- 1Department of Obstetrics, Guangdong Provincial Key Laboratory of Major Obstetric Diseases, Guangdong Provincial Clinical Research Center for Obstetrics and Gynecology, Guangdong-Hong Kong-Macao Greater Bay Area Higher Education Joint Laboratory of Maternal-Fetal Medicine, The Third Affiliated Hospital of Guangzhou Medical University, Guangzhou, China
- 2Department of Obstetrics and Gynecology, Guangdong Provincial Key Laboratory of Major Obstetric Diseases, Guangdong Provincial Clinical Research Center for Obstetrics and Gynecology, Guangdong-Hong Kong-Macao Greater Bay Area Higher Education Joint Laboratory of Maternal-Fetal Medicine, The Third Affiliated Hospital of Guangzhou Medical University, Guangzhou, China
- 3Department of Clinical Medicine, The Third Clinical School of Guangzhou Medical University, Guangzhou, China
Purpose: This meta-analysis was conducted to assess the diagnostic performance of artificial intelligence (AI) based on imaging for detecting lymph node metastasis (LNM) among cervical cancer patients and to compare its performance with that of radiologists.
Methods: A comprehensive literature search was conducted across PubMed, Embase, and Web of Science to identify relevant studies published up to October 2024. The search followed the Preferred Reporting Items for Systematic Reviews and Meta-Analyses of Diagnostic Test Accuracy (PRISMA-DTA) guidelines. Studies evaluating the accuracy of AI models in detecting LNM in cervical cancer through computed tomography (CT), magnetic resonance imaging (MRI), and positron emission tomography/computed tomography (PET/CT) were included. Pathology served as the reference standard for validation. A bivariate random-effects model was employed to estimate pooled sensitivity and specificity, both presented alongside 95% confidence intervals (CIs). Bias was assessed with the revised Quality Assessment of Diagnostic Accuracy Studies-2 (QUADAS-2) tool. Study heterogeneity was examined through the I2 statistic. Meta-regression was conducted when significant heterogeneity (I2 > 50%) was observed.
Results: A total of 23 studies were included in this meta-analysis. The quality and bias of the included studies were acceptable. However, substantial heterogeneity was observed among the included studies. Internal validation sets comprised 23 studies and 1,490 patients. The pooled sensitivity, specificity, and the area under the curve (AUC) for detecting LNM in cervical cancer were 0.83 (95% CI: 0.78-0.87), 0.78 (95% CI: 0.74-0.82) and 0.87 (95% CI: 0.84-0.90), respectively. External validation sets comprised six studies and 298 patients. The pooled sensitivity, specificity, and AUC for detecting LNM were 0.70 (95% CI: 0.56-0.81), 0.85 (95% CI: 0.66-0.95) and 0.76 (95% CI: 0.72-0.79), respectively. For radiologists, eight studies and 644 patients were included; the pooled sensitivity, specificity, and AUC for detecting LNM were 0.54 (95% CI: 0.42-0.66), 0.79 (95% CI: 0.59-0.91) and 0.65 (95% CI: 0.60-0.69), respectively.
Conclusions: Imaging-based AI demonstrates higher diagnostic performance than radiologists. Prospective studies with rigorous standardization as well as further research with external validation datasets, are necessary to confirm the results and assess their practical clinical applicability.
Systematic Review Registration: https://www.crd.york.ac.uk/PROSPERO, identifier CRD42024607074.
1 Introduction
Cervical cancer is the fourth most prevalent malignancy among women worldwide, with approximately 604,000 new cases and 342,000 deaths reported each year (1). LNM is a critical prognostic factor that significantly influences survival outcomes. Early-stage patients without LNM have a five-year survival rate ranging from 80% to 100%, while this rate declines markedly to 47% to 78% for those with LNM (2). The International Federation of Gynecology and Obstetrics (FIGO) staging system is the primary framework for guiding treatment and management (3). Patients diagnosed with LNM frequently treated with radiotherapy and chemotherapy as the preferred treatment methods (3). Therefore, early, non-invasive assessment of lymph node status is essential for determining optimal treatment plans and prevent unnecessary surgical interventions, ultimately enhancing patient care and outcomes.
Conventional imaging diagnostic methods, including CT, MRI, and PET/CT, have been commonly used for detecting LNM in cervical cancer. However, these techniques have notable limitations. CT and MRI often exhibit restricted sensitivity and specificity, primarily due to their inability to detect normal-sized LNM smaller than 1 cm, making it difficult to identify micrometastases (4, 5). PET/CT, while offering relatively better performance for LNM detection, faces challenges distinguishing between metastatic and hypermetabolic benign lymph nodes (6, 7). Furthermore, its effectiveness in detecting normal-sized LNM and early-stage LNM is constrained, with sensitivity ranging from only 32% to 58% (8). Although pathological examination is often considered the gold standard for LNM detection due to its accuracy, it is not ideal as an initial diagnostic approach because of its invasive nature, procedural complexity, and associated patient risks.
The emergence of AI has transformed the diagnostic landscape for cervical cancer, particularly in predicting LNM (9). Radiomics, an AI-based technique, extracts numerous quantifiable features from medical imaging data to reveal microstructural characteristics of tumors or other tissues not visible to the naked eye (10). Studies have demonstrated that radiomic features derived from MRI, CT, and PET/CT images are effective in predicting LNM in cervical cancer patients (11–13). However, these studies face contradictions due to small sample sizes, limited cross-comparisons of imaging techniques, and challenges in model reproducibility (14). Furthermore, it remains unclear whether AI-based diagnostics methods can outperform the expertise of experienced radiologists in real-world clinical settings (15).
Thus, we conducted a meta-analysis to evaluate the diagnostic performance of different imaging-based AI methods for LNM in cervical cancer patients, and compared their performance with conventional radiologists.
2 Methods
The meta-analysis strictly followed the Preferred Reporting Items for Systematic Reviews and Meta-Analyses of Diagnostic Test Accuracy (PRISMA-DTA) guidelines (16). Additionally, the study protocol is registered with PROSPERO (CRD42024607074).
2.1 Search strategy
We performed a comprehensive search throughout PubMed, Embase, and Web of Science databases, completed on October 7, 2024, with an update on November 2, 2024, to ensure the inclusion of recent studies. The search strategy included three primary terms: “artificial intelligence”, “cervical cancer”, and “lymph node metastasis”, applying both keywords and MeSH terms to optimize coverage (Supplementary Table 1). Only studies published in English, with accessible full text, were eligible for inclusion. Additionally, reference lists of selected articles were manually reviewed to capture further relevant studies.
2.2 Inclusion and exclusion criteria
The inclusion criteria were established based on the PICOS framework. Population (P): Adult cervical cancer patients undergoing LNM evaluation. Intervention (I): Artificial intelligence models utilizing MRI, CT, or PET/CT imaging modalities. Comparison (C): Studies with no comparator or those comparing results with clinicians. Outcome (O): Primary outcomes were sensitivity, specificity, and area under the curve (AUC). Study design (S): Only retrospective and prospective studies were included. Additional criteria required studies to be published in English and to include at least 10 participants.
Exclusion criteria included: (1) irrelevant titles and abstracts; (2) non-eligible publication types, such as reviews, conference abstracts, case reports, and meta-analyses. Studies that did not meet these criteria were excluded to ensure the reliability and quality of data for the meta-analysis.
