- 1Department of Neurology, Wuhan Children's Hospital, Tongji Medical College, Huazhong University of Science and Technology, Wuhan, China
- 2Department of Pediatrics, Tongji Hospital, Tongji Medical College, Huazhong University of Science and Technology, Wuhan, China
- 3Department of Hematology, Wuhan Children's Hospital, Tongji Medical College, Huazhong University of Science and Technology, Wuhan, China
- 4Xiangyang Central Hospital, Affiliated Hospital of Hubei University of Arts and Science, Xiangyang, China
- 5Department of Cardiology, Union Hospital, Tongji Medical College, Huazhong University of Science and Technology, Wuhan, China
- 6Aegicare Technology Co., Ltd. Shenzhen, China
- 7Clinic Center of Human Gene Research, Union Hospital, Tongji Medical College, Huazhong University of Science and Technology, Wuhan, China
Background: Epileptic encephalopathies (EEs) are a pediatric entity with highly phenotypic and genetic heterogeneity. Both single nucleotide variants (SNVs)/Indels and copy number variations (CNVs) could be the causes. Whole exome sequencing (WES) is widely applied to detect SNVs/Indels, but the bioinformatics approach for detecting CNVs is still limited and weak. In the current study, the possibility of profiling both disease-causing SNVs/Indels and CNVs in a single test based on WES in EEs was evaluated.
Methods: The infants diagnosed with EEs were enrolled from a single pediatric epilepsy center between January 2018 and February 2020. Demographic and clinical data were collected. In WES data, the pathogenic SNVs were identified through an in-house pipeline, and pathogenic CNVs were identified by CNVkit. The diagnostic rate was evaluated, and the molecular findings were characterized.
Results: A total of 73 infants were included; 36 (49.32%) of them were males. The median age was 7 months. Thirty-two (43.84%) infants had been diagnosed with epilepsy syndrome. The most common type of syndrome was West syndrome (22/73, 30.1%), followed by Dravet syndrome (20/77, 27.4%). Fifty-four (73.97%) had intellectual development delay. The genetic cause of EEs, pathogenic or likely pathogenic variants, were successfully discovered in 46.6% (34/73) of the infants, and 29 (39.7%) infants carried SNVs/Indels, while 5 (6.8%) carried CNVs. The majority of the disease-causing variants were inherited in de novo pattern (25, 71.4%). In addition to showing that the variants in the ion channel encoding genes accounted for the main etiology, we discovered and confirmed two new disease-causing genes, CACNA1E and WDR26. Five discovered CNVs were deletions of 2q24.3, 1p36, 15q11-q13, 16p11.2, and 17p13.3, and all were confirmed by array comparative genomic hybridization.
Conclusion: The application of both SNVs/Indels and CNVs detection in a single test based on WES yielded a high diagnosis rate in EEs. WES may serve as a first-tier test with cost-effective benefit in EEs.
Introduction
Epileptic encephalopathies (EEs) are characterized by frequent severe seizures, severe electroencephalography (EEG) abnormalities, and intellectual/developmental disabilities (1–3). EEs commonly occur during infancy and early childhood, with poor clinical outcome. They are highly heterogeneous in clinical features, including West syndrome (WS), Dravet syndrome (DS), Ohtahara syndrome (OS), migrating partial epilepsy of infancy (MPEI), and other related epilepsy syndromes (4–6). A range of etiologic spectra in epilepsy has been recognized, including structural, genetic, infectious, metabolic, and immune etiologies (7). Among them, genetic factors play an important role in the pathogenesis of EEs. Many genes have been linked to EEs, and a significant proportion of them is ion channel genes which are particularly relevant to epilepsy (1, 8). Identifying the etiology of EEs is a primary clinical objective in the management of the disorder. Up to now, over 30 genes with precision medicine implications have been described in existing published literature (9, 10).
Sequencing-based studies have identified risk genes for rare and severe epilepsies and revealed a role of rare deleterious variation in common epilepsies (11). Whole exome sequencing (WES) is used routinely to detect sequence variants among the infants with EEs [single nucleotide variants (SNVs) and short insertions and deletions, SNPs/Indels] in clinical genetic laboratories (12, 13). However, the sequencing-based methods for identifying SNPs/Indels are not optimized for detecting the potential copy number variation (CNV), which is also involved in the pathogenesis of epilepsy, particularly early infantile epileptic encephalopathy (EIEE) (14, 15). This deficiency requires additional samples and application of genetic tests such as SNP arrays or array comparative genomic hybridization (aCGH) to discover the CNVs. Currently, many algorithms have been developed to obtain copy number information directly from the WES data (14, 16). This exome-based CNV analytic strategy has been proven to improve the diagnostic yields with cost-effective benefit (17). In this study, we evaluated the clinical application of profiling both SNPs/Indels and CNVs in a cohort of 73 infants with EEs who underwent WES as a diagnostic test.
Methods
Study Populations
A retrospective case study was performed in a single pediatric epilepsy center at Wuhan Children's Hospital, Tongji Medical College, Huazhong University of Science and Technology, Wuhan, China. The infants diagnosed with EEs were recruited between January 2018 and December 2019. All infants underwent a neurological examination, electromyography (EMG), and magnetic resonance imaging (MRI) for brain. And intellectual disabilities were assessed according to the criteria of the Diagnostic and Statistical Manual of Mental Disorders-5 (DSM-5) (18). All these clinical data were collected and independently reviewed by two neurologists (DS and YL).
WES
Genomic DNA samples were extracted from peripheral blood using QIAamp® Blood Mini Kit (Qiagen, Hilden, Germany). The quality of genomic DNA was evaluated by agarose gel electrophoresis analysis, and the quantity was measured by NanoDrop2000 and Qubit3.0. DNA was sheared with M220 Focused-ultrasonicator (Covaris, Woburn, MA, USA). DNA target regions were captured by hybridizing the genomic DNA sample library with the xGen® Exome Research Panel v1.0 (IDT, USA). The captured and amplified DNA samples were sequenced using Illumina NovaSeq6000 (Illumina, San Diego, CA, USA) with 150 base-paired end reads.
