- 1School of Public Health, Dalian Medical University, Dalian, China
- 2School of International Education, Dalian Medical University, Dalian, China
- 3Department of Psychology, Dalian Medical University, Dalian, China
Background: Nowadays, e-learning significantly affects college students’ academic life. This study aims to examine the factors that influence college students’ satisfaction with online learning outcomes.
Method: The study population consisted of undergraduate students from Dalian Medical University, with a total of 715 college students participating in the study. Out of these participants, 602 valid questionnaires were obtained. Demographic data was analyzed using SPSS.22, and the data was cleaned and prepared for testing the research hypotheses. The proposed research framework was examined using structural equation modeling (SEM) through Smart-PLS 3.0.
Results: The results of the study showed that student satisfaction with learning outcomes was positively correlated with several factors: quality of teacher instruction (β = 0.100, p < 0.0001), quality of e-learning platforms (β = 0.059, p < 0.0001), individual learner factors such as learning motivation (β = 0.112, p < 0.001), and e-learning environment (β = 0.469, p < 0.001). Additionally, self-learning efficacy (β = 0.081, p < 0.0001), learning strategies (β = 0.031, p < 0.001), and learning motivation (β = 0.039, p < 0.001) were found to have mediating effects.
Conclusion: Understanding the satisfaction of college students with the effect of e-learning holds great significance in coping with teaching methods in unexpected situations. It enables adjustments to teaching strategies, improvements to learning platforms, and mobilization of students’ motivation. Thus, it serves as a valuable reference in addressing unexpected teaching scenarios.
1. Introduction
1.1. Research background
E-learning has become a necessary mode of learning in the current education system. The rapid development of online courses has been fueled by the increasing demand for both new and existing courses. Studies have shown that about 66% of institutions in the U.S. reported a rise in demand for new online courses (Allen and Seaman, 2010). Similarly, approximately 73% of educational institutions observed an increase in demand for existing online courses (Alqurashi, 2016). The COVID-19 pandemic, along with other global health crises, has further highlighted the limitations of traditional education, such as spatial constraints and limitations in timeliness. This has exposed the contradiction between existing intelligent educational technology and the current needs of higher education, thereby accelerating the progress of online courses (Pokhrel and Chhetri, 2021). To meet the demands of the digital era, higher education institutions have integrated modern information technology with teaching activities, constructing new teaching platforms that utilize e-learning (Ma, 2017). As a result, e-learning has become an indispensable part of college students’ daily study lives.
The pandemic has provided an opportunity for the rapid development of innovative and alternative education systems and assessment strategies, paving the way for the introduction and promotion of digital learning (Xie et al., 2020). Consequently, much research has been focused on optimizing e-learning, which includes improving the learning environment, reforming teaching methods, upgrading educational resources, and fostering students’ learning expectations (Wang et al., 2021). However, despite these advancements, various factors continue to impact college students’ experience in e-learning, leading to persistent challenges. Some of these challenges include the absence of an effective online learning atmosphere (Zhang, 2021), low learning atmosphere, inadequate interactivity in virtual classrooms, and limited teacher-student interaction (Dai and Lin, 2020). Furthermore, the use of a single teaching method, the lack of effective assessment methods, insufficient online course resources (Zhou et al., 2020), and inadequate learning platforms and technical support contribute to the ongoing issues with e-learning (Bao, 2020).
According to studies, continuous exposure to shortcomings in e-learning can increase the pressure on learners’ perceived ease of use and usefulness of new technologies. This, in turn, affects users’ satisfaction with e-learning and may lead to negative attitudes or rejection of e-learning (Kauffman, 2015; Chen et al., 2020; Alqahtani et al., 2022). Additionally, a survey by Maria S Abbasi found that satisfaction with e-learning is higher in developed countries than in developing countries. Most participants agreed that e-learning provides satisfactory access to knowledge (Abbasi et al., 2020). Zhang et al. (2021) demonstrated a significant correlation between college students’ self-efficacy, motivation to learn, and student satisfaction. Consequently, it is crucial to explore and enhance the factors that enable learners to persist in e-learning in order to increase satisfaction. This will be particularly important in regional education systems with an equal level of economic development, as it will facilitate a successful educational process (Elshami et al., 2021).
1.2. Online education hotspot cluster analysis
In general, the number of articles related to online learning research has increased significantly due to the influence of COVID-19 from January 2020 to January 2023, as evidenced by records from Web of Science and PUB MED database. As shown in Figure 1, the research hotspots within this field, identified through keyword co-occurrence and clustering analysis, mainly revolve around the learning effect of e-learning, teaching strategies, independent learning, and student performance. However, despite the growing importance of online learning, little attention has been given to the factors that impact student satisfaction with their learning outcomes. Consequently, it is crucial to address this research gap. Notably, e-learning research has gained attention in various regions, such as North America (e.g., Canada), Europe (e.g., United Kingdom), Asia (e.g., China, India, Australia), and Africa. Building upon this background, the current study primarily aims to investigate the factors influencing satisfaction with e-learning outcomes comprehensively.
1.3. Satisfaction with the effectiveness of e-learning
Traditionally, a student’s subjective evaluation of their educational experience is known as student satisfaction (Elliot and Shin, 2002). Satisfaction is measured by students after performing a learning activity (Li, 2018). Student satisfaction reflects learning outcomes between students and teachers (Yunusa and Umar, 2021). Nelson sees learning satisfaction as a combination of good perceptions and positive attitudes (Nelson, 2022). This is because students can combine the two to meet their individual needs in the learning process. Students’ satisfaction with online learning requires advanced pedagogical methods and technological know-how to capture students’ attention and teaching (Baber, 2020). Learning satisfaction is a feeling and attitude toward the learning process; this feeling and attitude is formed by the pleasure students feel when the learning activity or process meets their physical and mental needs (Li, 2018). Gopal et al.’s (2021) study found that the perceived quality of the instructor, course design, instructor feedback, and student expectations were the most important predictors of student satisfaction. Satisfaction with the effectiveness of online learning among college students is an important variable to explore, which is important to facilitate the understanding of the current state of learning among college students.
1.4. Stimulus-Organism-Response (S-O-R) theoretical framework
The S-O-R model, developed by Mehrabian and Russell (1974), suggests that when a person is exposed to an external stimulus, certain cognitive or affective states will be evoked and an individual response will be triggered. This model involves three theoretical components: stimulus, organism, and response. It assumes that stimuli (S) in the external environment lead to changes in people’s internal organisms (O), which in turn affects their behavioral responses (R). The model has been widely used in various domains, including retail purchasing behavior, social media engagement, online user behavior, and educational instruction, to explain how external characteristics (i.e., stimulus variables) influence human internal thoughts (i.e., organism variables) and behavioral responses (i.e., response variables) (Zhao et al., 2020). In this theoretical framework, stimuli appear in different forms, such as environmental factors and interpersonal relationships (Animesh et al., 2011). As the global COVID-19 pandemic has led to a shift from offline to online classrooms, students are now forced to experiment with multimedia tools for learning (Khan et al., 2017). Yan Zhan and colleagues found that the S-O-R model is suitable for studying the context of online learning user behavior (Zhai et al., 2020). Their study concluded that the stimuli perceived during e-learning can be considered as stimuli from the external environment that stimulate the learner’s behavior, including motivation, learning strategies, and self-directed learning ability, and ultimately affect the learner’s learning outcomes.