2.3 Quality assessment
Two reviewers conducted independent assessments of bias using a modified quality assessment tool, resolving disagreements through consensus to ensure rigor and objectivity in the evaluation. To enhance the tool’s relevance, we adapted the original QUADAS-2 by incorporating criteria from the PROBAST (Prediction model Risk of Bias Assessment Tool), targeting potential biases unique to AI-based LNM prediction (17, 18). This revised tool focused on four domains: (1) patient selection, (2) index test (AI algorithm), (3) reference standard, and (4) analysis. Applicability concerns were also assessed within the first three domains.
2.4 Data extraction
Two reviewers independently assessed study eligibility and conducted data extraction, with any disagreements resolved by consensus involving a third reviewer as an adjudicator for accuracy. Extracted data included (1) study details: first author’s name, publication year, study design, country of origin, and reference standard; (2) patient data: number of patients in training, internal and external validation sets, age distribution, and number of patients with positive LNM; (3) AI algorithm details: imaging modality and algorithm type.
2.5 Outcome measures
The primary outcome measures included data from both internal and external validation sets, as well as sensitivity, specificity, and AUC for radiologists. Sensitivity was defined as the ratio of true positives (TP) to the sum of true positives (TP) and false negatives (FN), while specificity was defined as the ratio of true negatives (TN) to the sum of true negatives (TN) and false positives (FP). The AUC, representing the area under the summary receiver operating characteristic (SROC) curve, summarizes the model’s ability to distinguish between positive and negative cases. As a crucial metric for evaluating the accuracy of diagnostic tests, the AUC provides a quantitative measure of performance. Higher values indicate superior diagnostic efficiency and reliability (19). We extracted AI performance data from validation sets, prioritizing the model with the highest AUC. Additionally, radiologists’ diagnostic data were collected for comparative evaluation.
2.6 Statistical analysis
We utilized a bivariate random-effects model to estimate pooled sensitivity and specificity for both imaging-based AI and clinician assessments, each reported with 95% CIs. To evaluate diagnostic accuracy, we used SROC model to generate SROC curves and calculate the AUC. The SROC model integrates diagnostic data from multiple studies, illustrating the trade-off between sensitivity and specificity in diagnostic tests. Each point on the curve represents the result of a specific diagnostic test, with its sensitivity and specificity values visually depicted (19). The Fagan plot was used to explain the link between pre-probability, post-probability, and likelihood ratio, which can estimate the application of imaging-based AI in clinical practice (20). Heterogeneity across studies was evaluated using the I2 statistic, where values of 0%-25%, 25%-50%, 50%-75%, and >75% signified very low, low, moderate, and high heterogeneity, respectively. For internal validation datasets exceeding 10 studies, meta-regression was conducted when high heterogeneity (I2 > 50%) was observed, exploring variables such as imaging type (MRI vs. non-MRI), patient number (>50 vs. ≤50), country (China vs. other countries), and algorithm type (deep learning vs. machine learning). Subgroup analyses were performed for distinct imaging modalities (CT, MRI, and PET/CT).
Publication bias was evaluated using Deeks’ funnel plot asymmetry test, which evaluates bias by examining the symmetry of the funnel plot and performing quantitative analysis (21). Statistical analyses were conducted using Stata 15.1, while study quality was evaluated using RevMan 5.4. Statistical significance was defined as P < 0.05.
3 Results
3.1 Study selection
A comprehensive literature search was conducted across three databases. Initially, 828 articles were identified as potentially eligible through the database search. Following the removal of 318 duplicate records, 510 unique articles remained. Of these, 463 studies were excluded due to failure to meet the inclusion criteria. Full-text reviews were conducted on the remaining 47 articles. Subsequently, 24 studies were excluded due to the inability to extract essential data (TP, TN, FP, FN) (n = 21), non-cervical cancer (n = 1), or non-English full text (n = 2). Ultimately, 23 studies were included in the final meta-analysis (9, 11–13, 22–40). The article selection process is illustrated in Figure 1, following the PRISMA flow diagram format.
3.2 Study description and quality assessment
A total of 23 eligible studies published between 2018 and 2024 were included. The internal validation cohorts across these studies comprised 1,490 patients, with study sample sizes ranging from 28 to 141 participants. Six studies incorporated external validation, involving 298 patients (ranging from 29 to 69) (12, 22, 26, 29, 31, 32); however, one study lacked sufficient data for extraction (22). Eight studies provided comparisons with radiologists, including 644 patients (ranging from 29 to 141) (9, 13, 24–26, 32, 34, 37). All studies were retrospective. The imaging modalities used were predominantly MRI (17 studies) (9, 11, 22–25, 28, 30–37, 39, 40), followed by PET/CT (4 studies) (13, 27, 29, 38), and contrast-enhanced CT (2 studies) (12, 26). Pathology was employed as the reference standard. A summary of patient characteristics is presented in Table 1.
Bias was evaluated using the QUADAS-2-Revised tool, with individual risk assessments illustrated in Figure 2. Five studies were rated as having a “high risk” for patient selection due to inappropriate exclusions (29, 33, 36, 38, 39). Four studies were identified as “high risk” for the index test due to inadequate details regarding the artificial intelligence model (11, 12, 22, 26). Overall, despite some areas of concern, the quality of the included studies was deemed acceptable.
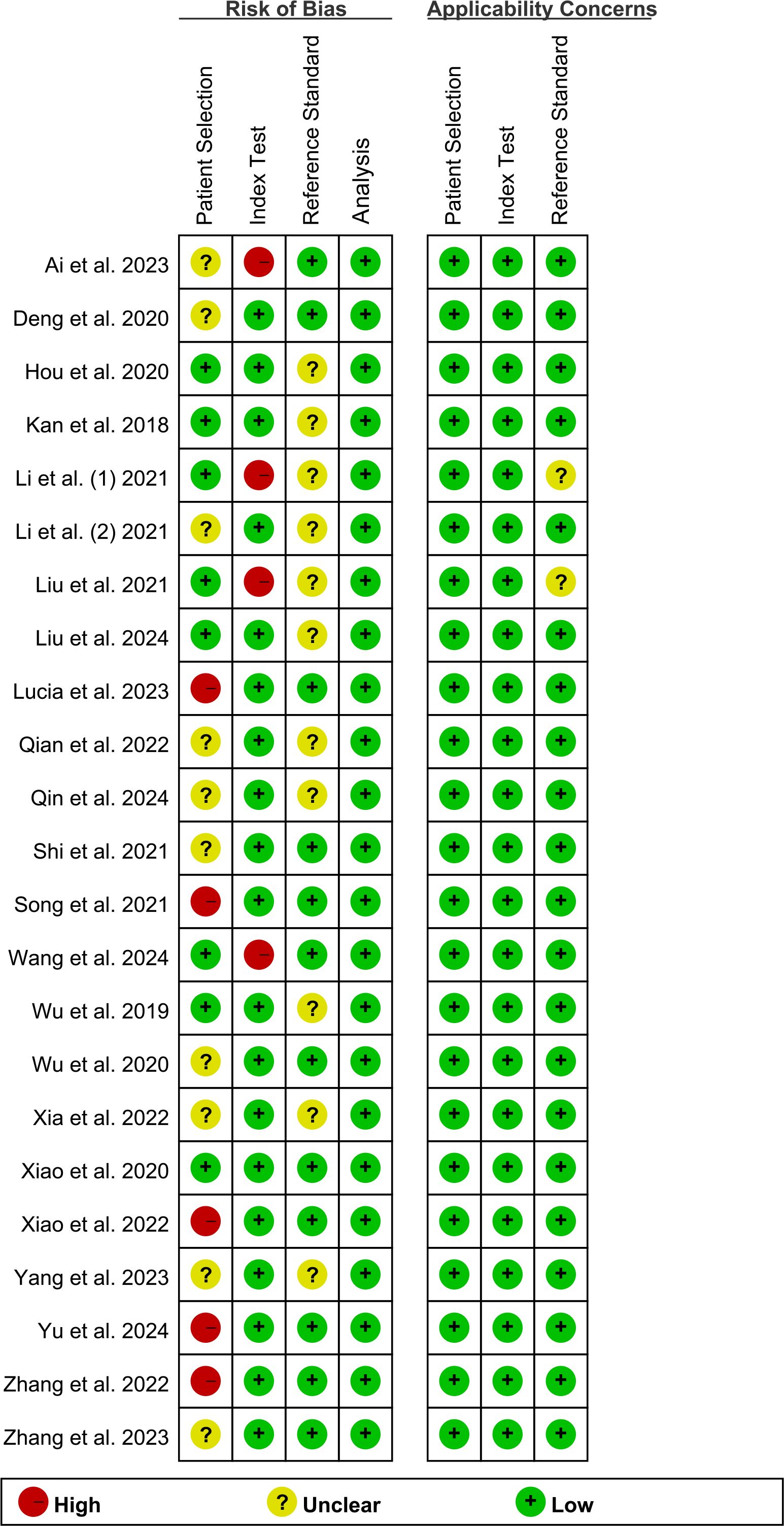
Figure 2. Risk of bias and applicability concerns of the included studies using the Quality Assessment of Diagnostic Performance Studies QUADAS-2 revised tool.
