- 1W. M. Keck Science Department, Scripps College, Claremont, CA, United States
- 2W. M. Keck Science Department, Pitzer College, Claremont, CA, United States
- 3Physics and Biophysics Department, University of San Diego, San Diego, CA, United States
The cytoskeleton–a composite network of biopolymers, molecular motors, and associated binding proteins–is a paradigmatic example of active matter. Particle transport through the cytoskeleton can range from anomalous and heterogeneous subdiffusion to superdiffusion and advection. Yet, recapitulating and understanding these properties–ubiquitous to the cytoskeleton and other out-of-equilibrium soft matter systems–remains challenging. Here, we combine light sheet microscopy with differential dynamic microscopy and single-particle tracking to elucidate anomalous and advective transport in actomyosin-microtubule composites. We show that particles exhibit multi-mode transport that transitions from pronounced subdiffusion to superdiffusion at tunable crossover timescales. Surprisingly, while higher actomyosin content increases the range of timescales over which transport is superdiffusive, it also markedly increases the degree of subdiffusion at short timescales and generally slows transport. Corresponding displacement distributions display unique combinations of non-Gaussianity, asymmetry, and non-zero modes, indicative of directed advection coupled with caged diffusion and hopping. At larger spatiotemporal scales, particles in active composites exhibit superdiffusive dynamics with scaling exponents that are robust to changing actomyosin fractions, in contrast to normal, yet faster, diffusion in networks without actomyosin. Our specific results shed important new light on the interplay between non-equilibrium processes, crowding and heterogeneity in active cytoskeletal systems. More generally, our approach is broadly applicable to active matter systems to elucidate transport and dynamics across scales.
1 Introduction
The cytoplasm is a crowded, heterogeneous, out-of-equilibrium material through which macromolecules and vesicles traverse to perform critical cellular processes such as mitosis, endocytosis, migration, and regeneration [1–4]. Macromolecules and particles diffusing through the cytoplasm and other similar materials have been shown to exhibit widely varying and poorly understood anomalous transport properties that deviate significantly from normal Brownian diffusion. In particular, the mean-squared displacement,
In steady-state, the thermal transport of particles through in vitro cytoskeletal systems exhibit varying degrees of subdiffusion and non-Gaussianity depending on the types and concentrations of filaments and crosslinking proteins [5, 6, 12, 13]. For example, single-particle tracking (SPT) of particles in composites of entangled actin filaments and microtubules, has revealed increasing degrees of subdiffusion (
Differential dynamic microscopy (DDM), which uses Fourier-space analysis to measure the timescales over which particle density fluctuations decay, has also been used to measure transport and quantify anomalous characteristics over larger spatiotemporal scales compared to SPT [5, 14, 15]. DDM analysis of a time-series of images provides a characteristic decay time
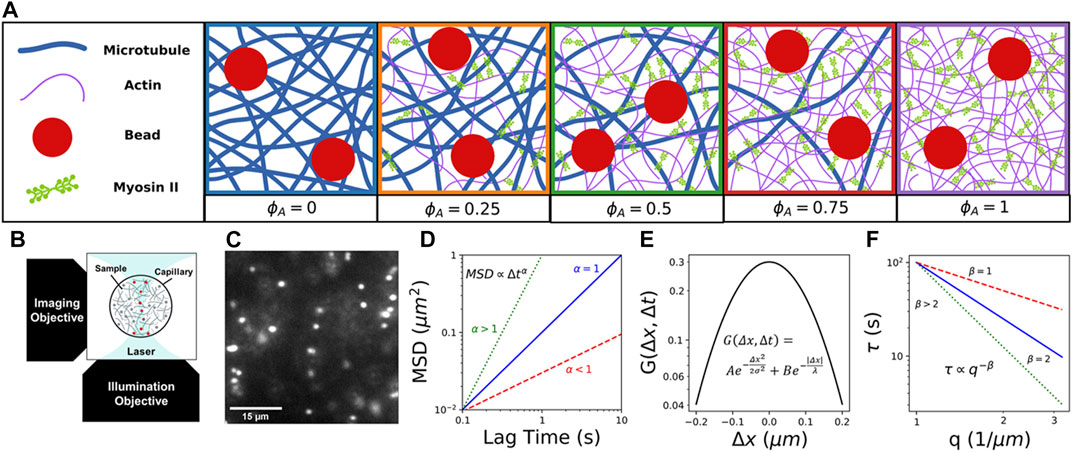
FIGURE 1. Combining light sheet microscopy with real-space single-particle tracking (SPT) and reciprocal-space differential dynamic microscopy (DDM) to characterize particle transport in active cytoskeletal composites. (A) We create composites of co-entangled microtubules (blue) and actin filaments (purple) driven out-of-equilibrium by myosin II minifilaments (green). We track the motion of embedded 1
Similar SPT and DDM experiments demonstrated that crosslinking of actin and/or microtubules introduced bi-phasic transport with the subdiffusive scaling exponents dropping from
Numerous studies have also investigated transport in non-equilibrium cytoskeleton networks, in which activity is introduced via motor proteins, such as actin-associated myosin II and microtubule-associated kinesin [2, 10, 18–21]. These studies have shown evidence of vesicle movement strongly tracking with actin movement, microtubule-dependent flow, and the simultaneous presence of subdiffusive and ballistic transport dynamics. While the majority of these active matter studies have been on systems of either actin or microtubules, recent studies have used DDM and optical tweezers microrheology to characterize the dynamics of actin-microtubule composites pushed out-of-equilibrium by myosin II minifilaments straining actin filaments [14, 22, 23]. These studies showed that active actin-microtubule composites exhibited ballistic-like (
However, particle transport through active actin-microtubule composites–likely dictated by the complex combination of active processes, crowding, and interactions between mechanically distinct filaments—has remained largely unexplored. The majority of studies that have examined the combined effect of activity and crowding have been in vivo [1, 24–29], where a large number of conflating variables that are difficult to tune make teasing out the effect of each contribution and mechanism highly non-trivial.