1.5. LICE model of online learning influencing factors
The LICE model of factors affecting e-learning, proposed by Chinese scholar Jiahua Zhang, categorizes these factors into four aspects: Learner, Instructor, Curriculum, and Environment (referred to as the LICE model by Zhang and Jianping, 2009). Based on this model, along with related research both domestically and internationally, this study proposes a model that describes the factors affecting network learning, as depicted in Figure 2. The model divides the influencing factors into four aspects: Learner, Instructor, Learning platform, and Learning environment, leading to the abbreviation LIPE model. In this model, the learner factor serves as the key internal influencing factor of network learning, while the Instructor is an important factor. Additionally, the learning environment serves as a guarantee factor, and the learning platform acts as an auxiliary factor. These three factors together constitute the external influencing factors of network learning. It is the collaboration between the internal and external influencing factors that ultimately impacts the effectiveness of e-learning.
This study combines the research hotspots in the field of online education and integrates the structure of the mature LICE online education evaluation model. It introduces the LIPE model for online learning satisfaction assessment and focuses on exploring the comprehensive factors affecting students’ satisfaction with the effect of online learning. With the help of the Stimulus-Organism-Response (S-O-R) theoretical framework, it provides realistic strategies for adjusting the teaching strategy, improving the learning platform, and mobilizing the students’ motivation for online learning. The results of the study promote the long-lasting and sustainable development of online education and provide value for coping with the sudden change of the teaching mode in unexpected situations.
2. Method
2.1. Research model and hypothesis
Based on the S-O-R model, this study aimed to investigate the effects of learning platform quality, instructor teaching quality, and learning environment as stimulus variables on learners’ satisfaction with e-learning outcomes when they are engaged in e-learning. To further identify the biological variables in this study, we integrated the LICE model with the S-O-R model, according to which four variables (i.e., instructional teacher factors, learning platform factors, learning environment, and learner factors) were considered as biological and environmental variables in the S-O-R framework. Based on this, this study developed a research hypothesis model combining the LICE model and the S-O-R model, as shown in Figure 3. Based on the research model, several hypotheses were developed.
2.1.1. Relationship between the quality of teaching by lecturers and individual learner factors
In the process of E-learning, the teaching quality of educators plays a crucial role in influencing the effectiveness of online education. Zhang et al. (2020) argue that online teaching not only helps to reduce cross-transmission in traditional classrooms but also measures online student satisfaction, thus ensuring the quality of online teaching during a pandemic. The effect of E-learning on students is also influenced by various factors such as the teaching style of educators, their interaction with students, and the courseware and teaching materials used. Manou et al. (2021) propose that an increased frequency of instructor-student interactions in e-learning courses leads to better course quality and improved student learning outcomes. Additionally, Horntvedt et al. (2018) found that the use of interactive instructional strategies further enhances students’ knowledge and skills. Based on these findings, it can be concluded that there is a positive relationship between the teaching quality of educators and both learning motivation and learning strategies in the process of E-learning. Therefore, the following hypotheses are proposed:
H1a: There is a positive relationship between the teaching quality of educators and learning motivation in the process of E-learning.
H1b: There is a positive relationship between the teaching quality of educators and learning strategies.
2.1.2. The relationship between the quality of e-learning platform and the personal factors of learners
In China, online education began in the 1990s. The e-learning platform, known as a comprehensive teaching service support system, offers features such as teaching tutorials, online self-learning, and online teacher-student communication. It provides an environment for distance learning. The widespread adoption of online learning was accelerated by the impact of the COVID-19 pandemic on offline teaching in 2019. As a result, various online learning platforms, such as Learning Pass, MOOC, Tencent Meeting, Moodle, and others, have continued to improve their development. Research studies have demonstrated that students have no difficulty using these platforms. Moreover, the effectiveness of the learning platforms, coupled with positive communication between instructors and students, has led to students expressing their desire for online learning to continue even after the pandemic (Adeyeye et al., 2022). This is further supported by the findings of Ogura et al. (2018) who discovered that medical students’ learning outcomes can be effectively enhanced through the use of e-learning platforms. Similarly, Yakin and Linden (2021) found that students taught via e-learning platforms performed better on laboratory exams compared to those who were not.
H2a: There is a positive relationship between the quality of e-learning platforms and learning motivation.
H2b: A positive correlation between the quality of e-learning platforms and Personal learning strategies.
H2c: A positive relationship between the quality of e-learning platforms and self-efficacy.
2.1.3. The relationship between online learning environments and personal factors of learners
Bandura’s Ternary Interactive Determinism (TID) considers that the environment, individual cognition, and behavior are independent, interdependent, and mutually influential, and emphasizes the influence of the individual’s environment on his or her behavior (Bandura, 1977). Findings suggest that the learning environment is an important determinant of students’ cognitive and affective outcomes (Eom et al., 2006). Many studies confirm the relationship between students’ perceptions of the learning environment and their affective learning outcomes (Cho and Cho, 2014). Junqi Zhu and other scholars believe that the effect of college students’ online learning is mainly influenced by potential variables such as online learning behavior, online learning cognition, and online learning environment (Zhu et al., 2022). Therefore, the environmental factors of e-learning are included in the influences that affect the effectiveness of e-learning.
H3a: There is a positive relationship between the online learning environment and learning motivation.
H3b: There is a positive relationship between the online learning environment and Personal learning strategies.
H3c: Online learning environment has a positive relationship with self-efficacy.
2.1.4. The relationship between learning strategies, learning motivation, and satisfaction with the effect of online learning
E-learning includes online lectures, audio-visual aids (AVA), PowerPoint slides, and recorded video lessons (Baber, 2020). Students’ feelings, attitudes, and hopes about the quality of the learning environment as an expression of satisfaction with learning (Wu et al., 2010), and were widely used indicators of students’ attitudes toward the online learning process (Graham and Scarborough, 2001). Motivation for learning is one of the important variables in emotion-based research and refers to the internal (intrinsic) and external (extrinsic) forces that empower students to learn effectively (Keller, 2007). Learning strategies are the process of acquiring, organizing, or transforming information (Alexander et al., 1998). Studies have shown a significant positive correlation between students’ use of these strategies and learning satisfaction (Choi, 2016). Muis et al. (2015) found that by effectively using learning strategies to removes barriers to learning, reduces negative emotions, and allow students to enjoy learning. Chang et al. (2017) showed that interest in utilizing e-learning resources as well as their performance and motivation influenced their desire to embrace e-learning. Voils et al. (2019) found positive associations between the use of instructor-created supplemental activities and classroom test scores, self-regulated learning behaviors, and overall course performance.
H4: There is a positive correlation between learners' motivation and satisfaction with learning outcomes.
H5: There is a positive correlation between learners' learning strategies and satisfaction with learning outcomes.
2.1.5. The relationship between self-efficacy and satisfaction with learning outcomes
Self-efficacy theory was proposed by Bandura, an American psychologist, who suggested that the interaction between environment, behavior, and person affects individual behavior, emphasizing the mediating and regulating role of self-factors on behavior (Bandura, 1977). Self-efficacy indicates the degree to which an individual possesses the ability to manage a task or stress, and the concept is also related to motivation levels, actions, and psychological states (Zimmerman and Kulikowich, 2016). In their study of the relationship between motivation and academic performance among medical students, Wu Hongbin and other scholars found that both intrinsic and extrinsic motivation predicted self-efficacy (Wu et al., 2020).
H6: A positive relationship between self-efficacy and satisfaction with the effectiveness of online learning.
