- 1Department of Biochemistry, Yong Loo Lin School of Medicine, National University of Singapore, Dundee, Singapore
- 2Duke-NUS Graduate School, Singapore, Singapore
- 3Division of Cellular and Molecular Research, Humphrey Oei Institute of Cancer Research, National Cancer Centre Singapore, Singapore, Singapore
- 4Department of Medicine, Yong Loo Lin School of Medicine, Cardiovascular Research Institute, National University of Singapore, Singapore, Singapore
- 5NovogeneAIT Genomics Singapore, Singapore, Singapore
- 6Department of Pediatrics, Yong Loo Lin School of Medicine, National University of Singapore, Singapore, Singapore
- 7Department of Cardiology, National Heart Centre Singapore, Singapore, Singapore
- 8Genome Institute of Singapore, Singapore, Singapore
- 9NUS Graduate School for Integrative Sciences and Engineering, National University of Singapore, Singapore, Singapore
- 10Translational Laboratory in Genetic Medicine, Singapore, Singapore
Statins can cause muscle symptoms resulting in poor adherence to therapy and increased cardiovascular risk. We hypothesize that combinations of potentially functional SNPs (pfSNPs), rather than individual SNPs, better predict myalgia in patients on atorvastatin. This study assesses the value of potentially functional single nucleotide polymorphisms (pfSNPs) and employs six machine learning algorithms to identify the combination of SNPs that best predict myalgia.
Methods: Whole genome sequencing of 183 Chinese, Malay and Indian patients from Singapore was conducted to identify genetic variants associated with atorvastatin induced myalgia. To adjust for confounding factors, demographic and clinical characteristics were also examined for their association with myalgia. The top factor, sex, was then used as a covariate in the whole genome association analyses. Variants that were highly associated with myalgia from this and previous studies were extracted, assessed for potential functionality (pfSNPs) and incorporated into six machine learning models. Predictive performance of a combination of different models and inputs were compared using the average cross validation area under ROC curve (AUC). The minimum combination of SNPs to achieve maximum sensitivity and specificity as determined by AUC, that predict atorvastatin-induced myalgia in most, if not all the six machine learning models was determined.
Results: Through whole genome association analyses using sex as a covariate, a larger proportion of pfSNPs compared to non-pf SNPs were found to be highly associated with myalgia. Although none of the individual SNPs achieved genome wide significance in univariate analyses, machine learning models identified a combination of 15 SNPs that predict myalgia with good predictive performance (AUC >0.9). SNPs within genes identified in this study significantly outperformed SNPs within genes previously reported to be associated with myalgia. pfSNPs were found to be more robust in predicting myalgia, outperforming non-pf SNPs in the majority of machine learning models tested.
Conclusion: Combinations of pfSNPs that were consistently identified by different machine learning models to have high predictive performance have good potential to be clinically useful for predicting atorvastatin-induced myalgia once validated against an independent cohort of patients.
Introduction
Cardiovascular disease is a leading cause of death worldwide (World Health Organization – Cardiovascular Disease, 2020). High blood cholesterol levels increase the risk of cardiovascular disease, making lipid-lowering medications such as statins important for the therapeutic management of this risk factor (SEARCH Collaborative Group et al., 2010; Cholesterol Treatment Trialists et al., 2012; Silverman et al., 2016). Statins, or 3-hydroxy-3-methylglutaryl CoA reductase inhibitors, are generally well tolerated. However up to 25% of individuals have reported some degree of statin-associated muscle symptoms (SAMS) (Bruckert et al., 2005; Cohen et al., 2012). These side effects range from myalgia (with or without elevations in serum creatine kinase) to severe rhabdomyolysis (Alfirevic et al., 2014). Although severe forms of muscle toxicity such as myopathy and rhabdomyolysis are rare, the most common event leading to discontinuation of statins are muscle symptoms, in particular those without significant elevation in creatine kinase (McGinnis et al., 2007; Wei et al., 2013; Stroes et al., 2015). As treatment of hypercholesterolaemia is life-long, poor adherence to prescribed statin therapy increases the risk of cardiovascular events (Chowdhury et al., 2013; Saxon and Eckel, 2016). It is therefore important to be able to identify patients with muscle symptoms of pharmacological origin so that they can receive appropriate management. These patients could also receive alternative non-statin therapies such as the more expensive PSCK9 inhibitors or ezetimibe (Bakar et al., 2018).
Previous pharmacogenomic studies have reported genetic variations that are associated with SAMS, most notably the rs4149056 polymorphism in the SLCO1B1 gene. (Link et al., 2008; Wilke et al., 2012). This polymorphism has been included in CPIC guidelines for simvastatin therapy. While the pharmacokinetic basis of rs4149056 and simvastatin-induced myopathy has been established in several clinical studies (Pasanen et al., 2006; Voora et al., 2009; Carr et al., 2013), it is unclear whether this variant is also associated with SAMS in patients on lower doses of statin, milder myalgia or from different populations (Donnelly et al., 2011.; Hubacek et al., 2015; Sai et al., 2016; Zhong et al., 2018). For instance, Donnelly et al. (2011) reported an association of SLCO1B1 variants with mild myalgia in patients receiving high doses of statin, but Huback et al. (2015) reported that SLCO1B1 polymorphisms were not associated with risk of myalgia in a Czech population. Furthermore, this association is strongest for simvastatin, and there are conflicting reports for atorvastatin treatment which is the most widely prescribed high-potency statin (Voora et al., 2009; Carr et al., 2013; Brunham et al., 2018). Atorvastatin, simvastatin and other statins differ in the ring that is attached to their active moieties as well as in the form that they are administered in (Turner and Pirmohamed, 2019; Ward et al., 2019). These statins therefore have different pharmacokinetic characteristics and involve different genes and SNPs for their metabolism and transport.
In addition to SNPs in the SLCO1B1 gene, SNPs in several other pathways including statin metabolism [e.g., cytochrome P450 (CYP) genes (Frudakis et al., 2007; Shek et al., 2017) and glycine amidinotransferase (GATM) (Mangravite et al., 2013)], statin transport [e.g., ATP binding cassette (ABC) transporters (Zhang et al., 2019)], and immune response (e.g human leukocyte antigen (HLA) (Sai et al., 2016) and leukocyte immunoglobulin-like receptor (LILR) (Siddiqui et al., 2017)] have also been implicated in SAMS (reviewed in Ward et al., 2019; Turner and Pirmohamed, 2019). For some of these SNPs, further studies have shown that the associations do not replicate (e.g., the GATM variant) (Floyd et al., 2014; Luzum et al., 2015). Clinical factors such as age, sex, ethnicity, daily dose, body mass index, drug-drug interactions, comorbidities, duration of statin use and use of concomitant medications have also been implicated with SAMS (SEARCH Collaborative Group et al., 2010; Cohen et al., 2012; Tournadre, 2020), although the association of these covariates again varies with each study.
Hence, this study aims to examine the role of genetic and clinical factors for predicting atorvastatin-induced myalgia in the Singapore population, which comprises mainly of individuals of Chinese, Malay and Indian descent. Genetic polymorphisms associated with myalgia were obtained by whole genome sequencing (WGS). Unlike exome or targeted sequencing technologies previously used in the discovery of statin associated myopathy variants (Ruano et al., 2007; Ruano et al., 2011; Bakar et al., 2018; Floyd et al., 2019), WGS allows for the detection of polymorphisms in both coding and non-coding regions. Furthermore, our group has found that non-coding regions contain a larger proportion of potentially functional SNPs compared to coding regions (Bachtiar et al., 2019a), which makes WGS a more suitable platform compared to other technologies. Floyd et al. (2019) reported that there was no evidence linking rare coding variants to adverse statin reactions, and given our small sample size, we have decided to focus on common variants in this study.
The potentially functional SNPs (Wang et al., 2011) uncovered from this study, as well as from other known genes in the atorvastatin pathway, were used for predicting myalgia using a variety of machine learning approaches. Machine learning has previously been used to predict drug response or dosage in fields such as cancer, psychiatry and cardiovascular disease (Liu et al., 2015; Huang et al., 2018; Athreya et al., 2019). To our knowledge, it has not been applied to predict the risk of statin-induced myalgia based on pharmacogenomic data. Insights gained from this study can therefore help to reveal important clinical and genetic risk factors that are predictive of atorvastatin-induced myalgia, as well as demonstrate the utility of machine learning approaches in pharmacogenomics.
