- 1Department of Radiology, Nanfang Hospital, Southern Medical University, Guangzhou, China
- 2Institute of Integrated Bioinformedicine and Translational Science, Hong Kong Baptist University, Hong Kong SAR, China
- 3Neurosurgery Center, Guangdong Provincial Key Laboratory on Brain Function Repair and Regeneration, Department of Cerebrovascular Surgery, Engineering Technology Research Center of Education Ministry of China on Diagnosis and Treatment of Cerebrovascular Disease, Zhujiang Hospital, Southern Medical University, Guangzhou, China
- 4Department of Biochemistry and Molecular Biology, School of Basic Medical Sciences, Southern Medical University, Guangzhou, China
- 5Guangdong Key Laboratory of Biochip Technology, Southern Medical University, Guangzhou, China
- 6Modern Research Center for Traditional Chinese Medicine, Shanxi University, Taiyuan, China
Breast cancer (BC) is one of the most common malignant tumors among women worldwide and can be treated using various methods; however, side effects of these treatments cannot be ignored. Increasing evidence indicates that compound kushen injection (CKI) can be used to treat BC. However, traditional Chinese medicine (TCM) is characterized by “multi-components” and “multi-targets”, which make it challenging to clarify the potential therapeutic mechanisms of CKI on BC. Herein, we designed a novel system pharmacology strategy using differentially expressed gene analysis, pharmacokinetics synthesis screening, target identification, network analysis, and docking validation to construct the synergy contribution degree (SCD) and therapeutic response index (TRI) model to capture the critical components responding to synergistic mechanisms of CKI in BC. Through our designed mathematical models, we defined 24 components as a high contribution group of synergistic components (HCGSC) from 113 potentially active components of CKI based on ADME parameters. Pathway enrichment analysis of HCGSC targets indicated that Rhizoma Heterosmilacis and Radix Sophorae Flavescentis could synergistically target the PI3K-Akt signaling pathway and the cAMP signaling pathway to treat BC. Additionally, TRI analysis showed that the average affinity of HCGSC and targets involved in the key pathways reached -6.47 kcal/mmol, while in vitro experiments proved that two of the three high TRI-scored components in the HCGSC showed significant inhibitory effects on breast cancer cell proliferation and migration. These results demonstrate the accuracy and reliability of the proposed strategy.
Introduction
Breast cancer (BC) is the most common cancer in women worldwide and is responsible for the second highest death rate among female patients with cancer (Siegel et al., 2020). Many options are available to treat breast cancer, such as surgical treatment (Zheng et al., 2020), radiation therapy (Balaji et al., 2016), neoadjuvant endocrine therapy (Yao et al., 2019), neoadjuvant chemotherapy (Vaidya et al., 2018), and anti-HER2 therapy (Yao and Fu, 2018). However, side effects of these treatments are often observed. For example, radiation therapy can cause radiation-induced fibrosis (Straub et al., 2015) and radiodermatitis (Singh et al., 2016). Fatigue, pain, and systemic side effects can occur as a result of surgical treatment (Enien et al., 2018). Adjuvant endocrine therapy can cause vasomotor symptoms and musculoskeletal and vulvovaginal symptoms (Condorelli and Vaz-Luis, 2018). These side effects mainly influence the organs and blood system, which aggravates the psychological burden on the patients. Side effect symptoms include loss of appetite, vomiting, diarrhea, nausea, and ulcers (Meng et al., 2017; Recht, 2017; Cochran et al., 2019). In recent years, traditional Chinese medicine (TCM) has become increasingly popular in the treatment of BC. Ganoderma lucidum from Ganoderma suppresses the proliferation and migration of breast cancer by inhibiting Wnt/β-catenin signaling (Zhang, 2017), while puerarin from Radix puerariae inhibits cell migration, invasion, and adhesion of LPS-induced BC by blocking NF-κB and Erk pathways (Liu et al., 2017). In addition to these herbal components, many formulas and proprietary Chinese medicines are widely used in the treatment of BC, such as compound kushen injection (CKI) (Liu et al., 2020), Shugan Jianpi decoction (Jingyuan et al., 2021), and Fangjihuangqi decoction (Guo et al., 2020). Based on these formulas and proprietary Chinese medicines, CKI is a commonly used antitumor treatment in clinical practice.
CKI is a TCM formulae extract from kushen (Radix Sophorae flavescentis) and baituling (Rhizoma Heterosmilacis) at a ratio of 7:3, in which exist hundreds of components including alkaloids and flavonoids (Cui et al., 2020). As approved by the China Food and Drug Administration, CKI can be employed for cancer treatment (Guo et al., 2015) and has several pharmacological functions, including anticancer properties, hemostasis, and immunity enhancement (Wang et al., 2015). CKI is widely being used in the treatment of cancers, such as breast cancer (Xu et al., 2011a; Qu et al., 2016; Cui et al., 2019; Nourmohammadi et al., 2019; Cui et al., 2020), acute myeloid leukemia (Jin et al., 2018), and hepatocellular carcinoma (Gao et al., 2018; Yang et al., 2021). Additionally, the function of CKI in treating breast cancer was also proved in in vitro and in vivo experiments. CKI could reduce the tumor formation rates and tumor volume by the downregulated Wnt/b-catenin pathway in the MCF-7 SP xenograft model (Xu et al., 2011a). Besides, CKI could impair the migration and invasiveness of MDA-MB-231 cell lines (Nourmohammadi et al., 2019) and influence the cell cycle of MDA-MB-231 cell lines in promoting cell death by increasing the proportion of cells in the G1 phase and decreasing the cells in the S and G2/M phase (Cui et al., 2019; Cui et al., 2020), while it could inhibit proliferation and induce cell apoptosis of MCF-7 cell lines (Qu et al., 2016). These in vitro and in vivo experiments had proved that CKI has anticancer pharmacological function, especially in breast cancer. In CKI, matrine, oxymatrine, and sophocarpine are the main components of Radix Sophorae flavescentis, which display antitumor, anti-inflammatory, and antiviral properties, as well as cardiovascular protective abilities (Wang et al., 2015). Rhizoma Heterosmilacis may play a role in promoting oxidative stress–induced apoptosis (Liu et al., 2017), reducing oxidative stress (Hong et al., 2014), deoxidation, and dampness relief (Liang et al., 2019). Increasing evidence has shown that CKI can be used to halt cancer migration (Nourmohammadi et al., 2019), reduce anticancer drug resistance (Hy et al., 2014), increase cancer cell apoptosis (Qu et al., 2016), suppress the cancer cell cycle (Cui et al., 2019), and inhibit cancer progression (Wang et al., 2019). Matrine upregulates Bax and downregulates Bcl-2 to inhibit proliferation and increase apoptosis of breast cancer cells (Li et al., 2015) and further downregulates the canonical pathway to suppress human breast cancer stem-like cells (Xu et al., 2011b). Oxymatrine exerts its effects by blocking the cell cycle, initiating apoptosis (Binggang et al., 2002) and suppressing the epithelial–mesenchymal transition (Jiaqin et al., 2018). These sporadic reports suggest that different components of CKI play a role in the treatment of BC, but there is still a lack of systematic and overall research on the synergistic mechanism of different components of Rhizoma Heterosmilacis and Radix Sophorae Flavescentis in CKI.
Since TCM is characterized by “multi-components” and “multi-targets”, it is difficult to reveal the associated mechanisms between herbs, components, genes, and disease through traditional experimental methods. As an effective tool to elucidate the synergistic and potential mechanisms of the networks between component–target and target–disease, systemic pharmacology provides a new perspective on the therapeutic mechanisms of TCM in the treatment of BC at the systematic level.
To explore the therapeutic mechanism of CKI in treating BC, a novel system pharmacology strategy (Figure 1), which integrates differentially expressed gene analysis (DEGs), pharmacokinetics synthesis screening, target identification, synergy contribution degree (SCD), network analysis, and therapeutic response index (TRI) calculation, was developed. The Cancer Genome Atlas (TCGA) database was used to explore the DEGs in BC. Subsequently, previously proposed absorption, distribution, metabolism, and excretion (ADME) screening models were employed to select potential active components; online tools were used to predict the targets of these potential active components, and a component–target (C-T) network was used for further network analysis. Network analysis of potential components combined with SCD was used to simulate the treatment effects of each component of CKI in BC and to explore a high contribution group of synergistic components (HCGSC). The virtual docking-aided TRI model was designed to determine whether highly reliable components may have a potential synergistic mechanism. Thereafter, GO enrichment and KEGG pathway enrichment analysis of BC, CKI, and highly reliable components in HCGSC were discussed to decode the potential synergistic mechanism analysis of CKI in the treatment of BC based on HCGSC. Ultimately, experimental validation was used to confirm the effect of high TRI-scored components in the HCGSC and evaluate the reliability of our model. We hope that these results will provide a strategy to reveal the therapeutic mechanism of TCM at the molecular level.
Materials and Methods
Chemical Components Collected From Database
All CKI components were collected from the Traditional Chinese Medicine Systems Pharmacology (TCMSP) database (http://www.tcmspw.com/tcmsp.php). TCMSP includes information from DrugBank, HIT, TTD, and PharmGKB. The pharmacokinetic properties of TCMSP include molecular weight (MW), AlogP, number of acceptor atoms for H-bonds (nHacc), number of donor atoms for H-bonds (nHdon), Caco-2 permeability (Caco-2), oral bioavailability (OB), drug-likeness (DL), blood–brain barrier (BBB), FASA-, and half-life (HL).
ADME Screening
In the process of modern drug development, many drug candidates fail during development because of inadequate ADME properties (Rojas-Aguirre and Medina-Franco, 2014). Therefore, evaluating the ADME of drug components in the early stages has become an essential process. Components with better pharmacokinetic properties can be obtained by ADME screening, and the potential drug–drug interactions can be minimized (Wang et al., 2018). Two ADME-related models were employed in the present study, namely Caco-2 permeability and drug-likeness, to screen the potential active components of CKI (Supplementary Figure S1).
As a model system for intestinal epithelial permeability, the human colon carcinoma cell line (Caco-2) (Ij et al., 1989) is currently considered the gold standard (Jacobsen et al., 2020). The transport rates of components (nm/s) in Caco-2 monolayers represent intestinal epithelial permeability in TCMSP (Ru et al., 2014). Components with Caco-2 < −0.4 are not permeable; thus, Caco-2 > −0.4 were selected as candidate components.
Drug-likeness (DL) may be defined as a complex balance of various molecular properties and structural features that determine whether a particular molecule is similar to known drugs. The drug similarity index of the new compound was calculated using the Tanimoto coefficient, which is defined as
In this equation, A represents the molecular descriptor of herbal components, and B is the average molecular property of all components in DrugBank (Tao et al., 2013). Based on the data from TCMSP, the average value of Radix Sophorae Flavescentis was 0.40, while that of Rhizoma Heterosmilacis was 0.25. Combined with other literature search criteria, we defined DL ≥ 0.18, as the screening criterion of DL.
Target Identification
Three commonly used online tools were employed to identify the targets of active components in CKI, namely, similarity ensemble approach (SEA) (Keiser et al., 2007), HitPick (Liu et al., 2013), and SwissTargetPrediction (Gfeller et al., 2014). Open Babel 3.0.0 (O Boyle et al., 2011) was used to convert the SDF format of all CKI potential active components into the SMILES format. Potential active components in the SMILES format were imported to the SEA, HitPick, and SwissTargetPrediction to predict targets of potential active components. The homologous genes of other species provided by online tools were included in the discussion.
Network Construction and Analysis
The C-T network was used as a frame to uncover the relationship between active components and targets. Cytoscape 3.8.0 (Shannon et al., 2003), an open-source software platform, was employed to visualize the networks.
GO Enrichment and KEGG Pathway Enrichment Analysis
To analyze the main function of targets, clusterProfiler (Yu et al., 2012) in the Bioconductor package (https://bioconductor.org/) based on the R language was used for GO-BP enrichment analysis and KEGG pathway analysis. FDR-adjusted p values were set at 0.05, as the cut-off criterion.
Synergy Contribution Degree Calculation
The SCD represents the contribution of a potential active component in the C-T network and its effectiveness in the treatment of BC. To evaluate the effect of CKI on the treatment of BC, we built a mathematical model to calculate the SCD of each active component in CKI:
In this equation, i is the number of components, and j is the number of targets. C represents the betweenness centrality of each component. T represents the betweenness centrality count of all targets of each component. Ce represents the edge count of each component. Te represents the edge count of all targets of each component. CB represents the betweenness centrality of each component only in Rhizoma Heterosmilacis, and CK represents the betweenness centrality of each component only in Radix Sophorae Flavescentis. If a component consists only of Radix Sophorae Flavescentis or Rhizoma Heterosmilacis,
Breast Cancer Differentially Expressed Genes Analysis
The TCGA database (https://portal.gdc.cancer.gov/) was used to explore the DEGs in BC. We used TCGAbiolinks (Colaprico et al., 2016) in the Bioconductor packages to access the HTSeq-Counts number of normal and BC samples from the TCGA-BRCA project of the TCGA program. DESeq (Anders and Huber, 2010) and limma (Ritchie et al., 2015) in the Bioconductor package were used to analyze the DEGs in BC. We set |log2FoldChange|≥2 and FDR adjusted the p value < 0.05, as the cut-off criterion. The results are presented and plotted using the gplots (Warnes et al., 2020) package.