2.1.6. The mediating role of learning motivation, learning strategies, and self-efficacy
Bandura’s Ternary Interactive Determinism (TID) posits that the environment, individual cognition, and behavior mutually influence each other, indicating that the individual’s environment has a significant impact on their behavior (Bandura, 1977). Consistent with this perspective, Mohd Satar et al. (2021) discovered that e-learning satisfaction and key outcomes were mediated by learning stress and motivation among students. Furthermore, Doménech-Betoret et al. (2017) found that self-efficacy plays a crucial role in influencing academic performance. Based on these findings, we propose the following hypothesis:
H7: Learning environment influences satisfaction with the effectiveness of online learning through self-efficacy.
H8: Quality of e-learning platforms influences satisfaction with the effectiveness of online learning through learning strategies.
H9: The teaching quality of educators affects satisfaction with the effectiveness of online learning through learning motivation.
2.2. Participants
A total of 715 people, comprising of undergraduate students in grades 1 to 5 on campus at a medical university in Dalian, were surveyed between October 2021 and December 2022. Among the 602 recovered valid questionnaires, the effective response rate was 84.2%. The demographic information of the college students interviewed is shown in Table 1. Out of the respondents, 312 were male, representing 51.8%, while 290 were female, representing 48.2%. The majority of the respondents, 89.9%, belonged to the ethnic group, while the remaining 10.1% were from other ethnic minorities. In terms of academic year, 47.5% were freshmen, 14.8% were sophomores, 6.8% were juniors, 12.8% were seniors, and the remaining 18.1% were seniors and above. Medical students constituted the largest group, accounting for 45.6% of the respondents, followed by management students at 12.5%, art students at 6.6%, humanities and social sciences students at 5.3%, law students at 5.0%, science and engineering students at 8.1%, and literature students at 9.6%.
2.3. Research design
2.3.1. Sampling and questionnaire distribution
To investigate college students’ satisfaction with learning outcomes and their influencing factors, this study commenced with face-to-face interviews and the distribution of questionnaires using stratified random and purposive sampling. The samples of this study primarily consisted of undergraduate students in the first to fifth year of a medical university in Dalian. A total of 715 questionnaires were distributed to universities in schools, and 602 valid questionnaires were recovered, resulting in an effective response rate of 84.2%. The purpose of this study was to test the aforementioned hypotheses and explore the relationship between latent and observed variables.
2.3.2. Inclusion and exclusion criteria
The following data inclusion and exclusion criteria were set to ensure data validity and minimize errors:
1. The questionnaires were collected for undergraduate students in the first to fifth year of a medical university in Dalian.
2. Studying the impact of e-learning on satisfaction with learning outcomes, so traditional ways of learning are ruled out.
3. Data from college students who had engaged in online learning were included, and students who had never had online learning experience were excluded.
2.4. Research tool
The questionnaire was divided into two parts. The first part included demographic information such as gender, major, grade level, etc. The second part consists of four dimensions, including four parts: learning environment, teacher teaching, learning platform, and individual learner, which are used to measure students’ satisfaction with the effect of e-learning. All four dimensions were utilized in the Rilicet Response Scale. The questionnaire used in this study was designed according to the needs of the study, therefore the reliability and validity of the questionnaire were tested.
2.4.1. Questionnaire reliability test
In this study, the internal consistency method was used to test the reliability of the variables and to analyze the reliability of the questionnaire items. The alpha reliability coefficient of the measured variables was calculated to be 0.993, which is an acceptable value with α > 0.7 indicating high reliability of the questionnaire items.
2.4.2. Questionnaire validity test
The study used factor analysis to analyze the validity of the questionnaire scale construct using KMO and Bartlett’s test of sphericity. The results showed that the KMO value of the scale was 0.967 (>0.8), which is close to 1, indicating that the sample size meets the requirements and the data are suitable for factor analysis, while Bartlett’s test of the sphericity significance level of p = 0.000 < 0.01 indicates that the original variables are meaningful and the scale data are suitable for factor analysis.
In summary, the questionnaire has good reliability and validity.
2.5. Data collection and data analysis
Data were collected from October 2021–December 2022 and the questionnaires were all electronic and distributed through the WJX platform. The data were then entered and managed using Excel and analyzed using SPSS.22 for descriptive statistical analysis and correlation analysis. Finally, the hypothesized model was validated with the help of Smart PLS and using SEM analysis.
3. Results
3.1. Multivariate analysis
3.1.1. Measurement model evaluation
Structural equation modeling (SEM) allows the use of multiple observations for each latent variable to explain measurement error in both the dependent and independent variables (Levine et al., 2005). First, a correlated validated factor measurement model with 8 variables was constructed using Smart PLS 3.0. Secondly, SEM analysis was performed to measure the path coefficients as well as the fit coefficients of the models. To test the reliability of the scale this study was validated in terms of measuring the item aspects and latent variables, respectively. The measurement item aspect was assessed using factors and coefficients. As shown in Table 2, all projects have loads above the 2010 Hair et al. (2010) recommendation of 0.50 and were significant at the 0.001 level. Latent variable validation was measured using the combined reliability and Cronbach’s α values, and both the combined reliability and ɑ values were taken to be greater than the suggested threshold of 0.7, and the AVE values for all items were greater than 0.5.
3.1.2. Discriminant validity
Validity is a measure of whether a comprehensive evaluation system accurately reflects the purpose and requirements of the evaluation. It refers to the degree of accuracy in measuring the specific characteristics to be measured. Higher validity indicates a better ability of the measurement tool to label the intended characteristic, and vice versa. Discriminant validity (DV) can be used to quantify concepts that are not conceptually related to each other. Discriminant validation aims to provide evidence of discrimination based on differences between all components (Campbell and Fiske, 1959). The distinctiveness of validity can be measured using the square root of the average variance extracted (AVE) compared to the magnitude of the correlation coefficient value. In Table 3, correlation coefficients between the constructs that are less than the square root of the AVE indicate good discriminant validity. The value of convergent validity, measured by AVE, in Table 3 and Figure 4, ranged from 0.603–0.723, which is greater than 0.5. Therefore, it can be concluded that the model demonstrates good validity and reliability.
3.2. Structural equation model
3.2.1. Model fitting
Structural modeling is a theoretical framework for evaluating internal path models using structural equations (Skrondal and Rabe-Hesketh, 2004), assuming a series of regression equations to estimate the structural model coefficients of the inter-construct relationships. Model fit is measured using the Standardized Root Mean Square Residual (SRMR), a standardized residual index used to assess model fit, chi-square, and the Normal Fit Index (NFI) (Brown, 2006; Chen, 2007). The SRMR value compares the observed covariance with the predicted matrix, and the result is better when it is less than or equal to 0.08. The predicted SRMR value is 0.053, which is a good model fit; the NFI is 0.808; and the chi-square value is 1587.353, as shown in Table 4.
3.2.2. Model results
When evaluating structural relationships, it is important to check for covariance to ensure that it does not bias the regression results; the variance inflation factor (VIF) is commonly used to assess the covariance of exogenous structures (Hair et al., 2019). In the study, the VIF values for each construct were below the threshold value of 5.0 and very close to 3. This indicates that the problem of covariance between constructs does not exist and is in line with Hair et al.’s VIF cutoff of 5.0, which is in line with Hair et al.’s recommendation that the VIF scores should be close to 3 and that a lower value is needed. Table 5 and Figure 5 show that almost all exogenous variables have positive and statistically significant relationships with the hypothesized endogenous variables.