Materials and Methods
Study Cohort
This study examined 183 subjects on atorvastatin therapy from the Surveillance and Pharmacogenomics Initiative for Adverse Drug Reactions (SAPhIRE) project. Written informed consent was obtained from all participants and the study protocol was approved by the National Healthcare Group Domain Specific Review Board (NHG DSRB). For patients who reported muscle pain, severity of symptoms was scored based on two criteria, regional distribution pattern and temporal pattern. The scoring for regional distribution is as follows - “non-specific, intermittent” was given a score of 1, “symmetric hip flexors/thigh aches” was given a score of 3 while “symmetric calf aches” and “symmetric upper proximal aches” were given a score of 2. For temporal pattern, “onset < 4 weeks” was given a score of 3, “4–12 weeks” was given a score of 2 and “>12 weeks” was given a score of 1. The scores for the two patterns were added with scores ranging from 0 (no muscle pain) to 6, and patients who responded with a score of 0–2 were defined as the statin tolerant group while those with a score of 4–6 were defined as the myalgia group. All 30 patients in the myalgia group were selected for further analysis, while 153 out of 946 patients were randomly selected from the atorvastatin tolerant group to form the controls. From this study cohort, 48% were self-reported Chinese, 31% Indian and 21% Malay, and patients had a mean age of 57.4 (95% CI: 55.9–59.0) years, although one patient did not have age data. Patients were treated with atorvastatin for 10–5,046 days with a daily dose ranging from 5 to 80 mg. Demographics (including age, sex, height and weight), comorbidities and medications of all patients were recorded. Each patient provided a venous blood sample which was transferred into EDTA tubes and stored at −80°C for genetic analyses.
Whole Genome Sequencing
Genomic DNA was extracted and purified from whole blood using the Omega Bio-Tek E. Z.N.A. Blood DNA mini kit (Norcross, GA, United States). DNA concentration was measured using Qubit® DNA Assay Kit in Qubit® 2.0 Flurometer (Life Technologies, CA, United States). Fragment distribution of DNA library was measured using the DNA Nano 6000 Assay Kit of Agilent Bioanalyzer 2100 system (Agilent Technologies, CA, United States). A total amount of 1.0 μg DNA per sample was used as input material for the DNA sample preparations. Sequencing libraries were generated using NEBNext® DNA Library Prep Kit following manufacturer’s recommendations and indices were added to each sample. The genomic DNA is randomly fragmented to a size of 350 bp by shearing, then DNA fragments were end polished, A-tailed, and ligated with the NEBNext adapter for Illumina sequencing, and further PCR enriched by P5 and indexed P7 oligos. The PCR products were purified (AMPure XP system) and resultant libraries were analyzed for size distribution by Agilent 2100 Bioanalyzer and quantified using real-time PCR. Sequencing was performed on the Illumina platform (HiSeq X) using a paired-end read length of 150 bp. Data files have been uploaded to the European Nucleotide Archive with accession number PRJEB40922.
Sequence Alignment and Data Processing
Read pairs with adapter contamination, more than 10% bases uncertainty or >50% low quality bases in either read were first discarded. Burrows-Wheeler Aligner (BWA) was utilized to map the paired-end reads to the human reference genome b37 (ftp.broadinstitute.org/bundle/b37/human_g1k_v37_decoy.fasta.gz) and duplicate reads marked using Picard (http://picard.sourceforge.net) (Li and Durbin, 2009). The BAM files were further processed following the GATK Best Practices Workflow (https://www.broadinstitute.org/gatk/guide/best-practices). Single-sample genotypes were called using GATK HaplotypeCaller (McKenna et al., 2010) followed by hard filtering with the following options: QualByDepth > 2.0, FisherStrand < 60.0, MappingQuality > 40, MappingQualityRankSumTest > − 12.5, ReadPosRankSumTest > − 8.0 and StrandOddsRatio < 3.0. Variants were annotated using ANNOVAR according to the hg19 reference genome (Wang et al., 2010). Downstream analyses were only performed on biallelic SNPs that passed all quality filters above, had less than 10% of genotype missingness, deviation from Hardy-Weinberg equilibrium p > 0.001 and minor allele frequency >10%. Genotypic data from myalgia patients, controls and 1,000 Genomes was used in a principal component analysis (PCA) using PLINK 1.9 (Chang et al., 2015) to identify racial stratification in our dataset, and figures were plotted in R.
Univariate and Single Variant Analysis
Statistical analyses for all clinical parameters (expressed as mean, 95% CI) were performed using R 3.6.1. Fisher’s exact tests were used for categorical variables and t-tests for continuous variables. Unlike the chi-squared test, Fisher’s exact test does not require the expected frequencies of cases and controls to be large, and was the more suitable test given the small sample size in this study. To determine the association of genetic polymorphisms with myalgia, binary logistic regression was performed on the 4,554,532 SNPs with known rs numbers using PLINK 1.9. Additive, dominant and recessive models for genotypes were separately tested. Sex was included as a covariate as it was found to be significantly associated with myalgia, and the first two principal components (PCs) were used to correct for population substructure. SNPs obtained from this single variant analysis were ranked according to the lowest p-value out of the three genotypic models tested.
For a 0.1 minor allele frequency cutoff, assuming a reported prevalence rate of 0.2 (prevalence has been reported to be up to 25%) and a case control ratio of 1:5, to detect an odds ratio of 5 with p < 5 × 10−8 and 80% statistical power, a sample size of 34 cases for the additive model and 35 cases for the dominant model is required. However, the prevalence rate of myalgia in this study may not be 0.2 as there were only 30 patients with myalgia out of the 976 patients on atorvastatin therapy whose clinical data was available. Assuming a prevalence rate of 30/976 = 0.03, 66 cases would be required to detect the above effects.
Selection of Potentially Functional SNPs
Potential functionalities of SNPs found in this study were evaluated using the pfSNP resource developed by our laboratory (Wang et al., 2011). pfSNPs include SNPs that reside within regions under natural selection forces, as well as those predicted to alter the expression, structure, function, or activity of the associated gene. For coding SNPs, functionality was determined based on whether the SNP resides within protein modification sites such as phosphorylation sites, within important protein domains/functional regions, or are predicted to affect exonic splice enhancer/silencer sites or nonsense-mediated decay. Furthermore, within the coding region, synonymous mutations were assessed for significant codon usage bias as this could potentially influence the speed of the translation process (Kimchi-Sarfaty et al., 2007), while predicted deleteriousness was used for selecting non-synonymous pfSNPs (Bachtiar et al., 2019b). In addition to the pfSNP resource, expression quantitative trait loci (eQTLs) from the GTEx database (gtexportal.org) (Carithers et al., 2015), the eqtlGen consortium (eqtlgen.org) (Võsa et al., 2018) and the Jansen study (eqtl.onderzoek.io) (Jansen et al., 2017) were also used to identify potentially functional SNPs (pfSNPs). Cumulative counts of potentially functional (pf) SNPs were compared with non-pf SNPs for the top 100 SNPs most associated with myalgia based on univariate association p-values.
Selection of Candidate SNPs for Prediction
Three separate groups of SNPs were used as inputs into the machine learning models. These were: 1) SNPs that were most highly associated with myalgia from our results, 2) SNPs residing in 128 genes in the atorvastatin pathway from the drug databases Drugbank, CHEMBL, CTD and PharmGKB as previously obtained by our group (Supplementary Table S1) (Bachtiar et al., 2019b), and 3) SNPs in nine genes reported to be associated with atorvastatin-induced myalgia from the literature (Supplementary Table S2) (Ruano et al., 2007; Ruano et al., 2011; Brunham et al., 2018). SNPs in these three groups were ranked by their p-value of association with myalgia from our univariate analysis, and the top 50 overall, pf and non-pf SNPs from these three groups were extracted and separately used for training the models. For genes found to be associated with myalgia from the literature, only 20 non-pf SNPs were found. SNPs with missing values as well as those with greater than 80% correlation with a more significant SNP were removed. Non-pf SNPs that had greater than 80% correlation with pfSNPs were also removed from the non-pf group.
