- 1National Forestry and Grassland Administration Engineering Research Centre for Southwest Forest and Grassland Fire Ecological Prevention, College of Forestry, Sichuan Agricultural University, Chengdu, China
- 2National Forestry and Grassland Administration Key Laboratory of Forest Resources Conservation and Ecological Safety on the Upper Reaches of the Yangtze River and Forestry Ecological Engineering in the Upper Reaches of the Yangtze River Key Laboratory of Sichuan Province, Chengdu, China
- 3Southwest Survey and Planning Institute of National Forestry and Grassland Administration, Kunming, China
Pinus yunnanensis Franch. (Pinaceae) is an important endemic tree species that serves as a critical constituent of the forest ecosystems and plays a significant role in forestry economic production in southwest China. P. yunnanensis comprises three varieties: var. yunnanensis, var. pygmaea and var. tenuifolia, with significant variation in traits, such as height and leaf size. This study aims to characterize the habitat conditions of the three varieties and predict their potential future distributions by employing MaxEnt model. Temperature seasonality (BIO4) emerged as the most influential factor affecting the distribution of var. yunnanensis; isothermally (BIO3) stands out as the most critical factor for the distribution of var. pygmaea; whereas mean annual fire occurrence (MAF) had the greatest impact on the distribution of var. tenuifolia. Under future climate conditions, the highly and moderately suitable habitats for all the three varieties are projected to decrease, while the lowly suitable habitats are projected to increase. The distribution centroids of all the three varieties are anticipated to shift to higher latitudes. Our study characterized the habitat conditions and predicted the potential future distribution of the three Pinus yunnanensis varieties, which could help the conservation and utilization of Pinus yunnanensis varieties.
1 Introduction
Pinus yunnanensis Franch. (Pinaceae) is a unique species that only can be found in southwest China (Wang et al., 2013), but also one of the most widespread species in this region. The P. yunnanensis forest is highly regarded in forestry production due to its high resin content and wood quality. Furthermore, it plays a crucial role in the ecological development of the Yangtze and Jinsha River basins (Cai et al., 2016). There are three varieties (var. yunnanensis, var. pygmaea and var. tenuifolia) in P. yunnanensis, and the three varieties showed considerable variations in traits (Sun et al., 2020). For example, var. yunnanensis and var. tenuifolia are trees, generally can grow above 15 m, while var. pygmaea is a dwarf shrub that typically grows no taller than 2–3 meters, hence it is not suitable for timber production (Figure 1). The length of var. yunnanensis leaves usually ranges from 10 to 30 cm, while the var. pygmaea has shorter leaves ranging from 7 to 13 cm; var. tenuifolia has the longest leaves, which can reach up to 36 cm (Flora Reipublicae Popularis Sanicae: http://frps.iplant.cn/). P. yunnanensis varieties also exhibit great variation in habitat conditions: var. yunnanensis grows at altitudes of 1,000–3,200 m elevation and occupies diverse habitats; var. pygmaea usually grows on dry and barren, sunny upper slopes, at altitudes of 2,200–3,100 m elevation; while var. tenuifolia grows at altitudes of 400–1,200 m elevation, along riversides (Chen et al., 2021). Exploring the variations in traits and habitat conditions across the three varieties can provide insight into evolutionary process of P. yunnanensis. P. yunnanensis growing in different environments displays substantial variations in its growth, which is attributed to variations in precipitation, temperature, and elevation (Zhou et al., 2022). Therefore, the variations in environmental conditions significantly impact timber production of P. yunnanensis (Shen et al., 2020). However, the exactly variation of habitat conditions and distribution across the three varieties are still unclear. What environmental factors are important influencers on their geographic distribution, and how climate change will affect the distribution of P. yunnanensis, thus affecting timber production, also have been scarcely studied.
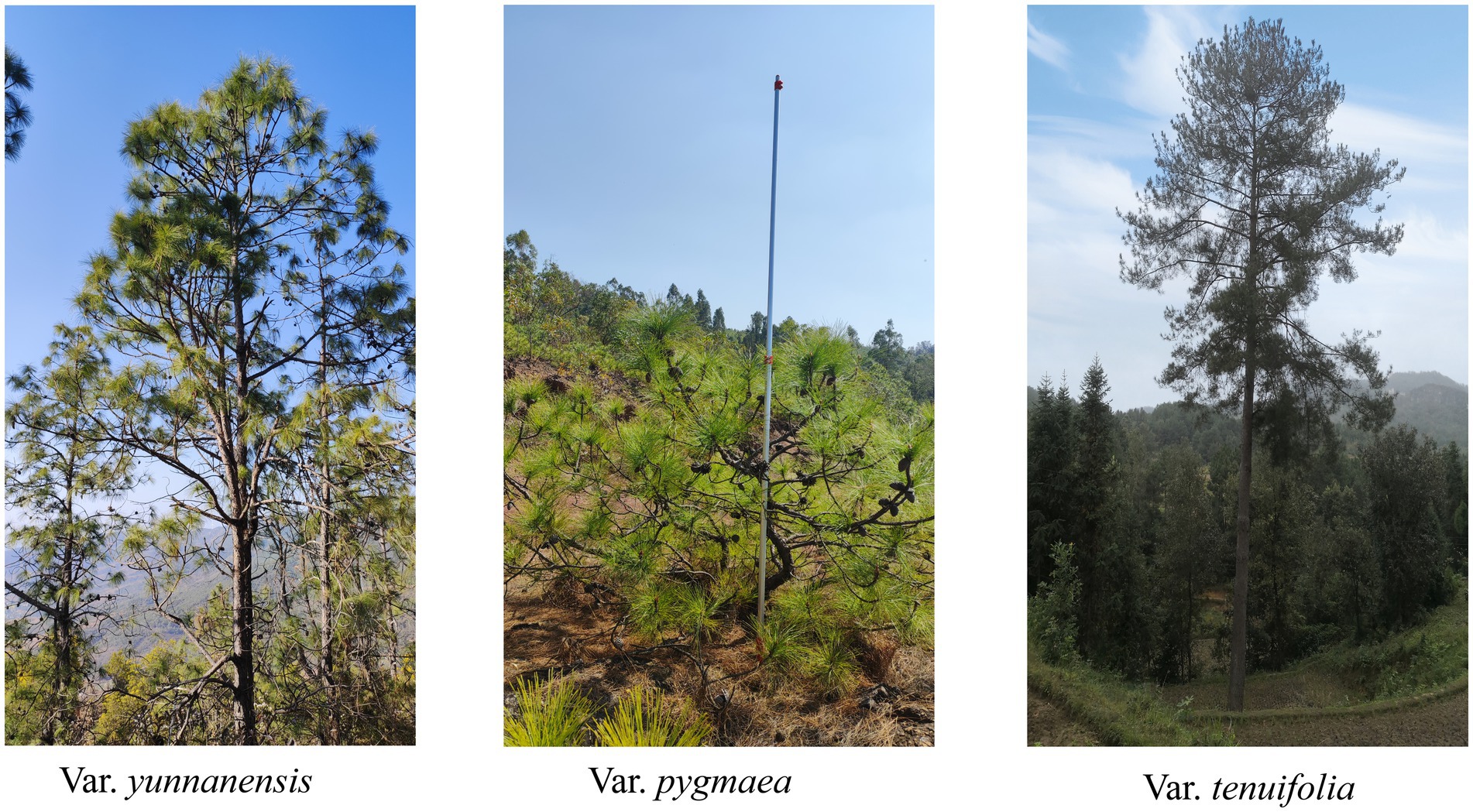
Figure 1. Photographs of var. yunnanensis, var. pygmaea and var. tenuifolia. The height of the stick next to var. pygmaea in the figure is 4.5 m.
