- 1Singapore General Hospital, Singapore
- 2Family Medicine, Duke-NUS Medical School, Singapore
- 3Saw Swee Hock School of Public Health, National University of Singapore, Singapore
- 4Centre for Quantitative Medicine, Duke-NUS Medical School, Singapore
Background: Residence in public rental housing is an area-level measure of socioeconomic status, but its impact as a social determinant of health in Singapore has not been studied. We therefore aimed to examine the association of public rental housing with readmission risk and increased utilization of hospital services in Singapore.
Methods: We conducted a retrospective cohort study using retrospective 2014 data from Singapore General Hospital’s electronic health records. Variables known to affect readmission risk and health-care utilization were identified a priori and include patient demographics, comorbidities, health-care utilization in the preceding 1 year and clinical variables from the index admission in 2014. Multivariate logistic regression was used to evaluate public rental housing as an independent risk factor for admission risk, emergency department (ED), and specialist outpatient clinic attendances.
Results: A total of 14,457 unique patients were analyzed, and 2,163 patients (15.0%) were rental housing residents. Rental housing patients were significantly more likely to be male; required financial assistance; have chronic obstructive pulmonary disease; usage of anti-depressant and anti-psychotic medications; longer length of hospital stay during the index admission; and higher Charlson Comorbidity Index scores. After adjusting for demographics and clinical variables, staying in public rental housing remained an independent risk factor for readmission within 15 and 30 days, frequent hospital admissions and ED attendances in Singapore.
Conclusion: Our study showed an association between public rental housing with readmission risk and increased utilization of hospital services in Singapore. A deeper understanding of the residents’ social circumstances and health seeking behavior would be insightful.
Introduction
Low socioeconomic status (SES) is well recognized as an independent risk factor for various adverse health outcomes, such as readmission risk (1–3) and hospital utilization (4). Differences in SES contribute significantly to variations in readmission rates between hospitals (5, 6). Most studies evaluating the role of SES have concentrated on individual-level measures of SES, such as educational level, income inequality (7), employment status, or requirement for financial assistance (2). Unfortunately, such detailed information on SES are rarely captured as part of routine clinical care. This limits their utility in estimating readmission risk or planning interventions for high risk patients for whom such information is often unavailable.
Health is determined by a complex interaction between individual characteristics, lifestyle, and the physical, social, and economic environment (8). The interaction of a person’s health with the health system and the community is also well documented in Wagner’s chronic care model (9). The aging population and high prevalence of chronic diseases in many countries had resulted in rapidly escalating demand for hospital services. There is increasing acceptance that adopting a population health approach that promotes healthy communities beyond the hospital setting is an essential component of the new paradigm (10). Some preliminary work on area-level or neighborhood SES measures suggest that these are sensitive indicators that can be used to estimate the SES effect on individual’s risk of hospital utilization (11, 12). Housing as an important social determinant of health is well acknowledged (13, 14). Disadvantaged housing condition is associated with poor health including a higher prevalence of infectious diseases, injuries, and chronic diseases (13). Poor housing conditions, such as poor sanitation, crowding, poor indoor air quality, and inadequate ventilation, contribute to communicable diseases, chronic diseases, injuries, and poor health outcomes. It is also closely related to low SES and social instability which by itself predisposes to malnutrition, lack of access to affordable health care, and poor health (15).
However, the use of area-level estimates of the SES effect may not be effective in communities where there is no clear geographical segregation of housing along SES lines. In Singapore, for example, housing policy had been used as a social engineering tool to prevent the formation of ghettos (16). There remain markers of low SES associated with housing that can be used to identify individuals at risk. A survey by George et al. suggests that patients staying in one to two room public rental flats in Singapore have higher health-care utilization (17), although the study was limited by the small sample size and self-reporting of outcomes. Nevertheless, residence in public rental housing appears to be as promising as an area-level measure of SES in Singapore.
