- 1Department of Nuclear Medicine, First Hospital of Shanxi Medical University, Taiyuan, China
- 2Molecular Imaging Precision Medical Collaborative Innovation Center, Shanxi Medical University, Taiyuan, China
Objective: This study aimed to evaluate the value of 18F-FDG PET/CT radiomics in predicting EGFR gene mutations in non-small cell lung cancer by meta-analysis.
Methods: The PubMed, Embase, Cochrane Library, Web of Science, and CNKI databases were searched from the earliest available date to June 30, 2023. The meta-analysis was performed using the Stata 15.0 software. The methodological quality and risk of bias of included studies were assessed using the Quality Assessment of Diagnostic Accuracy Studies 2 and Radiomics Quality Score criteria. The possible causes of heterogeneity were analyzed by meta-regression.
Results: A total of 17 studies involving 3763 non-small cell lung cancer patients were finally included. We analyzed 17 training cohorts and 10 validation cohorts independently. Within the training cohort, the application of 18F-FDG PET/CT radiomics in predicting EGFR mutations in NSCLC demonstrated a sensitivity of 0.76 (95% CI: 0.70-0.81) and a specificity of 0.78 (95% CI: 0.74-0.82), accompanied by a positive likelihood ratio of 3.5 (95% CI:3.0-4.2), a negative likelihood ratio of 0.31 (95% CI: 0.24-0.39), a diagnostic odds ratio of 11.0 (95% CI: 8.0-16.0), and an area under the curve (AUC) of 0.84 (95% CI: 0.80-0.87). In the validation cohort, the values included a sensitivity of 0.76 (95% CI: 0.67-0.83), a specificity of 0.75 (95% CI: 0.68-0.80), a positive likelihood ratio of 3.0 (95% CI:2.4-3.8), a negative likelihood ratio of 0.32 (95% CI: 0.24-0.44), a diagnostic odds ratio of 9 (95% CI: 6-15), and an AUC of 0.82 (95% CI: 0.78-0.85). The average Radiomics Quality Score (RQS) across studies was 10.47 ± 4.72. Meta-regression analysis identifies the application of deep learning and regions as sources of heterogeneity.
Conclusion: 18F-FDG PET/CT radiomics may be useful in predicting mutation status of the EGFR gene in non-small cell lung cancer.
Systematic review registration: https://www.crd.york.ac.uk/PROSPERO, identifier CRD42022385364.
1 Introduction
Lung cancer is one of the most prevalent malignant tumors with high incidence and mortality rates (1, 2). Non-small-cell lung cancer (NSCLC) accounts for approximately 85% of primary lung cancers, and most patients are diagnosed with an advanced stage of the disease, leading to a 5-year survival rate of less than 20%. The use of tyrosine kinase inhibitors targeting the epidermal growth factor receptor (EGFR) has been an effective treatment of improving the prognosis of NSCLC patients with EGFR mutation (3, 4). Therefore, the early identification of EGFR gene mutation status in NSCLC patients is critical. Nevertheless, gene detection methods usually require an invasive tissue or cell biopsy, a process that is time-consuming and possibly risky. Therefore, it is essential to develop a non-invasive and faster detection method to predict EGFR mutation status.18F-fluorodeoxyglucose positron emission tomography/computed tomography (18F-FDG PET/CT) is routinely used for tumor staging, treatment decision-making, and response monitoring in NSCLC patients (5, 6). Previous studies have shown that semi-quantitative parameters derived from 18F-FDG PET/CT, including maximum standard uptake value (SUVmax) and total lesion glycolysis (TLG), have reasonable diagnostic utility in detecting EGFR mutation status in NSCLC patients. However, meta-analysis indicates that SUVmax has a low pooled sensitivity and specificity in predicting EGFR mutation status in NSCLC patients (7, 8). Therefore, it is necessary to investigate additional parameters such as radiomic features of 18F-FDG PET/CT to predict EGFR mutation status in NSCLC.
Radiomics is an emerging field that analyzes quantitative medical images to extract a large number of objective and quantitative image features that are correlated with clinical, pathological, molecular, and genetic features that may reflect tumor genetic phenotypes by utilizing artificial intelligence algorithms (9). Previously published studies have exhibited significant variations in methodology and outcomes (10, 11). Thus, Consequently, the objective of this study is to conduct a meta-analysis of published studies on 18F-FDG radiomics for predicting EGFR mutation status in patients with NSCLC.
2 Materials and methods
This study followed the Preferred Reporting Item of the Guidelines for Systematic Reviews and Meta-Analysis (PRISMA) and was registered in PROSPER (CRD42022385364, https://www.crd.york.ac.uk/prospero).
2.1 Literature search
The PubMed, Embase, Web of Science, Cochrane Library, and CNKI databases were searched by two independent observers to identify eligible studies up to June 30, 2023. The searches used a combination of subject headings and free terms, including “radiomics”, “texture analysis”, “artificial intelligence”, “lung cancer”, “PET/CT”, “EGFR”, and “18F-FDG”. The search strategies are shown in Supplementary Tables S1-S5.
2.2 Inclusion and exclusion criteria
We selected publications for review if they met several of the following inclusion criteria: (1) All patients were NSCLC patients with pathology confirmation and underwent 18F-FDG PET/CT scans. (2) Radiomics or deep learning algorithms applied to predict EGFR mutation status. (3) The number of true positives (TP), false positives (FP), true negatives (TN), and false negatives (FN) must be reported or quantified in the studies. Exclusion criteria: (1) Reviews, meta-analyses, conference abstracts, editorials, and notes. (2) Duplicated and irrelevant studies. (3) Studies where diagnostic data could not be obtained.
2.3 Data extraction
Data from each eligible study were independently extracted by two reviewers. The following data were collected: first author, publication year, country/region, study object, study design, blindness to EGFR mutation results when reviewing the PET/CT image, sample size, EGFR mutation rate, patient characteristics (age, gender, and 18F-FDG injection dose), tumor segmentation, feature extraction, feature type, validation and radiomics algorithms, receiver operating characteristic curve (AUC), sensitivity, specificity, TP, FP, TN, and FN. In situations where a study reported accuracy data for multiple models, we utilized the 2 × 2 tables for the model with the highest AUC.
2.4 Quality assessment
Two independent investigators used the Quality Assessment for Diagnostic Accuracy Studies-2 (QUADAS-2) and the Radiomics Quality Score (RQS) to assess the methodological quality and risk of bias of the included studies (12, 13). Where there were differences of opinion, we had recourse to a third reviewer for conflict resolution. QUADAS-2 primarily includes assessment of risk of bias and clinical applicability. Risk of bias includes “patient selection”, “index test”, “reference standard”, and “flow and timing”, while clinical applicability requires assessment of the first three. The quality of radiomics processes and reports was evaluated using the Radiomics Quality Score (RQS), which comprised 16 dimensions. The total scores ranged between -8 and +36. A score between -8 and 0 was equivalent to 0%, while a score of 36 was equivalent to 100%. The inter-reviewer agreement for each item of the RQS was quantified using the modified Fleiss kappa statistic, tailored for ordered variables. Overall inter-reviewer agreement, including for the RQS, was assessed using intergroup correlation coefficients (ICCs), calculated via a single-source, two-way random effects model to ascertain absolute agreement between reviewers.