Based on the results presented in Figure 5 and Table 5, the quality of teacher’s instruction exhibits a positive correlation with both personal motivation to learn and personal learning strategies (β = 0.346, p < 0.001; β = 0.255, p < 0.001), thus confirming the validity of hypotheses H1a and H1b. Similarly, the quality of e-learning platforms demonstrates a positive correlation with individual motivation to learn, supporting hypothesis H2a (β = 0.245, p < 0.001). Additionally, the quality of e-learning platforms is positively associated with individual learning strategies and the efficacy of individual self-directed learning, reinforcing hypotheses H2b and H2c (β = 0.132, p < 0.005; β = 0.120, p < 0.005). Furthermore, a positive relationship emerges between the e-learning environment and learning motivation, learning strategies, and personal efficacy in self-directed learning, thereby confirming hypotheses H3a, H3b, and H3c (β = 0.247, p < 0.001; β = 0.341, p < 0.001; and β = 0.503, p < 0.001). Moreover, a positive correlation is observed between learning motivation and learning effectiveness, verifying hypothesis H4 (β = 0.113, p < 0.001). Similarly, a positive correlation exists between personal learning strategies and learning effectiveness, confirming hypothesis H5 (β = 0.238, p < 0.001). Finally, a positive correlation is found between personal independent learning efficacy and learning effectiveness, supporting hypothesis H6 (β = 0.612, p < 0.001).
3.2.3. Intermediary role
To examine the mediating role of learning strategies, motivation, and self-learning effectiveness, Baron and Kenny’s (1986) research process was employed in this study. The independent variables included learning environment, quality of learning platform, and quality of teachers’ teaching, while the mediating variables were learning strategies, motivation, and self-learning efficacy. The dependent variable was satisfaction with learning outcomes. Data analysis was conducted using Smart PLS 3.0, with the mediation effect assessed through Bootstrapping at a 95% confidence interval. The results in Table 6 show a positive correlation between the learning environment and personal independent learning efficacy (β = 0.503, p < 0.001), as well as between personal independent learning efficacy and satisfaction with learning outcomes (β = 0.612, p < 0.001). Furthermore, the quality of the learning platform had a positive effect on online learning strategies (β = 0.132, p < 0.001), which in turn positively influenced satisfaction with learning outcomes (β = 0.238, p < 0.001). Similarly, teacher teaching quality had a positive effect on online learning motivation (β = 0.346, p < 0.001), and online learning motivation had a positive impact on satisfaction with learning outcomes (β = 0.113, p < 0.001). The assessment of specific indirect effects in Table 7 revealed that self-learning efficacy mediated the relationship between the learning environment and satisfaction with learning outcomes (β = 0.089, p < 0.001), supporting research hypothesis H7. Additionally, learning strategies mediated the relationship between the quality of learning platforms and satisfaction with learning outcomes (β = 0.031, p < 0.001), confirming research hypothesis H8. Moreover, motivation played a significant mediating role in the relationship between teachers’ teaching quality and satisfaction with learning outcomes (β = 0.039, p < 0.001), establishing research hypothesis H9.
4. Discussion
With the continued resurgence or crossover of different risk pandemics, many schools are rapidly adopting online delivery models, enhancing the planning of e-learning technology and systems platforms so that students can continue to receive their education (Wang et al., 2022). This not only changes the traditional mode of learning for students but also makes the continued implementation of online learning an important issue for the sustainability of education in the post-epidemic era. Since the end of 2021, the associated risks and regular vaccinations have had a significant impact on the social and economic livelihoods of individuals in the post-COVID-19 era (Cheng et al., 2021), including in the area of online education. Therefore, exploring the factors that influence students to engage in online learning has become an important research topic. The results of this study showed that the quality of teachers’ teaching, the learning environment, the quality of the learning platform, and personal factors (learning motivation, learning strategies, and self-efficacy) have a great impact on the satisfaction of e-learning outcomes. Taking the students of a medical university in Dalian as a sample, the S-O-R model was used to conduct a substantial study to explore the influence of the above factors on the satisfaction of the e-learning effect. This study fills the gap of the influence factors of college students’ online learning affect satisfaction in the context of online learning (Zhang et al., 2021).
4.1. Factors affecting satisfaction with e-learning outcomes
4.1.1. Personal factors influencing the effectiveness of online learning among college students
The results of this study indicate that learners’ personal motivation, learning strategies, and self-efficacy when engaging in e-learning have an impact on learners’ satisfaction with learning outcomes. In a study on the impact of college students’ adaptability to blended learning in English, Shuhan Yang and other scholars also found that self-efficacy and motivation had a significant positive impact on the adaptability of non-English majors to the blended learning mode of English courses (Yang and Pu, 2022). Therefore, individual learner factors are crucial in influencing the satisfaction of e-learning outcomes and are very well guided in enhancing and promoting the user experience of the blended learning services offered. In addition, it was found that many students actively used e-learning platforms to acquire knowledge to further their studies and obtain certificates, etc. Interestingly, such groups tended to report higher e-learning effectiveness and satisfaction than those who passively accepted e-learning platforms (Figure 6). According to the results of the study we found that there is a significant positive correlation between motivation, learning strategies, self-efficacy, and e-learning effectiveness, which is consistent with the findings of Wlodkowski (1999), Honicke and Broadbent (2016) and other scholars. It was also found that motivation, learning strategies, and self-efficacy moderated the relationship between the quality of the e-learning platform, the quality of the instructor’s teaching, and the learning environment and satisfaction with the e-learning outcomes, which is consistent with the findings of Kuo et al. (2014), Bandura (1977), and Borkotoky and Borah (2021). Therefore, lecturers or learners can increase internal and external incentives to enhance the perceived value of e-learning during the process of e-learning (Yahiaoui et al., 2022). It is recommended that lecturers in online courses give comprehensive evaluations based on the number of interactive exchanges on the platform, students’ enthusiasm in answering questions, the status of group discussions, and appropriate rewards based on the evaluations to enhance students’ satisfaction and pride. Secondly, it should pay close attention to the learning needs of students, such as the needs of college students to find a job, the demand for graduate school, the demand for bonuses, etc., which can be adjusted according to the different needs of the students of the network courses, to stimulate the enthusiasm of the students of network learning (Zhang et al., 2021).
4.1.2. External factors affecting the effectiveness of college students’ online learning
Since 2020, the rapid development of e-learning platforms such as Tencent conference, MOOC, Rain Classroom, and Nail has provided students with a good learning platform. However, the quality of these platforms varies, and improvement is needed (Yuanyuan, 2022). This study has identified external factors that can directly or indirectly influence college students’ satisfaction with e-learning. These factors include the quality of the e-learning platform, the e-learning environment, and teacher support. Among these factors, the quality of the e-learning platform and teacher support are the most significant (Herrador-Alcaide et al., 2020). Therefore, long-lasting e-learning platforms should promptly adapt to societal and students’ learning needs by implementing technological changes and updating shared learning resources. Additionally, they should always maintain the innovative and social nature of e-learning resources. Furthermore, in terms of teacher support, interviews with students revealed that the teacher’s delivery style, choice of materials, teaching strategies, and proficiency in instructional tools significantly impact students’ satisfaction with learning outcomes, which supports the findings of Al Mamun et al. (2020) and Fraser et al. (1986). Hence, lecturers should strengthen interactive communication with students (Zhang et al., 2017), utilize pop-up functions and online Q&A during online lectures to encourage student participation and cultivate their interest. Moreover, teachers should adjust their teaching methods to enhance student engagement and optimize lesson design (Wang et al., 2021).