Singapore is an affluent Asian economy (gross domestic product of about S$73,000) with a multi-ethnic population of 5.6 million people. In Singapore, home ownership is a key local indicator of SES (18). For the needy, heavily subsidized public rental housing is available from the Housing Development Board. About 5.3% of resident households in Singapore reside in public rental housing in 2014 (19). To be eligible for public rental housing at highly subsidized rates, the total household gross income must be very low and not exceed S$1,500 per month. Those staying in public rental housing earn S$2,313 per month, while the national median household income is S$8,290 per month (20).
Therefore, public rental housing is a good area-level measure of SES in Singapore. Using public rental housing as a marker of low SES in this study, we sought to study the impact of residence in public rental housing on readmission risk and hospital services utilization [admissions, emergency department (ED) attendances, specialist outpatient clinic (SOC) attendance] in Singapore.
Materials and Methods
Study Design
This is an observational study using retrospective 2014 data from Singapore General Hospital (SGH) electronic health records (EHRs).
Setting and Study Population
Ministry of Health Singapore created six regional health systems (RHSs) in 2011, each being responsible to adopt a population health approach and integrate care for a specific geographic region. The Singapore Health Services (SingHealth) RHS is the largest health-care cluster in Singapore providing care for the South-central part of Singapore. Each RHS is anchored by an acute hospital, supported by a community hospital providing stepdown and rehabilitation care and complete with linkages to primary care and long-term care services in the region. SGH is the flagship hospital of the SingHealth RHS and the largest tertiary hospital in Singapore with 37 clinical specialties and 88,000 inpatient admissions each year.
To be eligible for inclusion in our study, patients must have at least one clinical encounter (admission or ED visit) to SGH in 2014. Patients who died in 2014 or are non-residents were excluded from analysis. We excluded patients who died as they would not have the whole of 2014 to accumulate readmissions and/or ED visits for our study outcomes. Similarly, we excluded patients who resided in areas where SGH is not the primary hospital, as these patients are likely to be cared for by a different RHS. Similarly, patients discharged to long-term residential care facilities were excluded as these could be located in geographical areas outside of the region served by the SingHealth RHS and confound the frequency of subsequent hospital admissions.
Data Collection, Variables, and Outcome Measures
Patient-related data (demographics, clinical data, health services utilization, and mortality) were extracted in a de-identified format from the hospital’s enterprise analytics platform.eHINTS (Electronic Health INTelligence System) is the enterprise data repository and analytical platform, which serves the analysis and reporting needs of finance, operations, and clinical users in SingHealth. It integrates financial, operational, and clinical data from multiple systems, including the EHR, across SingHealth institutions to provide users with comprehensive information. Its analytical tools, “self-service” drill down capabilities and location analytics enables faster and more efficient reporting and analysis.
We adjusted for the variables that can affect readmission risk and hospital utilization based on the previous literature to evaluate the independent association of public rental housing with readmission risk and hospital utilization (21–23).
Patient demographics extracted include age, gender, marital status, race, and admission ward class. Our study used residence in public rental housing (housing development board one and two room rental flats) as an indicator of SES in Singapore. Postal codes are unique for each apartment block in Singapore, and all flats in the same block share the same postal code instead of individual codes. In our dataset, public rental housing is recoded based on postal codes published by the Singapore Government (18). Hospital admission payment mode and medical insurance variables, including coinsurance, Medishield, Medical Claims Proration System (MCPS), Medifund, others, and self-payer, were also extracted.
