- 1School of Psychology, University of Auckland, Auckland, New Zealand
- 2Division of Child and Adolescent Psychiatry, Semel Institute for Neuroscience and Human Behavior, University of California, Los Angeles, Los Angeles, CA, United States
- 3Department of Psychiatry and Psychotherapy, Jena University Hospital, Jena, Germany
- 4Department of Neurology, Jena University Hospital, Jena, Germany
- 5Department of Neurology, University of California, Los Angeles, Los Angeles, CA, United States
- 6Brain Research Institute, University of California, Los Angeles, Los Angeles, CA, United States
- 7Laboratory of Neuro Imaging, School of Medicine, University of Southern California, Los Angeles, CA, United States
- 8Department of Women’s and Children’s Health, Uppsala University, Uppsala, Sweden
Attention-deficit hyperactivity disorder (ADHD) is a debilitating disorder with apparent roots in abnormal brain development. Here, we quantified the level of individual brain maturation in children with ADHD using structural neuroimaging and a recently developed machine learning algorithm. More specifically, we compared the BrainAGE index between three groups matched for chronological age (mean ± SD: 11.86 ± 3.25 years): 89 children diagnosed with ADHD, 34 asymptomatic siblings of those children with ADHD, and 21 unrelated healthy control children. Brains of children with ADHD were estimated significantly younger (−0.85 years) than brains of healthy controls (Cohen’s d = −0.33; p = 0.028, one-tailed), while there were no significant differences between unaffected siblings and healthy controls. In addition, more severe ADHD symptoms were significantly associated with younger appearing brains. Altogether, these results are in line with the proposed delay of individual brain maturation in children with ADHD. However, given the relatively small sample size (N = 144), the findings should be considered preliminary and need to be confirmed in future studies.
Introduction
Attention-Deficit/Hyperactivity Disorder (ADHD) is a neurodevelopmental disorder that affects approximately 5% of all children and adolescents worldwide, with a higher incidence in males compared to females (1–5). ADHD has been widely reported to be associated with smaller global, regional, and local brain measures, both on the cortical and subcortical level and with respect to both gray matter and white matter (1–3, 6–25). Importantly, such effects were not only reported in classic analyses of data obtained on a single site, but also in meta- and mega-analytic analyses in very large samples from multiple sites (13–19). Moreover, there is evidence from longitudinal studies for lagging trajectories of brain development in ADHD (20–22). Altogether, these findings have been interpreted as a neurodevelopmental delay in ADHD (2, 3, 11, 26, 27), which in turn might be reflected in a seemingly younger brain age.
To our knowledge, only two other studies (28, 29) have investigated brain age in ADHD and, indeed, one observed younger appearing brains in children and adolescents with ADHD albeit not significantly so (28). The other one observed older appearing brains in participants with ADHD but findings were not significant either (29). However, no brain age study was conducted with explicit focus on ADHD (the main motivation of the former study was to evaluate a new brain age classifier; the latter study investigated multiple psychiatric disorders), so further research in the framework of ADHD is clearly indicated. On this note, ADHD has a strong genetic component, with a heritability of over 70% and a 5–10-fold increased risk for siblings to develop ADHD (1–3, 30–33). Given that previous studies reported that unaffected siblings of children with ADHD had similar but less pronounced gray matter alterations than their affected siblings (24, 25), it would also be interesting to explore if a delayed brain maturation is similarly evident in the healthy siblings of the children with ADHD.
Therefore, to shed further light on these questions and contribute to an understudied field of research, here we applied a well-validated brain age estimation algorithm (34–39) in children with ADHD, their healthy siblings, as well as age-matched unrelated control children. We hypothesized that children with ADHD will be estimated younger (i.e., their BrainAGE index will be lower) than age-matched controls, in accordance with the outcomes of the majority of the aforementioned studies (1–3, 6–27) as well as with the classification of ADHD as a neurodevelopmental disorder (2, 3, 11, 26, 27). Moreover, we hypothesized negative correlations between individual ADHD symptoms and estimated brain age (i.e., the more severe the symptoms, the more negative the BrainAGE index). As far as the siblings (who share genetic material with the ADHD children) are concerned, we hypothesized them to have younger appearing brains than the age-matched controls as well, but that these differences will be smaller than when comparing the ADHD children to the controls (i.e., ADHD children < unaffected siblings < healthy controls).