Scholars such as Allen and Fraser (2007) have identified the learning environment as an important factor influencing the effectiveness of online learning. In terms of family environment, due to the COVID-19 epidemic, college students are currently constrained to their homes, making the family environment the primary setting for their learning (Nainggolan, 2021). It is crucial to create a quiet and comfortable learning environment within the family. Furthermore, parents and teachers should communicate promptly with students and enhance supervision to improve the effectiveness of learning (Playle and Mullarkey, 1998). When online learning is implemented in schools, it is vital to promptly establish improved corresponding policies, enhance supporting measures such as software and hardware suitable for online learning, and closely monitor the psychological and physical changes of students (Zhai et al., 2020). These actions will contribute to the creation of a favorable learning environment.
4.2. LICE model combined with S-O-R model to explain the effects of factors on satisfaction with online learning effectiveness
This study constructs the LIPE model, which combines the LICE model with the S-O-R model, to explore how students’ satisfaction with learning can be improved from various perspectives. In the LICE theoretical model, the individual factor is considered the key factor, the instructor factor is an important factor, and the learning environment acts as a safeguard. Additionally, the learning platform serves as a supporting factor, and these various influences interact with each other (Lin et al., 2020). The inclusion of the S-O-R model in this integrated model provides a more scientifically rigorous explanation of the relationship between these influences. It elucidates the changes in students’ psychological cognition during the learning process, as well as their subsequent learning intentions and behavioral responses. Moreover, it better explains how adjustments and refinements of external influences, along with the internal factors of college students, contribute to the enhancement of learning satisfaction in an environment that receives external stimuli. This finding is consistent with the conclusions drawn by Guihua Zhang and other scholars (Zhang et al., 2021).
The results of the study showed that the changes in the learning environment, learning styles, learning methods, teaching methods, and other factors, as well as the different learning motives, learning strategies, and learning abilities of college students themselves, have led to changes in satisfaction with the effectiveness of e-learning.
4.3. E-learning applications in education
In December 2022, Chinese provinces gradually abolished the nucleic acid standing inspection, and the social side gradually opened up the epidemic control, while countries such as the United States had already begun to liberalize the restrictions on the prevention and control of epidemics long before that. The COVID-19 pandemic is over, but public health emergencies and damage caused by unforeseen factors are unpredictable. Therefore, the end of the pandemic has translated these lessons into clear guidelines and practices so that preventive and corrective actions can be taken promptly when exceptional circumstances arise. Figure 7 shows a sharp increase in the number of online learners in the network from 2019 until 2022. Network learning has become a college student learning life and should not be taken as a lesser method of learning. With students returning to offline learning, the question arises about the future of online learning and whether it should be abandoned. However, more than three-quarters of teachers preferred online instruction during the pandemic, and in the post-pandemic era, most prefer physical and blended instruction (Saha et al., 2022). Therefore, the development of online learning needs to change with the times. It is necessary to combine traditional learning methods with online learning to create a comprehensive approach (Edem Adzovie and Jibril, 2022).
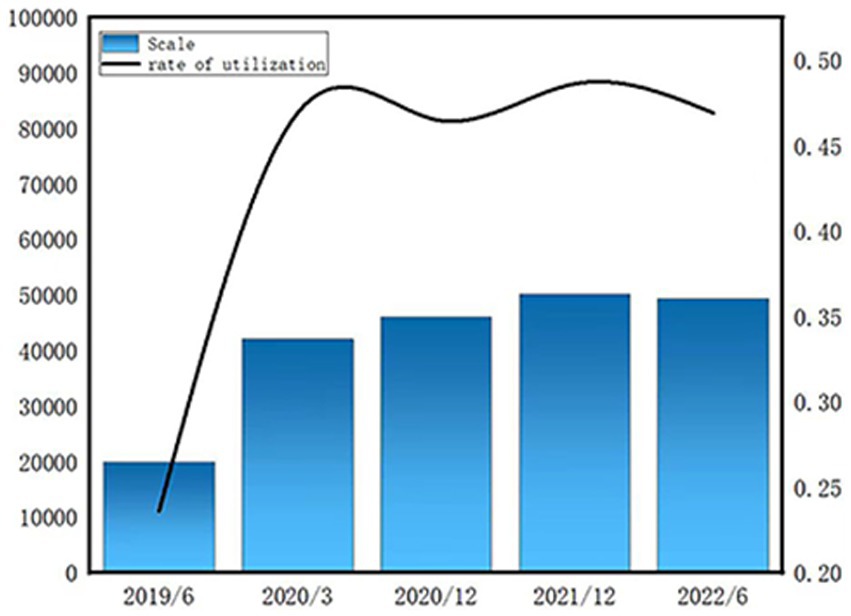
Figure 7. Scale and usage of online education from 2020 to 2022. Data from China’s 48th, 49th and 50th statistical report on the development of the Internet in China.
5. Conclusion
The main purpose of this study was to investigate the factors that influence satisfaction with online learning outcomes. It primarily used structural equation modeling to analyze the effects of various factors on e-learning satisfaction. Specifically, the study examined the quality of e-learning platforms, the quality of teachers’ teaching, the e-learning environment, individual motivation in e-learning, learning strategies, and the effectiveness of self-directed learning. According to previous research, the quality of e-learning platforms, the quality of teachers’ teaching, the e-learning environment, and personal factors are all key determinants of satisfaction with e-learning outcomes. The study also revealed that college students’ e-learning motivation, learning strategies, and self-directed learning efficacy serve as mediators in their evaluation of e-learning satisfaction.
This study further verifies the deepening and presents the LIPE model, which was created based on the LICE model developed by Zhang Jiahua scholars. The LIPE model demonstrates that the learner’s factors, such as e-learning motivation, learning strategies, and self-learning efficacy, contribute to the internal factors that enhance the effectiveness of network learning. On the other hand, the quality of the network learning platform, the quality of the teacher’s lectures, and the network learning environment are identified as external factors that affect network learning effectiveness. The study reveals that a higher quality e-learning platform, better teacher instruction, and improved learning environment result in better e-learning outcomes. Additionally, the study highlights that e-learning motivation, learning strategies, and self-learning efficacy have a significant positive impact on learning effectiveness. These findings provide valuable insights for students themselves, as well as for improving school policies, enhancing the learning environment, developing online teaching platforms, refining teaching methods, and enhancing teaching quality.
According to research, e-learning, although it was imposed as a solution in a particular era, has always existed as a convenient way of learning and will continue to exist beyond this era. Therefore we should maximize the benefits of each learning method and keep optimizing it to minimize the drawbacks of the learning process.
5.1. Limitations and future directions
When evaluating the current study, several limitations need to be addressed. Firstly, the study only investigated undergraduate students, excluding master’s degree, college, and junior and senior high school students. This limitation affects the perspective and scope of the study. In future research, it would be beneficial to include additional levels of scope to provide a more comprehensive understanding of the topic. Another limitation of the study is that it is a longitudinal study spanning two periods during and at the end of the COVID-19 epidemic. To ensure a more accurate examination of the e-learning status of college students, it is recommended to extend the observation period to continuously track their experiences over a longer period. This would allow for a more robust understanding of changes in satisfaction with e-learning effects. Furthermore, the study primarily focuses on four aspects: the quality of the e-learning platform, the quality of the teacher’s instruction, the e-learning environment, and personal factors. However, it is important to note that satisfaction with e-learning effects is influenced by various factors. Therefore, future studies should consider incorporating more refined and comprehensive factors to provide a more nuanced analysis.