Detection of SNVs/Indels From WES Data
To identify disease-associated SNVs/Indels (short insertions and deletions smaller than 50 bp), sequencing data were analyzed according to an in-house pipeline. Both public software and commercial packages were implemented during bioinformatics analysis. Specifically, raw FASTQ was processed with FASTP (https://github.com/OpenGene/fastp) to cut adapters and filter out bad reads of low quality. The clean reads were then aligned against human reference (GRCh37) with BWA (19). SNPs/Indels were discovered by HaplotypeCaller tool of GATK after the necessary post-processes on primary alignment, including removal of duplicated reads, realignment, and base recalibrating (20). VEP(Ensembl Variant Effect Predictor) was employed to identify the effect of all discovered variants followed by variant annotation with AnnoVar (21). Notably, each variant was compared against several public databases, gnomAD, 1000 genomes project, NHLBI Exome Sequencing Project 6500 (ESP6500), and Exome Aggregation Consortium (ExAC) to achieve allele frequency in the general population. To identify the known and reported pathogenic variants, each variant was also compared against ClinVar (https://www.ncbi.nlm.nih.gov/clinvar) and Human Gene Mutation Database (HGMD) (www.hgmd.cf.ac.uk). In terms of possible influence on the protein function, variants were evaluated by several popular prediction tools, including InterVar, SIFT, PolyPhen, ClinPred, and GERP++ (22–26). Based on the variant annotations, series of filtering strategies were applied to identify candidate SNVs/Indels associated with EEs, which had been previously described (27).
Detection of CNVs From WES Data
CNVs were detected by CNVkit (28). To create a stable and reliable CNV reference, in-house samples over the same sequencing protocol were selected for reference training in an iterative manner. Specifically, a set of 80 samples was used to create an initial CNV reference. CNV calling process was then run over this reference for each sample in the training set. Samples with any CNV event larger than 1 Mbp were excluded from the next iteration. In the next iteration, new samples were added in to make up the training set to be 80 samples. The iteration process was ended once no <50 samples were qualified. During the reference creation process, circular binary segmentation algorithm was chosen for CNV event segmentation, and the threshold parameter for copy number calling was set to be “−1.6, −0.8, 0.5, 1.” For better visualization investigation of CNV events, a tool was designed to plot copy number aligned with B-allele frequencies along the chromosome coordinates. The copy number was shown as log2 ratio obtained from bin level and segmented level CNV calling results. The B-allele frequencies were computed by samtools mpileup tool. AnnotSV and its annotation databases were locally installed to annotate detected CNV events for each tested sample for the following clinical interpretation (29).
Confirmation and Interpretation of the Candidate Variants
To confirm the candidate SNVs/Indels, PCR amplification of the genomic DNA fragments of infants and their parents was performed, then the samples were sequenced by Sanger sequencing. The candidate CNVs identified were further verified by aCGH such as chromosomal microarray analysis (CMA) or methylation-specific multiplex ligation-dependent probe amplification (MS-MLPA). CMA was performed on Affymetrix GeneChip System 3000Dx v.2 (Thermo Fisher Scientific, MA, USA) by using CytoScan™ HD Array Kit (Thermo Fisher Scientific, MA, USA). Array data were analyzed with Affymetrix Chromosome Analysis Suite Software (Thermo Fisher Scientific, MA, USA). MS-MLPA was carried out using MS-MLPA Kit ME028 (MRC Holland, Amsterdam, Netherlands), and data analyses were performed using Coffalyser Software (MRC Holland, Amsterdam, Netherlands). The American College of Medical Genetics and Genomics/Association for Molecular Pathology (ACMG/AMP) criteria were applied in the interpretation of the pathogenicity of all identified variants (30).
Statistical Analysis
Quantitative variables were expressed as median and interquartile range (IQR). Categorical variables were presented as numbers and percentages. Comparison of categorical variables was analyzed by Fisher's exact-test or chi-squared-test where appropriate. All statistical analyses were performed using SPSS software (version 23.0; SPSS Inc., Chicago, IL, USA).
Result
Demographic and Clinical Features of the Infants With EEs
Seventy-three infants with EEs admitted between January 2018 and February 2020 were enrolled in the single pediatric epilepsy center for the study. The median age was 7 months (range 0.03–12 months); 36 (49.32%) of them were males. Clinical features of the 73 infants are listed in Table 1. Thirty-two (43.84%) infants had been diagnosed with epilepsy syndrome. The most common type of syndrome was WS (22/73, 30.1%), followed by DS (20/77, 27.4%), OS (1/73, 1.4%), and MPEI (1/73, 1.4%). The remaining 29 patients (39.7%) were diagnosed as unclassified EIEE due to non-specific clinical manifestations. Fifty-four (73.97%) had intellectual development delay. Sixty-three (86.30%) infants had an abnormal record of EEG, and 25 (34.2%) had brain MRI abnormality. Focal seizure (31/73, 42.5%) was the most common onset feature, followed by tonic-clonic seizures (23/73, 31.5%) and tonic seizures (15/73, 20.5%).
Identification of Pathogenic SNVs/Indels
For each sample, an average of 43.9 M (±5.9 M) pairs of 150 bp raw reads of WES were obtained. The exonic target region capture efficiency was 84.5% (±2.5%) on average. The mean sequencing depth on exonic target regions with flanking 10 bp on either side was 151-folds (±20-folds), providing a fairly high coverage of targeted exonic regions. Such high average coverage also ensured the detection of CNVs according to the demand of CVNkit. On average, 80,753 variants were called in each sample, consisting of 72,522 SNVs and 8,231 Indels. After excluding low quality variants with depths lower than 20-folds, about 68,741 SNVs and 7,382 Indels were left per sample.
When interpreted by ACMG recommended standards and analyzed for clinical concordance, 30 SNVs/Indels in 29 infants (39.7%) were graded pathogenic or likely pathogenic variants associated with EEs (Table 2). Among these variants, 15 (50.0%) were missenses, and 15 (50.0%) were protein-truncating variants including 4 (13.3%) non-senses, 10 (33.3%) frameshift Indels, and 1 (3.3%) splicing site variant. The majority of the variants were inherited in de novo pattern (20, 66.7%), defined as variants present in the infants but not in the parents. Two variants (6.7%) were inherited in X-linked pattern, and one variant (3.3%) in the PCDH19 gene was inherited in male-sparing pattern. Moreover, 16 (53.3%) variants were previously unreported in variant databases.
