- 1The Central Laboratory of Birth Defects Prevention and Control, Ningbo Women and Children's Hospital, Ningbo City, Zhejiang, China
- 2Ningbo Women and Children's Hospital, Ningbo, Zhejiang, China
- 3Department of Endocrinology, Ningbo Women and Children's Hospital, Ningbo, Zhejiang, China
- 4Department of Children’s Healthcare Clinic, Women and Children’s Hospital of Ningbo University, Ningbo, Zhejiang, China
- 5Paediatric Surgery Centre, Women and Children’s Hospital of Ningbo University, Ningbo, Zhejiang, China
Background: Neonatal screening for inherited metabolic diseases (IMDs) has been revolutionized by tandem mass spectrometry (MS/MS). This study aimed to enhance neonatal screening for IMDs using machine learning (ML) techniques.
Methods: The study involved the analysis of a comprehensive dataset comprising 309,102 neonatal screening records collected in the Ningbo region, China. An advanced ML system model, encompassing nine distinct algorithms, was employed for the purpose of predicting the presence of 31 different IMDs. The model was compared with traditional cutoff schemes to assess its diagnostic efficacy. Additionally, 180 suspected positive cases underwent further evaluation.
Results: The ML system exhibited a significantly reduced positive rate, from 1.17% to 0.33%, compared to cutoff schemes in the initial screening, minimizing unnecessary recalls and associated stress. In suspected positive cases, the ML system identified 142 true positives with high sensitivity (93.42%) and improved specificity (78.57%) compared to the cutoff scheme. While false negatives emerged, particularly in heterozygous carriers, our study revealed the potential of the ML system to detect asymptomatic cases.
Conclusion: This research provides valuable insights into the potential of ML in pediatric medicine for IMD diagnosis through neonatal screening, emphasizing the need for accurate carrier detection and further research in this domain.
1 Introduction
Inherited metabolic diseases (IMDs) comprise a group of genetic disorders, including amino acid, organic acid, and fatty acid disorders (1). The application of tandem mass spectrometry (MS/MS) in neonatal screening has revolutionized the early identification of IMDs by analyzing and interpreting amino acids and acylcarnitines (2). At present, MS/MS technology enables the screening of approximately 50 metabolites, facilitating the detection of over 20 IMDs (3). However, the comprehensive metabolite measurement involved in MS/MS screening comes with limitations, particularly in terms of false-positive (FPs) and false-negative results (FNs) (4–7). The consequences of such inaccuracies were significant, often subjecting families to unnecessary stress and healthcare costs or, conversely, delaying vital treatment.
With the advancement of science and technology, computational and machine learning (ML) methodologies provide a promising approach for analyzing high-dimensional data (8, 9). Recent applications of ML techniques have extended to neonatal screening for the diagnosis of IMDs, improving screening sensitivity and specificity (10, 11). ML techniques have the potential to expedite the diagnosis of IMDs. In a previous study conducted by our collaborative partners, 9 ML algorithms were employed to predict 16 IMDs (12). As the repository of screening and diagnostic data steadily accumulates, the ML system model has now acquired the capacity to prognosticate the presence of 31 IMDs (13). The present study aimed to conduct a comprehensive evaluation of the diagnostic efficacy of the ML system model using neonatal screening data from the Ningbo area (China) in clinical practice.
2 Materials and methods
2.1 Patients’ data
The study population consisted of 309,102 neonatal screening data collected from the Central Laboratory of Birth Defects Prevention and Control Affiliated with the Ningbo Women and Children's Hospital (Ningbo, China) between July 2014 and March 2020. In the large dataset of screening results, a total of 3,608 cases commenced recall procedures due to their initial positive screening results. Subsequently, 398 cases exhibited abnormal metabolic concentration or metabolite concentration ratios during secondary screening, indicating their potential as cases of IMDs. Among these, 180 cases underwent next-generation sequencing (NGS) to confirm IMDs, while the remaining 218 suspected cases did not proceed with NGS due to various reasons. These reasons include normal results from subsequent tandem mass spectrometry tests and urine organic acid tests, or parental refusal of NGS testing for their children. While these patients were included in the machine learning (ML) analysis, the lack of genetic testing reports for them precludes a definitive exclusion of disease presence. Therefore, our analysis focused solely on the diagnostic efficiency of cases with a clear genetic diagnosis, to ensure the integrity and reliability of our findings.The study flowchart is depicted in Figure 1.
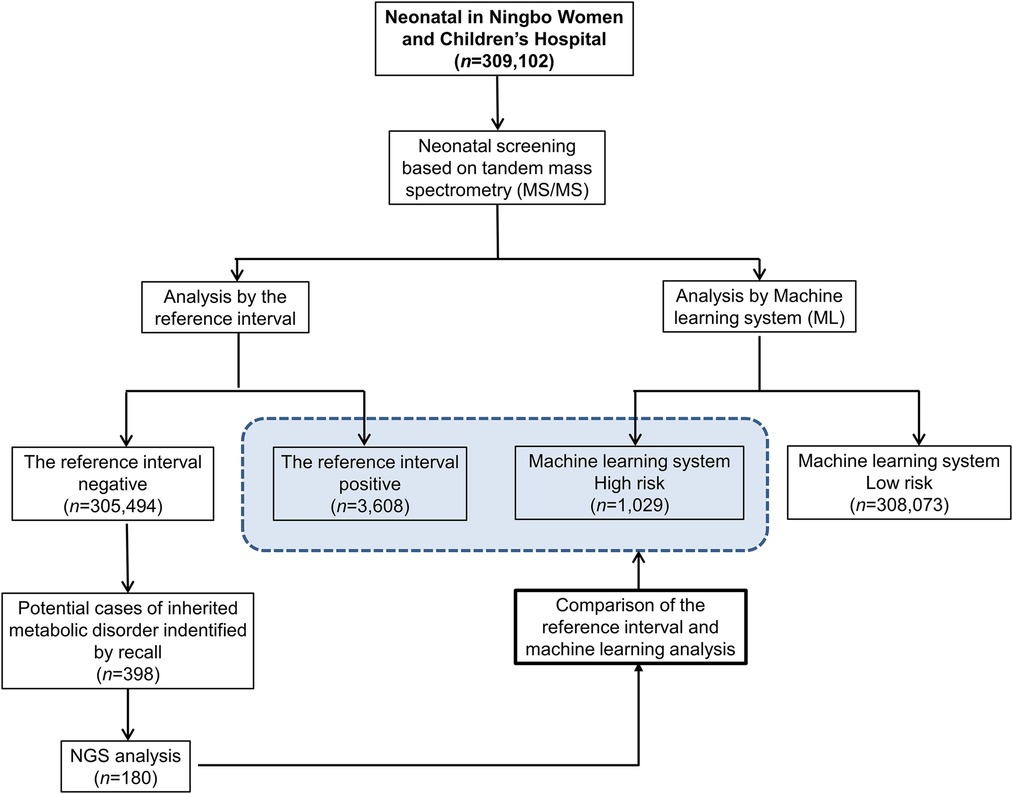
Figure 1. Workflow of the reference interval and machine learning for analyzing neonatal screening data of inherited metabolic diseases.