Clinical data extracted included major diseases listed under the Charlson Comorbidity Index (CCI) and the number of surgical procedures, length of stay of index admission, and urgency of index admission. The CCI consist of 17 major diseases, including myocardial infarction, congestive heart failure, cerebrovascular accident, dementia, and diabetes (24). These diseases were identified using International Classification of Diseases (ICD-10) codes in any primary or secondary diagnosis fields dating back to 1 year preceding the index admission. The CCI score was computed for each patient using the using the comorbidities.icd10 package (https://github.com/gforge/comorbidities.icd10) in R version 3.2.3 (R Foundation, Vienna, Austria) during preprocessing of the data. The LACE score (length of stay, acuity of admission, CCI, and ED visits in past 6 months) derived in Ontario, Canada is calculated by summing the points of the above four variables (25). To examine the effect of high risk clinical conditions, we derived the diagnoses of congestive cardiac failure, cerebrovascular disease, chronic obstructive pulmonary disease, and malignancies using ICD-10 codes (26) in our dataset. Depression or other mental conditions were inferred by identifying patients on anti-depressant treatment (mirtazapine, fluvoxamine, escitalopram, and fluoxetine) and anti-psychotic treatment (haloperidol, olanzapine, and risperidone) based on the discharge medications in the EHR. Prior health-care utilization (number of admissions, number of ED visits, and number of SOC visits) in the preceding 1 year were also extracted.
The outcomes studied were (1) readmission within 30 days of discharge; (2) readmission within 15 days of discharge; (3) frequent admissions in 2014, defined as a patient with three or more admissions in 1 year by the Ministry of Health Singapore with an average health-care cost per year approaching S$30,000 (27); (4) frequent ED attendances in 2014, defined as a patient with four or more ED attendances on 1 year by the Ministry of Health Singapore; and (5) frequent outpatient clinic attendance in 2014. These outcomes were chosen as they represent patients who are frequent users of the health-care system. This study was approved by the Singapore Health Services (SingHealth) Centralized Institutional Review Board (CIRB Ref: 2015/2076) with a waiver of patient consent.
Statistical Analysis
The demographics, clinical, and health services utilization data were compared between patients residing in rental housing and those who are not. Chi-square test or Fisher exact test was used to compare categorical factors associated with staying in rental housing where appropriate. Independent samples t-test was used to compare normally distributed continuous variables and Mann–Whitney U-test was used to assess the continuous variables, which are not normally distributed. We assessed the independent relationship between residing in rental housing and hospital reutilization and readmission outcomes after adjusting for the demographic and clinical characteristics documented in the previous literature (21–23, 28). We selected the included variables in the multivariate analyses based on whether they were significant at p < 0.05 in the univariate analysis and used a backward step-wise procedure in the multivariate models. The model selection was also assessed using the Akaike’s Information Criterion (AIC) and Bayesian information criterion (BIC). Modified Poisson regression model with a robust error variance was used to estimate the relative risk (RR) of the outcomes, since studies have shown that odds ratio (OR) from logistic regression models can substantially overestimate the RR when the outcome is common with the incidence of 10% or more (29, 30). We used the negative binomial model to assess the relationship between rental housing and frequent SOC attendance outcomes since the counts of frequent outpatient attendance were Poisson distributed and showed over-dispersion. Statistical significance was set at p < 0.05.
This study defined frequent hospital admissions as three or more hospital admission in 1 year (27). Frequent attenders to ED were defined as patients who attended the ED four or more times a year (31). All data analyses were performed with Stata version 13 (StataCorp, College Station, TX, USA).
Results
A total of 14,457 unique patients fulfilled both inclusion and exclusion criteria were analyzed, and 2,163 patients (15.0%) were rental housing residents. Table 1 shows the characteristics of included patients stratified by staying at rental housing. The majority of patients were Chinese and those with unknown marital status. Patients who stayed at rental housing were associated with younger age, male gender, non-Chinese ethnicity, requiring financial assistance, chronic obstructive pulmonary disease, usage of anti-depressant and psychotic medication, longer length of hospital stay during the index admission, higher LACE scores, higher proportion of patients with comorbidities, readmission within 15 and 30 days, frequent hospital admissions (three or more), and frequent ED visits (four or more). But, residing in rental housing was associated with fewer SOC visits.
After adjusting for demographics and clinical conditions known to affect readmission risk and hospital services utilization, the RR of readmission within 15 and 30 days associated with residence in public rental housing was 1.19 (1.02–1.39), p = 0.029 and 1.27 (1.12–1.43), p < 0.001, respectively (Table 2). Patients staying in public rental housing have a 19 and 27% higher odds of being readmitted within 15 and 30 days, respectively.