Molecular Docking Analysis of HCGSC in the Treatment of BC
The components of HCGSC were collected from the ZINC (Sterling and Irwin, 2015) and PubChem (https://pubchem.ncbi.nlm.nih.gov) databases in the MOL2 format. Human proteins were collected from the Protein Data Bank (PDB) (http://www.rcsb.org). AutoDock Tools (ADT) (Sanner, 1999) was used to pretreat the components and proteins. The pocket of the protein was automatically extracted from the space of the protein. AutoDock Vina (Trott and Olson, 2009) was used for docking. The seed of docking was 10,000, the energy range was 4, and the exhaustiveness was 4. The affinity (kcal/mol) index of each component–protein pair was used to estimate the docking results. The results were obtained using PyMOL (Schr Odinger, 2015).
Therapeutic Response Index Model Calculation
We developed a mathematical model to calculate the therapeutic response index (TRI) of each component in a highly reliable docking relationship (HRDR) between HCGSC and its targets.
In the above model, i is the number of components, j is the number of topology parameters, n is the collection of components, and m is the collection of components and topology parameters. CDI represents the docking index of HRDR. GI represents the number of genes targeted by each component. AI represents the average affinity of all component–protein binding relationships of each component. NI represents the C-T network topology parameters of HCGSC and their targets, including degree, betweenness centrality, closeness centrality, average shortest path length, eccentricity, radiality, neighborhood connectivity, and topological coefficient. SCD is the calculation result for each component from method 2.6. TRI represents the mechanism of CKI in the treatment of BC, which is the sum of normalized CDI and normalized SCD. NORM is a normalized equation.
Materials
High-glucose Dulbecco’s modified Eagle medium (DMEM; 4.5 g/L), fetal bovine serum (FBS), 0.25% trypsin, and 3-(4,5-dimethylthiazol-2-yl)-2,5-diphenyltetrazolium bromide (MTT) were purchased from Shanghai Sangon Biotechnology Co., Ltd. (Shanghai, China). The transwell invasion chamber was purchased from Corning costar company (Andover, MA, United States). Matrigel was purchased from BD (Biosciences, Bedford, MA, United States). Luteolin and trifolirhizin (≥98% purity by HPLC) were obtained from Nanjing Jingzu Biotech Co., Ltd. (Nanjing, China).
Cell Culture
The human breast cancer cell line MCF-7 was purchased from Procell Life Science & Technology Co., Ltd. Cells were cultured in an incubator at 37°C in DMEM containing 10% FBS. When the cells reached 80% confluency, they were exposed to different concentrations of luteolin and trifolirhizin (1, 2, and 4 mg/ml) for 24 h.
Cell Viability Assay
MCF-7 cells (1 × 104 cells/well) were seeded in 96-well plates and treated with 0, 5, 10, 20, 40, 80, and 100 μM luteolin and trifolirhizin for 24 h. MTT was added to a 96-well plate for 4 h, and the culture supernatant was removed. Finally, DMSO was used to dissolve the purple crystals. Absorbance at 570 nm was measured using a plate reader.
Transwell Assay
Referring to our previous method (Gao et al., 2018), luteolin (40 and 80 μM) and trifolirhizin (20 and 40 μM) were added to the lower compartments. The migrating cells were observed under a microscope.
Statistical Analysis
The R package ggpubr (Kassambara, 2020) was used to compare the molecular properties of all components in Rhizoma Heterosmilacis and Radix Sophorae Flavescentis. Data were analyzed using the Student’s t-test. Differences were considered statistically significant at p < 0.05.
Results
Based on the systematic pharmacological model, the mechanism of CKI in BC treatment was clarified and validated. First, DEGs that could be used as potential pathogenic genes were determined based on the data from the TCGA-BRCA project. Second, the CKI components were collected from the TCMSP database, and the ADME method was used to screen active components in CKI. Third, the active components and their targets, which were predicted using three online tools, were used to construct the C-T network. Fourth, a network analysis of potential components combined with SCD was used to explore HCGSC. Thereafter, GO enrichment and KEGG pathway enrichment analysis of BC, CKI, and highly reliable components in HCGSC were discussed to decode the potential synergistic mechanism of CKI in the treatment of BC. Subsequently, the virtual docking-aided TRI model was designed to determine whether highly reliable components may have a synergistic mechanism. Ultimately, experimental validation was used to confirm the effect of high TRI-scored components in HCGSC and evaluate the reliability of our model.
Differentially Expressed Genes Analysis of BC
To further analyze the mechanism of CKI in the treatment of BC, 113 normal breast samples and 1,102 breast cancer samples were extracted from the TCGA-BRCA project. A total of 979 DEGs were upregulated, and 990 were downregulated in cancer (Supplementary Table S2). The DEGs were used to determine the gene expression patterns of normal and tumor patients (Supplementary Figure S2). The results showed that the DEG pattern distinguished between diseased and normal states. From the expression pattern, we identified that the top 10 upregulated genes were FTHL17, CSAG1, MUC2, COX7B2, CGA, CSAG4, CST4, MAGEA12, MAGEA1, and ACTL8. Among these genes, MUC2 influences proliferation, apoptosis, and metastasis of breast cancer cells (Astashchanka et al., 2019), while the upregulation of MAGEA in patients revealed a higher risk of recurrence (Otte et al., 2001). The top 10 downregulated genes were LEP, GLYAT, AC087482.1, APOB, TRHDE-AS1, AQP7P2, FP325317.1, PLIN1, CA4, and AL845331.2. LEP which inhibit apoptosis of breast cancer cells by coding leptin, while PLIN1 inhibits invasion, migration, and proliferation of cells (Zhou et al., 2016; Crean-Tate and Reizes, 2018). The literature reports proved that these genes are related to the development of BC, which indicates that the DEGs were more likely to be pathogenic genes.
Component Comparisons in Rhizoma Heterosmilacis and Radix Sophorae Flavescentis
From the TCMSP database, 187 compounds were retrieved from Rhizoma Heterosmilacis (74) and Radix Sophorae Flavescentis (113). Detailed information on the components of Rhizoma Heterosmilacis and Radix Sophorae Flavescentis is provided in Supplementary Table S3. To further describe the differences between Rhizoma Heterosmilacis and Radix Sophorae Flavescentis, we compared nine properties of the two components, including drug likeness (DL), Caco-2, molecular weight (MW), AlogP, TPSA, FASA-, RBN, nHDon, and nHAcc, and drew boxplots using R packages ggplot2 (Wickham, 2016), ggpubr (Kassambara, 2020), and ggsci (Xiao, 2018). The results (Figure 2) showed most of chemical composition and properties between Rhizoma Heterosmilacis and Radix Sophorae Flavescentis had no statistical difference except for DL (p = 0.00013) and RBN (p = 0.015). These results indicate that Rhizoma Heterosmilacis and Radix Sophorae Flavescentis have similar chemical properties.
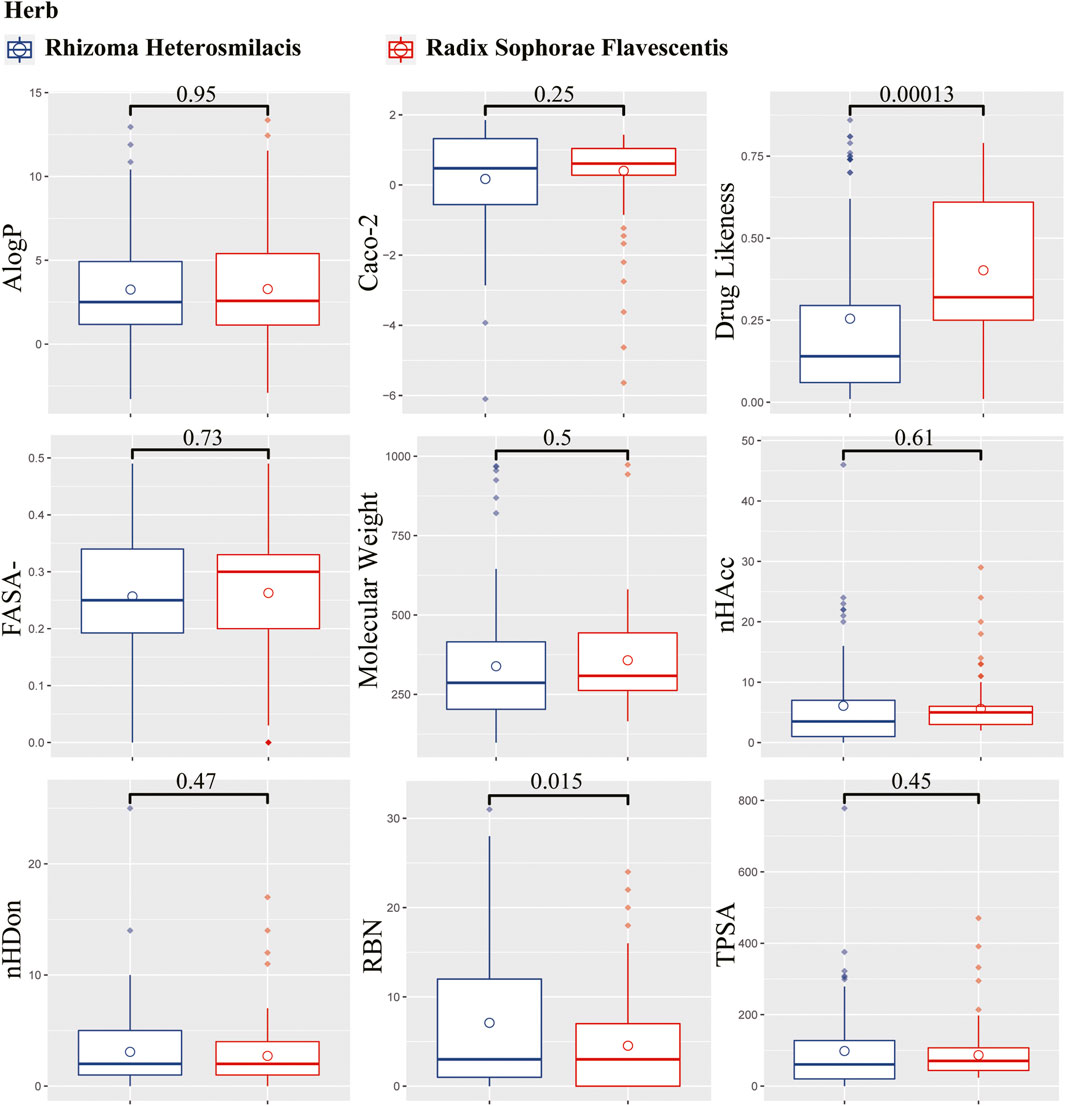
FIGURE 2. Boxplot of nine properties of the two components. The box in blue represents the value distribution of Radix Sophorae Flavescentis, and the box in red represents the value distribution of Rhizoma Heterosmilacis.
Potential Active Components in Rhizoma Heterosmilacis and Radix Sophorae Flavescentis
Traditional Chinese medicine uses a combination of herbs, each of which usually contains hundreds of components, and only a few of these components possess satisfactory pharmacodynamic and pharmacokinetic properties. In this study, two ADME-related properties, DL and Caco-2, were used to screen for active components. Through ADME screening, 111 active components (Radix Sophorae Flavescentis 90 and Rhizoma Heterosmilacis 21) were selected from the 187 components of CKI. Additionally, some components, such as N-methylcytisine and trifolirhizin, did not pass the ADME screening, but were frequently reported in previous studies, so we added them to the list of active components (Xiumei and Cen, 2004; Juan et al., 2007; Yue, 2012; Liang et al., 2013). Therefore, 113 active components (Radix Sophorae Flavescentis 92 and Rhizoma Heterosmilacis 21) were included for further analysis. Detailed information is provided in Supplementary Table S4.
Through ADME screening and literature review, 92 potential components containing ideal pharmacokinetic profiles were selected from Radix Sophorae Flavescentis. For example, as one of the major components of CKI, sophoridine (KS18, DL = 0.25, Caco-2 = 1.13) displays antitumor, anti-allergic, anti-inflammatory, anti-arrhythmia, and antiviral properties, and affects the central nervous system (Han et al., 2009; Cuiping, 2010; Huang et al., 2014; Hu et al., 2016). Anagyrine (KS34, DL = 0.24, Caco-2 = 1.16) and (+)-lupanine (KS83, DL = 0.24, Caco-2 = 1.16) inhibit the proliferation and induction of apoptosis in human cervical cancer cells (Merghoub et al., 2011). Oxysophocarpine (KS89, DL = 0.29, Caco-2 = 1.042) suppressed cell proliferation, migration, invasion, and angiogenesis, induced cell cycle arrest, and enhanced apoptosis in oral squamous cell carcinoma (Liu et al., 2018). IDO1 expression can be downregulated by kushenol E (KS76, DL = 0.59, Caco-2 = 0.58) and kushenol F (KS74, DL = 0.61, Caco-2 = 0.45) to inhibit tumor proliferation (Kwon et al., 2019) and induce apoptosis (Kwon et al., 2020).