3.3 Diagnostic performance of internal validation set for AI and radiologists in predicting lymph node metastasis of cervical cancer
For internal validation sets, the pooled sensitivity and specificity for detecting LNM in cervical cancer were 0.83 (95% CI: 0.78-0.87) and 0.78 (95% CI: 0.74-0.82), respectively (Figure 3), with an AUC of 0.87 (95% CI: 0.84-0.90) (Figure 4A). With a pre-test probability of 20%, the Fagan nomogram indicates a positive likelihood ratio of 49% and a negative likelihood ratio of 5% (Figure 5A). For radiologists, the sensitivity and specificity for detecting LNM in cervical cancer were 0.54 (95% CI: 0.42-0.66) and 0.79 (95% CI: 0.59-0.91), respectively (Figure 6), with an AUC of 0.65 (95% CI: 0.60-0.69) (Figure 4B). Using the same pre-test probability, the Fagan nomogram indicates a positive likelihood ratio of 39% and a negative likelihood ratio of 13% (Figure 5B). The overall diagnostic performance of internal validation, external validation, and radiologists is summarized in Table 2.
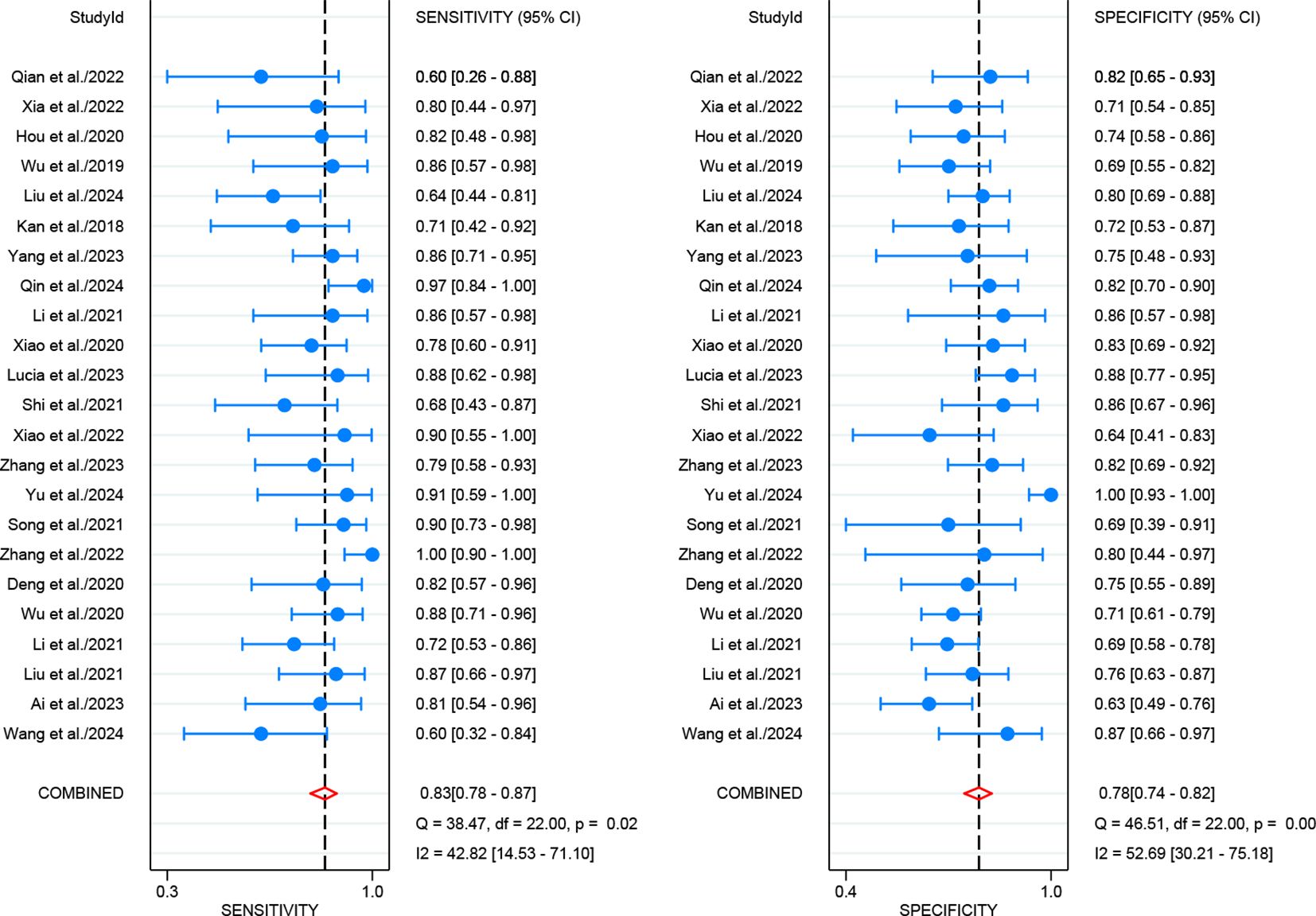
Figure 3. Forest plot of imaging-based artificial intelligence on the internal validation set for diagnosing lymph node metastasis in cervical cancer.