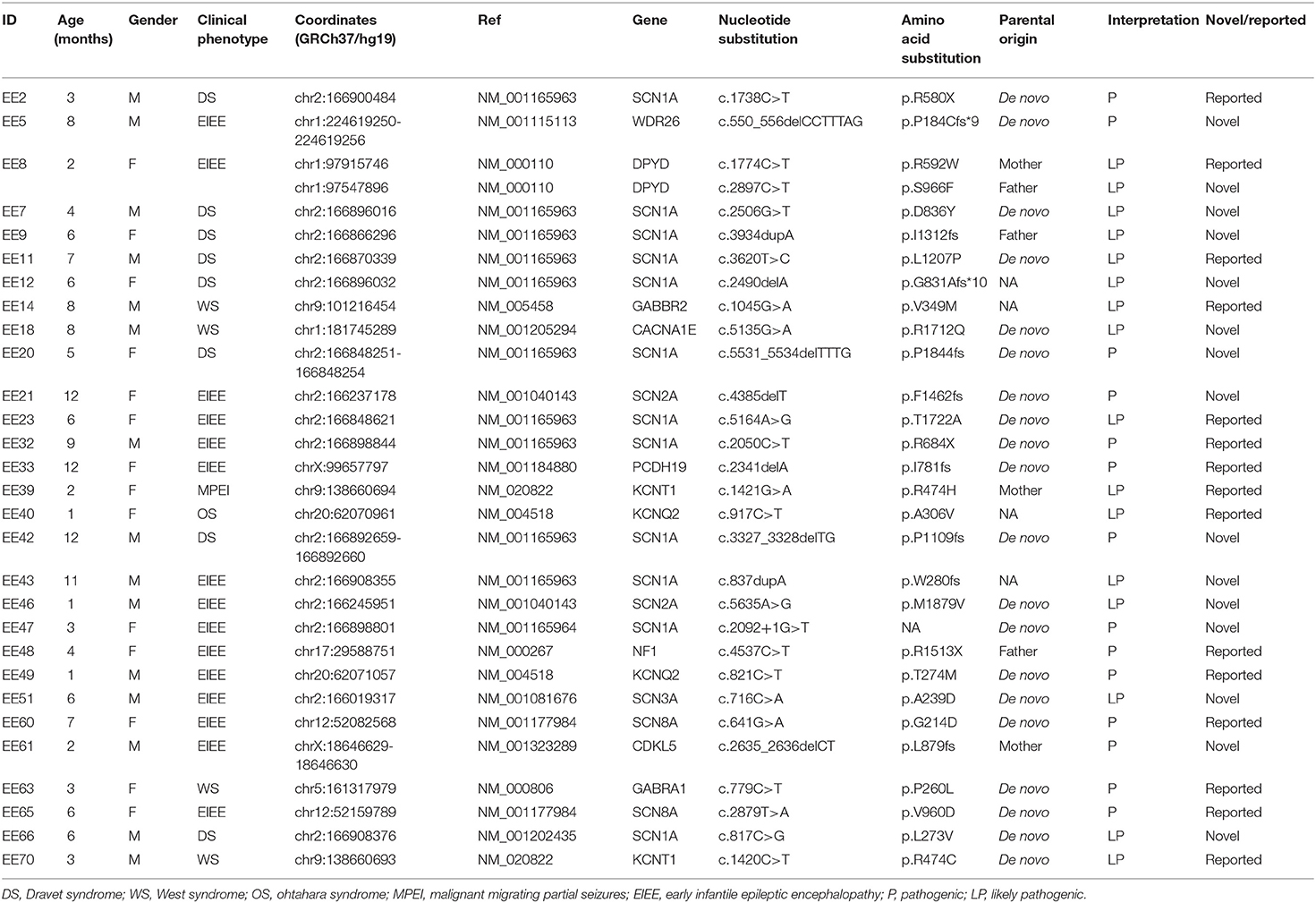
Table 2. Profiles of identified pathogenic or likely pathogenic sequence variants in the patients with EEs.
These pathogenic or likely pathogenic SNVs/Indels were located in 14 different genes. The genes, encoding sodium and potassium channels of neuron and maintaining neuron excitability, were the prevalent disease genes and account for 22 (30.1%) infants with EEs. Variants of the SCN1A gene, which encodes sodium voltage-gated channel alpha subunit 1, accounted for the largest proportion (12/73, 16.4%) of positively detected infants. And variants of the other ion channels genes, SCN2A, SCN8A, KCNQ2, and KCNT1, accounted for 2.7% (2/73), respectively. One pathogenic variant in de novo status was found in the CACNA1E gene, which encodes calcium voltage-gated channel subunit alpha1 E. The disease-causing variants in CACNA1E has recently been identified as a cause of developmental and epileptic encephalopathies (31). One variant each was found in both GABRA1 and GABRA2 genes, encoding Gamma-aminobutyric acid (GABA) type A receptor subunits. GABA type A receptors are pentameric chloride channels, which are the principal receptors that mediate the inhibitory synaptic transmission in the mammalian brain. Mutations in the genes encoding the GABA receptor subunits have been associated with a spectrum of neurological disorders including EEs. From the current study, four variants were located in the epilepsy-related genes, the DPYD gene, NF1 gene, and WDR26 gene. Two compound heterozygous variants were found in the DPYD gene, which encodes the rate-limiting enzyme for fluoropyrimidine catabolism and eliminates over 80% of administered 5-FU (32). The pathogenic homozygous or compound heterozygous variants within the DPYD gene are associated with dihydropyrimidine dehydrogenase (DPD) deficiency, and DPD-deficient infants may develop intellectual disability, motor retardation, and seizures. One non-sense variant was detected within the NF1 gene in the infant with WS. The NF1 gene is a tumor suppressor gene; therefore, loss of function due to a mutation leads to increase in cell proliferation and results in the development of neurofibromatosis type 1. Apart from tumors, patients with neurofibromatosis type 1 have an increased risk for WS (33). One novel de novo fame-shift variant was identified in the WDR26 gene, and WDR26 haploinsufficiency has been linked to a syndrome characterized by intellectual disability, seizures, abnormal gait, and distinctive facial features.