Here, we take advantage of the tunability of in vitro reconstituted cytoskeleton composites to systematically investigate the coupled effects of non-equilibrium activity, crowding, and heterogeneity on particle transport. We combine fluorescence light sheet microscopy (fLSM) with single-particle tracking (SPT) and differential dynamic microscopy (DDM) to examine the anomalous transport of micron-sized particles within active composites of myosin II minifilaments, actin filaments, and microtubules with varying molar fractions of actin and tubulin (Figure 1A). We leverage the optical sectioning and low excitation power of fLSM (Figure 1B) to capture particle trajectories with a temporal resolution of 0.1s for durations up to 400 s (Figure 1C). Using both SPT and DDM provides transport characterization over a broad spatiotemporal range that spans ∼10–1 -102 s and ∼10–1 - 10
2 Materials and methods
2.1 Protein preparation
We reconstitute rabbit skeletal actin (Cytoskeleton, Inc. AKL99) to 2 mg/ml in 5 mM Tris–HCl (pH 8.0), 0.2 mM CaCl2, 0.2 mM ATP, 5% (w/v) sucrose, and 1% (w/v) dextran; porcine brain tubulin (Cytoskeleton T240) to 5 mg/ml in 80 mM PIPES (pH 6.9), 2 mM MgCl2, 0.5 mM EGTA, and 1 mM GTP; and rabbit skeletal myosin II (Cytoskeleton MY02) to 10 mg/ml in 25 mM PIPES (pH 7.0), 1.25 M KCl, 2.5% sucrose, 0.5% dextran, and 1 mM DTT. We flash freeze all proteins in single-use aliquots and store at -80˚C. We reconstitute the UV-sensitive myosin II inhibitor, (-)-blebbistatin (Sigma B0560) in anhydrous DMSO and store at -20˚C for up to 6 months. Immediately prior to experiments, we remove enzymatically dead myosin II from aliquots using centrifugation clarification, as previously described [14, 22].
2.2 Composite network assembly
We prepare actin-microtubule composites by mixing actin monomers, tubulin dimers and a trace amount of 1 µm diameter carboxylated microspheres (Polysciences) in PEM-100 (100 mM PIPES, 2 mM MgCl2, and 2 mM EGTA), 0.1% Tween-20, 1 mM ATP, and 1 mM GTP, and incubating at 37°C for 30 min to allow for polymerization of actin filaments and microtubules. We coat microspheres (beads) with AlexaFluor594 BSA (Invitrogen) to visualize the particles and prevent nonspecific interactions with the composite [30, 31]. We fix the combined molar concentration of actin and tubulin to