Under climate changing, the risk of forest fires has become increasingly severe, posing a serious threat to global timber production (Bousfield et al., 2023). The southwestern region of China, where P. yunnanensis is situated, is prone to frequent wildfires. This has led to the development of varying adaptive strategies for dealing with different fire environments (Tang et al., 2013). Therefore, in addition to bioclimatic environmental variables, wildfires, as a significant environmental factor, may also influence the distribution of P. yunnanensis and its variants. Currently, there is limited research on this aspect, especially considering wildfires as an impacting variable. Consequently, gaining insights into the impacts of climate change and wildfires on the distribution of P. yunnanensis is paramount for formulating nuanced forest management policies aimed at the preservation and sustainable utilization of this species.
Climate change has great impacts on species distribution, ecosystem structure and biological community composition (Root et al., 2003; Huang et al., 2022; Ouyang et al., 2022). Many studies have showed that climate change will cause considerable changes in the distribution of plant species (Ji et al., 2020; Ab Lah et al., 2021; Zhang et al., 2021; Li et al., 2023). For example, Kalanchoe × houghtonii (Crassulaceae) is an invasive species that has rapidly expanded in recent years. To explore its distribution pattern, Herrando-Moraira et al. (2020) used the MaxEnt model to predict its future distribution and discussed the crucial variables influencing its distribution. Leucanthemum vulgare (ox-eye Daisy) is an invasive species with a wide global distribution, Ahmad et al. (2019) utilized species distribution models to predict the future distribution of Leucanthemum vulgare (ox-eye Daisy), enabling early detection of areas susceptible to invasion and preventing further spread of the species. Prediction of the potential distribution of regional species also been reported. For example, Ferreira et al. (2016) projected that 90% of the 206 local species on oceanic islands (Azores) would lose their climatic space in future. Similarly, Akebia trifoliata (Ranunculaceae) is a fruit found mainly in southern China, Zhang et al. (2022) employed the MaxEnt model to predict the distribution of wild Akebia trifoliata (Ranunculaceae), under different future climate scenarios and observed a shift of suitable habitat from south to north. Hu et al. (2019) examined the impact of climatic changes on the distribution patterns of six typical Kobresia forage species in the Tibetan Plateau, and found that climate warming would create unfavorable survival conditions for these species, resulting in their degradation. Predicting future distribution of plant species can facilitate understanding of the relationship between climate change and species distribution patterns, thereby guiding conservation measures.
Species distribution model (SDM) is developed on the basis of niche theory (Guisan and Thuiller, 2005), which is widely used to predict species distribution (Zhang et al., 2021; Li et al., 2022). It is a model that associates the distribution of the target species with the relevant environmental factors to calculate the ecological requirements for the distribution of the species through the algorithm, and to predict the potential distribution of the species in a certain area under specific space–time conditions (Elith et al., 2011; Dyderski et al., 2018). Currently, commonly used species distribution modeling (SDM) models include the bioclimatic model (BIOCLIM), ecological niche factor analysis model (ENFA), genetic algorithm for rule-set production model (GARP), and maximum entropy model (MaxEnt) (Tsoar et al., 2007; Bradie and Leung, 2017). The MaxEnt model offers several advantages, such as the ability to select control variables, estimate coefficients for environmental variables, and visualize analysis results effectively. Notably, the MaxEnt model incorporates a response curve function for each variable, providing a more intuitive representation of the threshold range of a single environmental factor’s impact on species distribution (Parveen et al., 2022). In addition, the MaxEnt model demonstrates high accuracy in predicting species distribution, particularly for species with small sample sizes and relatively concentrated distributions, thus making it as one of the most reliable SDM models (Wang et al., 2021; Huang et al., 2022).
Pinus yunnanensis Franch. (Pinaceae) is an important endemic tree species that serves as a critical constituent of the forest ecosystems and plays a significant role in forestry economic production in southwest China. However, the distribution of P. yunnanensis, especially the variation in distribution across its three varieties, has been scarcely studied. How the distribution of the three varieties will change under climate change is also unclear. In this study, we aim to predict both the present and future distribution patterns of P. yunnanensis varieties by employing the MaxEnt model, and to unravel the key environmental variables that exert significant influences on their distribution. The objectives of our research are: (1) To characterize the variation in the habitat conditions of the 3 P. yunnanensis varieties; (2) To identify the key environmental factors that has influenced the distribution of the 3 P. yunnanensis varieties; (3) To predict the current and future distribution areas of the 3 P. yunnanensis varieties (4) To elucidate the shifts of the distribution centers of the 3 P. yunnanensis varieties. We hope our study can facilitate the conservation and sustainable utilization of P. yunnanensis, and guide the management of P. yunnanensis forests.
2 Methods
2.1 Study area
This study was conducted in the southwestern region of China, including Sichuan, Yunnan, Guizhou, and Chongqing, Qinghai provinces and Tibet Autonomous Region, with a geographic range from 78°25′ to 112°08′ E longitude and 21°08′ to 36°19′ N latitude. Climate types in this region are diverse. For example, the Sichuan Basin has a mid-subtropical monsoon climate, the Yunnan-Guizhou Plateau has a south-central subtropical monsoon climate, and the mountains of the Qinghai-Tibet Plateau have a highland mountain climate. The average annual temperature in the southwest is about 16.5°C (Tang et al., 2021). The total annual precipitation in the southwest region is between 600 and 2,300 mm, mainly presenting a spatial distribution pattern of more precipitation in the east and less in the west, with more in the south and less in the north, accompanied by large differences in intra-annual distribution and distinct wet and dry seasons. Pinus yunnanenesis were mainly distributed in Yunnan-Guizhou Plateau, where has a subtropical monsoon climate with alternating warm dry season and hot wet seasons, creating conditions that favour wildfires (Wu et al., 2020).
2.2 Collection of occurrence records for the three Pinus yunnanensis varieties
Pinus yunnanensis Franch. (Pinaceae) is one of the most widespread indigenous tree species in south-west China (Chen et al., 2021). The occurrence records for P. yunnanensis varieties are mainly derived from China forest and grassland fire risk survey. A total of 681 occurrences with coordinate information (var. yunnanensis: 630; var. pygmaea: 32; var. tenuifolia: 19) were obtained (Supplementary Table S1; Figure 2). The occurrence records of the three varieties vary greatly, but the number of occurrence records for each variety is roughly correlated with its distribution range. Var. yunnanensis has the widest distribution, predominantly abundant in Yunnan and Sichuan, with scattered occurrences in Guangxi, Guizhou, Tibet. Var. pygmaea follows with a significantly smaller distribution, limited to the central part of Yunnan and southern Sichuan. Var. tenuifolia has the smallest distribution across the three varieties and exists only at the tri-provincial border of Guangxi, Guizhou, and Yunnan.
