- 1CSIRO, Brisbane, QLD, Australia
- 2Metro North Public Health Unit, Queensland Health, Brisbane, QLD, Australia
Background: In an effort to eliminate dengue, a successful technology was developed with the stable introduction of the obligate intracellular bacteria Wolbachia pipientis into the mosquito Aedes aegypti to reduce its ability to transmit dengue fever due to life shortening and inhibition of viral replication effects. An analysis of risk was required before considering release of the modified mosquito into the environment.
Methods: Expert knowledge and a risk assessment framework were used to identify risk associated with the release of the modified mosquito. Individual and group expert elicitation was performed to identify potential hazards. A Bayesian network (BN) was developed to capture the relationship between hazards and the likelihood of events occurring. Risk was calculated from the expert likelihood estimates populating the BN and the consequence estimates elicited from experts.
Results: The risk model for “Don’t Achieve Release” provided an estimated 46% likelihood that the release would not occur by a nominated time but generated an overall risk rating of very low. The ability to obtain compliance had the greatest influence on the likelihood of release occurring. The risk model for “Cause More Harm” provided a 12.5% likelihood that more harm would result from the release, but the overall risk was considered negligible. The efficacy of mosquito management had the most influence, with the perception that the threat of dengue fever had been eliminated, resulting in less household mosquito control, and was scored as the highest ranked individual hazard (albeit low risk).
Conclusions: The risk analysis was designed to incorporate the interacting complexity of hazards that may affect the release of the technology into the environment. The risk analysis was a small, but important, implementation phase in the success of this innovative research introducing a new technology to combat dengue transmission in the environment.
Introduction
Dengue remains a priority for public health authorities across the globe. The viral disease is transmitted primarily by the mosquito Aedes aegypti (Ae. aegypti), which also transmits a number of other viruses, including yellow fever and chikungunya viruses (1, 2). An estimated 390 million dengue infections occur annually as the virus expands into new geographic regions and affects both urban and rural settings (3, 4). Dengue is not considered endemic in Australia, and dengue activity is currently limited to some parts of Queensland, where Ae. aegypti is present (5, 6). In the absence of a vaccine, management of dengue comprises vector management and reduction of human exposure to mosquito bites through the use of repellents and behavioral modification (7). However, outbreaks still occur as a result of the repeated reintroduction of the virus through infected overseas travelers visiting or returning to Australia, especially during summer months.
Aedes aegypti females are responsible for transmitting dengue viruses between humans. However, the mosquito must first acquire the virus from a viremic individual through a blood meal. While Ae. aegypti is African in origin, it is now distributed in many tropical and subtropical regions across the globe. It is almost always associated with human habitats. Larval development most commonly occurs in artificial water containers, such as pot plant bases, discarded tires, and water tanks (8, 9), or natural containers, such as fallen palm fronds and coconut husks. The potential for larval production can be high, especially within urban centers of developing countries, where domestic water storage in containers is common (10, 11).
Wolbachia are obligate intracellular endosymbiotic bacteria found naturally in a wide range of invertebrates, including some species of mosquitoes, but not Ae. aegypti (12, 13). Wolbachia are maternally transmitted to the next generation through the eggs, infect reproductive tissues, and manipulate the host reproductive cycle to increase their spread (14–16). Reproductive strategies associated with Wolbachia infection include parthenogenesis, male killing or feminization, sex-ratio distortions (17–19), and cytoplasmic incompatibility (20, 21), which effectively reduce the ability for the dengue virus to infect other hosts by blocking virus replication (2). Many years of field-based and laboratory studies have resulted in the successful introduction of at least two Wolbachia strains (wMel and wMelPop-CLA strain) to Ae. aegypti populations [see Ref. (22) for details].
As part of a larger international project to reduce the incidence of dengue fever in Australia and elsewhere around the world, a trial field release of Wolbachia-infected Ae. aegypti was proposed for the 2010/11 wet season in far North Queensland, Australia. The biosafety of proposed release was assessed and approved by the Australian Government (23). This was the first time such a release had been considered and a key element in securing permission was the analysis of risks associated with the release. Thus, all elements of risk associated with the release of Ae. aegypti containing Wolbachia into naturally occurring populations needed to be identified and investigated before the release was approved to ensure that the field release would “cause no more harm” than that posed by natural Ae. aegypti populations. The novelty of the project meant that there were limited empirical data and subsequently high levels of uncertainty surrounding the potential for negative impacts. Where historical data relevant to assessing risk are lacking, elicitation of expert knowledge is an appropriate proxy for empirical data and is often used to address uncertainties in knowledge assumptions and limited datasets (24). Because of the novelty of the system, and potentially wide-ranging effects on individuals and communities in release areas, and in order to ensure transparency in the process, non-technical community experts were involved in the elicitation of risk estimates as well as technical experts.
Risk assessments are standard practice in many business practices and operational procedures of organizations today. A risk analysis determines the likelihood of an event occurring and the consequences of an event if it does occur. The level of risk is calculated from the product of the likelihood and consequence. A risk assessment needs measures to incorporate feedback opportunities to improve predictions and reduce uncertainty (25, 26).
The results of the risk analysis described herein were used to determine the approval of the proposed release. To assess the risk associated with the proposed release, two risk end points (undesirable states of a system) were considered. Here, we describe the tools, process, and methodology used in the risk analysis to identify and assess potential hazards of releasing Ae. aegypti mosquitoes containing a strain of Wolbachia into a naturally occurring population. We also describe the results of the risk analysis, including discussion of the specific hazard domains identified, the Bayesian networks (BNs) constructed to explore the hierarchal structure and relationship of the hazards, and the final expert-derived estimates of risk associated with the release of the modified Ae. aegypti in Australia. In addition, we list recommendations for developing risk analyses, which address novel technologies with diverse impacts.
Materials and Methods
Steps of Risk Analysis – Overall Methodology
The risk analysis for the proposed release assessed the risks associated with two end points: (1) that release would not occur within a set time frame due to logistical, regulatory, political, epidemiological, and community concerns, referred to as “Don’t Achieve Release” (DAR) and (2) that the release of the modified Ae. aegypti would result in more harm through impacts on the economy, social wellbeing and community health, future mosquito control effort, and/or adverse changes to the biology of the vector, Wolbachia or dengue viruses, in the release locations when compared with the current situation within a 30-year timeframe, known as “Cause More Harm” (CMH) (Table 1).
Following the formulation of the two end points, DAR and CMH, risk was assessed through four iterative stages: (i) hazard identification and development of a conceptual model, (ii) development of a predictive risk model, (iii) model scrutiny and update, and (iv) risk calculation. These stages were undertaken using various methods, including expert elicitation via workshops and email correspondence, and construction of a BN. A series of expert workshops were held in Cairns and Brisbane, QLD, Australia, in 2010. Each workshop is comprised a range of “experts” as participants, including academics, regulatory officials, and community members, to obtain the broadest knowledge possible. Technical experts were identified due to their association with the project or as local experts with relevant expertise on mosquitoes, vector control, arboviruses, and public health. Non-technical community experts and non-government organizations were identified through a community engagement program that was conducted in the proposed location of release (27).
The authors facilitated each workshop using an elicitation method adapted from Spetzler and von Holstein (28) and O’Hagan et al. (29) using breakout groups of three to four experts to encourage individual expert input, combined with entire group participation for feedback opportunity and group consensus. Averaging expert responses through group consensus counters individual variation in opinion (30) but does not account for outlier influence. Therefore, it took email correspondence and a further two workshops to reach consensus across each model.