2.2 MS/MS analysis
Quantification of amino acids and acylcarnitines in dried blood spots (DBS) was performed using the Xevo TQD tandem mass spectrometers (Waters Corp., Milford, MA, USA) in conjunction with the NeoBase Non-derivatized MSMS kit (PerkinElmer, Helsinki, Finland). The analysis comprehensively included 11 amino acids, encompassing a wide range of metabolites associated with the investigated amino acid disorders. In addition, 31 fatty acids (acylcarnitines) and succinylacetone were incorporated into the analysis, as they hold relevance to organic acidemias and fatty acid oxidation defects. Integral to our analysis, reference intervals for each metabolite were defined within the 0.5th to 99.5th percentile range, using a nonparametric ranking to address the non-normal distribution of our dataset. This careful selection ensures our screening's precision, with intervals refined as our sample pool expands, enhancing diagnosis accuracy by reducing false positives and negatives. The detailed information regarding the analyzed metabolites and their corresponding reference intervals can be found in Table S1.
2.3 Genetic testing and bioinformatics analysis
Genomic DNA was extracted from DBS or peripheral blood obtained from patients using the OMEGA Genomic DNA Extraction Kit (OMEGA Biotech, United States). Subsequently, targeted sequencing was conducted using the basic edition panel of IMDs (Genuine Diagnostic Laboratory, Hangzhou, China) to detect 94 genes, including SLC22A5, PAH, PTS, MUT, and other relevant genes. Target regions’ sequences were enriched through multiple probe hybridizations using the Agilent SureSelect Human Exon Sequence Capture Kit. Following enrichment, capture products were purified using Agencourt AMPure XP beads (Beckman Coulter). After purification and quality testing, the sequencing libraries were quantified using the Illumina DNA standard and Primer Premix Kit (Kapa), and subsequently subjected to massively parallel sequencing using the Illumina MiSeq platform. All potentially pathogenic variants were validated through Sanger sequencing utilizing specific primers. Polymerase chain reaction (PCR) conditions followed TaKaRa LA PCRTM Kit Ver.2.1 (TaKaRa). The trans status of all compound heterozygous variants was determined. Identified variants underwent scrutiny against databases such as the Human Gene Mutation (HGMD) Database, ClinVar, ExAC consortium, gnomAD, 1,000 Genome Project database, the laboratory's internal database (∼20,000 mutations), and relevant literature. Novel missense variants were further assessed for potential pathogenicity using tools integrated into VarSome, including SIFT, PolyPhen-2, and MutationTaster. Variant classification followed the standards and guidelines set forth by the American College of Medical Genetics and Genomics (ACMG).
2.4 Data collection and standardization for ML system model
a. Laboratory Background Information: This category covered various elements, including instrument models, types of reagents (commercial or self-made), types of quality control products, laboratory quality control rules, sizes of perforated blood spots, types of filter paper, cold chain transportation of blood samples, types of blood collection needles, succinylacetone treatment methods, normal population ranges, and interpretation rules.
b. Quality Control Data: This section comprised information such as quality control numbers, types of quality control, quality control batch numbers, amino acid internal standard batch numbers, acylcarnitine internal standard batch numbers, factory times, experimental times, and test values for each quality control analyte.
c. Screening Test Data: Included data on screening numbers, mother's ages, gestational ages, gravidities, parities, hyperthyroidism presence, anti-thyroid drug use, breastfeeding methods, milk consumption, native places (provinces and cities), places of residence (provinces and cities), baby genders, birth dates, birth weights, initial screening conclusions, recall review conclusions, sample numbers, screening times, blood collection dates, delivery dates, experimental dates, experimental methods (derivative or non-derivative), quality control numbers, and detection concentrations for each analyte.
d. Positive Case Data: This category covered screening numbers, confirmed diseases, urine organic acid tests, blood ammonia tests, blood gas analyses, blood routines, liver function tests, vitamin B12 tests, imaging examinations, genetic test information.
e. Standardized median multiple (multiple of the medium, MoM) methods were applied in which the median of the original concentration divided by the biochemical indicators was applied to the detection indicators to eliminate the influence of regional and laboratory differences. We then trained the disease model by combining the MoM, gestational week, neonatal blood collection interval, neonatal weight, and corresponding IMDs.
2.5 ML system model
The ML framework utilized in this study has been previously established and published by our collaborative partners, as detailed in the prior research (12, 13). This ML system model for IMDs was crafted by Zhejiang Biosan Biochemical Technologies Co. Ltd. To facilitate the training and testing of this model, an extensive dataset encompassing over 3.67 million neonatal screening data and 3,000 confirmed cases were collected from 31 hospitals between February 2010 and September 2019. Nine pertinent classification algorithms, comprising logistic regression, linear discriminant analysis, decision trees, random forest, extremely randomized trees, gradient boosting, adaptive boosting, support vector machine, and k-nearest neighbors, were systematically applied to analyze 31 IMDs. The detailed list of IMDs was presented in Table 1.