2.3 Environmental data collection and data analysis
Nineteen bioclimatic variables data and altitude data were obtained from the World Climate Database (WorldClim: https://www.worldclim.org/). Wildfire data were obtained from the moderate resolution imaging spectroradiometer (MODIS) products of The fire information for resource management system (FIRMS: https://firms.modaps.eosdis.nasa.gov/). The mean annual fire occurrence from 2001 to 2021 were extracted at a grid cell size of 1 km × 1 km. Soil data, including nitrogen content, organic carbon content, sandy soil content and soil silt content of 0-5 cm soil, were obtained from Soilgrids (https://soilgrids.org/) (Poggio et al., 2021). Finally, we gathered data for 25 environmental variables, all of which are at a resolution of 30′′ (Table 1). The variations in these variables among the three varieties were analyzed using a one-way analysis of variance in SPSS (IBM Corp, 2013). To assess whether there are significant differences in environmental variables among the three varieties statistically, the Least Significant Difference (LSD) method was employed to ascertain the significance of differences among these three varieties. For the prediction of further distribution of P. yunnanensis, we selected the global bioclimatic data under the shared socio-economic pathways SSP1-2.6 and SSP5-8.5 in the BCC-CSM2-MR atmospheric circulation model from 2040 to 2060 and 2060–2080 (Séférian et al., 2019). Because P. yunnanensis is mainly distributed in the southwest of China, the environment variables are cut to the southwest using ArcGIS10.8 and convert it to ASC II format that can be recognized by MaxEnt software.
2.4 MaxEnt model construction and result evaluation
We employed MaxEnt software (version 3.4.1) to develop the model, specifying a maximum of 500 iterations. We randomly assigned 25% of the species distribution points as the test dataset, while the remaining 75% were used as the training dataset. To assess the contribution and importance of each variable to the model construction, we conducted jackknife analysis (Fand et al., 2020; Baradevanal et al., 2023; Ullah et al., 2023). Additionally, we utilized the response curve function to determine the threshold range of the impact of individual environmental variables on the distribution of P. yunnanensis. Other model parameters were set to their default values, and the entire process was repeated 10 times. The final output of the model was imported into ArcGIS 10.8. To classify the distribution probability of the species, we employed the reclass tool to assign four grades ranging from 0 to 1: 0–0.1 represents unsuitable habitats, 0.1–0.4 represents lowly suitable habitats, 0.4–0.7 indicates moderately suitable habitats, and 0.7–1.0 represents highly suitable habitats.
The shift of distribution centers for the three varieties in future periods was analyzed by using the SDM toolbox, a Python-based tool for ArcGIS. After inputting files containing the model-predicted future species habitat and the current habitat, a vector file (line) is generated. This file illustrates the movement of the distribution center of each variety from the current center to a specific location in the future period.
The accuracy of the model is evaluated by Receiver Operating Characteristic (ROC) analysis (Merow et al., 2013). It is a method employed by MaxEnt software to assess model accuracy, with the Area Under the ROC Curve (AUC) serving as the evaluation metric (Phillips et al., 2006). One advantage is that the AUC provides a standardized measure of model performance, independent of the choice of any threshold. This metric is widely utilized in the evaluation of species distribution models (Phillips and Dudík, 2008). An AUC value exceeding 0.9 indicates excellent model accuracy, while an AUC above 0.8 suggests good model prediction. AUC values between 0.7 and 0.8 indicate moderate model accuracy. However, if the AUC value falls within the range of 0.5 to 0.6, the model’s predictions are considered to be unsuccessful. A flowchart of the methodology in this study was provide to illustrate the steps (Figure 3).
3 Results
3.1 Habitat conditions varied significantly across the three Pinus yunnanensis varieties
Among the three varieties of P. yunnanensis, significant differences in habitat conditions were observed across var. tenuifolia, var. yunnanensis and var. pygmaea (Table 2). Var. tenuifolia exhibited an average annual temperature of 17.9°C and an annual precipitation of 1262.1 mm, significantly higher than the other two varieties. Var. tenuifolia experienced a higher frequency of annual wildfires, while its elevation was significantly lower than that of var. yunnanensis and var. pygmaea. Based on the results, the habitat conditions of var. yunnanensis and var. pygmaea are generally consistent, with both species growing in higher elevations characterized by lower temperatures and precipitation. In contrast, var. tenuifolia thrives in lower elevations, in regions that are warmer and more humid.
3.2 Important environmental variables for the current distribution of the three Pinus yunnanensis varieties
The contribution rate of each environmental variable (for all the 25 variables) to the distribution of P. yunnanensis varieties were evaluated by using the jackknife method (Table 3). The main environmental variables that affected the potential distribution of var. yunnanensis are temperature seasonality (BIO4, 32.4%), mean annual fire occurrence (MAF, 22.2%), isothermality (BIO3, 19.1%), minus temperature of coldest month (BIO6, 9.2%) and elevation (ELEV, 8.8%), which contribute the most to the prediction model under current climate environment, and their total contribution rate reaches 91.7%; The main environmental variables that affected the potential distribution of var. pygmaea are isothermality (BIO3, 24.1%), mean annual fire occurrence (MAF, 18.6%), sandy soil content (SAND, 17.4%), temperature seasonality (BIO4, 13.2%), min temperature of coldest month (BIO6, 9%), and elevation (ELEV, 5.3%), with a total contribution rate of 87.6%; For var. tenuifolia, mean annual fire occurrence (MAF, 53.5%), isothermality (BIO3, 14.8%), soil nitrogen content (NIT, 9.9%), precision of driest month (BIO14, 8.2%), and elevation (ELEV, 7.6%) are the main environmental variables that have a cumulative contribution rate of 94%.
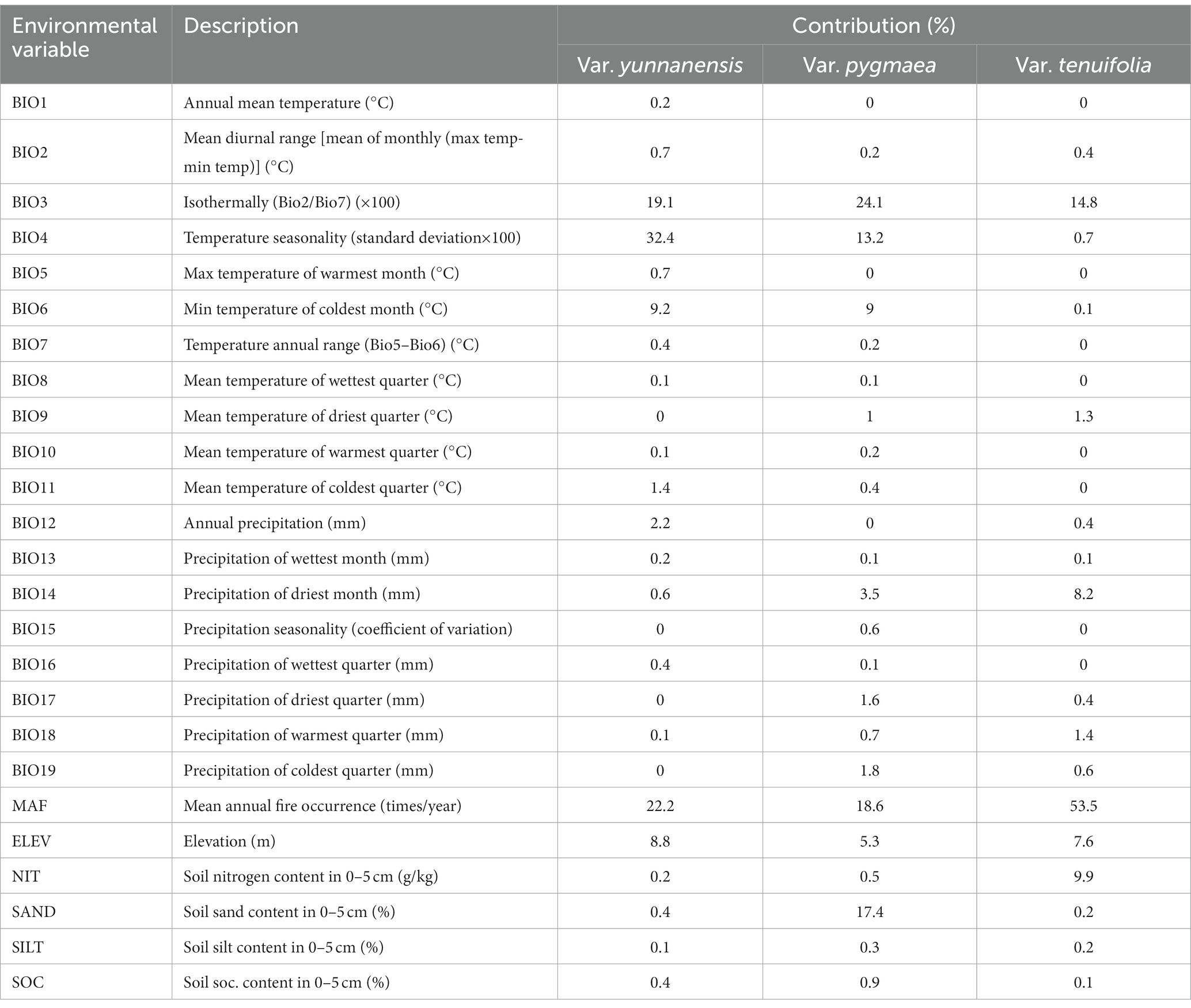
Table 3. The contribution rate of each environmental variable in determining the distribution of the three Pinus yunnanensis varieties.