Hazard Identification and Development of the Conceptual Model
The first step in any risk analysis is to identify all hazards associated with an event. An initial workshop was conducted with technical experts to describe all potential risks or hazards that may result from the proposed release relevant to each endpoint. The initial step elicited expert judgment from researchers associated with the Grand Challenges in Global Health (GCGH) initiative during a 2-h brain storming and hazard mapping session on May 20, 2009. Participants were provided the two end points and asked to describe relevant types of potential hazard under general categories before identifying specific hazards for each. Second, additional hazards of concern to the proposed release locations were elicited through the GCGH’s public engagement program through community seminars and media (27, 31).
Development of the Predictive Risk Model
A BN was used as the model structure to determine the risk of the end points using the information derived from the experts. A BN is an influence diagram that depicts logical or causal relationships of factors that can influence the likelihood of an outcome of a parameter (32). The BN influence diagram consists of a graph (network) with a set of connected nodes (representing hazards), where directed connections from “parent” nodes leading to a “child” node indicate a causal influence of the parent node(s) on the child node. Each node is discretized into categories (states) defining the range or value of information represented in each state for each node (33). Underlying each child node is a conditional probability table (CPT) that defines the dependencies between the parent nodes and their associated states (34). The likelihood of each state for each child node is updated when values for a parent node are specified (by expert estimates or empirical data). We used Netica 4.12 (35) to develop and compile the BN.
Experts were invited to critique the model structure of the BN and edit accordingly by adding, removing, or modifying nodes (hazards). Experts also formulated the definitions for the nodes and their associated states. Once the model structure was agreed upon, experts were asked to estimate the conditional probabilities for each combination of states possible under each child node. Subsequent discussion by email and two further workshops with experts resulted in consensus for the final BN models and underlying CPTs.
Model Analysis and Sensitivity Analysis
We ran sensitivity analysis within Netica 4.12 to examine the influence of each node on the two end points. Sensitivity analysis in Netica uses entropy reduction (the expected decrease in uncertainty of the node being queried due to information at the parent node) to determine how “sensitive” a model is to the changes in model variables (36). Hence, if prior findings or “priors,” such as knowledge from an expert’s experience, are supplied about the state of a parent node, this may reduce the maximum range of uncertainty around the likelihood distribution of the output child node (35, 37). Sensitivity analysis can also show how influential each parent node is toward its associated child node. This is determined by the likelihoods given in the CPTs populated by the experts.
Uncertainty was reduced by continually incorporating new information from emerging research as “findings” when it became available, approaching all potential experts for participation, educating all participating experts regarding the model language, jointly developing definitions for each of the model nodes and associated states, and having an independent committee overseeing each stage of the risk analysis. Email correspondence and further workshops with technical experts were conducted to address a number of issues, including reducing the uncertainty around nodes by narrowing their expected distributions (e.g., uncertainty range changes from 0.1–0.9 to 0.4–0.6 when new knowledge reduced the uncertainty around a variable). In the final workshop, experts were asked to come to a group consensus through discussion of the merits of the current individual likelihoods for each node and adjusting where necessary, thus reducing the uncertainty through group experience.
Risk Calculation
Risk was calculated by combining the probability or likelihood of an event occurring (or not occurring) and the impact or consequences of that event occurring (or not occurring). Hence, we considered the likelihood of not achieving release and that the event will cause more harm, as well as the consequences if these events occurred. Estimates for both likelihood and consequence were elicited from the technical experts for calculation of risk. These estimates were multiplied together to give a risk value. A scale was determined with experts to rank risk from negligible to very high (Table 2). These were combined into a risk matrix, which corresponded with the risk value.
Results
Hazard Identification and Development of the Conceptual Model
Fifty-two possible hazards associated with the release of the modified Ae. aegypti were initially identified at the first GCGH session across 13 categories, including regulatory compliance, community acceptance, and adverse public health effects, beyond that already caused by naturally occurring Ae. aegypti. The expert workshops further identified additional hazards. After workshops, consultations and community engagement, a total of 109 hazards were identified and defined (Supplementary Material). After removing hazards that were beyond the scope of the end points, the remaining hazards were refined by grouping similar hazards into themes: (i) logistics, compliance, and public opinion for DAR (comprising 18 nodes) and (ii) ecological impact, economic impact, mosquito management, social behavior changes, and public health impacts for CMH (comprising 30 nodes).
Predictive Risk Model
The hazard themes provided a BN framework for the endpoints, which was critiqued and modified by experts to best reflect causal relationships of each hazard (defined in Table 1). Experts estimated the likelihoods to populate each parent node. The CPTs are provided in Tables S1–S20 in Supplementary Material.
Overall, the conditional probability that the release would not occur on time due to a hazard failure was estimated at 45.9% (moderate likelihood) (Figure 1). Sensitivity analysis revealed DAR was the most sensitive to the “compliance” node (>60%), which was in turn strongly influenced by the “formal regulatory oversight” (45%) and to a lesser extent the “community” (20%) and “political” (7%) nodes (Figure 2). “Public opinion” (15%) was sensitive to the “community,” and the “logistical constraints” node was sensitive to the “release site,” which was in turn influenced by “epidemiological issues.”
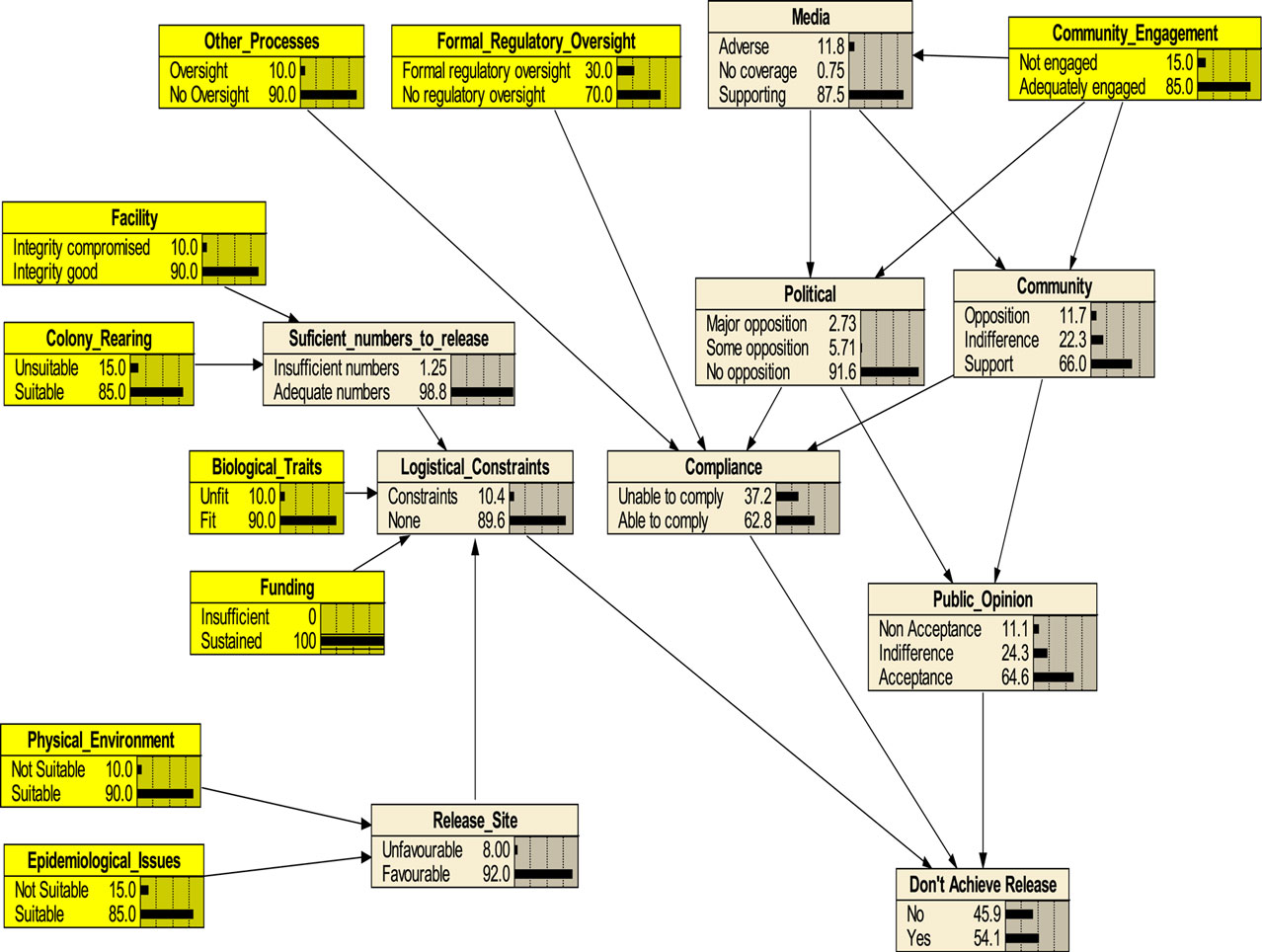
Figure 1. Bayesian belief network for the endpoint “Don’t Achieve Release.” Each node (box) and the states within nodes are described in Table 1 and Table S1 in Supplementary Material. Probabilities for terminal nodes are determined by expert estimates. Parent nodes are represented in yellow.