2.5 Statistical analysis
Meta-analysis was performed by utilizing Stata 15.0 software (Stata Corp, College Station, Texas, USA) and the MIDAS bivariate random-effects model. Heterogeneity among the studies included in our analysis was assessed using the Cochran Q test and the I2 statistic. The significance level was set at P<0.05. According to the Cochrane Handbook for Systematic Reviews of Diagnostic Test Accuracy, the I2 value reflects the degree of heterogeneity, with an I2 value of more than 50% indicating high heterogeneity (14). The effectiveness of 18F-FDG PET/CT in detecting EGFR mutation status in NSCLC patients was evaluated by calculating the pooled statistics of sensitivity (SEN), specificity (SPE), positive likelihood ratio (PLR), negative likelihood ratio (NLR), diagnostic odds ratio (DOR), and their corresponding 95% confidence intervals (CI). Predictive accuracy was also evaluated using summary receiver operating characteristic and area under the curves. Spearman’s correlation coefficient was calculated using Meta-Disc 1.4 software (Ramon y Cajal Hospital, Madrid, Spain) to investigate the potential threshold effect. A threshold effect was considered present if r > 0.5 and P < 0.05. Publication bias was assessed using the Deeks funnel plot, where significant asymmetry was indicated if P <0.10. Sensitivity analysis was to observe the stability of the synthetic results. In our meta-regression, aimed at pinpointing sources of heterogeneity, analyzed covariates such as blinding to EGFR mutation results in PET/CT reviews (yes or unclear), modeling methods (deep learning or radiomics algorithms), sample (<130 or ≥130), study focus (NSCLC or ADC only), and the radiomics software (Pyradiomics or others), publication year (before or after 2022). Factors in model construction included the integration of clinical information,gender number (≥50 or <50), number of smokers (≥100 or <100), Radiomics Quality Score (RQS ≥12 or <12), and region (mainland China or others). For assessing clinical utility, we calculated posttest probabilities and created Fagan plots.
3 Results
3.1 Literature search
A comprehensive search was conducted through various databases including PubMed, Embase, Cochrane Library, Web of Science, and CNKI. Initially, a total of 87 studies were found. After removing 29 duplicate articles, the remaining articles were screened by two independent reviewers based on their titles and abstracts. 5 conference abstracts, 15 reviews, 1 editorial, 13 irrelevant studies and 1 note were excluded subsequently. Further assessment of the full texts led to the exclusion of 4 studies with insufficient data and 3 studies without PET radiomics feature. Ultimately, 17 diagnostic studies that met the inclusion criteria were included in the analysis. The PRISMA flow-chart of the literature search of our systematic review and meta-analysis is presented in Figure 1. All studies included were retrospective cohort studies.
A total of 3763 patients were included, and the sample sizes of the studies ranged from 50-583, Our study’s training cohort comprised 2877 individuals, averaging 169 participants per study and a median size of 127, while the validation group encompassed 1021 individuals. In the training set, 1239 patients reported a smoking history, and 1321 were female. Additionally, nine of the studies integrated clinical characteristics, including demographic data (age, gender), smoking history, and tumor stage, to enhance EGFR mutation prediction accuracy. Our analysis encompassed 17 studies: 15 from China, one from Canada, and one utilizing public datasets (‘TCGA LUAD’ and ‘NSCLC Radiogenomics’). Of these, seven specifically investigated lung adenocarcinoma (ADC), while the others included ADC and other NSCLC subtypes. The radiomic features analyzed were diverse, spanning first-order, texture, shape, size, and deep learning features. Commonly employed feature types included Gray Level Co-occurrence Matrix, Gray Level Dependence Matrix, Gray Level Run Length Matrix, Gray Level Size Zone Matrix, and Neighborhood Gray Tone Difference Matrix. In radiomics analysis, five studies utilized Pyradiomics for feature extraction. Of the studies reviewed, thirteen used classical machine learning algorithms and four used deep learning approaches. The literature’s publication dates range from 2019 to 2023. The literature’s publication dates range from 2019 to 2023. The specific characteristics of the included literature are shown in Tables 1, 2.
3.2 Data quality assessment
The results of the QUADAS-2 quality assessment for the literature are shown in Figure 2. Due to inappropriate or incomplete exclusion criteria, eleven studies exhibited high or unclear risk of bias in terms of patient selection. Concerning the reference standard, ten studies showed an unclear risk of bias due to missing information on blindness compared to the reference test. Flow and timing introduced uncertainty regarding the risk of bias in six studies, as they exhibited an unclear risk of bias owing to ambiguity in the time interval between the index test and the reference standard. The patient selection of the included studies was of low applicability concern. One study had unclear applicability concerns because the index test was performed with different PET/CT scanners. Another study had high applicability concerns because no information on PET/CT acquisition was provided. Regarding the reference standard, none of the included studies showed an unclear or high risk of bias. Overall, most studies have low or unclear bias risks and moderate clinical applicability problems. The details of the QUADAS-2 assessment are presented in Figure 2 and Supplementary Figure S2.
The included studies achieved a mean ± standard deviation RQS of 10.47 ± 4.72, a median of 12, and a range of 3 to 19. The highest RQS score was 19 (52.8%). RQS scores showed improvement over time (Supplementary Figure S2). About half of the studies scored greater than 10. Since no study considered the three items “Phantom study on all scanners”, “Imaging at multiple time points”, and “Prospective study”, these three items received a score of zero. Most studies provided details about the items “Imaging protocol”, “Feature reduction”, “Non Radiomics”, “Biological correlates”, “Discrimination and resampling” and “Gold standard”. The other items that underperformed included “Multiple segmentation,” “Cutoff analysis,” “Calibration statistics,” “Validation,” “Clinical utility,” “Cost-effectiveness analysis,” and “Open science and data,” each with an average score of less than 15%. A detailed description of the RQS scores is provided in Supplementary Table S6, Supplementary Figure S1. Inter-reviewer agreement for the Radiomics Quality Score (RQS) was quantified using the ICC, which stood at 0.97 (95% CI 0.82-0.99). Across the seven RQS criteria, moderate agreement was observed, while nine items reached substantial or near-perfect concordance, as detailed in Table 3.
3.3 Meta-analysis combined results
We performed a meta-analysis to combine the results of the 17 included studies. For train cohort, the pooled SEN, SPE, PLR, NLR, and DOR for the radiomics based on 18F-FDG PET/CT in diagnose EGFR mutation status of NSCLC patients were 0.76(0.70,0.81), 0.78(0.74,0.82), 3.5(3.0,4.2), 0.31(0.24,0.39), and 11.0(8.0,16.0) respectively. The forest plot showed significant heterogeneity in sensitivity (I2 = 78.34, P<0.01) and specificity (I2 = 69.92, P=0.01). For the validation cohort of 10 studies, the pooled SEN, SPE, PLR, NLR and DOR were 0.76 (0.67,0.83), 0.75 (0.68–0.80), 3.0 (2.4,3.8), 0.32 (0.24,0.44) and 9 (6, 15). Figures 3, 4 show the forest plots for the training cohort and validation cohort, respectively. For the training and validation cohorts, the area under the curve (AUC) was 0.84 (95% CI: 0.80-0.87) and 0.82 (95% CI: 0.78-0.85), respectively (Figures 5A, B).