Among the 74 components in Rhizoma Heterosmilacis, 21 components met the screening criteria. For instance, diosgenin (BTL5, DL = 0.81, Caco-2 = 0.82), a steroid compound, has been shown to promote apoptosis and anticancer effects (Romero-Hernández et al., 2015; Jiang et al., 2016). Sitogluside (BTL1, DL = 0.62, Caco-2 = -0.14), beta-sitosterol (BTL2, DL = 0.75, Caco-2 = 1.32), sitosterol (BTL3, DL = 0.75, Caco-2 = 1.32), and stigmasterol (BTL4, DL = 0.75, Caco-2 = 1.45) can inhibit proliferation (Awad et al., 2000; Awad et al., 2003) and promote apoptosis (Awad et al., 2003), cell cycle arrest, and sphingomyelin cycle activation (Jia et al., 2018). Additionally, quercetin (BTL1, DL = 0.28, Caco-2 = 0.05) could be used to inhibit the proliferation of tumor cell lines (Ren et al., 2015) and induce apoptosis of tumor cells (Jia et al., 2018). Taxifolin (BTL13, DL = 0.27, Caco-2 = -0.23) inhibited proliferation, migration, and invasion of breast cancer cells by promoting EMT through β-catenin signal transduction (Li et al., 2019).
Target Prediction and Analysis of Rhizoma Heterosmilacis and Radix Sophorae Flavescentis
To evaluate the effect of the active components of CKI, we used SEA, HitPick, and SwissTargetPrediction tools to predict the targets of 113 active components of CKI. Finally, 780 targets were obtained from these tools (Supplementary Table S5). To explore the mechanism of CKI in the treatment of BC, 113 active components and 780 targets were used to construct the C-T network (Supplementary Figure S3). The C-T network results showed that 252 of all 780 targets were overlapped by the target list of Rhizoma Heterosmilacis and Radix Sophorae Flavescentis, and 320 targets were unique to Radix Sophorae Flavescentis, while 208 targets were unique to Rhizoma Heterosmilacis. A total of 4,592 component–target associations between 113 active components and 780 targets were contained in the C-T network. The average number of targets per component is 40.64, and the mean number of components per target is 5.89, which shows that CKI has multi-component and multi-target characteristics in treating BC.
Among all components, six components displayed a number of degrees above 120, namely palmitone (BTL15, degree = 149), quercetin (BTL1, degree = 144), quercetin (KS3, degree = 144), norartocarpetin (KS77, degree = 130), apigenin (KS2, degree = 129), and luteolin (KS1, degree = 125), all of which exhibit anticancer functions (Imran et al., 2019), and suppress migration and EMT (Cao et al., 2020). These six high-degree components only accounted for 5.3% of all components but covered 58.3% of all common targets. They all targeted common targets with high degrees, including ESR1 (degree = 65), MAPT (degree = 65), and ESR2 (degree = 63). It is worth noting that the top 25 targets with the highest degree were all dropped in the common targets list between Rhizoma Heterosmilacis and Radix Sophorae Flavescentis, most of which were related to the pathogenesis or treatment of BC. As the coding genes of the estrogen receptor, methylation of the ESR1 (degree = 65) promoter may be associated with shorter survival time and increased risk of drug resistance to anti-endocrine therapy (Kirn et al., 2018) and is commonly targeted by oxysophocarpine (KS89), kushenol E (KS76), and kushenol F (KS74) of Radix Sophorae Flavescentis and Stigmasterol (BTL5), quercetin (BTL1), and taxifolin (BTL13) of Rhizoma Heterosmilacis. Additionally, MAPT (degree = 65) is correlated with microtubule assembly and stabilization (Weingarten et al., 1975), which can promote bicalutamide resistance and is associated with survival in prostate cancer (Sekino et al., 2020). Our data analysis showed that (+)-Lupanine (KS76) and leachianone,g (KS74) from Radix Sophorae Flavescentis and quercetin (BTL1) from Rhizoma Heterosmilacis could target MAPT. We noted that several components of Rhizoma Heterosmilacis and Radix Sophorae Flavescentis can both activate common targets to play their role in the treatment of BC. Thus, these results suggest that Rhizoma Heterosmilacis and Radix Sophorae Flavescentis may act synergistically to treat BC via the common targets based on the “multi-component” and “multi-target” features and provide therapeutic targets for the cooperative treatment of BC.
To further decode the synergistic mechanism between Radix Sophorae Flavescentis and Rhizoma Heterosmilacis, we investigated the change in common target proportion under the condition of different degrees as thresholds. The results showed that following the increase in degree, the proportion of common target retention maintained a high growth trend. The proportion of common targets increased from 32.31 to 100% when the degree threshold increased from 1 to 28. Additionally, when the degree threshold increased from 1 to 28, the proportion of unique targets of Rhizoma Heterosmilacis decreased from 26.76 to 0%; however, when the degree threshold increased from 1 to 8, the proportion of specific targets of Radix Sophorae Flavescentis decreased from 41.02 to 0%. The results indicated that the common targets of Rhizoma Heterosmilacis and Radix Sophorae Flavescentis have relatively high degrees. A higher degree indicates that these targets have a higher influence among all targets, and it also suggests that Rhizoma Heterosmilacis and Radix Sophorae Flavescentis exert major synergistic effects by targeting common targets, which have a greater influence on the intervention response network.
To further decode the synergistic mechanism between them, we calculated the dynamic ratio change between common targets and unique targets of Radix Sophorae Flavescentis and Rhizoma Heterosmilacis using degree as the threshold. As shown in Figure 3, the proportion of common targets showed an overall upward trend from 32.31% (degree = 1) to 100% (degree = 28). However, the proportion of unique targets of Rhizoma Heterosmilacis and Radix Sophorae Flavescentis revealed a gradual downward trend. The ratio of unique targets of Radix Sophorae Flavescentis changed from 41.02 to 0% with a degree ranging from 1 to 28, while the ratio of unique targets of Rhizoma Heterosmilacis changed from 26.67 to 0% with a degree range from 1 to 7.
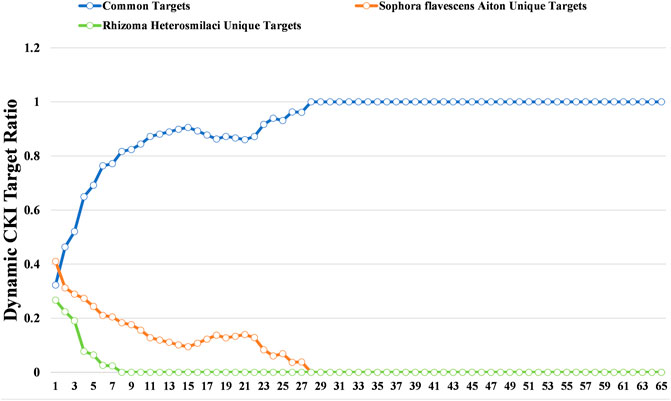
FIGURE 3. Dynamic ratio changes between common targets and unique targets of Rhizoma Heterosmilacis and Radix Sophorae Flavescentis.
In the C-T network, nodes with a higher degree usually represent the importance of all nodes. The results indicated that too much background noise may obscure the synergistic mechanism. Therefore, a model should be used to extract the core components.
Synergy Contribution Degree Calculation and Effect Verification
It is worth noting that Rhizoma Heterosmilacis and Radix Sophorae Flavescentis may play their roles through common targets based on the C-T network. However, the core component groups and mechanisms of synergy require further elucidation. To solve these problems, an SCD calculation method was designed that considers the synergy of both the topology of the components in the C-T network and the dose of the components. The SCD values of the active components of CKI are shown in Supplementary Table S6.
According to the calculation results, the top two components with an SCD sum of 49.05% were oxymatrine (KS80) and matrine (KS20). Twenty-four components contribute to the effects of CKI on BC, with a total of 95.03%. Surprisingly, 24 components accounted for only 21.23% of all active components in CKI and could cover 631 targets (80.89% of all CKI targets) (Figure 4A). Thus, we define these 24 components as the high contribution group of synergistic components (HCGSC). These results show that HCGSC plays key roles in all active components of CKI and may clarify why the herbs in CKI generate synergistic and combination effects on BC.
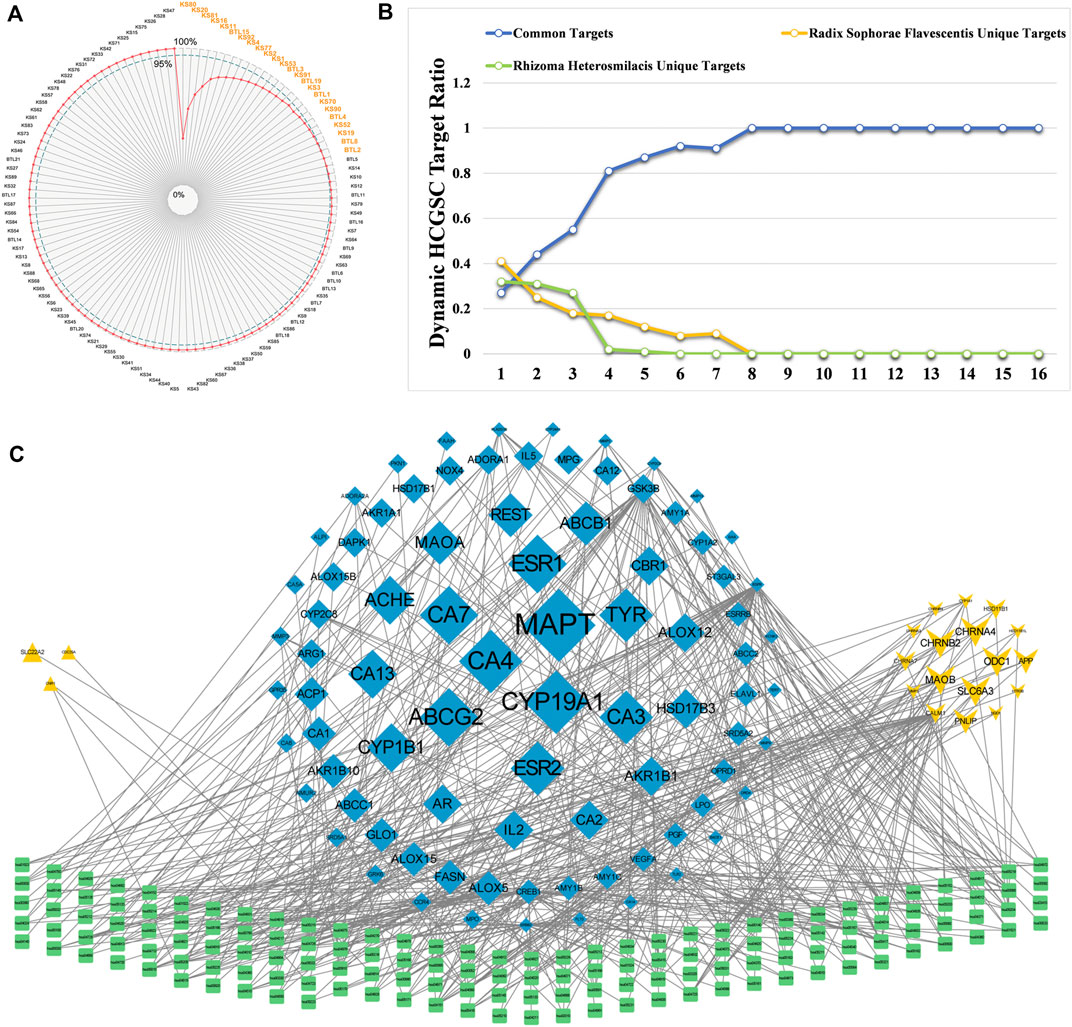
FIGURE 4. (A) Cumulative radar chart of the ratio between component accumulation targets and the targets of CKI. The label within the chart represented the ratio (%) between component accumulation targets and the targets of CKI. (B) The dynamic ratio changes between common targets of HCGSC and unique targets of Radix Sophorae Flavescentis, and Rhizoma Heterosmilacis of HCGSC. (C) The network with targets and their enriched pathways (the degrees of the targets were higher than the third quartile of the targets in the C-T network of HCGSC) and their enriched pathways.