The construction of the ML system model followed a structured progression:
a. Indicator Construction and Selection: Core indicators were selected using information gain and correlation coefficients, culminating in the creation of feature combinations.
b. Model Selection and Training: During the model training phase, the screening data was partitioned into training and test sets, adhering to an 8:2 ratio. Subsequently, the most proficient model was identified through the integration of various learning models, including random forest, gradient boosting, and artificial neural network algorithm.
c. Model Evaluation: Initially, a 100% identification rate for positive cases was successfully achieved by the model. Subsequently, the FP rates were compared to discern and select the optimal training model. Additionally, within the risk assessment process, risk predictions for test samples were conducted by the ML model, estimating the risk associated with samples affected by various inherited metabolic diseases. These predictions were systematically converted into scores ranging from 0 to 100 via a dedicated risk value mapping algorithm. Distinct risk assessment reference interval was established, guided by the risk score and the disease prevalence specific to each location.
Finally, we used our ML technique to design an easy-to-operate Web-based screening system for neonatal metabolic diseases. This system was specifically designed to assess the risk of specific IMDs in each screening dataset, where high- and low-risk cases were classified as positive and negative, respectively.
3 Results
3.1 Neonatal screening and children with positive diagnosis
In our research, a primary screening of 309,102 neonates led to the identification of 3,608 infants with positive screening results, constituting a positive rate of approximately 1.17%. Further investigation of this group uncovered 398 infants who presented abnormal results during the secondary screening phase, prompting considerations of IMDs. Consequently, NGS was employed in 180 of these cases, to confirm the presence of IMDs.
The results showed that 152 out of 180 patients who underwent NGS testing were positive for IMDs, of which 72 were homozygous or compound heterozygous mutations and the other 80 were heterozygous carriers. The results of our neonatal screening displayed a broad spectrum of metabolic disorders, encompassing 16 types of IMDs. These included phenylalanine hydroxylase deficiency (PAHD, 23 cases), tetrahydrobiopterin deficiency (BH4D, 1 case), primary carnitine deficiency (PCD, 30 cases), methylmalonic acidemia (MMA, 6 cases), short-chain acyl-CoA dehydrogenase deficiency (SCADD, 8 cases), Isobutyryl-CoA dehydrogenase deficiency (IBDD, 9 cases), 3-methylcrotonyl-CoA carboxylase deficiency (3-MCCD, 41 cases), 3-hydroxy-3-methylglutaryl CoA lyase deficiency (3-HMGD, 4 cases), neonatal intrahepatic cholestasis caused by citrin deficiency (NICCD, 3 cases), citrullinemia type I (CIT-I, 8 cases), hypermethioninemia (HMET, 9 cases), 2-methylbutyryl-CoA dehydrogenase deficiency (2-MBDD, 4 cases), medium-chain acyl-CoA dehydrogenase deficiency (MCADD, 3 cases), glutaric acidemia type II (GA-II, 1case), ornithine transcarbamoylase deficiency (OTCD, 1 case), and argininemia (HARG, 1 case) (Figure 2). Other IMDs were not found in our study.
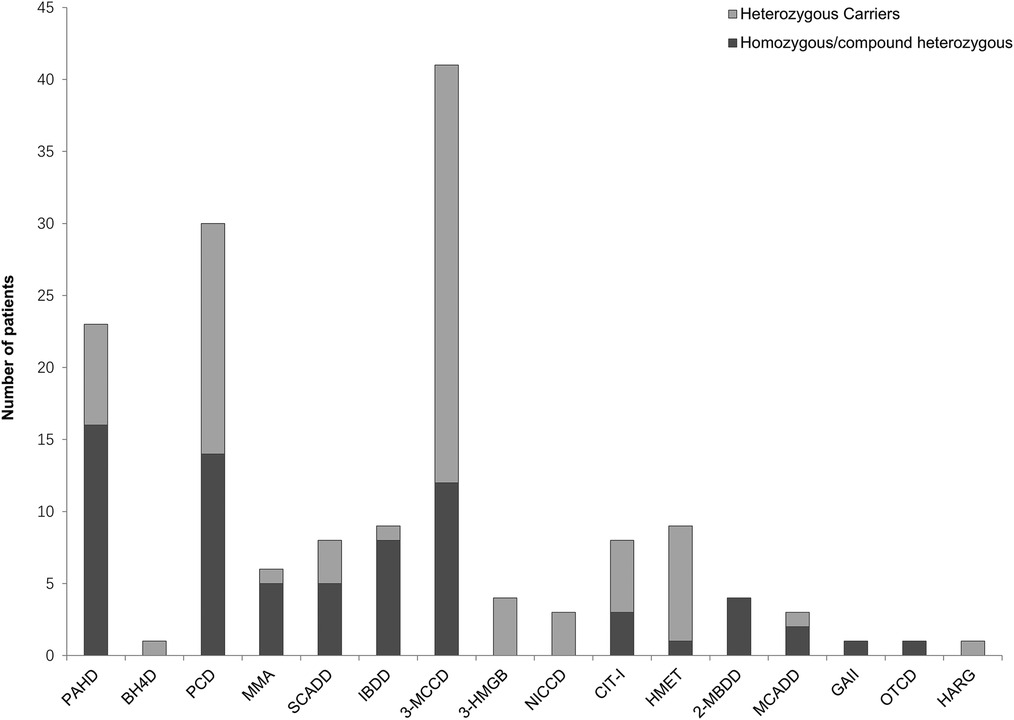
Figure 2. Number of patients with inherited metabolic diseases identified by next-generation sequencing. A total of 152 patients were confirmed to be positive for inherited metabolic diseases, covering a diverse spectrum of 16 types of inherited metabolic diseases. ML, machine Learning; TP, true-positive; PAHD, phenylalanine hydroxylase deficiency; BH4D, tetrahydrobiopterin deficiency; PCD, primary carnitine deficiency; MMA, methylmalonic acidemia; SCADD, short-chain acyl-CoA dehydrogenase deficiency; IBDD, Isobutyryl-CoA dehydrogenase deficiency; 3-MCCD, 3-methylcrotonyl-CoA carboxylase deficiency; 3-HMGD, 3-hydroxy-3-methylglutaryl CoA lyase deficiency; NICCD, neonatal intrahepatic cholestasis caused by citrin deficiency; CIT-I, citrullinemia type I; HMET, hypermethioninemia; 2-MBDD, 2-methylbutyryl-CoA dehydrogenase deficiency; MCADD, medium-chain acyl-CoA dehydrogenase deficiency; GA-II, glutaric acidemia type II; OTCD, ornithine transcarbamoylase deficiency; HARG, argininemia; PHE, phenylalanine; CIT, citrulline; MET, methionine; ARG, arginine; C0, free carnitine; C3, propionylcarnitine; C4, butyrylcarnitine; C5OH, 3-hydroxy (OH) isovalerylcarnitine; C6, hexanoylcarnitine; C8, octanoylcarnitine; C10, decanoylcarnitine; C12, dodecanoylcarnitine; C14, myristoylcarnitine; C14:1, myristoleylcarnitine; C14:2, tetradecadienoylcarnitine.