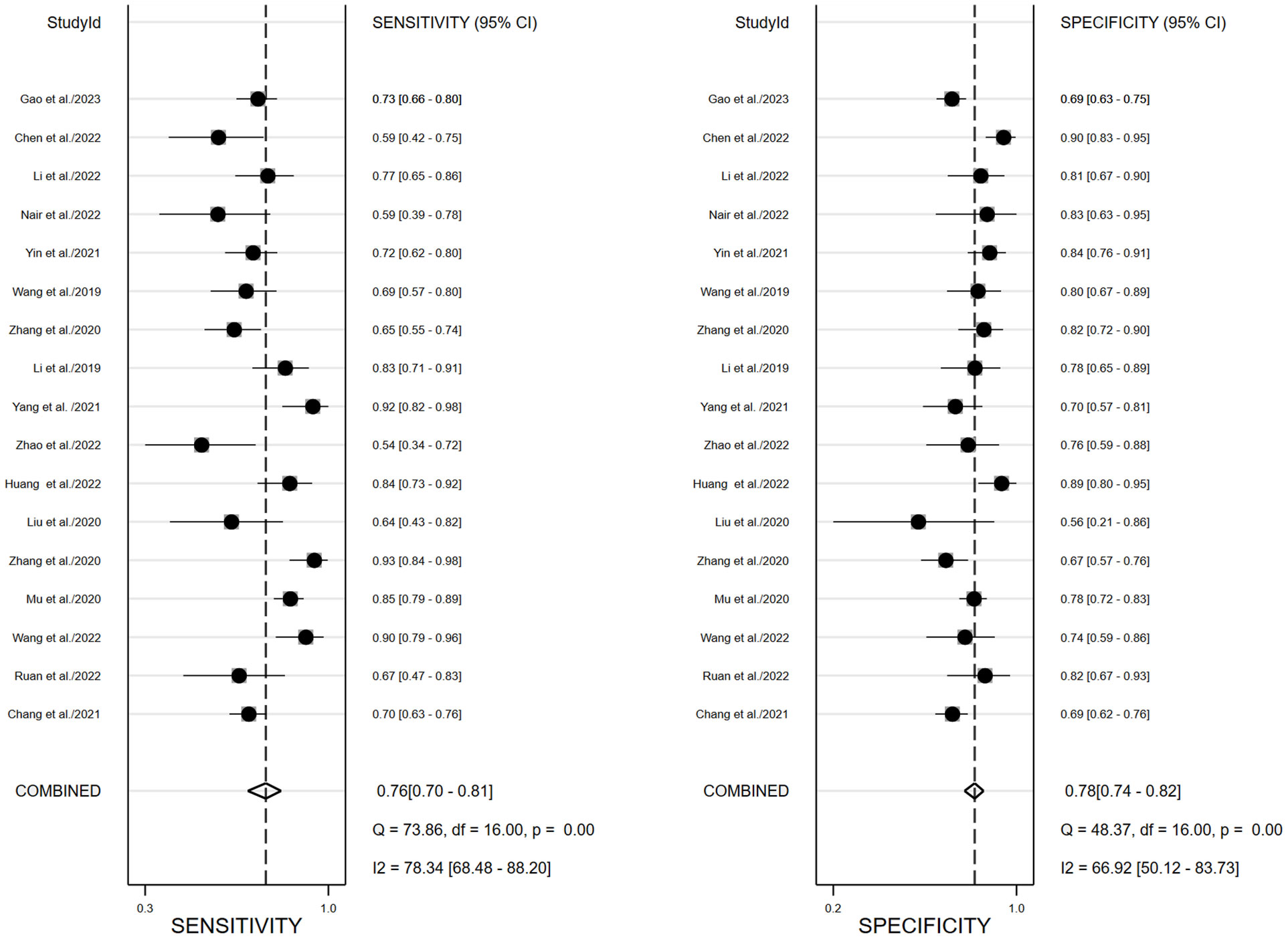
Figure 3 Forest plots of pooled sensitivity and specificity of 18F-FDG PET/CT radiomics diagnostic performance of predicting EGFR mutations in NSCLC patients for training cohort.
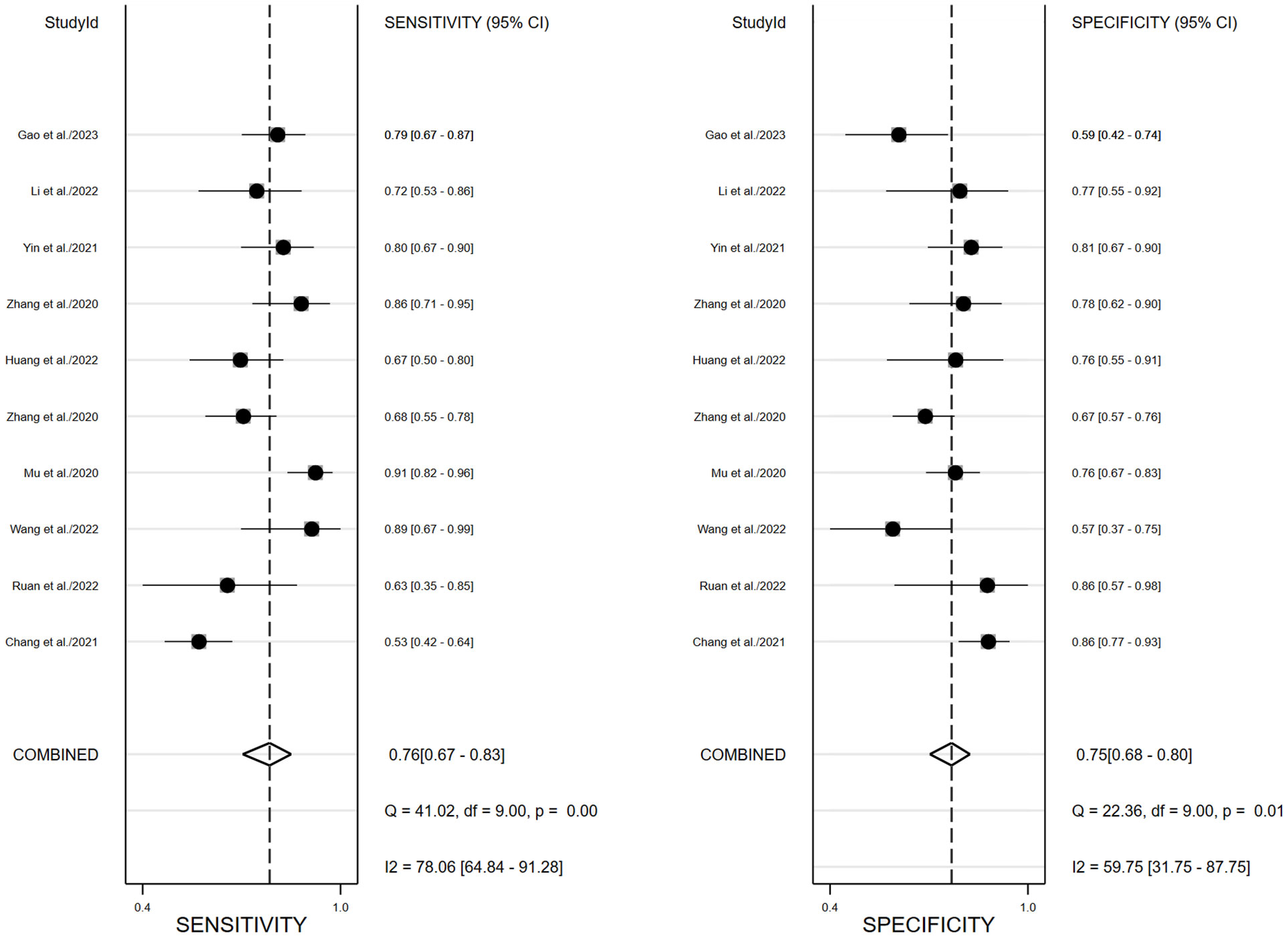
Figure 4 Forest plots of pooled sensitivity and specificity of 18F-FDG PET/CT radiomics diagnostic performance of predicting EGFR mutations in NSCLC patients for validation cohort.