To evaluate the reliability of HCGSC, we defined two references for further comparison: the first reference was 70 genes, which overlapped with DEGs and CKI targets, and the second reference was the 17 pathways, which are overlapped by enriched DEGs pathways and CKI targets. The targets of HCGSC contained 62 genes, which could cover 88.57% (Supplementary Figure S4A) of the first reference, while the HCGSC targets enriched 158 pathways, which could cover 94.11% (Supplementary Figure S4B) of the second reference. Of these 62 overlapped genes, 39 were targeted by Rhizoma Heterosmilacis, while 43 were targeted by Radix Sophorae Flavescentis. These results confirm the reliability and accuracy of the HCGSC selection model. Two networks were constructed to analyze HCGSC. The first is a C-T network based on 24 components and 631 targets. The second is the target–pathway network based on 532 targets and 158 enriched pathways.
In the first network, the degree of targets ranged from 1 to 16, with the third quartile equal to 3. The change in the proportion of common targets under different conditions showed that the common targets had a higher degree (Figure 4B). The proportion of common targets increased from 27.26% to 100%. Additionally, with the increase in the threshold of the degree, the proportion of unique targets of Rhizoma Heterosmilacis decreased from 32.17 to 0%, while the proportion of specific targets of Radix Sophorae Flavescentis decreased from 40.57 to 0%. In the second network, we assigned the degree from the first network to the targets; targets with a degree higher than 3 (the third quartile of the targets in the C-T network) and their enriched pathways were selected to construct the network (Figure 4C). Visualization showed that the size of the target was positively related to the degree. We noted that the common targets (in blue diamond) displayed more connections with pathways and larger sizes than the others. However, the unique targets of Rhizoma Heterosmilacis (yellow triangle) and Radix Sophorae Flavescentis (yellow arrow) not only showed fewer connections with pathways, but were also smaller than the common targets. These two networks indicated that the common targets of Rhizoma Heterosmilacis and Radix Sophorae Flavescentis in HCGSC have relatively high degrees, which shows that Rhizoma Heterosmilacis and Radix Sophorae Flavescentis have a synergistic effect on the nodes with higher influence.
Potential Synergistic Mechanism Analysis of CKI in BC Treatment Based on HCGSC
GO Enrichment Analysis for CKI Based on HCGSC
To further interpret the potential synergistic mechanisms of Rhizoma Heterosmilacis and Radix Sophorae Flavescentis, we performed GO-BP enrichment analysis for the targets of Radix Sophorae Flavescentis and Rhizoma Heterosmilacis in HCGSC. Radix Sophorae Flavescentis targets in HCGSC were enriched in 1517 GO-BP terms, while Rhizoma Heterosmilacis targets in HCGSC were enriched in 1394 GO-BP terms. A total of 927 joint GO-BP terms were found between them (Supplementary Figure S4C).
We selected the GO-BP terms with an FDR-adjusted p value lower than that of the first quartile of all GO-BP terms to build a network (Figure 5A). We found that 86.49% of the selected GO-BP terms belong to the commonly enriched GO-BP terms between the targets of Radix Sophorae Flavescentis and Rhizoma Heterosmilacis in HCGSC. This suggests that Rhizoma Heterosmilacis and Radix Sophorae Flavescentis have potential synergistic mechanisms in the treatment of BC through these highly reliable, commonly enriched GO-BP terms based on HCGSC.
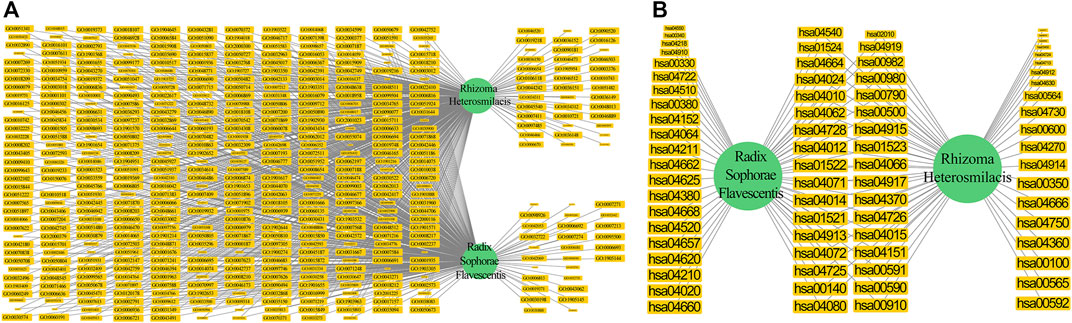
FIGURE 5. Components were represented in green. The GO-BP terms and pathways were represented in yellow. The size of the pathways was related to the FDR adjust p value. The lower FDR adjust p value represented the larger size. (A) The network of selected GO-BP terms based on the first quartile value of all GO-BP terms FDR adjust p value. The left of the network was the commonly enriched GO-BP terms. The upper right of the network was the unique GO-BP terms of Rhizoma Heterosmilacis. The lower right of the network was the unique GO-BP terms of Radix Sophorae Flavescentis. (B) The network of pathways between Rhizoma Heterosmilacis and Radix Sophorae Flavescentis. The center of the network was the commonly enriched pathways. The right of the network was the unique pathways of Rhizoma Heterosmilacis. The left of the network was the unique pathways of Radix Sophorae Flavescentis.
It is worth noting that most of the joint GO-BP terms were closely related to BC, such as the steroid metabolic process (GO:0008202, FDR adjusted p value = 2.24E-27) and steroid biosynthetic process (GO:0006694, FDR adjusted p value = 1.23E-18). Regarding the genes of these GO terms, estrone (E1) can protect women from breast cancer, while estradiol (E2) and estriol (E3) may enhance the risk (Lemon et al., 1966; Cohn et al., 2017). The expression of ATGL is correlated with tumor aggressiveness in vivo (Wang et al., 2017), which is related to the lipid catabolic process (GO:0016042, FDR adjust p value = 1.46E-22), lipid transport (GO:0006869, FDR adjusted p value = 4.04E-17), and lipid localization (GO:0010876, FDR adjusted p value = 1.16E-16). Including TRPCs, TPRVs, TRPMs, TRPA1, TRPPs, and TRPMLs (Berridge et al., 2003; Rohacs, 2005; Monteith et al., 2007; Venkatachalam and Montell, 2007; Doherty et al., 2015; Vangeel and Voets, 2019), calcium homeostasis plays an important role in the occurrence, development, and metastasis of breast cancer, which is related to the response to metal ions (GO:0010038, FDR adjust p value = 9.17E-13).
Additionally, the other GO-BP terms of targets for Radix Sophorae Flavescentis and Rhizoma Heterosmilacis in HCGSC, respectively, are also related to BC treatment. The ERK/MAPK and JAK2/PI3K signaling cascades in breast cancer cells can be activated by α7-nAChR activation (Chen et al., 2006; Nishioka et al., 2011; Kalantari-Dehaghi et al., 2015), while α9-nAChR overexpression is observed in tumor tissues compared with adjacent normal tissues (Lee et al., 2010). These genes are involved in synaptic transmission, cholinergic (GO:0007271, FDR adjust p value = 3.54E-19), and acetylcholine receptor signaling pathways (GO:0095500, FDR adjust p value = 1.91E-10) of Radix Sophorae Flavescentis in HCGSC. For the remaining GO-BP terms of Rhizoma Heterosmilacis in HCGSC, some steroid hormones, such as vitamin D, may have anticancer properties, while others may favor cancer progression, including estrogens and androgens (Restrepo-Angulo et al., 2020), which are related to regulation of steroid metabolic processes (GO:0019218, FDR adjusted p value = 2.49E-13).
Pathway Analyses Exploring Therapeutic Mechanisms of CKI Based on HCGSC
To further dissect the potential synergistic mechanisms of Rhizoma Heterosmilacis and Radix Sophorae Flavescentis, we performed the KEGG pathway enrichment analysis for the targets of Radix Sophorae Flavescentis and Rhizoma Heterosmilacis in HCGSC, respectively. Targets of Radix Sophorae Flavescentis in HCGSC were enriched in 55 pathways, while those of Rhizoma Heterosmilacis were enriched in 54 pathways. Thirty-four commonly enriched pathways were found between them (Supplementary Figure S4D).
We used pathways to build a network (Figure 5B). Surprisingly, 45.33% of pathways belonged to the commonly enriched pathways between targets of Radix Sophorae Flavescentis and Rhizoma Heterosmilacis in HCGSC. This suggests that Rhizoma Heterosmilacis and Radix Sophorae Flavescentis have potential synergistic mechanisms in the treatment of BC through these commonly enriched pathways based on HCGSC.
As shown in Figure 5B, most of the commonly enriched pathways were closely related to BC. Arachidonic acid metabolism (hsa00590, FDR adjusted p value = 4.47E-10), as a metabolic process, and the arachidonic acid (AA) pathway play key roles in carcinogenesis (Yarla et al., 2016). Additionally, PLA2s, COXs, LOXs, CYP-dependent monooxygenases, and their metabolites are known to play key roles in carcinogenesis (Tong et al., 2002; Go et al., 2015; Wu et al., 2015; Yarla et al., 2016). The migration and invasion of MDA-MB-231 breast cancer cells may be induced by linoleic acid (Serna-Marquez et al., 2017), which is related to linoleic acid metabolism (hsa00591, FDR adjusted p value = 4.47E-10). The PI3K-Akt signaling pathway (hsa04151, FDR adjusted p value = 2.78E-07) plays an important role in the tumorigenesis of breast cancer (Yuan and Cantley, 2008), and its activation may promote tumor progression in mice (Mei et al., 2018).
Additionally, other pathways of targets for Radix Sophorae Flavescentis and Rhizoma Heterosmilacis in HCGSC, respectively, are also related to BC treatment. For other pathways of Radix Sophorae Flavescentis in HCGSC, cellular Ca2+ signals have been implicated in the induction of apoptosis and regulation of apoptotic pathways (Berridge et al., 1998; Sergeev, 2005), which is related to the calcium signaling pathway (hsa04020, FDR adjusted p value = 1.43E-04). Regarding other pathways of Rhizoma Heterosmilacis in HCGSC, the higher alpha-linolenic acid content may reduce the risk of breast cancer, which is related to alpha-linolenic acid metabolism (hsa00592, FDR adjusted p value = 3.13E-06).
To further explain the synergistic mechanisms, we obtained 34 commonly enriched pathways between targets for Radix Sophorae Flavescentis SC and Rhizoma Heterosmilacis in HCGSC and 25 DEG-enriched pathways. By analyzing these pathways, we found that five pathways overlapped between 34 and 25 pathways, which we considered highly reliable pathways. These include the PI3K-Akt signaling pathway (hsa04151), cAMP signaling pathway (hsa04024), neuroactive ligand–receptor interaction (hsa04080), dopaminergic synapse (hsa04728), and ABC transporters (hsa02010). The PI3K-Akt and cAMP signaling pathways are reportedly related to BC, with a greater number of published studies (Mei et al., 2018; Dong et al., 2015). Next, we constructed a comprehensive pathway (Figure 6), including the PI3K-Akt and cAMP signaling pathways, using CyKEGGParser to explore the synergetic mechanisms of Rhizoma Heterosmilacis and Radix Sophorae Flavescentis in the treatment of BC using CKI (Nersisyan et al., 2014).
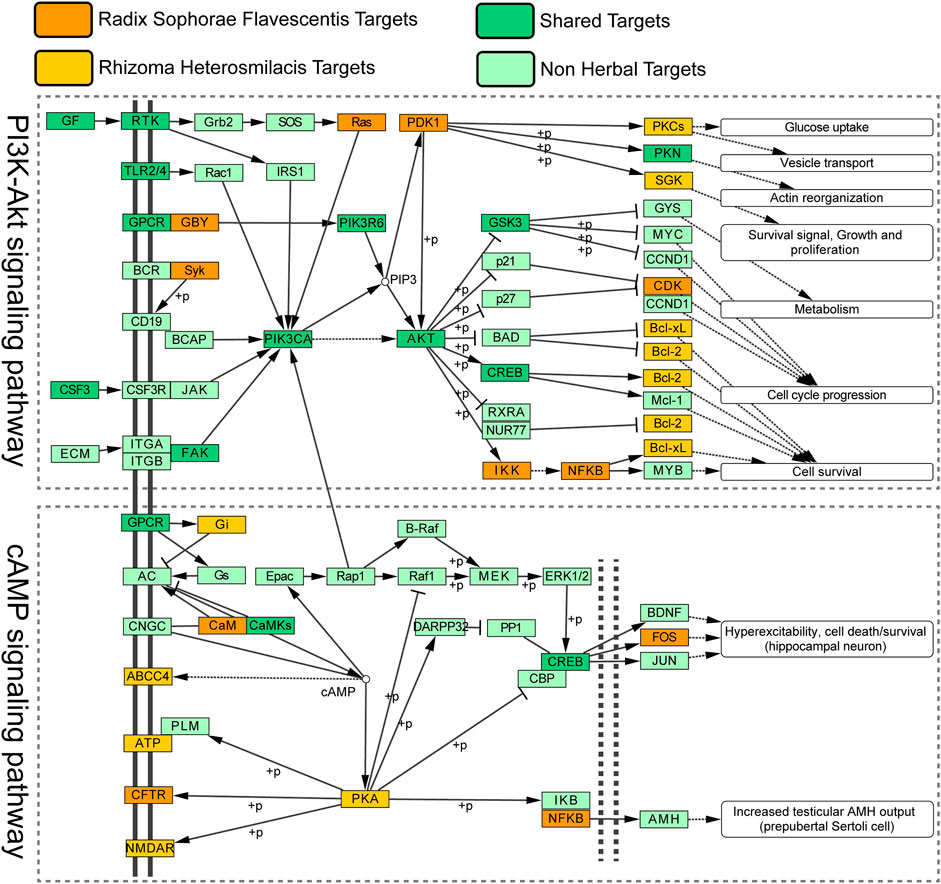
FIGURE 6. Distribution of target proteins of CKI on the compressed BC pathway. The colors of the blanks represent different types of targets.