3.2 ML system model vs. the reference interval in the initial screening
To assess the effectiveness of the ML system model, this study utilizes extensive neonatal screening data. Initially, we analyzed the positive rate between the ML system model and pediatricians using a predefined reference interval in the initial screening process. The findings indicate that, based on the reference interval, 3,608 newborns received a positive diagnosis, while 305,494 cases were categorized as negative during the initial screening phase, resulting in a positive rate of 1.17%. On the other hand, the ML system model identified 1,029 positive cases and 308,073 negative cases in the initial screening, representing a positive rate of 0.33%. The results showed that the positive rate of initial screening significantly decreased from 1.17% to 0.33% compared with the results achieved by the reference interval. This could minimize false positives in the initial screening that cause unnecessary family stress as well as potentially enable cost-effective screening.
3.3 Diagnostic efficiency of ML system model for suspected positive cases
To interpret the potential of ML in enhancing diagnostic efficiency, we employed data from 180 suspected positive cases with NGS results. The application of ML system model diagnosed 142 true-positives (TPs) and 22 true-negatives (TNs). Compared with the reference interval, 144 TPs and 2 TNs were identified. In addition, the application of ML system model detected 6 FPs and 10 FNs, while the utilization of reference interval detected 26 FPs and 8 FNs.
The application of ML system model significantly reduced the number of FPs from 26 to 6, and the detailed numbers of TNs, TPs, FNs, and FPs were presented in Table 2. The sensitivity of the ML system model and the reference interval was 93.42% and 94.74%, respectively. The specificity of the ML system model was 78.57%, while that of the reference interval was 7.14%. Furthermore, the ML system exhibited a positive predictive value (PPV) of 95.95% and a negative predictive value (NPV) of 68.75%, whereas the reference interval yielded a PPV of 84.71% and an NPV of 20%. The ML system model showed a significant increase in both PPV and NPV. The ML system exhibited a higher accuracy. The values of sensitivity, specificity, PPV, NPV, and accuracy were summarized in Table 3.

Table 2. Comparison of diagnostic performance metrics between the ML system model and reference interval in suspected cases.
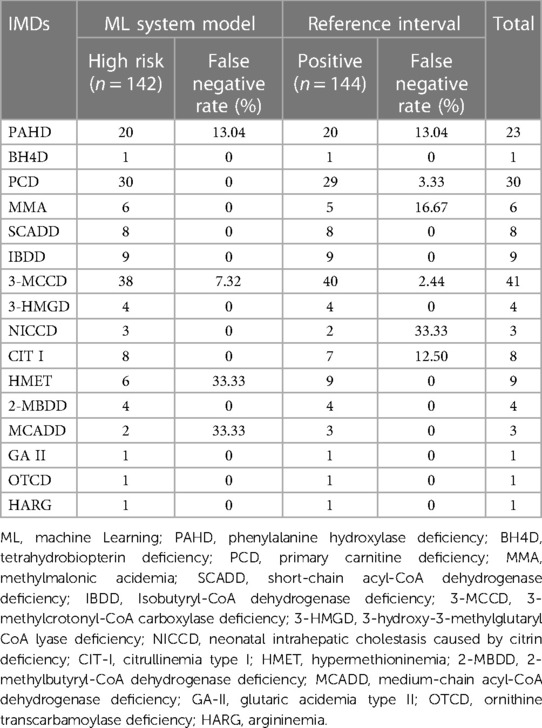
Table 3. Diagnosis and false negative rates of different IMDs: comparison between ML system and reference interval for neonatal screening.
3.4 Comparative analysis of ML system model and reference interval reveals misdiagnosis in suspected inherited metabolic disease cases
Different disorders showed varying diagnostic results when using the ML system model (Table 3). BH4D, PCD, MMA, SCADD, IBDD, NICCD, CPT I, 2-MBDD, GA II, OTCD, and HARG accurately predicted positive cases. Nonetheless, a comprehensive assessment revealed 10 instances of misdiagnosis. There were 3 cases of PAHD, 3 cases of 3-MCCD, 3 cases of HMET, and 1 case of MCADD, and the FN rates were 13.04%, 7.32%, 33.33%, and 33.33%, respectively. In the reference interval, a total of 8 cases of misdiagnosis were found, including 3 cases of PAHD, 1 case of PCD, 1 case of MMA, 1 case of 3-MCCD, 1 case of NICCD, and 1 case of CIT I, and the FP rates were 13.04%, 3.33%, 16.67%, 2.44%, 33.33% and 12.50%, respectively (Table 3). Remarkably, two cases of PAHD were missed by both methods. Table 4 presents an overview of all of 16 misdiagnosed cases and their metabolic indices. Among them, 3 cases were identified with homozygous or compound heterozygous mutations, and the remaining 13 cases were heterozygous carriers. This result suggests that heterozygous carriers are easily misdiagnosed regardless of whether the ML method or the reference interval scheme is used for analysis. It is important to note that misdiagnosis occurs mainly among heterozygous carriers. In some heterozygous carriers, metabolic alterations were observed despite a low-risk designation by the ML system. In contrast, in other heterozygous carriers, indicators of normal metabolism were classified as high risk by the ML system.