2.3 Sample preparation and imaging
We pipet prepared composites into capillary tubing with an inner diameter of x=800 μm and length of y=10 mm, then seal with epoxy. Microspheres are imaged using a custom-built fLSM with a 10
2.4 Single-particle tracking
We use the Python package Trackpy [34] to track particle trajectories and measure the
Additionally, we compute van Hove probability distributions of particle displacements,
2.5 Differential dynamic microscopy
We obtain the image structure function
where
We also examine the stretching exponent
3 Results and discussion
To elucidate the combined effects of non-equilibrium activity and steric hindrance on particle transport in crowded active matter, we leverage the tunability of reconstituted cytoskeleton composites [42] and the power of coupling real-space (SPT) and reciprocal space (DDM) transport analysis, to robustly characterize particle transport as a function of active substrate content. Specifically, we tune the composition of actomyosin-microtubule composites to display a wide range of transport characteristics by varying the molar fraction of actomyosin, which we denote by the molar actin fraction
In Figure 2A, we plot the ensemble-averaged
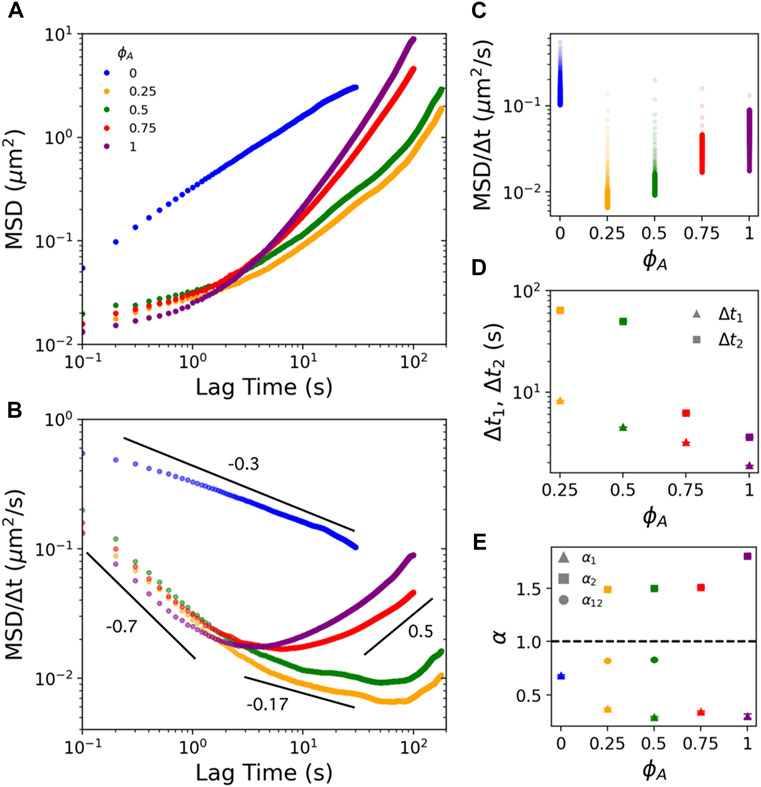
FIGURE 2. Actomyosin activity in actin-microtubule composites endows multi-phasic particle transport marked by pronounced subdiffusion at short lag times and superdiffusion at long lag times. (A) Mean-squared displacements (
To evaluate the
To understand this phenomenon, we consider that active ballistic transport would only be detectable at timescales in which the network motion can move a bead more than the minimum resolvable displacement:
To determine the extent to which motor-driven transport and confinement contribute to the particle dynamics, we next evaluate the anomalous scaling exponents in the short, intermediate, and long
To understand the lower
Examining the long-time regime,
To shed further light on the mechanisms underlying the anomalous transport shown in Figure 2, we compute van Hove distributions
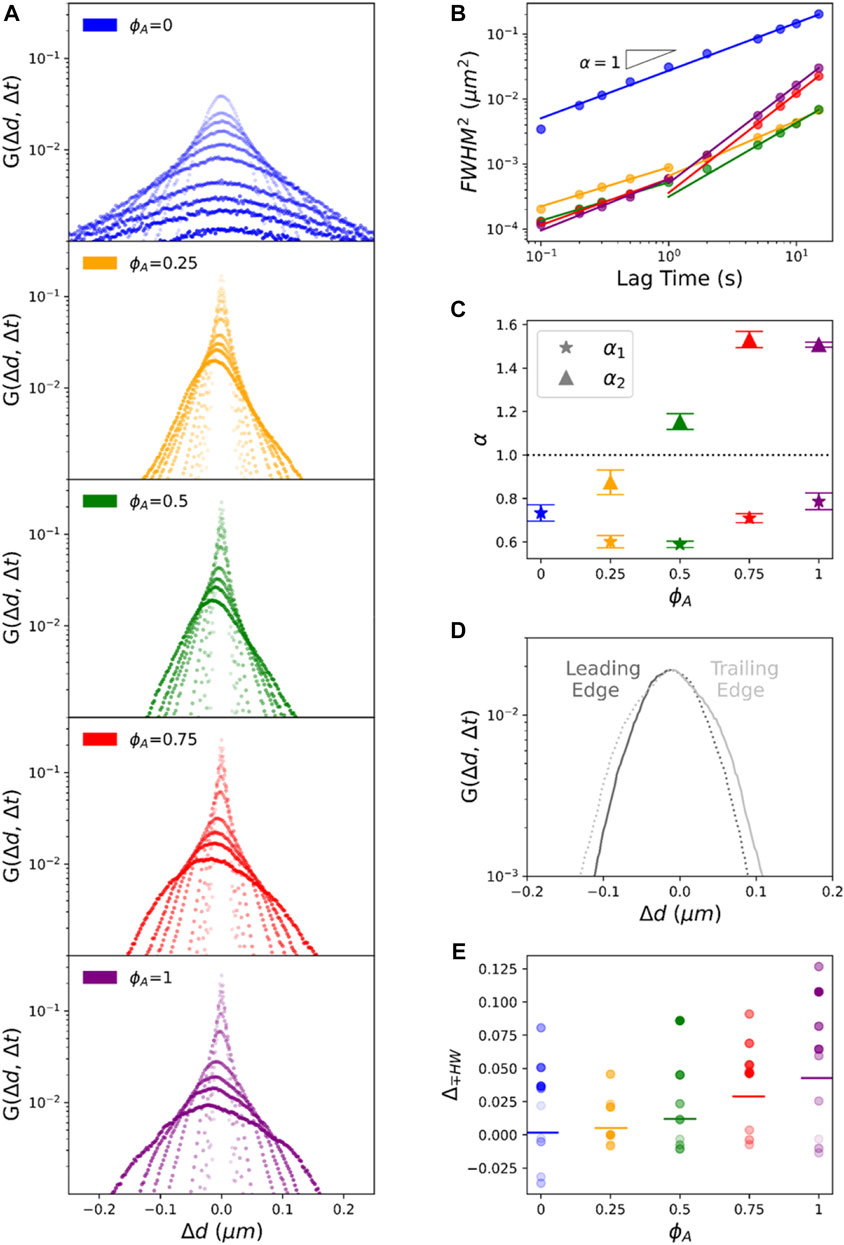
