in current research to extend the research results of soil quality from small scale to large-scale research under the numbers of samples.
Conclusion
Based on the soil samples collected from different afforestation years of 2, 5, 11, 15, and 31 years and natural sample plots with similar site conditions in the same region, the gray target model and CRITIC method are used to evaluate the soil quality of the sample plots with different afforestation years and the corresponding natural sample plots on the Tibet Plateau. The results show that:
(1) The most positive soil physical and chemical indexes increase with the increase in afforestation years. The contents of SOM, TN, and nitrogen increased with the increase in the afforestation years, except for the sample plot of 11 years. The content of most soil physical and chemical indexes is greater than that in the corresponding natural sample plots in 31 years of afforestation.
(2) The target degree of the soil quality in the study area is divided into five grades according to the principle of equal division and integration. The soil quality grade of the corresponding sample plot after 15 years of afforestation is grade 4. The other plots are grade 5.
(3) The SQI generally increases with the increase of in forestation years. The different afforestation years indicate SQI (0.709) of 15 years > SQI (0.662) of 31 years > SQI (0.426) of 11 years > SQI (0.348) of 2 years > SQI (0.309) of 5 years. The full data set method verifies the effectiveness of the gray target model. The two soil quality evaluation methodologies are consistent and can verify each other’s accuracy. The correlation coefficient of the two results reaches 0.957.
(4) The long-term afforestation timescales have improved the soil quality of watershed valley. Available phosphorus, silt, soil water content are the main factors impacting the soil quality in the afforestation timescales.
Data availability statement
The original contributions presented in this study are included in the article/supplementary material, further inquiries can be directed to the corresponding authors.
Author contributions
HY: conceptualization. BY: data curation. LJ and JX: formal analysis and writing—review and editing. ZZ: methodology. XC: resources. JX: supervision. JH and ZX: validation. HY and SW: writing—original draft. All authors have read and agreed to the published version of the manuscript.
Funding
This work was financially supported by the Second Tibetan Plateau Scientific Expedition and Research Program (STEP) (2019QZKK0405), the Study on the Support System of Modern Forestry Technology in Tibet (2018xz503118002), the Original Innovation Project of the Basic Frontier Scientific Research Program, Chinese Academy of Sciences (ZDBS-LY-DQC031), the National Natural Science Foundation of China (42071259), the Natural Science Foundation of Xinjiang Uygur Autonomous Region (2021D01E01), the Third Batch of Tianshan Talents Program of Xinjiang Uygur Autonomous Region (2021-2023), the Youth Innovation Promotion Association of the Chinese Academy of Sciences (2019430), the Investigation on Resource Environment and Biodiversity of Typical Mountainous Areas in Different Climatic Zones (2019FY101601-2), and the Study on the Model of Planting and Breeding Circular Agriculture in Tibet.
Conflict of interest
The authors declare that the research was conducted in the absence of any commercial or financial relationships that could be construed as a potential conflict of interest.
Publisher’s note
All claims expressed in this article are solely those of the authors and do not necessarily represent those of their affiliated organizations, or those of the publisher, the editors and the reviewers. Any product that may be evaluated in this article, or claim that may be made by its manufacturer, is not guaranteed or endorsed by the publisher.
References
Abdel-Basset, M., and Mohamed, R. (2019). A novel plithogenic TOPSIS- CRITIC model for sustainable supply chain risk management. J. Clean. Prod. 247:119586. doi: 10.1016/j.jclepro.2019.119586
Alinezhad, A., and Khalili, J. (2019). New methods and applications in multiple attribute decision making (MADM). International series in operations research & management science, Vol. 277. Cham: Springer. doi: 10.1007/978-3-030-15009-9
Bastida, A. F., Zsolnay, A., Hernández, T., and García, C. (2008). Past, present and future of soil quality indices: A biological perspective. Geoderma 147, 159–171. doi: 10.1016/j.geoderma.2008.08.007
Brady, N. C., and Weil, R. R. (2007). The nature and properties of soils. Hoboken, NJ: Prentice Hall.