3.5 Diagnostic acccuracy and alterations in metabolic indicators in patients with homozygous and compound heterozygous mutations vs. heterozygous carriers
The results of the present study revealed a remarkable diagnostic accuracy of 97.22% in a cohort of 72 patients with homozygous and compound heterozygous mutations, as assessed by the ML system. Similarly, the ML system demonstrated a diagnostic accuracy of 88.75% for 80 heterozygous carriers. Importantly, the metabolic indicators revealed significant differences between patients harboring homozygous mutations and those harboring compound heterozygous mutations compared with heterozygous carriers. Patients with IMDs harboring homozygous mutations and compound heterozygous mutations exhibited more significant alterations in metabolic indicators.
Table 5 provides a comprehensive overview of the diagnostic outcomes and alterations in metabolic indicators identified in patients with IMDs harboring homozygous and compound heterozygous mutations, as well as heterozygous carriers. This table presents detailed insights into the diagnostic efficacy and metabolic changes within these populations.
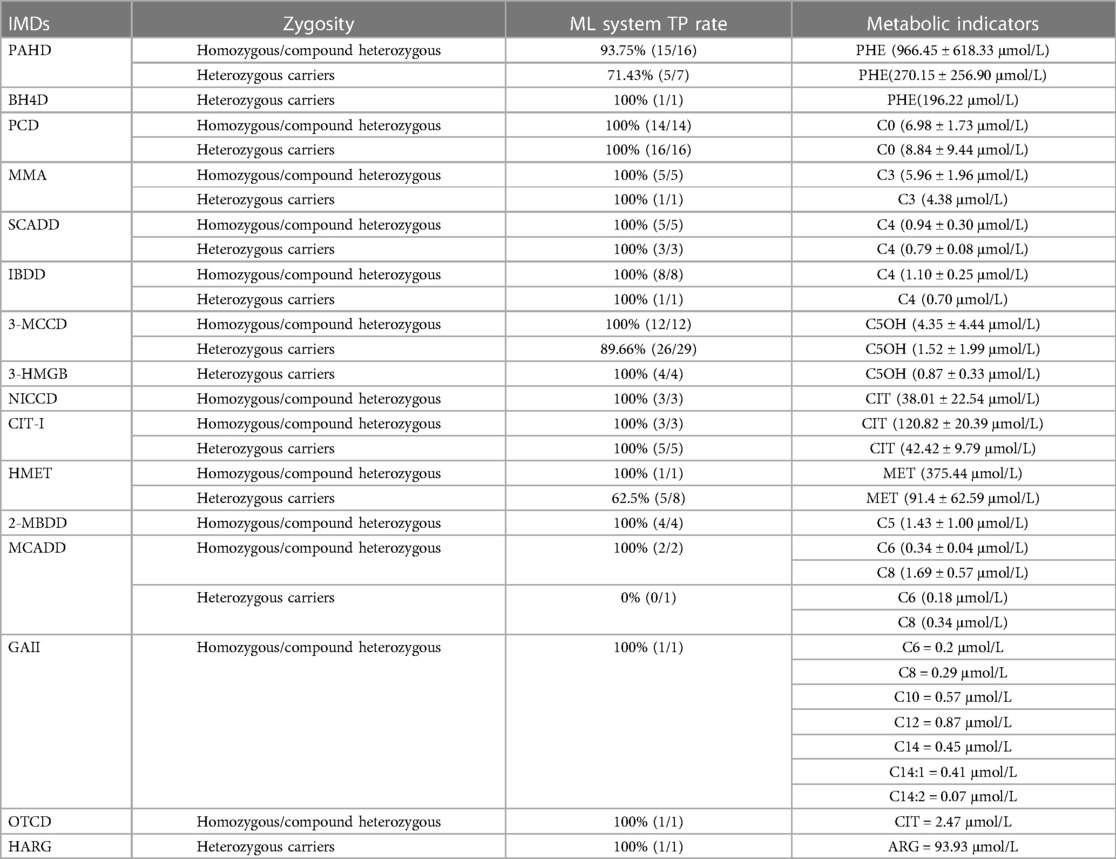
Table 5. Ml system true positive rates, and metabolic indicators in homozygous/compound heterozygous and heterozygous carriers for inherited metabolic disorders.
4 Discussion
In our study, a total of 309,102 newborns underwent screening, leading to the identification of 152 cases with IMDs. The incidence of IMDs with our study population was 1: 2,034, a finding that consistently mirrors the reported rates in Suzhou (1: 3,163) (14) and Quanzhou (1: 2,804) (15). Notably, the most frequent IMDs within our cohort included PADH and 3-MCCD, exhibiting incidence rates of 1 in 13,429 and 1 in 7,539, respectively. It is pertinent to acknowledge that the incidence rates of IMDs exhibit substantial variations when examined across diverse geographical regions.
Recent research endeavors have witnessed the application of ML methodologies in the study of IMDs, albeit often focusing on individual or limited categories of IMDs (16). For instance, Zaunseder et al. (17) endeavored to enhance specificity in neonatal screening for IVA through the adept utilization of machine learning techniques. Similarly, Zhu et al. (18) pioneered the development of a machine learning logistic regression analysis model aimed at ameliorating the diagnostic accuracy associated with Phenylketonuria. Furthermore, Peng et al. (19) conducted an insightful analysis of the Random Forest machine's performance concerning GA-I, MMA, OTCD, and VLCADD. In contrast, our investigation adopts a holistic approach, harnessing the potential of nine distinct algorithms that collectively empower our ML system model to predict and scrutinize 31 distinct IMDs across a broad spectrum. This comprehensive strategy not only extends the scope of applicability but also augments the clinical value of our study, promising multifaceted insights into IMDs diagnosis and management.
It is imperative to acknowledge that ML techniques commonly exhibit enhanced predictive capabilities when applied to substantial datasets (20). In our study, the ML system showcased a notable diminished the initial positive screening rate in contrast to the traditional reference interval. These observations not only signify a reduction in the count of suspected cases but also hold promise for curtailing the necessity of patient recall.