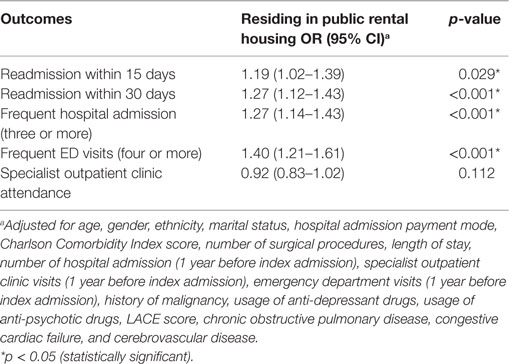
Table 2. The impact of residing in public rental housing on readmission risk and hospital services utilization.
The RR of frequent hospital admissions and frequent ED attendances associated with residence in public rental housing was 1.27 (1.14–1.43), p < 0.001 and 1.40 (1.21–1.61), p < 0.001, respectively (Table 2). Patients staying in public rental housing have a 27 and 40% higher risk of being a frequent hospital admitter and frequent ED attendee, respectively. Staying in public rental housing showed a 8% lower risk per one SOC visit, but the result was statistically non-significant, 0.92 (0.83–1.02), p = 0.112 (Table 2).
Discussion
We set out to study the association of public rental housing as an area-level measure of SES with readmission risk and hospital services utilization. We found that residents in public rental housing have higher risk of 15-day, 30-day readmissions and being a frequent admitter and frequent ED attendee, even after adjustment for demographics and clinical factors. The consistent trend across four outcomes shows a strong, consistent link between residence in public rental housing and increased hospital utilization. Although the Singapore model of housing, health-care structure, and financing is unique from other countries, our findings add to existing literature on an association between low SES and increased hospital utilization. Our study also highlights the importance and possibility of building healthier communities beyond hospital walls to reduce utilization of hospital services.
Singapore offers universal health-care coverage but adheres to the philosophy of individual responsibility where citizens are expected to make co-payment on top of government subsidies. This co-payment amount, in turn, may be reduced further by three major financing schemes, such as Medisave, MediShield Life, and Medifund. Medisave is a mandatory health savings account and can be withdrawn to pay the hospital bills of the account holder and immediate family members. Medishield Life is a basic health insurance plan, which helps to pay for large hospital bills and selected costly outpatient treatments, such as dialysis and chemotherapy for cancer. The premiums are subsidized by the government and can be paid for through the patients’ own Medisave account. Finally, Medifund is a safety net that is designed as a funding of last resort for patients who are needy and unable to pay despite optimal use of the other financial support schemes. This is to ensure that no citizen is denied appropriate essential health care. In a ranking of health-care systems of the world with a strong emphasis on access, equality and health-care financing, Singapore was ranked 6th out of 191 countries (32). Therefore, affordability and access to health care are unlikely to have a major impact on health-care utilization pattern of patients with low SES in Singapore.
In medicine, there is a preoccupation with developing health-care policies targeting medical determinants of health, such as genetic predisposition and disease-specific risk factors. There is now increasing awareness of the importance developing health policies in the context of the social environment and the social determinants of health (33). Singapore’s public housing policy is considered to be one of the most progressive in the world with 82% of its population living in high quality public housing. It has the highest home ownership rate in the world at 93%. Even under such a favorable setting, housing remains a strong determinant of health (34). The implications of our findings are that policy makers need to engage rental housing residents to obtain a deeper insightful understanding of the social circumstances, health seeking behavior, and health literacy. Addressing these actionable risk factors may reduce unnecessary health-care utilization.