Bünemann, E. K., Bongiorno, G., Bai, Z., Creamer, R. E., and Brussaard, L. (2018). Soil quality: A critical review. Soil Biol. Biochem. 120, 105–125. doi: 10.1016/j.soilbio.2018.01.030
Cetin, M., and Jawed, A. A. (2022). Variation of Ba concentrations in some plants grown in Pakistan depending on traffic density. Biomass Conv. Bioref. (in press) 1–8.
Chen, F. Z., Ren, J., Liu, S. H., and Hu, K. L. (2021). Comprehensive assessment of soil fertility of cultivated land in southern Dongting Lake Basin based on minimum data set. Chin. J. Soil Sci. 52, 1348–1359. doi: 10.19336/j.cnki.trtb.2021040901
Chen, H., Zhu, Q., Peng, C., Wu, N., Wang, Y., Fang, X., et al. (2013). The impacts of climate change and human activities on biogeochemical cycles on the Qinghai-Tibetan Plateau. Glob. Change Biol. 19, 2940–2955. doi: 10.1111/gcb.12277
Chen, H. S., Liu, S. P., Yang, W. Q., and Liang, G. Q. (2022). Structure and diversity of bacterial community in rhizosphere soil of four dominant species along the bank of the lower reaches of Yarlung Zangbo River. Acta Ecol. Sin. 42, 1527–1537.
Deng, J. L. (2002). Basis of grey theory. Wuhan: Huazhong University of Science & Technology Press, 172–176.
Diakoulaki, D., Mavrotas, G., and Papayannakis, L. (1995). Determining objective weights in multiple criteria problems: The critic method. Comput. Oper. Res. 22, 763–770. doi: 10.1016/0305-0548(94)00059-h
Doran, J. W., Coleman, D. C., Bezdicek, D. F., Stewart, B. A., Doran, J. W., and Parkin, T. B. (1994). “Defining and assessing soil quality,” in Defining soil quality for a sustainable environment, eds J. W. Doran, D. C. Coleman, D. F. Bezdicek, and B. A. Stewart (Madison, WI: Soil Science Society of America. Publication Inc), 3–21. doi: 10.2136/sssaspecpub35.c1
Doran, J. W., Jones, A. J., Doran, J. W., and Parkin, T. B. (1996). “Quantitative indicators of soil quality: A minimum data set,” in Methods for assessing soil quality, SSSA special publication no. 49, ed. J. W. Doran (Madison, WI: Soil Science Society of America Special Publication), 25–37. doi: 10.2136/sssaspecpub49.c2
Du, J., Liu, S., and Liu, Y. (2021). Grey target negotiation consensus model based on super conflict equilibrium. Group Decis. Negot. 30, 915–944. doi: 10.1007/s10726-021-09742-1
Du, J., and Yang, Z. G. (2013). Tibet autonomous region county-level climate regionalization. Beijing: China Meteorological Press.
Fu, B. J., Liu, S. L., Chen, L. D., Lu, Y. H., and Qiiu, J. (2004). Soil quality regime in relation to land cover and slope position across a highly modified slope landscape. Ecol. Res. 19, 111–118. doi: 10.1111/j.1440-1703.2003.00614.x
Gao, Q., Wan, Y., Xu, H., Li, Y., Jiangcun, W., and Borjigidai, A. (2010). Alpine grassland degradation index and its response to recent climate variability in Northern Tibet, China. Quat. Int. 226, 143–150. doi: 10.1016/j.quaint.2009.10.035
Graves, A. R., Morris, J., Deeks, L. K., Rickson, R. J., Kibblewhite, M. G., Harris, J. A., et al. (2015). The total costs of soil degradation in England and Wales. Ecol. Econ. 119, 399–413. doi: 10.1016/j.ecolecon.2015.07.026
Guan, X. Y., Wang, S. L., Gao, Z. Y., Lv, Y., and Fu, X. J. (2012). Spatio-temporal variability of soil salinity and its relationship with the depth to groundwater in salinization irrigation district. Acta Ecol. Sin. 32, 1202–1210.
He, F. H., Huang, M. B., and Dang, T. T. (2003). Distribution characteristic of dried soil layer in Wangdonggou watershed in gully region of the Loess Plateau. J. Nat. Resour. 18, 30–36.