Materials and methods
Participants
A total of 144 participants (ADHD children, unaffected siblings, and control children) were recruited from the community, drafted from other ongoing studies of ADHD at the University of California, Los Angeles (UCLA), or referred to the study by their physicians. All children were evaluated for ADHD and other psychiatric disorders based on an interview with their primary caretaker using the Kiddie-Schedule for Affective Disorders and Schizophrenia–Present and Lifetime (K-SADS-PL) (40). In addition, direct interviews were performed with children who were 8 years and older. To supplement the interview(s), parent ratings on the Swanson, Nolan, and Pelham, Version IV (SNAP-IV) Rating Scale (41) were obtained. Children with ADHD were enrolled together with their siblings. If the siblings met the full diagnostic criteria for ADHD, they were enrolled in the ADHD group; if they did not meet diagnostic criteria for ADHD, they were enrolled as unaffected siblings. Unrelated children who did not meet diagnostic criteria for ADHD or any other psychiatric disorder were enrolled as control children; all of those were singletons. Exclusion criteria for all children (ADHD, siblings, and controls) were neurological disorders, significant head injuries, premature birth (≤34 weeks gestation), low IQ (<70 Full-Scale), lifetime diagnosis of schizophrenia or of autism, and any contraindications to magnetic resonance imaging (MRI). Overall this resulted in the inclusion of 89 children with ADHD, 34 siblings without ADHD (hereafter referred to as siblings), and 21 controls. Table 1 provides an overview on the sample characteristics. Importantly, there were no significant differences in chronological age across the three groups (p = 0.363). However, the distribution of boys and girls differed significantly between the three groups (p < 0.001), with a significantly higher male-to-female ratio in children with ADHD compared to siblings and controls. All participants provided written informed consent/assent and the study was approved by the UCLA Institutional Review Board. Additional approval for the data analysis was obtained from the University of Auckland Health Research Ethics Committee.
Data acquisition and preprocessing
All brain images were acquired on a 3T Siemens Trio Scanner at the UCLA Ahmanson-Lovelace Brain Mapping Center using a 21-channel head coil and the following parameters: TR = 1,900 ms, TE = 3.26 ms, flip angle = 9°, 176 axial sections, voxel size = 0.98 mm × 0.98 mm × 1 mm. These T1-weighted images were then processed in Matlab1 using SPM122 and the CAT12 toolbox (42). As detailed elsewhere (35, 36, 38, 39), all images were corrected for magnetic field inhomogeneities, tissue-classified into gray matter, white matter, and cerebrospinal fluid, spatially normalized to MNI space using affine transformations and convoluted using a Gaussian Kernel. These processed images provided the input for the BrainAGE analysis (see next section).
Estimating brain age
The BrainAGE approach used in our study was specifically validated in the context of neurodevelopment (35) and shown to be robust and reliable across datasets, age ranges, and scanner types (34, 36). The algorithm is based on a Relevance Vector Regression Machine (35, 43), and has been successfully applied in a number of studies (e.g., 34, 37, 38, and 39). The current study used the same workflow as described elsewhere (34, 35), but the model was trained on an even larger sample of 879 healthy children and adolescents aged 5–22 years (mean age 12.3 years, NIH Pediatric MRI Data Repository 4th release). This trained algorithm was applied to the processed gray matter images of the current sample (see previous section) to estimate each brain’s age. The difference between the estimated age and the chronological age yielded the brain age gap estimate (BrainAGE) index (see Supplementary Figure 1 for further details). The BrainAGE index is negative if a brain is estimated younger than its chronological age (positive if older than its chronological age). Thus, a negative BrainAGE index is consistent with a delay in brain maturation. Importantly, to ensure that the BrainAGE index is not correlated with age, any links between the two variables were removed (44, 45) as described elsewhere (37).
Statistical analyses
Main analyses
To compare BrainAGE across the three groups, the BrainAGE indices were the dependent variable and group status (i.e., ADHD, siblings, and controls) was the independent variable. Sex was included as a covariate because the distribution of boys and girls differed between the groups, and “family” was included as a random variable because several of the participants were siblings. In addition, we tested whether there was a significant group-by-sex interaction because the prevalence of ADHD during childhood and adolescence is higher in males than in females (4). However, the inclusion of a group-by-sex interaction did not improve the model (p = 0.332) but, instead, increased the Akaike Information Criterion suggesting that the model without the interaction term was a better fit. Moreover, the interaction did not reach significance [F (2,138) = 1.11, p = 0.332]. Thus, the model was conducted without the group-by-sex interaction. Given our hypothesis that children with ADHD as well as their siblings (albeit to a lesser degree) would present with a delay in brain maturation (i.e., younger appearing brains) compared to control children, one-tailed T-tests were applied. Assumptions for parametric testing were confirmed using a Lilliefors test determining the normal distribution of the residuals and a Bartlett test determining equality of variance.
To investigate correlations between BrainAGE and measures of symptom severity, the BrainAGE indices were the dependent variable, the five symptom measures were the independent variable (a separate model was run for each), sex was a covariate, and “family” was a random variable. Again, the addition of a sex interaction did not improve the models and also did not reach significance for any of the symptom measures (see Supplementary Table 1); it was thus omitted. The aforementioned five symptom measures were (1) the Parent SNAP-IV hyperactivity score, (2) the Parent SNAP-IV inattention score, (3) the Parent SNAP-IV combined score, (4) the number of hyperactivity symptoms, and (5) the number of inattention symptoms. Eight participants (one control, four siblings, and three ADHD children) did not have scores for the SNAP, and three controls did not have scores for the number of symptoms and were therefore excluded from the correlation analyses. Given our hypothesis of a negative correlation between the number of symptoms and brain maturation (the more symptoms, the younger the brain), one-tailed T-tests were applied. Given the five different models for the five different symptom scores, corrections for multiple comparisons were applied using the false discovery rate (FDR) (46). Assumptions for parametric testing were confirmed using Lilliefors tests determining the normal distribution of the residuals.
