- 1Department of Dermatology, Yueyang Hospital of Integrated Traditional Chinese and Western Medicine, Shanghai University of Traditional Chinese Medicine, Shanghai, China
- 2Institute of Dermatology, Shanghai Academy of Traditional Chinese Medicine, Shanghai, China
- 3Shanghai Skin Disease Hospital of Tongji University, Shanghai, China
- 4Center for Translational Medicine, Huaihe Hospital of Henan University, Kaifeng, China
- 5Shanghai Applied Protein Technology Co., Ltd., Shanghai, China
Sheng-Ji Hua-Yu (SJHY) formula has been proved to reduce the severity of diabetic wound healing without significant adverse events in our previous clinical trials. However, based on multi-target characteristics, the regulatory network among herbs, ingredients, and hub genes remains to be elucidated. The current study aims to identify the biomarkers of the SJHY formula for the treatment of diabetic wound healing. First, a network of components and targets for the SJHY formula was constructed using network pharmacology. Second, the ClusterONE algorithm was used to build a modular network and identify hub genes along with kernel pathways. Third, we verified the kernel targets by molecular docking to select hub genes. In addition, the biomarkers of the SJHY formula were validated by animal experiments in a diabetic wound healing mice model. The results revealed that the SJHY formula downregulated the mRNA expression of Cxcr4, Oprd1, and Htr2a, while upregulated Adrb2, Drd, Drd4, and Hrh1. Besides, the SJHY formula upregulated the kernel pathways, neuroactive ligand–receptor interaction, and cAMP signaling pathway in the skin tissue homogenate of the diabetic wound healing mice model. In summary, this study identified the potential targets and kernel pathways, providing additional evidence for the clinical application of the SJHY formula for the treatment of diabetic wound healing.
1 Introduction
Diabetic wounds are a severe health problem, while the prevalence of diabetes worldwide and foot ulceration is approximately 3% in a community-based cohort clinical study (Zhang et al., 2017; Narres et al., 2017). It is estimated that up to 50% of patients with diabetic foot ulceration have peripheral arterial disease in high- or middle-income countries (Prompers et al., 2007; Morbach et al., 2012), whereas a higher incidence of neuropathic ulcers was observed in low-income countries (Rigato et al., 2018; Younis et al., 2018). Chronic ulcers, resulted in amputation, are among the most frequent complications corresponding to diabetes, which account for over 200 billion USD in annual in healthcare costs (den Dekker et al., 2019).
In the field of clinical treatment, platelet-derived growth factor was the only drug approved by U.S. Food and Drug Administration for the treatment of diabetic wound to date. However, availability of it was limited due to heavy cost (Papanas and Maltezos, 2007; Barrientos et al., 2008). As recommended in guidelines (Guo et al., 2020), arterial reconstruction of the lower limbs is the moose vital measure, while patients undergoing surgical revascularization are at increased risk of surgical mortality (Hinchliffe et al., 2016). Consequently, appropriate interventions targeted as diabetic wound should be taken to improve long-term clinical efficacy.
The clinical application and research of traditional Chinese medicine (TCM)–treated diabetic wound healing have been extensively investigated and broadly applied. The SJHY formula is an effective compound preparation of Chinese herbal medicine for treating diabetic wounds, which was formulated on the basis of TCM theories of “qu-fu-sheng-xin (removing necrotic tissues to stimulate the growth of new skin)” (Li et al., 2012) and “sheng-ji-hua-yu (promoting the growth of new tissues and simultaneously melting the stasis)” (Li et al., 2011). SJHY formula is summed up according to clinical practice, while consisting of Sheng-ji recipe and Hua-yu recipe (Li, 2000). Herbal formula treatment is efficient in the management of diabetic skin ulcers, and healing time is 2–3 days shorten than western medicine treatment (mupirocin ointment, growth factor (bFGF), and Vaseline gauze for external use and with basic therapies), which are consistment with our previous animal experiment reported (Li et al., 2011; Kuai et al., 2018; Xiang et al., 2021). Additionally, the SJHY formula has been validated to be efficacious to treat diabetic wound healing via higher order Markov chain set pair analysis (Kuai et al., 2020) and cloud model set pair analysis (Kuai et al., 2019). However, further investigations are required to elucidate the regulation mechanisms of SJHY formula–treated diabetic wound healing.
The effectiveness of single-target therapy is inadequate for complex diseases recently (Lord and Ashworth, 2010). Due to the characteristics of complexity of diseases with multiple targets, modular pharmacology, which employed multiple modules according to combination therapy, was developed, which has been considered to be the next paradigm in drug discovery (Wang et al., 2012). Taking into consideration topological overlap, ClusterONE can improve the performance of module detection, together with an effect in predicting functional modules (Cheng et al., 2019; Amjad et al., 2020). Hence, the approach of modular pharmacology using the ClusterONE tool has been used for research on TCM and natural medicine (Chen et al., 2016).
Molecular docking, a computational technique extensively adopted in drug discovery, which established in silico structure-based docking enables predicting bond conformations and free binding energies, and places small molecule ligands to targets predicting ligand–target interactions at a molecular level (Pinzi and Rastelli, 2019).
Based on the evidences, the current studies applied the ClusterONE algorithm for modular pharmacology to identify biomarkers of SJHY formula on the treatment of diabetic wound healing. Furthermore, we verified the targets by molecular docking and experiments in vivo (Figure 1).
2 Methods
2.1 Components and Corresponding Targets of SJHY Formula
The search was performed on TCMSP (https://www.tcmspw.com, Ru et al., 2014), the comprehensive database of TCMID (http://www.megabionet.org/tcmid/, Huang et al., 2018), and BATMAN-TCM (http://bionet.ncpsb.org/batman-tcm, Liu et al., 2016), using “Huang Qi,” “Dan Shen,” “Bai Zhi,” “Da Huang,” “Zi Cao,” “Xue Jie,” “Lu Gan Shi,” and “Zhen Zhu Fen” as keywords. Table 1 shows Latin name or English name of each herb. Information of molecular molecule IDs, molecule names, and target names for each herb has been downloaded. The gene names of targets were largely annotated on the UniProt database (https://www.uniprot.org), while subsequently normalized to gene symbol.
2.2 Acquisition Targets of Diabetic Wound Healing
Corresponding targets associated with the disease of diabetic wound healing were collected from the GeneCards database (https://www.genecards.org, Lin and Hu, 2021), Online Mendelian Inheritance in Man database (OMIM, https://www.omim.org, Amberger and Hamosh, 2017), PharmGKB database (https://www.pharmgkb.org, Barbarino et al., 2018), Therapeutic Targets Database (TTD, db.idrblab.net, Wang Y. et al., 2020), and DrugBank database (https://www.drugbank.com, Wishart et al., 2018). An intersection of targets associated with diabetic wound healing diseases and the targets of SJHY formula was constructed, as potential targets for subsequent gene annotation and an enrichment analysis.
2.3 Component–Target Network Construction
According to the pairs of herbs components and component–target, the SJHY formula network image was produced using Cytoscape software (version 3.6.0). Nodes of different colors represented core ingredients or targets, and the edges were used to describe the interaction relationship between each Chinese medicine and the ingredients along with the targets.
2.4 Gene Annotation and Function Enrichment
In the current study, the gene annotation and the function enrichment analysis were performed using Metascape (http://metascape.org) (Zhou et al., 2019), which consist of the Gene Ontology (GO) function enrichment analysis, including the biological process, cellular component, molecular function, reactome gene sets, canonical pathways, CORUM, and Kyoto Encyclopedia of Genes and Genomes (KEGG). A p-value < 0.01 and an enrichment score of >1.5 were considered to be statistically significant.
2.5 Protein–Protein Interaction Network
Protein–protein interaction (PPI) networks are used as viable tools for understanding disease mechanisms, drug discovery, or repositioning drugs (Vella et al., 2018). The STRING database (https://string-db.org/) was particularly adept at collection, scoring, and integrating all publicly available sources. The present study conducted a PPI network study based on potential targets of SJHY formula and diabetic wound healing to investigate the interactions with the highest confidence (0.900). Furthermore, the network analyzer of network topology parameters was used as a tool to calculate the average degree of freedom, and the PPI data was obtained for further modular analysis.