He, X. Y., Nie, Y., Wang, P., Yin, C., and Yu, L. (2022). Cultivated land quality evaluation method and demonstration based on improved Grey Target model. Acta Pedol. Sin. (in press). doi: 10.11766/trxb202109170501
Idowu, O. J., Es, H. M. V., Abawi, G. S., Wolfe, D. W., Ball, J. I., Gugino, B. K., et al. (2008). Farmer-oriented assessment of soil quality using field, laboratory, and VNIR spectroscopy methods. Plant Soil 307, 243–253. doi: 10.1007/s11104-007-9521-0
Kumaraswamy, M., and Ramaswamy, R. (2016). Performance evaluation of software projects using criteria importance through Inter-criteria correlation technique. Int. J. Soft Comput. Softw. Eng. 6, 28–36.
Lal, R. (2015). Restoring soil quality to mitigate soil degradation. Sustainability 7, 5875–5895. doi: 10.3390/su7055875
Lal, R. (2016). Soil health and carbon management. Food Energy Secur. 5, 212–222. doi: 10.1002/fes3.96
Larson, W. E., and Pierce, F. J. (1991). Conservation and enhancement of soil quality: Evaluation for sustainable land management in the developing world. Bangkok: International Board for Soil Research and Management Inc, 175–203.
Larson, W. E., and Pierce, F. J. (1994). The dynamics of soil quality as a measure of sustainable management. Soil Sci. 551, 37–51. doi: 10.2136/sssaspecpub35.c3
Lehmann, J., Bossio, D. A., Kögel-Knabner, I., and Rillig, M. C. (2020). The concept and future prospects of soil health. Nat. Rev. Earth Enviorn. 1, 544–553. doi: 10.1038/s43017-020-0080-8
Li, H., Shen, W., Zou, C., Jiang, J., Fu, L., and She, G. (2013). Spatio-temporal variability of soil moisture and its effect on vegetation in a desertified aeolian riparian ecotone on the Tibetan Plateau, China. J. Hydrol. 479, 215–225. doi: 10.1016/j.jhydrol.2012.12.002
Li, S., Du, J. K., Zhang, Z. W., and Xing, H. H. (2021). Risk regionalization for flood hazard in southern Shaanxi Province based on grey target model and GIS. Yangtze River 52, 17–21. doi: 10.16232/j.cnki.1001-4179.2021.07.003
Li, X., Zhang, W. J., Wu, L., Ren, Y., Zhang, J. D., and Xu, M. G. (2021). Advance in indicator screening and methodologies of soil quality evaluation. Sci. Agric. Sin. 54, 3043–3056. doi: 10.1001/jamaoncol.2021.6987
Liu, X., Wang, Y. B., and Lu, M. X. (2018). Soil quality assessment of alpine grassland in permafrost region of Tibetan Plateau based on principal component analysis. J. Glaciol. Geocryol. 40, 469–479. doi: 10.7522/j.issn.1000-0240.2018.0052
Liu, Z. F., Fu, B. J., Liu, G. H., and Zhu, Y. G. (2006). Soil quality: Concept, indicators and its assessment. Acta Ecol. Sin. 26, 276–288.