Supplemental analyses
No significant sex interaction was found, neither in the group comparisons nor the correlation analyses. However, given the higher prevalence of ADHD in males during childhood and adolescence (4), we conducted an additional set of exploratory analyses repeating the main analyses described above in males and females separately. Moreover, we repeated the correlation analyses for both sexes combined but only in children with ADHD.
Results
Main findings
Figure 1 depicts the individual and group-specific BrainAGE indices with negative numbers indicating a delay in brain maturation (i.e., brains are estimated younger than their chronological age). On average, the BrainAGE index was 0.0 ± 1.95 years in controls (blue), −0.71 ± 1.78 in siblings (cyan), and −0.85 ± 1.87 in children with ADHD (yellow). There was a delay in brain maturation of 0.71 years (which corresponds to 8.5 months) in siblings and of 0.85 years (which corresponds to 10.2 months) in children with ADHD. The delay in brain maturation did not reach significance in siblings compared to controls [Cohen’s d = −0.23, t (140) = −1.38, p = 0.086], but was significant for children with ADHD compared to controls [Cohen’s d = −0.33, t (140) = −1.93, p = 0.028]. The difference in brain maturation between children with ADHD and siblings was not significant [Cohen’s d = −0.08, t (140) = −0.49, p = 0.313].
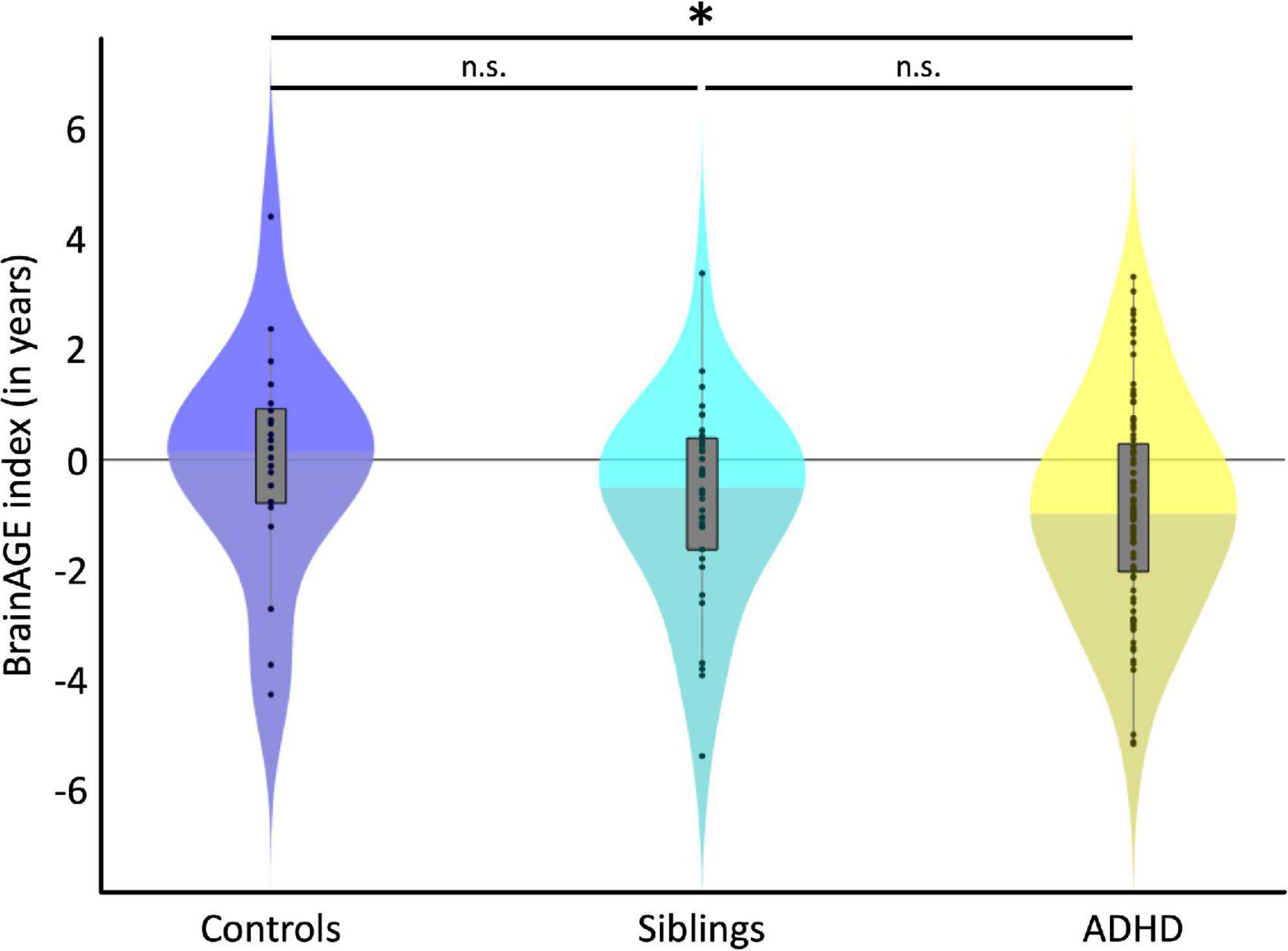
Figure 1. Group Differences. Violin plots depict the BrainAGE distribution for each group. The black dots show individual estimates, the gray boxes show the group-specific interquartile ranges, and the whiskers show the group-specific 1.5 interquartile ranges. Shading for each group’s violin plot changes at the median. On average, children with ADHD (yellow) were estimated significantly younger than control children (blue), as indicated by the asterisk. Siblings unaffected by ADHD (cyan) were also estimated younger than control children (blue), but this difference did not reach statistical significance (n.s.).