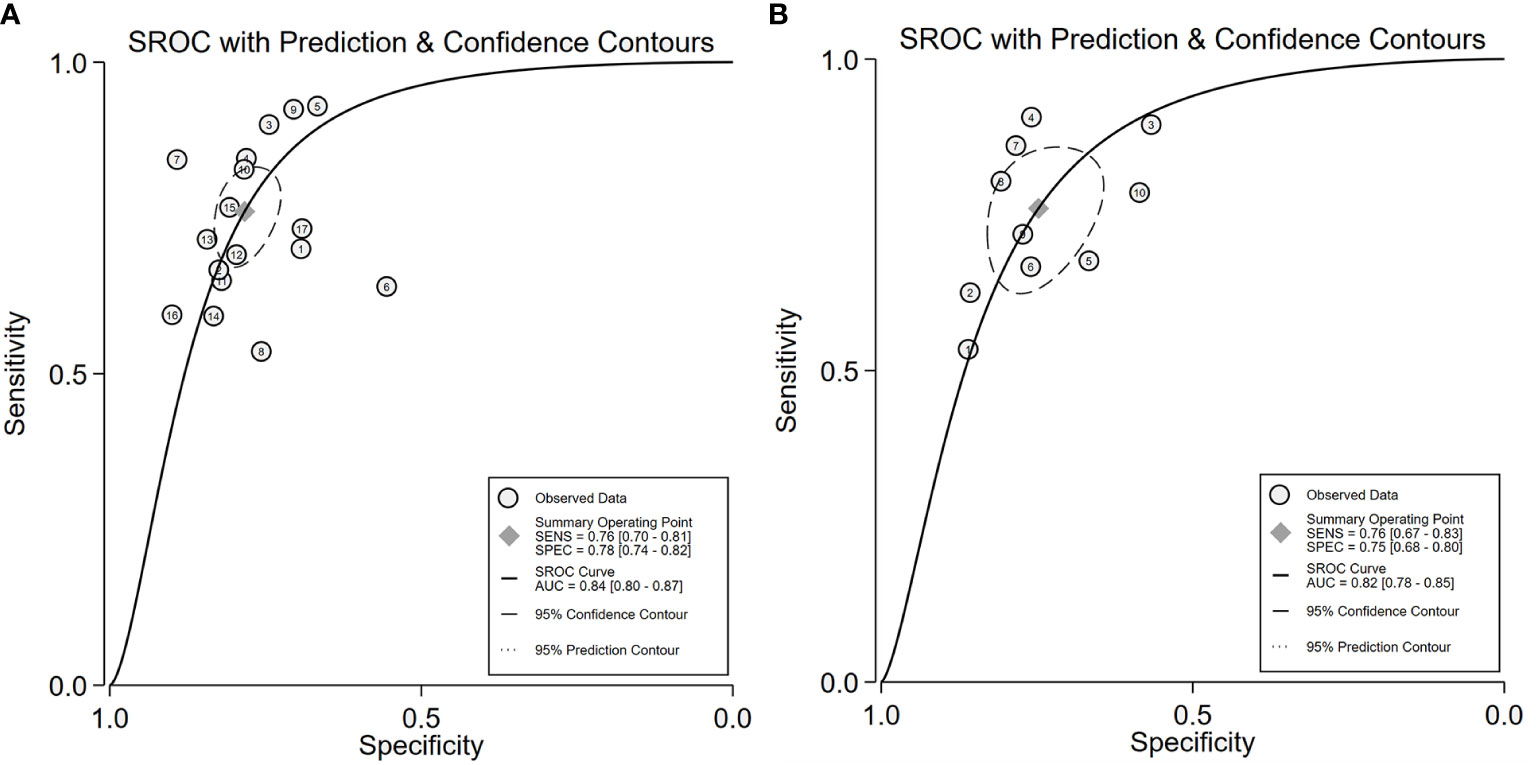
Figure 5 SROC of for the 18F-FDG PET/CT radiomics for the prediction of EGFR mutation status in NSCLC, In both training (A) and validation cohorts (B).
3.4 Sensitivity analysis and publication bias
In the included study, the Deek’s test was used to investigate potential publication bias; however, the funnel chart asymmetry test did not show significant publication bias in both training cohorts (t=0.33, p=0.75, Figure 6A) or validation t=0.01, p=0.99, Figure 6B). We deleted each study individually and combined the rest of the studies to summarize the effect again. The results show that the comprehensive effect of each index changes little, indicating that the stability of the literature is good and the reliability of the results is high (Supplementary Figure S4).
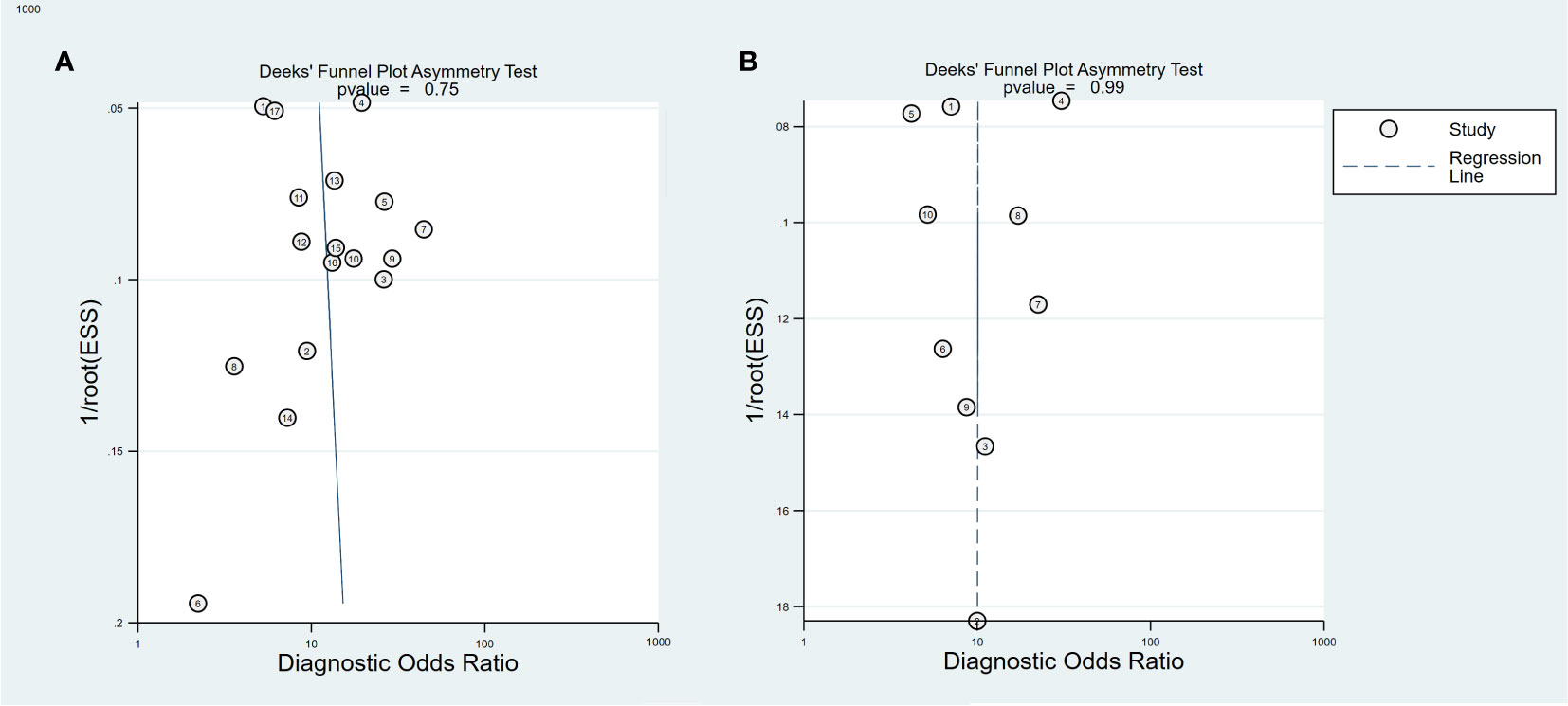
Figure 6 Deeks funnel plot for the publication bias test of 18F-FDG PET/CT radiomics for the prediction of EGFR mutation status in NSCLC, In both training (A) and validation cohorts (B).