Predictions Using Machine Learning
Six classifiers were selected for predicting myalgia. These include regression based methods such as logistic regression and elastic nets; tree based methods such as random forests and boosted trees and other popular machine learning approaches such as neural networks and support vector machines. As there is currently no consensus as to which approach is best for genomic data, these six models were selected as a broad representation of popular machine learning models used for prediction. SNPs that performed well on most or all models represent SNPs that are able to predict myalgia to a high degree of confidence. As the different models use different approaches for learning and prediction, consistent results from the majority of models would increase our confidence about the validity of the results. All predictions were made using the R caret package in conjunction with the glm, glmnet, rf, gbm, nnet and svmRadial packages for training the individual models (Kuhn, 2008). Default caret training settings were used and sex was included as a predictor in all models. Predictive performance using the top 5–50 (in intervals of 5) overall, pf and non-pf SNPs from all three groups were separately obtained using the average 5-round 5-fold cross validation area under ROC curve (AUC) as the performance score. The unpaired t-test with Bonferroni correction (n = 3) was used to determine if there was a significant difference in mean AUC values of models using pfSNPs, non-pf SNPs and all SNPs. All six models were also trained without SNP data using 1) only sex as a predictor and 2) all clinical characteristics as predictors for determining the baseline model.
Results
Demographic and Clinical Characteristics
There were 88 Chinese, 57 Indians and 38 Malays in the dataset and patients ranged in age from 25 to 81 years (mean = 57.4, CI = 55.9–59.0). The ethnic distribution in the study cohort is generally reflective of the Singapore population, although there was a lower percentage of Chinese and a higher percentage of Indians in the study cohort. This can be attributed to the higher prevalence of coronary heart disease in Singapore Indians requiring statin pharmacotherapy resulting in a higher proportion of Indians among statin users (Hughes et al., 1990; Ounpuu and Yusuf, 2003). All patients were treated with atorvastatin and the demographic and clinical characteristics of patients according to myalgia status is shown in Table 1. Of these characteristics, only sex was found to be significant (p < 0.05), with females more likely to have statin induced myopathy than males (Table 1). None of the comorbidities and drug treatments were found to be significantly associated with myalgia.
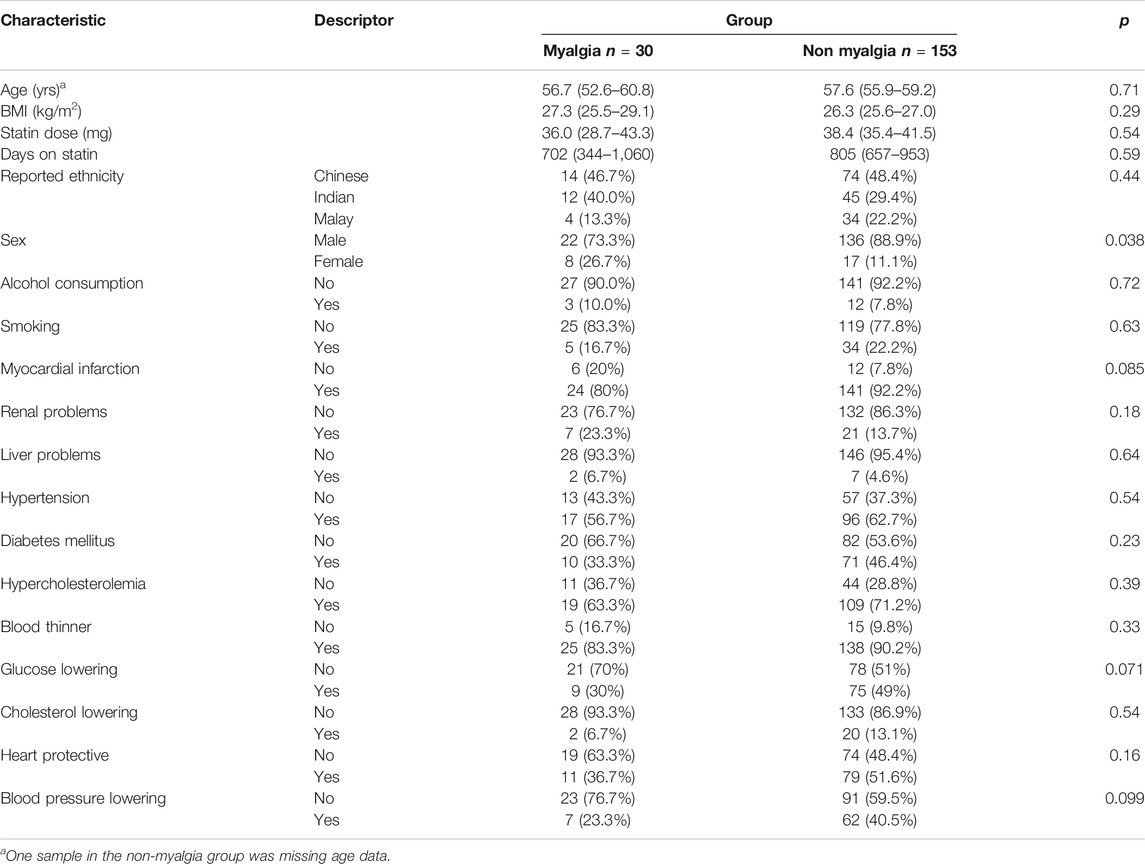
TABLE 1. Clinical/demographic characteristics of myalgia (cases) and non-myalgia (controls) subjects.
Population Stratification
PCA analyses showed that Chinese patients from our dataset clustered more closely with 1,000 Genomes East Asian populations, and Indian patients from our dataset clustered more closely with 1,000 Genomes South Asian populations (Supplementary Figure S1A). Chinese, Malay and Indian patients from our dataset were also fairly well separated when projected on to the first two principal components (Supplementary Figure S1B), although there was some overlap between Chinese and Malay patients due to genetic admixture between the two ethnicities (Deng et al., 2015).
Single Variant Analyses
4,554,532 SNPs with known rs numbers passed quality control in our dataset, with the majority of variants residing in intergenic and intronic regions (Supplementary Figure S2). To identify single SNP variants that might be associated with statin induced myalgia, logistic regression adjusting for the first two principal components and sex was performed. Most of the SNPs that were highly associated with myalgia were located outside exons and untranslated (UTR) regions (Figure 1A), highlighting an important limitation of exome based platforms. A p-value of 5 × 10−8 is commonly used to determine significance in genome wide studies, based on an assumption of 1,000,000 independent tests and patterns of linkage disequilibrium in individuals of European descent (Fadista et al., 2016). Although none of the variants in our analyses met this p-value threshold, 15 suggestive SNPs (p < 1 × 10−5) were found, with genes RHOBTB1 on chromosome 10 and SUSD1 on chromosome 9 containing the most number of suggestive SNPs, all of which were potentially functional (Figure 1B; Table 2). The top SNP for RHOBTB1, rs10821852, is an intronic SNP with an odds ratio (OR) of 5.66 (95% CI: 2.70–11.8, p: 4.23 × 10−6, assuming an additive genotypic model) while the top SNP for SUSD1, rs10981237 is an intronic SNP with an OR of 21.67 (95% CI: 5.68–82.8, p: 6.81 × 10−6, assuming a recessive genotypic model) (Table 2).