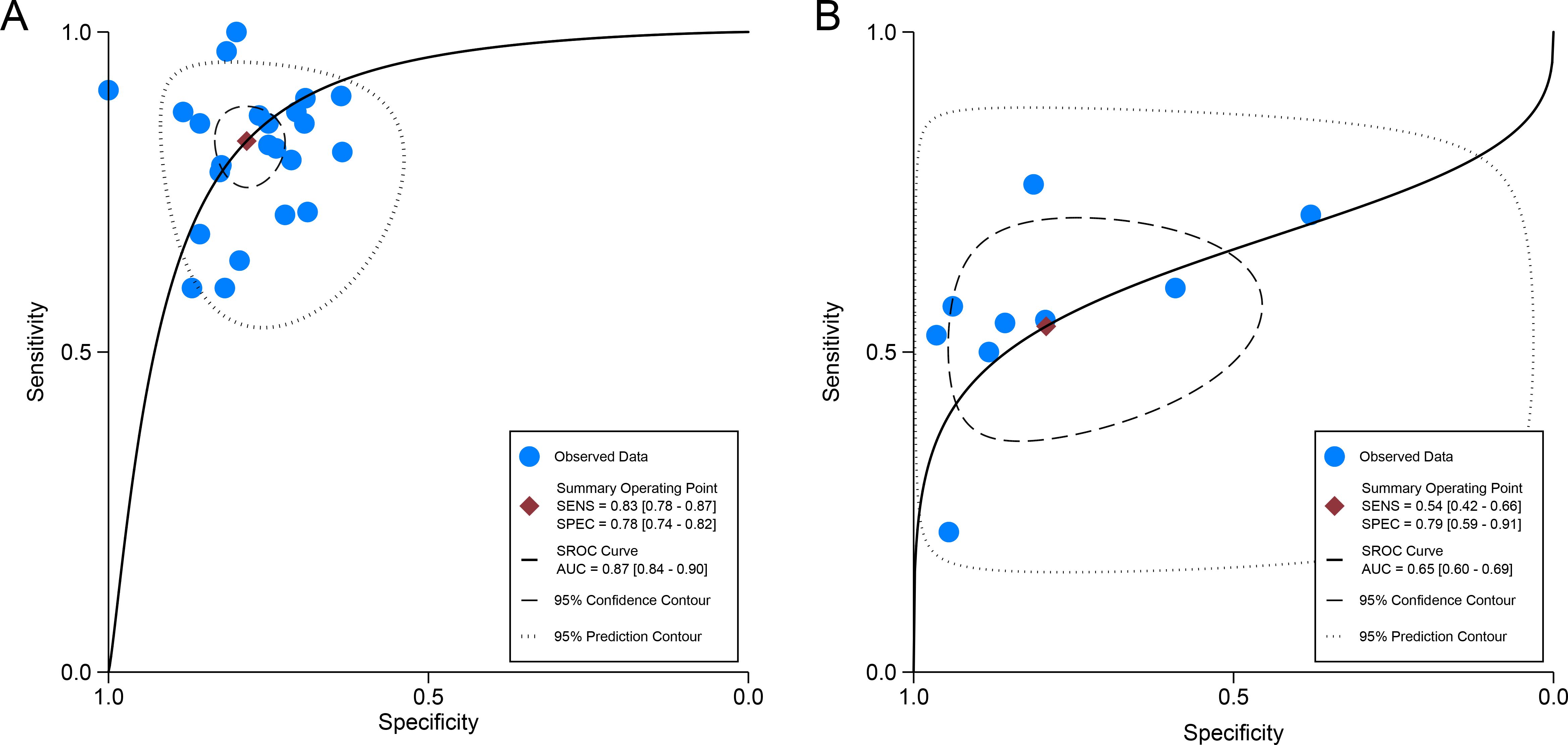
Figure 4. Summary receiver operating characteristic (SROC) curves of imaging-based artificial intelligence on the internal validation set (A) and radiologists (B) for diagnosing lymph node metastasis in cervical cancer.
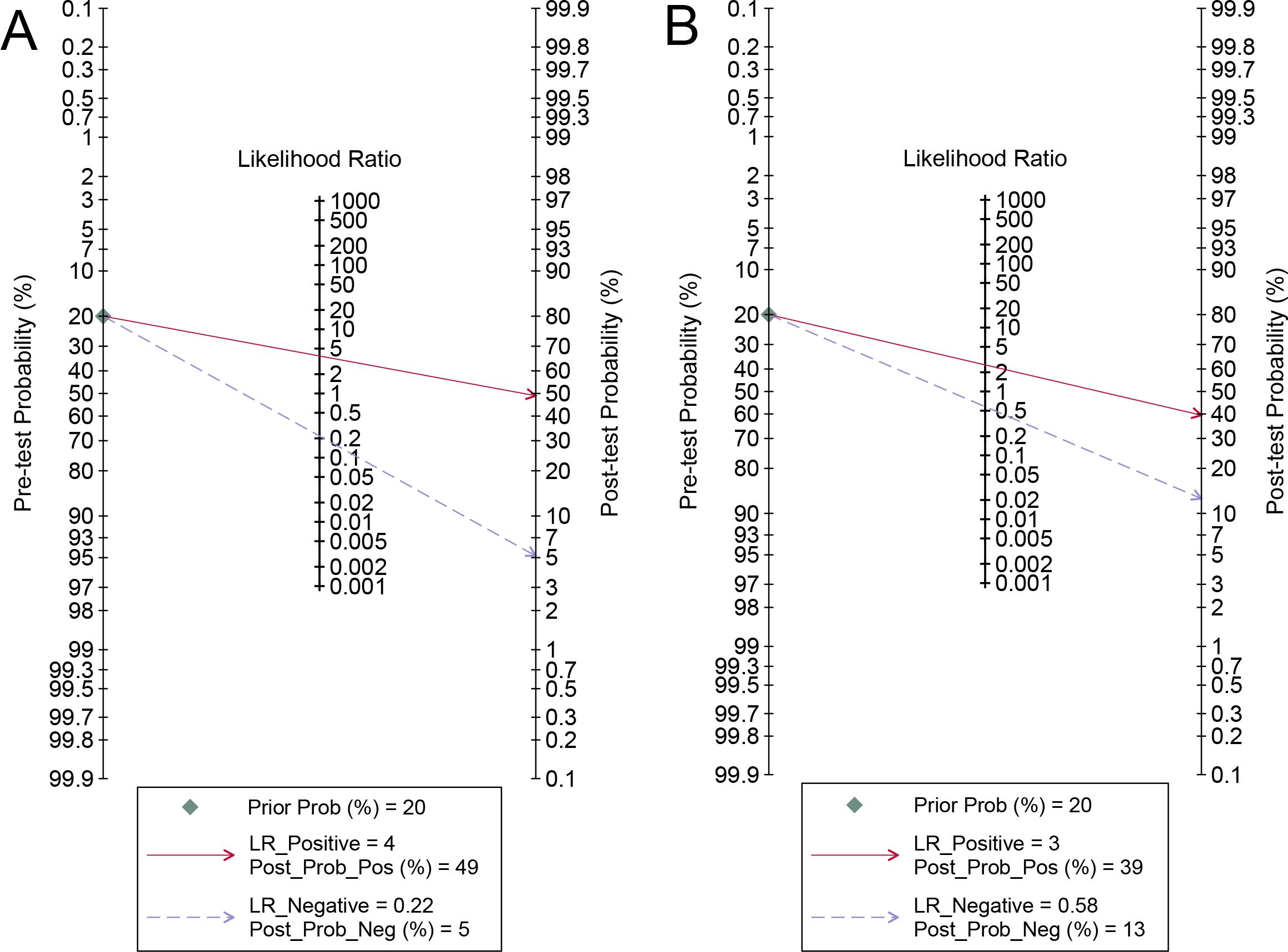
Figure 5. Fagan’s nomogram of imaging-based artificial intelligence on the internal validation set (A) and radiologists (B) for diagnosing lymph node metastasis in cervical cancer.