In our investigation, ML model was successfully deployed, accumulating data from the Ningbo region. The experimental outcomes furnish compelling evidence substantiating the effectiveness of the ML system in the diagnosis of IMDs via neonatal screening. In direct comparison with the traditional reference interval, our ML system exhibited a sensitivity level akin to that of 93.42%, thereby maintaining diagnostic acumen. However, it notably improved specificity, elevating it from a mere 7.14% to a substantial 78.57%. This enhancement translated into a noteworthy reduction in the number of FPs, diminishing their count from 26 to a mere 6. This achievement is in alignment with prior research conducted by Peng et al. (19).
Furthermore, our ML system demonstrated a markedly heightened PPV of 95.95%, outperforming the traditional reference interval which yielded a PPV of 84.71%. These findings are congruent with the work of Zhu et al. (18), who reported a notable increase in the PPV for PAHD through the application of an ML model, surging from 19.14% to an impressive 32.16%. The substantial reductions in FPs and the concurrent augmentation of PPV underscore the significant improvements in screening efficiency realized through the application of ML methodologies.
However, in the relentless pursuit of augmented diagnostic precision and efficiency, it becomes imperative to address and comprehend the emergence of FNs, a pivotal concern inherent to any diagnostic framework. In the work of Tang et al. (21), four instances of NICCD were erroneously overlooked, signifying the susceptibility to FN outcomes. Similarly, Lin et al. (22) reported the misdiagnosis of a MADD patient whose acylcarnitine levels resided within the normal reference range upon recall. Within our findings, it's noteworthy that our ML model exhibited the omission of 10 cases, while the traditional reference interval missed 8 cases. The ML system model demonstrated an elevated false negative rate for 3-MCCD, HMET, and MCADD in comparison to the reference interval.
To elucidate the underpinnings of these FNs within the ML system model, we conducted a comprehensive analysis encompassing genetic results and metabolite concentrations for the missed cases. Within the cohort of cases missed by the ML model, which includes 3 cases of HMET, 3 cases of 3-MCCD, 3 cases of PAHD, and 1 case of MCADD, all cases, except for one PAHD case harboring a compound heterozygous mutation in PAH, were identified as heterozygous carriers of these conditions. This observation suggests a proclivity for heterozygous carriers to be susceptible to misclassification by the ML system model, emphasizing the intricacies of carrier identification within diagnostic frameworks.
This study stands as an innovative endeavor in the realm of medical science, pioneering the application of ML techniques for the precise diagnosis of patients afflicted with IMDs harboring pathogenic mutations. Among the cohort of 80 patients classified as heterozygous carriers, the ML system displayed a remarkable capacity by accurately identifying 71 cases, thus underscoring its consistent performance across a spectrum of diverse metabolic indices. It is imperative to note that heterozygous carriers, particularly those bearing partially functional alleles, exhibited discernible variations in metabolic profiles when compared to cases characterized by classical mutations (23–25). The presence of a single mutated allele induced noteworthy alterations in associated proteins and enzymes, thereby engendering variations in pertinent metabolic markers. A compelling observation emerged, revealing that the concentration of metabolites in heterozygous carriers registered a significant reduction when juxtaposed with patients harboring homozygous or compound heterozygous mutations. This revelation has profound implications for heterozygous carriers, particularly within the domain of neonatal screening practices as administered by pediatricians. Our findings provide cogent evidence to posit that heterozygous carriers may manifest variations in metabolic indicators, thereby precipitating potential misclassification. To surmount this challenge, it becomes imperative to embark on further research endeavors aimed at the refinement and augmentation of ML algorithms, with a specific emphasis on enhancing the capacity for accurate carrier detection.
The differences in metabolite profiles observed in heterozygous carriers, especially in PAHD patients, may affect gene expression due to variations in noncoding regions or deep introns, thereby forming compound heterozygous mutations affecting gene function, which also highlights the limitations of targeted region capture sequencing in the detection of noncoding regions and deep intron regions. These undetected mutations significantly affected metabolic profiles, suggesting that genetic interactions are more complex than previously understood and that the availability of NGS could make whole-exome and whole-genome sequencing more affordable and compensate for the lack of targeted region capture. In order to cope with these limitations, ML has become an indispensable supplement. Its ability to analyze large data sets, including metabolic profiles and clinical measures, improves predictive accuracy and reduces diagnostic errors. Newborn screening could be more effective by combining the pattern recognition and prediction capabilities of machine learning with the genetic insights provided by NGS.
To address the risk of missed diagnoses in newborns with initially normal screening results, as well as those born prematurely or with low birth weight, our institution has implemented a seven-year follow-up program. This method is aimed at maximizing the detection of late-onset symptoms of metabolic disorders that may not be evident in initial screenings. Specifically, this long-term monitoring ensures that children who may show symptoms of IMDs are promptly identified and assessed, significantly reducing the risk of missed diagnoses. By adopting this comprehensive follow-up, our study addresses the concerns regarding the limitations of DBS acquisition. The follow-up program was guided by the ‘Expert Consensus on Chinese Pediatric Health Examination’ to monitor and ensure the health of newborns (26). Two key assessments were initiated within the first month after discharge, and during the first year, children underwent quarterly examinations that included a history review, physical examination, physical measurements (e.g., height, weight, body-mass index, and head circumference), laboratory and imaging studies, and cognitive, neuromotor, developmental, language, hearing, vision, and dental health. Subsequently, the follow-up period was a biannual assessment, which was gradually changed to annual. Children with an increased risk of metabolic diseases due to genetic factors should be examined and evaluated intensively.
An intriguing case in our study involved a patient with MMA who harbored a homozygous mutation of the MUT gene c.1663G>A, despite no abnormal changes in metabolic indicators (C3 = 3.96 μmol/L). This highlights that certain IMDs may not manifest obvious changes in MS/MS neonatal screening during the early stages, resulting in FN results. As more cases of this nature are unveiled, the capability of our ML system to identify asymptomatic cases will be increasingly evident, emphasizing its potential toimprove the detection of such cases.