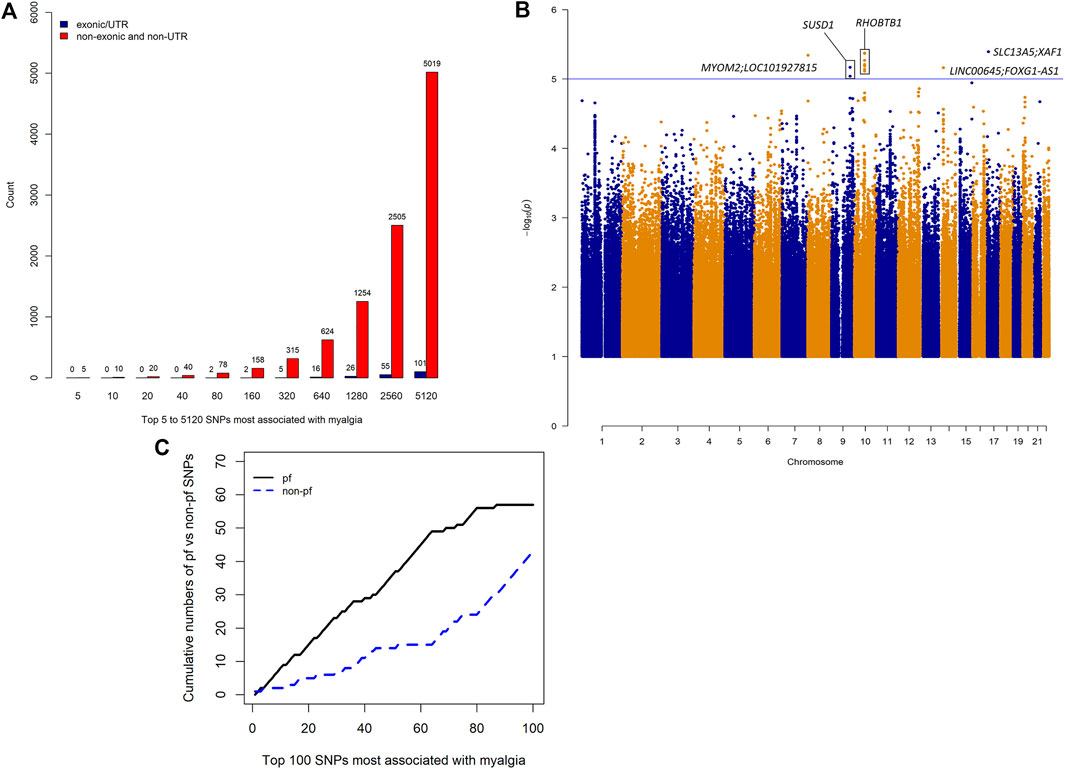
FIGURE 1. Whole genome sequencing results. (A) Number of exonic/UTR variants vs non-exonic/non-UTR variants in the top 5 to 5,120 SNPs most associated with myalgia. (B) Manhattan plot of association of SNPs with atorvastatin-induced myalgia. The line indicates a p-value threshold of 1 × 10−5, which can be considered to be a suggestive threshold of genome wide significance for small sample sizes. (C) Cumulative numbers of potentially functional (pf) and non-pf SNPs in the top 100 SNPs most associated with myalgia.
Distribution of Potentially Functional SNPs
Of the 4,554,532 SNPs with known rs numbers, approximately 60% (2,774,804) were potentially functional. The cumulative number of pfSNPs was consistently higher than that of non-pf SNPs in the top 100 SNPs most associated with myalgia (Figure 1C).
Good Predictive Performance Using 15 SNPs
Predictive performance was greatest when using SNPs that were highly associated with myalgia from this study (highest AUC: 1, Figure 2) followed by SNPs in atorvastatin pathway genes (highest AUC: 0.936, Figure 3) and SNPs in myalgia associated genes from previous studies (highest AUC: 0.794, Figure 4). For all models and inputs, close to maximal AUCs were generally achieved when 15 SNPs were used, after which there was either minimal increase in predictive performance, or a decrease in AUC values (Figures 2–4). However, for SNPs in myalgia associated genes from previous studies, mean AUC values did not increase with increasing number of SNPs, suggesting that most of these SNPs were not predictive (Figure 4). Out of the top five pfSNPs in this group, four were within the ABCG2 gene while one was at the HTR3B locus. In terms of the best performing machine learning model when 15 SNPs were used as inputs, the best model was support vector machine (AUC: 0.990) for SNPs found from this study (Figure 2), random forest (AUC: 0.89) for atorvastatin pathway SNPs (Figure 3) and boosted tree (AUC:0.790) for SNPs in genes from previous studies (Figure 4).
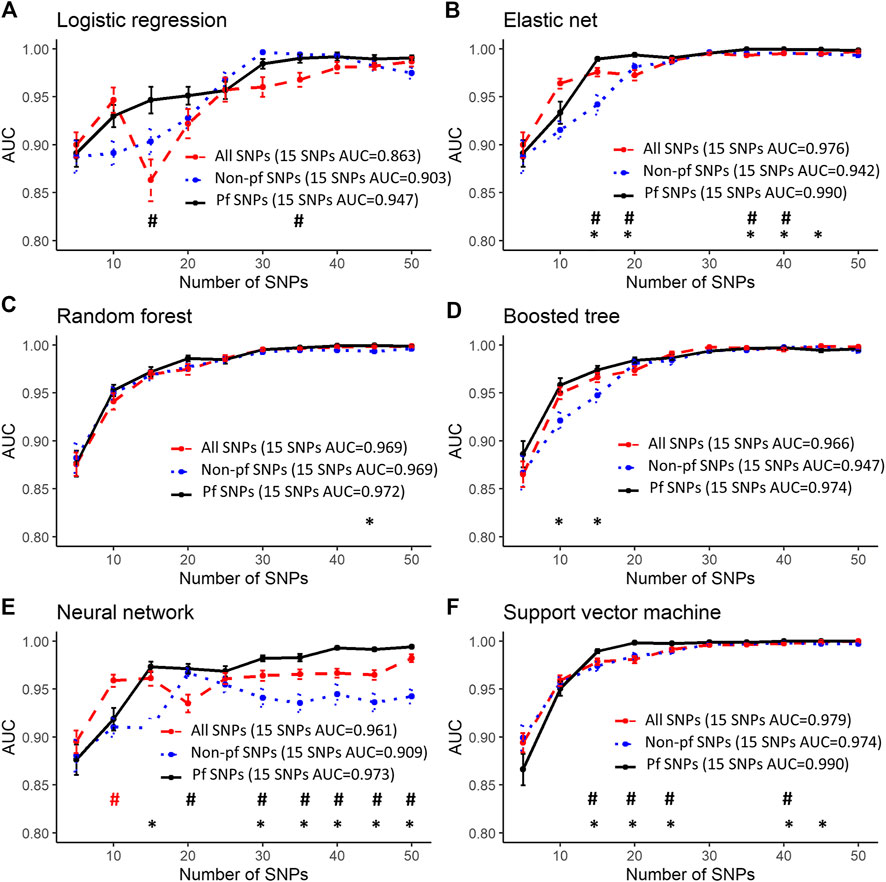
FIGURE 2. Predictive performance using the top 5 to 50 SNPs most associated with myalgia from our dataset. Error bars denote the standard error of the mean. #’s indicate statistical significance when comparing between all SNPs and pfSNPs while *’s indicate statistical significance when comparing between non-pf SNPs and pfSNPs. Statistical significance when comparing between all SNPs and non-pfSNPs is not shown. The colors represent the input set with the higher AUC (red—all SNPs, blue—non-pf SNPs and black—pfSNPs). Bonferroni corrected unpaired t-test p-values (p < 0.05) were used for determining statistical significance.
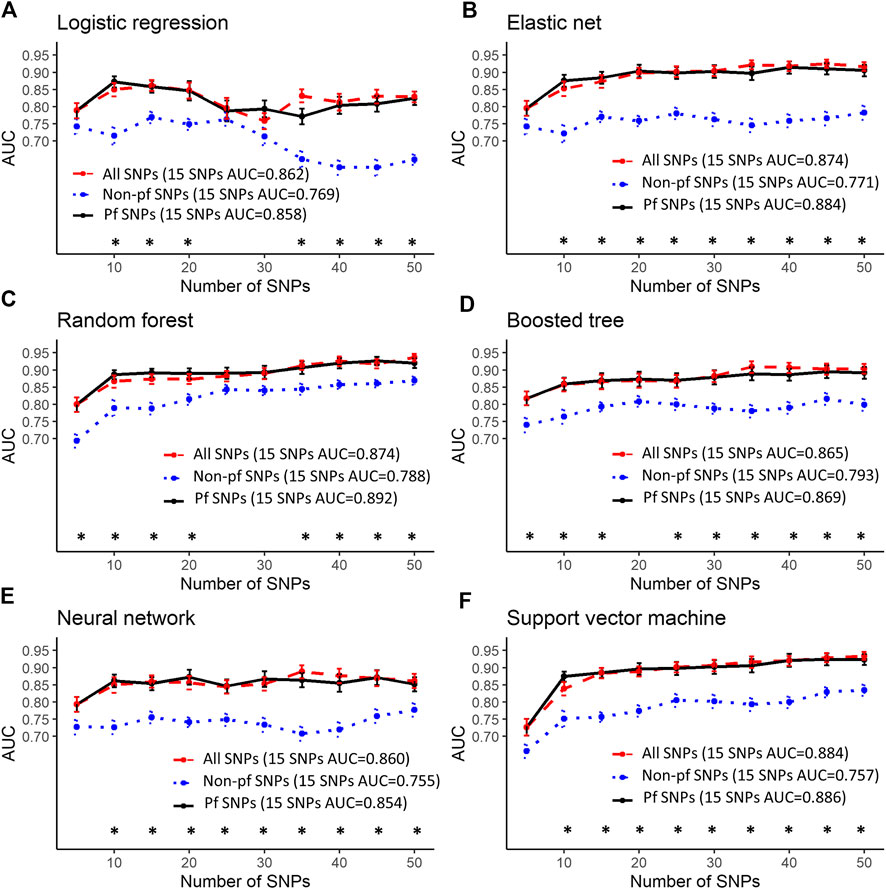
FIGURE 3. Predictive performance using the top 5–50 SNPs in atorvastatin pathway genes. Error bars denote the standard error of the mean. #’s indicate statistical significance when comparing between all SNPs and pfSNPs while *’s indicate statistical significance when comparing between non-pf SNPs and pfSNPs. Statistical significance when comparing between all SNPs and non-pfSNPs is not shown. The colors represent the input set with the higher AUC (red—all SNPs, blue—non-pf SNPs and black—pfSNPs). Bonferroni corrected unpaired t-test p-values (p < 0.05) were used for determining statistical significance.