Table 2. Diagnostic performance of internal validation set, external validation set, and radiologists.
For internal validation sets, moderate heterogeneity was observed for both sensitivity (I2 = 43%) and specificity (I2 = 53%). Meta-regression analysis indicated that heterogeneity was primarily due to differences in the number of patients (>50 vs. ≤50, P = 0.01 for sensitivity, P < 0.001 for specificity). Additionally, algorithm type (deep learning vs. machine learning, P = 0.03 for sensitivity, P < 0.001 for specificity) and imaging modality (MRI vs. non-MRI, P = 0.03 for sensitivity, P = 0.01 for specificity) were also identified as potential sources of heterogeneity for both sensitivity and specificity. These findings are further detailed in Table 3.
3.4 Diagnostic performance of external validation sets for AI in predicting lymph node metastasis in cervical cancer
For external validation sets, the pooled sensitivity and specificity for detecting LNM in cervical cancer were 0.70 (95% CI: 0.56-0.81) and 0.85 (95% CI: 0.66-0.95) (Supplementary Figure 1), with an AUC of 0.76 (95% CI: 0.72-0.79) (Supplementary Figure 2). With a pre-test probability of 20%, the Fagan nomogram demonstrates a positive likelihood ratio of 55% and a negative likelihood ratio of 8% (Supplementary Figure 3).
3.5 Diagnostic performance of different imaging techniques for AI in predicting lymph node metastasis in cervical cancer
For MRI-based AI, 17 studies in internal validation were pooled, the sensitivity in detecting LNM of cervical cancer was 0.82 (95% CI: 0.78-0.86), and the specificity was 0.76 (95% CI: 0.72-0.79), with an AUC of 0.85 (95% CI: 0.81-0.88) (Table 4). Two studies in external validation were pooled; the sensitivity in detecting LNM of cervical cancer was 0.59 (95% CI: 0.41-0.76), and the specificity was 0.86 (95% CI: 0.76-0.94) (Table 4).
For PET/CT-based AI, four studies in internal validation were pooled, the sensitivity in detecting LNM of cervical cancer was 0.87 (95% CI: 0.78-0.93), and the specificity was 0.91 (95% CI: 0.85-0.95), with an AUC of 0.93 (95% CI: 0.88-0.97) (Table 4). However, an analysis of the external validation set could not be performed owing to the unavailability of sufficient data.
For CT-based AI, two studies in internal validation were pooled; the sensitivity in detecting LNM of cervical cancer was 0.78 (95% CI: 0.65-0.87), and the specificity was 0.72 (95% CI: 0.63-0.79) (Table 4). Two studies in external validation were pooled, the sensitivity in detecting LNM of cervical cancer was 0.80 (95% CI: 0.56-0.94), and the specificity was 0.68 (95% CI: 0.57-0.77) (Table 4).
3.6 Publication bias
Deeks’ funnel plot asymmetry test indicated no significant publication bias for the internal validation sets for AI and radiologists (P = 0.69, 0.50) (Figures 7A, B). Similarly, no significant publication bias was identified for the external validation sets (P = 0.18) (Supplementary Figure 4).
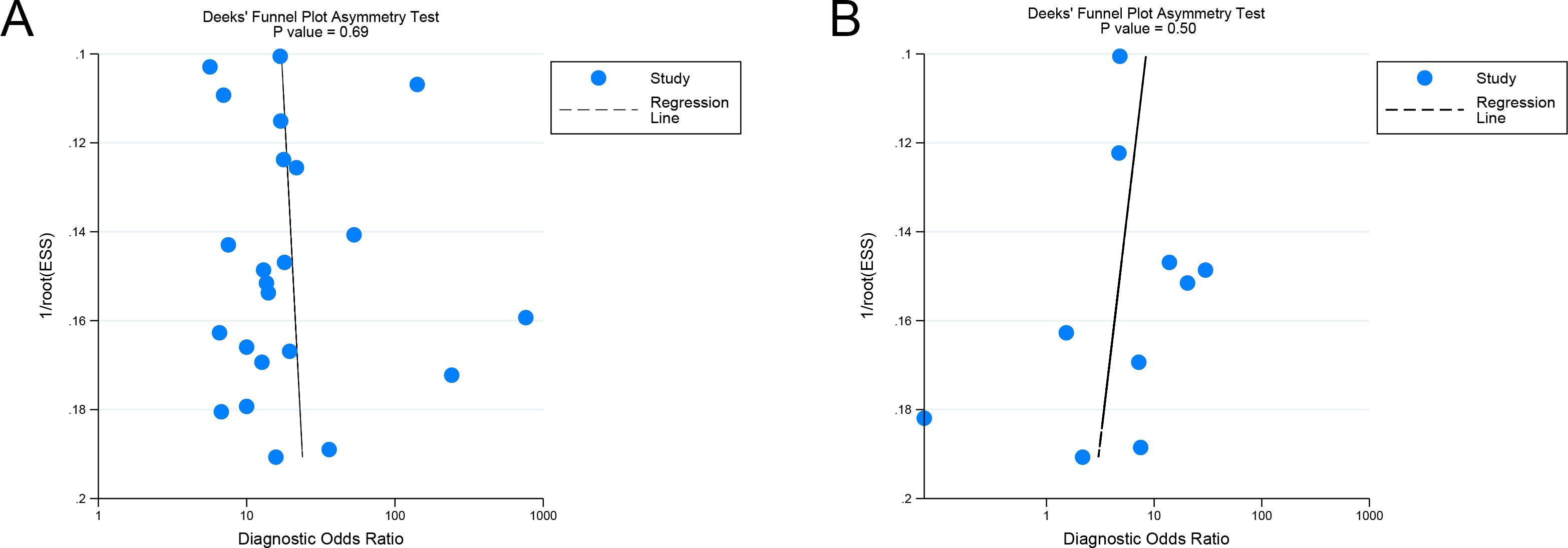
Figure 7. Deek’s funnel plot of imaging-based artificial intelligence on the internal validation set (A) and radiologists (B) for diagnosing lymph node metastasis in cervical cancer.
4 Discussion
In recent years, advancements in AI have led to the development of models aimed at assessing LNM in cervical cancer using imaging techniques, incorporating technologies such as MRI, CT, and PET/CT (12, 25, 29). However, despite this promising integration, the diagnostic performance of imaging-based AI compared with radiologists, has demonstrated variability across studies. For instance, research by Kan et al. highlighted that MRI-based AI models exhibited superior diagnostic accuracy for detecting LNM in cervical cancer compared to traditional radiologist assessments, suggesting the potential for enhanced precision through AI implementation (25). Conversely, findings from other studies, such as those by Shi et al., indicated that MRI-based AI models did not surpass the specificity achieved by experienced clinical radiologists, reflecting inconsistencies in diagnostic outcomes (32). These discrepancies underscore the need for further investigation into the comparative diagnostic efficacy of AI and human radiologists in this domain.
This study presents a meta-analysis of the diagnostic performance of imaging-based AI in detecting LNM in cervical cancer. Our findings demonstrate that AI models in internal validation cohorts achieved higher sensitivity (0.83 versus 0.54) and AUC (0.87 versus 0.65) compared to radiologists while maintaining comparable specificity (0.79 versus 0.78). The higher sensitivity and AUC of AI models may be attributed to their ability to detect subtle imaging features that may be overlooked by human observers, enhancing diagnostic accuracy (14). Radiologists demonstrate specificity comparable to that of AI models, possibly due to their ability to utilize clinical context and experiential judgment, which helps to reduce false-positive results (41). Overall, imaging-based AI exhibits enhanced diagnostic performance, particularly in accurately identifying patients with LNM.