In the PI3K-Akt signaling pathway, Radix Sophorae Flavescentis acts on targets of the upstream pathway, such as Ras, GBY, and Syk, while Rhizoma Heterosmilacis acts on downstream targets, such as PKCs, SGK, Bcl-2, and Bcl-xL. These results indicate that Rhizoma Heterosmilacis and Radix Sophorae Flavescentis have synergistic and complementary effects on cell survival, cell cycle progression, metabolism, survival signal, growth and proliferation, actin reorganization, vesicle transport, and glucose uptake. Additionally, in the cAMP signaling pathway, Rhizoma Heterosmilacis acts as a target of the upstream pathway, including Gi and PKA, while Radix Sophorae Flavescentis acts as a target of the downstream pathway, including FOS, NFKB, and CFTR, which are associated with pathways of hyperexcitability, cell death or survival (hippocampal neuron), and increased testicular AMH output (prepubertal Sertoli cells). As the pathogenic factors of BC are related to metabolism, cell survival, proliferation, cell death, and cell cycle (Jia et al., 2018), the above results suggest that Rhizoma Heterosmilacis and Radix Sophorae Flavescentis can exert a synergistic effect on BC at multiple pathways.
Molecular Docking Validation of HCGSC in the Treatment of BC
To evaluate the function of HCGSC in BC treatment, molecular docking was employed to simulate the interaction between the ligands and the protein. Twenty-four components from the HCGSC of CKI and 85 proteins coded by 30 genes were used for molecular docking, and 18,254 docking relationships were obtained from the docking results (Supplementary Table S7). The result with the lowest affinity value in each component-protein was selected as the best docking relationship (2040 docking relationships), of which the affinity value ranged from −11.3 kcal/mol to −0.5 kcal/mol. Among the best docking relationships, three exhibited affinity values lower than −11.0 kcal/mol, BTL3 and BTL4 had the lowest affinity values of −11.3 kcal/mol, binding with protein 3ovv coded by PKA and protein 2w73 coded by CaM, respectively. BTL3 could bind 2w73 coded by CaM with the affinity values of −11.2 kcal/mol thereafter (Figures 7A–C). Based on the literature reports, improved binding between component–protein interactions should have a lower value of affinity (Elhenawy et al., 2019), and the histogram revealed that most of the results were concentrated in the lower affinity value position (Figure 7D).
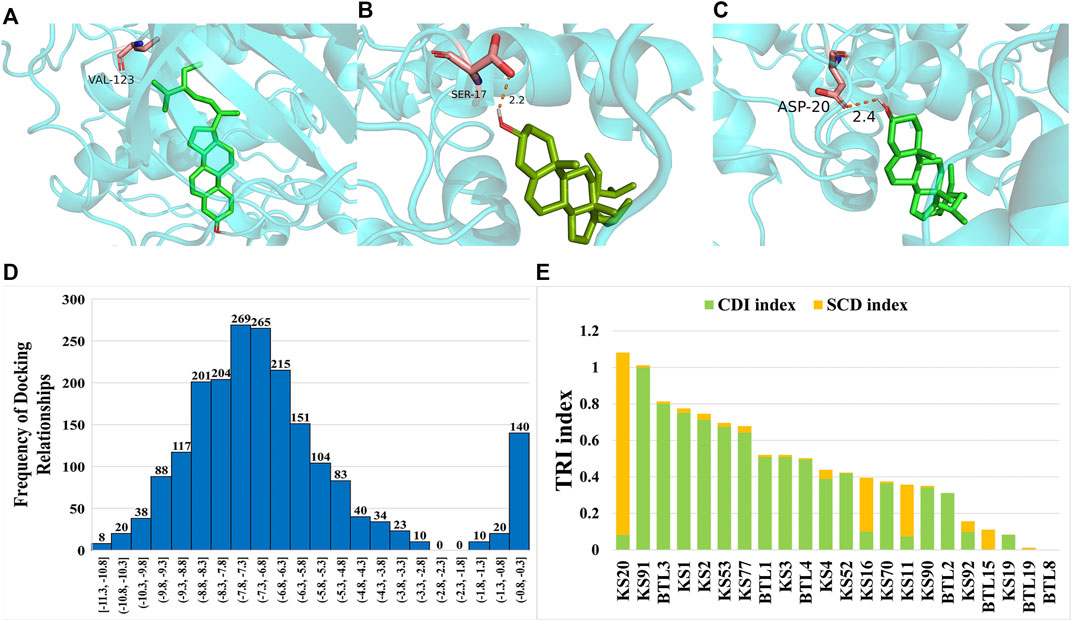
FIGURE 7. (A) Docking result visualization of BTL3-3ovv (coded by PKA). (B) Docking result visualization of BTL4-2w73 (coded by CaM). (C) Docking result visualization of BTL3-2w73 (coded by Cam). (D) The frequency histogram of the affinity value. (E) The bar plot to display the composition of CDI and SCD in each TRI.
To reduce the influence of background noise of docking relationships with high affinity values, we selected the docking relationships that were lower than the average affinity value (−6.743) from all the best docking relationships as a highly reliable docking relationship (HRDR). Twenty-two components and 82 proteins coded by 29 genes were identified in HRDR with 1,255 docking relationships. Among the 22 components, 15 components were from Radix Sophorae Flavescentis, including KS1, KS2, KS3, KS4, KS11, KS16, KS19, KS20, KS52, KS53, KS70, KS77, KS90, KS91, and KS92, and seven components were from Rhizoma Heterosmilacis, including BTL1, BTL2, BTL3, BTL4, BTL8, BTL15, and BTL19. The 15 components of Radix Sophorae Flavescentis could bind 82 proteins coded by 29 genes, and the seven components of Rhizoma Heterosmilacis could bind 81 proteins coded by 29 genes. These results suggest that Rhizoma Heterosmilacis and Radix Sophorae Flavescentis exert a synergistic effect on BC at multiple pathways.
To evaluate the therapeutic potential of components in HRDR, we designed a mathematical model to calculate the TRI of each component in the HRDR from the HCGSC. To further decode the mechanism of CKI in treating BC comprehensively, we integrated the CDI and SCD to calculate the TRI, which not only considered synergistic effects, but also considered the docking relationship. Detailed information on the TRI is listed in Supplementary Table S8. As shown in Figure 7E, KS20 (TRI = 1.08), KS91 (TRI = 1.01), BTL3 (TRI = 0.81), and KS1 (TRI = 0.78) were the top four components with TRI scores higher than 0.75. Among these four components, matrine (KS20), with the highest TRI, was confirmed by a number of published studies related to BC therapy (Li et al., 2015). The following three components with higher TRIs were selected for in vitro experiments to validate the reliability of our proposed strategy.
Luteolin and Trifolirhizin Inhibited Proliferation and Metastasis of MCF-7 Cells In Vitro
Based on the TRI results, trifolirhizin (KS91), beta-sitosterol (BTL3), and luteolin (KS1) were selected for in vitro experiments. The in vitro experiments proved that two of the three core components of HCGSC showed significant inhibitory effects on breast cancer cell proliferation and migration. The effects of luteolin and trifolirhizin on the viability of MCF-7 cells were determined using the MTT assay. As shown in Figures 8A,B, the proliferation of MCF-7 cells was suppressed by luteolin and trifolirhizin in a dose-dependent manner. Subsequently, the effects of luteolin and trifolirhizin on the migration of MCF-7 cells were investigated. As presented in Figures 7C,D, luteolin (40 and 80 Μm) and trifolirhizin (20 and 40 Μm) dramatically inhibited the migration of MCF-7 cells. These results indicated that luteolin and trifolirhizin markedly inhibited the proliferation and migration of MCF-7 cells.
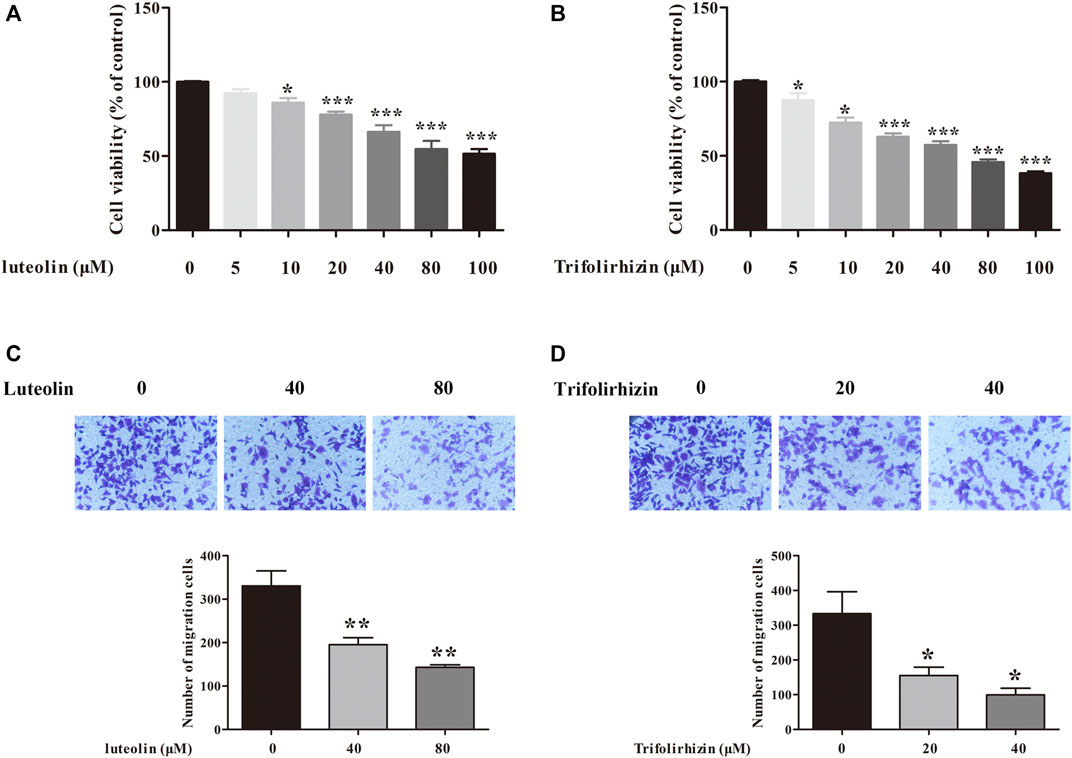
FIGURE 8. Inhibitory effects of luteolin (A) and trifolirhizin (B) on the proliferation of MCF-7 cells. Inhibitory effects of luteolin (C) and trifolirhizin (D) on migration in MCF-7 cells. Data are represented as mean ± SEM (n = 3). *p < 0.05, **p < 0.01, ***p < 0.001 as compared with that of the control group.
Discussion
Breast cancer is one of the most common malignant tumors among women worldwide, with the second highest death rate in female cancer patients (Siegel et al., 2020). At present, research regarding cancer treatment using TCM is still in the exploratory stage, and CKI has a wide range of applications in the treatment of tumors. However, there are few studies on CKI in the treatment of BC through systematic pharmacology. Therefore, we designed a systematic pharmacology strategy combining differentially expressed gene analysis, pharmacokinetics synthesis screening, target identification, synergy contribution degree, network analysis, therapeutic response index calculation, and experimental validation and provided a reference for this new method.
In this study, we examined the synergistic effect of CKI on BC at three levels. At the first level, the target coincidence of Rhizoma Heterosmilacis and Radix Sophorae Flavescentis in CKI indicates that Rhizoma Heterosmilacis and Radix Sophorae Flavescentis may act synergistically to exert a therapeutic effect in treating BC. First, we constructed a C-T network and found that most targets with a higher degree were mapped on the joint targets between Rhizoma Heterosmilacis and Radix Sophorae Flavescentis. These results suggest that Rhizoma Heterosmilacis and Radix Sophorae Flavescentis may act synergistically to treat BC via common targets and provide therapeutic targets for the cooperative treatment of BC. However, in the complex C-T network, we know neither the key synergistic components in treating BC nor their synergistic mechanisms.