FIGURE 3. Asymmetric non-Gaussian van Hove distributions reveal a combination of heterogeneous subdiffusion and advective transport of particles in active composites. (A) van Hove distributions
While our analysis described above assumes Gaussian distributions, Figure 3A shows that nearly all distributions have distinct non-Gaussian features similar to those reported for steady-state actin-microtubule composites [5, 7]. In particular,
The distributions for active composites are even more complex, with asymmetries and peaks at
We also note that the asymmetry shown in Figure 3A is always in the negative direction (to the left). To determine the directionality of this asymmetry we evaluate the distributions of
To determine if this directionality is a reproducible microscale bias or is an ensemble result of averaging over trials with positive and negative anisotropies, we evaluate the van Hove distributions for each trial of the
To more quantitatively characterize the rich transport phenomena revealed in Figure 3, we first fit each
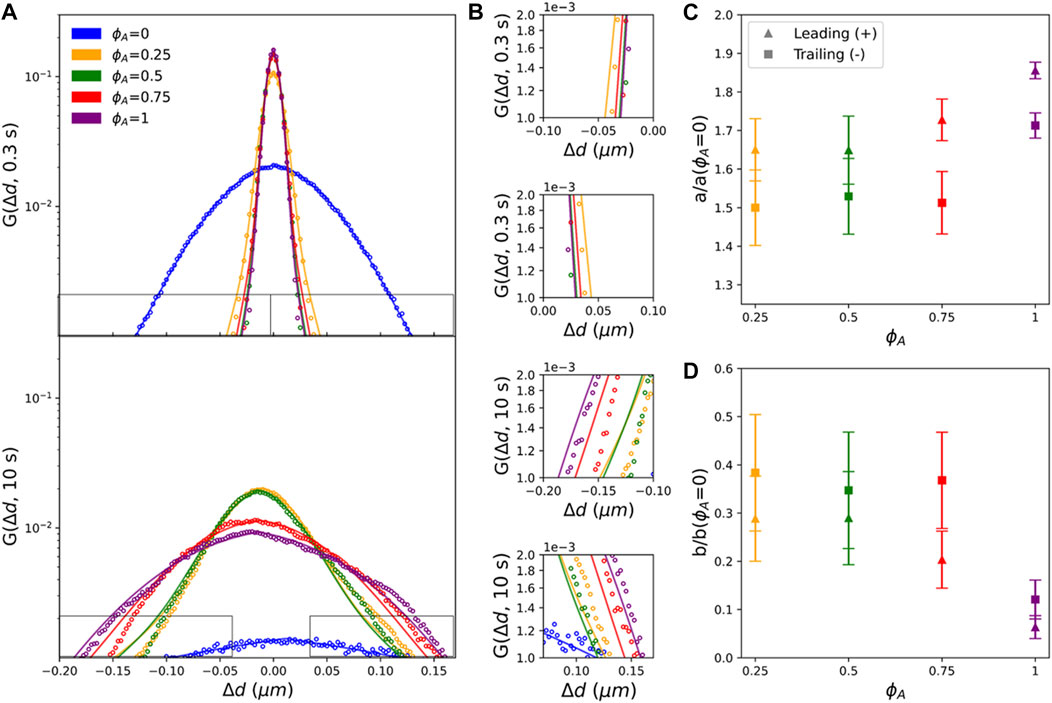
FIGURE 4. Actomyosin activity reduces heterogeneous non-Gaussian diffusivity and endows Gaussian-like advective transport. (A) Comparing van Hove distributions of composites with different
As shown in Figures 4C,D, in which
Conversely, the increased contribution from the exponential term for the trailing edge, implies that displacements comprising these exponential tails are dominated by heterogeneous transport modes such as hopping between heterogeneously distributed cages [6, 7]. To better understand this effect, we recall that particle displacements comprising the trailing edge are lagging behind the bulk translational motion of the composite. As the composite moves and restructures, a fraction of the particles remain caged in the moving composite and thus move along with it, corresponding to displacements comprising the leading edge, whereas a fraction of the particles are squeezed out or hop out of composite cages and into new ‘trailing’ cages. It is also likely that as the composite contracts and forms more heterogeneous structures and larger open voids that characteristic ‘hopping’ displacements, as well as displacements withing cages, may increase and become more heterogeneous, thereby enhancing exponential tails.