3.5 Meta-regression subgroup analysis
To elucidate heterogeneity causes, we conducted a meta-regression analysis, detailed in Figure 7. Modeling method and region emerged as significant heterogeneity factors, with respective p-values of 0.01 and 0.04. Subgroup analysis indicated that studies (n=10) covering both ADC and other NSCLC subtypes showed enhanced sensitivity (78% vs. 73%, p=0.06) and specificity (81% vs. 75%, p<0.01) compared to ADC-only studies (n=7). Deep learning studies (n=4) outperformed radiomics algorithm studies (n=13) in specificity (85% vs. 74%, p<0.01). Blinded studies (n=7) achieved higher specificity (82% vs. 70%, p=0.10) compared to those with unclear blinding (n=10). Intriguingly, studies utilizing Pyradiomics for feature extraction (n=5) demonstrated superior sensitivity (82% vs. 73%, p=0.15) compared to those employing other software (n=6). Higher RQS studies (n=11) demonstrated increased sensitivity (77%) and specificity (81%) over lower RQS studies (n=6; sensitivity: 74%, p=0.06; specificity: 79%, p<0.01). Larger sample sizes (≥130) were associated with higher sensitivity (78% vs.72%,p=0.57) and specificity (79% vs.78%, p<0.01). Studies with fewer smokers (<100, n=12) outperformed those with more smokers (≥100, n=4) in both sensitivity (75% vs. 73%, p=0.02) and specificity (80% vs. 77%, p<0.01). Similarly, studies with a higher proportion of female participants (n=12) reported better sensitivity (78% vs. 69%, p=0.31) and specificity (78% vs. 77%, p<0.01) than those with fewer females (n=5). Notably, studies integrating clinical data in their radiomics models (n=9) achieved higher specificity (80% vs. 76%, p<0.01) in EGFR mutation prediction.
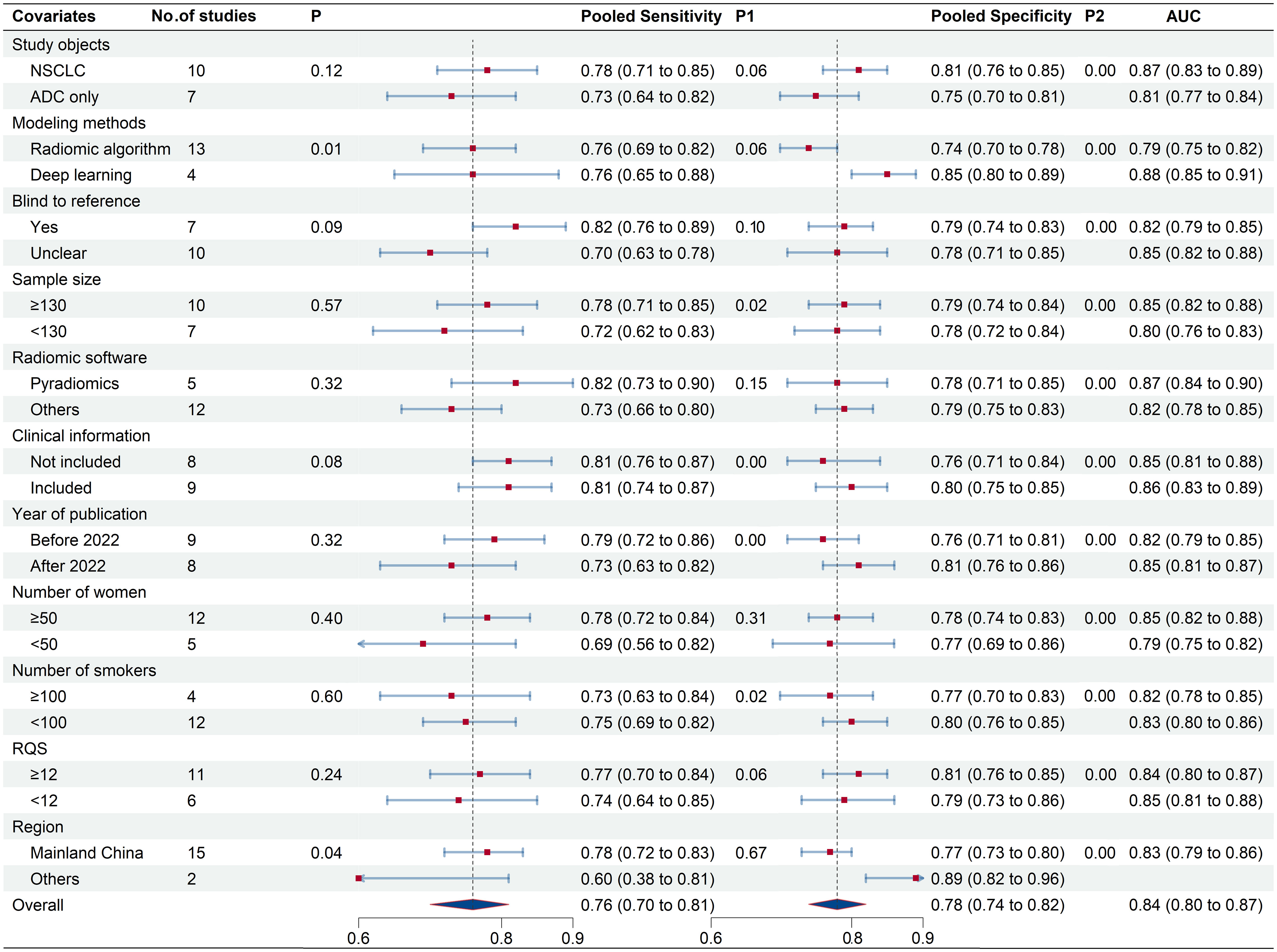
Figure 7 Meta-regression results of 18F-FDG PET/CT radiomics for prediction of EGFR mutation in NSCLC.
3.6 Clinical utility
The Fagan plot analysis for the training cohort (Figure 8A) demonstrates that 18F-FDG PET/CT-based radiomics increased the post-test probability of an EGFR mutation prediction from 20% to 47% with a positive likelihood ratio (PLR) of 5. Conversely, a negative pre-test reduced the post-test probability to 6% with a negative likelihood ratio (NLR) of 0.31. Comparable outcomes were observed in the validation cohort (Figure 8B).
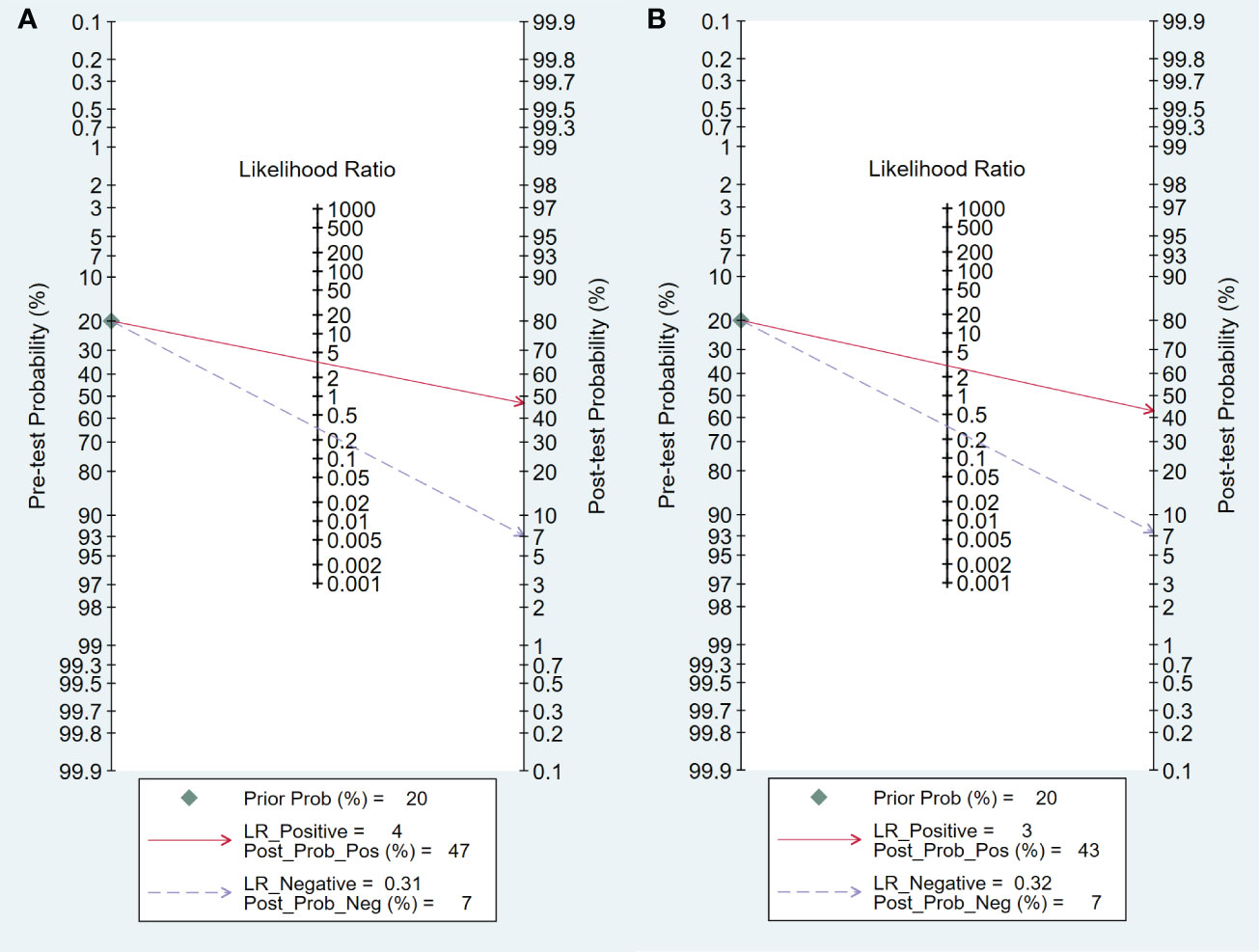
Figure 8 Fagan plots for assessing the clinical utility, In both training (A) and validation (B) cohorts.