2.6. Modular Analysis Using ClusterONE Algorithm
The ClusterONE algorithm aims to calculate the topology graph of the PPI network (Chen et al., 2016) and identifies modules in protein interaction networks (Cheng et al., 2019). The determined clusters were considered as kernel targets, which were defined as the node size >5, p-value < 0.0001, quality >0.60, and density >0.05.
Next, genes were sorted according to the degree of freedom in each inner cluster, and we identified three markers per cluster in the modular-based approach as hub genes of SJHY formula for the treatment of diabetic wound healing. Furthermore, we constructed a target pathway network map based on kernel clusters, and selected kernel pathways according to the size of nodes. Furthermore, the kernel targets of each cluster were used for the GO network and the KEGG target network analysis.
2.7 Molecular Docking Verification
We downloaded the compound SDF structure from the PubChem database (https://pubchem.ncbi.nlm.nih.gov/, Kim et al., 2021), used Chem3D energy minimization to save it in mol2 format, and AutodockTools 1.5.6 to open the small ligand molecule and saved it as a pdbqt file. Furthermore, we selected human-derived protein with a co-crystal structure and high resolution as the protein used for docking in this subject from Uniprot (https://www.uniprot.org/, The UniProt Consortium. 2017). The three-dimensional structures of the protein were downloaded from the RCSB database (www.rcsb.org/, Burley et al., 2018). AutodockTools 1.5.6 was adopted to add all hydrogen atoms, calculated Gasteiger charge, defined as an acceptor, and saved as a pdbqt file. Autodock Vina was used for semi-flexible docking. The lowest docking energy was considered as a condition to select for the docking binding mode analysis, the force was analyzed using Discovery Studio, and Pymol was used for mapping.
2.8 Experimental Verification
2.8.1 SJHY Formula Preparation
SJHY formula consists of eight Chinese herbs (Supplementary Table S1). The herbs of SJHY formula were approved and obtained from the pharmacy department. The preparation process of SJHY formula was in accordance with our previous study (Kuai et al., 2018). The effective components of SJHY formula were extracted with 95% ethanol and mixed with carbomer. The mixture was stored at 4°C. For the LC-MS/MS analysis, the SJHY formula (50 μl) was first diluted with 40% methanol solution (950 μl, pre-cooled) and labeled as di20. Then we adulterated the di20 (50 μl) with 40% methanol solution (450 μl, pre-cooled) and stamped it as di200. All the diluted samples were centrifuged at 4°C at 16,000 rpm for 15 min, and the supernatant was transferred to the liquid vial and stored in the refrigerator at 4°C.
2.8.2 Liquid Chromatography Tandem–Mass Spectrometer (LC-MS/MS)
LC-MS/MS analysis was performed for quality control of SJHY formula utilizing Waters ACQUITY UPLC I-Class coupled with a 5500 QTRAP mass spectrometer (SCIEX) at the column temperature of 35°C. The mobile phase (A: aqueous solution containing 0.1% formic acid; B: acetonitrile solution containing 0.1% formic acid) was delivered at a speed of 0.3 ml/min: 0–3 mins (5%–25%B); 3–8.5 mins (25%–45%B); 8.5–12 mins (45%–95%B); 12–15 mins (95%–98%B); 15–15.2 mins (98%–5%B); and 15.2–18.2 mins (5%B).
2.8.3 Animals
The current study used C57BL/6 mice with the specific pathogen-free (SPF), placed at a temperature of 21–25°C for 16 h and dark for 8 h, provided by the Shanghai Medical Experimental Animal Center. The experiment was approved by the Ethics Committee of Yueyang Hospital (No.19964, Supplementary Figure S1). The mice were distributed into three groups (n = 4 for each group) randomly, as follows: control group, disease group, and SJHY group.
2.8.4 Model Establishment
A diabetic skin wound mice model was established in the current study. Animals in the disease group and SJHY group were intraperitoneally injected with 2% streptozotocin (dissolved in a citrate buffer solution). The condition of the diabetic model was blood glucose (BG) over 16.7 mmol/L. After shaving, a sterile punch was adopted to make full-thickness excisional skin wounds (Kuai et al., 2018; Xiang et al., 2021). The intervention measures of the disease group and the control group were vehicle ointment, while SJHY ointment was applied to the SJHY group. The mice were executed and skin tissues were taken on day 9 after punching.
2.8.5 qPCR
Total RNA was isolated from mouse skin tissues with a Trizol reagent (Invitrogen, USA). Real-time PCR was performed by the FastStart Universal SYBR Green Master (Rox) (Roche). Non-specific amplifications have been monitored using melting curves. The relative expression level was calculated according to the 2-ΔΔCt method. PCR primer sequences are displayed in Supplementary Table S2.
2.8.6 ELISA
ELISA was performed using mice skin tissue. The conditions include incubation at 4°C and centrifugation for 5 min. cAMP mouse ELISA kit (CSB-E15061m, CUSABIO) and AGTR1 mouse ELISA kit (LS-F18244, LSBio) were used to monitor cAMP and AGTR1 protein content.
2.8.7 Statistical Methods
Data involved in experimental validation was analyzed with GraphPad Prism 8. The T test was produced to compare among the groups, and a p-value of less than 0.05 was used as the condition for statistically significant difference.
3 Results
3.1 Identification of SJHY Formula and Disease Targets
To obtain compound disease common targets, we retrieved on the TCMSP, TCMID, BATMAN-TCM, GeneCards, OMIM, PharmGKB, TTD, and DrugBank databases. 529 components were obtained, and 659 SJHY formula corresponding targets of these components were retrieved after screening on the TCMSP database (Supplementary Table S3). 5,028 genes associated with diabetic wound healing were collected from GeneCards, OMIM, PharmGKB, TTD, and DrugBank databases. After the restriction of conditions (by intersecting the targets associated with diabetic wound healing diseases and the targets of SJHY formula), 328 targets were identified (Figures 2A,B).
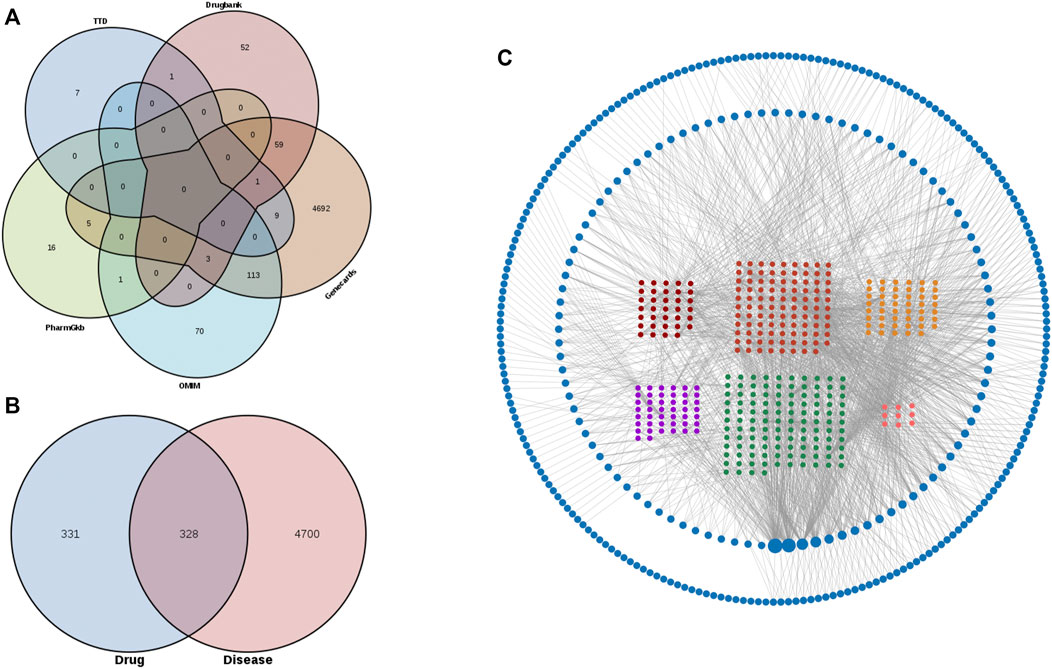
FIGURE 2. Potential targets and the enrichment analysis of diabetic wound healing and SJHY formula identification. (A) Venn graph of potential targets of diabetic wound healing using five databases. (B) Venn graph of the intersection of diabetic wound healing and SJHY formula potential targets. (C) A network of components and targets construction.