There were 21 candidate variants in 15 infants (20.5%) that were evaluated to be variants of uncertain significance (VUS) by ACMG/AMP criteria (Supplementary Table 1). These variants were kept through filtering steps because of pathogenicity prediction inconsistency in multiple perdition tools and limited published literature information.
Identification of Pathogenic CNVs
Through CNV analysis, six CNVs were linked to the clinical phenotype among six cases (8.2%) (Table 2). All of these variants were in de novo status. Five discovered CNVs were deletions of 2q24.3, 1p36, 15q11–q13, 16p11.2, and 17p13.3. Patient 73, who was a 10-month-old female, was admitted for infantile spasms, and brain MRI disclosed the lissencephaly. Both the CNV analysis and CMA found a de novo heterozygous deletion of 17p13.3 which was indicated by a value log2 ratio = −1 and missing value of 0.5 in BAF (Figure 1). In the deletion region, the YWHAE and CRK genes on the telomeric end of chromosome 17p have been considered as epilepsy-causing genes in previous clinical reports, and the deletion was interpreted as pathogenic (34, 35). The clinical findings and genetic test supported the diagnosis of Miller–Dieker syndrome. All these five deletions were classified as pathogenic, which included genes that are well-known to cause epilepsy (Table 2).
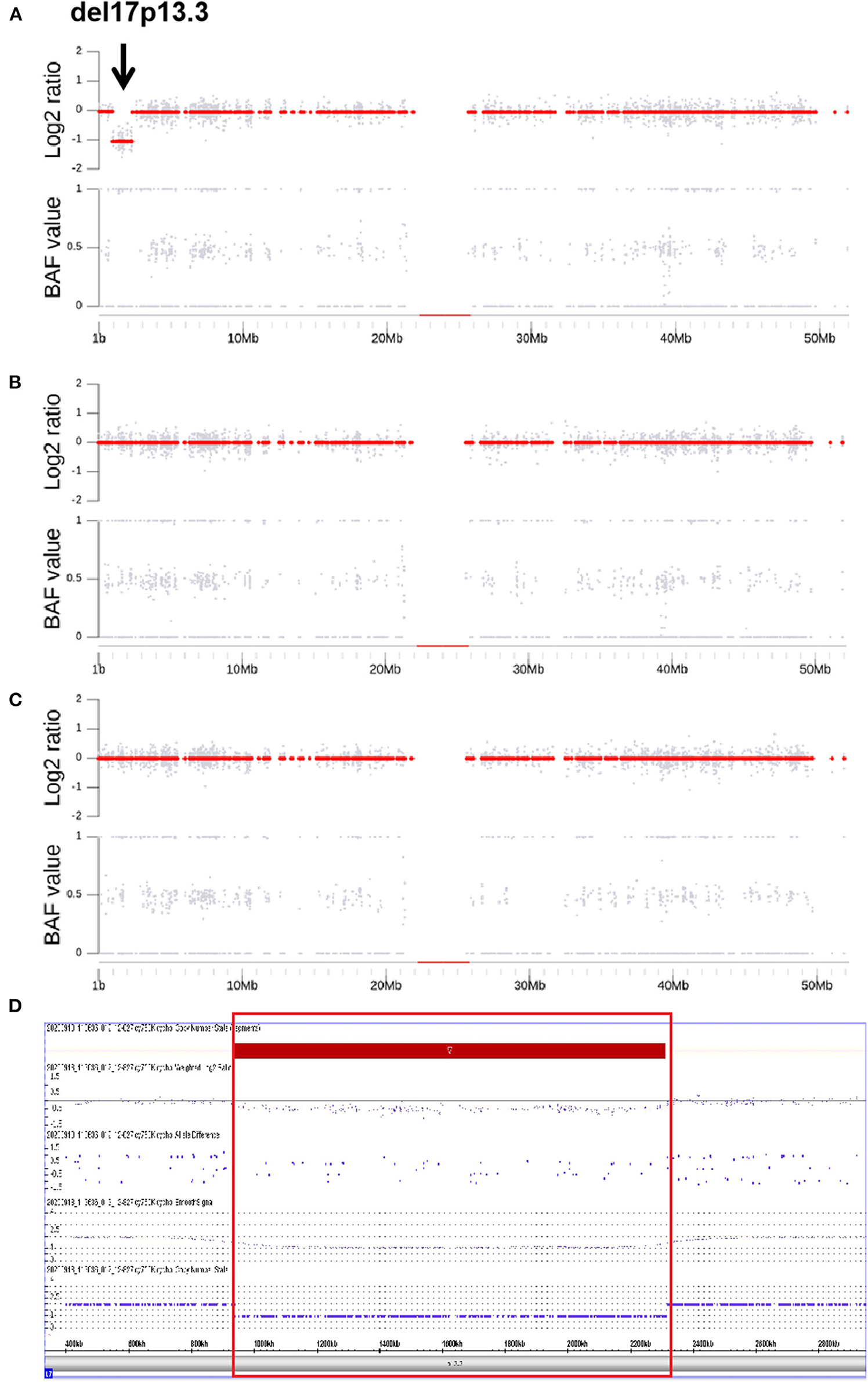
Figure 1. B allele Frequency, Log2 Ratio plots and CMA of a de novo heterozygous deletion of 17p13.3 in the patient with Miller-Dieker syndrome. In (A–C), the upper and the lower subplots are the copy number estimation and the B allele Frequency (BAF, allelic copy ratio) in the relevant regions. The copy number estimation is provided as log2 ratio (the upper subplots) which is calculated by log2 (Ne/Nd), where Ne is the estimated copy number and Nd is the normal copy number of the diploid (i.e., 2 for autosome). The gray dots show the Log2 Ratios of the bins and the red dots are the ones of the segments. (A–C) are the copy number Log2 Ratio and BAF plots for the proband, father and mother, respectively. The proband (A), the values of Log2 Ratio in detected region are around −1 and being recognized as a segment (red dots of −1). Meanwhile, the BAF are all closing to 0 or 1, indicating the homozygosity of this segment. A deletion of 17p13.3 is indicated by BAF band along with the lack of change in Log2 Ratio (black arrow, A). Trio analysis confirms that the deletion has occurred de novo in the proband. In (D), the deletion was verified by MS-MLPA and the loss in 17p13.3 is indicated by red rectangle.