Figure 2 depicts the link between the individual BrainAGE indices and symptom scores. All correlations were negative indicating that a lower BrainAGE index is associated with more severe symptoms. As detailed in Table 2, the correlations were significant for all symptom severity scores, except for the number of hyperactivity symptoms.
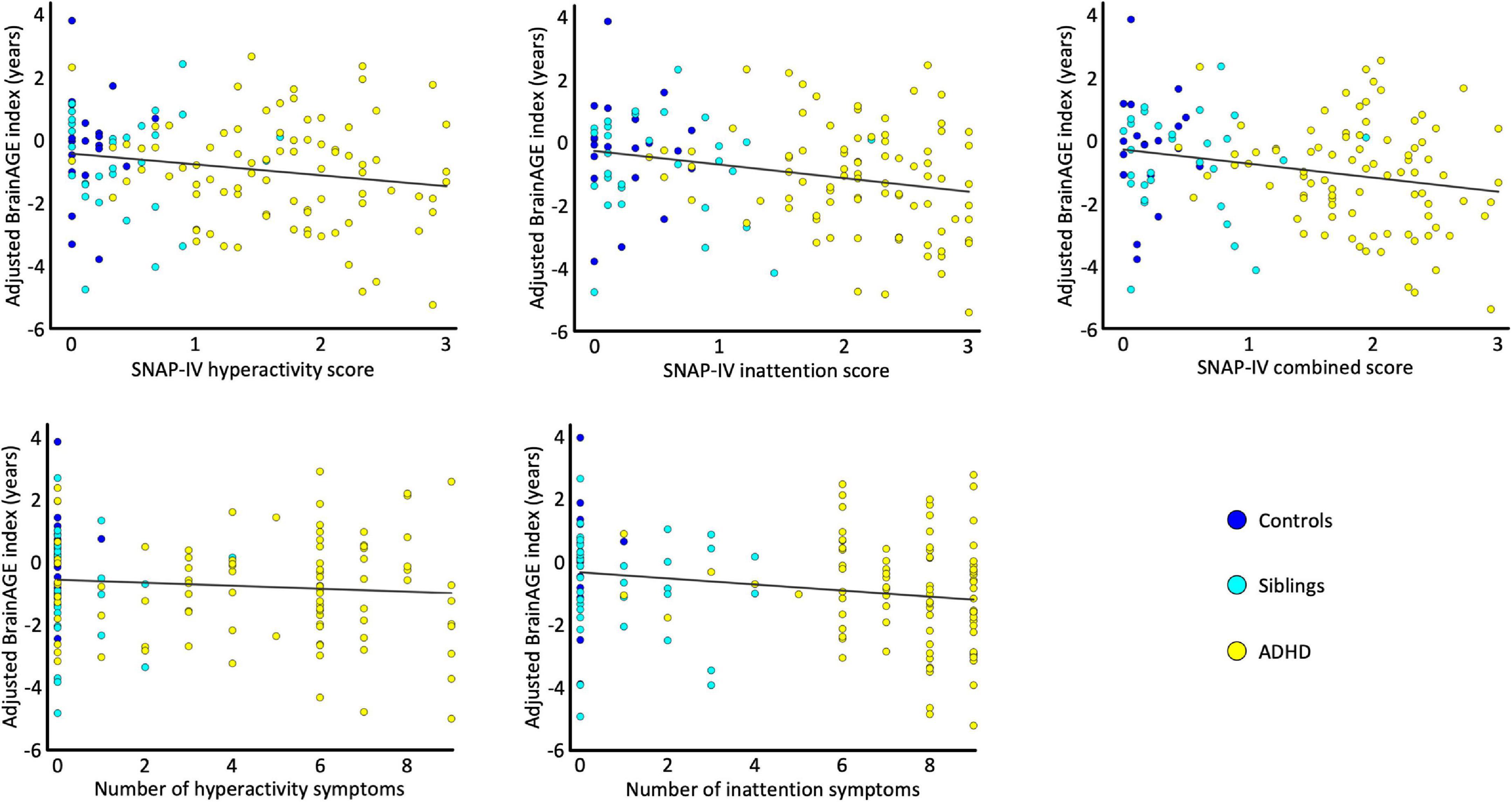
Figure 2. Correlations. The scatterplots depict the association between the sex-adjusted BrainAGE index and the SNAP-IV hyperactivity scores, the SNAP-IV inattention scores, the SNAP-IV combined scores, the number of hyperactivity symptoms, and the number of inattention symptoms. For each scatterplot the estimated association is plotted as a least-square regression line. All data points are color-coded by group (control children in blue, unaffected siblings in cyan, and children with ADHD in yellow).