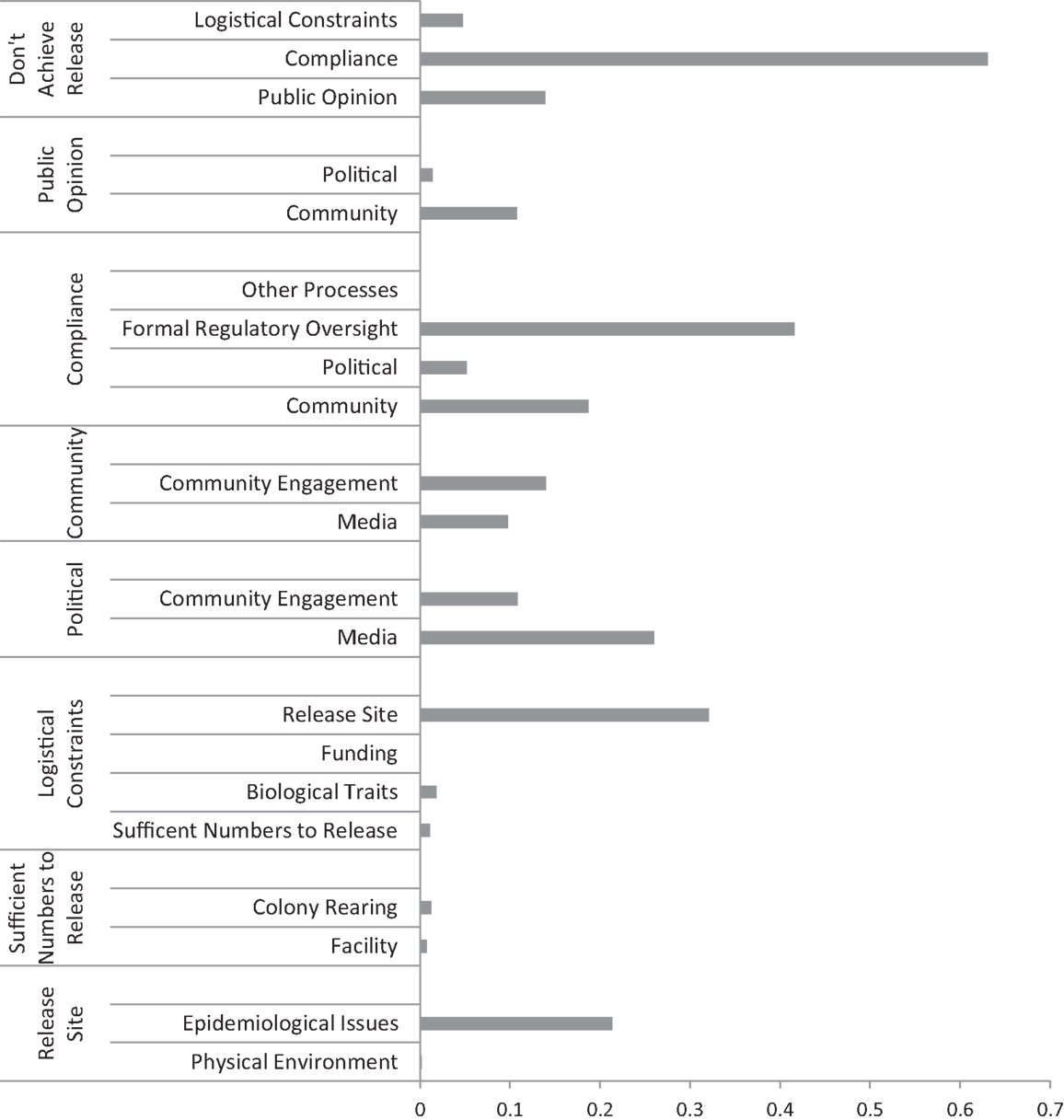
Figure 2. Sensitivity results (measured as entropy reduction) for the Bayesian belief network for the “Don’t Achieve Release” endpoint. It shows the sensitivity of the key variables (logistical constraints, compliance, and public opinion) along with the sensitivity of the variables inputting these and of the “Don’t Achieve Release” output. The longer the bar length, the more influence the variable had on the model within each category.
The probability that some forms of additional harm could eventuate over a 30-year time frame from the date of release was 12.5% (Figure 3). Sensitivity analysis (Figure 4) revealed CMH was most sensitive to the “efficacy of mosquito management” (18%), which in turn was influenced strongly by “household control.” “Standard of public health” (10%) had some influence, followed by “economic effects” (6%) and “avoidance strategies” (4%) nodes. The node “Ecology” had little effect. “Standard of public health” was strongly affected by “dengue transmission,” which in turn was heavily influenced by “dengue evolution.” Change in “tourism” had the strongest effect on the “economic effects” node (Figure 4).