3.3 Current potential suitable habitat areas for the three Pinus yunnanensis varieties
The highly and moderately suitable area for var. yunnanensis span approximately 10.27 × 104 km2 and 15.63 × 104 km2, predominantly located in north-central Yunnan and southern Sichuan, ranging from 98.3–105.3° E and 23.3–28.8° N (Figure 4A1). Var. pygmaea exhibits a highly and moderate suitable area of around 2.12 × 104 km2 and 3.95 × 104 km2, mainly distributed in central Yunnan and along the Yunnan-Sichuan border, spanning from 100.1–104.1° E and 24.1–27.8° N (Figure 4B1). As for var. tenuifolia, the highly and moderately suitable area encompass approximately 2.84 × 104 km2 and 3.21 × 104 km2, with a primary distribution along the border of Guangxi, Yunnan, and Guizhou, ranging from 103.7–107.5° E and 23.2–25.8° N (Figure 4C1).
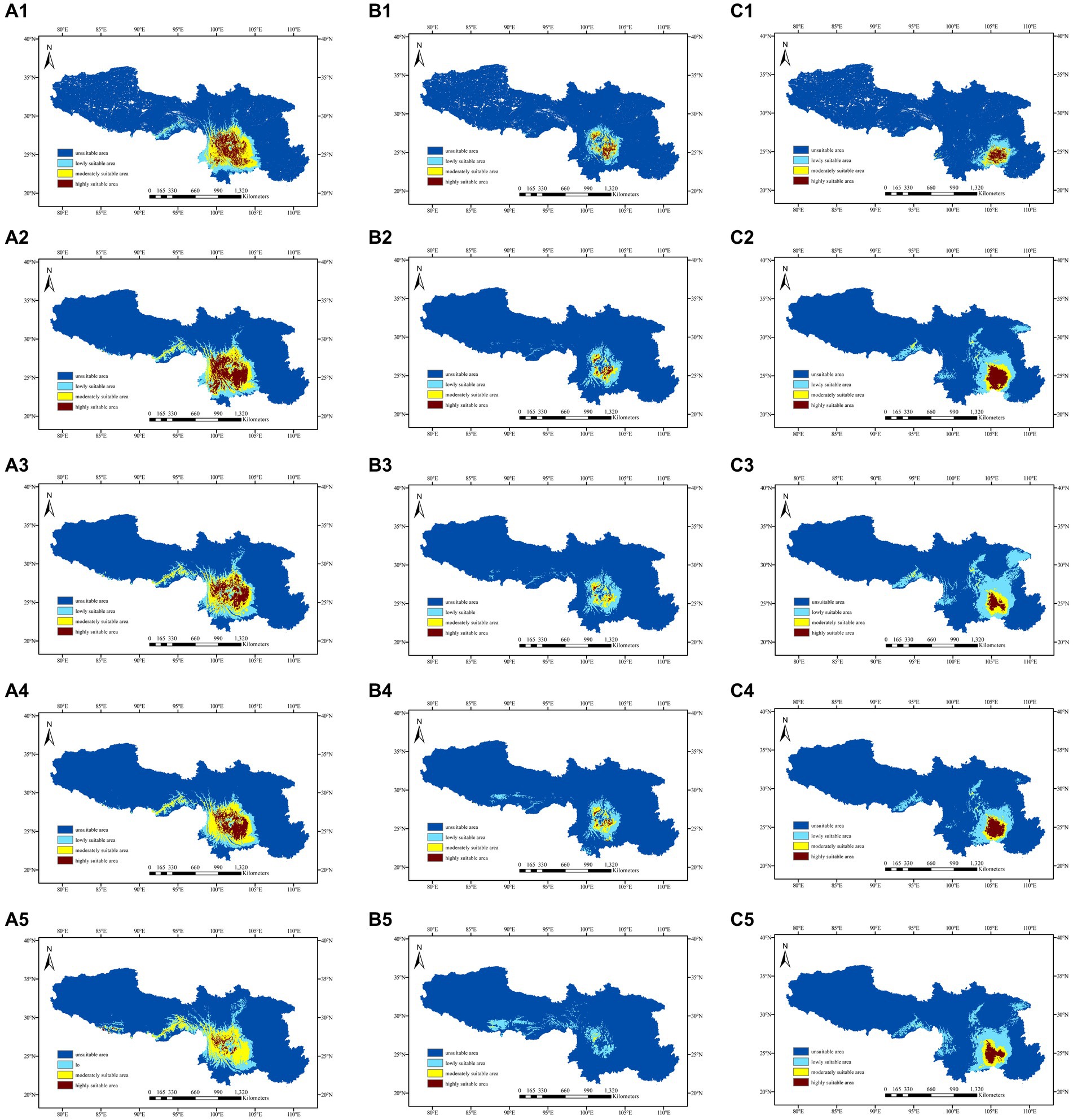
Figure 4. Predicted current and future potential distribution of three P. yunnanensis varieties under different scenarios. (A) var. yunnanensis; (B) var. pygmaea; (C) var. tenuifolia; (1) current climate; (2) future climate scenario SSP 1–2.6 in 2040–2060; (3) future climate scenario SSP 5–8.5 in 2040–2060; (4) future climate scenario SSP 1–2.6 in 2060–2080; (5) future climate scenario SSP 5–8.5 in 2060–2080.
3.4 Future changes in suitable habitat areas for the three Pinus yunnanensis varieties
We conducted predictions on the future suitable habitat area for the three varieties under two shared socio-economic pathways for two future periods (Figure 4; Table 4). According to the future climate model, it is projected that the total habitat area for all three species will increase under both emission concentrations between 2040 and 2060. The variation in habitat range for var. yunnanensis and var. pygmaea is relatively small, while the suitable habitat area for var. tenuifolia has approximately doubled compared to the current climate. From 2060 to 2080, the total habitat area for the three species exhibited smaller change compared to the period of 2040–2060, with a tendency to become narrower. Over time, there is an overall increasing trend followed by a subsequent decreasing trend in the suitable habitat area for the three varieties.