4 Discussion
EGFR gene is a critical factor in determining the treatment and prognosis of patients with non-small cell lung cancer (NSCLC). EGFR has received increasing attention in recent years as it is frequently overexpressed and directly associated with prolonged survival. EGFR tyrosine kinase inhibitors (TKIs) are more effective in patients with EGFR-mutated NSCLC. Medical imaging techniques such as CT and PET scans are more cost-effective and convenient than biopsies for predicting mutation status. Nguyen et al. first systematic review to the diagnostic accuracy of AI-based radiomics algorithms in predicting EGFR mutation status in lung cancer. The results showed satisfactory diagnostic accuracy with an overall AUC value of 0.789 (31). The combined sensitivity and specificity were 72.2% and 73.3%, respectively. Subgroup analysis revealed that diagnostic performance can be improved by using the radiomics model of PET/CT.
In this review, we detailed synthesize findings from 18F-FDG PET/CT radiomics studies targeting EGFR mutation prediction in non-small cell lung cancer. The results of the 17 training cohorts showed that the 18F-FDG PET/CT radiomics method was promising for EGFR mutation prediction with a combined sensitivity, specificity, and AUC of 0.74, 0.78, and 0.84, respectively. The corresponding values for the ten independent validation cohorts were 0.76, 0.75, and 0.82, respectively. The results of our study indicated that 18F-FDG PET/CT radiomics model can enhance the precision in identifying EGFR mutations in NSCLC patients, aiding clinicians in tailoring treatment regimens, and potentially improving patient outcomes.
The training cohort exhibited significant heterogeneity, necessitating an exploration of its sources. To begin with, threshold effects were considered as they can lead to an overestimation of diagnostic performance. Spearman’s correlation coefficient was evaluated to eliminate the possibility of threshold effects. The results indicated that threshold effects were unlikely to be a source of heterogeneity (r=0.316, p=0.216). Besides, in evidence-based medicine, publication bias can significantly influence the results of meta-analysis, potentially leading to distorted or misleading conclusions. We used Deek’s funnel plot to assess publication bias in the included literature. Despite the subjective limitations of Deek’s funnel plot, our observation of a statistically insignificant slope coefficient suggests a low probability of publication bias. We conducted the sensitivity analysis that confirmed the stability of the included literature and the reliability of our findings. Lastly, we analyzed other potential sources of heterogeneity through univariate meta-regression and identified several relevant variables.
Recent systematic evaluations have revealed that the predictive performance of radiomics models in lung cancer is influenced by the inclusion of varying radiomics algorithms and clinical features (31, 32), which explains the heterogeneity of the results based on meta-regression. This variation underpins the heterogeneity observed in our meta-analysis. Notably, in predicting EGFR mutations in lung cancer, deep learning methodologies demonstrate superior performance over conventional machine learning techniques. This enhanced efficacy can be attributed to the advanced capabilities of deep learning models. Unlike traditional machine learning, deep learning can process original imaging data through intricate linear and non-linear transformations utilizing a complex, multi-layer neural network. This approach allows for the extraction of more sophisticated and potentially revealing features from the images. Additionally, deep learning algorithms bypass the need for labor-intensive tumor edge annotation. It can directly learn from the original images, effectively eliminating the requirement for complex preliminary steps such as detailed tumor boundary segmentation, feature extraction, and selection, simplifying the overall process of model development (21). EGFR mutations exhibit a robust association with clinicopathological features, including gender, smoking status, and pathological type. Our subgroup analysis aligns with prior research (33, 34). These results underscore the efficacy of integrating radiomics with clinical data in improving the precision of EGFR mutation predictions. Enriching the model with additional clinical parameters can further elevate diagnostic accuracy of 18F-FDG PET/CT radiomics.
Divergences in radiomics feature extraction software can introduce biases in research outcomes. Our subgroup analysis reveals that studies utilizing Pyradiomics for feature extraction demonstrated superior diagnostic efficacy in identifying EGFR mutations, compared to those using alternative software. This variability stems from the distinct algorithmic methodologies and parameter configurations inherent to each software. These findings emphasize the critical influence of feature extraction software selection on study results, underscoring the necessity for clear recognition, comprehension, and transparent reporting of the software’s impact in radiomics research.
Sample size and blinding to reference standards are pivotal in meta-analyses, significantly influencing study quality and contributing to heterogeneity. Adequate sample sizes and reference blinding enhance study reliability and interpretability, while effectively mitigating selection bias. Our analysis indicates that studies blinded to reference standards exhibited notably lower specificity compared to those where blinding status was ambiguous, potentially due to measurement bias in readers aware of the reference standard (8). Furthermore, studies with large sample sizes demonstrated superior diagnostic performance than those with smaller cohorts. Consequently, sample sizes and ensuring blinding to reference standards are critical steps for enhancing the diagnostic accuracy of 18F-FDG PET/CT radiomics in identifying EGFR mutations.
The dynamic progression of study typically places greater emphasis on recent studies for their applicability to contemporary clinical practice. In our subgroup analysis, studies published after 2022 show improved predictive accuracy for EGFR mutations, possibly due to advancements in imaging equipment, radiomics algorithms, and enhanced study quality. (35). Recognizing and addressing these temporal variations is crucial for deriving precise and relevant meta-analysis conclusions.
The quality of the studies included in this review was assessed using QUADAS-2 and RQS. QUADAS-2 was used for diagnostic accuracy studies, while RQS was used for quality assessment of radiomics studies. QUADAS-2 revealed high or unclear risks in reference standards, flow, and timing in many studies. Over two-thirds had unclear risks due to non-specifics about participant sampling methods, and three studies showed high selection bias risk from inappropriate exclusions. The timing of 18F-FDG PET/CT relative to biopsy, a crucial factor in flow and timing domains, was often ambiguously reported, leading to unclear temporal domain risks. Nevertheless, patient selection, index testing, and reference standards generally had low applicability concerns, indicating a broadly acceptable quality of the included studies.