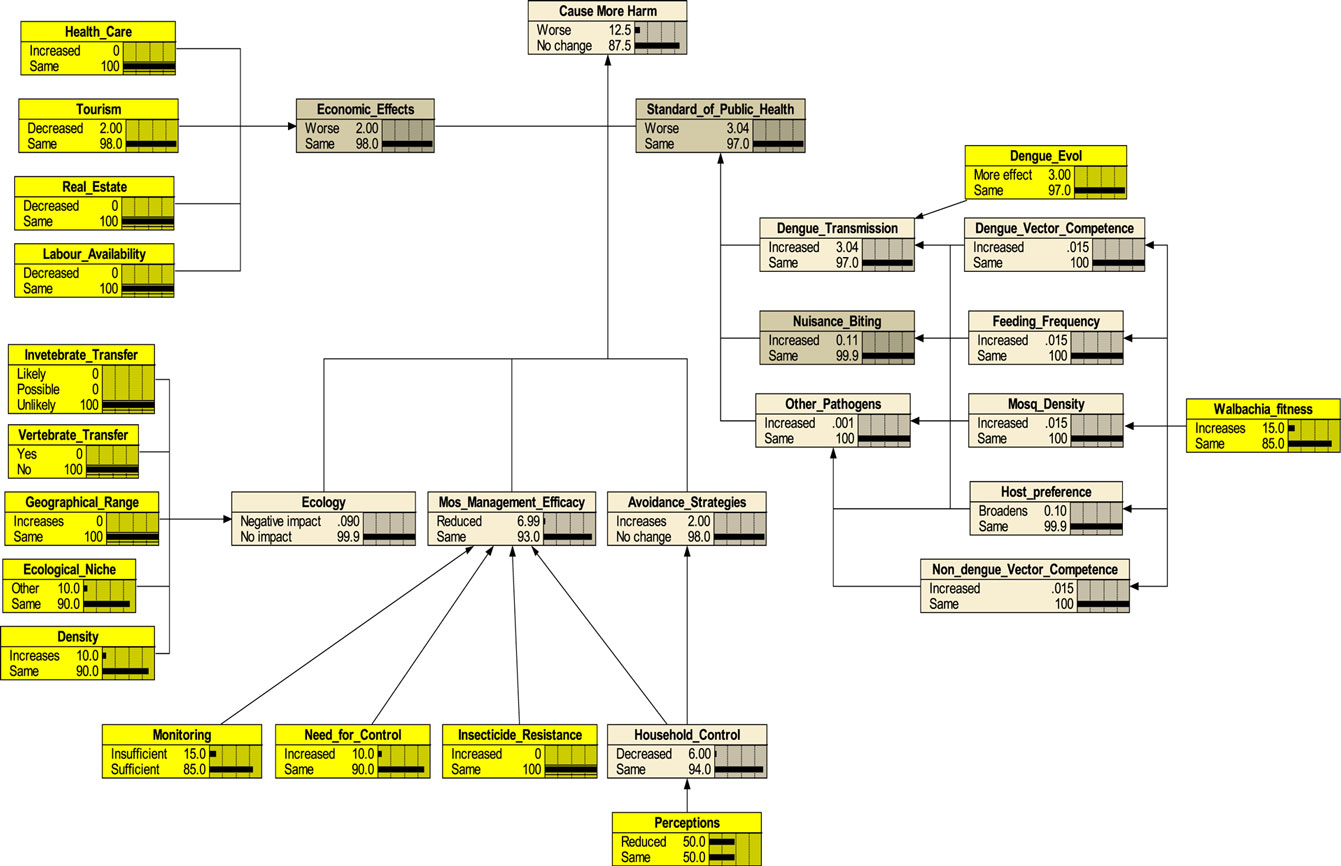
Figure 3. Bayesian belief network for the endpoint “Cause More Harm.” Each node (box) and the states within nodes are described in Table 1 and Table S1 in Supplementary Material. Probabilities for terminal nodes are determined by experts. Parent nodes are represented in yellow.
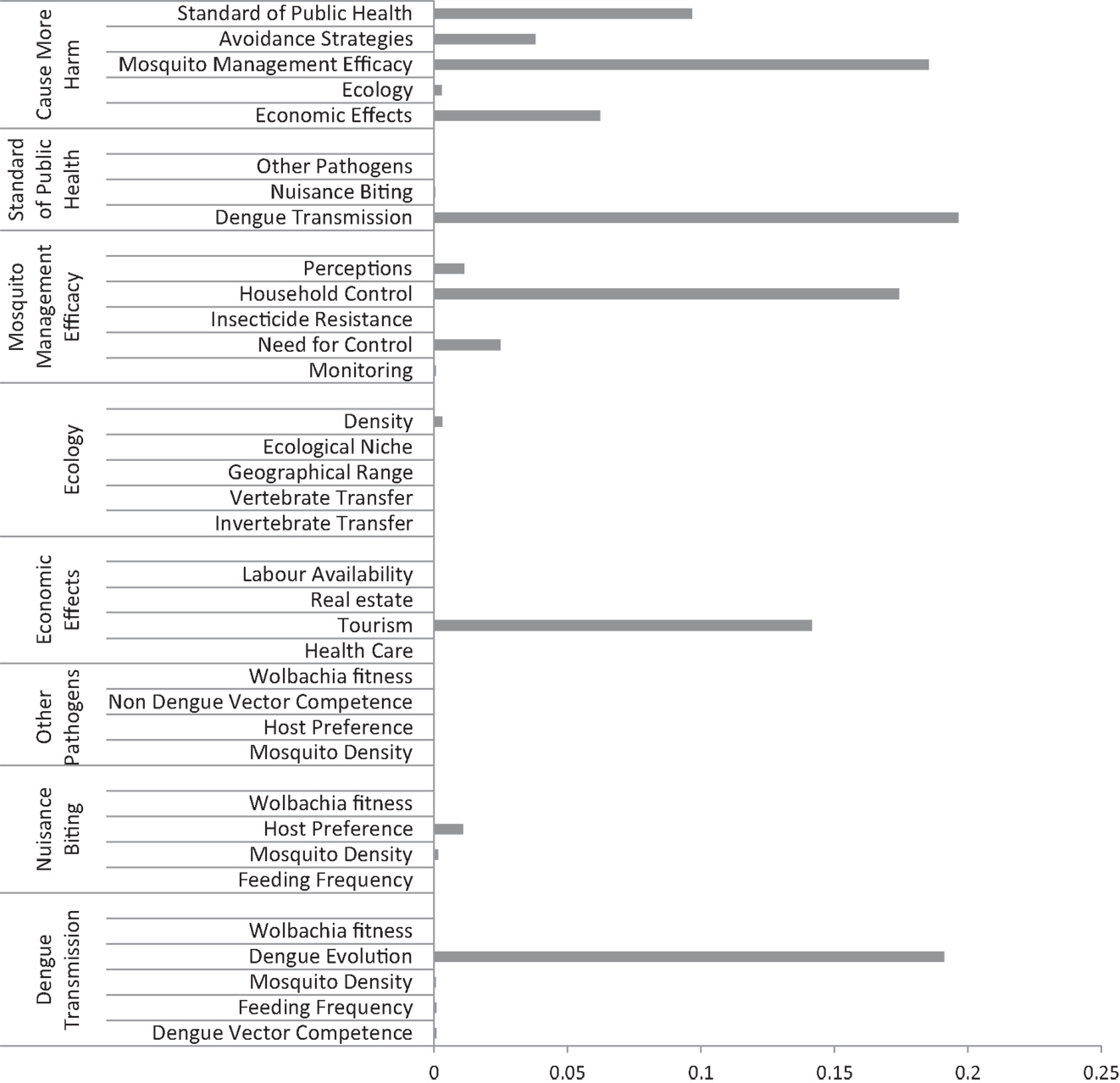
Figure 4. Sensitivity results (measured as entropy reduction) for the Bayesian belief network for the “Cause More Harm” endpoint. It shows the sensitivity of the key variables (standard of public health, avoidance strategies, mosquito management efficacy, ecology, and economic effects) along with the sensitivity of the variables inputting these and of the “Cause More Harm” output. The longer the bar length, the more influence the variable had on the model within each category.