Under the SSP1-2.6 climate scenario, the highly suitable area for var. yunnanensis is projected to be approximately 14.07 × 104 km2 during the period of 2040–2060, marking an increase of around 37% compared to the current distribution area. This highly suitable area is primarily concentrated in Yunnan and Sichuan. However, by 2060–2080, the highly suitable area decreases to 9.14 × 104 km2. The moderately suitable and lowly suitable areas for var. yunnanensis is projected to undergo minor changes. During 2060–2080, the highly suitable area and lowly suitable area of var. yunnanensis decrease by 4.93 × 104 km2 and 1.88 × 104 km2, respectively, compared to the period of 2040–2060. Meanwhile, the moderately suitable area increases by 1.61 × 104 km2. In the case of var. pygmaea, the highly suitable area is projected to be around 1.40 × 104 km2 from 2040 to 2060, indicating a decrease of approximately 33% compared to the current distribution area. This highly suitable area continues to decline to 0.90 × 104 km2 by 2060–2080. The moderately suitable area for var. pygmaea shows small changes under the SSP1-2.6 climate scenario, while the lowly suitable area experiences a slight increase, expanding toward southern Tibet in the future. For var. tenuifolia, the total suitable area increases by 18.1 × 104 km2 from 2040 to 2060, with a significant increase in the highly suitable area, which more than doubles. The lowly suitable area also expands by 13.31 × 104 km2. By 2060–2080, the suitable areas in higher latitudes for var. tenuifolia will decrease, resulting in a reduction of the total suitable area by 8.39 × 104 km2.
Under the SSP5-8.5 climate scenario, from 2040 to 2060, both the highly and moderately suitable areas of var. yunnanensis are projected to decrease by approximately 5%, while the lowly suitable area is expected to increase by 10%. The highly suitable area exhibits a significant decline in the period from 2060 to 2080, with a 73% decrease compared to 2040–2060, whereas the moderately and lowly suitable areas in Yunnan province will experience an increase (Figure 4). From 2040 to 2060, the highly suitable area for var. pygmaea is reduced by 74%. The moderately suitable area decreases by 8%, while the lowly suitable area increases by 38%. By 2060–2080, the highly and moderately suitable areas of var. pygmaea have nearly disappeared, with the emergence of lowly suitable areas in higher elevation regions, such as southern Tibet and parts of Sichuan. The total suitable area for var. tenuifolia undergoes an approximate 1.6-fold increase from 2040 to 2060, reaching an area of 40.64 × 104 km2. Among them, the lowly suitable area demonstrates the most significant growth, with an increase of 23.14 × 104 km2. The highly and moderately suitable areas also show an increase, albeit relatively small in terms of area. By 2060–2080, the total suitable area decreases by 6.11 × 104 km2. The highly suitable area experiences a 30% increase, reaching 3.84 × 104 km2, while the moderately suitable area and the lowly suitable area decrease by 3 and 21%, respectively. Under the high emission scenario SSP5-8.5, the high-suitability areas for the three varieties will significantly decrease. In 2060–2080, the high-suitability area for var. pygmaea will virtually disappear, which may be attributed to its narrower ecological niche.
3.5 The shift of the distribution centers for the three Pinus yunnanensis varieties
The sdmtoolbox within the ArcGIS software was utilized to analyze the alteration of distribution centers of P. yunnanensis varieties. Currently, the distribution center of var. yunnanensis is situated at 101.10° E and 25.83° N in Yunnan Province. However, the centroid of its distribution is projected to shift toward the northeast under future climate scenarios. Between 2040 and 2060, it is anticipated to relocate to 100.69° E and 26.00° N under the SSP1-2.6 scenario, and to 100.65° E and 26.36° N under the SSP5-8.5 scenario. By 2060–2080, the projected distribution center of var. yunnanensis is 100.80° E and 16.17° N under the SSP1-2.6 scenario, and 99.74° E and 26.97° N under the SSP5-8.5 scenario. The current distribution center of var. pygmaea, situated in Yunnan province, is situated at 101.97° E and 26.04° N. The distribution centers are anticipated to shift northward in the future. Between 2040 and 2060, it is anticipated to relocate to 101.91° E and 26.06° N under the SSP1-2.6 scenario, and to 101.57° E and 26.28° N under the SSP5-8.5 scenario. By 2060–2080, the projected distribution center of var. pygmaea is 100.80° E and 26.25° N under the SSP1-2.6 scenario, and 96.49° E and 27.88° N under the SSP5-8.5 scenario. The current distribution center of var. tenuifolia is situated at 105.14° E and 24.80° N in Guangxi province. Similarly, the centroid of its distribution is projected to shift toward the northwest in the future. Between 2040 and 2060, it is anticipated to relocate to 104.22° E and 26.19° N under the SSP1-2.6 scenario, and to 104.28° E and 27.01° N under the SSP5-8.5 scenario. By 2060–2080, the projected shift is 103.95° E and 25.89° N under the SSP1-2.6 scenario, and 103.75° E and 26.33° N under the SSP5-8.5 scenario. Our study showed that all the 3 P. yunnanensis varieties are likely to expand their range toward higher latitudes as temperatures rises. In future, the distribution range of the three varieties of P. yunnanensis is projected to shift northward, encompassing new habitats in Guizhou, Chongqing, and parts of Tibet that are currently outside their distribution range (Figure 5).
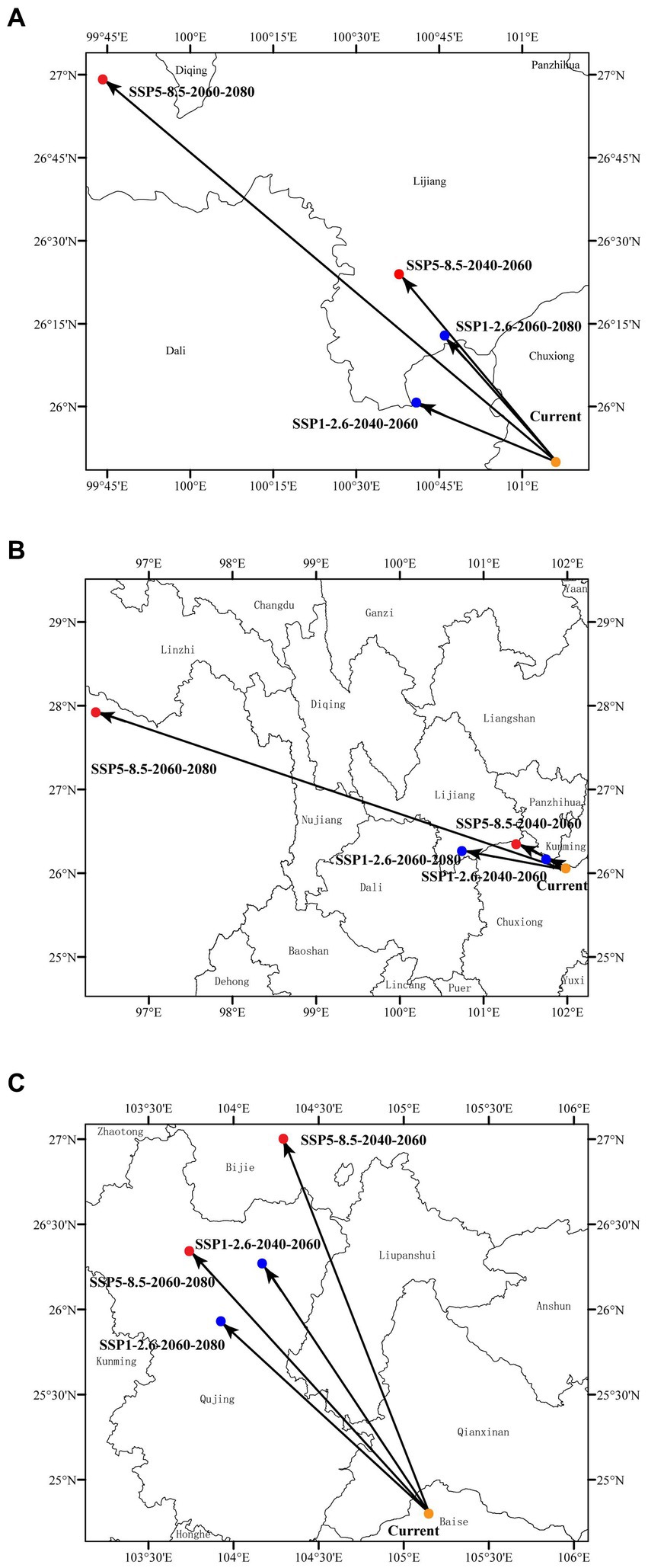
Figure 5. The shift of distributional centers under different climate scenario for the three Pinus yunnanensis varieties. (A) var. yunnanensis; (B) var. pygmaea; (C) var. tenuifolia; Arrow indicates magnitude and direction of predicted change through time.