3.2 Component–Target Network Construction
To clarify how the SJHY formula may act against diabetic wound healing, we used Cytoscape software to build an ingredient–target network (Figure 2C; Supplementary Table S4). A node represented each protein, and the node’s size was proportional to the node’s degree. The edges denoted that nodes could interact with each other. Here, 328 targets after intersection were included in the compound–target interaction network.
3.3 Gene Annotation and Enrichment Analysis
To illuminate potential functions of these potential targets (328 target genes after intersection), gene annotation and a function enrichment analysis has been performed. The top twenty representative terms were displayed (Figure 3A). Furthermore, a network based on subsets was constructed, with a condition of similarity >0.3 (Figures 3B,C). Specifically, the genes enriched in SJHY formula for the treatment of diabetic wound healing were associated with the neuroactive ligand–receptor interaction and the cAMP signaling pathway, along with the pathway in cancer.
3.4 PPI and Kernel Cluster Network Construction
In order to further investigate the hub genes, a new modular network was established according to the ClusterONE algorithm for determining the kernel therapeutic targets (Figures 4A,B). Based on the conditions, a total of three clusters were selected for a subsequent analysis (Table 2). Following the filter condition of each cluster, CXCR4, DRD4, and OPRD1could be considered as kernel targets. ADRB2 reached the highest degree of freedom in Cluster 2, followed by HRH1, HTR2A, and ADRA1A. Except for ADRB2 and DRD1, Cluster 3 contained ADRB3. Taken together, these results suggested that those kernel targets could be considered as the kernel targets of SJHY formula–treated diabetic wound healing (Table 3).
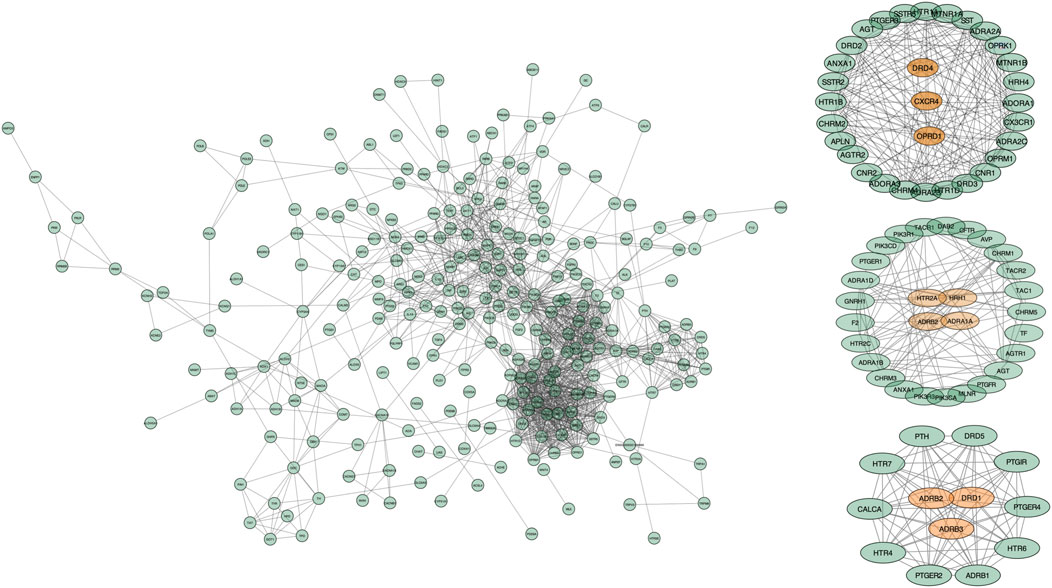
FIGURE 4. Modular pharmacology and the kernel cluster analysis based on the ClusterONE algorithm. The points represent genes, and the edges represent protein–protein interaction. The points in orange color represent the kernel genes, and the green points represent the genes that interacted with kernel genes.
Further analysis of the GO network was conducted on Cytoscape software, and the data revealed the main outcomes of GO characterization: vitamin B6 binding, response to alkaloid, and G protein–coupled amine receptor activity (Figure 5). To further investigate the regulatory relationship between the targets, we have drawn a pathway–target network analysis using screening conditions: BC > Avg (BC), CC > Avg (CC), and De > Avg (De). As Figure 6, it manifested that the neuroactive ligand–receptor interaction (Supplementary Figure S2) and the cAMP signaling pathway (Supplementary Figure S3) could be considered as the kernel pathways of the SJHY formula–treated diabetic wound healing.
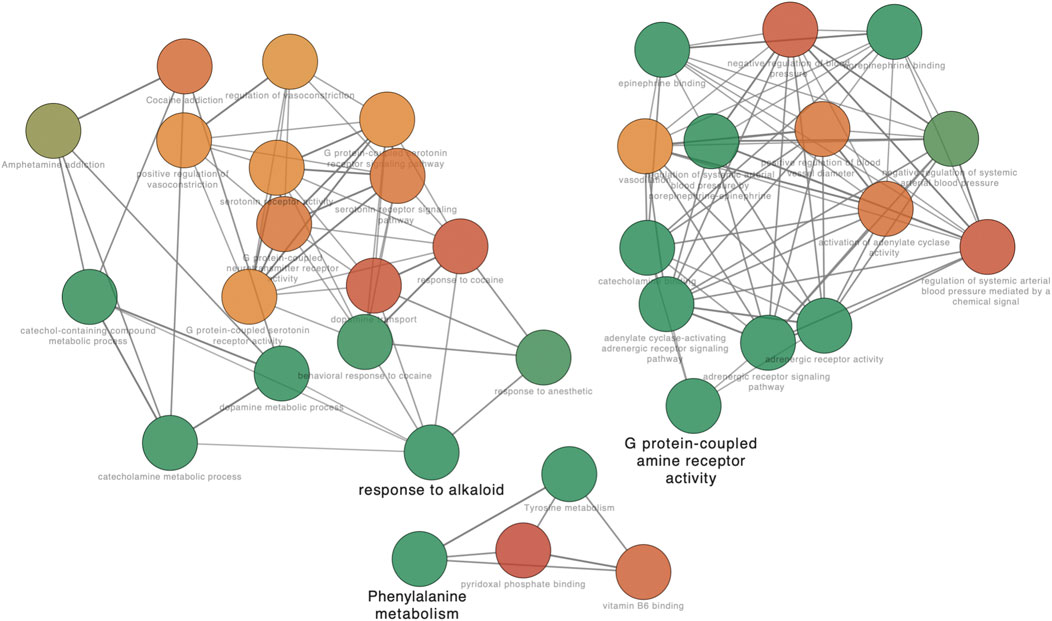
FIGURE 5. GO network analysis based on kernel targets. The nodes in different colors represent different enrichment degree. Red color means high enriched GO terms, and green color means low enrichment degree.
3.5 Molecular Docking
Molecular docking was adopted for predicting bond conformations and free binding energies in the current study. The relevant results in binding energy (BE) of the small molecule and macromolecule were considered as an evaluation index. BE values less than −7 kcal/mol were considered to represent significant binding affinity, while BE < −8.5 kcal/mol were chosen for molecular docking. The results of the binding energy computations can be obtained from Table 4, while the correlations analysis with bivariate correlations can be obtained from Figure 7. Based on the evidences from molecular docking, we considered that the structure of CXCR4–Astragaloside (BE = −8.8 kcal/mol; Figure 8A), HRH1–Lobelanidine (BE = −8.7 kcal/mol; Figure 8B), HTR2A–Dauricine (BE = −9.8 kcal/mol; Figure 8C), and OPRD1–Lobelanidine (BE = −9.4 kcal/mol; Figure 8D) were most stable, while DRD1, DRD4, and ADRB2 were also proposed as kernel targets of SJHY formula–treated diabetic wound healing.