Model Updating
Owing to the technical nature of the research and the use of a range of experts from different backgrounds and their varied knowledge of the project, different types and levels of uncertainty were evident (Murray et al., in preparation). Uncertainty arose from a number of sources, including linguistic interpretation of the hazard definitions (Table 1). Uncertainty was particularly apparent around knowledge gaps and when experts were unfamiliar with terminology, techniques, and the modeling process. Uncertainty was typically evident by a broad distribution between low and high values in elicited likelihoods (e.g., event will occur between the range of 0.1 and 0.9 likelihood). Reducing uncertainty narrows the range of possible likelihoods. Throughout the workshops and correspondence, experts were continually encouraged to provide updates on any new data arising from research currently underway and work toward consensus and removing any divergence and outliers. Effort was also invested in educating experts to reach a common linguistic understanding of node definitions. Models were continually updated when consensus was reached or new knowledge became available. For example, new information was released to reduce the uncertainty around infecting non-target species and feeding on other hosts (38).
Risk Calculation
Consensus of expert opinion on the endpoint for DAR resulted in a low likelihood (0.20) and a moderate consequence (0.35), ensuing DAR to be of very low risk (Table 3). “Biological traits” that were not suitable, a compromised “facility” and “insufficient numbers to release,” had high consequence but a very low likelihood, resulting in very low risk. This was the same level of risk for “insufficient funding,” which had a very high consequence but negligible likelihood. “Public opinion,” “community compliance,” “political,” “media,” “physical environment,” and “other processes” had negligible risk. The remaining nodes had very low risk except for problems encountered with “colony rearing,” which had a high consequence and low likelihood resulting in low risk (Table 3).
Consensus of expert opinion on the endpoint for CMH resulted in a very low likelihood (0.10) and a very low consequence (0.10), ensuing CMH to be of negligible risk (Table 4). Community “perceptions” had the highest risk with moderate likelihood (0.50) and moderate consequences (0.4) combined to render low risk. Community “perceptions” directly affected “household control.” However, “household control,” along with “avoidance strategies,” “mosquito density,” and “Wolbachia fitness,” had low likelihood and moderate consequence leading to very low risk. The remaining nodes relevant to CMH had negligible associated risk (Table 4).
Discussion
Overall Risk
Managing risk is an important component in most research and management endeavors. A risk analysis was undertaken on the proposal to release Wolbachia-infected Ae. aegypti in North Queensland, Australia, to reduce the transmission of dengue. Risk analysis considers the adverse potential outcomes that could eventuate from an event. Thus, we were only interested in whether release was not achieved and whether additional harm may result from this process. The risk assessment revealed that there was a higher likelihood of the project not achieving release than causing more harm, although the risk for both were very low. The potential risk for CMH was primarily influenced by the efficacy of alternative mosquito management actions, and the potential risk of DAR was mainly affected by whether regulatory compliance was obtained.
Don’t Achieve Release
Regulatory compliance, either formal or informal, was considered a hazard because of potential delay in the approval for the release and, hence, the release not being achieved within the designated timeframe. Compliance, in turn, was affected by the lack of formal regulatory oversight. The novelty of the proposed release was reflected in the initial failure to identify an appropriate regulatory body, which would accept governance over the proposed release and prescribe an appropriate risk analysis framework. Genetically modified organisms (GMOs) are controlled by regulatory structures (39). Notably, the modified Ae. aegypti was determined not to be a GMO by the relevant organization [The Office of the Gene Technology Regulator (OGTR)] on the basis that the method of modification did not involve recombinant technology. Nonetheless, the decision was made to use the OGTR risk analysis framework for GMOs (40) as best practice in the absence of regulatory guidance. In addition, an independent panel of local and international experts in risk analysis, biological control, and regulation were convened to oversee the methods used. The complete risk analysis was made publicly available and used to support the search for a regulator. This is an unusual situation as the risk analysis was completed before a regulator, and their requirements had been identified. Furthermore, an unusual regulatory solution was eventually found with the consideration of Wolbachia as a veterinary chemical substance governed under existing legislation (23).
The risk that public opinion may prevent release was also considered influential as it was difficult to preempt the community response to the project, and community engagement was considered vital for project success. To address this risk, long-term research had been conducted using an engagement strategy and communication materials specific to the local sociopolitical context for the communities at the release sites. Information sessions were run for the community, discussing past control methods for dengue and their limitations, the fundamentals of the project, and the expectations regarding outcomes of the project. The communities were involved and their concerns addressed at every step, enabling a reduction in the risk of opposition to the project (27, 31).
Cause More Harm
Causing more harm was considered a negligible risk. The greatest concern was whether general “mosquito management efficacy” would be maintained or if it was potentially reduced by households decreasing their efforts of mosquito control. As a consequence of the local population considering the threat of dengue transmission to be reduced following the release of w Ae. aegypti, residents may decrease efforts to minimize mosquito breeding around their home. This concern highlights the potential need for more effort and education directed toward the community to ensure that ongoing mosquito management is maintained at the household level (41, 42). Cliff and Campbell (43) also considered it was important to include perceptions, particularly behavioral intent and concern, within a biosecurity risk assessment to provide a more effective and efficient understanding of risk.
The standard of public health was also of some concern due to the potential risk of the release causing possible evolution of the dengue viruses to become a greater threat to public health. However, the likelihood of this occurring was deemed low by the technical experts. While Bennett et al. (44) suggest that dengue virus evolution can occur rapidly, the experts agreed that there is little evidence that the presence of Wolbachia in the mosquito will increase this risk, especially as evolutionary dynamics can only occur when the virus is transmitted successfully. In this case, the Wolbachia infection itself reduces the likelihood that transmission will occur (22).
Expert Elicitation
Releasing mosquitoes into the environment, in this case around human habitation, affects local communities where the release sites are targeted. This necessitates the involvement of the community through the whole process, from providing information sessions and literature (27) to inclusion in expert panels. Community experts were able to provide valuable contribution to assessing the likelihoods describing the economic effects and avoidance strategies for CMH. Community experts were also encouraged to participate in populating the other nodes but found them to be irrelevant to their personal experiences or difficult to understand the technicalities underlying the other nodes.
Because of the novelty of the technique, scientific experts were needed to populate technical nodes, such as ecology, dengue transmission, and Wolbachia fitness. The complexity of the model required a number of workshops to obtain full understanding and consensus for the different technical components of the model, especially those describing dengue evolution and Wolbachia fitness.
Risk Analysis Methodology
The four stages of the risk analysis were necessary to accommodate the complexity of the events being modeled and the uncertainty that arose from considering such innovative techniques (Murray et al., in preparation). This stepwise process provided an opportunity to collect feedback on model structure and relationships and reduce the uncertainty around nodes captured in the risk model. The brainstorming enabled all hazards to be identified and be sorted into appropriate themes directed toward each negative endpoint. Once hazards were identified and the model structure determined, BNs provided opportunity for recording likelihoods of events. It was important to minimize unnecessary complexity and keep models simple, wherever possible (45). Careful editing of models can include hazard grouping to represent overall relationships and removing rare or irrelevant hazards to allow streamlining of the model structure. The BNs can capture complex interactions between different hazards in a simplified and intuitive way. When involving non-technical community experts, it is especially important to provide them with a platform that encourages understanding with limited prior knowledge.