Data availability statement
The raw data supporting the conclusions of this article will be made available by the authors, without undue reservation.
Ethics statement
Ethical review and approval were not required for the study on human participants by the local legislation and institutional requirements. The patients/participants provided their written informed consent to participate in this study. Written informed consent was obtained from the individual (s) for the publication of any potentially identifiable images or data included in this article.
Author contributions
XD, RRW, and XFH participated in the design, investigation, data analysis, and writing of the manuscript. XXW, YTH, YL, YW, and CYG participated in data collection and analysis. RQK participated in the investigation and evaluation of the study. YZ and BG participated in the design, investigation, and evaluation of the study and contributed to critical revision. All authors contributed to the article and approved the submitted version.
Funding
Research on the Construction of a Regional Joint Prevention and Control System for Public Health Emergencies in Liaoning Province Based on Blockchain Technology, LJKZR20220102, Liaoning Provincial Department of Education 2022 Basic Scientific Research Projects for Universities, Key Research Project, 25,000 supported by the university, 2022.11; Impact of Cross-border Movement of People and Management Countermeasures in the Perspective of Prevention and Control of New Coronary Pneumonia Epidemic, 21ZSYZYB16, Higher Education Scientific Research Planning Project of China Higher Education Society in 2022, 2021, 12; Teaching Path of SPOC Mode Interaction Design under OBE Concept, General Project of Liaoning Province General Higher Education Undergraduate Teaching Reform Research in 2021, Project No. 542, 2021.12–2023.12; path of improving the competitiveness of medical college discipline culture under the view of three comprehensive education, 2022lslwzzkt-011, Liaoning Province Social Science Association Project. 2021.08-2022.08 (2022 reported workload) 2022 Liaoning Province Economic and Social Development Research Project; and Optimization research on the supervision and early warning of the new crown pneumonia epidemic in Liaoning Province based on data mining technology and LSTM network, 2020 scientific and technological activities support project for the candidates of the province’s “100 million talents project,” Liaoning Provincial Department of Human Resources and Social Security, 2021.09-2022.09.
Conflict of interest
The authors declare that the research was conducted in the absence of any commercial or financial relationships that could be construed as a potential conflict of interest.
Publisher’s note
All claims expressed in this article are solely those of the authors and do not necessarily represent those of their affiliated organizations, or those of the publisher, the editors and the reviewers. Any product that may be evaluated in this article, or claim that may be made by its manufacturer, is not guaranteed or endorsed by the publisher.
References
Abbasi, M. S., Ahmed, N., Sajjad, B., Alshahrani, A., Saeed, S., Sarfaraz, S., et al. (2020). E-learning perception and satisfaction among health sciences students amid the COVID-19 pandemic. Work 67, 549–556. doi: 10.3233/WOR-203308
Adeyeye, B., Ojih, S. E., Bello, D., Adesina, E., Yartey, D., Ben-Enukora, C., et al. (2022). Online learning platforms and covenant university students’ academic performance in practical related courses during COVID-19 pandemic. Sustainability 14:878. doi: 10.3390/su14020878
Alexander, P. A., Graham, S., and Harris, K. R. (1998). A perspective on strategy research: progress and prospects. Educ. Psychol. Rev. 10, 129–154. doi: 10.1023/A:1022185502996
Allen, D., and Fraser, B. J. (2007). Parent and student perceptions of classroom learning environment and its association with student outcomes. Learn. Environ. Res. 10, 67–82. doi: 10.1007/s10984-007-9018-z
Allen, I. E., and Seaman, J. (2010). Learning on demand: online education in the United States, 2009. Newburyport, MA: ERIC.
Al Mamun, M. A., Lawrie, G., and Wright, T. (2020). Instructional design of scaffolded online learning modules for self-directed and inquiry-based learning environments. Comput. Educ. 144:103695. doi: 10.1016/j.compedu.2019.103695
Alqahtani, T. M., Yusop, F. D., and Halili, S. H. (2022). Dataset on the relationships between flipped classroom approach, students’ learning satisfaction and online learning anxiety in the context of Saudi Arabian higher education institutions. Data Brief 45:108588. doi: 10.1016/j.dib.2022.108588
Alqurashi, E. (2016). Self-efficacy in online learning environments: a literature review. Contemp. Issues Educ. Res. 9, 45–52. doi: 10.19030/cier.v9i1.9549
Animesh, A., Pinsonneault, A., Yang, S.-B., and Oh, W. (2011). An odyssey into virtual worlds: exploring the impacts of technological and spatial environments on intention to purchase virtual products. MIS Q. 35, 789–810. doi: 10.2307/23042809
Baber, H. (2020). Determinants of students' perceived learning outcome and satisfaction in online learning during the pandemic of COVID-19. Educat. e-Learn 7, 285–292. doi: 10.20448/journal.509.2020.73.285.292
Bandura, A. (1977). Self-efficacy: toward a unifying theory of behavioral change. Psychol. Rev. 84, 191–215. doi: 10.1037/0033-295X.84.2.191
Bao, W. (2020). COVID-19 and online teaching in higher education: a case study of Peking University. Hum. Behav. Emerg. Technol. 2, 113–115. doi: 10.1002/hbe2.191
Baron, R. M., and Kenny, D. A. (1986). The moderator-mediator variable distinction in social psychological research: Conceptual, strategic, and statistical considerations. J. Pers. Soc. Psychol. 51, 1173–1182.
Borkotoky, D. K., and Borah, G. (2021). The impact of online education on the university students of Assam in covid times. Indian J. Sci. Technol. 14, 1028–1035. doi: 10.17485/IJST/v14i13.1809
Brown, T. A . (2006). Confirmatory factor analysis for applied research. New York, NY: Guilford Publications
Campbell, D. T., and Fiske, D. W. (1959). Convergent and discriminant validation by the multitrait-multimethod matrix. Psychol. Bull. 56, 81–105. doi: 10.1037/h0046016
Chang, H. H., Fu, C. S., and Huang, C. Y. (2017). Willingness to adopt or reuse an e-learning system: the perspectives of self-determination and perceived characteristics of innovation. Innov. Educ. Teach. Int. 54, 511–520. doi: 10.1080/14703297.2016.1194768
Chen, F. F. (2007). Sensitivity of goodness of fit indexes to lack of measurement invariance. Struct. Equ. Model. 14, 464–504. doi: 10.1080/10705510701301834
Cheng, Y., Liu, H., Wang, S., Cui, X., and Li, Q. (2021). Global action on SDGs: policy review and outlook in a post-pandemic era. Sustainability 13:6461. doi: 10.3390/su13116461
Chen, T., Peng, L., Yin, X., Rong, J., Yang, J., and Cong, G. (2020). Analysis of user satisfaction with online education platforms in China during the COVID-19 pandemic. Healthcare 8:200. doi: 10.3390/healthcare8030200
Choi, B. (2016). How people learn in an asynchronous online learning environment: the relationships between graduate students’ learning strategies and learning satisfaction | comment apprennent les gens dans un environnement d’apprentissage en ligne asynchrone. CJLT/RCAT 42. doi: 10.21432/T24K7R
Cho, M. H., and Cho, Y. (2014). Instructor scaffolding for interaction and students’ academic engagement in online learning: mediating role of perceived online class goal structures. Internet High. Educ. 21, 25–30. doi: 10.1016/j.iheduc.2013.10.008
Dai, D., and Lin, G. (2020). Online home study plan for postponed 2020 spring semester during the COVID-19 epidemic: a case study of Tangquan middle school in Nanjing, Jiangsu Province, China. Best Evid. Chin Educ. 4, 543–547.