One large duplication of 4q11–q13.1 was detected in a 12-month-old female with generalized tonic-clonic seizures and growth retardation. A previous study had detected duplication at 4q11–q13.1 in patients with idiopathic short stature, and TMEM165 and POLR2B in this region were suspected as candidate genes for growth retardation (36, 37). The duplication also included the SRD5A3 gene, the cause of Kahriz syndrome, and the null mutation of SRD5A3 having been reported to be associated with the epileptic phenotype, WS (38). In the duplicated regions, we also considered the REST gene as another candidate disease-causing gene because of its potential to control fundamental transcription patterns that drive circuit excitability, seizures, and epilepsy (39). However, due to the large size of duplication, it was difficult to determine the disease-causing gene(s), and the duplication was interpreted as VUS.
Altogether, the genetic causes (pathogenic or likely pathogenic variants) of EEs were successfully discovered for 34 (46.6%) infants. Twenty-nine (39.7%) infants carried the SNVs/Indels, and five (6.8%) carried CNVs related to the EEs. And the majority of the disease-causing variants were inherited in de novo pattern (25, 71.4%).
Genotype to Phenotype Correlation
In 20 clinical cases of DS, half of them (10/20, 50%) were found to arise from pathogenic or likely pathogenic SCN1A variants, and none of the disease-causing variants were identified in the other genes. In contrast, in 22 infants with WS, 8 cases (7/22, 31.8%) were caused by pathogenic or likely pathogenic variants from a variety of genes, including GABRA1, GABRA2, KCNT1, SCN3A, CACNA1E, PHGDH, and PRRT2 (in del 16p11.2). One infant was diagnosed with OS, and a likely pathogenic variant was found in KCNQ2. Additionally, another one infant was diagnosed with MPEI, and a likely pathogenic variant was found in KCNT1. As shown in Table 3, three pathogenic CNVs del1p36, del15q11–q13, and del17p13.3 corresponded to 1p36 deletion syndrome, Angelman syndrome, and Miller–Dieker syndrome, respectively.
Discussion
In the study, 73 infants with EEs for both SNPs/Indels and CNVs were evaluated by analyzing the WES data generated from a commercial human exome panel, and 34 mutations were found. SNVs/Indels accounted for 39.7% cases, and the detection of CNVs by using CNVkit increased a diagnostic rate of 6.8%. This is the first report of detecting both SNPs/Indels and CNVs in a single test for genetic diagnosis of EEs.
Through the current study, pathogenic and likely pathogenic SNPs/Indels were identified in 14 different disease-causing genes, and pathogenic CNVs were associated with at least 9 potential disease-causing genes. Altogether, 23 genes were involved in the pathogenesis of EEs in the current cohort. Similar to previous studies, ion channel-related genes, especially sodium ion channels and potassium ion channels, accounted for the large proportion of pathogenic and likely pathogenic variants. Indeed, in half of these genes, variants have been recorded to produce truncating proteins (40). One novel pathogenic variant (c.5135G>A/p.R1712Q) in de novo status was identified in a calcium channel-related gene, CACNA1E, which encodes a functionally critical subunit of a high voltage-activated, rapidly inactivating R-type calcium channel and initiates rapid synaptic transmission in the brain. The infant harboring the variant had intellectual disability and hypsarrhythmia on EEG, consistent with a diagnosis of WS. Helbig et al. had identified de novo CACNA1E variants in 30 individuals with EEs, characterized by refractory infantile-onset seizures, severe hypotonia, and profound developmental impairment (31). Most of the pathogenic variant clusters within the cytoplasmic ends of all the four S6 segments (Domains I, II, III, and IV) form the presumed CaV2.3 channel activation gate. The p.R1712Q variants also located in the S6 segment (Domain IV) are supposed to play a gain-of-function effect comprising facilitated voltage-dependent activation and slowed inactivation (31). One novel fame-shift deletion (c.550_556delCCTTTAG/p.P184Cfs*9) in the WDR26 gene was detected in an infant with absence seizures and febrile seizures, ventriculomegaly, intellectual/developmental disabilities, congenital heart disease, facial dysmorphism, and yellow hair. As WDR26 is located in chromosomal region 1q42, this showed that the patient shared some clinical features with 1q41q42 microdeletion syndrome, such as seizures, intellectual/developmental disabilities, and facial dysmorphism (41). Therefore, the truncation was supposed to lead to a haploinsufficiency of WDR26 to cause EEs like pathogenic mechanism of 1q41q42 microdeletion (41).
The majority of positive cases with EEs resulted to de novo pathogenic or likely pathogenic variants. Twenty SNPs/Indels and five CNVs in de novo status accounted for 71.4% positive findings and explained about one third of the infants with EEs overall. However, the current study was unable to exclude the possible parental mosaicism, which remains undetected by current methods. Parental mosaicism had been shown to present significantly higher recurrence risk in future pregnancies than the apparent de novo variants. It was identified in ~10% of families with children with an apparent de novo SCN1A variant in EEs (42). For further genetic counseling with apparent de novo variants in EEs, there is a need to consider higher recurrence risk especially for families who may wish to have another baby, who should therefore be subjected to more tests to confirm the status of de novo.
In our cohort, WS showed great heterogeneity in genetic etiology, with seven genes accounting for seven cases. And both SNP/Indels or CNVs were identified as causatives in WS. Currently, the routine treatment includes adrenocorticotropic hormone, vigabatrin, and corticosteroids. However, with the understanding of disease-causing genes and related pathogenesis, new therapies that target specific pathways of pathogenesis to correct the underlying molecular dysfunction will be developed in the near future. For example, there are a number of trials including rapamycin/everolimus, targeting the mTOR pathway, for the treatment of tuberous sclerosis complex and related WS (43); retigabine for the treatment of KCNQ2-related WS (44); and topiramate for the treatment of CACNA1E-related WS (31).