When estimating risk, capturing the likelihoods of an event is only half the story. An event may have a high likelihood of occurring but the consequences of that event may be minimal. Hence, combining the BN likelihood results with the associated consequences within a matrix provided a realistic estimate of the risk associated with that event (46–48). For example, “community perceptions” of dengue control had the highest risk for CMH, but this was still considered a low risk due to a minimal consequence of this outcome.
Limitations
In this assessment, expert’s opinion was used to provide likelihoods of hazard failures as a surrogate for incomplete or absent data, but this approach has limitations. For example, expert judgment is based on observation and experience, which varies both between and within the research and community representatives (49). How individuals perceive and quantify numerical risk also varies (50). Uncertainty was evident in different forms [including variability and epistemic and linguistic uncertainty (29, 51)] and although steps were taken to minimize its effect, in some cases, these efforts may have enhanced it. For example, the hazard definitions were intended to be succinct and accessible to both science and community representatives and included a glossary of key terms to avoid vagueness (Table 1; Supplementary Material). However, the elicitation exercise sent via email was notable for the linguistic difficulty some respondents experienced regarding the definitions – particularly if they had not participated in the earlier stages of model development. This manifested as highly divergent hazard scoring (broad ranges or outliers) and in some cases a lack of confidence in assigning a likelihood estimate to a hazard. The inability to easily discuss aspects, such as definitions and reach consensus on interpretation, and the occasionally low response rates are failings of this individual-focused remote approach (52). However, the value of an individual approach lies in the fact that a set of estimates from prior knowledge (known as “priors”) can be rapidly obtained without group influence. Individual priors may represent more breadth of knowledge than priors determined through group consensus. The last expert workshop was designed in response to these issues with an aim to reduce uncertainty and obtain a consensus set of priors, which adequately reflected individual expert opinion. The priors also represent a baseline for examination of the likelihoods of hazard failure and the consequences of failure. These priors can be updated when new information becomes available to further inform expert estimates or when new data can be used to replace expert estimates. For instance, where robust scientific data from laboratory testing are available on horizontal transfer rates, it may be more accurate than the equivalent expert opinion.
Risk matrices have been used extensively in risk analysis and management but suffer some limitations. Cox (53) notes that risk matrices can have poor resolution by only comparing a fraction of hazard pairs, can erroneously assign higher risks to qualitative rather than quantitative risks, and can lead to ambiguous inputs and outputs if consequences are uncertain. During our risk analysis process, we endeavored to counter these limitations. Using BNs allowed the modeling of interactions between all associated risks so all hazards, and their potential codependent hazards are accounted for within the risk matrix. The BNs were also populated entirely by expert elicitation; therefore, quantitative data were not used. However, we continually sought feedback from experts to ensure that the latest knowledge and understanding was captured in the models. Lastly, we chose technical experts with great expertise within the field to quantify consequences both individually and as a group.
While it is difficult to test and validate risk assessment models, the model results informed the independent scientific committee and the regulatory body who subsequently approved the project. Post-project results showed that the project members were able to overcome any potential risks for DAR by successfully producing the modified Ae. aegypti populations for the designated release timeframe (23). Wolbachia-infected mosquito populations to date have also been successfully established into their natural environment around the release sites (54). However, risks associated with CMH need to be monitored over the next 30 years.
Conclusion
The risk analysis highlighted factors that needed to be addressed before the release of Wolbachia-infected mosquitoes could occur. It also highlighted the uncertainty that arises from innovative research and the employment of expert opinion to populate models. We make the following five recommendations:
• Overall community engagement and media coverage need to play a significant role in obtaining project approval, especially if there are direct implications to the community.
• Inclusion of both technical and non-technical community experts captures the diversity of opinions relevant to different hazards. This is especially important when community support is essential.
• An iterative model continually updated with new knowledge can help reduce the uncertainty associated with risks as well as highlight where new research is needed to clarify issues.
• As in this case, innovative research may not be covered by existing regulatory bodies and this may lead to delays. Understanding this early can provide an opportunity and time for project managers to find solutions.
• Understanding the role that individual and community perceptions play when introducing new technology for which there is no prior example of application is very important, particularly when modeling future human behavior. In this case, introducing this technology may have led to individuals believing the dengue threat had been alleviated and thus reducing the amount of household mosquito control they conduct. Thus, adopting ethical public participation and engagement sessions to both inform and answer direct questions from members of the community can reduce this risk (27, 42, 55).
Ethics
Human research ethics was approved through the Human Research Ethics Committee (James Cook University) Approval H3555, modifying mosquito population age structure to eliminate dengue transmission: Part 1 population attributes of the dengue vector and Part 2 social research and community engagement. Institutional Biosafety was approved through the University of Queensland Institutional Biosafety Committee Approval IBC/Biosafety17/SBS/2010, field testing of Wolbachia Aedes aegypti to control dengue transmission in North Queensland.
Author Notes
JM is an ecologist with extensive experience in expert elicitation and BNs. CJ is an entomologist with extensive knowledge in mosquito research and vector-borne diseases. PDB is the Research Director of the CSIRO Health and Biosecurity Business Unit’s Risk Evaluation and Preparedness Programme with a broad knowledge in many areas, including vector ecology associated with human health and risk analysis.
Author Contributions
PDB conceived the idea and chose the methodology for the study. All authors took part in running the expert workshops. JM and CJ analyzed the model data and JM interpreted the results. All authors contributed to the writing of the manuscript.
Conflict of Interest Statement
Dr. JM reports a grant from the University of Queensland. CSIRO was contracted by the University of Queensland to undertake an independent risk assessment of the proposed release of Wolbachia Aedes aegypti. The Wolbachia Aedes aegypti research was funded by the Foundation for the National Institutes of Health through the Grand Challenges in Global Health Initiative of the Bill & Melinda Gates Foundation as part of Eliminate Dengue.
Acknowledgments
The authors thank all experts who consistently donated their time to contribute to the knowledge gathering and model building elicitation sessions. Brendan Murphy is acknowledged for his contribution in planning and running the workshops and initial data analysis. Keith Hayes is acknowledged for his advice regarding risk methodologies and Rieks van Klinken for advice on the Bayesian network. We also thank the members of the Dengue Consultation Group (DCG). We acknowledge the support of Darlene McNaughton who identified potential community participants for the workshop and provided the hazards elicited from the community, Scott Ritchie for providing data on the Ae. aegypti density for Cairns, and Jennifer Sutton for brokering contacts with the FNIH/Gates Foundation project. We are grateful to the independent panel members for their valuable feedback and to the two internal CSIRO reviewers and the anonymous reviewers for their helpful comments.
Funding
The Bill & Melinda Gates Foundation provided funds through the Foundation for the National Institutes for Health (Grand Challenges in Global Health) to The University of Queensland to contract CSIRO to run an independent risk assessment completed before the release of the modified mosquito into its natural environment to help combat dengue.
Supplementary Material
A glossary of terms, the definitions of the nodes and states for each end point, the conditional probability tables, and the complete fault trees for the two endpoints can be found at the CSIRO data access portal (http://doi.org/10.4225/08/56806A85E3E65) (56). The email elicitation template is also provided there as Supplementary Information.