It is similar to recent systematic evaluations of the quality of radiomics studies in other fields, the median RQS score of included studies was 12 (33.3% of the total score), which is overall relatively low (31, 36). In radiomics research, the RQS critically influences study results. Studies that garner low RQS ratings are often plagued by methodological defects. These deficiencies can introduce significant biases into the meta-analysis, consequently diminishing the reliability and validity of the research findings. The methodological rigor of the included studies was inconsistent. A notable absence of prospective designs and, often, multicenter independent validation cohorts hindered the assessment of model reproducibility. Furthermore, limited biological relevance in investigations and a scarcity of publicly open data could impede a thorough understanding of how 18F-FDG PET/CT radiomic features influence EGFR prediction. Our meta-regression found no significant link between the RQS and result heterogeneity. While study quality metrics are important, low RQS scores should be interpreted as indicators of areas for improvement rather than outright poor quality. Notably, deep learning studies may be disadvantaged by RQS, which is more suited to hand-crafted radiomics (21), indicating RQS might not be the optimal tool for radiomics quality assessment. In contrast, new checklists like CLEAR have shown efficacy in reporting radiomics modeling components, which provides a more thorough coverage of study aspects, enhancing the comprehensive evaluation of study quality and reliability in radiomics research (37).
This study has several limitations. Predominantly, the population analyzed was Chinese, with only two of the 17 studies based in the USA and Canada, while the rest were conducted in China. This led to significant geographical variations contributing to the heterogeneity of our results. EGFR mutations were found in 15.4% of North American NSCLC patients, in contrast to 49.1% in Asian patients, highlighting substantial regional differences (38). Moreover, the retrospective nature of all included studies underscores the need for prospective research to enhance findings’ quality and relevance. Additionally, the exclusion of gray literature and studies with inadequate data may result in biased meta-analysis, necessitating careful consideration in study selection and analysis to ensure the validity and accuracy of the results.
In conclusion, our meta-analysis demonstrates that 18F-FDG PET/CT radiomics is a promising tool for predicting EGFR mutations in NSCLC. Deep learning algorithms particularly stand out, offering enhanced predictive accuracy. However, the pooled AUCs for both validation and training cohorts in our study fall below 0.90, suggesting that this field is still in its developmental phase. This early stage of research limits the wider clinical application of these noninvasive methods for EGFR mutation assessment. Therefore, the development of more advanced deep learning features based on 18F-FDG PET/CT is essential for improving the predictive accuracy for EGFR mutations in NSCLC.
Data availability statement
The original contributions presented in the study are included in the article/Supplementary Material, further inquiries can be directed to the corresponding author.
Author contributions
NM: Conceptualization, Methodology, Writing – original draft, Writing – review & editing. WY: Writing – original draft, Data curation. QW: Data curation, Writing – review & editing. CC: Writing – review & editing, Methodology. YH: Writing – review & editing. ZW: Funding acquisition, Supervision, Writing – review & editing.
Funding
The author(s) declare financial support was received for the research, authorship, and/or publication of this article. This work was supported by National Natural Science Foundation (Grant No.81971655) and Postgraduate Education Innovation Program of Shanxi Province (Grant No.2022Y437).
Conflict of interest
The authors declare that the research was conducted in the absence of any commercial or financial relationships that could be construed as a potential conflict of interest.
Publisher’s note
All claims expressed in this article are solely those of the authors and do not necessarily represent those of their affiliated organizations, or those of the publisher, the editors and the reviewers. Any product that may be evaluated in this article, or claim that may be made by its manufacturer, is not guaranteed or endorsed by the publisher.
Supplementary material
The Supplementary Material for this article can be found online at: https://www.frontiersin.org/articles/10.3389/fonc.2024.1281572/full#supplementary-material
References
1. Siegel RL, Miller KD, Wagle NS, Jemal A. Cancer statistics, 2023. CA: A Cancer J Clin (2023) 73(1):17–48. doi: 10.3322/caac.21763
2. Zheng R, Zhang S, Zeng H, Wang S, Sun K, Chen R, et al. Cancer incidence and mortality in China. J Natl Cancer Center (2022) 2(1):1–9. doi: 10.1016/j.jncc.2022.02.002
3. Jia Y, Yun CH, Park E, Ercan D, Manuia M, Juarez J, et al. Overcoming egfr(T790m) and egfr(C797s) resistance with mutant-selective allosteric inhibitors. Nature (2016) 534(7605):129–32. doi: 10.1038/nature17960
4. Gainor JF, Varghese AM, Ou S-HI, Kabraji S, Awad MM, Katayama R, et al. Alk rearrangements are mutually exclusive with mutations in egfr or kras: an analysis of 1,683 patients with non–small cell lung cancer. Clin Cancer Res (2013) 19(15):4273–81. doi: 10.1158/1078-0432.CCR-13-0318
5. Sasaki R, Komaki R, Macapinlac H, Erasmus J, Allen P, Forster K, et al. [18f]Fluorodeoxyglucose uptake by positron emission tomography predicts outcome of non–small-cell lung cancer. J Clin Oncol (2005) 23(6):1136–43. doi: 10.1200/jco.2005.06.129
6. China GOoNHCotPsRo. Clinical practice guideline for primary lung cancer(2022 version). Med J Peking Union Med Coll Hosp (2022) 13(4):549–70. doi: 10.12290/xhyxzz.2022-0352
7. Bulin D, Shu W, Yan C, Guanghui L, Xuena L, Yaming L. Can 18F-FDG PET/CT predict egfr status in patients with non-small cell lung cancer? A systematic review and meta-analysis. BMJ Open (2021) 11(6):e044313. doi: 10.1136/bmjopen-2020-044313
8. Guo Y, Zhu H, Yao Z, Liu F, Yang D. The diagnostic and predictive efficacy of 18F-FDG PET/CT metabolic parameters for egfr mutation status in non-small-cell lung cancer: A meta-analysis. Eur J Radiol (2021) 141:109792. doi: 10.1016/j.ejrad.2021.109792
9. Bi WL, Hosny A, Schabath MB, Giger ML, Birkbak NJ, Mehrtash A, et al. Artificial intelligence in cancer imaging: clinical challenges and applications. CA: A Cancer J Clin (2019) 69(2):127–57. doi: 10.3322/caac.21552
10. Yang B, Ji H, Zhong J, Ma L, Zhong J, Dong H, et al. Value of 18F-FDG PET/CT-based radiomics nomogram to predict survival outcomes and guide personalized targeted therapy in lung adenocarcinoma with egfr mutations. Front Oncol (2020) 10:567160. doi: 10.3389/fonc.2020.567160
11. Yin G, Wang Z, Song Y, Li X, Chen Y, Zhu L, et al. Prediction of egfr mutation status based on 18F-FDG PET/CT imaging using deep learning-based model in lung adenocarcinoma. Front Oncol (2021) 11:709137. doi: 10.3389/fonc.2021.709137
12. Whiting F, Rutjes AW, Westwood ME, Mallett S, Deeks JJ, Reitsma JB, et al. Quadas-2: A revised tool for the quality assessment of diagnostic accuracy studies. Ann Internal Med (2011) 155(8):529–36. doi: 10.7326/0003-4819-155-8-201110180-00009