The reasons behind poor outcomes in patients residing in public rental housing are many. Disadvantaged housing condition is both a direct cause of poor health through poor household conditions, such as sanitation, overcrowding, and poor indoor air quality, contributing to communicable diseases and exacerbations of chronic illnesses and a marker of low SES and social instability that compromises access to health care (13, 35). In our study, patients staying in public rental housing in Singapore have more comorbidities as indicated by their higher CCI scores. They are more likely to be on anti-depressant treatment, consistent with findings from Wee et al. (36). Interestingly, they do not appear to be more likely to have cancer, congestive heart failure, or stroke, but the prevalence of chronic obstructive pulmonary disease is higher and statistically significant. Unfortunately, we do not have data on smoking habit of the subjects, which might provide possible explanation of the observed difference. On the other hand, this could also mean that housing has a direct impact on the prevalence and optimal control of COPD. Indoor air pollution had been shown to be associated with chronic lung diseases (37). Other studies had shown that COPD as a chronic disease is most sensitive to the effects of social deprivation (38, 39). This is consistent with our findings.
In our study, patients in public rental housing are more likely to be single, widowed, or divorced. There is an over-representation of minority ethnic groups in comparison to the general population. These are factors that are known to be associated with poorer health outcome. Housing therefore may be a good composite indicator of socioeconomic disadvantage and explains its importance as a social determinant of health. Health-care policies aimed at rationalizing the use of hospital resources must take socioeconomic factors into consideration. Data about housing are unobtrusive and readily available in most hospital administrative datasets. Our study showed that housing can be associated with high health-care needs and utilization of hospital resources. This knowledge can help us to allocate resources and develop medical and social interventions to help such individuals and improve programs aimed at optimizing cost effectiveness of health-care systems.
For patients of low SES, the hospital discharge plan prescribed by health-care workers are often confusing, unrealistic, and impractical in the face of significant socioeconomic constraints and more pressing needs that such patients may face in their daily life (40). In-depth interviews by Kangovi et al. found that residents in high poverty areas were unable to access the care and accommodations needed to cope with post-hospital frailty. As a result, medical disability was amplified by socioeconomic disability (41).
We found it interesting that rental housing residents in our cohort have a different pattern of utilization of health-care resources. They visited fewer specialists in the outpatient setting. There are few studies that evaluated the impact of SES on outpatient specialist use and Filc et al. reported that patients with higher SES visited more specialists (4), which is similar to our results. Although our data do not include primary care utilization, a study by Wee et al. found that western-trained physicians are not the first choice of lower income Singaporeans seeking primary care. Many preferred alternative medicine or self-medication (42). Barriers, such as poor knowledge, costs, and characteristics of primary care practices, must be addressed if better outcomes were to be achieved.
Limitations
Although our study was carefully prepared, there were some limitations. First, our dataset did not include patients who could have utilized only outpatient services in our health system. However, these patients are low risk, and our aim was to study the association of housing with readmission risk after an index hospital admission and subsequent hospital services utilization. Second, variables in our dataset are restricted to those routinely collected in the EHR and administrative databases. As such, the granularity of social determinant variables is restricted to housing and payment modes, and functional status was not available. By excluding patients who were discharged to long-term institutional care, we likely minimized gross differences in function between rental housing and non-rental housing patients. Third, due to the retrospective nature of the study, we were unable to prove a causal association between rental housing and health outcomes. In addition, although our study was conducted at the largest health system in Singapore, we were unable to account for readmissions to other health systems. We minimized such bias by excluding patients who stay in geographical locations served by other health systems. Finally, although our study adds to existing literature that housing is a good proxy marker of SES and is independently associated with increased hospital services utilization, and this should be interpreted within the context of Singapore’s unique housing and health-care policies.
Conclusion
Our study found that public rental housing as an area-level measure of SES in Singapore is independently associated with increased readmission risk and being a frequent hospital admitter and ED user. Policy makers and the health system need to engage rental housing residents to obtain a deeper insightful understanding of the social circumstances, health seeking behavior, and health literacy. Intervening in these modifiable risk factors may reduce unnecessary health-care utilization.
Ethics Approval
This study was approved by the Singapore Health Services (SingHealth) Centralized Institutional Review Board (CIRB Ref: 2015/2076) with a waiver of patient consent.