Abbreviations
BN, Bayesian network; CMH, Cause More Harm; CPT, conditional probability table; DAR, Don’t Achieve Release; GCGH, Grand Challenges in Global Health; GMO, genetically modified organism; OGTR, Office of the Gene Technology Regulator.
References
1. Bosio CF, Fulton RE, Salasek ML, Beaty BJ, Black WC. Quantitative trait loci that control vector competence for dengue-2 virus in the mosquito Aedes aegypti. Genetics (2000) 156:687–98.
2. Moreira LA, Iturbe-Ormaetxe I, Jeffery JA, Lu G, Pyke AT, Hedges LM, et al. A Wolbachia symbiont in Aedes aegypti limits infection with dengue, chikungunya, and plasmodium. Cell (2009) 139:1268–78. doi: 10.1016/j.cell.2009.11.042
3. Bhatt S, Gething PW, Brady OJ, Messina JP, Farlow AW, Moyes CL, et al. The global distribution and burden of dengue. Nature (2013) 496:504–7. doi:10.1038/nature12060
4. World Health Organisation. Dengue: Guidelines for Diagnosis, Treatment, Prevention and Control. Geneva: World Health Organisation and the Special Programme for Research and Training in Tropical Diseases (2009).
5. Russell RC, Currie BJ, Lindsay MD, Mackenzie JS, Ritchie SA, Whelan PI. Dengue and climate change in Australia: predictions for the future should incorporate knowledge from the past. Med J Aust (2009) 190:265–8.
6. Messina JP, Brady OJ, Scott TW, Zou C, Pigott DM, Duda KA, et al. Global spread of dengue virus types: mapping the 70 year history. Trends Microbiol (2014) 22:138–46. doi:10.1016/j.tim.2013.12.011
7. Queensland Health. Queensland Dengue Management Plan. Queensland Government (2011). Available from: https://www.health.qld.gov.au/publications/clinical-practice/guidelines-procedures/diseases-infection/governance/dengue-mgt-plan.pdf
8. Chadee DD. Key premises, a guide to Aedes aegypti (Diptera: Culicidae) surveillance and control. Bull Entomol Res (2004) 94:201–7. doi:10.1079/BER2004297
9. Vezzani D, Rubio A, Velazquez SM, Schweigmann N, Wiegand T. Detailed assessment of microhabitat suitability for Aedes aegypti (Diptera: Culicidae) in Buenos Aires, Argentina. Acta Trop (2005) 95:123–31. doi:10.1016/j.actatropica.2005.03.010
10. Chan KL, Ho BC, Chan YC. Aedes aegypti (L.) and Aedes albopictus (Skuse) in Singapore city 2. Larval habitats. Bull World Health Organ (1971) 44:629–33.
11. Wen T-H, Lin M-H, Teng H-J, Chang N-T. Incorporating the human-Aedes mosquito interactions into measuring the spatial risk of urban dengue fever. Appl Geogr (2015) 62:256–66. doi:10.1016/j.apgeog.2015.05.003
12. Sun LV, Riegler M, O’Neill SL. Development of a physical and genetic map of the virulent Wolbachia strain wMelPop. J Bacteriol (2003) 185:7077–84. doi:10.1128/JB.185.24.7077-7084.2003
13. Perlman SJ, Hunter MS, Zchori-Fein E. The emerging diversity of Rickettsia. Proc R Soc B Biol Sci (2006) 273:2097–106. doi:10.1098/rspb.2006.3541
14. Stevens L, Giordano R, Fialho RF. Male-killing, nematode infections, bacteriophage infection, and virulence of cytoplasmic bacteria in the genus Wolbachia. Annu Rev Ecol Syst (2001) 32:519–45. doi:10.1146/annurev.ecolsys.32.081501.114132
15. Tram U, Feree PM, Sullivan W. Identification of Wolbachia – host interacting factors through cytological analysis. Microbes Infect (2003) 5:999–1011. doi:10.1016/S1286-4579(03)00192-8
16. Werren JH. Biology of Wolbachia. Annu Rev Entomol (1997) 42:587–609. doi:10.1146/annurev.ento.42.1.587
17. Cook JM, Butcher RDJ. The transmission and effects of Wolbachia bacteria in parasitoids. Res Popul Ecol (1999) 41:15–28. doi:10.1007/PL00011978
18. Dyson EA, Kamath MK, Hurst GDD. Wolbachia infection associated with all-female broods in Hypolimnas bolina (Lepidoptera: Nymphalidae): evidence for horizontal transmission of a butterfly male killer. Heredity (2002) 88:166–71. doi:10.1038/sj.hdy.6800021
19. Hurst GDD, Jiggins FM, Pomiankowski A. Which way to manipulate host reproduction? Wolbachia that cause cytoplasmic incompatibility are easily invaded by sex ratio-distorting mutants. Am Nat (2002) 160:360–73. doi:10.1086/341524
20. McGraw EA, O’Neill SL. Wolbachia pipientis: intracellular infection and pathogenesis in Drosophila. Curr Opin Microbiol (2004) 7:67–70. doi:10.1016/j.mib.2003.12.003
21. Turelli M. Evolution on incompatibility-inducing microbes and their hosts. Evolution (1994) 48:1500–13. doi:10.2307/2410244
22. Walker T, Johnson PH, Moreira LA, Iturbe-Ormaetxe I, Frentiu FD, McMeniman CJ, et al. The wMel Wolbachia strain blocks dengue and invades caged Aedes aegypti populations. Nature (2011) 476:450–U101. doi:10.1038/nature10355
23. De Barro PJ, Murphy B, Jansen CC, Murray J. The proposed release of the yellow fever mosquito, Aedes aegypti containing a naturally occurring strain of Wolbachia pipientis, a question of regulatory responsibility. J Consum Protect Food Saf (2011) 6(Suppl 1):33–40. doi:10.1007/s00003-011-0671-x
24. Burgman MA, Lindenmayer DB, Elith J. Managing landscapes for conservation under uncertainty. Ecology (2005) 86:2007. doi:10.1890/04-0906
25. Walshe T, Burgman M. A framework for assessing and managing risks posed by emerging diseases. Risk Anal (2010) 30:236–49. doi:10.1111/j.1539-6924.2009.01305.x
26. Gibbs M. Ecological risk assessment, prediction and assessing risk predictions. Risk Anal (2011) 31:1784–8. doi:10.1111/j.1539-6924.2011.01605.x
27. McNaughton D. The importance of long-term social research in enabling participation and developing engagement strategies for new dengue control technologies. PLoS Negl Trop Dis (2012) 6(8):e1785. doi:10.1371/journal.pntd.0001785
28. Spetzler CS, von Holstein CS. Probability encoding in decision analysis. Manag Sci (1975) 22:340–58. doi:10.1287/mnsc.22.3.340
29. O’Hagan A, Buck CE, Daneshkhah A, Eiser JR, Garthwaite PH, Jenkinson DJ, et al. Uncertain Judgements: Eliciting Experts’ Probabilities. West Sussex: John Wiley & Sons (2006).