Supplemental findings
The exploratory analyses investigating group differences and associations with symptom scores in boys and girls separately suggest that effects are primarily found in boys. However, given that group-by-sex interactions and symptom-by-sex interactions in the main analyses were not significant, these findings will solely be provided as a reference for future studies without interpreting them (see Supplementary Tables 2, 3). When confining the correlation analyses to the ADHD group, the associations remained negative for all but the number of hyperactivity symptoms but only reached significance (albeit uncorrected) when stratified by sex. Similarly to the sex-stratified group differences and correlations in the whole group, these findings are also provided solely as a reference (see Supplementary Table 4).
Discussion
Originally catalyzed by a competition (47), a number of studies have used machine learning tools to predict the diagnosis of ADHD based on MRI scans of the brain (48). Deriving at a diagnosis for ADHD based on machine learning (or neuroimaging in general) still poses an ongoing challenge but has since led to promising advances in the field (48). Importantly, while the BrainAGE approach is also based on machine learning it was not designed to diagnose ADHD. Instead, it was applied to test for a potentially delayed brain maturation in ADHD. More specifically, this was achieved by estimating the individual brain ages and calculating their deviation from the chronological ages—revealing the BrainAGE indices (34–39)—which were then compared between children with ADHD and age-matched controls as well as related to ADHD symptoms. We detected significantly younger appearing brains in children with ADHD compared to age-matched controls and we also observed significant negative links between ADHD symptom severity and BrainAGE. Altogether, these findings are in strong agreement with the majority of previous observations (1–3, 6–28), suggesting a neurodevelopmental delay in ADHD (2, 3, 10, 11, 26, 27). Moreover, as deviations from normal brain development have been proposed as defining traits of neurodevelopmental disorders (26, 27), the present results also support the classification of ADHD as a neurodevelopmental disorder (2, 3, 11, 26, 27). Of note, the direction of the currently observed effect is also in agreement with the outcomes of another brain age estimation study reporting younger brain ages in children with ADHD compared to controls (28), albeit the effect in that study was not significant. In contrast, the findings of the current study as well as most other existing findings (1–3, 6–25) seem to disagree with the outcomes of yet another brain age study, which revealed older brain ages in participants with ADHD (29), albeit effects were not significant either. Given the conflicting findings as well as the limited number of brain age studies overall, further research is clearly necessary.
In addition to children with ADHD and control children, the present study also included unaffected siblings of children with ADHD. The difference between siblings and controls did not reach significance, but the brains of siblings were estimated 8.5 months younger than the brains of controls. Given that the brains of children with ADHD were estimated 10.2 months younger than the brains of controls, the unaffected siblings were overall closer to their siblings with ADHD than to the controls. This finding seems to be consistent with the observation that ADHD runs in families, with siblings of children with ADHD having a 5–10-fold increased risk of developing ADHD when compared to the general population (2, 30, 31). It also corroborates the outcomes of previous imaging studies that revealed similar but less pronounces brain alterations in siblings (24, 25).
Study limitations and implications for future research
Since the brain age estimation is a relatively novel approach, our study complements and extends the existing field of research. However, future studies are needed to replicate the present findings and address some study limitations: First of all, the sample size is rather small and would benefit substantially from an increase, particularly in the control group. This might not only enhance statistical power but also allow to stratify analyses by sex and to interpret sex-specific findings with confidence. For example, it is certainly noteworthy that our supplemental analyses revealed a lower BrainAGE index (compared to controls) only in boys with ADHD as well as in unaffected brothers, while no effects were evident in girls with ADHD or unaffected sisters. However, due to the non-significant sex-by-group interaction in the main analysis, which is potentially due to the limited statistical power, any interpretations would be conjecture. Moreover, while the current cross-sectional study captured apparent delays in brain maturation, future longitudinal studies may not only shed light on the causality of the effects but also on the onset as well as the longevity/stability of the effects. For example, ADHD has been reported to manifest in early childhood, middle childhood, adolescence, or even adulthood, where adulthood ADHD may or may not be a continuation of childhood ADHD (49–51). In the same vein, symptoms may increase, decrease, or stagnate over time (3, 26) and all this might be captured by changes in the BrainAGE index (or changes in brain anatomy in general). On that last note, it will certainly also be worthwhile finding a sustainable way to conduct more comprehensive studies in the field of ADHD linking a number of relevant aspects (i.e., brain structure, brain function, cognition, behavior, genes, and environment) rather than being restricted to one or two single aspects.
Data availability statement
The data analyzed in this study is subject to the following licenses/restrictions: We do not have permission to share the data. Requests to access these datasets should be directed to FK, Zi5rdXJ0aEBhdWNrbGFuZC5hYy5ueg==.