The detection of CNVs by using CNVkit increased the diagnostic rate by 6.8% in our cohort. The traditional approach for detecting genome-wide CNVs includes karyotyping, fluorescence in situ hybridization (FISH), SNP array, and aCGH. Among them, aCGH has been proven as an indispensable approach to screen CNVs associated with epilepsy, intellectual disability, developmental delay, or congenital anomalies in children (45). In the past decade, WES, which refers to sequencing of all protein-coding exons, has been widely used in genetic testing in Mendelian disorders as well as other complex diseases (46). It is powerful to detect SNVs/Indels in enriched protein-coding regions. Recently, many bioinformatics tools have been developed to do CNVs calling from WES data. These included XHMM (47), using hidden Markov model, and CoNIFER (48), using singular value decomposition, whereas others such as cn.MOPS use Bayesian inference (49). CNVkit is a toolkit that uses both the targeted reads and the non-specifically captured off-target reads to achieve highly accurate and reliable copy ratio estimates across the genome (28). All these CNV calling tools based on WES data could be considered as a complementary way with only computational effort to improve the diagnostic yields in EEs. In our study, aCGH served as a confirmatory tool rather than a screening tool and could bring cost-effective benefit for clinical diagnosis.
There are various limitations of the current study that need to be addressed. First, phenotypic details for the parents and familial disease history were lacking in some cases, which is a common issue with retrospective studies. Second, the current study approach was to identify known reported disease-causing genes and their rare variants and cloud not identify the variants in new disease-causing genes and common variants as genetic risks for EEs. Third, there were 22 variants (21 SNPs/Indels and 1 CNV) in 16 infants that were interpreted as VUS in EEs. Most of them were novel findings when they were compared with disease variants database or published literature. As more evidence emerges through function analysis, some VUS will be reclassified as the pathogenic variants. All these limitations may influence genetic diagnostic rate under the current study.
In summary, the application of both SNPs/Indels and CNVs detection in a single test based on WES yielded diagnosis in 46.6% of the infants with EEs, which demonstrates the utility of this pipeline as a diagnostic test for pediatric patients with a disease presenting a highly phenotypic and genetic heterogeneity. Importantly, a number of novel variants involved in EEs are being reported here. Although there are technical challenges with CNVs calling as well as challenges for pathogenic classification of identified variants, WES may serve as a first-tier test with cost-effective benefit in EEs.
Data Availability Statement
The raw sequence data reported in this paper have been deposited in the Genome Sequence Archive in National Genomics Data Center, China National Center for Bioinformation/Beijing Institute of Genomics, Chinese Academy of Sciences (https://bigd.big.ac.cn/). After publication of study findings, the data will be available for others to request. The research team will provide accession number of the database once the data are approved to be shared with others. The proposal with description of study objectives will be needed for evaluation of the reasonability to request for the data. The corresponding authors and the Ethical Committee of Wuhan Children's Hospital will make a decision based on these materials.
Ethics Statement
The studies involving human participants were reviewed and approved by The Ethical Committee of Wuhan Children's Hospital, Tongji Medical College, Huazhong University of Science and Technology, Wuhan, China. Written informed consent to participate in this study was provided by the participants' legal guardian/next of kin.
Author Contributions
FZ and DS conceived, supervised this study, and wrote the manuscript. DS, YaL, WC, and ZL evaluated and cared for the patients. JM, KN, and MC collected clinical data. CW and YZ performed the WES test. FZ and YoL performed the bioinformatics analysis of WES data. All authors contributed to the article and approved the submitted version.
Funding
This work was supported by grants from the National Natural Science Foundation of China (No. 81570348) and the National Key Research and Development Program of China (No. 2016YFC1306202).
Conflict of Interest
YoL and YZ are employees of company Aegicare Technology Co., Ltd. (Shenzhen, China).
The remaining authors declare that the research was conducted in the absence of any commercial or financial relationships that could be construed as a potential conflict of interest.
Acknowledgments
We are thankful to the patients and their families for their participation in the study.
Supplementary Material
The Supplementary Material for this article can be found online at: https://www.frontiersin.org/articles/10.3389/fped.2021.635703/full#supplementary-material
References
1. McTague A, Howell KB, Cross JH, Kurian MA, Scheffer IE. The genetic landscape of the epileptic encephalopathies of infancy and childhood. Lancet Neurol. (2016) 15:304–16. doi: 10.1016/S1474-4422(15)00250-1
2. Hamdan FF, Myers CT, Cossette P, Lemay P, Spiegelman D, Laporte AD, et al. High rate of recurrent de novo mutations in developmental and epileptic encephalopathies. Am J Hum Genet. (2017) 101:664–85. doi: 10.1016/j.ajhg.2017.09.008