Subgroup analysis of different imaging modalities in internal validation revealed that MRI, PET/CT, and CT demonstrated sensitivities of 0.82, 0.87, and 0.78, respectively, with specificities of 0.76, 0.91, and 0.72. The AUC values were 0.85 for MRI and 0.93 for PET/CT, while the AUC for CT could not be evaluated due to insufficient data. Our findings indicate that PET/CT-based AI showed superior diagnostic performance compared to MRI and CT. This enhanced performance is likely due to the integration of metabolic information with anatomical imaging in PET/CT and its ability to extract high-throughput imaging features reflecting metabolic characteristics (27, 42). In contrast, MRI-based and CT-based AI primarily rely on anatomical features alone.
This meta-analysis represents the first effort to evaluate the diagnostic performance of imaging-based AI models and directly compare their performance with that of radiologists in predicting LNM in cervical cancer patients. A meta-analysis by He et al. on traditional imaging techniques (MRI vs. PET/CT) reported a sensitivity of 0.65 (0.60–0.69) and specificity of 0.93 (0.91–0.94) for PET/CT, along with a sensitivity of 0.58 (0.54–0.63) and specificity of 0.91 (0.90–0.92) for MRI (43). Compared with the internal validation results of our study, their findings show lower sensitivity. These results are consistent with our comparison of imaging-based AI models and radiologists, further demonstrating the robustness of our findings.
Our study introduces an innovative approach by incorporating both internal and external validation datasets to evaluate the generalizability and reliability of AI models. A previous meta-analysis by Li et al., which evaluated the diagnostic performance of MRI-based AI in detecting lymph node metastasis in cervical cancer, reported a sensitivity of 0.80, specificity of 0.76, and AUC of 0.83 (44), results that are closely aligned with our findings for MRI-based AI models. However, we extended the scope beyond MRI to include multiple imaging modalities (CT and PET/CT). This broader approach offers novel and clinically relevant insights into AI applications in diverse imaging methods, providing actionable strategies for optimizing diagnostic workflows.
Imaging-based AI models present significant advantages in predicting LNM in cervical cancer patients, particularly due to their higher sensitivity compared to traditional methods, which can enhance detection performance. Our results demonstrates that AI achieves superior diagnostic performance (AUC: 0.87 versus 0.65 for radiologists), suggesting its potential to reduce healthcare providers’ workload and enhance patient outcomes through early detection and timely intervention. Notably, PET/CT-based AI showed superior diagnostic performance, warranting future studies to compare AI models across different imaging modalities.
The clinical significance of imaging-based AI lies in its ability to enable rapid detection and its high acceptance among patients. Although previous studies have explored other invasive methods for diagnosing and treating early cervical cancer patients, concerns regarding surgical trauma and complications remain substantial (45). Integrating imaging-based AI with these methods represents a potential direction for future clinical practice. Additionally, the study by Mereu et al. on locally advanced cervical cancer demonstrates that the treatment approach combining neoadjuvant chemotherapy with radical surgery shows limited effectiveness for patients with LNM, as it does not result in significant improvements in disease-free survival or overall survival (46). Consequently, the early detection of LNM in cervical cancer is crucial in clinical practice to prevent unnecessary surgery and chemotherapy and to develop appropriate strategies, such as precise resection or radiotherapy (12). However, the high heterogeneity of our results highlights the need for further research and external validation to confirm their robustness.
Several limitations of this meta-analysis must be considered when interpreting the results. First, the high heterogeneity among the included studies may have affected the overall sensitivity and specificity of AI models in both internal and external datasets. Meta-regression identified patient numbers, algorithm types, and imaging modalities as potential sources of heterogeneity. Notably, heterogeneity may also be attributed to variations in study design methodologies, patient demographic characteristics, tumor staging criteria, institutional imaging protocols, image acquisition parameters, and differences in radiologist experience levels and training backgrounds. Additionally, to reduce variability in the research, our study focused exclusively on imaging-based AI models and did not evaluate AI models incorporating other factors, such as clinical variables. The primary objective was to assess LNM detection in cervical cancer; other pathological factors, such as lymphovascular space invasion (LVSI), were not included in this analysis. Although some studies have explored the diagnostic performance of these pathological factors, integrating them into a comprehensive analysis remains a critical avenue for future research (47). Second, all included studies were retrospective, which introduces potential biases. Well-designed prospective studies with external datasets are necessary to validate our findings. Additionally, the majority of the studies were from China, which may also contribute to potential bias. Third, external validation was insufficient. Only six of the 23 studies included external testing. External validation is critical to address overfitting, a common issue in AI development, where models perform well on internal data but may underperform on external datasets. This discrepancy underscores the importance of following AI development guidelines that emphasize external validation before clinical application (48). Future research should prioritize rigorous external validation to ensure the durability and practical utility of AI algorithms in real-world clinical applications.
5 Conclusion
Imaging-based AI demonstrates higher diagnostic performance than radiologists. Prospective studies with rigorous standardization as well as further research with external validation datasets, are necessary to confirm the results and assess their practical clinical applicability.
Data availability statement
The original contributions presented in the study are included in the article/Supplementary Material. Further inquiries can be directed to the corresponding author.
Author contributions
CJ: Conceptualization, Data curation, Investigation, Methodology, Project administration, Supervision, Validation, Visualization, Writing – original draft, Writing – review & editing. XL: Validation, Visualization, Writing – review & editing. ZZ: Data curation, Validation, Visualization, Writing – review & editing. QX: Writing – original draft. LY: Project administration, Supervision, Writing – review & editing.
Funding
The author(s) declare that no financial support was received for the research, authorship, and/or publication of this article.
Conflict of interest
The authors declare that the research was conducted in the absence of any commercial or financial relationships that could be constructed as a potential conflict of interest.
Generative AI statement
The author(s) declare that no Generative AI was used in the creation of this manuscript.
Publisher’s note
All claims expressed in this article are solely those of the authors and do not necessarily represent those of their affiliated organizations, or those of the publisher, the editors and the reviewers. Any product that may be evaluated in this article, or claim that may be made by its manufacturer, is not guaranteed or endorsed by the publisher.
Supplementary material
The Supplementary Material for this article can be found online at: https://www.frontiersin.org/articles/10.3389/fonc.2025.1532698/full#supplementary-material
Abbreviations
AI, artificial intelligence; LNM, lymph node metastasis; CT, computed tomography; MRI, magnetic resonance imaging; PET/CT, positron emission tomography/computed tomography; QUADAS-2, Quality Assessment of Diagnostic Accuracy Studies-2; ROC, receiver operating characteristic; SROC, summary receiver operating characteristic.