30. McBride MF, Fidler F, Burgman MA. Evaluating the accuracy and calibration of expert predictions under uncertainty: predicting the outcomes of ecological research. Divers Distrib (2012) 18:782–94. doi:10.1111/j.1472-4642.2012.00884.x
31. Popovici J, Moreira LA, Poinsignon A, Iturbe-Ormaetxe I, McNaughton D, O’Neill SL. Assessing key safety concerns of a Wolbachia-based strategy to control dengue transmission by Aedes mosquitoes. Mem Inst Oswaldo Cruz (2010) 105:957–64. doi:10.1590/S0074-02762010000800002
32. Marcot BG, Holthausen RS, Raphael MG, Rowland MM, Wisdom MJ. Using Bayesian belief networks to evaluate fish and wildlife population viability under land management alternatives from an environmental impact statement. For Ecol Manage (2001) 153:29–42. doi:10.1016/S0378-1127(01)00452-2
33. Stewart-Koster B, Bunn SE, Mackay SJ, Poff NL, Naiman RJ, Lake PS. The use of Bayesion networks to guide investments in flow and catchment restoration for impaired river ecosystems. Freshw Biol (2010) 55:243–60. doi:10.1111/j.1365-2427.2009.02219.x
34. Ames DP, Neilson BT, Stevens DK, Lall U. Using Bayesian networks to model watershed management decisions: an East Canyon Creek case study. J Hydroinf (2005) 7(4):267–82.
35. Norsys Software Corp. Netica 4.12 (2009). Available from: http://www.norsys.com
36. Murray JV, Stokes KE, van Klinken RD. Predicting the potential distribution of a riparian invasive plant: the effects of changing climate, flood regimes and land-use patterns. Glob Chang Biol (2012) 18:1738–53. doi:10.1111/j.1365-2486.2011.02621.x
37. Nash D, Hannah M, Robertson F, Rifkin PA. Bayesian network for comparing dissolved nitrogen exports from high rainfall cropping in southeastern Australia. J Environ Qual (2010) 39:1699–710. doi:10.2134/jeq2009.0348
38. McMeniman CJ, Hughes GL, O’Neill SL. A Wolbachia symbiont in Aedes aegypti disrupts mosquito egg development to a greater extent when mosquitoes feed on nonhuman versus human blood. J Med Entomol (2011) 48:76–84.
39. Ramsey J, Bond J, Macotela M, Facchinelli L, Valerio L, Brown D, et al. A regulatory structure for working with genetically modified mosquitoes: lessons from Mexico. PLoS Negl Trop Dis (2014) 8:e2623. doi:10.1371/journal.pntd.0002623
40. OGTR. Risk Analysis Framework. Australian Government – Office of the Gene Technology Regulator (2009). Available from: http://www.ogtr.gov.au/internet/ogtr/publishing.nsf/Content/raf-3/$FILE/raffinal4.pdf
41. Jeelani S, Sabesan S, Subramanian S. Community knowledge, awareness and preventive practices regarding dengue fever in Puducherry – South India. Public Health (2015) 129:790–6. doi:10.1016/j.puhe.2015.02.026
42. Dhar-Chowdhury P, Haque CE, Driedger SM. Dengue disease risk mental models in the city of Dhaka, Bangladesh: juxtapositions and gaps between the public and experts. Risk Anal (2015). doi:10.1111/risa.12501
43. Cliff N, Campbell ML. Perception as a tool to inform aquatic biosecurity risk assessments. Aquat Invasions (2012) 7:387–404. doi:10.3391/ai.2012.7.3.010
44. Bennett SN, Drummond AJ, Kapan DD, Suchard MA, Munoz-Jordan JL, Pybus OG, et al. Epidemic dynamics revealed in dengue evolution. Mol Biol Evol (2010) 27:811–8. doi:10.1093/molbev/msp285
45. Zellner A, Keuzenkamp HA, McAleer M, editors. Simplicity, Inference and Modelling: Keeping It Sophisticatedly Simple. Cambridge: Cambridge University Press (2001).
46. Xiong W, Feng Z, Matsu T, Foxwell AR. Risk assessment of human infection with a novel bunyavirus in China. Western Pac Surveill Response J (2012) 3:61–6. doi:10.5365/WPSAR.2012.3.4.002
47. Campbell ML. Organism impact assessment: risk analysis for post-incursion management. ICES J Mar Sci (2008) 65:795–804. doi:10.1093/icesjms/fsn083
48. Iverson LR, Matthews SN, Prasad AM, Peters MP, Yohe G. Development of risk matrices for evaluating climatic change responses of forested habitats. Clim Change (2012) 114:231–43. doi:10.1007/s10584-012-0412-x
49. Regan HM, Colyvan M, Burgman MA. A taxonomy and treatment of uncertainty for ecology and conservation biology. Ecol Appl (2002) 12:618–28. doi:10.1890/1051-0761(2002)012[0618:ATATOU]2.0.CO;2
50. Peters E. Numeracy and the perception and communication of risk. Ann N Y Acad Sci (2008) 1128:1–7. doi:10.1196/annals.1399.001
51. Speirs-Bridge A, Fidler F, Mcbride M, Flander L, Cumming G, Burgman M. Reducing overconfidence in the interval judgements of experts. Risk Anal (2010) 30:512–23. doi:10.1111/j.1539-6924.2009.01337.x
52. Martin TG, Burgman MA, Fidler F, Kuhnert PM, Low-Choy S, McBride M, et al. Eliciting expert knowledge in conservation science. Conserv Biol (2012) 26:29–38. doi:10.1111/j.1523-1739.2011.01806.x
53. Cox LA. What’s wrong with risk matrices? Risk Anal (2008) 28(2):497–512. doi:10.1111/j.1539-6924.2008.01030.x
54. Hoffmann AA, Montgomery BL, Popovici J, Iturbe-Ormaetxe I, Johnson PH, Muzzi F, et al. Successful establishment of Wolbachia in Aedes populations to suppress dengue transmission. Nature (2011) 476:454–U107. doi:10.1038/nature10356
55. Arellano C, Castro L, Daiz-Caravantes RE, Ernst KC, Hayden M, Reyes-Castro P. Knowledge and beliefs about dengue transmission and their relationship with prevention practices in Hermosillo, Sonora. Front Public Health (2015) 3:142. doi:10.3389/fpubh.2015.00142
56. Murray J, De Barro P. Supplementary Information for Risk Analysis on Releasing Wolbachia-Infected Aedes aegypti Mosquitoes into the Environment. v3. CSIRO. Data Collection (2013). Available from: http://doi.org/10.4225/08/56806A85E3E65
Keywords: impact assessment, dengue, Wolbachia, Aedes aegypti, release, risk analysis
Citation: Murray JV, Jansen CC and De Barro P (2016) Risk Associated with the Release of Wolbachia-Infected Aedes aegypti Mosquitoes into the Environment in an Effort to Control Dengue. Front. Public Health 4:43. doi: 10.3389/fpubh.2016.00043
Received: 31 December 2015; Accepted: 04 March 2016;
Published: 22 March 2016
Edited by:
Fabrice Merien, Auckland University of Technology, New ZealandReviewed by:
Steven Sinkins, Lancaster University, UKRubén Bueno-Marí, University of Valencia, Spain
Copyright: © 2016 Murray, Jansen and De Barro. This is an open-access article distributed under the terms of the Creative Commons Attribution License (CC BY). The use, distribution or reproduction in other forums is permitted, provided the original author(s) or licensor are credited and that the original publication in this journal is cited, in accordance with accepted academic practice. No use, distribution or reproduction is permitted which does not comply with these terms.
*Correspondence: Justine V. Murray, justine.murray@csiro.au