Furthermore, ML algorithms typically benefit from large datasets, as larger datasets theoretically result in improved predictive performance. As our model continues to be implemented in Ningbo, we anticipate that the utilization and advancement of ML algorithms will gain increasing popularity in the near future. This trend is expected to further enhance the prediction accuracy and computational performance of risk assessment models for neonatal IMDs.
5 Conclusions
In conclusion, the application of our ML system exhibited promising effectiveness in pediatric diagnostic screening of IMDs. The model achieved a sensitivity of 93.42% and a specificity of 78.57%, surpassing the performance of the reference interval. Furthermore, the ML system demonstrated increased PPV and NPV. Notably, the ML system proved to be valuable in identifying carrier patients, providing novel insights into the application of ML in pediatric medical practice for diagnosing IMDs through neonatal screening.
Data availability statement
The raw data supporting the conclusions of this article will be made available by the authors without further reservation. The datasets generated and/or analysed during the current study are not publicly available due to regulation of Women and Children's Hospital of Ningbo University and protecting patient personal information. Requests to access these datasets should be directed to the corresponding author HL, Email:bGloYWliby03NzVAMTYzLmNvbQ==.
Ethics statement
The studies involving humans were approved by the Ethics Committee of Ningbo Women and Children’s Hospital. The studies were conducted in accordance with the local legislation and institutional requirements. Written informed consent for participation in this study was provided by the participants’ legal guardians/next of kin. Written informed consent was obtained from the individual(s), and minor(s)' legal guardian/next of kin, for the publication of any potentially identifiable images or data included in this article.
Author contributions
XY: Conceptualization, Methodology, Writing – original draft, Writing – review & editing. SD: Resources, Writing – review & editing. JZ: Resources, Writing – review & editing. ZH: Resources, Writing – review & editing. DZ: Formal Analysis, Funding acquisition, Writing – review & editing. FW: Data curation, Methodology, Writing – review & editing. SW: Supervision, Writing – review & editing. CC: Conceptualization, Funding acquisition, Project administration, Writing – review & editing. HL: Conceptualization, Funding acquisition, Project administration, Writing – review & editing.
Funding
The author(s) declare that financial support was received for the research, authorship, and/or publication of this article.
This work was supported by Social Development Public Welfare Foundation of Ningbo (Grant Nos. 202002N3150 and 2022S035), Innovation Project of Distinguished Medical Team in Ningbo (Grant No. 2022020405), First Municipal Medical and Health Brand Foundation of Ningbo (Grant No. PPXK2018-06), Ningbo Key Research and Development Program (Grant No. 2023Z178), and Medical Health Science and Technology Project of Zhejiang Provincial Health Commission (Grant No. 2023KY1121).
Acknowledgments
The authors gratefully acknowledge the valuable contributions of Zhen Yang from BGI Genomics Co., Ltd. (Shenzhen, China), and Chenggang Huang from Biosan Co., Ltd. (Hangzhou, China). The valuable assistance provided by their involvement in the experiments and their insightful discussions significantly contributed to the success of this study.
Conflict of interest
The authors declare that the research was conducted in the absence of any commercial or financial relationships that could be construed as a potential conflict of interest.
Publisher's note
All claims expressed in this article are solely those of the authors and do not necessarily represent those of their affiliated organizations, or those of the publisher, the editors and the reviewers. Any product that may be evaluated in this article, or claim that may be made by its manufacturer, is not guaranteed or endorsed by the publisher.
Supplementary material
The Supplementary Material for this article can be found online at: https://www.frontiersin.org/articles/10.3389/fped.2024.1366891/full#supplementary-material
References
1. Ferreira CR, van Karnebeek C. Inborn errors of metabolism. Handb Clin Neurol. (2019) 162:449–81. doi: 10.1016/B978-0-444-64029-1.00022-9
2. Gelb MH, Basheeruddin K, Burlina A, Chen HJ, Chien YH, Dizikes G, et al. Liquid chromatography-tandem mass spectrometry in newborn screening laboratories. Int J Neonatal Screen. (2022) 8(4):62. doi: 10.3390/ijns8040062
3. Zhang H, Wang Y, Qiu Y, Zhang C. Expanded newborn screening for inherited metabolic disorders by tandem mass spectrometry in a northern Chinese population. Front Genet. (2022) 13:801447. doi: 10.3389/fgene.2022.801447
4. Peng G, Tang Y, Gandotra N, Enns GM, Cowan TM, Zhao H, et al. Ethnic variability in newborn metabolic screening markers associated with false-positive outcomes. J Inherit Metab Dis. (2020) 43(5):934–43. doi: 10.1002/jimd.12236
5. Yang L, Zhang Y, Yang J, Huang X. Effects of birth weight on profiles of dried blood amino-acids and acylcarnitines. Ann Clin Biochem. (2018) 55(1):92–9. doi: 10.1177/0004563216688038
6. Bo R, Awano H, Nishida K, Fujioka K, Nishiyama A, Miyake O, et al. False positive cases of elevated tetradecenoyl carnitine in newborn mass screening showed significant loss of body weight. Mol Genet Metab Rep. (2020) 24:100634. doi: 10.1016/j.ymgmr.2020.100634
7. Wilson C, Knoll D, de Hora M, Kyle C, Glamuzina E, Webster D. The risk of fatty acid oxidation disorders and organic acidemias in children with normal newborn screening. JIMD Rep. (2017) 35:53–8. doi: 10.1007/8904_2016_25
8. Sultan AS, Elgharib MA, Tavares T, Jessri M, Basile JR. The use of artificial intelligence, machine learning and deep learning in oncologic histopathology. J Oral Pathol Med. (2020) 49(9):849–56. doi: 10.1111/jop.13042
9. Rauschert S, Raubenheimer K, Melton PE, Huang RC. Machine learning and clinical epigenetics: a review of challenges for diagnosis and classification. Clin Epigenetics. (2020) 12(1):51. doi: 10.1186/s13148-020-00842-4
10. Usha RG, Kadali S, Kurma RB, Shaheena D, Naushad SM. Application of machine learning tools and integrated OMICS for screening and diagnosis of inborn errors of metabolism. Metabolomics. (2023) 19(5):49. doi: 10.1007/s11306-023-02013-x
11. Zhou M, Deng L, Huang Y, Xiao Y, Wen J, Liu N, et al. Application of the artificial intelligence algorithm model for screening of inborn errors of metabolism. Front Pediatr. (2022) 10:855943. doi: 10.3389/fped.2022.855943
12. Lin B, Yin J, Shu Q, Deng S, Li Y, Jiang P, et al. Integration of machine learning techniques as auxiliary diagnosis of inherited metabolic disorders: promising experience with newborn screening data. In: Wang X, Gao H, Iqbal M, Min G, editors. Collaborative Computing: Networking, Applications and Worksharing: 15th EAI International Conference. London, UK: Springer International Publishing (2019). p. 334–49.