Author Contributions
Conceived and designed the study: LL and KL. Performed the study: LL, WW, and NL. Analyzed the data: WW and LL. Interpreted the results: LL and KL. Wrote the paper: LL, WW, and KL. Principal Investigator of this study and supervised this study: LL. Revised the paper critically and give final approval for publication: all authors.
Conflict of Interest Statement
The authors declare that the research was conducted in the absence of any commercial or financial relationships that could be construed as a potential conflict of interest.
Funding
This is an investigator-initiated study, and no grant funding is obtained.
Abbreviations
CCI, Charlson Comorbidity Index; ED, emergency department; RHS, Regional Health System; SES, socioeconomic status; SGH, Singapore General Hospital.
References
1. Krumholz HM, Bernheim SM. Considering the role of socioeconomic status in hospital outcomes measures. Ann Intern Med (2014) 161(11):833–4. doi:10.7326/M14-2308
2. Arbaje AI, Wolff JL, Yu Q, Powe NR, Anderson GF, Boult C. Postdischarge environmental and socioeconomic factors and the likelihood of early hospital readmission among community-dwelling Medicare beneficiaries. Gerontologist (2008) 48(4):495–504. doi:10.1093/geront/48.4.495
3. Hu J, Gonsahn MD, Nerenz DR. Socioeconomic status and readmissions: evidence from an urban teaching hospital. Health Aff (Millwood) (2014) 33(5):778–85. doi:10.1377/hlthaff.2013.0816
4. Filc D, Davidovich N, Novack L, Balicer RD. Is socioeconomic status associated with utilization of health care services in a single-payer universal health care system? Int J Equity Health (2014) 13(1):115. doi:10.1186/s12939-014-0115-1
5. Nagasako EM, Reidhead M, Waterman B, Dunagan WC. Adding socioeconomic data to hospital readmissions calculations may produce more useful results. Health Aff (Millwood) (2014) 33(5):786–91. doi:10.1377/hlthaff.2013.1148
6. Barnett ML, Hsu J, McWilliams JM. Patient characteristics and differences in hospital readmission rates. JAMA Intern Med (2015) 175(11):1803–12. doi:10.1001/jamainternmed.2015.4660
7. Lindenauer PK, Lagu T, Rothberg MB, Avrunin J, Pekow PS, Wang Y, et al. Income inequality and 30 day outcomes after acute myocardial infarction, heart failure, and pneumonia: retrospective cohort study. BMJ (2013) 346:f521. doi:10.1136/bmj.f521
8. Fund TKs. Broader Determinants of Health. (2015). Available from: http://www.kingsfund.org.uk/time-to-think-differently/trends/broader-determinants-health
9. Austin B, Wagner E, Hindmarsh M, Davis C. Elements of effective chronic care: a model for optimizing outcomes for the chronically ill. Epilepsy Behav (2000) 1(4):S15–20. doi:10.1006/ebeh.2000.0105
10. Goldman TR. Building healthy communities beyond the hospital walls. Health Aff (Millwood) (2014) 33(11):1887–9. doi:10.1377/hlthaff.2014.1142
11. Kind AJ, Jencks S, Brock J, Yu M, Bartels C, Ehlenbach W, et al. Neighborhood socioeconomic disadvantage and 30-day rehospitalization: a retrospective cohort study. Ann Intern Med (2014) 161(11):765–74. doi:10.7326/M13-2946
12. Bikdeli B, Wayda B, Bao H, Ross JS, Xu X, Chaudhry SI, et al. Place of residence and outcomes of patients with heart failure: analysis from the telemonitoring to improve heart failure outcomes trial. Circ Cardiovasc Qual Outcomes (2014) 7(5):749–56. doi:10.1161/CIRCOUTCOMES.113.000911
13. Krieger J, Higgins DL. Housing and health: time again for public health action. Am J Public Health (2002) 92(5):758–68. doi:10.2105/AJPH.92.5.758
14. Dunn JR, Hayes MV, Hulchanski JD, Hwang SW, Potvin L. Housing as a socio-economic determinant of health: findings of a national needs, gaps and opportunities assessment. Can J Public Health (2006) 97:S11–5. doi:10.17269/cjph.97.1559
15. Regidor E. Social determinants of health: a veil that hides socioeconomic position and its relation with health. J Epidemiol Community Health (2006) 60(10):896–901. doi:10.1136/jech.2005.044859
16. Phang S-Y. The Singapore Model of Housing and the Welfare State. In: Groves R, Murie A, Watson C, editors. Housing and the New Welfare State: Perspectives from East Asia and Europe, Research Collection School of Economics. Aldershot: Ashgate (2007). p. 15.