Mausel, P. W. (1971). Soil quality in Illinois-an example of a soils geography resource analysis. Prof. Geogr. 23, 127–136. doi: 10.1111/j.0033-0124.1971.00127.x
Mu, X., Kun, J., Zhao, W., Liu, S., Wei, X., and Wang, B. (2021). Spatio-temporal changes of ecological vulnerability across the Qinghai-Tibetan Plateau. Ecol. Indic. 123:107274. doi: 10.1016/j.ecolind.2020.107274
Pekkan, O. I., Senyel Kurkcuoglu, M. A., Cabuk, S. N., Aksoy, T., Yilmazel, B., Kucukpehlivan, T., et al. (2021). Assessing the effects of wind farms on soil organic carbon. Environ. Sci. Pollut. Res. 28, 18216–18233. doi: 10.1007/s11356-020-11777-x
Qin, Y., Wu, T., Wu, X., Li, R., Xie, C., Qiao, Y., et al. (2017). Assessment of reanalysis soil moisture products in the permafrost regions of the central of the Qinghai-Tibet Plateau. Hydrol. Process. 31, 4647–4659. doi: 10.1002/hyp.11383
Rahmanipour, F., Marzaioli, R., Bahrami, H. A., Fereidouni, Z., and Bandarabadi, S. R. (2014). Assessment of soil quality indices in agricultural lands of Qazvin Province, Iran. Ecol. Indic. 40, 19–26. doi: 10.1016/j.ecolind.2013.12.003
Rairsi, F. (2017). A minimum data set and soil quality index to quantify the effect of land use conversion on soil quality and degradation in native rang lands of upland arid and semiarid regions. Ecol. Indic. 75, 307–320. doi: 10.1016/j.ecolind.2016.12.049
Schoenholtz, S. H., Miegroet, H. V., and Burger, J. A. (2000). A review of chemical and physical properties as indicators of forest soil quality: Challenges and opportunities. For. Ecol. Manage. 138, 335–356. doi: 10.1016/s0378-1127(00)00423-0
Shi, H. W., and Li, W. Q. (2009). “The grey relational analysis on building construction duration cases,” in Proceedings of the 2009 international conference on future biomedical information engineering (FBIE), Sanya, 358–361. doi: 10.1109/fbie.2009.5405849
Smith, J. L., Halvorson, J. J., and Papendick, R. I. (1993). Using multiple-variable indicator kriging for evaluating soil quality. Soil Sci. Soc. Am. J. 57, 743–749. doi: 10.2136/sssaj1993.03615995005700030020x
Smith, P., Bustamante, M., House, J. I., Sobocká, J., Harper, R., Pan, G. X., et al. (2016). Global change pressures on soils from land use and management. Glob. Change Biol. 22, 1008–1028. doi: 10.1111/gcb.13068
Sun, Y., Wang, Y., Yang, W., Sun, Z., and Zhao, J. (2019). Variation in soil hydrological properties on shady and sunny slopes in the permafrost region, Qinghai-Tibetan plateau. Environ. Earth Sci. 78:100. doi: 10.1007/s12665-019-8067-9
Tan, J. J., Deng, J., Fan, J. R., Xu, J. H., and Deji, S. Z. (2019). Study on soil erosion classification index of small watershed in middle reaches of Yarlung Zangbo River. Soil Water Conserv. China 08, 56–59. doi: 10.14123/j.cnki.swcc.0219
Varol, T., Emir, T., Akgul, M., Ozel, H. B., Acar, H. H., and Cetin, M. (2020). Impacts of small-scale mechanized logging equipment on soil compaction in forests. J. Soil Sci. Plant Nutr. 20, 953–963. doi: 10.1007/s42729-020-00182-5
Wang, G., Wang, Y., Li, Y., and Cheng, H. (2007). Influences of alpine ecosystem responses to climatic change on soil properties on the Qinghai-Tibet Plateau, China. Catena 70, 506–514. doi: 10.1016/j.catena.2007.01.001
Wang, T., Si, W. T., Yan, R. Q., Li, H. D., Lin, N. F., and Shen, W. S. (2020). The effects of Hedysarum scoparium restoration on physical and chemical properties of sot in hillside shifting sandy land in middle reaches of Yarlung Zangbo River, China. J. Ecol. Rural Environ. 36, 1619–1625. doi: 10.19741/j.issn.1673-4831.2020.0371
Wang, X. Z., He, Q. R., Li, S., and Wei, X. H. (2009). Assessment of land degradation on Qinghai-Tebet Plateau. Res. Soil Water Conserv. 16, 14–18.