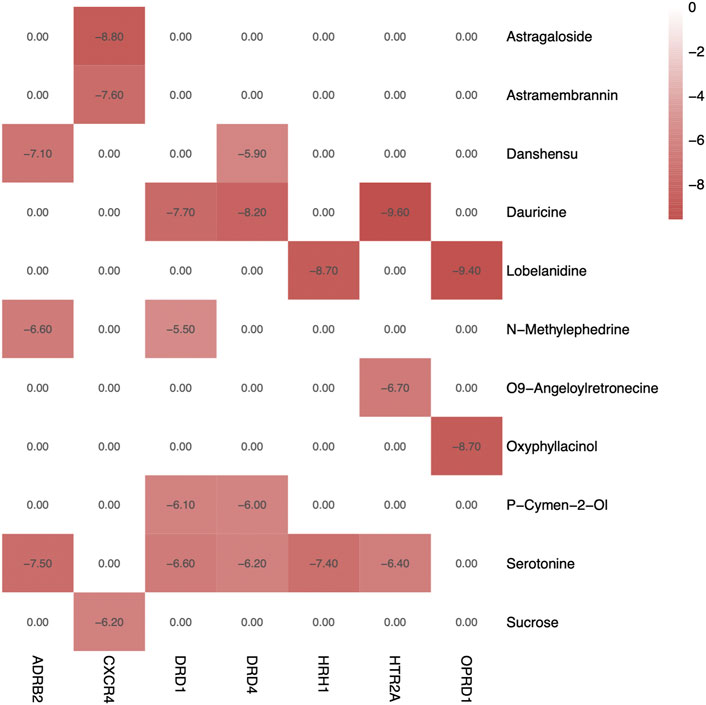
FIGURE 7. Heatmap of binding energy of compounds and targets. Color of red represents high BE values, and white and pink colors mean a low value of binding affinity.
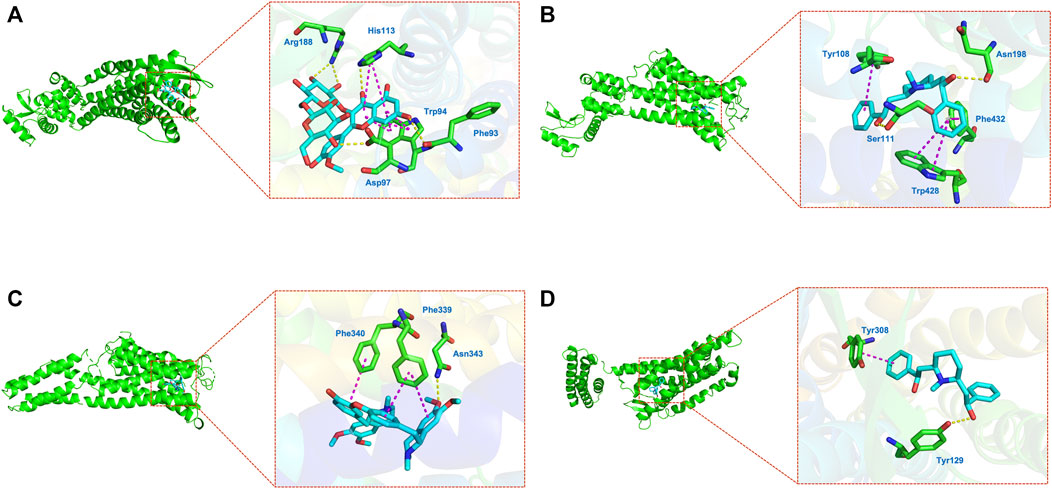
FIGURE 8. Molecular docking on hub genes. (A) CXCR4–astragaloside; (B, HRH1–lobelanidine; (C) HTR2A–dauricine; (D) OPRD1–lobelanidine.
3.6 Experimental Verification
3.6.1 Quantitative Analysis of SJHY Formula by LC-MS/MS
LC-MS/MS analysis was performed to establish the quality control of SJHY formula by identifying and quantifying ingredients involving loureirin A, loureirin B, paeonol, formononetin, and liquiritin. The structure of the aforementioned compounds is displayed in Figure 9A, and the general ion flow chromatographic of SJHY formula is shown in Figure 9B. The content of loureirin A, loureirin B, paeonol, formononetin, and liquiritin in the SJHY formula was 113.41 ug/ml, 129.39 ug/ml, 21.82 ug/ml, 13.99 ug/ml, and 5.00 ug/ml, respectively (Table 5).
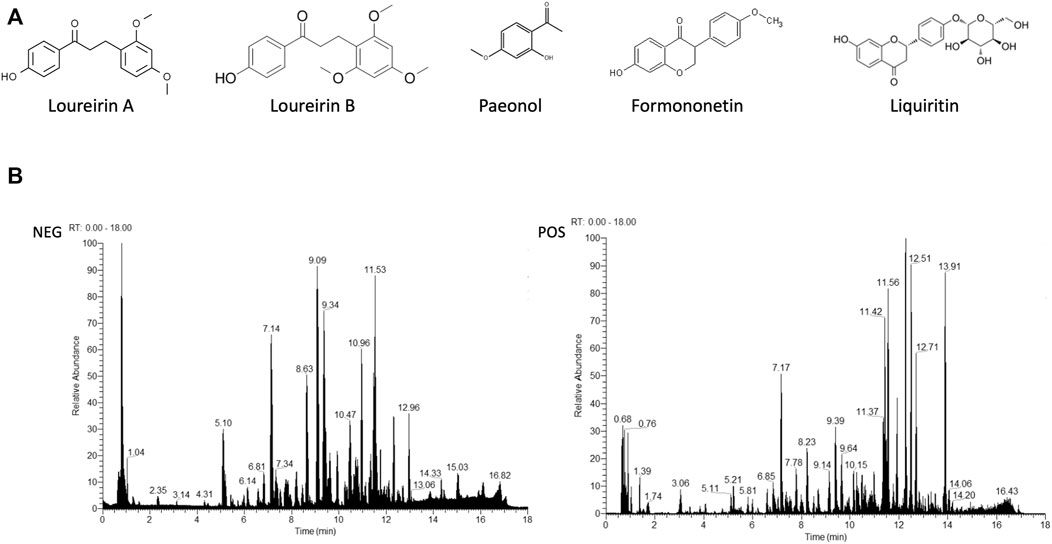
FIGURE 9. Analysis of components of SJHY formula. (A) Chemical structure of loureirin A, loureirin B, paeonol, formononetin, and liquiritin, respectively; (B) Total ion chromatogram of SJHY formula separated by a positive and negative ion mode.
3.6.2 SJHY Formula Regulated mRNA Expression of Kernel Genes
To verify the kernel network, the mRNA levels of the target genes was quantified by real-time PCR, and all seven genes reached statistical significance. The results of qPCR showed that the SJHY formula downregulated Cxcr4, Oprd1, and Htr2a with statistical significance, on comparison with the disease group. At the same time, the mRNA expression levels of Adrb2, Drd1, Drd4, and Hrh1 were significantly increased. Together these results provided important insights that the mRNA expression level of hub genes might help evaluate the efficacy of SJHY formula for the treatment of diabetic wound healing (Figure 10A).
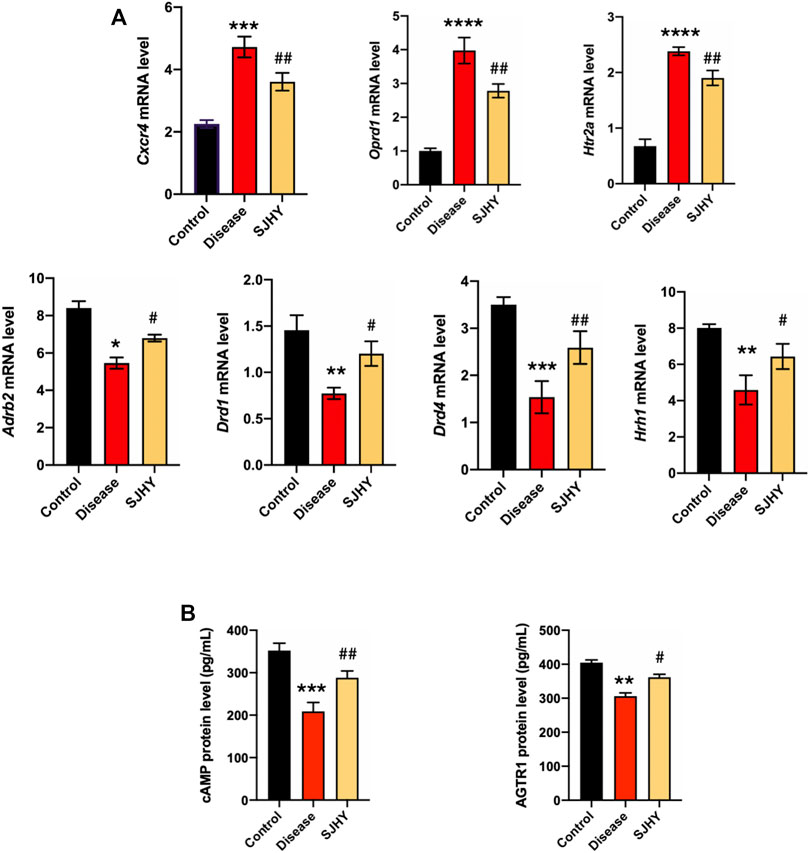
FIGURE 10. Experiment validation of hub genes and kernel pathways on day 9 after punching. (A) Detection of expression of hub genes using qPCR; (B) Validation of kernel pathways using ELISA. #p < 0.05, ##p < 0.01, compared with the control group. *p < 0.05, **p < 0.01, ***p < 0.001, **** p < 0.0001 compared with the disease group.