3.6 MaxEnt model accuracy verification
The MaxEnt model was utilized to predict the current potential distribution of the 3P. yunnanensis varieties in the current climate environment. The accuracy of models was evaluated by using the Receiver Operating Characteristic (ROC) analysis. The Area Under the Curve (AUC) values for the three species were 0.928, 0.982, and 0.984, respectively (Figure s1). All AUC values exceeded 0.9, indicating excellent accuracy in model’s predictions.
4 Discussion
Environmental conditions shaped the distribution of plant species. We found that temperature-related factors played more important roles than precipitation in affecting the distribution of the three varieties of P. yunnanensis (Table 3), indicating that that temperature has a more pronounced impact on the distribution of P. yunnanensis compared to precipitation. Similar findings have been reported for other species. For instance, Wang et al. (2013) found that temperature-related variables played more crucial roles compared to precipitation factors in examining the correlation with the geographical distribution of three taxa of Akebia Decne. Tang et al. (2021) also found that pine species exhibit a higher degree of sensitivity toward temperature fluctuations than other environmental factors. Besides temperature, fire also played an important role in the distribution of P. yunnanensis varieties. Wildfire is an ancient natural phenomenon that is an important driver of species distribution and community succession in ecosystems (Magadzire et al., 2019). The central Yunnan Plateau ecosystem, where the P. yunnanensis is located, is a fire-dependent ecosystem (Su et al., 2015). P. yunnanensis is frequently exposed to forest fires and is a dominant species in such fire-prone environments. Fire-adapted traits have developed in P. yunnanensis and these fire-adapted traits exhibited obvious intraspecific variation (Pausas, 2015). For example, the shrubby variety pygmea has a higher serotiny level and stronger resprouting ability than the tree-forming varieties var. yunnanensis and var. tenuifolia. Tree-forming varieties have thicker bark than the shrub-forming variety, and have self-pruning ability, which is absent in the shrub-forming variety. The intraspecific variation of fire adaptations allowed P. yunnanensis varieties adapted to different fire regimes. Most species distribution studies have focused on environmental factors, such as climate and soils, with little consideration of the effects of wildfire conditions on species distribution patterns. Our study proved that fire regime is a critical factor that can affect the distribution of plant species.
Climate change can lead to rearrange species distributions, as plants tend to follow their familiar climates (Madsen-Hepp et al., 2023). For var. yunnanensis, the probability of presence expands as temperature seasonality (Bio4) increases, while for var. pygmaea, the probability of presence decreases when isothermally (Bio3) increasing (Supplementary Figure S2). This discrepancy probably due to the narrower ecological niche and smaller range of temperature suitability of var. pygmaea compared to var. yunnanensis. Previous studies showed that the distribution of var. pygmaea is strongly affected by annual mean temperature and mean temperature of coldest quarter temperature changes (Chen et al., 2021), indicating that var. pygmaea needs more stable seasonal temperatures. Var. tenuifolia shows a gradual increase in the probability of presence with an increase in mean annual fire occurrence (MAF), indicating that var. tenuifolia can survive and regenerate in fire-prone environment. Var. tenuifolia is characterized by thick bark and tall crowns, allowing it has a significant advantage in survival surface fires and a greater competitive edge within the community (Pausas et al., 2017). As the probability of presence of var. yunnanensis is predicted to be expanded, which will undoubtedly be beneficial to the timber economy. However, for var. pygmaea, suitable areas, especially highly suitable areas, will decrease indicating that carrying out some effective protection measures are necessary for var. pygmaea. In future, P. yunnanensis varieties are likely to expand their range toward higher latitudes as temperatures rises, encompassing new habitats that are currently outside their distribution range. The predictions are consistent with the view that climate warming is driving many species to move to higher latitudes (Guo et al., 2023; Wang et al., 2023).
Ecological niche modeling has been recognized as an effective method to make predictions and control measures for species distribution patterns, but this method still has drawbacks (Li et al., 2023) (Kolanowska, 2023). For example, predicting species distribution maybe inexact when relying solely on a limited set of environmental variables and the results may vary with the environmental variables being used in the model. In this study, we considered the effects of climate, elevation, soil and fire on the distribution of the three varieties of P. yunnanensis. In realty, there are many other factors that can influence the distribution of species (Ramachandran et al., 2020). For example, pests, diseases, and human activities are also important factors affecting the distribution of P. yunnanensis (Huang et al., 2022; Liu et al., 2022). Further research is needed in order to better evaluate the factors effecting the distribution of P. yunnanensis and predict the dynamic in its distribution changes. The combined effects of geographic and environmental factors have created the genetic and phenotypic differentiation among P. yunnanensis varieties (Wang et al., 2013). In addition to climatic conditions, the distribution of species is also influenced by specific traits of the species itself (Bradie and Leung, 2017). For example, high serotiny level facilitated var. pygmaea to survive in wildfires (Tang et al., 2013). Therefore, how trait variations affect the distribution of P. yunnanensis varieties are also necessary to explore.
5 Conclusion
For this study, temperature seasonality (BIO4) emerged as the most influential factor affecting var. yunnanensis; isothermally (BIO3) stands out as the most critical factor for var. pygmaea, whereas mean annual fire occurrence (MAF) had the greatest impact on var. tenuifolia. Fire affected the distribution of all the three varieties, indicating that fire regime played a vital role in shaping the distribution of P. yunnanensis varieties. Under global warming, the suitable habitat range for the three varieties is expected to expand, and the distribution centroids for all three varieties are projected to shift toward to higher latitudes. Our study can facilitate the conservation and utilization of P. yunnanensis varieties. However, our study is constrained by a limited set of environmental variables. To better assess the further distribution of P. yunnanensis varieties, additional factors should be considered. For varieties with decreasing suitable areas, conservation measures should be implemented to protect them.
Data availability statement
The original contributions presented in the study are included in the article/Supplementary material, further inquiries can be directed to the corresponding author/s.
Author contributions
JF: Writing – original draft. BW: Writing – review & editing. MX: Writing – review & editing. SZ: Writing – review & editing. CH: Writing – review & editing. XC: Writing – review & editing.
Funding
The author(s) declare that financial support was received for the research, authorship, and/or publication of this article. This research was funded by the National Natural Science Foundation of China (grant number 32101532), and the Natural Science Foundation of Sichuan Province (grant number 2023NSFSC1278).
Conflict of interest
The authors declare that the research was conducted in the absence of any commercial or financial relationships that could be construed as a potential conflict of interest.