Ethics statement
The studies involving human participants were reviewed and approved by the UCLA Institutional Review Board and University of Auckland Health Research Ethics Committee. Written informed consent to participate in this study was provided by the participants’ legal guardian/next of kin.
Author contributions
EL, FK, and JL planned the present analysis of the dataset, which was conceived, acquired, and curated by JL, SL, JO’N, JA, and KN. CG processed the MRI data and calculated the BrainAGE indices. FK conducted the statistical analyses. FK and EL wrote the manuscript. JO’N contributed to the writing and editing of the manuscript. All authors contributed to the article and approved the final manuscript.
Funding
This research was supported by grants from the National Institutes of Health (NIMH RC1 MH088507 and NIAAA R01 AA025066 to JL), from Cure Kids (3919 to EL), as well as from the Humboldt Foundation (to EL).
Conflict of interest
The authors declare that the research was conducted in the absence of any commercial or financial relationships that could be construed as a potential conflict of interest.
Publisher’s note
All claims expressed in this article are solely those of the authors and do not necessarily represent those of their affiliated organizations, or those of the publisher, the editors and the reviewers. Any product that may be evaluated in this article, or claim that may be made by its manufacturer, is not guaranteed or endorsed by the publisher.
Supplementary material
The Supplementary Material for this article can be found online at: https://www.frontiersin.org/articles/10.3389/fpsyt.2022.1019546/full#supplementary-material
Footnotes
References
1. Faraone SV, Banaschewski T, Coghill D, Zheng Y, Biederman J, Bellgrove MA, et al. The world federation of ADHD International Consensus Statement: 208 evidence-based conclusions about the disorder. Neurosci Biobehav Rev. (2021) 128:789–818. doi: 10.1016/j.neubiorev.2021.01.022
2. Faraone SV, Asherson P, Banaschewski T, Biederman J, Buitelaar JK, Ramos-Quiroga JA, et al. Attention-deficit/hyperactivity disorder. Nat Rev Dis Primers. (2015) 1:15020.
3. Posner J, Polanczyk GV, Sonuga-Barke E. Attention-deficit hyperactivity disorder. Lancet. (2020) 395:450–62.
4. Polanczyk G, de Lima MS, Horta BL, Biederman J, Rohde LA. The worldwide prevalence of ADHD: a systematic review and metaregression analysis. Am J Psychiatry. (2007) 164:942–8.
5. Polanczyk GV, Willcutt EG, Salum GA, Kieling C, Rohde LA. ADHD prevalence estimates across three decades: an updated systematic review and meta-regression analysis. Int J Epidemiol. (2014) 43:434–42. doi: 10.1093/ije/dyt261
6. Hoogman M, van Rooij D, Klein M, Boedhoe P, Ilioska I, Li T, et al. Consortium neuroscience of attention deficit/hyperactivity disorder and autism spectrum disorder: the ENIGMA adventure. Hum Brain Mapp. (2022) 43:37–55. doi: 10.1002/hbm.25029
7. Firouzabadi FD, Ramezanpour S, Firouzabadi MD, Yousem IJ, Puts NAJ, Yousem DM. Neuroimaging in attention-deficit/hyperactivity disorder: recent advances. AJR Am J Roentgenol. (2022) 218:321–32.
8. Franke B, Michelini G, Asherson P, Banaschewski T, Bilbow A, Buitelaar JK, et al. Live fast, die young? A review on the developmental trajectories of ADHD across the lifespan. Eur Neuropsychopharmacol. (2018) 28:1059–88. doi: 10.1016/j.euroneuro.2018.08.001
9. Albajara Saenz A, Villemonteix T, Massat I. Structural and functional neuroimaging in attention-deficit/hyperactivity disorder. Dev Med Child Neurol. (2019) 61:399–405.
12. Durston S. A review of the biological bases of ADHD: what have we learned from imaging studies? Ment Retard Dev Disabil Res Rev. (2003) 9:184–95. doi: 10.1002/mrdd.10079
13. Hoogman M, Bralten J, Hibar DP, Mennes M, Zwiers MP, Schweren LSJ, et al. Subcortical brain volume differences in participants with attention deficit hyperactivity disorder in children and adults: a cross-sectional mega-analysis. Lancet Psychiatry. (2017) 4:310–9.
14. Hoogman M, Muetzel R, Guimaraes JP, Shumskaya E, Mennes M, Zwiers MP, et al. Brain Imaging of the Cortex in ADHD: a coordinated analysis of large-scale clinical and population-based samples. Am J Psychiatry. (2019) 176:531–42. doi: 10.1176/appi.ajp.2019.18091033
15. Valera EM, Faraone SV, Murray KE, Seidman LJ. Meta-analysis of structural imaging findings in attention-deficit/hyperactivity disorder. Biol Psychiatry. (2007) 61:1361–9.