Doménech-Betoret, F., Abellán-Roselló, L., and Gómez-Artiga, A. (2017). Self-efficacy, satisfaction, and academic achievement: the mediator role of students' expectancy-value beliefs. Front. Psychol. 8:1193. doi: 10.3389/fpsyg.2017.01193
Edem Adzovie, D., and Jibril, A. B. (2022). Assessment of the effects of COVID-19 pandemic on the prospects of e-learning in higher learning institutions: the mediating role of academic innovativeness and technological growth. Cogent Educ. 9:2041222. doi: 10.1080/2331186X.2022.2041222
Elliot, K. M., and Shin, D. (2002). Student satisfaction: an alternative approach to assessing this important concept. J. High. Educ. Policy Manag. 24, 197–209. doi: 10.1080/1360080022000013518
Elshami, W., Taha, M. H., Abuzaid, M., Saravanan, C., Al Kawas, S., and Abdalla, M. E. (2021). Satisfaction with online learning in the new normal: perspective of students and faculty at medical and health sciences colleges. Med. Educ. Online 26:1920090. doi: 10.1080/10872981.2021.1920090
Eom, S. B., Josephwen, H., and Ashill, N. (2006). The determinants of students’ perceived learning outcomes and satisfaction in university online education: an empirical investigation. Decis. Sci. 4, 215–235. doi: 10.1111/dsji.12097
Fraser, B. J., Treagust, D. F., and Dennis, N. C. (1986). Development of an instrument for assessing classroom psychosocial environment at universities and colleges. Stud. High. Educ. 11, 43–54. doi: 10.1080/03075078612331378451
Gopal, R., Singh, V., and Aggarwal, A. (2021). Impact of online classes on the satisfaction and performance of students during the pandemic period of COVID 19. Educ. Inf. Technol. 26, 6923–6947. doi: 10.1007/s10639-021-10523-1
Graham, M., and Scarborough, H. (2001). Enhancing the learning environment for distance education students. Distance Educ. 22, 232–244. doi: 10.1080/0158791010220204
Hair, J. F., Risher, J. J., Sarstedt, M., and Ringle, C. M. (2019). When to use and how to report the results of PLS-SEM. Eur. Bus. Rev. 31, 2–24. doi: 10.1108/EBR-11-2018-0203
Hair, Joseph F, Anderson, Rolph E, Babin, Barry J, and Black, William C (2010). Multivariate data analysis: a global perspective. Upper Saddle River, NJ: Pearson Education Inc.
Herrador-Alcaide, T. C., Hernández-Solís, M., and Hontoria, J. F. (2020). Online learning tools in the era of m-learning: utility and attitudes in accounting college students. Sustainability 12:5171. doi: 10.3390/su12125171
Honicke, T., and Broadbent, J. (2016). The influence of academic self-efficacy on academic performance: a systematic review. Educ. Res. Rev. 17, 63–84. doi: 10.1016/j.edurev.2015.11.002
Horntvedt, M.-E. T., Nordsteien, A., Fermann, T., and Severinsson, E. (2018). Strategies for teaching evidence-based practice in nursing education: a thematic literature review. BMC Med. Educ. 18, 1–11. doi: 10.1186/s12909-018-1278-z
Kauffman, H. (2015). A review of predictive factors of student success in and satisfaction with online learning. Res. Learn. Technol. 23:26507. doi: 10.3402/rlt.v23.26507
Keller, J. M. (2007). The systematic process of motivational design. Perform. Improv. 26, 1–8. doi: 10.1002/pfi.4160260902
Khan, I. U., Hameed, Z., Yu, Y., and Khan, S. U. (2017). Assessing the determinants of flow experience in the adoption of learning management systems: the moderating role of perceived institutional support. Behav. Inform. Technol. 36, 1162–1176. doi: 10.1080/0144929X.2017.1362475
Kuo, Y. C., Walker, A., Belland, B., Schroder, K., and Kuo, Y. T. (2014). A case study of integrating interwise: interaction, internet self-efficacy, and satisfaction in synchronous online learning environments. Int. Rev. Res. Open Distrib. Learn. 15, 161–181. doi: 10.19173/irrodl.v15i1.1664
Levine, S. Z., Petrides, K. V., Davis, S., Jackson, C. J., and Howell, P. (2005). The use of structural equation modeling in stuttering research: concepts and directions. Stammering Res. 1, 344–363.
Lin, J., Lin, S., Turel, O., and Xu, F. (2020). The buffering effect of flow experience on the relationship between overload and social media users’ discontinuance intentions. Telematics Inform. 49:101374. doi: 10.1016/j.tele.2020.101374
Li, Y. (2018). Effects of the application of mobile learning to criminal law education on learning attitude and learning satisfaction. Technol. Educ. 14, 3355–3362. doi: 10.29333/ejmste/91730
Ma, J. (2017). The study on design and development of network multi-medium teaching system based on bs mode. Agro Food Ind Hi Tech 28, 1532–1535.
Manou, E., Lazari, E.-C., Thomopoulou, G.-E., Agrogiannis, G., Kavantzas, N., and Lazaris, A. C. (2021). Participation and interactivity in synchronous e-learning pathology course during the COVID-19 pandemic. Adv. Med. Educ. Pract. 12, 1081–1091. doi: 10.2147/AMEP.S317854
Mehrabian, A., and Russell, J. A. (1974). An approach to environmental psychology. Cambridge, MA: MIT Press.
Mohd Satar, N. S., Dastane, D. O., and Morshidi, A. H. (2021). E-learning satisfaction during COVID-19 pandemic lockdown: analyzing key mediators. Int. J. Manag. Acc. Econ. 8, 542–560. doi: 10.5281/zenodo.5731664
Muis, K. R., Pekrun, R., Sinatra, G. M., Azevedo, R., Trevors, G., Meier, E., et al. (2015). The curious case of climate change: testing a theoretical model of epistemic beliefs, epistemic emotions, and complex learning. Learn. Instr. 39, 168–183. doi: 10.1016/j.learninstruc.2015.06.003
Nainggolan, S. (2021). Evaluating of digital platforms related online learning during Covid-19 pandemic: students’ satisfaction view. AL-ISHLAH Jurnal Pendidikan 13, 1358–1365.
Nelson, C (2022). Continued growth in accessibility of AACSB-accredited online degrees. Publication no. Available at: http://aacsbblogs.typepad.com/dataandresearch/2016/10/index.html.