3.6.3 SJHY Formula Increased Protein Expressed Levels of Kernel Pathway–Related Genes in Mice
The ELISA was adopted to evaluate the expression of cAMP and AGTR1, neuroactive ligand–receptor interaction, and cAMP signaling pathway–related genes in the tissue homogenate sample after SJHY formula treatment, and these proteins showed that the expression of cAMP and AGTR1 were increased with statistically significance (Figure 10B).
4 Discussion
Wound healing is a major complication of diabetes which usually leads to limb loss or disability (den Dekker et al., 2019). Currently, the Food and Drug Administration (FDA) declared for the clinical use of a platelet-derived growth factor, which was the only drug for the treatment of diabetic wound. Additionally, alternative therapies such as SJHY formula, could improve clinical response rate and shorten the clinical course by 2–3 days compared with Western medicine treatment, which are increasingly getting clinical applications worldwide. The therapeutic effect of TCM on diabetic wound healing is mainly through the interaction between multiple molecules.
We have previously demonstrated the therapeutic effects of SJHY formula on diabetic wound healing (Li et al., 2011; Kuai et al., 2018). Notably, the LC-MS/MS analysis was utilized to identify and quantify the compounds of SJHY formula, involving loureirin A, loureirin B, paeonol, formononetin, and liquiritin (Figure 9; Table 5). Loureirin A play an active role in wound healing by promoting hair follicle stem cells to repair skin epidermis (Li et al., 2018). Interestingly, loureirin B may downregulate the mRNA and protein levels of several fibrosis-related molecules and suppress the proliferation of hypertrophic scar (HS) fibroblasts, thus reducing scar formation (Bai et al., 2015). Paeonol, a compound that possesses anti-inflammatory, analgesic, antioxidant, and anti-allergic properties, has been proved to treat inflammation-related diseases, allergies, and cancer (Liu et al., 2014). Furthermore, formononetin inhibits NO production, inflammatory infiltration, and improve blood microcirculation, resulting in accelerated wound healing (Huh et al., 2011; Lai et al., 2013). As for liquiritin, it has been applied to treat against skin inflammation, various cancers, and digestive disorders by reducing inflammation, oxidative stress, apoptosis, and metabolic alterations (Xiong et al., 2021; Han et al., 2019). Taken together, these evidences may explain the clinical phenomenon that SJHY formula ameliorates retarded wound healing of diabetes and simultaneously reduces scar formation.
In the present study, the ClusterONE algorithm and molecular docking were applied to the compound prescription of TCM-treated diabetic wound healing. According to the networks, 529 components of SJHY formula were selected by the TCMSP database. Furthermore, we obtained 659 corresponding targets. Moreover, disease-related targets were determined using the five databases. After the intersection of drug targets and disease targets, 328 targets were obtained. As the modular network reveals and molecular docking experiment validates, we inferred that the following proteins could be considered as hub genes of SJHY formula: OPRD1, CXCR4, HTR2A, HRH1, DRD1, DRD4, and ADRB2.
The diabetic wounds have multiple factors, broadly divided into three categories: neuropathy, chronic inflammation, and angiopathy (Moura et al., 2019). Peripheral neuropathy is routinely used in risk assessment, presently in roughly half of non-healing wound cases and neurological evaluation (Moura et al., 2019). The present study indicated that SJHY formula might regulate multiple neurotransmitters levels to treat diabetic wound healing. OPRD1, a target of many regulators to modulate its neurotransmission for the role in neurosecretion, was significantly upregulated following SJHY formula. These results were in keeping with previous animal experiment studies, which indicated that OPRD1 was significantly regulated by nerve damage in the ganglion, and upregulated on the 4th day after the injury (Korczeniewska et al., 2020). Besides the decreased pain sensation, neuropathy also decreases neuropeptide expression (da Silva et al., 2010), which could regulating inflammation in the process of wound healing (Curtis and Radek, 2012).
Chronic inflammation is considered as a center link in the progression of diabetic wound healing disease (Moura et al., 2019). C-X-C motif chemokine receptor 4 (CXCR4), thought to serve a critical role in the regulation of inflammation and cancer biology, has gained a lot of attention in recent years (Kircher et al., 2018). The expression of CXCR4 was upregulated in the blood vessels, macrophages, and skin tissue of K14-VEGF-A induced mice (Zgraggen et al., 2014), and indicated the pivotal role in early inflammation (Konrad et al., 2017). CXCR4 was identified as a hub gene of SJHY formula with one of the lowest binding energy values in molecular docking, while qPCR verified that Cxcr4 was decreased after treatments. These results were consistent with those in previous studies.
The results on mRNA expression of Htr2a reflected those in the previous study of Yang et al., who had also found that accumulation of macrophages and neutrophils along with increased levels of inflammatory cytokines, were associated with inflammation (Yang et al., 2020). Meanwhile, the absence of 5-HT suppressed hepatic lipid load and the expression of inflammatory factors, including TNF-α, IL-6, and MCP1 (Wang L. et al., 2020). Another animal experiment also validated that HTR2A was upregulated significantly after inflammation/pain in the dorsal root ganglion following disc puncture in rats (Fujioka et al., 2016).
Histamine and histamine receptors (HRHs) have been confirmed as a kernel molecule in inflammation (Tanaka et al., 2016). Histamine promotes function of keratinocytes in inflammation, and keratinocytes receptors get involved in histamine-induced inflammation, predominantly mediated by HRH1 (Gutowska-Owsiak et al., 2014). Besides, adrenergic receptor (ADR)-agonists have indicated anti-inflammatory characteristics in immune or non-immune cell among various tissues. The present study confirmed several previous findings. Their anti-inflammatory properties are primarily mediated by overexpression of ADRB2 (Dorotea and Ha, 2021). The previous reports are in accordance with our experiment results.
Dopamine receptors are expressed in many immune cells and suppress inflammations (Liu et al., 2021). Our results of DRD1 and DRD4 corroborate the findings of a great deal of the previous study. One previous study reported that it inhibited NLRP3 inflammasome activation via DRD1, which negatively regulated NLRP3 inflammasome by cAMP, promoting ubiquitination of NLRP3 together with its degradation. Furthermore, it has been observed that DRD1 prevented NLRP3 inflammasome–dependent inflammation in vivo (Diaz-Espinosa et al., 2020). Further studies found that DRD4 downregulated the expression of cAMP, which further inhibited the PKA/p38 signaling pathway and downregulated the inflammation factors in a mouse model (Liu et al., 2021). The evidences on qPCR attributed that SJHY formula upregulated the expression of DRD1 and DRD4.
The neuroactive ligand–receptor interaction contained a few pairs, including HTR, DRD, and ADR, which had been considered as hub genes of SJHY formula treated diabetic wound healing as well as played a regulatory role in peripheral nerve disease and chronic inflammation. Besides, the current study detected protein expression of AGTR1, and the result validated that SJHY formula could significantly regulate a relevant neuroactive ligand–receptor interaction.