To expand the range of length and time scales over which we probe the non-equilibrium transport, and provide an independent measure of the dynamics, we complement our real-space SPT analysis with Fourier-space DDM analysis, as described in the Methods and previously [5, 6, 14]. Briefly, we compute the radially-averaged image structure function
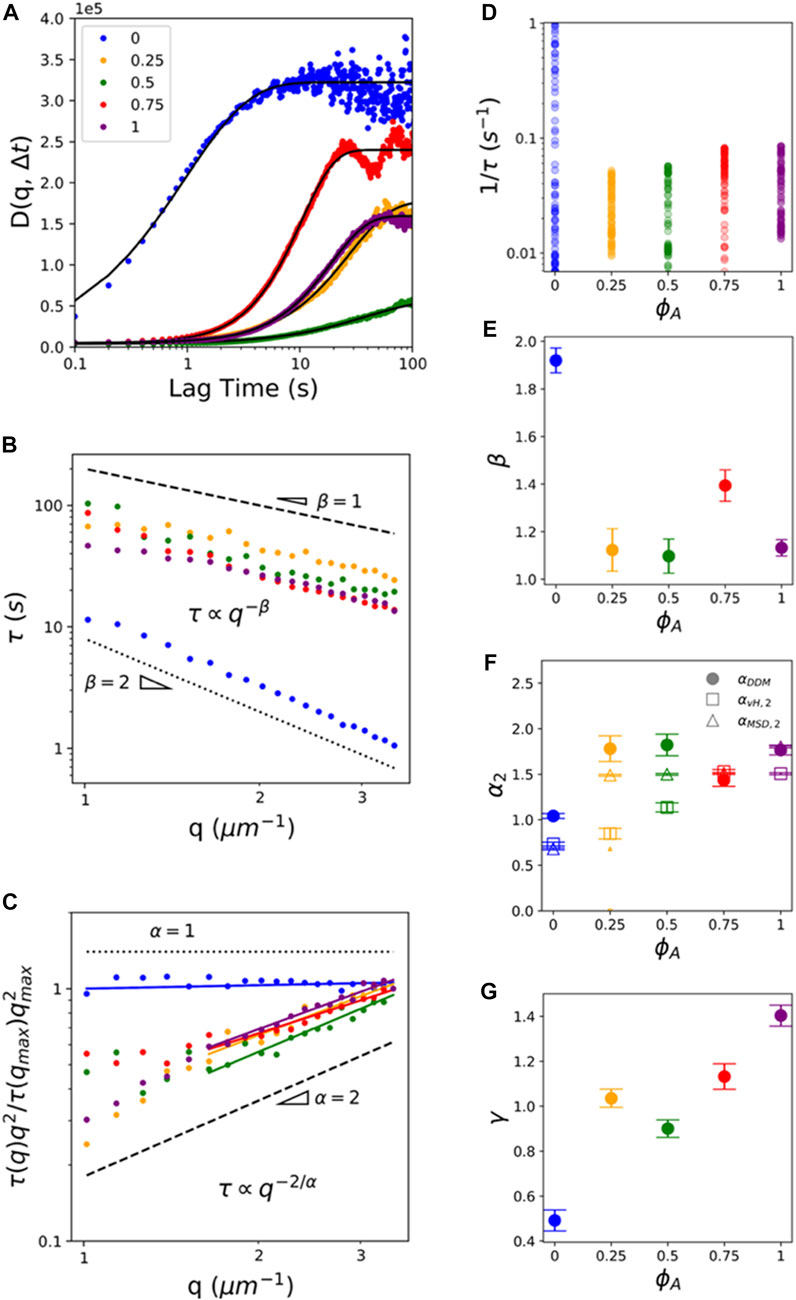
FIGURE 5. DDM analysis reveals ballistic-like transport of particles entrained in active composites at mesoscopic spatiotemporal scales. (A) Sample image structure functions
As shown in Figure 5B,
To better visualize differences in
Finally, to shed light on the competing contributions from motor-driven dynamics versus confinement and crowding to transport at larger spatiotemporal scales, we evaluate the dependence of the stretching exponent
We note that while other active systems display a return to Gaussian dynamics at long timescales (e.g., 46), we find no evidence of this return due to the relatively slow active network dynamics compared to other active systems. However, our inactive system (
4 Conclusion
Here, we couple real-space SPT and Fourier-space DDM to characterize particle transport across three decades in time (∼10–1–102 s) and two decades in space (∼10–1—10
Figure 6 summarizes and compares the key metrics we present in Figures 2–5 that characterize these complex transport properties. Importantly, as highlighted in Figure 6, while there is clear difference between the inactive and active networks for nearly all of the transport metrics we present, we emphasize that there are very few clear monotonic dependences on
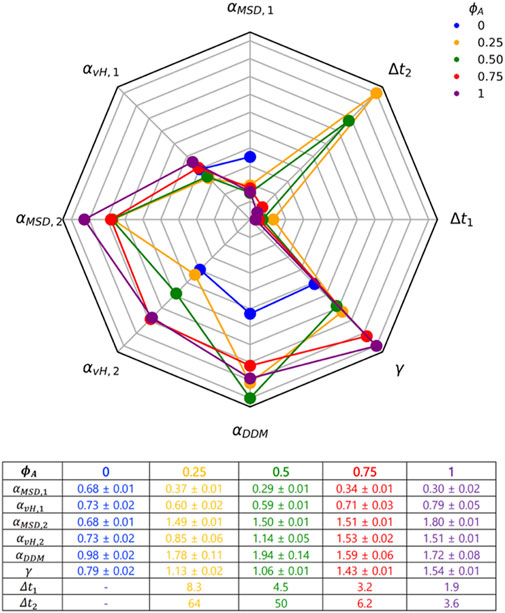
FIGURE 6. A robust suite of metrics reveals complex scale-dependent transport resulting from competition between motor-driven active dynamics, crowding and network connectivity. The 8-variable spider plot shows how the key metrics we use to characterize transport depend on
Data availability statement
The datasets generated and analyzed for this manuscript are available from the corresponding author on reasonable request.
Author contributions
JS analyzed and interpreted the data and wrote the paper; JG collected and helped analyze the data; SK and BL helped analyze data and prepare figures; RM conceived and guided the project, developed analysis code, helped analyze and interpret the data, and helped write the paper; RR-A conceived and guided the project, interpreted the data, and wrote the paper.
Funding
This work was supported by National Institutes of Health R15 Awards (R15GM123420, 2R15GM123420-02) to RMR-A and RJM, William M. Keck Foundation Research Grant to RMR-A, and NSF RUI Award (DMR-2203791) to JYS.
Acknowledgments
We thank AX, NB, and JK for help with data analysis and visualization. We thank GL for help with composite design. We thank DA for insightful discussions.
Conflict of interest
The authors declare that the research was conducted in the absence of any commercial or financial relationships that could be construed as a potential conflict of interest.
Publisher’s note
All claims expressed in this article are solely those of the authors and do not necessarily represent those of their affiliated organizations, or those of the publisher, the editors and the reviewers. Any product that may be evaluated in this article, or claim that may be made by its manufacturer, is not guaranteed or endorsed by the publisher.