Ogura, A., Hayashi, N., Negishi, T., and Watanabe, H. (2018). Effectiveness of an e-learning platform for image interpretation education of medical staff and students. J. Digit. Imaging 31, 622–627. doi: 10.1007/s10278-018-0095-6
Playle, J. F., and Mullarkey, K. (1998). Parallel process in clinical supervision: enhancing learning and providing support. Nurse Educ. Today 18, 558–566. doi: 10.1016/S0260-6917(98)80006-3
Pokhrel, S., and Chhetri, R. (2021). A literature review on impact of COVID-19 pandemic on teaching and learning. Higher Educ. Fut. 8, 133–141. doi: 10.1177/2347631120983481
Saha, S. M., Pranty, S. A., Rana, M. J., Islam, M. J., and Hossain, M. E. (2022). Teaching during a pandemic: do university teachers prefer online teaching? Heliyon 8:e08663. doi: 10.1016/j.heliyon.2021.e08663
Skrondal, A, and Rabe-Hesketh, S (2004). Generalized latent variable modeling: multilevel, longitudinal, and structural equation models. 1st Ed. New York, NY: Chapman and Hall/CRC.
Voils, S. A., Childs-Kean, L. M., and Thomas, A. (2019). Relationship between pharmacy students’ use of self-regulated learning strategies and course outcomes. Am. J. Pharm. Educ. 83:7566. doi: 10.5688/ajpe7566
Wang, R., Han, J., Gao, C., and Liu, C. (2021). Chinese university students’ perceptions of facilitation strategies, learning motivation, and satisfaction in cloud-based virtual classrooms. Front. Psychol. 12:801191. doi: 10.3389/fpsyg.2021.801191
Wang, S., Shi, G., Lu, M., Lin, R., and Yang, J. (2021). Determinants of active online learning in the smart learning environment: an empirical study with PLS-SEM. Sustainability 13:9923. doi: 10.3390/su13179923
Wang, Z., Qadir, A., Asmat, A., Aslam Mian, M. S., and Luo, X. (2022). The advent of coronavirus disease 2019 and the impact of mobile learning on student learning performance: the mediating role of student learning behavior. Front. Psychol. 12:796298. doi: 10.3389/fpsyg.2021.796298
Wlodkowski, R. J. (1999). Enhancing adult motivation to learn: a comprehensive guide for teaching all adults, 2nd Edn. San Francisco, CA: Jossey-Bass Higher and Adult Education Series.
Wu, H., Li, S., Zheng, J., and Guo, J. (2020). Medical students’ motivation and academic performance: the mediating roles of self-efficacy and learning engagement. Med. Educ. Online 25:1742964. doi: 10.1080/10872981.2020.1742964
Wu, J.-H., Tennyson, R. D., and Hsia, T.-L. (2010). A study of student satisfaction in a blended e-learning system environment. Comput. Educ. 55, 155–164. doi: 10.1016/j.compedu.2009.12.012
Xie, X., Siau, K., and Nah, F. F.-H. (2020). COVID-19 pandemic–online education in the new normal and the next normal. J. Inform. Technol. Case Appl. Res. 22, 175–187. doi: 10.1080/15228053.2020.1824884
Yahiaoui, F., Aichouche, R., Chergui, K., Brika, S. K. M., Almezher, M., Musa, A. A., et al. (2022). The impact of e-learning systems on motivating students and enhancing their outcomes during COVID-19: a mixed-method approach. Front. Psychol. 13:874181. doi: 10.3389/fpsyg.2022.874181
Yakin, M., and Linden, K. (2021). Adaptive e-learning platforms can improve student performance and engagement in dental education. J. Dent. Educ. 85, 1309–1315. doi: 10.1002/jdd.12609
Yang, S., and Pu, R. (2022). The effects of contextual factors, self-efficacy and motivation on learners’ adaptability to blended learning in college English: a structural equation modeling approach. Front. Psychol. 13:847342. doi: 10.3389/fpsyg.2022.847342
Yuanyuan, Z. (2022). MOOC teaching model of basic education based on fuzzy decision tree algorithm. Comput. Intell. Neurosci. 2022, 1–7. doi: 10.1155/2022/3175028
Yunusa, A. A., and Umar, I. N. (2021). A scoping review of critical predictive factors (CPFs) of satisfaction and perceived learning outcomes in E-learning environments. Educ. Inf. Technol. 26, 1223–1270. doi: 10.1007/s10639-020-10286-1
Zhai, X., Wang, M., and Ghani, U. (2020). The SOR (stimulus-organism-response) paradigm in online learning: an empirical study of students’ knowledge hiding perceptions. Interact. Learn. Environ. 28, 586–601. doi: 10.1080/10494820.2019.1696841
Zhang, D. J., Allon, G., and Van Mieghem, J. A. (2017). Does social interaction improve learning outcomes? Evidence from field experiments on massive open online courses. Manuf. Serv. Oper. Manag. 19, 347–367. doi: 10.1287/msom.2016.0615
Zhang, G., Yue, X., Ye, Y., and Peng, M. Y.-P. (2021). Understanding the impact of the psychological cognitive process on student learning satisfaction: combination of the social cognitive career theory and SOR model. Front. Psychol. 12:712323. doi: 10.3389/fpsyg.2021.712323
Zhang, J. Z., and Jianping,. (2009). Influencing factors of e-learning and its LICE model. Electrochemical Education Research.
Zhang, T. (2021). Chinese parents’ perception of emergency remote K-12 teaching-learning in China during the COVID-19 pandemic. Asian J. Dis. Educ. 16:16.
Zhang, Y., Zhang, P., Yang, H., Zhao, K., and Han, C. (2020). Influencing factors of students’ online learning satisfaction during the COVID-19 outbreak: An empirical study based on random forest algorithm. Paper Presented at the International Symposium on Emerging Technologies for Education, Cham.
Zhao, Y., Wang, A., and Sun, Y. (2020). Technological environment, virtual experience, and MOOC continuance: a stimulus–organism–response perspective. Comput. Educ. 144:103721. doi: 10.1016/j.compedu.2019.103721
Zhou, L., Wu, S., Zhou, M., and Li, F. (2020). 'School’s out, but class’ on', the largest online education in the world today: taking China’s practical exploration during the COVID-19 epidemic prevention and control as an example. Best. Evid. Chin. Educ. 4, 501–519. doi: 10.15354/bece.20.ar023
Zhu, J., Zhao, H., Wang, X., Yang, L., Qin, Z., and Geng, J. (2022). Effects of online learning on college students in eastern China: a structural equation model. Front. Public Health 10:853928. doi: 10.3389/fpubh.2022.853928
Keywords: S-O-R model, LICE model, online learning, learning motivation, online learning effect satisfaction
Citation: Dai X, Wang Rr, Huang Xf, Wang Xx, Huang Yt, Li Y, Wu Y, Guan Cy, Kazembe RQ, Zhang Y and Gao B (2024) An analytical model of college students’ self-assessed satisfaction with the effectiveness of online learning: a structural equation model integrating LICE and S-O-R models. Front. Psychol. 14:1248729. doi: 10.3389/fpsyg.2023.1248729
Edited by:
Urvashi Tandon, Chitkara University, IndiaReviewed by:
Ramazan Yilmaz, Bartin University, TürkiyeMuhammad Azeem Ashraf, Hunan University, China
Copyright © 2024 Dai, Wang, Huang, Wang, Huang, Li, Wu, Guan, Kazembe, Zhang and Gao. This is an open-access article distributed under the terms of the Creative Commons Attribution License (CC BY). The use, distribution or reproduction in other forums is permitted, provided the original author(s) and the copyright owner(s) are credited and that the original publication in this journal is cited, in accordance with accepted academic practice. No use, distribution or reproduction is permitted which does not comply with these terms.
*Correspondence: Yuanyuan Zhang, zhangyuan7981@126.com; Bo Gao, gaobo_915@126.com
†These authors have contributed equally to this work