Another kernel pathway, the cAMP signaling pathway, has been reported in previous studies that it has a role in proliferation, inflammation, and migration, which are present on the progression through distinct yet overlapping phases of wound healing (Boniakowski et al., 2017). cAMP has a dual effect on cell proliferation in the early G0 or G1 phase. Second, cAMP binds to NLRP3 and promotes its ubiquitination together with degradation through the E3 ubiquitin ligase MARCH7. Besides, an increase of cyclic adenosine monophosphate in adult neurons will cause them to grow like newborn neurons in the presence of protein myelin-associated glycoprotein. Consistent with the previous validation, the protein expression level of cAMP was significantly reduced following SJHY formula in the present study, which suggested that the cAMP signaling pathway could be the kernel pathways of SJHY formula treated diabetic wound healing.
Nevertheless, the current study also has some limitations. First, the evidences of molecular docking provided several pairs of TCM ingredients and target, which required further inquiry. Second, genetic function and rescue experiments on hub genes and kernel pathways were required in future research. Third, clinical sample validation should be further conducted.
5 Conclusion
The current study used modular pharmacology analysis to identify the hub genes and pathways of SJHY formula–treated diabetic wound healing. The present study proved that SJHY formula downregulated the mRNA expression of Cxcr4, Oprd1, and Htr2a, while upregulated Adrb2, Drd, Drd4, and Hrh1. Furthermore, kernel cluster enrichment analysis indicated that the SJHY formula could regulate the neuroactive ligand–receptor interaction and the cAMP signaling pathway to ameliorate skin lesions of diabetic wound healing–like mice model.
Data Availability Statement
The raw data supporting the conclusions of this article will be made available by the authors, without undue reservation, to any qualified researcher.
Ethics Statement
The animal study was reviewed and approved by the Ethics Committee of Yueyang Hospital of Integrated Traditional Chinese and Western Medicine.
Author Contributions
J-SJ, YZ, and YiL contributed equally. BL and LK conceived and designed the study. YiL, YR, YuL, and X-YF performed data curation. J-SJ, YZ, J-KS, X-JD, ZZ, DY, H-PZ, and T-YL performed experimental work. J-SJ, YZ, and S-YY prepared the original draft. BL and LK reviewed and edited the manuscript. All authors have read and approved the final manuscript.
Funding
This work was supported by grants from the National Youth Foundation of China (grant numbers 81904214, 82004235), National Key Research and Development Program of China (grant number 2018YFC1705305), NSFC of China (grant numbers 81671959, 81973860), Xinglin Youth Scholar of Shanghai University of Traditional Chinese Medicine (grant number RY411.33.10), Innovative Training Program for Graduate Students in Shanghai University of Traditional Chinese Medicine (grant number JY611.02.03.83) the Shanghai Development Office of TCM (No. ZY(2018-2020)-FWTX-4010, ZY(2018-2020)-FWTX- 1008), and Dermatology Department of Traditional Chinese Medicine, Clinical Key Specialty Construction Project of Shanghai (No. shslczdzk05001) and Shanghai Sailing Program (Grant number 21YF1448100).
Conflict of Interest
Authors H-PZ and T-YL were employed by the company Shanghai Applied Protein Technology Co., Ltd.
The remaining authors declare that the research was conducted in the absence of any commercial or financial relationships that could be construed as a potential conflict of interest.
Publisher’s Note
All claims expressed in this article are solely those of the authors and do not necessarily represent those of their affiliated organizations, or those of the publisher, the editors, and the reviewers. Any product that may be evaluated in this article, or claim that may be made by its manufacturer, is not guaranteed or endorsed by the publisher.
Supplementary Material
The Supplementary Material for this article can be found online at: https://www.frontiersin.org/articles/10.3389/fphar.2021.726158/full#supplementary-material
Supplementary Figure 1 | Approval of animal experiment ethic application.
Supplementary Figure 2 | Map of the neuroactive ligand–receptor interaction.
Supplementary Figure 3 | Map of the cAMP signaling pathway.
Abbreviations
GO, gene ontology; KEGG, Kyoto Encyclopedia of Genes and Genomes; LC-MS/MS, Liquid chromatography tandem–mass spectrometer; OMIM, Online Mendelian Inheritance in Man; PPI, protein–protein interaction; SJHY, Sheng-JiHua-Yu; SPF, specific pathogen-free; TCM, traditional Chinese medicine; TCMSP, traditional Chinese medicine systems pharmacology database and analysis platform; TTD, Therapeutic Target Database.
References
Amberger, J. S., and Hamosh, A. (2017). Searching Online Mendelian Inheritance in Man (OMIM): A Knowledgebase of Human Genes and Genetic Phenotypes. Curr. Protoc. Bioinformatics 58, 1.2.1–1.2.12. doi:10.1002/cpbi.27
Amjad, E., Asnaashari, S., Sokouti, B., and Dastmalchi, S. (2020). Impact of Gene Biomarker Discovery Tools Based on Protein-Protein Interaction and Machine Learning on Performance of Artificial Intelligence Models in Predicting Clinical Stages of Breast Cancer. Interdiscip. Sci. 12, 476–486. doi:10.1007/s12539-020-00390-8
Bai, X., He, T., Liu, J., Wang, Y., Fan, L., Tao, K., et al. (2015). Loureirin B Inhibits Fibroblast Proliferation and Extracellular Matrix Deposition in Hypertrophic Scar via TGF-β/Smad Pathway. Exp. Dermatol. 24 (5), 355–360. doi:10.1111/exd.12665
Barbarino, J. M., Whirl-Carrillo, M., Altman, R. B., and Klein, T. E. (2018). PharmGKB: A Worldwide Resource for Pharmacogenomic Information. Wiley Interdiscip. Rev. Syst. Biol. Med. 10 (4), e1417. doi:10.1002/wsbm.1417
Barrientos, S., Stojadinovic, O., Golinko, M. S., Brem, H., and Tomic-Canic, M. (2008). Growth Factors and Cytokines in Wound Healing. Wound Repair Regen. 16 (5), 585–601. doi:10.1111/j.1524-475X.2008.00410.x
Boniakowski, A. E., Kimball, A. S., Jacobs, B. N., Kunkel, S. L., and Gallagher, K. A. (2017). Macrophage-Mediated Inflammation in Normal and Diabetic Wound Healing. J. Immunol. 199, 17–24. doi:10.4049/jimmunol.1700223
Burley, S. K., Berman, H. M., Christie, C., Duarte, J. M., Feng, Z., Westbrook, J., et al. (2018). RCSB Protein Data Bank: Sustaining a Living Digital Data Resource that Enables Breakthroughs in Scientific Research and Biomedical Education. Protein Sci. 27 (1), 316–330. doi:10.1002/pro.3331
Chen, Q., Wu, F., Wang, M., Dong, S., Liu, Y., Lu, Y., et al. (2016). Transcriptional Profiling and miRNA-Target Network Analysis Identify Potential Biomarkers for Efficacy Evaluation of Fuzheng-Huayu Formula-Treated Hepatitis B Caused Liver Cirrhosis. Int. J. Mol. Sci. 17, 883. doi:10.3390/ijms17060883