To solve this issue, at the second level, we designed an SCD model with the advantages of reflecting the topology character of the C-T network and the component dose, which implemented a semi-quantitative system pharmacology model. Through the analysis of the SCD model, 24 components compose HCGSC, which can contribute to the effects of CKI on BC with a sum of 95.03% and map 80.89% (631/780) of all targets of CKI. Two references were employed to confirm the reliability and accuracy of the HCGSC selection model, including genes and pathways. The targets of HCGSC contained 62 genes, which could cover 88.57% of the first reference, while the HCGSC targets enriched 81 pathways, which could cover 70.59% of the second reference. Third, we performed GO-BP and KEGG enrichment analysis for Radix Sophorae Flavescentis and Rhizoma Heterosmilacis targets of HCGSC. We found that most of the overlapped GO-BP terms and pathways between them affect BC treatment, and most of them have a lower FDR adjusted p value.
At the third level, to further understand the synergistic mechanisms of CKI and infer the function of synergistic components and verify their effectiveness, three aspects were employed. First, a comprehensive pathway was constructed to explore the synergetic mechanism of Rhizoma Heterosmilacis and Radix Sophorae Flavescentis in the treatment of BC using CKI. In the PI3K-Akt and cAMP signaling pathways, Rhizoma Heterosmilacis and Radix Sophorae Flavescentis can activate upstream and downstream targets to play their role in the treatment of BC. These results suggest that CKI can produce a combined effect on BC. Second, we designed a TRI model, which provides the results of SCD and docking, to comprehensively evaluate the synergy contribution rate of the components in HCGSC and the binding ability of the components and proteins. TRI calculation results showed that four components displayed a TRI score higher than 0.75, including matrine (KS20, TRI = 1.08), trifolirhizin (KS91, TRI = 1.01), beta-sitosterol (BTL3, TRI = 0.81), and luteolin (KS1, TRI = 0.78), which may be the core components in the treatment of BC with synergistic mechanisms. Third, MTT and Transwell assays were employed to determine the function of luteolin and trifolirhizin. The results showed that they markedly inhibited the proliferation and migration of MCF-7 cells.
In this study, we proposed two novel mathematical models for the speculation of synergistic components and mechanisms. The method provided a methodological reference for decoding the synergistic mechanism of TCM in treating complex diseases. However, several limitations must be mentioned. 1) The precise synergistic mechanisms based on the calculation predictions warrant further validation. 2) Because the interaction between herbs is extremely complex, it may be incomplete to explore the synergistic mechanisms based on our model. Multiple synergistic effects combining with other techniques should be further considered. 3) The TRI model is proposed based on network topology methodology, which is more suitable for the case that herbs have a complex network. This algorithm used undirected network, which ignores the activation or inhibition effects of the disease targets.
Data Availability Statement
Publicly available datasets were analyzed in this study. This data can be found here: https://portal.gdc.cancer.gov/ https://tcmsp-e.com/.
Author Contributions
YL and KW contributed equally to this article. Conceptualization: YL, WC, and DG.; methodology: DG and GQ; Software: YL and JC; validation: XQ and AL; investigation: XQ and AL; data curation: YL and YC; writing—original draft: YL and KW; writing—review and editing: DG, WC, and GQ; visualization: YL and KW; funding acquisition: DG and WC. All authors have read and agreed to the published version of the manuscript.
Funding
This study was financially supported by the Natural Science Foundation of China (grant No. 82171929), the Natural Science Foundation of Guangdong Province (grant No. 2019A1515011168), the Startup fund from the Southern Medical University (grant No. G820282016), the Natural Science Foundation Council of China (grant No. 31501080, 32070676), the Natural Science Foundation of Guangdong Province (grant No. 2021A1515010737), Hong Kong Baptist University Strategic Development Fund (grant No. SDF13-1209-P01, SDF15-0324-P02(b) and SDF19-0402-P02), Hong Kong Baptist University Interdisciplinary Research Matching Scheme (grant No. RC/IRCs/17-18/04).
Conflict of Interest
The authors declare that the research was conducted in the absence of any commercial or financial relationships that could be construed as a potential conflict of interest.
Publisher’s Note
All claims expressed in this article are solely those of the authors and do not necessarily represent those of their affiliated organizations, or those of the publisher, the editors, and the reviewers. Any product that may be evaluated in this article, or claim that may be made by its manufacturer, is not guaranteed or endorsed by the publisher.
Supplementary Material
The Supplementary Material for this article can be found online at: https://www.frontiersin.org/articles/10.3389/fphar.2021.723147/full#supplementary-material
Supplementary Figure S1 | The process of ADME screening.
Supplementary Figure S2 | The heatmap of DEGs of breast cancer. The red color represents the high expression of the gene and the green color represents the low expression of the gene.
Supplementary Figure S3 | The C-T network of Rhizoma Heterosmilacis and Radix Sophorae Flavescentis. The targets were in yellow and the components were in the green. The common targets were placed at the center of the network. The targets at the left were the unique targets of Radix Sophorae Flavescentis, while those at the right were the unique targets of Rhizoma Heterosmilacis. The size of the targets and components were related to the degree. The higher degree represented the larger size.
Supplementary Figure S4 | (A) The Venn diagram of the joint targets between BC and CKI and the targets of HCGSC. (B) The Venn diagram of the joint KEGG pathways between BC and CKI and the KEGG pathways of HCGSC. (C) The Venn diagram of joint GO-BP terms between Radix Sophorae Flavescentis in HCGSC and Rhizoma Heterosmilacis in HCGSC. (D) The Venn diagram of the joint pathway between Radix Sophorae Flavescentis in HCGSC and Rhizoma Heterosmilacis in HCGSC.
Abbreviations
ADME, absorption, distribution, metabolism, and excretion; BC, breast cancer; CKI, compound kushen injection; C-T network, component–target network; DEGs, differentially expressed gene analysis; HCGSC, high contribution group of synergistic components; HPLC, high performance liquid chromatography; HRDR, highly reliable docking relationship; SCD, synergy contribution degree; TCGA, The Cancer Genome Atlas; TCM, traditional Chinese medicine; TRI, therapeutic response index.
References
Anders, S., and Huber, W. (2010). Differential expression analysis for sequence count data. Genome Biol. 11 (10), R106. doi:10.1186/gb-2010-11-10-r106
Astashchanka, A., Shroka, T. M., and Jacobsen, B. M. (2019). Mucin 2 (MUC2) modulates the aggressiveness of breast cancer. Breast Cancer Res. Treat. 173 (2), 289–299. doi:10.1007/s10549-018-4989-2
Awad, A. B., Downie, A. C., and Fink, C. S. (2000). Inhibition of growth and stimulation of apoptosis by beta-sitosterol treatment of MDA-MB-231 human breast cancer cells in culture. Int. J. Mol. Med. 5 (5), 541–545. doi:10.3892/ijmm.5.5.541
Awad, A. B., Roy, R., and Fink, C. S. (2003). Beta-sitosterol, a plant sterol, induces apoptosis and activates key caspases in MDA-MB-231 human breast cancer cells. Oncol. Rep. 10 (2), 497–500. doi:10.3892/or.10.2.497
Balaji, K., Subramanian, B., Yadav, P., Anu Radha, C., and Ramasubramanian, V. (2016). Radiation therapy for breast cancer: Literature review. Med. Dosim. 41 (3), 253–257. doi:10.1016/j.meddos.2016.06.005
Berridge, M. J., Bootman, M. D., and Lipp, P. (1998). Calcium--a life and death signal. Nature 395 (6703), 645–648. doi:10.1038/27094
Berridge, M. J., Bootman, M. D., and Roderick, H. L. (2003). Calcium signalling: dynamics, homeostasis and remodelling. Nat. Rev. Mol. Cel Biol 4 (7), 517–529. doi:10.1038/nrm1155
Binggang, Z., Jing, L., Yuzhuo, F., and Gang, S. (2002). Experimental study on apoptosis of human breast cancer cell line MCF-7 induced by oxymatrine. Chin. Pharmacol. Bull. (06), 689–691.
Cao, D., Zhu, G. Y., Lu, Y., Yang, A., Chen, D., Huang, H. J., et al. (2020). Luteolin suppresses epithelial-mesenchymal transition and migration of triple-negative breast cancer cells by inhibiting YAP/TAZ activity. Biomed. Pharmacother. 129, 110462. doi:10.1016/j.biopha.2020.110462
Chen, Z. B., Liu, C., Chen, F. Q., Li, S. Y., Liang, Q., and Liu, L. Y. (2006). Effects of tobacco-specific carcinogen 4-(methylnitrosamino)-1-(3-pyridyl)-1-butanone (NNK) on the activation of ERK1/2 MAP kinases and the proliferation of human mammary epithelial cells. Environ. Toxicol. Pharmacol. 22 (3), 283–291. doi:10.1016/j.etap.2006.04.001
Cochran, J. M., Busch, D. R., Leproux, A., Zhang, Z., O'Sullivan, T. D., Cerussi, A. E., et al. (2019). Tissue oxygen saturation predicts response to breast cancer neoadjuvant chemotherapy within 10 days of treatment. J. Biomed. Opt. 24 (02), 1–11. doi:10.1117/1.JBO.24.2.021202
Cohn, B. A., Cirillo, P. M., Hopper, B. R., and Siiteri, P. K. (2017). Third Trimester Estrogens and Maternal Breast Cancer: Prospective Evidence. J. Clin. Endocrinol. Metab. 102 (10), 3739–3748. doi:10.1210/jc.2016-3476
Colaprico, A., Silva, T. C., Olsen, C., Garofano, L., Cava, C., Garolini, D., et al. (2016). TCGAbiolinks: an R/Bioconductor package for integrative analysis of TCGA data. Nucleic Acids Res. 44 (8), e71. doi:10.1093/nar/gkv1507
Condorelli, R., and Vaz-Luis, I. (2018). Managing side effects in adjuvant endocrine therapy for breast cancer. Expert Rev. Anticancer Ther. 18 (11), 1101–1112. doi:10.1080/14737140.2018.1520096
Crean-Tate, K. K., and Reizes, O. (2018). Leptin Regulation of Cancer Stem Cells in Breast and Gynecologic Cancer. Endocrinology 159 (8), 3069–3080. doi:10.1210/en.2018-00379
Cui, J., Qu, Z., Harata-Lee, Y., Nwe Aung, T., Shen, H., Wang, W., et al. (2019). Cell cycle, energy metabolism and DNA repair pathways in cancer cells are suppressed by Compound Kushen Injection. BMC Cancer 19 (1), 103. doi:10.1186/s12885-018-5230-8
Cui, J., Qu, Z., Harata-Lee, Y., Shen, H., Aung, T. N., Wang, W., et al. (2020). The effect of compound kushen injection on cancer cells: Integrated identification of candidate molecular mechanisms. PLoS One 15 (7), e0236395. doi:10.1371/journal.pone.0236395
Cuiping, Y. (2010). Review on Pharmacological Researches of Sophoridine. Chin. J. Exp. Traditional Med. Formulae.
Doherty, A. H., Ghalambor, C. K., and Donahue, S. W. (2015). Evolutionary Physiology of Bone: Bone Metabolism in Changing Environments. Physiology (Bethesda) 30 (1), 17–29. doi:10.1152/physiol.00022.2014
Dong, H., Claffey, K. P., Brocke, S., and Epstein, P. M. (2015). Inhibition of breast cancer cell migration by activation of cAMP signaling. Breast Cancer Res. Treat. 152 (1), 17–28. doi:10.1007/s10549-015-3445-9
Elhenawy, A. A., Al-Harbi, L. M., El-Gazzar, M. A., Khowdiary, M. M., and Moustfa, A. (2019). Synthesis, molecular properties and comparative docking and QSAR of new 2-(7-hydroxy-2-oxo-2H-chromen-4-yl)acetic acid derivatives as possible anticancer agents. Spectrochim Acta A. Mol. Biomol. Spectrosc. 218, 248–262. doi:10.1016/j.saa.2019.02.074
Enien, M. A., Ibrahim, N., Makar, W., Darwish, D., and Gaber, M. (2018). Health-related quality of life: Impact of surgery and treatment modality in breast cancer. J. Cancer Res. Ther. 14 (5), 957–963. doi:10.4103/0973-1482.183214
Gao, L., Wang, K. X., Zhou, Y. Z., Fang, J. S., Qin, X. M., and Du, G. H. (2018). Uncovering the anticancer mechanism of Compound Kushen Injection against HCC by integrating quantitative analysis, network analysis and experimental validation. Sci. Rep. 8 (1), 624. doi:10.1038/s41598-017-18325-7
Gfeller, D., Grosdidier, A., Wirth, M., Daina, A., Michielin, O., and Zoete, V. (2014). SwissTargetPrediction: a web server for target prediction of bioactive small molecules. Nucleic Acids Res. 42, W32–W38. (Web Server issue). doi:10.1093/nar/gku293
Go, R. E., Hwang, K. A., and Choi, K. C. (2015). Cytochrome P450 1 family and cancers. J. Steroid Biochem. Mol. Biol. 147, 24–30. doi:10.1016/j.jsbmb.2014.11.003
Guo, Y., Fan, Y., and Pei, X. (2020). Fangjihuangqi Decoction inhibits MDA-MB-231 cell invasion in vitro and decreases tumor growth and metastasis in triple-negative breast cancer xenografts tumor zebrafish model. Cancer Med. 9 (7), 2564–2578. doi:10.1002/cam4.2894
Guo, Y., Huang, Y., Shen, H., Sang, X., Ma, X., Zhao, Y., and et alXiao, X. (2015). Efficacy of Compound Kushen Injection in Relieving Cancer-Related Pain: A Systematic Review and Meta-Analysis. United States: Hindawi Publishing Corporation, 840742–840748.