13. Yang RL, Yang YL, Wang T, Xu WZ, Yu G, Yang JB, et al. Establishment of an auxiliary diagnosis system of newborn screening for inherited metabolic diseases based on artificial intelligence technology and a clinical trial. Zhonghua Er Ke Za Zhi. (2021) 59(4):286–93. doi: 10.3760/cma.j.cn112140-20201209-01089
14. Wang T, Ma J, Zhang Q, Gao A, Wang Q, Li H, et al. Expanded newborn screening for inborn errors of metabolism by tandem mass spectrometry in Suzhou, China: disease spectrum, prevalence, genetic characteristics in a Chinese population. Front Genet. (2019) 10:1052. doi: 10.3389/fgene.2019.01052
15. Lin Y, Zheng Q, Zheng T, Zheng Z, Lin W, Fu Q. Expanded newborn screening for inherited metabolic disorders and genetic characteristics in a southern Chinese population. Clin Chim Acta. (2019) 494:106–11. doi: 10.1016/j.cca.2019.03.1622
16. Zaunseder E, Haupt S, Mütze U, Garbade SF, Kölker S, Heuveline V. Opportunities and challenges in machine learning-based newborn screening-a systematic literature review. JIMD Rep. (2022) 63(3):250–61. doi: 10.1002/jmd2.12285
17. Zaunseder E, Mütze U, Garbade SF, Haupt S, Feyh P, Hoffmann GF, et al. Machine learning methods improve specificity in newborn screening for isovaleric aciduria. Metabolites. (2023) 13(2):304. doi: 10.3390/metabo13020304
18. Zhu Z, Gu J, Genchev GZ, Cai X, Wang Y, Guo J, et al. Improving the diagnosis of phenylketonuria by using a machine learning-based screening model of neonatal MRM data. Front Mol Biosci. (2020) 7:115. doi: 10.3389/fmolb.2020.00115
19. Peng G, Tang Y, Cowan TM, Enns GM, Zhao H, Scharfe C. Reducing false-positive results in newborn screening using machine learning. Int J Neonatal Screen. (2020) 6(1):16. doi: 10.3390/ijns6010016
20. Beam AL, Kohane IS. Big data and machine learning in health care. JAMA. (2018) 319(13):1317–8. doi: 10.1001/jama.2017.1839
21. Tang C, Tan M, Xie T, Tang F, Liu S, Wei Q, et al. Screening for neonatal inherited metabolic disorders by tandem mass spectrometry in Guangzhou. Zhejiang Da Xue Xue Bao Yi Xue Ban. (2021) 50(4):463–71. doi: 10.3724/zdxbyxb-2021-0260
22. Lin Y, Zhang W, Chen Z, Lin C, Lin W, Fu Q, et al. Newborn screening and molecular features of patients with multiple acyl-CoA dehydrogenase deficiency in Quanzhou, China. J Pediatr Endocrinol Metab. (2021) 34(5):649–52. doi: 10.1515/jpem-2020-0694
23. Weiss KJ, Berger U, Haider M, Wagner M, Märtner EMC, Regenauer-Vandewiele S, et al. Free carnitine concentrations and biochemical parameters in medium-chain acyl-CoA dehydrogenase deficiency: genotype-phenotype correlation. Clin Genet. (2023) 103(6):644–54. doi: 10.1111/cge.14316
24. Macchione F, Salviati L, Bordugo A, Vincenzi M, Camilot M, Teofoli F, et al. Multiple acyl-COA dehydrogenase deficiency in elderly carriers. J Neurol. (2020) 267(5):1414–9. doi: 10.1007/s00415-020-09729-z
25. Lin Y, Zheng W, Chen Y, Huang C, Fu Q, Chen D, et al. Incorporating second-tier genetic screening for multiple acyl-CoA dehydrogenase deficiency. Clin Chim Acta. (2022) 537:181–7. doi: 10.1016/j.cca.2022.10.024
Keywords: machine learning, neonatal screening, inherited metabolic diseases, diagnostic efficiency, carrier
Citation: Yang X, Ding S, Zhang J, Hu Z, Zhuang D, Wang F, Wu S, Chen C and Li H (2024) The significance of machine learning in neonatal screening for inherited metabolic diseases. Front. Pediatr. 12:1366891. doi: 10.3389/fped.2024.1366891
Received: 7 January 2024; Accepted: 11 March 2024;
Published: 20 March 2024.
Edited by:
Generoso Andria, University of Naples Federico II, ItalyReviewed by:
Hidde Huidekoper, Erasmus Medical Center, NetherlandsAlessandro Rossi, University of Naples Federico II, Italy
© 2024 Yang, Ding, Zhang, Hu, Zhuang, Wang, Wu, Chen and Li. This is an open-access article distributed under the terms of the Creative Commons Attribution License (CC BY). The use, distribution or reproduction in other forums is permitted, provided the original author(s) and the copyright owner(s) are credited and that the original publication in this journal is cited, in accordance with accepted academic practice. No use, distribution or reproduction is permitted which does not comply with these terms.
*Correspondence: Changshui Chen Y2hlbmNzQDEzOS5jb20= Haibo Li bGloYWliby03NzVAMTYzLmNvbQ==
†These authors have contributed equally to this work and share first authorship