17. George PP, Heng BH, De Castro Molina JA, Wong LY, Wei Lin NC, Cheah JT. Self-reported chronic diseases and health status and health service utilization – results from a community health survey in Singapore. Int J Equity Health (2012) 11:44. doi:10.1186/1475-9276-11-44
18. Singapore HaDB. Map to Find Location of Rental Flats. (2015). Available from: https://services2.hdb.gov.sg/webapp/AA11EMAP/AA11PMainPage
19. Singapore DoS. Population Trends 2015. (2015). Available from: http://www.singstat.gov.sg/publications/publications-and-papers/population-and-population-structure/population-trends
20. Singapore DoS. Key Household Income Trends, 2014. (2015). Available from: http://www.singstat.gov.sg/publications/publications-and-papers/population#household_income_and_expenditure
21. Low LL, Lee KH, Hock Ong ME, Wang S, Tan SY, Thumboo J, et al. Predicting 30-day readmissions: performance of the LACE index compared with a regression model among general medicine patients in Singapore. Biomed Res Int (2015) 2015:169870. doi:10.1155/2015/169870
22. Tan SY, Low LL, Yang Y, Lee KH. Applicability of a previously validated readmission predictive index in medical patients in Singapore: a retrospective study. BMC Health Serv Res (2013) 13:366. doi:10.1186/1472-6963-13-366
23. Kansagara D, Englander H, Salanitro A, Kagen D, Theobald C, Freeman M, et al. Risk prediction models for hospital readmission: a systematic review. JAMA (2011) 306(15):1688–98. doi:10.1001/jama.2011.1515
24. Deyo RA, Cherkin DC, Ciol MA. Adapting a clinical comorbidity index for use with ICD-9-CM administrative databases. J Clin Epidemiol (1992) 45(6):613–9. doi:10.1016/0895-4356(92)90133-8
25. van Walraven C, Dhalla IA, Bell C, Etchells E, Stiell IG, Zarnke K, et al. Derivation and validation of an index to predict early death or unplanned readmission after discharge from hospital to the community. CMAJ (2010) 182(6):551–7. doi:10.1503/cmaj.091117
26. Quan H, Sundararajan V, Halfon P, Fong A, Burnand B, Luthi JC, et al. Coding algorithms for defining comorbidities in ICD-9-CM and ICD-10 administrative data. Med Care (2005) 43(11):1130–9. doi:10.1097/01.mlr.0000182534.19832.83
27. Saxena N, You AX, Zhu Z, Sun Y, George PP, Teow KL, et al. Singapore’s regional health systems – a data-driven perspective on frequent admitters and cross utilization of healthcare services in three systems. Int J Health Plann Manage (2015). doi:10.1002/hpm.2300
28. Shadmi E, Flaks-Manov N, Hoshen M, Goldman O, Bitterman H, Balicer RD. Predicting 30-day readmissions with preadmission electronic health record data. Med Care (2015) 53(3):283–9. doi:10.1097/MLR.0000000000000315
29. McNutt LA, Wu C, Xue X, Hafner JP. Estimating the relative risk in cohort studies and clinical trials of common outcomes. Am J Epidemiol (2003) 157(10):940–3. doi:10.1093/aje/kwg074
30. Zou G. A modified Poisson regression approach to prospective studies with binary data. Am J Epidemiol (2004) 159(7):702–6. doi:10.1093/aje/kwh090
31. Locker TE, Baston S, Mason SM, Nicholl J. Defining frequent use of an urban emergency department. Emerg Med J (2007) 24(6):398–401. doi:10.1136/emj.2006.043844
32. Organization WH. The World Health Report 2000: Health Systems: Improving Performance. Geneva: World Health Organization (2000).