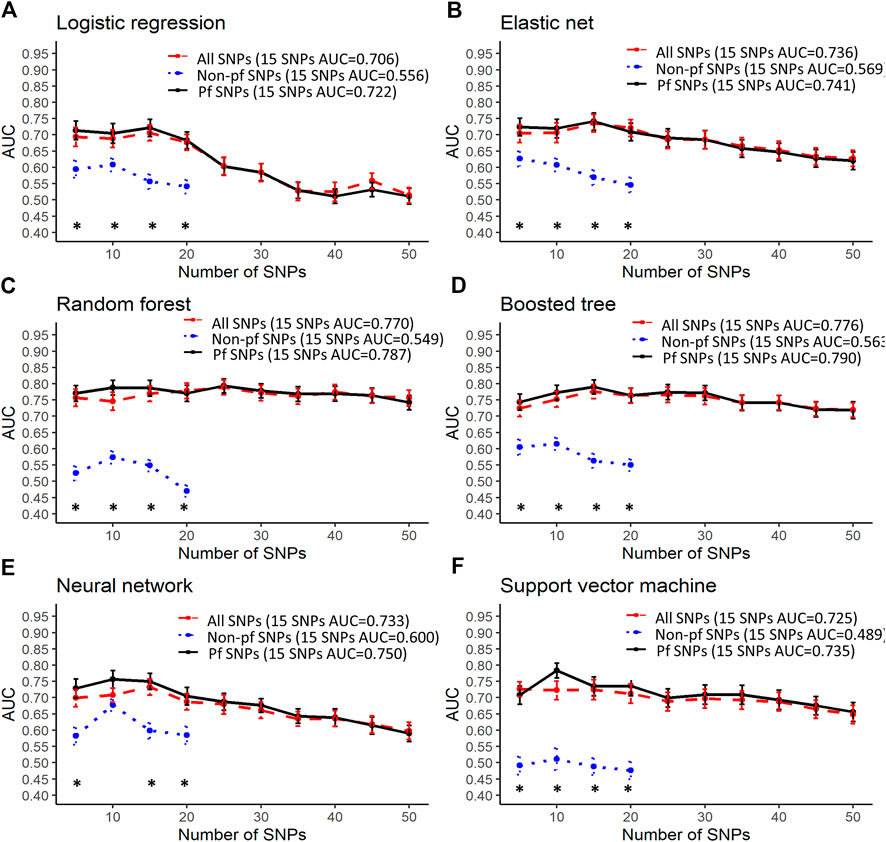
FIGURE 4. Predictive performance using the top 5–50 SNPs in genes found to be associated with myalgia from previous studies. Error bars denote the standard error of the mean. #’s indicate statistical significance when comparing between all SNPs and pfSNPs while *’s indicate statistical significance when comparing between non-pf SNPs and pfSNPs. Statistical significance when comparing between all SNPs and non-pfSNPs is not shown. The colors represent the input set with the higher AUC (red—all SNPs, blue—non-pf SNPs and black—pfSNPs). Bonferroni corrected unpaired t-test p-values (p < 0.05) were used for determining statistical significance. Only 20 non-pf SNPs were found in genes associated with myalgia from the literature.
Robust Performance of Potentially Functional SNPs in Predicting Myalgia
The best performing models described above for a 15 SNP input were obtained when using only pfSNPs, and not when using all SNPs or non-pf SNPs. Furthermore, when comparing pfSNPs to combined SNPs, in four of the machine learning models (logistic regression, elastic net, neural network, support vector machine), pfSNPs outperformed combined SNPs when 15 or more SNPs were used (Figures 2A, B, E, F, # indicates Bonferroni corrected p < 0.05). The predictive performance of pfSNPs was only significantly lower than the combined SNPs when a small number of 10 SNPs was used in the neural network model (Figure 2E, # indicates Bonferroni corrected p < 0.05). However, the AUC achieved using this 10 combined SNPs was 0.959 which was lower than when 15 pfSNPs were used in the same neural network model (AUC: 0.973). In the remaining models (Figures 2C,D) as well as for atorvastatin SNPs (Figure 3) and literature review SNPs (Figure 4), there was no significant difference between using pfSNPs and using combined SNPs. When comparing pfSNPs to non-pf SNPs, pfSNPs outperformed non-pf SNPs in almost all models and input sets (Figures 2–4, * indicates Bonferroni corrected p < 0.05). Additionally, the baseline performance of models only incorporating sex as a predictor (best AUC: 0.58) or using all clinical variables (best AUC: 0.57) (Supplementary Table S3) was significantly poorer than models incorporating both pfSNPs and sex (Figures 2–4).
Discussion
In this study, we hypothesize that rather than individual SNPs, a combination of several potentially functional SNPs (pfSNPs) can better predict myalgia in patients on atorvastatin. Among the demographic and clinical characteristics examined, only sex was significantly (p < 0.05) associated with myalgia, with females having a higher risk. This is concordant with reports from previous studies (Link et al., 2008; Bakar et al., 2018; Tournadre, 2020). Through whole genome association analyses with sex as a covariate, we first demonstrated that among the top 100 SNPs that were most associated with myalgia, the cumulative number of pfSNPs was consistently higher than that of non-pf SNPs (Figure 1C) highlighting the importance of pfSNPs. To identify the combination of pfSNPs/non-pfSNPs that can predict atorvastatin-induced myalgia, six different, but commonly used machine learning models were employed to identify the minimum number of pfSNPs/non-pfSNPs necessary to achieve optimal sensitivity and specificity, determined through the area under ROC curve (AUC), in most, if not all the six models. pfSNPs consistently outperforms non-pfSNPs in predicting myalgia. To our knowledge, this is the first study examining pfSNPs and utilizing machine learning models in the prediction of myalgia.
From the whole genome sequencing results, potentially functional SNPs in RHOBTB1 and SUSD1 were found to be highly associated with atorvastatin-induced myalgia. RHOBTB1 is a member of the Rho GTPase family of signaling proteins with high levels of expression in the stomach, skeletal muscle, placenta, kidney and testis (Ramos et al., 2002). RHOBTB1 is a tumor suppressor gene involved in head and neck cancer and is also involved in protecting against hypertension by improving vasodilator function (Xiao et al., 2017; Mukohda et al., 2019). Knockdown of RHOBTB1 was also found to promote cardiomyocyte proliferation (Xiao et al., 2017). Given its high expression in skeletal muscle, as well as its role in cardiomyocyte proliferation and preventing vascular smooth muscle dysfunction, it is possible that this gene is also involved in preventing myalgia. Not much is known about SUSD1, which encodes for the sushi domain-containing protein 1 precursor. The sushi domain has been found in a number of proteins and is a motif for protein-protein interactions (Wei et al., 2001). SNPs in SUSD1 has been previously associated with venous thromboembolism (Tang et al., 2013) and neurocognitive disabilities (Nilsson et al., 2017).
Most of the machine learning models gave similar AUC values making it difficult to draw definitive conclusions as to which model performs best. However, models including only clinical factors (Supplementary Table S3) were found to have a poorer performance than models incorporating sex and genetic factors (Figures 2–4), demonstrating the higher predictive potential of SNPs compared to clinical factors. When 15 or more SNPs were used, elastic net, neural network and support vector machine models with potentially functional SNPs as inputs had significantly better mean AUCs compared to the same models incorporating non-pf SNPs and total SNPs as seen in Figure 2. Furthermore, the overall best models at the 15 SNP level for each of the three datasets (associated SNPs from this study, SNPs in artovastatin pathway genes and SNPs in genes found from the literature) all utilized pfSNPs as inputs (Figures 2–4). Taken together, these results suggest that SNP functionality is an important factor to consider for improving predictive performance. The importance of SNP functionality was also underscored by the fact that the raw count of pfSNPs was higher than non-pf SNPs in the top 100 variants most associated with myalgia.