3. Helbig I, Tayoun AA. Understanding genotypes and phenotypes in epileptic encephalopathies. Mol Syndromol. (2016) 7:172–81. doi: 10.1159/000448530
4. Auvin S, Cilio MR, Vezzani A. Current understanding and neurobiology of epileptic encephalopathies. Neurobiol Dis. (2016) 92:72–89. doi: 10.1016/j.nbd.2016.03.007
5. Trivisano M, Specchio N. “Early-onset epileptic encephalopathies,” Clinical Electroencephalography ed M. Oriano (New York, NY: Springer), (2019) 405–411. doi: 10.1007/978-3-030-04573-9
6. Shbarou R, Mikati MA. The expanding clinical spectrum of genetic pediatric epileptic encephalopathies. Semin Pediatr Neurol. (2016) 23:134–42. doi: 10.1016/j.spen.2016.06.002
7. Scheffer IE, Berkovic S, Capovilla G, Connolly MB, French J, Guilhoto L, et al. ILAE classification of the epilepsies: position paper of the ILAE commission for classification and terminology. Epilepsia. (2017) 58:512–21. doi: 10.1111/epi.13709
8. Kaplan DI, Isom LL, Petrou S. Role of sodium channels in epilepsy. Cold Spring Harb Perspect Med. (2016) 6:a022814. doi: 10.1101/cshperspect.a022814
9. Truty R, Patil N, Sankar R, Sullivan J, Millichap J, Carvill G, et al. Possible precision medicine implications from genetic testing using combined detection of sequence and intragenic copy number variants in a large cohort with childhood epilepsy. Epilepsia Open. (2019) 4:397–408. doi: 10.1002/epi4.12348
10. Lin Lee V, Kar Meng Choo B, Chung YS, Kundap UP, Kumari Y, Shaikh MF. Treatment, therapy and management of metabolic epilepsy: a systematic review. Int J Mol Sci. (2018) 19:871. doi: 10.3390/ijms19030871
11. Milh M, Riccardi F, Denis J. Genetics of neonatal onset epilepsies: an overview. Rev Neurol (Paris). (2020) 176:2–9. doi: 10.1016/j.neurol.2019.01.396
12. Ostrander BEP, Butterfield RJ, Pedersen BS, Farrell AJ, Layer RM, Ward A, et al. Whole-genome analysis for effective clinical diagnosis and gene discovery in early infantile epileptic encephalopathy. NPJ Genom Med. (2018) 3:22. doi: 10.1038/s41525-018-0061-8
13. Palmer EE, Schofield D, Shrestha R, Kandula T, Macintosh R, Lawson JA, et al. Integrating exome sequencing into a diagnostic pathway for epileptic encephalopathy: evidence of clinical utility and cost effectiveness. Mol Genet Genomic Med. (2018) 6:186–99. doi: 10.1002/mgg3.355
14. Tsang MH, Leung GK, Ho AC, Yeung KS, Mak CC, Pei SL, et al. Exome sequencing identifies molecular diagnosis in children with drug-resistant epilepsy. Epilepsia Open. (2019) 4:63–72. doi: 10.1002/epi4.12282
15. Epi25 Collaborative. Electronic address: cy5iZXJrb3ZpY0B1bmltZWxiLmVkdS5hdQ==. Ultra-rare genetic variation in the epilepsies: a whole-exome sequencing study of 17,606 individuals. Am J Hum Genet. (2019) 105:267–82. doi: 10.1016/j.ajhg.2019.05.020
16. Striano P, Coppola A, Paravidino R, Malacarne M, Gimelli S, Robbiano A, et al. Clinical significance of rare copy number variations in epilepsy: a case-control survey using microarray-based comparative genomic hybridization. Arch Neurol. (2012) 69:322–30. doi: 10.1001/archneurol.2011.1999
17. Epilepsy Phenome/Genome Project Epi KC. Copy number variant analysis from exome data in 349 patients with epileptic encephalopathy. Ann Neurol. (2015) 78:323–8. doi: 10.1002/ana.24457
18. Association AP. Diagnostic and Statistical Manual of Mental Disorders (DSM-5®). American Psychiatric Pub (2013).
19. Li H, Durbin R. Fast and accurate short read alignment with Burrows-Wheeler transform. Bioinformatics (Philadelphia: Oxford, England). (2009) 25:1754–60. doi: 10.1093/bioinformatics/btp324
20. McKenna A, Hanna M, Banks E, Sivachenko A, Cibulskis K, Kernytsky A, et al. The genome analysis toolkit: a MapReduce framework for analyzing next-generation DNA sequencing data. Genome Res. (2010) 20:1297–303. doi: 10.1101/gr.107524.110
21. McLaren W, Gil L, Hunt SE, Riat HS, Ritchie GR, Thormann A, et al. The ensembl variant effect predictor. Genome Biol. (2016) 17:122. doi: 10.1186/s13059-016-0974-4
22. Ng PC, Henikoff S. SIFT: predicting amino acid changes that affect protein function. Nucleic Acids Res. (2003) 31:3812–4. doi: 10.1093/nar/gkg509
23. Adzhubei IA, Schmidt S, Peshkin L, Ramensky VE, Gerasimova A, Bork P, et al. A method and server for predicting damaging missense mutations. Nat Methods. (2010) 7:248–9. doi: 10.1038/nmeth0410-248
24. Li Q, Wang K. InterVar: clinical interpretation of genetic variants by the 2015 ACMG-AMP guidelines. Am J Hum Genet. (2017) 100:267–80. doi: 10.1016/j.ajhg.2017.01.004
25. Alirezaie N, Kernohan KD, Hartley T, Majewski J, Hocking TD. ClinPred: prediction tool to identify disease-relevant nonsynonymous single-nucleotide variants. Am J Hum Genet. (2018) 103:474–83. doi: 10.1016/j.ajhg.2018.08.005
26. Davydov EV, Goode DL, Sirota M, Cooper GM, Sidow A, Batzoglou S. Identifying a high fraction of the human genome to be under selective constraint using GERP++. PLoS Comput Biol. (2010) 6:e1001025. doi: 10.1371/journal.pcbi.1001025
27. Zhou P, He N, Zhang JW, Lin ZJ, Wang J, Yan LM, et al. Novel mutations and phenotypes of epilepsy-associated genes in epileptic encephalopathies. Genes Brain Behav. (2018) 17:e12456. doi: 10.1111/gbb.12456
28. Talevich E, Shain AH, Botton T, Bastian BC. CNVkit: genome-wide copy number detection and visualization from targeted DNA sequencing. PLoS Comput Biol. (2016) 12:e1004873. doi: 10.1371/journal.pcbi.1004873
29. Geoffroy V, Herenger Y, Kress A, Stoetzel C, Piton A, Dollfus H, et al. AnnotSV: an integrated tool for structural variations annotation. Bioinformatics (Oxford, England). (2018) 34:3572–4. doi: 10.1093/bioinformatics/bty304