Cheng, L., Liu, P., Wang, D., and Leung, K. S. (2019). Exploiting Locational and Topological Overlap Model to Identify Modules in Protein Interaction Networks. BMC Bioinformatics 20, 23. doi:10.1186/s12859-019-2598-7
Curtis, B. J., and Radek, K. A. (2012). Cholinergic Regulation of Keratinocyte Innate Immunity and Permeability Barrier Integrity: New Perspectives in Epidermal Immunity and Disease. J. Invest. Dermatol. 132, 28–42. doi:10.1038/jid.2011.264
da Silva, L., Carvalho, E., and Cruz, M. T. (2010). Role of Neuropeptides in Skin Inflammation and its Involvement in Diabetic Wound Healing. Expert Opin. Biol. Ther. 10, 1427–1439. doi:10.1517/14712598.2010.515207
den Dekker, A., Davis, F. M., Kunkel, S. L., and Gallagher, K. A. (2019). Targeting Epigenetic Mechanisms in Diabetic Wound Healing. Transl Res. 204, 39–50. doi:10.1016/j.trsl.2018.10.001
Diaz-Espinosa, A. M., Link, P. A., Sicard, D., Jorba, I., Tschumperlin, D. J., and Haak, A. J. (2020). Dopamine D1 Receptor Stimulates Cathepsin K-dependent Degradation and Resorption of Collagen I in Lung Fibroblasts. J. Cel Sci 133, jcs248278. doi:10.1242/jcs.248278
Dorotea, D., and Ha, H. (2021). Activation of β2 Adrenergic Receptor Signaling Modulates Inflammation: a Target Limiting the Progression of Kidney Diseases. Arch. Pharm. Res. 44, 49–62. doi:10.1007/s12272-020-01280-9
Fujioka, Y., Stahlberg, A., Ochi, M., and Olmarker, K. (2016). Expression of Inflammation/pain-Related Genes in the Dorsal Root Ganglion Following Disc Puncture in Rats. J. Orthop. Surg. (Hong Kong) 24, 106–112. doi:10.1177/230949901602400124
Guo, G., Zhu, F., Min, D., Huang, Y., and Wu, J. (2020). National Expert Consensus on Surgical Treatment of Diabetic Foot Complicated with Refractory Wounds (2020 Version). Chin J. Inj. Repair Wound Healing 15, 256–263. doi:10.1111/ced.12256
Gutowska-Owsiak, D., Selvakumar, T. A., Salimi, M., Taylor, S., and Ogg, G. S. (2014). Histamine Enhances Keratinocyte-Mediated Resolution of Inflammation by Promoting Wound Healing and Response to Infection. Clin. Exp. Dermatol. 39, 187–195. doi:10.1111/ced.12256
Han, Y., Kang, B., Yang, E., Choi, M., and Song, I. (2019). Simultaneous Determination and Pharmacokinetic Characterization of Glycyrrhizin, Isoliquiritigenin, Liquiritigenin, and Liquiritin in Rat Plasma Following Oral Administration of Glycyrrhizae Radix Extract. Molecules 24, 1816. doi:10.3390/molecules24091816
Hinchliffe, R. J., Brownrigg, J. R., Andros, G., Apelqvist, J., Boyko, E. J., Fitridge, R., et al. (2016). Effectiveness of Revascularization of the Ulcerated Foot in Patients with Diabetes and Peripheral Artery Disease: a Systematic Review. Diabetes Metab. Res. Rev. 32 (Suppl. 1), 136–144. doi:10.1002/dmrr.2705
Huang, L., Xie, D., Yu, Y., Liu, H., Shi, Y., Shi, T., et al. (2018). TCMID 2.0: a Comprehensive Resource for TCM. Nucleic Acids Res. 46, D1117–D1120. doi:10.1093/nar/gkx1028
Huh, J. E., Nam, D. W., Baek, Y. H., Kang, J. W., Park, D. S., Choi, D. Y., et al. (2011). Formononetin Accelerates Wound Repair by the Regulation of Early Growth Response Factor-1 Transcription Factor through the Phosphorylation of the ERK and P38 MAPK Pathways. Int. Immunopharmacol 11 (1), 46–54. doi:10.1016/j.intimp.2010.10.003
Kim, S., Chen, J., Cheng, T., Gindulyte, A., He, J., He, S., et al. (2021). PubChem in 2021: New Data Content and Improved Web Interfaces. Nucleic Acids Res. 49, D1388–D1395. doi:10.1093/nar/gkaa971
Kircher, M., Herhaus, P., Schottelius, M., Buck, A. K., Werner, R. A., Wester, H. J., et al. (2018). CXCR4-directed Theranostics in Oncology and Inflammation. Ann. Nucl. Med. 32, 503–511. doi:10.1007/s12149-018-1290-8
Konrad, F. M., Meichssner, N., Bury, A., Ngamsri, K. C., and Reutershan, J. (2017). Inhibition of SDF-1 Receptors CXCR4 and CXCR7 Attenuates Acute Pulmonary Inflammation via the Adenosine A2B-Receptor on Blood Cells. Cell Death Dis 8, e2832. doi:10.1038/cddis.2016.482
Korczeniewska, O. A., Katzmann Rider, G., Gajra, S., Narra, V., Ramavajla, V., Chang, Y. J., et al. (2020). Differential Gene Expression Changes in the Dorsal Root versus Trigeminal Ganglia Following Peripheral Nerve Injury in Rats. Eur. J. Pain 24, 967–982. doi:10.1002/ejp.1546
Kuai, L., Fei, X. Y., Xing, J. Q., Zhang, J. T., Zhao, K. Q., Ze, K., et al. (2020). An Efficacy Predictive Method for Diabetic Ulcers Based on Higher-Order Markov Chain-Set Pair Analysis. Evid. Based Complement. Alternat Med. 2020, 5091671. doi:10.1155/2020/5091671
Kuai, L., Xing, J. Q., Zhang, J. T., Xu, X. Z., Wu, M. F., Zhao, K. Q., et al. (2019). Application of a Cloud Model-Set Pair Analysis in Efficacy Assessment for Diabetic Ulcers. Evid. Based Complement. Alternat Med. 2019, 8450397. doi:10.1155/2019/8450397
Kuai, L., Zhang, J. T., Deng, Y., Xu, S., Xu, X. Z., Wu, M. F., et al. (2018). Sheng-ji Hua-Yu Formula Promotes Diabetic Wound Healing of Re-epithelization via Activin/Follistatin Regulation. BMC Complement. Altern. Med. 18, 32. doi:10.1186/s12906-017-2074-8
Lai, P. K., Chan, J. Y., Cheng, L., Lau, C. P., Han, S. Q., Leung, P. C., et al. (2013). Isolation of Anti-inflammatory Fractions and Compounds from the Root of Astragalus Membranaceus. Phytother Res. 27 (4), 581–587. doi:10.1002/ptr.4759
Li, B. (2000). Observation on the Efficacy of Sheng-Ji Hua-Yu Recipe in the Treatment of Chronic Ulcer of Lower Limbs. Liaoning J. traditional Chin. Med. 27 (8), 359–360. doi:10.13192/j.ljtcm.2000.08.26.lib.014
Li, F. L., Deng, H., Wang, H. W., Xu, R., Chen, J., Wang, Y. F., et al. (2011). Effects of External Application of Chinese Medicine on Diabetic Ulcers and the Expressions of β-catenin, C-Myc and K6. Chin. J. Integr. Med. 17, 261–266. doi:10.1007/s11655-011-0703-5
Li, F. L., Wang, Y. F., Li, X., Li, F., Xu, R., Chen, J., et al. (2012). Characteristics and Clinical Managements of Chronic Skin Ulcers Based on Traditional Chinese Medicine. Evid. Based Complement. Alternat Med. 2012, 930192. doi:10.1155/2012/930192
Li, X., Wu, Y., Xie, F., Zhang, F., Zhang, S., Zhou, J., et al. (2018). miR-339-5p Negatively Regulates Loureirin A-induced H-air F-ollicle S-tem C-ell D-ifferentiation by T-argeting DLX5. Mol. Med. Rep. 18 (2), 1279–1286. doi:10.3892/mmr.2018.9110
Lin, Y., and Hu, Z. (2021). Bioinformatics Analysis of Candidate Genes Involved in Ethanol-Induced Microtia Pathogenesis Based on a Human Genome Database: GeneCards. Int. J. Pediatr. Otorhinolaryngol. 142, 110595. doi:10.1016/j.ijporl.2020.110595
Liu, M. H., Lin, A. H., Lee, H. F., Ko, H. K., Lee, T. S., and Kou, Y. R. (2014). Paeonol Attenuates Cigarette Smoke-Induced Lung Inflammation by Inhibiting ROS-Sensitive Inflammatory Signaling. Mediators Inflamm. 2014, 651890. doi:10.1155/2014/651890