Interestingly, we found that SNPs in genes previously reported to be associated with myalgia had the poorest predictive performance of the three groups. Furthermore, predictive AUCs in this group did not increase as the number of SNPs used was increased. Genes in this group include the serotonin receptor genes HTR3B and HTR7 (Ruano et al., 2007), efflux transporter ABCG2, uptake transporter SLCO1B1, cytochrome P450 genes CYP3A4 and CYP2D6, and other candidate genes such as COQ2, ATP2B1, DMPK (Ruano et al., 2011). Our results suggest that only a few SNPs in this group had predictive value, with ABCG2 and HTR3B being the strongest candidate genes. It is also interesting to note that the rs4149056 variant in the SLCO1B1 gene had a relatively high uncorrected p-value of 0.1 in our study. Furthermore, the minor allele frequencies of this variant were higher in controls than in cases for Singaporeans of Chinese, Malay and Indian ethnicities (Supplementary Table S4). These findings suggest that the rs4149056 variant may not have the same effect for milder myalgia, in non-European populations, or due to the type of statin used. These reasons were also alluded to in the review by Turner and Pirmohamed (2019) when discussing the role of SLCO1B1 in statin-related myotoxicity.
There are however some limitations to this study. The relatively small number of samples, with only 30 patients reporting definitive myalgia, limits the discovery p-value to only a suggestive threshold, and could be a possible reason why SLCO1B1 was not detected to be significant. Nevertheless, smaller sample sizes are not unusual in pharmacogenomic association studies due to the large effect sizes of pharmacogenomic variants, unlike complex disease association analyses (Maranville and Cox, 2016). Furthermore, in this study, being unable to achieve genome wide significance for single SNPs is not pertinent as the univariate p-values were merely used for the ranking of SNPs to facilitate the identification of a combination of multiple potentially functional SNPs that best predict atorvastatin-induced myalgia using six different machine learning algorithms. The combination of pfSNPs that were found by most, if not all, of the six different machine learning models to show high sensitivity and specificity in predicting myalgia highlights the robustness of our strategy. A second caveat is that predictive performance of the machine learning models, while achieving good cross validation AUCs, should ideally be validated against an independent test set. Nonetheless, cross validation is a useful indicator of the generalizability of the model and by utilizing the lowest number of SNPs with good AUCs, which we found to be in the 15 SNP range, we hope to minimize overfitting. We aim to validate these SNPs in an independent test set in a future study. The results of this study, while limited by the small sample size, represent a proof of concept of the potential of both machine learning methods and potentially functional polymorphisms in the prediction of drug response.
In conclusion, machine learning models with potentially functional SNPs were found to have good and robust properties for predicting atorvastatin-induced myalgia. However, SNPs in candidate genes previously reported to be associated with myalgia did not show good predictive properties, at least in this Singapore population. Combinations of pfSNPs that were consistently identified by different machine learning models to have high predictive performance have good potential to be clinically useful for predicting atorvastatin-induced myalgia once validated against an independent cohort of patients.
Data Availability Statement
The datasets presented in this study can be found in online repositories. The names of the repository/repositories and accession number(s) can be found below: https://www.ebi.ac.uk/ena, PRJEB40922.
Ethics Statement
The studies involving human participants were reviewed and approved by the National Healthcare Group Domain Specific Review Board (NHG DSRB). The patients/participants provided their written informed consent to participate in this study.
Author Contributions
BO: data processing, statistical analysis, machine learning modeling, data interpretation, and writing of manuscript. RR and YJ: data processing and statistical analysis. AY: machine learning modeling and writing of manuscript. YK and SY: data acquisition and data processing. JHSL: whole genome sequencing and data processing. SC: data interpretation and cirtical review of manuscript. JT and JJL: Patient recruitment, data collection, study plan and critical review of manuscript throughout the editorial process. CL and CD: study plan, data acquisition, data interpretation, critical review of the manuscript throughout the editorial process, and approval of the final manuscript draft submitted for publication.
Funding
This work was supported by grants from the Technology Acceleration Program (TAP) Grant (National University of Singapore) to Caroline Lee and Chester Drum; and block funding Duke-NUS Graduate Medical School to Caroline Lee. The funders had no role in study design, data collection and analysis, decision to publish, or preparation of the manuscript.
Conflict of Interest
The authors declare that the research was conducted in the absence of any commercial or financial relationships that could be construed as a potential conflict of interest.
Acknowledgments
All library preparation and sequencing was done by Novogene, Singapore. We thank Novogene for their kind support.
Supplementary Material
The Supplementary Material for this article can be found online at: https://www.frontiersin.org/articles/10.3389/fphar.2021.605764/full#supplementary-material
References
Alfirevic, A., Neely, D., Armitage, J., Chinoy, H., Cooper, R. G., Laaksonen, R., et al. (2014). Phenotype standardization for statin-induced myotoxicity. Clin. Pharmacol. Ther. 96 (4), 470–476. doi:10.1038/clpt.2014.121
Athreya, A. P., Neavin, D., Carrillo‐Roa, T., Skime, M., Biernacka, J., Frye, M. A., et al. (2019). Pharmacogenomics‐driven prediction of antidepressant treatment outcomes: a machine‐learning approach with multi‐trial replication. Clin. Pharmacol. Ther. 106 (4), 855–865. doi:10.1002/cpt.1482
Bachtiar, M., Jin, Y., Wang, J., Tan, T. W., Chong, S. S., Ban, K. H. K., et al. (2019a). Architecture of population-differentiated polymorphisms in the human genome. PLoS One 14 (10), e0224089. doi:10.1371/journal.pone.0224089
Bachtiar, M., Ooi, B. N. S., Wang, J., Jin, Y., Tan, T. W., Chong, S. S., et al. (2019b). Towards precision medicine: interrogating the human genome to identify drug pathways associated with potentially functional, population-differentiated polymorphisms. Pharmacogenomics J. 19 (6), 516–527. doi:10.1038/s41397-019-0096-y
Bakar, N. S., Neely, D., Avery, P., Brown, C., Daly, A. K., and Kamali, F. (2018). Genetic and clinical factors are associated with statin-related myotoxicity of moderate severity: a case-control study. Clin. Pharmacol. Ther. 104 (1), 178–187. doi:10.1002/cpt.887
Bruckert, E., Hayem, G., Dejager, S., Yau, C., and Bégaud, B. (2005). Mild to moderate muscular symptoms with high-dosage statin therapy in hyperlipidemic patients -the PRIMO study. Cardiovasc. Drugs Ther. 19 (6), 403–414. doi:10.1007/s10557-005-5686-z