Supplementary material
The Supplementary Material for this article can be found online at: https://www.frontiersin.org/articles/10.3389/fphy.2022.1055441/full#supplementary-material
References
1. Mogre SS, Brown AI, Koslover EF. Getting around the cell: Physical transport in the intracellular world. Phys Biol (2020) 17:061003. doi:10.1088/1478-3975/aba5e5
2. Brangwynne CP, Koenderink GH, MacKintosh FC, Weitz DA. Intracellular transport by active diffusion. Trends Cel Biol (2009) 19:423–7. doi:10.1016/j.tcb.2009.04.004
3. Burute M, Kapitein LC. Cellular logistics: Unraveling the interplay between microtubule organization and intracellular transport. Annu Rev Cel Dev Biol (2019) 35:29–54. doi:10.1146/annurev-cellbio-100818-125149
4. Gardel ML, Schneider IC, Aratyn-Schaus Y, Waterman CM. Mechanical Integration of actin and Adhesion dynamics in cell migration. Annu Rev Cel Dev Biol (2010) 26:315–33. doi:10.1146/annurev.cellbio.011209.122036
5. Anderson SJ, Garamella J, Adalbert S, McGorty RJ, Robertson-Anderson RM. Subtle changes in crosslinking drive diverse anomalous transport characteristics in actin–microtubule networks. Soft Matter (2021) 17:4375–85. doi:10.1039/d1sm00093d
6. Anderson SJ, Matsuda C, Garamella J, Peddireddy KR, Robertson-Anderson RM, McGorty R. Filament Rigidity Vies with mesh size in determining anomalous diffusion in cytoskeleton. Biomacromolecules (2019) 20:4380–8. doi:10.1021/acs.biomac.9b01057
7. Wang B, Kuo J, Bae SC, Granick S. When Brownian diffusion is not Gaussian. Nat Mater (2012) 11:481–5. doi:10.1038/nmat3308
8. Sokolov IM. Models of anomalous diffusion in crowded environments. Soft Matter (2012) 8:9043. doi:10.1039/c2sm25701g
9. Burla F, Mulla Y, Vos BE, Aufderhorst-Roberts A, Koenderink GH. From mechanical resilience to active material properties in biopolymer networks. Nat Rev Phys (2019) 1:249–63. doi:10.1038/s42254-019-0036-4
10. Koenderink GH, Dogic Z, Nakamura F, Bendix PM, MacKintosh FC, Hartwig JH, et al. An active biopolymer network controlled by molecular motors. Proc Natl Acad Sci U S A (2009) 106:15192–7. doi:10.1073/pnas.0903974106
12. Regan K, Wulstein D, Rasmussen H, McGorty R, Robertson-Anderson RM. Bridging the spatiotemporal scales of macromolecular transport in crowded biomimetic systems. Soft Matter (2019) 15:1200–9. doi:10.1039/c8sm02023j
13. Wulstein DM, Regan KE, Garamella J, McGorty RJ, Robertson-Anderson RM. Topology-dependent anomalous dynamics of ring and linear DNA are sensitive to cytoskeleton crosslinking. Sci Adv (2019) 5:eaay5912. doi:10.1126/sciadv.aay5912
14. Lee G, Leech G, Rust MJ, Das M, McGorty RJ, Ross JL, et al. Myosin-driven actin-microtubule networks exhibit self-organized contractile dynamics. Sci Adv (2021) 7:eabe4334. doi:10.1126/sciadv.abe4334
15. Cerbino R, Cicuta P. Perspective: Differential dynamic microscopy extracts multi-scale activity in complex fluids and biological systems. J Chem Phys (2017) 147:110901. doi:10.1063/1.5001027
16. Cerbino R, Trappe V. Differential Dynamic Microscopy: Probing wave vector dependent dynamics with a microscope. Phys Rev Lett (2008) 100:188102. doi:10.1103/physrevlett.100.188102
17. Burov S, Jeon J-H, Metzler R, Barkai E. Single particle tracking in systems showing anomalous diffusion: The role of weak ergodicity breaking. Phys Chem Chem Phys (2011) 13:1800–12. doi:10.1039/c0cp01879a