Publisher’s note
All claims expressed in this article are solely those of the authors and do not necessarily represent those of their affiliated organizations, or those of the publisher, the editors and the reviewers. Any product that may be evaluated in this article, or claim that may be made by its manufacturer, is not guaranteed or endorsed by the publisher.
Supplementary material
The Supplementary material for this article can be found online at: https://www.frontiersin.org/articles/10.3389/ffgc.2023.1308416/full#supplementary-material
References
Ab Lah, N. Z., Yusop, Z., Hashim, M., Mohd Salim, J., and Numata, S. (2021). Predicting the habitat suitability of Melaleuca cajuputi based on the MaxEnt species distribution model. Forests 12:1449. doi: 10.3390/f12111449
Ahmad, R., Khuroo, A. A., Charles, B., Hamid, M., Rashid, I., and Aravind, N. A. (2019). Global distribution modelling, invasion risk assessment and niche dynamics of Leucanthemum vulgare (ox-eye daisy) under climate change. Sci. Rep. 9:11395. doi: 10.1038/s41598-019-47859-1
Baradevanal, G., Chander, S., Singh, H. S., Reddy, D. S., and Rajan, S. (2023). Mapping the risk of quarantine pest Sternochetus mangiferae under different climate change scenarios through species distribution modelling. Int. J. Trop. Insect Sci. 43, 919–932. doi: 10.1007/s42690-023-01000-y
Bousfield, C. G., Lindenmayer, D. B., and Edwards, D. P. (2023). Substantial and increasing global losses of timber-producing forest due to wildfires. Nat. Geosci. 16, 1145–1150. doi: 10.1038/s41561-023-01323-y
Bradie, J., and Leung, B. (2017). A quantitative synthesis of the importance of variables used in MaxEnt species distribution models. J. Biogeogr. 44, 1344–1361. doi: 10.1111/jbi.12894
Cai, N., Xu, Y., Chen, S., He, B., Li, G., Li, Y., et al. (2016). Variation in seed and seedling traits and their relations to geo-climatic factors among populations in Yunnan pine (Pinus yunnanensis). J. For. Res. 27, 1009–1017. doi: 10.1007/s11676-016-0228-z
Chen, J., Zhang, S., Luo, T., Zheng, W., Yang, W., Li, J., et al. (2021). Distribution pattern of Pinus yunnanensis and P. yunnanensis var. pygmaea and related key ecological factors. J. Northeast For. Univ. 49, 8–14. doi: 10.13759/j.cnki.dlxb.2021.09.002
Dyderski, M. K., Paź, S., Frelich, L. E., and Jagodziński, A. M. (2018). How much does climate change threaten European forest tree species distributions? Glob. Change Biol. 24, 1150–1163. doi: 10.1111/gcb.13925
Elith, J., Phillips, S. J., Hastie, T., Dudík, M., Chee, Y. E., and Yates, C. J. (2011). A statistical explanation of MaxEnt for ecologists. Divers. Distrib. 17, 43–57. doi: 10.1111/j.1472-4642.2010.00725.x
Fand, B. B., Shashank, P. R., Suroshe, S. S., Chandrashekar, K., Meshram, N. M., and Timmanna, H. N. (2020). Invasion risk of the south American tomato pinworm Tuta absoluta (Meyrick) (Lepidoptera: Gelechiidae) in India: predictions based on MaxEnt ecological niche modelling. Int. J. Trop. Insect Sci. 40, 561–571. doi: 10.1007/s42690-020-00103-0
Ferreira, M. T., Cardoso, P., Borges, P. A. V., Gabriel, R., De Azevedo, E. B., Reis, F., et al. (2016). Effects of climate change on the distribution of indigenous species in oceanic islands (Azores). Clim. Chang. 138, 603–615. doi: 10.1007/s10584-016-1754-6
Guisan, A., and Thuiller, W. (2005). Predicting species distribution: offering more than simple habitat models. Ecol. Lett. 8, 993–1009. doi: 10.1111/j.1461-0248.2005.00792.x
Guo, Y., Zhao, Z., Yuan, S., and Li, X. (2023). A greener loess plateau in the future: moderate warming will expand the potential distribution areas of woody species. Environ. Res. Lett. 18:034027. doi: 10.1088/1748-9326/acb9a8
Herrando-Moraira, S., Vitales, D., Nualart, N., Gómez-Bellver, C., Ibáñez, N., Massó, S., et al. (2020). Global distribution patterns and niche modelling of the invasive Kalanchoe × houghtonii (Crassulaceae). Sci. Rep. 10:3143. doi: 10.1038/s41598-020-60079-2
Hu, Z., Guo, K., Jin, S., and Pan, H. (2019). The influence of climatic changes on distribution pattern of six typical Kobresia species in Tibetan plateau based on MaxEnt model and geographic information system. Theor. Appl. Climatol. 135, 375–390. doi: 10.1007/s00704-018-2373-0
Huang, B., Mao, J., Zhao, Y., Sun, Y., Cao, Y., and Xiong, Z. (2022). Similar pattern of potential distribution of Pinus yunnanensis Franch and Tomicusyunnanensis Kirkendall under climate change in China. Forests 13:1379. doi: 10.3390/f13091379
Ji, W., Han, K., Lu, Y., and Wei, J. (2020). Predicting the potential distribution of the vine mealybug, Planococcus ficus under climate change by MaxEnt. Crop Prot. 137:105268. doi: 10.1016/j.cropro.2020.105268
Kolanowska, M. (2023). Future distribution of the epiphytic leafless orchid (Dendrophylax lindenii), its pollinators and phorophytes evaluated using niche modelling and three different climate change projections. Sci. Rep. 13:15242. doi: 10.1038/s41598-023-42573-5
Li, S., Wang, Z., Zhu, Z., Tao, Y., and Xiang, J. (2023). Predicting the potential suitable distribution area of Emeia pseudosauteri in Zhejiang Province based on the MaxEnt model. Sci. Rep. 13:1806. doi: 10.1038/s41598-023-29009-w
Li, X., Yao, Z., Yuan, Q., Xing, R., Guo, Y., Zhang, D., et al. (2023). Prediction of potential distribution area of two Parapatric species in Triosteum under climate change. Sustainability 15:5604. doi: 10.3390/su15065604
Li, Y., Shao, W., and Jiang, J. (2022). Predicting the potential global distribution of Sapindus mukorossi under climate change based on MaxEnt modelling. Environ. Sci. Pollut. Res. 29, 21751–21768. doi: 10.1007/s11356-021-17294-9
Liu, M., Zhang, Z., Liu, X., Li, M., and Shi, L. (2022). Trend analysis of coverage variation in Pinus yunnanensis Franch. Forests under the influence of pests and abiotic factors. Forests 13:412. doi: 10.3390/f13030412
Madsen-Hepp, T. R., Franklin, J., McFaul, S., Schauer, L., and Spasojevic, M. J. (2023). Plant functional traits predict heterogeneous distributional shifts in response to climate change. Funct. Ecol. 37, 1449–1462. doi: 10.1111/1365-2435.14308
Magadzire, N., de Klerk, H. M., Esler, K. J., and Slingsby, J. A. (2019). Fire and life history affect the distribution of plant species in a biodiversity hotspot. Divers. Distrib. 25, 1012–1023. doi: 10.1111/ddi.12921
Merow, C., Smith, M. J., and Silander, J. A. Jr. (2013). A practical guide to MaxEnt for modeling species’ distributions: what it does, and why inputs and settings matter. Ecography 36, 1058–1069. doi: 10.1111/j.1600-0587.2013.07872.x