30. Richards S, Aziz N, Bale S, Bick D, Das S, Gastier-Foster J, et al. Standards and guidelines for the interpretation of sequence variants: a joint consensus recommendation of the American College of Medical Genetics and Genomics and the Association for Molecular Pathology. Genet Med. (2015) 17:405–24. doi: 10.1038/gim.2015.30
31. Helbig KL, Lauerer RJ, Bahr JC, Souza IA, Myers CT, Uysal B, et al. De novo pathogenic variants in CACNA1E cause developmental and epileptic encephalopathy with contractures, macrocephaly, and dyskinesias. Am J Hum Genet. (2018) 103:666–78. doi: 10.1016/j.ajhg.2018.09.006
32. Wigle TJ, Tsvetkova EV, Welch SA, Kim RB. DPYD and fluorouracil-based chemotherapy: mini review and case report. Pharmaceutics. (2019) 11:199. doi: 10.3390/pharmaceutics11050199
33. Ruggieri M, Iannetti P, Clementi M, Polizzi A, Incorpora G, Spalice A, et al. Neurofibromatosis type 1 and infantile spasms. Childs Nerv Syst. (2009) 25:211–6. doi: 10.1007/s00381-008-0706-5
34. Noor A, Bogatan S, Watkins N, Meschino WS, Stavropoulos DJ. Disruption of YWHAE gene at 17p13.3 causes learning disabilities and brain abnormalities. Clin Genet. (2018) 93:365–7. doi: 10.1111/cge.13056
35. Blazejewski SM, Bennison SA, Smith TH, Toyo-Oka K. Neurodevelopmental genetic diseases associated with microdeletions and microduplications of chromosome 17p13.3. Front Genet. (2018) 9:80. doi: 10.3389/fgene.2018.00080
36. Schulte Althoff S, Gruneberg M, Reunert J, Park JH, Rust S, Muhlhausen C, et al. TMEM165 deficiency: postnatal changes in glycosylation. JIMD Rep. (2016) 26:21–9. doi: 10.1007/8904_2015_455
37. Hu G, Fan Y, Wang L, Yao RE, Huang X, Shen Y, et al. Copy number variations in 119 Chinese children with idiopathic short stature identified by the custom genome-wide microarray. Mol Cytogenet. (2016) 9:16. doi: 10.1186/s13039-016-0225-0
38. Tuysuz B, Pehlivan D, Ozkok A, Jhangiani S, Yalcinkaya C, Zeybek CA, et al. Phenotypic expansion of congenital disorder of glycosylation due to SRD5A3 null mutation. JIMD Rep. (2016) 26:7–12. doi: 10.1007/8904_2015_478
39. Hwang JY, Zukin RS. REST, a master transcriptional regulator in neurodegenerative disease. Curr Opin Neurobiol. (2018) 48:193–200. doi: 10.1016/j.conb.2017.12.008
40. Oyrer J, Maljevic S, Scheffer IE, Berkovic SF, Petrou S, Reid CA. Ion channels in genetic epilepsy: from genes and mechanisms to disease-targeted therapies. Pharmacol Rev. (2018) 70:142–73. doi: 10.1124/pr.117.014456
41. Skraban CM, Wells CF, Markose P, Cho MT, Nesbitt AI, Au PYB, et al. WDR26 haploinsufficiency causes a recognizable syndrome of intellectual disability, seizures, abnormal gait, and distinctive facial features. Am J Hum Genet. (2017) 101:139–48. doi: 10.1016/j.ajhg.2017.06.002
42. Epi KC. De novo mutations in SLC1A2 and CACNA1A are important causes of epileptic encephalopathies. Am J Hum Genet. (2016) 99:287–98. doi: 10.1016/j.ajhg.2016.06.003
43. Franz DN, Belousova E, Sparagana S, Bebin EM, Frost MD, Kuperman R, et al. Long-term use of everolimus in patients with tuberous sclerosis complex: final results from the EXIST-1 study. PLoS ONE. (2016) 11:e0158476. doi: 10.1371/journal.pone.0158476
44. Kalappa BI, Soh H, Duignan KM, Furuya T, Edwards S, Tzingounis AV, et al. Potent KCNQ2/3-specific channel activator suppresses in vivo epileptic activity and prevents the development of tinnitus. J Neurosci. (2015) 35:8829–42. doi: 10.1523/JNEUROSCI.5176-14.2015
45. Miller DT, Adam MP, Aradhya S, Biesecker LG, Brothman AR, Carter NP, et al. Consensus statement: chromosomal microarray is a first-tier clinical diagnostic test for individuals with developmental disabilities or congenital anomalies. Am J Hum Genet. (2010) 86:749–64. doi: 10.1016/j.ajhg.2010.04.006
46. Han JY, Lee IG. Genetic tests by next-generation sequencing in children with developmental delay and/or intellectual disability. Clin Exp Pediatr. (2020) 63:195–202. doi: 10.3345/kjp.2019.00808
47. Fromer M, Purcell SM. Using XHMM software to detect copy number variation in whole-exome sequencing data. Curr Protoc Hum Genet. (2014) 81:7.23.1–21. doi: 10.1002/0471142905.hg0723s81
48. Krumm N, Sudmant PH, Ko A, O'Roak BJ, Malig M, Coe BP, et al. Copy number variation detection and genotyping from exome sequence data. Genome Res. (2012) 22:1525–32. doi: 10.1101/gr.138115.112
Keywords: epileptic encephalopathies, whole exome sequencing, genetics, copy number variation, variant
Citation: Sun D, Liu Y, Cai W, Ma J, Ni K, Chen M, Wang C, Liu Y, Zhu Y, Liu Z and Zhu F (2021) Detection of Disease-Causing SNVs/Indels and CNVs in Single Test Based on Whole Exome Sequencing: A Retrospective Case Study in Epileptic Encephalopathies. Front. Pediatr. 9:635703. doi: 10.3389/fped.2021.635703
Received: 30 November 2020; Accepted: 24 March 2021;
Published: 13 May 2021.
Edited by:
Tieliu Shi, East China Normal University, ChinaReviewed by:
Jeffrey Dennis Calhoun, Northwestern University, United StatesCristina Skrypnyk, Arabian Gulf University, Bahrain
Copyright © 2021 Sun, Liu, Cai, Ma, Ni, Chen, Wang, Liu, Zhu, Liu and Zhu. This is an open-access article distributed under the terms of the Creative Commons Attribution License (CC BY). The use, distribution or reproduction in other forums is permitted, provided the original author(s) and the copyright owner(s) are credited and that the original publication in this journal is cited, in accordance with accepted academic practice. No use, distribution or reproduction is permitted which does not comply with these terms.
*Correspondence: Zhisheng Liu, bGl1enNjQDEyNi5jb20=; Feng Zhu, emh1ZmVuZ0BodXN0LmVkdS5jbg==
†These authors have contributed equally to this work