16. Hutchinson AD, Mathias JL, Banich MT. Corpus callosum morphology in children and adolescents with attention deficit hyperactivity disorder: a meta-analytic review. Neuropsychology. (2008) 22:341–9. doi: 10.1037/0894-4105.22.3.341
17. Ellison-Wright I, Ellison-Wright Z, Bullmore E. Structural brain change in attention deficit hyperactivity disorder identified by meta-analysis. BMC Psychiatry. (2008) 8:51. doi: 10.1186/1471-244X-8-51
18. Nakao T, Radua J, Rubia K, Mataix-Cols D. Gray matter volume abnormalities in ADHD: voxel-based meta-analysis exploring the effects of age and stimulant medication. Am J Psychiatry. (2011) 168:1154–63. doi: 10.1176/appi.ajp.2011.11020281
19. Frodl T, Skokauskas N. Meta-analysis of structural MRI studies in children and adults with attention deficit hyperactivity disorder indicates treatment effects. Acta Psychiatr Scand. (2012) 125:114–26. doi: 10.1111/j.1600-0447.2011.01786.x
20. Castellanos FX, Lee PP, Sharp W, Jeffries NO, Greenstein DK, Clasen LS, et al. Developmental trajectories of brain volume abnormalities in children and adolescents with attention-deficit/hyperactivity disorder. JAMA. (2002) 288: 1740–8.
21. Shaw P, Eckstrand K, Sharp W, Blumenthal J, Lerch JP, Greenstein D, et al. Attention-deficit/hyperactivity disorder is characterized by a delay in cortical maturation. Proc Natl Acad Sci U.S.A. (2007) 104:19649–54. doi: 10.1073/pnas.0707741104
22. Shaw P, Malek M, Watson B, Sharp W, Evans A, Greenstein D. Development of cortical surface area and gyrification in attention-deficit/hyperactivity disorder. Biol Psychiatry. (2012) 72:191–7.
23. Luders E, Narr KL, Hamilton LS, Phillips OR, Thompson PM, Valle JS, et al. Decreased callosal thickness in attention-deficit/hyperactivity disorder. Biol Psychiatry. (2009) 65:84–8.
24. Durston S, Hulshoff Pol HE, Schnack HG, Buitelaar JK, Steenhuis MP, Minderaa RB, et al. Magnetic resonance imaging of boys with attention-deficit/hyperactivity disorder and their unaffected siblings. J Am Acad Child Adolesc Psychiatry. (2004) 43:332–40.
25. Greven CU, Bralten J, Mennes M, O’Dwyer L, van Hulzen KJ, Rommelse N, et al. Developmentally stable whole-brain volume reductions and developmentally sensitive caudate and putamen volume alterations in those with attention-deficit/hyperactivity disorder and their unaffected siblings. JAMA Psychiatry. (2015) 72:490–9. doi: 10.1001/jamapsychiatry.2014.3162
26. Shaw P, Polanczyk GV. Combining epidemiological and neurobiological perspectives to characterize the lifetime trajectories of ADHD. Eur Child Adolesc Psychiatry. (2017) 26:139–41. doi: 10.1007/s00787-017-0944-8
28. Niu X, Taylor A, Shinohara RT, Kounios J, Zhang F. Multidimensional brain-age prediction reveals altered brain developmental trajectory in psychiatric disorders. Cereb Cortex. (2022) [Epub ahead of print]. doi: 10.1093/cercor/bhab530
29. Kaufmann T, van der Meer D, Doan NT, Schwarz E, Lund MJ, Agartz I, et al. Common brain disorders are associated with heritable patterns of apparent aging of the brain. Nat Neurosci. (2019) 22:1617–23.
30. Biederman J, Faraone SV, Keenan K, Benjamin J, Krifcher B, Moore C, et al. Further evidence for family-genetic risk factors in attention deficit hyperactivity disorder. Patterns of comorbidity in probands and relatives psychiatrically and pediatrically referred samples. Arch Gen Psychiatry. (1992) 49:728–38. doi: 10.1001/archpsyc.1992.01820090056010
31. Biederman J, Faraone SV, Keenan K, Knee D, Tsuang MT. Family-genetic and psychosocial risk factors in DSM-III attention deficit disorder. J Am Acad Child Adolesc Psychiatry. (1990) 29:526–33. doi: 10.1097/00004583-199007000-00004
32. Larsson H, Chang Z, D’Onofrio BM, Lichtenstein P. The heritability of clinically diagnosed attention deficit hyperactivity disorder across the lifespan. Psychol Med. (2014) 44:2223–9.
33. Faraone SV, Perlis RH, Doyle AE, Smoller JW, Goralnick JJ, Holmgren MA, et al. Molecular genetics of attention-deficit/hyperactivity disorder. Biol Psychiatry. (2005) 57:1313–23.
34. Franke K, Gaser C. Ten Years of BrainAGE as a neuroimaging biomarker of brain aging: what insights have we gained? Front Neurol. (2019) 10:789. doi: 10.3389/fneur.2019.00789
35. Franke K, Luders E, May A, Wilke M, Gaser C. Brain maturation: Predicting individual BrainAGE in children and adolescents using structural MRI. Neuroimage. (2012) 63:1305–12.