Brunham, L. R., Baker, S., Mammen, A., Mancini, G. B. J., and Rosenson, R. S. (2018). Role of genetics in the prediction of statin-associated muscle symptoms and optimization of statin use and adherence. Cardiovasc. Res. 114 (8), 1073–1081. doi:10.1093/cvr/cvy119
Carithers, L. J., Ardlie, K., Barcus, M., Branton, P. A., Britton, A., Buia, S. A., et al. (2015). A novel approach to high-quality postmortem tissue procurement: the GTEx project. Biopreservation and Biobanking 13 (5), 311–319. doi:10.1089/bio.2015.0032
Carr, D. F., O’Meara, H., Jorgensen, A. L., Campbell, J., Hobbs, M., McCann, G., et al. (2013). SLCO1B1 genetic variant associated with statin-induced myopathy: a proof-of-concept study using the clinical practice research datalink. Clin. Pharmacol. Ther. 94 (6), 695–701. doi:10.1038/clpt.2013.161
Chang, C. C., Chow, C. C., Tellier, L. C., Vattikuti, S., Purcell, S. M., and Lee, J. J. (2015). Second-generation PLINK: rising to the challenge of larger and richer datasets. GigaSci 4, 7. doi:10.1186/s13742-015-0047-8
Cholesterol Treatment Trialists, C., Mihaylova, B., Emberson, J., Blackwell, L., Keech, A., Simes, J., et al. (2012). The effects of lowering LDL cholesterol with statin therapy in people at low risk of vascular disease: meta-analysis of individual data from 27 randomised trials. Lancet 380 (9841), 581–590. doi:10.1016/S0140-6736(12)60367-5
Chowdhury, R., Khan, H., Heydon, E., Shroufi, A., Fahimi, S., Moore, C., et al. (2013). Adherence to cardiovascular therapy: a meta-analysis of prevalence and clinical consequences. Eur. Heart J. 34 (38), 2940–2948. doi:10.1093/eurheartj/eht295
Cohen, J. D., Brinton, E. A., Ito, M. K., and Jacobson, T. A. (2012). Understanding Statin Use in America and Gaps in Patient Education (USAGE): an internet-based survey of 10,138 current and former statin users. J. Clin. Lipidol. 6 (3), 208–215. doi:10.1016/j.jacl.2012.03.003
Deng, L., Hoh, B.-P., Lu, D., Saw, W.-Y., Twee-Hee Ong, R., Kasturiratne, A., et al. (2015). Dissecting the genetic structure and admixture of four geographical Malay populations. Sci. Rep. 5, 14375. doi:10.1038/srep14375
Donnelly, L. A., Doney, A. S. F., Tavendale, R., Lang, C. C., Pearson, E. R., Colhoun, H. M., et al. (2011). Common nonsynonymous substitutions in SLCO1B1 predispose to statin intolerance in routinely treated individuals with type 2 diabetes: a go-DARTS study. Clin. Pharmacol. Ther. 89 (2), 210–216. doi:10.1038/clpt.2010.255
Fadista, J., Manning, A. K., Florez, J. C., and Groop, L. (2016). The (in)famous GWAS P-value threshold revisited and updated for low-frequency variants. Eur. J. Hum. Genet. 24 (8), 1202–1205. doi:10.1038/ejhg.2015.269
Floyd, J. S., Bis, J. C., Brody, J. A., Heckbert, S. R., Rice, K., and Psaty, B. M. (2014). GATM locus does not replicate in rhabdomyolysis study. Nature 513 (7518), E1–E3. doi:10.1038/nature13629
Floyd, J. S., Bloch, K. M., Brody, J. A., Maroteau, C., Siddiqui, M. K., Gregory, R., et al. (2019). Pharmacogenomics of statin-related myopathy: meta-analysis of rare variants from whole-exome sequencing. PLoS One 14 (6), e0218115. doi:10.1371/journal.pone.0218115
Frudakis, T. N., Thomas, M. J., Ginjupalli, S. N., Handelin, B., Gabriel, R., and Gomez, H. J. (2007). CYP2D6*4 polymorphism is associated with statin-induced muscle effects. Pharmacogenet Genomics 17 (9), 695–707. doi:10.1097/FPC.0b013e328012d0a9
Huang, C., Clayton, E. A., Matyunina, L. V., McDonald, L. D., Benigno, B. B., Vannberg, F., et al. (2018). Machine learning predicts individual cancer patient responses to therapeutic drugs with high accuracy. Sci. Rep. 8 (1), 16444. doi:10.1038/s41598-018-34753-5
Hubáček, J. A., Dlouhá, D., Adámková, V., Zlatohlavek, L., Viklický, O., Hrubá, P., et al. (2015). SLCO1B1 polymorphism is not associated with risk of statin-induced myalgia/myopathy in a Czech population. Med. Sci. Monit. 21, 1454–1459. doi:10.12659/MSM.893007
Hughes, K., Lun, K. C., Yeo, P. P., Thai, A. C., Sothy, S. P., Wang, K. W., et al. (1990). Cardiovascular diseases in Chinese, Malays, and Indians in Singapore. I. Differences in mortality. J. Epidemiol. Community Health 44 (1), 24–28. doi:10.1136/jech.44.1.24
Jansen, R., Hottenga, J.-J., Nivard, M. G., Abdellaoui, A., Laport, B., de Geus, E. J., et al. (2017). Conditional eQTL analysis reveals allelic heterogeneity of gene expression. Hum. Mol. Genet. 26 (8), 1444–1451. doi:10.1093/hmg/ddx043
Kimchi-Sarfaty, C., Oh, J. M., Kim, I.-W., Sauna, Z. E., Calcagno, A. M., Ambudkar, S. V., et al. (2007). A “silent” polymorphism in the MDR1 gene changes substrate specificity. Science 315 (5811), 525–528. doi:10.1126/science.1135308
Kuhn, M. (2008). Building predictive models inRUsing thecaretPackage. J. Stat. Soft. 28 (5), 26. doi:10.18637/jss.v028.i05
Li, H., and Durbin, R. (2009). Fast and accurate short read alignment with Burrows-Wheeler transform. Bioinformatics 25 (14), 1754–1760. doi:10.1093/bioinformatics/btp324
Link, E., Link, E., Parish, S., Armitage, J., Bowman, L., Heath, S., et al. (2008). SLCO1B1 variants and statin-induced myopathy–a genomewide study. N. Engl. J. Med. 359 (8), 789–799. doi:10.1056/NEJMoa0801936
Liu, R., Li, X., Zhang, W., and Zhou, H.-H. (2015). Comparison of nine statistical model based warfarin pharmacogenetic dosing algorithms using the racially diverse international warfarin pharmacogenetic consortium cohort database. PLoS One 10 (8), e0135784. doi:10.1371/journal.pone.0135784
Luzum, J. A., Kitzmiller, J. P., Isackson, P. J., Ma, C., Medina, M. W., Dauki, A. M., et al. (2015). GATM polymorphism associated with the risk for statin-induced myopathy does not replicate in case-control analysis of 715 dyslipidemic individuals. Cel Metab. 21 (4), 622–627. doi:10.1016/j.cmet.2015.03.003
Mangravite, L. M., Engelhardt, B. E., Medina, M. W., Smith, J. D., Brown, C. D., Chasman, D. I., et al. (2013). A statin-dependent QTL for GATM expression is associated with statin-induced myopathy. Nature 502 (7471), 377–380. doi:10.1038/nature12508
Maranville, J. C., and Cox, N. J. (2016). Pharmacogenomic variants have larger effect sizes than genetic variants associated with other dichotomous complex traits. Pharmacogenomics J. 16 (4), 388–392. doi:10.1038/tpj.2015.47
McGinnis, B., Olson, K. L., Magid, D., Bayliss, E., Korner, E. J., Brand, D. W., et al. (2007). Factors related to adherence to statin therapy. Ann. Pharmacother. 41 (11), 1805–1811. doi:10.1345/aph.1K209
McKenna, A., Hanna, M., Banks, E., Sivachenko, A., Cibulskis, K., Kernytsky, A., et al. (2010). The Genome Analysis Toolkit: a MapReduce framework for analyzing next-generation DNA sequencing data. Genome Res. 20 (9), 1297–1303. doi:10.1101/gr.107524.110
Mukohda, M., Fang, S., Wu, J., Agbor, L. N., Nair, A. R., Ibeawuchi, S.-R. C., et al. (2019). RhoBTB1 protects against hypertension and arterial stiffness by restraining phosphodiesterase 5 activity. J. Clin. Invest. 129 (6), 2318–2332. doi:10.1172/JCI123462
Nilsson, D., Pettersson, M., Gustavsson, P., Förster, A., Hofmeister, W., Wincent, J., et al. (2017). Whole-genome sequencing of cytogenetically balanced chromosome translocations identifies potentially pathological gene disruptions and highlights the importance of microhomology in the mechanism of formation. Hum. Mutat. 38 (2), 180–192. doi:10.1002/humu.23146
Ounpuu, S., and Yusuf, S. (2003). Singapore and coronary heart disease: a population laboratory to explore ethnic variations in the epidemiologic transition. Eur. Heart J. 24 (2), 127–129. doi:10.1016/s0195-668x(02)00611-5