Ouyang, X., Lin, H., Bai, S., Chen, J., and Chen, A. (2022). Simulation the potential distribution of Dendrolimus houi and its hosts, Pinus yunnanensis and Cryptomeria fortunei, under climate change in China. Front. Plant Sci. 13:1054710. doi: 10.3389/fpls.2022.1054710
Parveen, S., Kaur, S., Baishya, R., and Goel, S. (2022). Predicting the potential suitable habitats of genus Nymphaea in India using MaxEnt modeling. Environ. Monit. Assess. 194:853. doi: 10.1007/s10661-022-10524-8
Pausas, J. G. (2015). Evolutionary fire ecology: lessons learned from pines. Trends Plant Sci. 20, 318–324. doi: 10.1016/j.tplants.2015.03.001
Pausas, J. G., Keeley, J. E., and Schwilk, D. W. (2017). Flammability as an ecological and evolutionary driver. J. Ecol. 105, 289–297. doi: 10.1111/1365-2745.12691
Phillips, S. J., Anderson, R. P., and Schapire, R. E. (2006). Maximum entropy modeling of species geographic distributions. Ecol. Model. 190, 231–259. doi: 10.1016/j.ecolmodel.2005.03.026
Phillips, S. J., and Dudík, M. (2008). Modeling of species distributions with Maxent: new extensions and a comprehensive evaluation. Ecography 31, 161–175. doi: 10.1111/j.0906-7590.2008.5203.x
Poggio, L., de Sousa, L. M., Batjes, N. H., Heuvelink, G. B. M., Kempen, B., Ribeiro, E., et al. (2021). SoilGrids 2.0: producing soil information for the globe with quantified spatial uncertainty. Soil 7, 217–240. doi: 10.5194/soil-7-217-2021
Ramachandran, R. M., Roy, P. S., Chakravarthi, V., Joshi, P. K., and Sanjay, J. (2020). Land use and climate change impacts on distribution of plant species of conservation value in Eastern Ghats, India: a simulation study. Environ. Monit. Assess. 192:86. doi: 10.1007/s10661-019-8044-5
Root, T. L., Price, J. T., Hall, K. R., Schneider, S. H., Rosenzweig, C., and Pounds, J. A. (2003). Fingerprints of global warming on wild animals and plants. Nature 421, 57–60. doi: 10.1038/nature01333
Séférian, R., Nabat, P., Michou, M., Saint-Martin, D., Voldoire, A., Colin, J., et al. (2019). Evaluation of CNRM earth system model, CNRM-ESM2-1: role of earth system processes in present-day and future climate. J. Adv. Model. Earth Syst. 11, 4182–4227. doi: 10.1029/2019MS001791
Shen, J., Li, Z., Gao, C., Li, S., Huang, X., Lang, X., et al. (2020). Radial growth response of Pinus yunnanensis to rising temperature and drought stress on the Yunnan plateau, southwestern China. For. Ecol. Manag. 474:118357. doi: 10.1016/j.foreco.2020.118357
Su, W.-H., Shi, Z., Zhou, R., Zhao, Y.-J., and Zhang, G.-F. (2015). The role of fire in the Central Yunnan plateau ecosystem, southwestern China. For. Ecol. Manag. 356, 22–30. doi: 10.1016/j.foreco.2015.05.015
Sun, Y.-Q., Zhao, W., Xu, C.-Q., Xu, Y., El-Kassaby, Y. A., De La Torre, A. R., et al. (2020). Genetic variation related to high elevation adaptation revealed by common garden experiments in Pinus yunnanensis. Front. Genet. 10:1405. doi: 10.3389/fgene.2019.01405
Tang, C. Q., He, L.-Y., Su, W.-H., Zhang, G.-F., Wang, H.-C., Peng, M.-C., et al. (2013). Regeneration, recovery and succession of a Pinus yunnanensis community five years after a mega-fire in Central Yunnan. China. For. Ecol. Manag. 294, 188–196. doi: 10.1016/j.foreco.2012.07.019
Tang, X., Yuan, Y., Li, X., and Zhang, J. (2021). Maximum entropy modeling to predict the impact of climate change on pine wilt disease in China. Front. Plant Sci. 12:2500. doi: 10.3389/fpls.2021.652500
Tsoar, A., Allouche, O., Steinitz, O., Rotem, D., and Kadmon, R. (2007). A comparative evaluation of presence-only methods for modelling species distribution. Divers. Distrib. 13, 397–405. doi: 10.1111/j.1472-4642.2007.00346.x
Ullah, F., Zhang, Y., Gul, H., Hafeez, M., Desneux, N., Qin, Y., et al. (2023). Estimation of the potential geographical distribution of invasive peach fruit fly under climate change by integrated ecological niche models. CABI Agric. Biosci. 4:46. doi: 10.1186/s43170-023-00187-x
Wang, B., Mao, J.-F., Zhao, W., and Wang, X.-R. (2013). Impact of geography and climate on the genetic differentiation of the subtropical pine Pinus yunnanensis. PLoS One 8:e67345. doi: 10.1371/journal.pone.0067345
Wang, X., Zhang, W., Zhao, X., Zhu, H., Ma, L., Qian, Z., et al. (2021). Modeling the potential distribution of three taxa of Akebia decne. under climate change scenarios in China. Forests 12:1710. doi: 10.3390/f12121710
Wang, Y., Xie, L., Zhou, X., Chen, R., Zhao, G., and Zhang, F. (2023). Prediction of the potentially suitable areas of Leonurus japonicus in China based on future climate change using the optimized MaxEnt model. Ecol. Evol. 13:e10597. doi: 10.1002/ece3.10597
Wu, Z., He, H. S., Keane, R. E., Zhu, Z., Wang, Y., and Shan, Y. (2020). Current and future patterns of forest fire occurrence in China. Int. J. Wildland Fire 29, 104. doi: 10.1071/WF19039
Zhang, J., Peng, X., Song, M., Li, Z., Xu, X., and Wang, W. (2022). Effects of climate change on the distribution of wild Akebia trifoliata. Ecol. Evol. 12:e8714. doi: 10.1002/ece3.8714
Zhang, Y., Tang, J., Ren, G., Zhao, K., and Wang, X. (2021). Global potential distribution prediction of Xanthium italicum based on Maxent model. Sci. Rep. 11:16545. doi: 10.1038/s41598-021-96041-z
Keywords: Pinus yunnanensis, MaxEnt model, distribution, climate change, habitat conditions
Citation: Feng J, Wang B, Xian M, Zhou S, Huang C and Cui X (2023) Prediction of future potential distributions of Pinus yunnanensis varieties under climate change. Front. For. Glob. Change. 6:1308416. doi: 10.3389/ffgc.2023.1308416
Edited by:
Yashwant Singh Rawat, Federal Technical and Vocational Education and Training Institute, EthiopiaReviewed by:
Augusta Costa, National Institute for Agricultural and Veterinary Research, PortugalPrashant Sharma, Dr. Yashwant Singh Parmar University of Horticulture and Forestry, India
Copyright © 2023 Feng, Wang, Xian, Zhou, Huang and Cui. This is an open-access article distributed under the terms of the Creative Commons Attribution License (CC BY). The use, distribution or reproduction in other forums is permitted, provided the original author(s) and the copyright owner(s) are credited and that the original publication in this journal is cited, in accordance with accepted academic practice. No use, distribution or reproduction is permitted which does not comply with these terms.
*Correspondence: Xinglei Cui, eGluZ2xlaS5jdWlAc2ljYXUuZWR1LmNu