36. Franke K, Ziegler G, Kloppel S, Gaser C. Estimating the age of healthy subjects from T1-weighted MRI scans using kernel methods: exploring the influence of various parameters. Neuroimage. (2010) 50:883–92. doi: 10.1016/j.neuroimage.2010.01.005
37. Cherbuin N, Walsh EI, Shaw M, Luders E, Anstey KJ, Sachdev PS, et al. Optimal blood pressure keeps our brains younger. Front Aging Neurosci. (2021) 13:694982. doi: 10.3389/fnagi.2021.694982
38. Luders E, Cherbuin N, Gaser C. Estimating brain age using high-resolution pattern recognition: Younger brains in long-term meditation practitioners. Neuroimage. (2016) 134:508–13. doi: 10.1016/j.neuroimage.2016.04.007
39. Luders E, Gingnell M, Poromaa IS, Engman J, Kurth F, Gaser C. Potential brain age reversal after pregnancy: younger brains at 4-6 weeks postpartum. Neuroscience. (2018) 386:309–14. doi: 10.1016/j.neuroscience.2018.07.006
40. Kaufman J, Birmaher B, Brent D, Rao U, Flynn C, Moreci P, et al. Schedule for Affective Disorders and Schizophrenia for School-Age Children-Present and Lifetime Version (K-SADS-PL): initial reliability and validity data. J Am Acad Child Adolesc Psychiatry. (1997) 36:980–8. doi: 10.1097/00004583-199707000-00021
41. Swanson JM. School-based Assessments and Interventions for ADD Students. Irvine, CA: K.C. Publishing (1992).
42. Gaser C, Dahnke R, Thompson PM, Kurth F, Luders E. CAT – a computational anatomy toolbox for the analysis of structural MRI data. bioRxiv [Preprint]. (2022) doi: 10.1101/2022.06.11.495736
43. Tipping ME. Sparse bayesian learning and the relevance vector machine. J Mach Learn Res. (2001) 1:211–44.
44. Smith SM, Vidaurre D, Alfaro-Almagro F, Nichols TE, Miller KL. Estimation of brain age delta from brain imaging. Neuroimage. (2019) 200:528–39.
45. Le TT, Kuplicki RT, McKinney BA, Yeh HW, Thompson WK, Paulus MP, et al. A nonlinear simulation framework supports adjusting for age when analyzing brainAGE. Front Aging Neurosci. (2018) 10:317. doi: 10.3389/fnagi.2018.00317
46. Hochberg Y, Benjamini Y. More powerful procedures for multiple significance testing. Stat Med. (1990) 9:811–8.
47. HD-200 Consortium. The ADHD-200 consortium: a model to advance the translational potential of neuroimaging in clinical neuroscience. Front Syst Neurosci. (2012) 6:62. doi: 10.3389/fnsys.2012.00062
48. Uddin LQ, Dajani DR, Voorhies W, Bednarz H, Kana RK. Progress and roadblocks in the search for brain-based biomarkers of autism and attention-deficit/hyperactivity disorder. Transl Psychiatry. (2017) 7:e1218. doi: 10.1038/tp.2017.164
49. Agnew-Blais JC, Polanczyk GV, Danese A, Wertz J, Moffitt TE, Arseneault L. Evaluation of the persistence, remission, and emergence of attention-deficit/hyperactivity disorder in young adulthood. JAMA Psychiatry. (2016) 73:713–20. doi: 10.1001/jamapsychiatry.2016.0465
50. Moffitt TE, Houts R, Asherson P, Belsky DW, Corcoran DL, Hammerle M, et al. Is Adult ADHD a childhood-onset neurodevelopmental disorder? evidence from a four-decade longitudinal cohort study. Am J Psychiatry. (2015) 172:967–77. doi: 10.1176/appi.ajp.2015.14101266
Keywords: ADHD, brain, BrainAGE, development, machine learning, relevance vector, sex, siblings
Citation: Kurth F, Levitt JG, Gaser C, Alger J, Loo SK, Narr KL, O’Neill J and Luders E (2022) Preliminary evidence for a lower brain age in children with attention-deficit/hyperactivity disorder. Front. Psychiatry 13:1019546. doi: 10.3389/fpsyt.2022.1019546
Received: 15 August 2022; Accepted: 14 November 2022;
Published: 02 December 2022.
Edited by:
Laura K. M. Han, The University of Melbourne, AustraliaReviewed by:
Danai Dima, City, University of London, United KingdomNiousha Dehestani, Deakin University, Australia
Lisa Todd Eyler, University of California, San Diego, United States
Copyright © 2022 Kurth, Levitt, Gaser, Alger, Loo, Narr, O’Neill and Luders. This is an open-access article distributed under the terms of the Creative Commons Attribution License (CC BY). The use, distribution or reproduction in other forums is permitted, provided the original author(s) and the copyright owner(s) are credited and that the original publication in this journal is cited, in accordance with accepted academic practice. No use, distribution or reproduction is permitted which does not comply with these terms.
*Correspondence: Florian Kurth, Zi5rdXJ0aEBhdWNrbGFuZC5hYy5ueg==