33. Wilkinson RG, Marmot MG. Social Determinants of Health: The Solid Facts. Geneva: World Health Organization (2003).
34. Planning IFfHa. Singapore’s Successful Long-Term Public Housing Strategies. (2012). Available from: http://www.ifhp.org/ifhp-blog/singapore’s-successful-long-term-public-housing-strategies
35. Galobardes B, Shaw M, Lawlor DA, Lynch JW, Davey Smith G. Indicators of socioeconomic position (part 1). J Epidemiol Community Health (2006) 60(1):7–12. doi:10.1136/jech.2004.023531
36. Wee LE, Yong YZ, Chng MW, Chew SH, Cheng L, Chua QH, et al. Individual and area-level socioeconomic status and their association with depression amongst community-dwelling elderly in Singapore. Aging Ment Health (2014) 18(5):628–41. doi:10.1080/13607863.2013.866632
37. Gordon SB, Bruce NG, Grigg J, Hibberd PL, Kurmi OP, Lam KB, et al. Respiratory risks from household air pollution in low and middle income countries. Lancet Respir Med (2014) 2(10):823–60. doi:10.1016/S2213-2600(14)70168-7
38. Prieto-Centurion V, Gussin HA, Rolle AJ, Krishnan JA. Chronic obstructive pulmonary disease readmissions at minority-serving institutions. Ann Am Thorac Soc (2013) 10(6):680–4. doi:10.1513/AnnalsATS.201307-223OT
39. McAllister DA, Morling JR, Fischbacher CM, MacNee W, Wild SH. Socioeconomic deprivation increases the effect of winter on admissions to hospital with COPD: retrospective analysis of 10 years of national hospitalisation data. Prim Care Respir J (2013) 22(3):296–9. doi:10.4104/pcrj.2013.00066
40. Kangovi S, Barg FK, Carter T, Levy K, Sellman J, Long JA, et al. Challenges faced by patients with low socioeconomic status during the post-hospital transition. J Gen Intern Med (2014) 29(2):283–9. doi:10.1007/s11606-013-2571-5
41. Kangovi S, Levy K, Barg FK, Carter T, Long JA, Grande D. Perspectives of older adults of low socioeconomic status on the post-hospital transition. J Health Care Poor Underserved (2014) 25(2):746–56. doi:10.1353/hpu.2014.0111
Keywords: social determinant of health, readmission, hospital utilization, housing
Citation: Low LL, Wah W, Ng MJ, Tan SY, Liu N and Lee KH (2016) Housing as a Social Determinant of Health in Singapore and Its Association with Readmission Risk and Increased Utilization of Hospital Services. Front. Public Health 4:109. doi: 10.3389/fpubh.2016.00109
Received: 18 March 2016; Accepted: 16 May 2016;
Published: 30 May 2016
Edited by:
Quan Zou, The George Washington University, USAReviewed by:
Timothy Joe Wade, United States Environmental Protection Agency, USAYoussef Oulhote, Harvard School of Public Health, USA
Xian Sun, The George Washington University, USA
Copyright: © 2016 Low, Wah, Ng, Tan, Liu and Lee. This is an open-access article distributed under the terms of the Creative Commons Attribution License (CC BY). The use, distribution or reproduction in other forums is permitted, provided the original author(s) or licensor are credited and that the original publication in this journal is cited, in accordance with accepted academic practice. No use, distribution or reproduction is permitted which does not comply with these terms.
*Correspondence: Lian Leng Low, bG93LmxpYW4ubGVuZ0BzaW5naGVhbHRoLmNvbS5zZw==