Pasanen, M. K., Neuvonen, M., Neuvonen, P. J., and Niemi, M. (2006). SLCO1B1 polymorphism markedly affects the pharmacokinetics of simvastatin acid. Pharmacogenet Genomics 16 (12), 873–879. doi:10.1097/01.fpc.0000230416.82349.90
Ramos, S., Khademi, F., Somesh, B. P., and Rivero, F. (2002). Genomic organization and expression profile of the small GTPases of the RhoBTB family in human and mouse. Gene 298 (2), 147–157. doi:10.1016/s0378-1119(02)00980-0
Ruaño, G., Thompson, P. D., Windemuth, A., Seip, R. L., Dande, A., Sorokin, A., et al. (2007). Physiogenomic association of statin-related myalgia to serotonin receptors. Muscle Nerve 36 (3), 329–335. doi:10.1002/mus.20871
Ruaño, G., Windemuth, A., Wu, A. H. B., Kane, J. P., Malloy, M. J., Pullinger, C. R., et al. (2011). Mechanisms of statin-induced myalgia assessed by physiogenomic associations. Atherosclerosis 218 (2), 451–456. doi:10.1016/j.atherosclerosis.2011.07.007
Sai, K., Kajinami, K., Akao, H., Iwadare, M., Sato-Ishida, R., Kawai, Y., et al. (2016). A possible role for HLA-DRB1*04:06 in statin-related myopathy in Japanese patients. Drug Metab. Pharmacokinet. 31 (6), 467–470. doi:10.1016/j.dmpk.2016.09.002
Saxon, D. R., and Eckel, R. H. (2016). Statin intolerance: a literature review and management strategies. Prog. Cardiovasc. Dis. 59 (2), 153–164. doi:10.1016/j.pcad.2016.07.009
SEARCH Collaborative Group Armitage, J., Bowman, L., Wallendszus, K., Bulbulia, R., et al. (2010). Intensive lowering of LDL cholesterol with 80 mg versus 20 mg simvastatin daily in 12,064 survivors of myocardial infarction: a double-blind randomised trial. Lancet 376 (9753), 1658–1669. doi:10.1016/S0140-6736(10)60310-8
Shek, A. B., Kurbanov, R. D., Abdullaeva, G. J., Nagay, A. V., Hoshimov, S. U., Nizamov, U. I., et al. (2017). Simvastatin intolerance genetic determinants: some features in ethnic Uzbek patients with coronary artery disease. amsad 2, 68–75. doi:10.5114/amsad.2017.70597
Siddiqui, K. M., Maroteau, C., Veluchamy, A., Tornio, A., Tavendale, R., Carr, F., et al. (2017). A common missense variant of LILRB5 is associated with statin intolerance and myalgia. Eur. Heart J. 38 (48), 3569–3575. doi:10.1093/eurheartj/ehx467
Silverman, M. G., Ference, B. A., Im, K., Wiviott, S. D., Giugliano, R. P., Grundy, S. M., et al. (2016). Association between lowering LDL-C and cardiovascular risk reduction among different therapeutic interventions. JAMA 316 (12), 1289–1297. doi:10.1001/jama.2016.13985
Stroes, E. S., Thompson, P. D., Corsini, A., Vladutiu, G. D., Raal, F. J., Ray, K. K., et al. (2015). Statin-associated muscle symptoms: impact on statin therapy-European atherosclerosis society consensus panel statement on assessment, aetiology and management. Eur. Heart J. 36 (17), 1012–1022. doi:10.1093/eurheartj/ehv043
Tang, W., Teichert, M., Chasman, D. I., Heit, J. A., Morange, P.-E., Li, G., et al. (2013). A genome-wide association study for venous thromboembolism: the extended cohorts for heart and aging research in genomic epidemiology (CHARGE) consortium. Genet. Epidemiol. 37 (5), 512–521. doi:10.1002/gepi.21731
Tournadre, A. (2020). Statins, myalgia, and rhabdomyolysis. Jt. Bone Spine 87 (1), 37–42. doi:10.1016/j.jbspin.2019.01.018
Turner, R. M., and Pirmohamed, M. (2019). Statin-related myotoxicity: a comprehensive review of pharmacokinetic, pharmacogenomic and muscle components. Jcm 9 (1), 22. doi:10.3390/jcm9010022
Voora, D., Shah, S. H., Spasojevic, I., Ali, S., Reed, C. R., Salisbury, B. A., et al. (2009). The SLCO1B1*5Genetic variant is associated with statin-induced side effects. J. Am. Coll. Cardiol. 54 (17), 1609–1616. doi:10.1016/j.jacc.2009.04.053
Võsa, U., Claringbould, A., Westra, H.-J., Bonder, M. J., Deelen, P., Zeng, B., et al. (2018). Unraveling the polygenic architecture of complex traits using blood eQTL metaanalysis. bioRxiv, 447367. doi:10.1101/447367
Wang, J., Ronaghi, M., Chong, S. S., and Lee, C. G. L. (2011). pfSNP: an integrated potentially functional SNP resource that facilitates hypotheses generation through knowledge syntheses. Hum. Mutat. 32 (1), 19–24. doi:10.1002/humu.21331
Wang, K., Li, M., and Hakonarson, H. (2010). ANNOVAR: functional annotation of genetic variants from high-throughput sequencing data. Nucleic Acids Res. 38 (16), e164. doi:10.1093/nar/gkq603
Ward, N. C., Watts, G. F., and Eckel, R. H. (2019). Statin toxicity. Circ. Res. 124 (2), 328–350. doi:10.1161/CIRCRESAHA.118.312782
Wei, M. Y., Ito, M. K., Cohen, J. D., Brinton, E. A., and Jacobson, T. A. (2013). Predictors of statin adherence, switching, and discontinuation in the USAGE survey: understanding the use of statins in America and gaps in patient education. J. Clin. Lipidol. 7 (5), 472–483. doi:10.1016/j.jacl.2013.03.001
Wei, X.-q., Orchardson, M., Gracie, J. A., Leung, B. P., Gao, B.-m., Guan, H., et al. (2001). The sushi domain of soluble IL-15 receptor α is essential for binding IL-15 and inhibiting inflammatory and allogenic responses in vitro and in vivo. J. Immunol. 167 (1), 277–282. doi:10.4049/jimmunol.167.1.277
Wilke, R. A., Ramsey, L. B., Johnson, S. G., Maxwell, W. D., McLeod, H. L., Voora, D., et al. (2012). The clinical pharmacogenomics implementation consortium: CPIC guideline for SLCO1B1 and simvastatin-induced myopathy. Clin. Pharmacol. Ther. 92 (1), 112–117. doi:10.1038/clpt.2012.57
World Health Organization – Cardiovascular Disease (2020). https://www.who.int/health-topics/cardiovascular-diseases/#tab=tab_1 (Accessed February 13, 2020).
Xiao, J., Liu, H., Cretoiu, D., Toader, D. O., Suciu, N., Shi, J., et al. (2017). miR-31a-5p promotes postnatal cardiomyocyte proliferation by targeting RhoBTB1. Exp. Mol. Med. 49 (10), e386. doi:10.1038/emm.2017.150
Zhang, L., Lv, H., Zhang, Q., Wang, D., Kang, X., Zhang, G., et al. (2019). Association of SLCO1B1 and ABCB1 genetic variants with atorvastatin-induced myopathy in patients with acute ischemic stroke. Cpd 25 (14), 1663–1670. doi:10.2174/1381612825666190705204614
Keywords: statin, myalgia, whole genome sequencing, machine learning, pharmacogenomics
Citation: Ooi BNS, Raechell , Ying AF, Koh YZ, Jin Y, Yee SWL, Lee JHS, Chong SS, Tan JWC, Liu J, Lee CG and Drum CL (2021) Robust Performance of Potentially Functional SNPs in Machine Learning Models for the Prediction of Atorvastatin-Induced Myalgia. Front. Pharmacol. 12:605764. doi: 10.3389/fphar.2021.605764
Received: 13 September 2020; Accepted: 08 March 2021;
Published: 22 April 2021.
Edited by:
Jasmine Luzum, University of Michigan, United StatesReviewed by:
Tao Jiang, University of Illinois at Urbana-Champaign, United StatesXiaohui Li, Lundquist Institute for Biomedical Innovation, United States
Moneeza Kalhan Siddiqui, University of Dundee, United Kingdom
Copyright © 2021 Ooi, Raechell, Ying, Koh, Jin, Yee, Lee, Chong, Tan, Liu, Lee and Drum. This is an open-access article distributed under the terms of the Creative Commons Attribution License (CC BY). The use, distribution or reproduction in other forums is permitted, provided the original author(s) and the copyright owner(s) are credited and that the original publication in this journal is cited, in accordance with accepted academic practice. No use, distribution or reproduction is permitted which does not comply with these terms.
*Correspondence: Caroline G. Lee, bchleec@nus.edu.sg; Chester L. Drum, mdccld@nus.edu.sg