18. Scholz M, Weirich KL, Gardel ML, Dinner AR. Tuning molecular motor transport through cytoskeletal filament network organization. Soft Matter (2020) 16:2135–40. doi:10.1039/c9sm01904a
19. Needleman D, Dogic Z. Active matter at the interface between materials science and cell biology. Nat Rev Mater (2017) 2:17048–14. doi:10.1038/natrevmats.2017.48
20. Foster PJ, Fürthauer S, Shelley MJ, Needleman DJ. Active contraction of microtubule networks. eLife (2015) 4:e10837. doi:10.7554/elife.10837
21. Vale RD. The molecular motor toolbox for intracellular transport. Cell (2003) 112:467–80. doi:10.1016/s0092-8674(03)00111-9
22. Sheung JY, Achiriloaie DH, Currie C, Peddireddy K, Xie A, Simon-Parker J, et al. Motor-driven restructuring of cytoskeleton composites leads to tunable time-varying Elasticity. ACS Macro Lett (2021) 10:1151–8. doi:10.1021/acsmacrolett.1c00500
23. Lee G, Leech G, Lwin P, Michel J, Currie C, Rust MJ, et al. Active cytoskeletal composites display emergent tunable contractility and restructuring. Soft Matter (2021) 17:10765–76. doi:10.1039/d1sm01083b
24. Drechsler M, Giavazzi F, Cerbino R, Palacios IM. Active diffusion and advection in Drosophila oocytes result from the interplay of actin and microtubules. Nat Commun (2017) 8:1520. doi:10.1038/s41467-017-01414-6
25. Rezaul K, Gupta D, Semenova I, Ikeda K, Kraikivski P, Yu J, et al. Engineered Tug-of-War between kinesin and Dynein controls direction of microtubule Based transport in vivo. Traffic (2016) 17:475–86. doi:10.1111/tra.12385
26. Brangwynne CP, Koenderink GH, MacKintosh FC, Weitz DA. Cytoplasmic diffusion: Molecular motors mix it up. J Cel Biol (2008) 183:583–7. doi:10.1083/jcb.200806149
27. Ganguly S, Williams LS, Palacios IM, Goldstein RE. Cytoplasmic streaming in Drosophila oocytes varies with kinesin activity and correlates with the microtubule cytoskeleton architecture. Proc Natl Acad Sci U S A (2012) 109:15109–14. doi:10.1073/pnas.1203575109
28. Goldstein RE, van de Meent J-W. A physical perspective on cytoplasmic streaming. Interf Focus (2015) 5:20150030. doi:10.1098/rsfs.2015.0030
29. Kural C, Serpinskaya AS, Chou YH, Goldman RD, Gelfand VI, Selvin PR. Tracking melanosomes inside a cell to study molecular motors and their interaction. Proc Natl Acad Sci U S A (2007) 104:5378–82. doi:10.1073/pnas.0700145104
30. Valentine MT, Perlman Z, Gardel M, Shin J, Matsudaira P, Mitchison T, et al. Colloid Surface Chemistry critically Affects multiple particle tracking measurements of biomaterials. Biophys J (2004) 86:4004–14. doi:10.1529/biophysj.103.037812
31. Weigand WJ, Messmore A, Tu J, Morales-Sanz A, Blair DL, Deheyn DD, et al. Active microrheology determines scale-dependent material properties of Chaetopterus mucus. PLOS ONE (2017) 12:e0176732. doi:10.1371/journal.pone.0176732
32. Jordan MA, Wilson L. Microtubules as a target for anticancer drugs. Nat Rev Cancer (2004) 4:253–65. doi:10.1038/nrc1317
33. Jordan MA, Toso RJ, Thrower D, Wilson L. Mechanism of mitotic block and inhibition of cell proliferation by taxol at low concentrations. Proc Natl Acad Sci U S A (1993) 90:9552–6. doi:10.1073/pnas.90.20.9552
34. Allan DB, Caswell T, Keim NC, van der Wel CM, Verweij RW. soft-matter/trackpy: Trackpy v0.5.0 (2021). doi:10.5281/zenodo.4682814