References
1. Sung H, Ferlay J, Siegel RL, Laversanne M, Soerjomataram I, Jemal A, et al. Global cancer statistics 2020: GLOBOCAN estimates of incidence and mortality worldwide for 36 cancers in 185 countries. CA Cancer J Clin. (2021) 71:209–49. doi: 10.3322/caac.21660
2. Gien LT, Covens A. Lymph node assessment in cervical cancer: prognostic and therapeutic implications. J Surg Oncol. (2009) 99:242–7. doi: 10.1002/jso.21199
3. Koh WJ, Abu-Rustum NR, Bean S, Bradley K, Campos SM, Cho KR, et al. Cervical cancer, version 3.2019, NCCN clinical practice guidelines in oncology. J Natl Compr Canc Netw. (2019) 17:64–84. doi: 10.6004/jnccn.2019.0001
4. Choi HJ, Ju W, Myung SK, Kim Y. Diagnostic performance of computer tomography, magnetic resonance imaging, and positron emission tomography or positron emission tomography/computer tomography for detection of metastatic lymph nodes in patients with cervical cancer: meta-analysis. Cancer Sci. (2010) 101:1471–9. doi: 10.1111/j.1349-7006.2010.01532.x
5. Scheidler J, Hricak H, Yu KK, Subak L, Segal MR. Radiological evaluation of lymph node metastases in patients with cervical cancer. A meta-analysis. Jama. (1997) 278:1096–101. doi: 10.1001/jama.1997.03550130070040
6. Nguyen NC, Beriwal S, Moon CH, D’Ardenne N, Mountz JM, Furlan A, et al. Diagnostic value of FDG PET/MRI in females with pelvic Malignancy-A systematic review of the literature. Front Oncol. (2020) 10:519440. doi: 10.3389/fonc.2020.519440
7. Zheng M, Xie D, Pan C, Xu Y, Yu W. Diagnostic value of 18F-FDG PET/MRI in recurrent pelvis Malignancies of female patients: a systematic review and meta-analysis. Nucl Med Commun. (2018) 39:479–85. doi: 10.1097/MNM.0000000000000839
8. Khiewvan B, Torigian DA, Emamzadehfard S, Paydary K, Salavati A, Houshmand S, et al. Update of the role of PET/CT and PET/MRI in the management of patients with cervical cancer. Hell J Nucl Med. (2016) 19:254–68. doi: 10.1967/s002449910409
9. Wu Q, Wang S, Zhang S, Wang M, Ding Y, Fang J, et al. Development of a deep learning model to identify lymph node metastasis on magnetic resonance imaging in patients with cervical cancer. JAMA Netw Open. (2020) 3(7):e2011625. doi: 10.1001/jamanetworkopen.2020.11625
10. Lambin P, Rios-Velazquez E, Leijenaar R, Carvalho S, van Stiphout RG, Granton P, et al. Radiomics: extracting more information from medical images using advanced feature analysis. Eur J Cancer. (2012) 48:441–6. doi: 10.1016/j.ejca.2011.11.036
11. Wang T, Li YY, Ma NN, Wang PA, Zhang B. A MRI radiomics-based model for prediction of pelvic lymph node metastasis in cervical cancer. World J Surg Oncol. (2024) 22(1):55. doi: 10.1186/s12957-024-03333-5
12. Liu Y, Fan H, Dong D, Liu P, He B, Meng L, et al. Computed tomography-based radiomic model at node level for the prediction of normal-sized lymph node metastasis in cervical cancer. Trans Oncol. (2021) 14(8):101113. doi: 10.1016/j.tranon.2021.101113
13. Yang S, Zhang W, Liu C, Li C, Hua K. Predictive value and potential association of PET/CT radiomics on lymph node metastasis of cervical cancer. Ann Med Surge. (2024) 86:805–10. doi: 10.1097/MS9.0000000000001412
14. Bi WL, Hosny A, Schabath MB, Giger ML, Birkbak NJ, Mehrtash A, et al. Artificial intelligence in cancer imaging: Clinical challenges and applications. CA Cancer J Clin. (2019) 69:127–57. doi: 10.3322/caac.21552
15. Shen J, Zhang CJP, Jiang B, Chen J, Song J, Liu Z, et al. Artificial intelligence versus clinicians in disease diagnosis: systematic review. JMIR Med Inform. (2019) 7:e10010. doi: 10.2196/10010
16. McInnes MDF, Moher D, Thombs BD, McGrath TA, Bossuyt PM, Clifford T, et al. Preferred reporting items for a systematic review and meta-analysis of diagnostic test accuracy studies: the PRISMA-DTA statement. Jama. (2018) 319:388–96. doi: 10.1001/jama.2017.19163
17. Whiting PF, Rutjes AW, Westwood ME, Mallett S, Deeks JJ, Reitsma JB, et al. QUADAS-2: a revised tool for the quality assessment of diagnostic accuracy studies. Ann Intern Med. (2011) 155:529–36. doi: 10.7326/0003-4819-155-8-201110180-00009
18. Moons KGM, Wolff RF, Riley RD, Whiting PF, Westwood M, Collins GS, et al. PROBAST: A tool to assess risk of bias and applicability of prediction model studies: explanation and elaboration. Ann Intern Med. (2019) 170:W1–w33. doi: 10.7326/M18-1377
19. Moses LE, Shapiro D, Littenberg B. Combining independent studies of a diagnostic test into a summary ROC curve: data-analytic approaches and some additional considerations. Stat Med. (1993) 12:1293–316. doi: 10.1002/sim.4780121403
20. Hellmich M, Lehmacher W. A ruler for interpreting diagnostic test results. Methods Inf Med. (2005) 44:124–6. doi: 10.1055/s-0038-1633930
21. Deeks JJ, Macaskill P, Irwig L. The performance of tests of publication bias and other sample size effects in systematic reviews of diagnostic test accuracy was assessed. J Clin Epidemiol. (2005) 58:882–93. doi: 10.1016/j.jclinepi.2005.01.016
22. Ai C, Zhang L, Ding W, Zhong S, Li Z, Li M, et al. A nomogram-based optimized Radscore for preoperative prediction of lymph node metastasis in patients with cervical cancer after neoadjuvant chemotherapy. Front Oncol. (2023) 13. doi: 10.3389/fonc.2023.1117339
23. Deng X, Liu M, Sun J, Li M, Liu D, Li L, et al. Feasibility of MRI-based radiomics features for predicting lymph node metastases and VEGF expression in cervical cancer. Eur J Radiol. (2021) 134:109429. doi: 10.1016/j.ejrad.2020.109429
24. Hou L, Zhou W, Ren J, Du X, Xin L, Zhao X, et al. Radiomics analysis of multiparametric MRI for the preoperative prediction of lymph node metastasis in cervical cancer. Front Oncol. (2020) 10. doi: 10.3389/fonc.2020.01393
25. Kan Y, Dong D, Zhang Y, Jiang W, Zhao N, Han L, et al. Radiomic signature as a predictive factor for lymph node metastasis in early-stage cervical cancer. J Magnetic Resonance Imaging. (2019) 49:304–10. doi: 10.1002/jmri.26209
26. Li P, Feng B, Liu Y, Chen Y, Zhou H, Chen Y, et al. Deep learning nomogram for predicting lymph node metastasis using computed tomography image in cervical cancer. Acta Radiol. (2023) 64:360–9. doi: 10.1177/02841851211058934
27. Li XR, Jin JJ, Yu Y, Wang XH, Guo Y, Sun HZ. PET-CT radiomics by integrating primary tumor and peritumoral areas predicts E-cadherin expression and correlates with pelvic lymph node metastasis in early-stage cervical cancer. Eur Radiol. (2021) 31:5967–79. doi: 10.1007/s00330-021-07690-7