Han, F. M., Zhu, M. M., Chen, H. X., and Chen, Y. (2009). Identification of Sophoridine and its Metabolites in Rat Urine by Liquid Chromatography-Tandem Mass Spectrometry. Anal. Lett. 43 (1), 45–54. doi:10.1080/00032710903276471
Hong, Q., Yu, S., Mei, Y., Lv, Y., Chen, D., Wang, Y., et al. (2014). Smilacis Glabrae Rhizoma Reduces Oxidative Stress Caused by Hyperuricemia via Upregulation of Catalase. Cell. Physiol. Biochem. 34 (5), 1675–1685. doi:10.1159/000366369
Hu, S. T., Shen, Y. F., Gong, J. M., and Yang, Y. J. (2016). Effect of sophoridine on Ca²⁺ induced Ca²⁺ release during heart failure. Physiol. Res. 65, 43–52. doi:10.33549/physiolres.933052
Huang, X., Li, B., and Shen, L. (20142014). Studies on the Anti-inflammatory Effect and its Mechanisms of Sophoridine. J. Anal. Methods Chem. 2014, 502626–6. doi:10.1155/2014/502626
Hy, L., and Qy, H. (2014). Mechanisms of yanshu injection for overcoming multidrug resistance in breast carcinoma MCF-7 cells: an experimental research. Chin. J. integrated traditional West. Med. 34 (3), 324–328.
Ij, H., Tj, R., and Rt, B. (1989). Characterization of the human colon carcinoma cell line (Caco-2) as a model system for intestinal epithelial permeability. Gastroenterology 96 (3), 736–749.
Imran, M., Rauf, A., Abu-Izneid, T., Nadeem, M., Shariati, M. A., Khan, I. A., et al. (2019). Luteolin, a flavonoid, as an anticancer agent: A review. Biomed. Pharmacother. 112, 108612. doi:10.1016/j.biopha.2019.108612
Jacobsen, A. C., Nielsen, S., Brandl, M., and Bauer-Brandl, A. (2020). Drug Permeability Profiling Using the Novel Permeapad® 96-Well Plate. Pharm. Res. 37 (6), 93. doi:10.1007/s11095-020-02807-x
Jia, L., Huang, S., Yin, X., Zan, Y., Guo, Y., and Han, L. (2018). Quercetin suppresses the mobility of breast cancer by suppressing glycolysis through Akt-mTOR pathway mediated autophagy induction. Life Sci. 208, 123–130. doi:10.1016/j.lfs.2018.07.027
Jiang, S., Fan, J., Wang, Q., Ju, D., Feng, M., Li, J., et al. (2016). Diosgenin induces ROS-dependent autophagy and cytotoxicity via mTOR signaling pathway in chronic myeloid leukemia cells. Phytomedicine 23 (3), 243–252. doi:10.1016/j.phymed.2016.01.010
Jiaqin, C., Xiaoxia, W., Xuhui, H., Jie, Z., and Hong, S. (2018). Oxymatrine inhibits invasion and metastasis of triple negative breast cancer cells by regulating epithelial-mesenchymal transformation. Chin. J. Clin. Pharmacol. Ther. 23 (01), 13–17.
Jin, Y., Yang, Q., Liang, L., Ding, L., Liang, Y., Zhang, D., et al. (2018). Compound kushen injection suppresses human acute myeloid leukaemia by regulating the Prdxs/ROS/Trx1 signalling pathway. J. Exp. Clin. Cancer Res. 37 (1), 277. doi:10.1186/s13046-018-0948-3
Jingyuan, W., Bowen, X., Jie, L., Weilu, C., Taicheng, L., and Luchang, C. (2021). [Exploring the Molecular Biological Mechanism of Shugan Jianpi Decoction in the Treatment of Depression-related Breast Cancer Based Treatment of Depression-related Breast Cancer Based]. Hainan: Journal of Hainan Medical University, 1–16. doi:10.13210/j.cnki.jhmu.20210315.001
Juan, T., Weihao, W., Huimin, G., and Zhimin, W. (2007). Determination of matrine, sophoridine and oxymatrine in compound Sophora flavescens injection by HPLC. China J. Chin. Materia Med. (03), 222–224.
Kalantari-Dehaghi, M., Parnell, E. A., Armand, T., Bernard, H. U., and Grando, S. A. (2015). The nicotinic acetylcholine receptor-mediated reciprocal effects of the tobacco nitrosamine NNK and SLURP-1 on human mammary epithelial cells. Int. Immunopharmacol. 29 (1), 99–104. doi:10.1016/j.intimp.2015.04.041
Keiser, M. J., Roth, B. L., Armbruster, B. N., Ernsberger, P., Irwin, J. J., and Shoichet, B. K. (2007). Relating protein pharmacology by ligand chemistry. Nat. Biotechnol. 25 (2), 197–206. doi:10.1038/nbt1284
Kirn, V., Strake, L., Thangarajah, F., Richters, L., Eischeid, H., Koitzsch, U., et al. (2018). ESR1-promoter-methylation status in primary breast cancer and its corresponding metastases. Clin. Exp. Metastasis 35 (7), 707–712. doi:10.1007/s10585-018-9935-5
Kwon, M., Jang, M., Kim, G. H., Oh, T., Ryoo, I. J., Ryu, H. W., et al. (2020). Kushenol E inhibits autophagy and impairs lysosomal positioning via VCP/p97 inhibition. Biochem. Pharmacol. 175, 113861. doi:10.1016/j.bcp.2020.113861
Kwon, M., Ko, S. K., Jang, M., Kim, G. H., Ryoo, I. J., Son, S., et al. (2019). Inhibitory effects of flavonoids isolated from Sophora flavescens on indoleamine 2,3-dioxygenase 1 activity. J. Enzyme Inhib. Med. Chem. 34 (1), 1481–1488. doi:10.1080/14756366.2019.1640218
Lee, C. H., Huang, C. S., Chen, C. S., Tu, S. H., Wang, Y. J., Chang, Y. J., et al. (2010). Overexpression and activation of the alpha9-nicotinic receptor during tumorigenesis in human breast epithelial cells. J. Natl. Cancer Inst. 102 (17), 1322–1335. doi:10.1093/jnci/djq300
Lemon, H. M., Wotiz, H. H., Parsons, L., and Mozden, P. J. (1966). Reduced estriol excretion in patients with breast cancer prior to endocrine therapy. JAMA 196 (13), 1128–1136. doi:10.1001/jama.1966.03100260066020
Li, H., Li, X., Bai, M., Suo, Y., Zhang, G., and Cao, X. (2015). Matrine inhibited proliferation and increased apoptosis in human breast cancer MCF-7 cells via upregulation of Bax and downregulation of Bcl-2. Int. J. Clin. Exp. Pathol. 8 (11), 14793–14799.
Li, J., Hu, L., Zhou, T., Gong, X., Jiang, R., Li, H., et al. (2019). Taxifolin inhibits breast cancer cells proliferation, migration and invasion by promoting mesenchymal to epithelial transition via β-catenin signaling. Life Sci. 232, 116617. doi:10.1016/j.lfs.2019.116617
Liang, G., Nie, Y., Chang, Y., Zeng, S., Liang, C., Zheng, X., et al. (2019). Protective effects of Rhizoma smilacis glabrae extracts on potassium oxonate- and monosodium urate-induced hyperuricemia and gout in mice. Phytomedicine 59, 152772. doi:10.1016/j.phymed.2018.11.032
Liu, C., Kang, Y., Zhou, X., Yang, Z., Gu, J., and Han, C. (2017). Rhizoma smilacis glabrae protects rats with gentamicin-induced kidney injury from oxidative stress-induced apoptosis by inhibiting caspase-3 activation. J. Ethnopharmacol. 198, 122–130. doi:10.1016/j.jep.2016.12.034
Liu, R., Peng, J., Wang, H., Li, L., Wen, X., Tan, Y., et al. (2018). Oxysophocarpine Retards the Growth and Metastasis of Oral Squamous Cell Carcinoma by Targeting the Nrf2/HO-1 Axis. Cel. Physiol. Biochem. 49 (5), 1717–1733. doi:10.1159/000493615
Liu, S., Hu, X., Fan, X., Jin, R., Yang, W., Geng, Y., et al. (2020). A Bioinformatics Research on Novel Mechanism of Compound Kushen Injection for Treating Breast Cancer by Network Pharmacology and Molecular Docking Verification. Evid.-based Compl. Alt. 2020, 1–14. doi:10.1155/2020/2758640
Liu, X., Vogt, I., Haque, T., and Campillos, M. (2013). HitPick: a web server for hit identification and target prediction of chemical screenings. Bioinformatics 29 (15), 1910–1912. doi:10.1093/bioinformatics/btt303
Liu, X., Zhao, W., Wang, W., Lin, S., and Yang, L. (2017). Puerarin suppresses LPS-induced breast cancer cell migration, invasion and adhesion by blockage NF-Κb and Erk pathway. Biomed. Pharmacother. 92, 429–436. doi:10.1016/j.biopha.2017.05.102
Mei, Y., Yang, J. P., Lang, Y. H., Peng, L. X., Yang, M. M., Liu, Q., et al. (2018). Global expression profiling and pathway analysis of mouse mammary tumor reveals strain and stage specific dysregulated pathways in breast cancer progression. Cell Cycle 17 (8), 963–973. doi:10.1080/15384101.2018.1442629
Meng, G., Tang, X., Yang, Z., Benesch, M. G. K., Marshall, A., Murray, D., et al. (2017). Implications for breast cancer treatment from increased autotaxin production in adipose tissue after radiotherapy. FASEB J. 31 (9), 4064–4077. doi:10.1096/fj.201700159R
Merghoub, N., Benbacer, L., El Btaouri, H., Ait Benhassou, H., Terryn, C., Attaleb, M., et al. (2011). In vitro antiproliferative effect and induction of apoptosis by Retama monosperma L. extract in human cervical cancer cells. Cel Mol Biol (Noisy-le-grand) 57 Suppl (Suppl. l), OL1581–91. doi:10.5772/30025
Miller, K. D., Fidler-Benaoudia, M., Keegan, T. H., Hipp, H. S., Jemal, A., and Siegel, R. L. (2020). Cancer statistics for adolescents and young adults, 2020. CA Cancer J. Clin. 70 (1), 443–459. doi:10.3322/caac.2159010.3322/caac.21637
Monteith, G. R., Mcandrew, D., Faddy, H. M., and Roberts-Thomson, S. J. (2007). Calcium and cancer: targeting Ca2+ transport. Nat. Rev. Cancer 7 (7), 519–530. doi:10.1038/nrc2171
Nersisyan, L., Samsonyan, R., and Arakelyan, A. (2014). CyKEGGParser: tailoring KEGG pathways to fit into systems biology analysis workflows. F1000Res 3, 145. doi:10.12688/f1000research.4410.210.12688/f1000research.4410.1
Nishioka, T., Kim, H. S., Luo, L. Y., Huang, Y., Guo, J., and Chen, C. Y. (2011). Sensitization of epithelial growth factor receptors by nicotine exposure to promote breast cancer cell growth. Breast Cancer Res. 13 (6), R113. doi:10.1186/bcr3055
Nourmohammadi, S., Aung, T. N., Cui, J., Pei, J. V., De Ieso, M. L., Harata-Lee, Y., et al. (2019). Effect of Compound Kushen Injection, a Natural Compound Mixture, and its Identified Chemical Components on Migration and Invasion of Colon, Brain, and Breast Cancer Cell Lines. Front. Oncol. 9, 314. doi:10.3389/fonc.2019.00314
O'Boyle, N. M., Banck, M., James, C. A., Morley, C., Vandermeersch, T., and Hutchison, G. R. (2011). Open Babel: An open chemical toolbox. J. Cheminform 3 (1), 33–14. doi:10.1186/1758-2946-3-33
Otte, M., Zafrakas, M., Riethdorf, L., Pichlmeier, U., Löning, T., Jänicke, F., et al. (2001). MAGE-A gene expression pattern in primary breast cancer. Cancer Res. 61 (18), 6682–6687.