Wen, L., Dong, S., Li, Y., Wang, X., Li, X., Shi, J., et al. (2013). The impact of land degradation on the C pools in alpine grasslands of the Qinghai-Tibet Plateau. Plant Soil 368, 329–340. doi: 10.1007/s11104-012-1500-4
Wu, Q., Hou, Y., Yun, H., and Liu, Y. (2015). Changes in active-layer thickness and near-surface permafrost between 2002 and 2012 in alpine ecosystems, Qinghai-Xizang (Tibet) Plateau, China. Glob. Planet. Change 124, 149–155. doi: 10.1016/j.gloplacha.2014.09.002
Xie, X. F., Pu, L. J., Zhu, M., Wu, T., and Xu, Y. (2019). Assessment of soil quality in coastal tidal flat reclamation area based on MDS-TOPSIS model. Environ. Sci. 40, 5484–5492. doi: 10.13227/j.hjkx.201905129
Yang, X. Y., Fu, Q., Wang, B. H., and Jiang, Q. X. (2008). Construction and application of fuzzy neural networks model in evaluation on the soil quality changes of Sanjiang plain. Res. Soil Water Conserv. 15, 54–57. doi: 10.1109/WiCom.2008.2570
Yu, J., Fang, L., Cang, D., Zhu, L., and Bian, Z. (2012). Evaluation of land eco-security in Wanjiang district base on entropy weight and matter element model. Trans. Chin. Soc. Agric. Eng. 28, 260–266.
Zha, X. C., and Tang, K. L. (2003). Change about soil erosion and soil properties in reclaimed forestland of loess hilly region. Acta Geogr. Sin. 3, 464–469. doi: 10.11821/xb200303017
Zhang, W., Xue, X., and Peng, F. (2019). Meta-analysis of the effects of grassland degradation on plant and soil properties in the alpine meadows of the Qinghai-Tibetan Plateau. Glob. Ecol. Conserv. 20:e00774. doi: 10.1016/j.gecco.2019.e00774
Zhang, Z. W., Yin, H., Chang, J. J., and Xue, J. (2022). Spatial variability of surface soil water content and its influencing factors on shady and sunny slopes of an alpine meadow on the Qinghai–Tibetan Plateau. Glob. Ecol. Conserv. 34:e02035. doi: 10.1016/j.gecco.2022.e02035
Zhang, Z. W., Yin, H. Y., Zhao, Y., Wang, S. P., Han, J. H., Yu, B., et al. (2021). Spatial heterogeneity and driving factors of soil moisture in alpine desert using the geographical detector method. Water 13:2652. doi: 10.3390/w13192652
Zhu, J. J., and Hipel, K. W. (2012). Multiple stages grey target decision making method with incomplete weight based on multi-granularity linguistic label. Inform. Sci. 212, 15–32. doi: 10.1016/j.ins.2012.05.011
Zhu, M. M., Xu, J. H., Chen, G. Y., Li, P., Cheng, Q. M., and Chen, C. (2021). Assessment on soil quality under different land use patterns in karst area based on minimum data set. Acta Agrestia Sin. 29, 2323–2331. doi: 10.11733/j.issn.1007-0435.2021.10.024
Keywords: afforestation, soil quality, gray target model, CRITIC method, Tibet Plateau
Citation: Yin H, Yu B, Zhang Z, Jia L, Xue J, Chen X, Wang S, Han J and Xing Z (2022) Evaluating the impact of different afforestation timescales on the soil quality in a typical watershed valley using the criteria importance through intercriteria correlation method and gray target model. Front. For. Glob. Change 5:997949. doi: 10.3389/ffgc.2022.997949
Received: 19 July 2022; Accepted: 31 August 2022;
Published: 29 September 2022.
Edited by:
Mehrdad Zarafshar, Agricultural Research, Education and Extension Organization (AREEO), IranReviewed by:
Abolfazl Jaafari, Research Institute of Forests and Rangelands, IranYu Liu, Northwest A&F University, China
Elham Nouri, Agricultural Research, Education and Extension Organization (AREEO), Iran
Maryam Teimouri, Research Institute of Forests and Rangelands, Iran
Copyright © 2022 Yin, Yu, Zhang, Jia, Xue, Chen, Wang, Han and Xing. This is an open-access article distributed under the terms of the Creative Commons Attribution License (CC BY). The use, distribution or reproduction in other forums is permitted, provided the original author(s) and the copyright owner(s) are credited and that the original publication in this journal is cited, in accordance with accepted academic practice. No use, distribution or reproduction is permitted which does not comply with these terms.
*Correspondence: Lizhi Jia, amlhbGl6aGkxMDAwMEAxMjYuY29t; Jie Xue, eHVlamllMTFAbXMueGpiLmFjLmNu