28. Liu J, Dong L, Zhang X, Wu Q, Yang Z, Zhang Y, et al. Radiomics analysis for prediction of lymph node metastasis after neoadjuvant chemotherapy based on pretreatment MRI in patients with locally advanced cervical cancer. Front Oncol. (2024) 14. doi: 10.3389/fonc.2024.1376640
29. Lucia F, Bourbonne V, Pleyers C, Dupré PF, Miranda O, Visvikis D, et al. Multicentric development and evaluation of 18F-FDG PET/CT and MRI radiomics models to predict para-aortic lymph node involvement in locally advanced cervical cancer. Eur J Nucl Med Mol Imaging. (2023) 50:2514–28. doi: 10.1007/s00259-023-06180-w
30. Qian W, Li Z, Chen W, Yin H, Zhang J, Xu J, et al. RESOLVE-DWI-based deep learning nomogram for prediction of normal-sized lymph node metastasis in cervical cancer: a preliminary study. BMC Med Imaging. (2022) 22:221. doi: 10.1186/s12880-022-00948-6
31. Qin F, Sun X, Tian M, Jin S, Yu J, Song J, et al. Prediction of lymph node metastasis in operable cervical cancer using clinical parameters and deep learning with MRI data: a multicentre study. Insights Into Imaging. (2024) 15(1):56. doi: 10.1186/s13244-024-01618-7
32. Shi J, Dong Y, Jiang W, Qin F, Wang X, Cui L, et al. MRI-based peritumoral radiomics analysis for preoperative prediction of lymph node metastasis in early-stage cervical cancer: A multi-center study. Magnetic Resonance Imaging. (2022) 88:1–8. doi: 10.1016/j.mri.2021.12.008
33. Song J, Hu Q, Ma Z, Zhao M, Chen T, Shi H. Feasibility of T2WI-MRI-based radiomics nomogram for predicting normal-sized pelvic lymph node metastasis in cervical cancer patients. Eur Radiol. (2021) 31:6938–48. doi: 10.1007/s00330-021-07735-x
34. Wu Q, Wang S, Chen X, Wang Y, Dong L, Liu Z, et al. Radiomics analysis of magnetic resonance imaging improves diagnostic performance of lymph node metastasis in patients with cervical cancer. Radiother Oncol. (2019) 138:141–8. doi: 10.1016/j.radonc.2019.04.035
35. Xia X, Li D, Du W, Wang Y, Nie S, Tan Q, et al. Radiomics based on nomogram predict pelvic lymphnode metastasis in early-stage cervical cancer. Diagnostics. (2022) 12(10):2446. doi: 10.3390/diagnostics12102446
36. Xiao M, Ma F, Li Y, Li Y, Li M, Zhang G, et al. Multiparametric MRI-based radiomics nomogram for predicting lymph node metastasis in early-stage cervical cancer. J Magnetic Resonance Imaging. (2020) 52:885–96. doi: 10.1002/jmri.27101
37. Xiao ML, Wei Y, Zhang J, Jian JM, Song Y, Lin ZJ, et al. MRI texture analysis for preoperative prediction of lymph node metastasis in patients with nonsquamous cell cervical carcinoma. Acad Radiol. (2022) 29:1661–71. doi: 10.1016/j.acra.2022.01.005
38. Yu Y, Li X, Du T, Rahaman M, Grzegorzek MJ, Li C, et al. Increasing the accuracy and reproducibility of positron emission tomography radiomics for predicting pelvic lymph node metastasis in patients with cervical cancer using 3D local binary pattern-based texture features. Intelligent Med. (2024) 4:153–60. doi: 10.1016/j.imed.2024.03.001
39. Zhang Y, Zhang KY, Jia HD, Fang X, Lin TT, Wei C, et al. Feasibility of predicting pelvic lymph node metastasis based on IVIM-DWI and texture parameters of the primary lesion and lymph nodes in patients with cervical cancer. Acad Radiol. (2022) 29:1048–57. doi: 10.1016/j.acra.2021.08.026
40. Zhang Z, Wan X, Lei X, Wu Y, Zhang J, Ai Y, et al. Intra- and peri-tumoral MRI radiomics features for preoperative lymph node metastasis prediction in early-stage cervical cancer. Insights Into Imaging. (2023) 14(1):65. doi: 10.1186/s13244-023-01405-w
41. Waite S, Grigorian A, Alexander RG, Macknik SL, Carrasco M, Heeger DJ, et al. Analysis of perceptual expertise in radiology - current knowledge and a new perspective. Front Hum Neurosci. (2019) 13:213. doi: 10.3389/fnhum.2019.00213
42. Yousefirizi F, Jha AK, Brosch-Lenz J, Saboury B, Rahmim A. Toward high-throughput artificial intelligence-based segmentation in oncological PET imaging. PET Clin. (2021) 16:577–96. doi: 10.1016/j.cpet.2021.06.001
43. He T, Sun J, Wu J, Wang H, Liang C, Wang H, et al. PET-CT versus MRI in the diagnosis of lymph node metastasis of cervical cancer: A meta-analysis. Microsc Res Tech. (2022) 85:1791–8. doi: 10.1002/jemt.24039
44. Li L, Zhang J, Zhe X, Tang M, Zhang X, Lei X, et al. A meta-analysis of MRI-based radiomic features for predicting lymph node metastasis in patients with cervical cancer. Eur J Radiol. (2022) 151:110243. doi: 10.1016/j.ejrad.2022.110243
45. Ronsini C, De Franciscis P, Carotenuto RM, Pasanisi F, Cobellis L, Colacurci N. The oncological implication of sentinel lymph node in early cervical cancer: A meta-analysis of oncological outcomes and type of recurrences. Med (Kaunas). (2022) 58(11):1539. doi: 10.3390/medicina58111539
46. Mereu L, Pecorino B, Ferrara M, Tomaselli V, Scibilia G, Scollo P. Neoadjuvant chemotherapy plus radical surgery in locally advanced cervical cancer: retrospective single-center study. Cancers (Basel). (2023) 15(21):5207. doi: 10.3390/cancers15215207
47. Ronsini C, Anchora LP, Restaino S, Fedele C, Arciuolo D, Teodorico E, et al. The role of semiquantitative evaluation of lympho-vascular space invasion in early stage cervical cancer patients. Gynecol Oncol. (2021) 162:299–307. doi: 10.1016/j.ygyno.2021.06.002
Keywords: cervical cancer, lymph node metastasis, artificial intelligence, radiomic, meta-analysis
Citation: Jiang C-Q, Li X-J, Zhou Z-Y, Xin Q and Yu L (2025) Imaging based artificial intelligence for predicting lymph node metastasis in cervical cancer patients: a systematic review and meta-analysis. Front. Oncol. 15:1532698. doi: 10.3389/fonc.2025.1532698
Received: 22 November 2024; Accepted: 10 February 2025;
Published: 28 February 2025.
Edited by:
Emanuele Perrone, Agostino Gemelli University Polyclinic (IRCCS), ItalyReviewed by:
Carlo Ronsini, Università degli Studi della Campania “Luigi Vanvitelli”, ItalyBasilio Pecorino, Kore University of Enna, Italy
Copyright © 2025 Jiang, Li, Zhou, Xin and Yu. This is an open-access article distributed under the terms of the Creative Commons Attribution License (CC BY). The use, distribution or reproduction in other forums is permitted, provided the original author(s) and the copyright owner(s) are credited and that the original publication in this journal is cited, in accordance with accepted academic practice. No use, distribution or reproduction is permitted which does not comply with these terms.
*Correspondence: Lin Yu, eXVsaW4wNDZAMTYzLmNvbQ==
†These authors have contributed equally to this work and share first authorship