Liu, Q., Zhang, R., Zhang, X., Liu, J., Wu, H., Li, Y., et al. (2021). Dopamine Improves Chemotherapeutic Efficacy for Pancreatic Cancer by Regulating Macrophage-Derived Inflammations. Cancer Immunol. Immunother. 70, 2165–2177. doi:10.1007/s00262-020-02816-0
Liu, Z., Guo, F., Wang, Y., Li, C., Zhang, X., Li, H., et al. (2016). BATMAN-TCM: a Bioinformatics Analysis Tool for Molecular mechANism of Traditional Chinese Medicine. Sci. Rep. 6, 21146. doi:10.1038/srep21146
Lord, C. J., and Ashworth, A. (2010). Biology-driven Cancer Drug Development: Back to the Future. BMC Biol. 8, 38. doi:10.1186/1741-7007-8-38
Morbach, S., Furchert, H., Gröblinghoff, U., Hoffmeier, H., Kersten, K., Klauke, G. T., et al. (2012). Long-Term Prognosis of Diabetic Foot Patients and Their Limbs: Amputation and Death over the Course of a Decade. Diabetes Care 35, 2021–2027. doi:10.2337/dc12-0200
Moura, J., Madureira, P., Leal, E. C., Fonseca, A. C., and Carvalho, E. (2019). Immune Aging in Diabetes and its Implications in Wound Healing. Clin. Immunol. 200, 43–54. doi:10.1016/j.clim.2019.02.002
Narres, M., Kvitkina, T., Claessen, H., Droste, S., Schuster, B., Morbach, S., et al. (2017). Incidence of Lower Extremity Amputations in the Diabetic Compared with the Non-diabetic Population: A Systematic Review. PLoS ONE 12, e0182081. doi:10.1371/journal.pone.0182081
Papanas, N., and Maltezos, E. (2007). Growth Factors in the Treatment of Diabetic Foot Ulcers: New Technologies, Any Promises. Int. J. Low Extrem Wounds 6 (1), 37–53. doi:10.1177/1534734606298416
Pinzi, L., and Rastelli, G. (2019). Molecular Docking: Shifting Paradigms in Drug Discovery. Int. J. Mol. Sci. 20, 4331. doi:10.3390/ijms20184331
Prompers, L., Huijberts, M., Apelqvist, J., Jude, E., Piaggesi, A., Bakker, K., et al. (2007). High Prevalence of Ischaemia, Infection and Serious Comorbidity in Patients with Diabetic Foot Disease in Europe. Baseline Results from the Eurodiale Study. Diabetologia 50, 18–25. doi:10.1007/s00125-006-0491-1
Rigato, M., Pizzol, D., Tiago, A., Putoto, G., Avogaro, A., and Fadini, G. P. (2018). Characteristics, Prevalence, and Outcomes of Diabetic Foot Ulcers in Africa. A Systemic Review and Meta-Analysis. Diabetes Res. Clin. Pract. 142, 63–73. doi:10.1016/j.diabres.2018.05.016
Ru, J., Li, P., Wang, J., Zhou, W., Li, B., Huang, C., et al. (2014). TCMSP: a Database of Systems Pharmacology for Drug Discovery from Herbal Medicines. J. Cheminform 6, 13. doi:10.1186/1758-2946-6-13
Tanaka, T., Kochi, T., Shirakami, Y., Mori, T., Kurata, A., Watanabe, N., et al. (2016). Cimetidine and Clobenpropit Attenuate Inflammation-Associated Colorectal Carcinogenesis in Male ICR Mice. Cancers (Basel) 8, 25. doi:10.3390/cancers8020025
The UniProt Consortium (2017). UniProt: the Universal Protein Knowledgebase. Nucleic Acids Res. 45, D158–D169. doi:10.1093/nar/gkw1099
Vella, D., Marini, S., Vitali, F., Di Silvestre, D., Mauri, G., and Bellazzi, R. (2018). MTGO: PPI Network Analysis via Topological and Functional Module Identification. Sci. Rep. 8, 5499. doi:10.1038/s41598-018-23672-0
Wang, L., Fan, X., Han, J., Cai, M., Wang, X., Wang, Y., et al. (2020b). Gut-Derived Serotonin Contributes to the Progression of Non-alcoholic Steatohepatitis via the Liver HTR2A/PPARγ2 Pathway. Front. Pharmacol. 11, 553. doi:10.3389/fphar.2020.00553
Wang, Y., Zhang, S., Li, F., Zhou, Y., Zhang, Y., Wang, Z., et al. (2020a). Therapeutic Target Database 2020: Enriched Resource for Facilitating Research and Early Development of Targeted Therapeutics. Nucleic Acids Res. 48, D1031–D1041. doi:10.1093/nar/gkz981
Wang, Z., Liu, J., Yu, Y., Chen, Y., and Wang, Y. (2012). Modular Pharmacology: the Next Paradigm in Drug Discovery. Expert Opin. Drug Discov. 7, 667–677. doi:10.1517/17460441.2012.692673
Wishart, D. S., Feunang, Y. D., Guo, A. C., Lo, E. J., Marcu, A., Grant, J. R., et al. (2018). DrugBank 5.0: a Major Update to the DrugBank Database for 2018. Nucleic Acids Res. 46, D1074–D1082. doi:10.1093/nar/gkx1037
Xiang, Y., Kuai, L., Ru, Y., Jiang, J., Li, X., Li, F., et al. (2021). Transcriptional Profiling and circRNA-miRNA-mRNA Network Analysis Identify the Biomarkers in Sheng-Ji Hua-Yu Formula Treated Diabetic Wound Healing. J. Ethnopharmacol 268, 113643. doi:10.1016/j.jep.2020.113643
Xiong, X., Huang, C., Wang, F., Dong, J., Zhang, D., Jiang, J., et al. (2021). Qingxue Jiedu Formulation Ameliorated DNFB-Induced Atopic Dermatitis by Inhibiting STAT3/MAPK/NF-κB Signaling Pathways. J. Ethnopharmacol 270, 113773. doi:10.1016/j.jep.2020.113773
Yang, T., Wang, H., Li, Y., Zeng, Z., Shen, Y., Wan, C., et al. (2020). Serotonin Receptors 5-HTR2A and 5-HTR2B Are Involved in Cigarette Smoke-Induced Airway Inflammation, Mucus Hypersecretion and Airway Remodeling in Mice. Int. Immunopharmacol 81, 106036. doi:10.1016/j.intimp.2019.106036
Younis, B. B., Shahid, A., Arshad, R., Khurshid, S., Ahmad, M., and Yousaf, H. (2018). Frequency of Foot Ulcers in People with Type 2 Diabetes, Presenting to Specialist Diabetes Clinic at a Tertiary Care Hospital, Lahore, Pakistan. BMC Endocr. Disord. 18, 53. doi:10.1186/s12902-018-0282-y
Zgraggen, S., Huggenberger, R., Kerl, K., and Detmar, M. (2014). An Important Role of the SDF-1/CXCR4 axis in Chronic Skin Inflammation. PLoS One 9, e93665. doi:10.1371/journal.pone.0093665
Zhang, P., Lu, J., Jing, Y., Tang, S., Zhu, D., and Bi, Y. (2017). Global Epidemiology of Diabetic Foot Ulceration: a Systematic Review and Meta-Analysis †. Ann. Med. 49, 106–116. doi:10.1080/07853890.2016.1231932
Keywords: diabetic wound healing, SJHY formula, modular pharmacology, molecular docking, experimental verification
Citation: Jiang J-S, Zhang Y, Luo Y, Ru Y, Luo Y, Fei X-Y, Song J-K, Ding X-J, Zhang Z, Yang D, Yin S-Y, Zhang H-P, Liu T-Y, Li B and Kuai L (2021) The Identification of the Biomarkers of Sheng-Ji Hua-Yu Formula Treated Diabetic Wound Healing Using Modular Pharmacology. Front. Pharmacol. 12:726158. doi: 10.3389/fphar.2021.726158
Received: 16 June 2021; Accepted: 11 October 2021;
Published: 16 November 2021.
Edited by:
Tao Xu, Anhui Medical University, ChinaReviewed by:
Xinkui Liu, Beijing University of Chinese Medicine, ChinaKe Lv, China Astronaut Research and Training Center, China
Copyright © 2021 Jiang, Zhang, Luo, Ru, Luo, Fei, Song, Ding, Zhang, Yang, Yin, Zhang, Liu, Li and Kuai. This is an open-access article distributed under the terms of the Creative Commons Attribution License (CC BY). The use, distribution or reproduction in other forums is permitted, provided the original author(s) and the copyright owner(s) are credited and that the original publication in this journal is cited, in accordance with accepted academic practice. No use, distribution or reproduction is permitted which does not comply with these terms.
*Correspondence: Bin Li, 18930568129@163.com; Le Kuai, mjbubu@qq.com
†These authors have contributed equally to this work