13. Lambin P, Leijenaar RTH, Deist TM, Peerlings J, de Jong EEC, van Timmeren J, et al. Radiomics: the bridge between medical imaging and personalized medicine. Nat Rev Clin Oncol (2017) 14(12):749–62. doi: 10.1038/nrclinonc.2017.141
14. Leeflang MMG, Deeks JJ, Takwoingi Y, Macaskill P. Cochrane diagnostic test accuracy reviews. Systematic Rev (2013) 2(1):82. doi: 10.1186/2046-4053-2-82
15. Chang C, Zhou S, Yu H, Zhao W, Ge Y, Duan S, et al. A clinically practical radiomics-clinical combined model based on pet/ct data and nomogram predicts egfr mutation in lung adenocarcinoma. Eur Radiol (2021) 31(8):6259–68. doi: 10.1007/s00330-020-07676-x
16. Ruan D, Fang J, Teng X. Efficient 18f-fluorodeoxyglucose positron emission tomography/computed tomography-based machine learning model for predicting epidermal growth factor receptor mutations in non-small cell lung cancer. Q J Nucl Med Mol Imaging (2022). doi: 10.23736/s1824-4785.22.03441-0
17. Wang J, Lv X, Huang W, Quan Z, Li G, Wu S, et al. Establishment and optimization of radiomics algorithms for prediction of kras gene mutation by integration of nsclc gene mutation mutual exclusion information. Front Pharmacol (2022) 13:862581. doi: 10.3389/fphar.2022.862581
18. Mu W, Jiang L, Zhang J, Shi Y, Gray JE, Tunali I, et al. Non-invasive decision support for nsclc treatment using pet/ct radiomics. Nat Commun (2020) 11(1):5228. doi: 10.1038/s41467-020-19116-x
19. Zhang M, Bao Y, Rui W, Shangguan C, Liu J, Xu J, et al. Performance of 18F-FDG PET/CT radiomics for predicting egfr mutation status in patients with non-Small cell lung cancer. Front Oncol (2020) 10:568857. doi: 10.3389/fonc.2020.568857
20. Hanbing L, Jie Z, Chunfeng H, Yankai M. The application of pet/ct combined texture analysis in predicting egfr gene mutation in non-small cell lung cancer. J Clincal Radiol (2020) 39(09):1759–63. doi: 10.13437/j.cnki.jcr.2020.09.018
21. Huang W, Wang J, Wang H, Zhang Y, Zhao F, Li K, et al. Pet/ct based egfr mutation status classification of nsclc using deep learning features and radiomics features. Front Pharmacol (2022) 13:898529. doi: 10.3389/fphar.2022.898529
22. Zhao HY, Su YX, Zhang LH, Fu P. Prediction model based on 18F-FDG PET/CT radiomic features and clinical factors of egfr mutations in lung adenocarcinoma. Neoplasma (2022) 69(1):233–41. doi: 10.4149/neo_2021_201222N1388
23. Yang T, Yin Z, Shuyi L, Zehui L, Hubing W, Quanshi W. Ability of 18F-FDG PET/CT radiomic features to differentiate egfr mutation status in patients with lung adenocarcinoma. Chinsese J Nucl Med Mol Imaging (2021) 41(2):65–70. doi: 10.3760/cma.j.cn321828-20191108-00255
24. Li XF, Yin GT, Zhang YF, Dai D, Liu JJ, Chen PH, et al. Predictive power of a radiomic signature based on F-18-fdg pet/ct images for egfr mutational status in nsclc. Front IN Oncol (2019) 9:1062. doi: 10.3389/fonc.2019.01062
25. Zhang J, Zhao X, Zhao Y, Zhang J, Zhang Z, Wang J, et al. Value of pre-therapy 18F-FDG PET/CT radiomics in predicting egfr mutation status in patients with non-small cell lung cancer. Eur J Nucl Med Mol Imaging (2020) 47(5):1137–46. doi: 10.1007/s00259-019-04592-1
26. Wang Z, Xingchi C, Jingbai H, Dasheng Q. Predictive power of radiomic features combined with clinical features for gene mutations in non-small cell lung cancer patients. J Clincal Radiol (2019) 38(06):1033–7. doi: 10.13437/j.cnki.jcr.2019.06.022
27. Nair JKR, Saeed UA, McDougall CC, Sabri A, Kovacina B, Raidu BVS, et al. Radiogenomic models using machine learning techniques to predict egfr mutations in non-small cell lung cancer. Can Assoc Radiologists J (2021) 72(1):109–19. doi: 10.1177/0846537119899526
28. Li S, Li Y, Zhao M, Wang P, Xin J. Combination of 18f-fluorodeoxyglucose pet/ct radiomics and clinical features for predicting epidermal growth factor receptor mutations in lung adenocarcinoma. Korean J Radiol (2022) 23(9):921–30. doi: 10.3348/kjr.2022.0295
29. Chen S, Han X, Tian G, Cao Y, Zheng X, Li X, et al. Using stacked deep learning models based on pet/ct images and clinical data to predict egfr mutations in lung cancer. Front Med (2022) 9:1041034. doi: 10.3389/fmed.2022.1041034
30. Gao J, Niu R, Shi Y, Shao X, Jiang Z, Ge X, et al. The predictive value of [18f]Fdg pet/ct radiomics combined with clinical features for egfr mutation status in different clinical staging of lung adenocarcinoma. EJNMMI Res (2023) 13(1):26. doi: 10.1186/s13550-023-00977-4
31. Nguyen HS, Ho DKN, Nguyen NN, Tran HM, Tam KW, Le NQK. Predicting egfr mutation status in non-small cell lung cancer using artificial intelligence: A systematic review and meta-analysis. Acad Radiol (2023). doi: 10.1016/j.acra.2023.03.040
32. Tabnak P, HajiEsmailPoor Z, Baradaran B, Pashazadeh F, Aghebati Maleki L. MRI-based radiomics methods for predicting ki-67 expression in breast cancer: A systematic review and meta-analysis. Academic Radiol (2023). doi: 10.1016/j.acra.2023.10.010
33. Le VH, Kha QH, Minh TNT, Nguyen VH, Le VL, Le NQK. Development and validation of ct-based radiomics signature for overall survival prediction in multi-organ cancer. J digital Imaging (2023) 36(3):911–22. doi: 10.1007/s10278-023-00778-0
34. Liu L, Xiong X. Clinicopathologic features and molecular biomarkers as predictors of epidermal growth factor receptor gene mutation in non-small cell lung cancer patients. Curr Oncol (Toronto Ont) (2021) 29(1):77–93. doi: 10.3390/curroncol29010007
35. Jia LL, Zhao JX, Zhao LP, Tian JH, Huang G. Current status and quality of radiomic studies for predicting kras mutations in colorectal cancer patients: A systematic review and meta−Analysis. Eur J Radiol (2023) 158:110640. doi: 10.1016/j.ejrad.2022.110640
36. Chen Q, Zhang L, Mo X, You J, Chen L, Fang J, et al. Current status and quality of radiomic studies for predicting immunotherapy response and outcome in patients with non-small cell lung cancer: A systematic review and meta-analysis. Eur J Nucl Med Mol Imaging (2021) 49(1):345–60. doi: 10.1007/s00259-021-05509-7
37. Kocak B, Baessler B, Bakas S, Cuocolo R, Fedorov A, Maier-Hein L, et al. Checklist for evaluation of radiomics research (Clear): A step-by-step reporting guideline for authors and reviewers endorsed by esr and eusomii. Insights into Imaging (2023) 14(1):75. doi: 10.1186/s13244-023-01415-8
Keywords: non-small cell lung cancer, EGFR mutation, 18F-FDG PET/CT, meta-analysis, radiomics
Citation: Ma N, Yang W, Wang Q, Cui C, Hu Y and Wu Z (2024) Predictive value of 18F-FDG PET/CT radiomics for EGFR mutation status in non-small cell lung cancer: a systematic review and meta-analysis. Front. Oncol. 14:1281572. doi: 10.3389/fonc.2024.1281572
Received: 22 August 2023; Accepted: 15 January 2024;
Published: 01 February 2024.
Edited by:
Xin Tang, Hangzhou Wuyunshan Hospital, ChinaReviewed by:
Jingmian Zhang, The Fourth Hospital of Hebei Medical University, ChinaNguyen Quoc Khanh Le, Taipei Medical University, Taiwan
Copyright © 2024 Ma, Yang, Wang, Cui, Hu and Wu. This is an open-access article distributed under the terms of the Creative Commons Attribution License (CC BY). The use, distribution or reproduction in other forums is permitted, provided the original author(s) and the copyright owner(s) are credited and that the original publication in this journal is cited, in accordance with accepted academic practice. No use, distribution or reproduction is permitted which does not comply with these terms.
*Correspondence: Zhifang Wu, d3V6aGlmYW5nMDFAMTYzLmNvbQ==