35. Wulstein DM, Regan KE, Robertson-Anderson RM, McGorty R. Light-sheet microscopy with digital Fourier analysis measures transport properties over large field-of-view. Opt Express (2016) 24:20881–94. doi:10.1364/oe.24.020881
36. Jacob JDC, He K, Retterer ST, Krishnamoorti R, Conrad JC. Diffusive dynamics of nanoparticles in ultra-confined media. Soft Matter (2015) 11:7515–24. doi:10.1039/c5sm01437a
37. He K, Babaye Khorasani F, Retterer ST, Thomas DK, Conrad JC, Krishnamoorti R. Diffusive dynamics of nanoparticles in Arrays of Nanoposts. ACS Nano (2013) 7:5122–30. doi:10.1021/nn4007303
38. Burla F, Sentjabrskaja T, Pletikapic G, van Beugena J, Koenderink GH. Particle diffusion in extracellular hydrogels. Soft Matter (2020) 16:1366–76. doi:10.1039/c9sm01837a
39. Cipelletti L, Manley S, Ball RC, Weitz DA. Universal aging features in the restructuring of fractal colloidal gels. Phys Rev Lett (2000) 84:2275–8. doi:10.1103/physrevlett.84.2275
40. Giavazzi F, Trappe V, Cerbino R. Multiple dynamic regimes in a coarsening foam. J Phys : Condens Matter (2021) 33:024002. doi:10.1088/1361-648x/abb684
41. Alvarado J, Cipelletti L, Koenderink GH. Uncovering the dynamic precursors to motor-driven contraction of active gels. Soft Matter (2019) 15:8552–65. doi:10.1039/c9sm01172b
42. Ricketts N, Gurmessa B, Robertson-Anderson M, Microscale R. Mechanics of Plug-and-Play in vitro cytoskeleton networks. In: Antonio Bastidas Pacheco G, Ali Kamboh A, editors. Parasitology and microbiology research. IntechOpen (2020). doi:10.5772/intechopen.84401
43. Ricketts SN, Francis ML, Farhadi L, Rust MJ, Das M, Ross JL, et al. Varying crosslinking motifs drive the mesoscale mechanics of actin-microtubule composites. Sci Rep (2019) 9:12831. doi:10.1038/s41598-019-49236-4
44. Gal N, Lechtman-Goldstein D, Weihs D. Particle tracking in living cells: A review of the mean square displacement method and beyond. Rheol Acta (2013) 52:425–43. doi:10.1007/s00397-013-0694-6
45. Cho JH, Cerbino R, Bischofberger I. Emergence of Multiscale dynamics in colloidal gels. Phys Rev Lett (2020) 124:088005. doi:10.1103/physrevlett.124.088005
Keywords: fluorescence microscopy, myosin, single-particle tracking, differential dynamic microscopy, cytoskeleton, actin, microtubule
Citation: Sheung JY, Garamella J, Kahl SK, Lee BY, McGorty RJ and Robertson-Anderson RM (2022) Motor-driven advection competes with crowding to drive spatiotemporally heterogeneous transport in cytoskeleton composites. Front. Phys. 10:1055441. doi: 10.3389/fphy.2022.1055441
Received: 27 September 2022; Accepted: 31 October 2022;
Published: 18 November 2022.
Edited by:
Roberto Cerbino, University of Vienna, AustriaReviewed by:
Fabio Giavazzi, University of Milan, ItalyPaolo Malgaretti, Helmholtz Institute Erlangen-Nürnberg, Germany
Copyright © 2022 Sheung, Garamella, Kahl, Lee, McGorty and Robertson-Anderson. This is an open-access article distributed under the terms of the Creative Commons Attribution License (CC BY). The use, distribution or reproduction in other forums is permitted, provided the original author(s) and the copyright owner(s) are credited and that the original publication in this journal is cited, in accordance with accepted academic practice. No use, distribution or reproduction is permitted which does not comply with these terms.
*Correspondence: Janet Y. Sheung, jsheung@scrippscollege.edu