Qi, L., Zhang, J., and Zhang, Z. (2013). Determination of four alkaloids in Compound Kushen Injection by high performance liquid chromatography with ionic liquid as mobile phase additive. Se Pu 31 (03), 249–253. doi:10.3724/sp.j.1123.2012.10039
Qu, Z., Cui, J., Harata-Lee, Y., Aung, T. N., Feng, Q., Raison, J. M., et al. (2016). Identification of candidate anti-cancer molecular mechanisms of Compound Kushen Injection using functional genomics. Oncotarget 7 (40), 66003–66019. doi:10.18632/oncotarget.11788
Recht, A. (2017). Radiation-Induced Heart Disease after Breast Cancer Treatment: How Big a Problem, and How Much Can-and Should-We Try to Reduce it. J. Clin. Oncol. 35 (11), 1146–1148. doi:10.1200/JCO.2016.71.4113
Ren, M. X., Deng, X. H., Ai, F., Yuan, G. Y., and Song, H. Y. (2015). Effect of quercetin on the proliferation of the human ovarian cancer cell line SKOV-3 in vitro. Exp. Ther. Med. 10 (2), 579–583. doi:10.3892/etm.2015.2536
Restrepo-Angulo, I., Bañuelos, C., and Camacho, J. (2020). Ion Channel Regulation by Sex Steroid Hormones and Vitamin D in Cancer: A Potential Opportunity for Cancer Diagnosis and Therapy. Front. Pharmacol. 11, 152. doi:10.3389/fphar.2020.00152
Ritchie, M. E., Phipson, B., Wu, D., Hu, Y., Law, C. W., Shi, W., et al. (2015). limma powers differential expression analyses for RNA-sequencing and microarray studies. Nucleic Acids Res. 43 (7), e47. doi:10.1093/nar/gkv007
Rohacs, T. (2005). Teaching resources. TRP channels. Sci. STKE 2005 (282), tr14. doi:10.1126/stke.2822005tr14
Rojas-Aguirre, Y., and Medina-Franco, J. L. (2014). Analysis of structure-Caco-2 permeability relationships using a property landscape approach. Mol. Divers. 18 (3), 599–610. doi:10.1007/s11030-014-9514-x
Romero-Hernández, L. L., Merino-Montiel, P., Montiel-Smith, S., Meza-Reyes, S., Vega-Báez, J. L., Abasolo, I., et al. (2015). Diosgenin-based thio(seleno)ureas and triazolyl glycoconjugates as hybrid drugs. Antioxidant and antiproliferative profile. Eur. J. Med. Chem. 99, 67–81. doi:10.1016/j.ejmech.2015.05.018
Ru, J., Li, P., Wang, J., Zhou, W., Li, B., Huang, C., et al. (2014). TCMSP: a database of systems pharmacology for drug discovery from herbal medicines. J. Cheminform 6 (1), 13–16. doi:10.1186/1758-2946-6-13
Sanner, M. F. (1999). Python: a programming language for software integration and development. J. Mol. Graph. Model. 17 (1), 57–61.
Sekino, Y., Han, X., Babasaki, T., Goto, K., Inoue, S., Hayashi, T., et al. (2020). Microtubule-associated protein tau (MAPT) promotes bicalutamide resistance and is associated with survival in prostate cancer. Urol. Oncol. Semin. Original Invest. 38, e1–795. doi:10.1016/j.urolonc.2020.04.032
Sergeev, I. N. (2005). Calcium signaling in cancer and vitamin D. J. Steroid Biochem. Mol. Biol. 97 (1-2), 145–151. doi:10.1016/j.jsbmb.2005.06.007
Serna-Marquez, N., Diaz-Aragon, R., Reyes-Uribe, E., Cortes-Reynosa, P., and Salazar, E. P. (2017). Linoleic acid induces migration and invasion through FFAR4- and PI3K-/Akt-dependent pathway in MDA-MB-231 breast cancer cells. Med. Oncol. 34 (6), 111. doi:10.1007/s12032-017-0969-3
Shannon, P., Markiel, A., Ozier, O., Baliga, N. S., Wang, J. T., Ramage, D., et al. (2003). Cytoscape: a software environment for integrated models of biomolecular interaction networks. Genome Res. 13 (11), 2498–2504. doi:10.1101/gr.1239303
Singh, M., Alavi, A., Wong, R., and Akita, S. (2016). Radiodermatitis: A Review of Our Current Understanding. Am. J. Clin. Dermatol. 17 (3), 277–292. doi:10.1007/s40257-016-0186-4
Sterling, T., and Irwin, J. J. (2015). ZINC 15--Ligand Discovery for Everyone. J. Chem. Inf. Model. 55 (11), 2324–2337. doi:10.1021/acs.jcim.5b00559
Straub, J. M., New, J., Hamilton, C. D., Lominska, C., Shnayder, Y., and Thomas, S. M. (2015). Radiation-induced fibrosis: mechanisms and implications for therapy. J. Cancer Res. Clin. Oncol. 141 (11), 1985–1994. doi:10.1007/s00432-015-1974-6
Tao, W., Xu, X., Wang, X., Li, B., Wang, Y., Li, Y., et al. (2013). Network pharmacology-based prediction of the active ingredients and potential targets of Chinese herbal Radix Curcumae formula for application to cardiovascular disease. J. Ethnopharmacol. 145 (1), 1–10. doi:10.1016/j.jep.2012.09.051
Tong, W. G., Ding, X. Z., and Adrian, T. E. (2002). The mechanisms of lipoxygenase inhibitor-induced apoptosis in human breast cancer cells. Biochem. Biophys. Res. Commun. 296 (4), 942–948. doi:10.1016/S0006-291X(02)02014-4
Trott, O., and Olson, A. J. (2009). AutoDock Vina: Improving the speed and accuracy of docking with a new scoring function, efficient optimization, and multithreading. J. Comput. Chem., NA. doi:10.1002/jcc.21334
Vaidya, J. S., Massarut, S., Vaidya, H. J., Alexander, E. C., Richards, T., Caris, J. A., et al. (2018). Rethinking neoadjuvant chemotherapy for breast cancer. BMJ 360, j5913. doi:10.1136/bmj.j5913
Vangeel, L., and Voets, T. (2019). Transient Receptor Potential Channels and Calcium Signaling. Cold Spring Harb Perspect. Biol. 11 (6). doi:10.1101/cshperspect.a035048
Venkatachalam, K., and Montell, C. (2007). TRP channels. Annu. Rev. Biochem. 76, 387–417. doi:10.1146/annurev.biochem.75.103004.142819
Wang, H., Hu, H., Rong, H., and Zhao, X. (2019). Effects of compound Kushen injection on pathology and angiogenesis of tumor tissues. Oncol. Lett. 17 (2), 2278–2282. doi:10.3892/ol.2018.9861
Wang, L. H., Zeng, X. A., Wang, M. S., Brennan, C. S., and Gong, D. (2018). Modification of membrane properties and fatty acids biosynthesis-related genes in Escherichia coli and Staphylococcus aureus: Implications for the antibacterial mechanism of naringenin. Biochim. Biophys. Acta Biomembr 1860 (2), 481–490. doi:10.1016/j.bbamem.2017.11.007
Wang, W., You, R. L., Qin, W. J., Hai, L. N., Fang, M. J., Huang, G. H., et al. (2015). Anti-tumor activities of active ingredients in Compound Kushen Injection. Acta Pharmacol. Sin. 36 (6), 676–679. doi:10.1038/aps.2015.24
Wang, Y. Y., Attané, C., Milhas, D., Dirat, B., Dauvillier, S., Guerard, A., et al. (2017). Mammary adipocytes stimulate breast cancer invasion through metabolic remodeling of tumor cells. JCI Insight 2 (4), e87489. doi:10.1172/jci.insight.87489
Warnes, G. R., Bolker, B., Bonebakker, L., Gentleman, R., Huber, W., Liaw, A., et al. (2020). gplots: Various R Programming Tools for Plotting Data. R package version 3.
Weingarten, M. D., Lockwood, A. H., Hwo, S. Y., and Kirschner, M. W. (1975). A protein factor essential for microtubule assembly. Proc. Natl. Acad. Sci. U S A. 72 (5), 1858–1862. doi:10.1073/pnas.72.5.1858
Wu, K., Fukuda, K., Xing, F., Zhang, Y., Sharma, S., Liu, Y., et al. (2015). Roles of the Cyclooxygenase 2 Matrix Metalloproteinase 1 Pathway in Brain Metastasis of Breast Cancer. J. Biol. Chem. 290 (15), 9842–9854. doi:10.1074/jbc.M114.602185
Xiao, N. (2018). ggsci: Scientific Journal and Sci-Fi Themed Color Palettes for 'ggplot2'. R package version 2.9.
Xiumei, Z., and Cen, S. (2004). Simultaneous determination of three Alkaloids in Compound Kushen Injection by HPLC. China J. Chin. Materia Med. (07), 99–100.
Xu, W., Lin, H., Zhang, Y., Chen, X., Hua, B., Hou, W., et al. (2011a). Compound Kushen Injection suppresses human breast cancer stem-like cells by down-regulating the canonical Wnt/β-catenin pathway. J. Exp. Clin. Cancer Res. 30, 103. doi:10.1186/1756-9966-30-103
Xu, W., Lin, H., Zhang, Y., Chen, X., Hua, B., Hou, W., et al. (2011b). Compound Kushen Injection suppresses human breast cancer stem-like cells by down-regulating the canonical Wnt/β-catenin pathway. J. Exp. Clin. Cancer Res. 30, 103. doi:10.1186/1756-9966-30-103
Yang, Y., Sun, M., Li, W., Liu, C., Jiang, Z., Gu, P., et al. (2021). Rebalancing TGF-β/Smad7 signaling via Compound kushen injection in hepatic stellate cells protects against liver fibrosis and hepatocarcinogenesis. Clin. Transl Med. 11 (7), e410. doi:10.1002/ctm2.410
Yao, L. T., Wang, M. Z., Wang, M. S., Yu, X. T., Guo, J. Y., Sun, T., et al. (2019). Neoadjuvant endocrine therapy: A potential strategy for ER-positive breast cancer. World J. Clin. Cases 7 (15), 1937–1953. doi:10.12998/wjcc.v7.i15.1937
Yao, M., and Fu, P. (2018). Advances in anti-HER2 therapy in metastatic breast cancer. Chin. Clin. Oncol. 7 (3), 27. doi:10.21037/cco.2018.05.04
Yarla, N. S., Bishayee, A., Sethi, G., Reddanna, P., Kalle, A. M., Dhananjaya, B. L., et al. (2016). Targeting arachidonic acid pathway by natural products for cancer prevention and therapy. Semin. Cancer Biol. 40-41, 48–81. doi:10.1016/j.semcancer.2016.02.001
Yu, G., Wang, L. G., Han, Y., and He, Q. Y. (2012). clusterProfiler: an R Package for Comparing Biological Themes Among Gene Clusters. OMICS 16 (5), 284–287. doi:10.1089/omi.2011.0118
Yuan, T. L., and Cantley, L. C. (2008). PI3K pathway alterations in cancer: variations on a theme. Oncogene 27 (41), 5497–5510. doi:10.1038/onc.2008.245
Zhang, Y. (2017). Ganoderma lucidum (Reishi) suppresses proliferation and migration of breast cancer cells via inhibiting Wnt/β-catenin signaling. Biochem. Biophys. Res. Commun. 488 (4), 679–684. doi:10.1016/j.bbrc.2017.04.086
Zheng, Y., Zhong, G., Yu, K., Lei, K., and Yang, Q. (2020). Individualized Prediction of Survival Benefit from Locoregional Surgical Treatment for Patients with Metastatic Breast Cancer. Front. Oncol. 10, 148. doi:10.3389/fonc.2020.00148
Keywords: breast cancer, compound kushen injection, traditional Chinese medicine, molecular docking, synergistic mechanism, system pharmacology
Citation: Li Y, Wang K, Chen Y, Cai J, Qin X, Lu A, Guan D, Qin G and Chen W (2021) A System Pharmacology Model for Decoding the Synergistic Mechanisms of Compound Kushen Injection in Treating Breast Cancer. Front. Pharmacol. 12:723147. doi: 10.3389/fphar.2021.723147
Received: 10 June 2021; Accepted: 15 October 2021;
Published: 16 November 2021.
Edited by:
Haiyang Yu, Tianjin University of Traditional Chinese Medicine, ChinaReviewed by:
Wei Wang, Shanxi Zhendong Pharmaceutical, ChinaTara Louise Pukala, University of Adelaide, Australia
Copyright © 2021 Li, Wang, Chen, Cai, Qin, Lu, Guan, Qin and Chen. This is an open-access article distributed under the terms of the Creative Commons Attribution License (CC BY). The use, distribution or reproduction in other forums is permitted, provided the original author(s) and the copyright owner(s) are credited and that the original publication in this journal is cited, in accordance with accepted academic practice. No use, distribution or reproduction is permitted which does not comply with these terms.
*Correspondence: Daogang Guan, guandg0929@hotmail.com; Genggeng Qin, zealotq@smu.edu.cn; Weiguo Chen, chen1999@smu.edu.cn
†These authors have contributed equally to this work and share first authorship