- 1Department of Clinical Pharmacy and Outcomes Sciences, University of South Carolina, Columbia, SC, United States
- 2Department of Clinical Pharmacy and Translational Science, University of Tennessee Health Science Center, Memphis, TN, United States
- 3Department of Pharmacy Administration, Fudan University, Shanghai, China
- 4Department of Sociology, Anthropology and Criminal Justice, Clemson University, Clemson, SC, United States
- 5Department of Health Promotion, Education, and Behaviour, Arnold School of Public Health, University of South Carolina, Columbia, SC, United States
Objective: To identify trends in the prevalence of mild cognitive impairment (MCI) and dementia, and to determine risk factors associated with the early detection of dementia among U.S. middle-aged and older adults.
Methods: We used 10-year nationally representative longitudinal data from the Health and Retirement Study (HRS) (2006–2016). Adults aged 55 years or older were included to examine the trend. To identify the associated factors, adults aged 55 years or older in 2006 who developed MCI or dementia in subsequent waves until the 2016 wave were included. Early and late detection of dementia were identified using the Langa-Weir classification of cognitive function. Multivariate logistic regression models were used to identify factors associated with the early detection of dementia.
Results: The sample size for the analysis of the prevalence of MCI and dementia ranged from 14,935 to 16,115 in the six survey years, and 3,729 individuals were identified to determine associated factors of the early detection of dementia. Among them, participants aged 65 years or older accounted for 77.9%, and male participants accounted for 37.2%. The 10-year prevalence of MCI and dementia was 14.5 and 6.6%, respectively. We also found decreasing prevalence trends in MCI (from 14.9 to 13.6%) and dementia (from 7.4 to 6.0%) overall in the past decade. Using logistic regression controlling for the year, non-Hispanic black (MCI: OR = 2.83, P < 0.001; dementia: OR = 2.53, P < 0.001) and Hispanic (MCI: OR = 2.52, P < 0.001; dementia: OR = 2.62, P < 0.001) had a higher prevalence of both MCI and dementia than non-Hispanic white participants. In addition, men had a lower prevalence of MCI (OR = 0.94, P = 0.035) and dementia (OR = 0.84, P < 0.001) compared to women. Associated factors of the early detection of dementia include age, gender, race, educational attainment, stroke, arthritis diseases, heart problems, and pensions.
Conclusion: This study found a decreasing trend in the prevalence of MCI and dementia in the past decade and associated racial/ethnic and gender disparities among U.S. middle-aged and older adults. Healthcare policies and strategies may be needed to address health disparities in the prevalence and take the associated factors of the early detection of dementia into account in clinical settings.
Introduction
Dementia is a broad term used to describe a range of symptoms related to the decline in cognitive functions that impair an individual's daily and independent ability (1–3). Due to the increasing aging population, it is expected that the number of participants with dementia will triple in 2050 compared to 2010 (4). The total direct medical costs of dementia are estimated to exceed $1 trillion in the United States in 2050 (5).
Given that there is still no curative treatment for dementia, reducing risk in high-risk populations is essential to slow the surge in the prevalence of dementia and to reduce economic burdens (6, 7). Mild cognitive impairment (MCI) is a transition state between the cognitive decline of normal aging and dementia, with which individuals appear to have a significantly higher risk of dementia (8). Compared with dementia, the most important distinguishing feature of MCI is that it is reversible and treatable (9, 10). Participants with MCI progress at the rate of 5–10% yearly and about one-third may revert to normal (9). Several elements could have an impact on the reversion (10). Evidence has shown that participants with milder symptoms and without apolipoprotein E (APOE) ε4 allele are more likely to reverse to normal cognition, and healthy lifestyles including exercise and diet can prevent or reverse cognitive decline (10–12). Due to the unavailability of a specific test to confirm, MCI is generally diagnosed by doctors based on symptoms and information provided subjectively (13, 14). As a result, individuals without MCI may sometimes be misdiagnosed and treated incorrectly (10).
Detecting MCI and treating correspondingly could generate economic benefits to the healthcare system and middle-aged and older adults. A recent report from the Alzheimer's Association shows that if all individuals with Alzheimer's disease who were living in the U.S. in 2018 had been detected at the MCI stage, a total of $8 trillion would have been saved (5). However, the detection of MCI is difficult (15). Common screening tools used to detect dementia in clinical practices are not reliable in detecting MCI because the decline in the cognition of individuals with MCI can be subtle (15). Comprehensive assessments for detecting MCI based on brain imaging markers or blood-based neurochemical biomarkers are usually costly, unmodifiable, and lack the sensitivity and specificity required to detect MCI (16–18). Thus, it is critical to determine some less costly and modifiable associated factors of the detection of MCI. In addition to determining associated factors of the detection of MCI, identifying the trends in it is of great importance to implications for the risk reduction and management of dementia (16). However, there have been no reports on trends in the prevalence of MCI and its disparities. To fill the gap in the literature, we aimed to determine associated factors for the detection of MCI, and trends in its prevalence using the Health and Retirement Study (HRS) (19), a large nationally representative prospective cohort study of U.S. middle-aged and older adults.
Methods
Data
This study followed the STROBE guidelines for observational studies. We used 10-year longitudinal data from waves 8 (2006) to 13 (2016) from the original HRS dataset and the RAND HRS v1 longitudinal file 2018. HRS is an ongoing longitudinal population-based survey sponsored by the National Institute on Aging (NIA) and Social Security Administration (SSA), which includes the representative data for adults aged 55 years old in the U.S. for our study period (19). The RAND HRS is a subset of the HRS published by the RAND Corporation with cleaned versions of variables of the HRS (19, 20). The sample of HRS is built over time. Initially, HRS recruited an initial cohort in 1992 with participants born in 1931–1941 (21). Two years later, another cohort born before 1924 was recruited. In 1998, two additional birth cohorts, born in 1924–1930 and 1942–1947, respectively, were recruited to fill the age gap and to create a representative sample of the US population over 50 (21). After that, HRS added a new cohort every 6 years to maintain the sample's representativeness (21). In addition, in 2010, HRS expanded the minority sample, which is referred to as minority oversample (21). The data and materials for HRS can be accessed at its website (http://hrsonline.isr.umich.edu/). Multistage probability sampling design with clustering was used in the HRS to identify household units as the primary sampling unit. Interviews of the HRS respondents, and their spouses if available, were conducted every 2 years (22). The HRS collects information on demographic and socioeconomic factors, health status, and cognition (19, 22). Telephone Interview for Cognitive Status (TICS) in the HRS is specifically used to identify the cognition status of participants (23). In addition, to ensure representativeness at the population level, HRS provides sampling weights for differential selection probabilities for racial/ethnic and birth cohorts and to correct for differential non-response (24). The sampling weights that the HRS provides can also be used to calculate national estimates (24).
Study sample
To have nationally representative samples (25, 26), adults aged 55 years or older in wave 8 (2006) and the refreshment samples of waves 10 (2010) and 13 (2016) were included to examine trends in the prevalence of MCI and dementia. For identifying associated factors of the early detection of dementia, adults aged 55 years or older in wave 8 (2006) were used as the baseline, while the subsequent waves through 2016 were referred to as the follow-up. Participants were included if they had no MCI or dementia at baseline and developed MCI or dementia during the follow-up. Those with missing information on associated factors and non-positive weight information at the baseline were excluded.
Measurement
The early and late detection of dementia were measured using the Langa-Weir Classification of Cognitive Function (LWCCF) based on TICS scores (20, 23). The total TICS score ranges from 0 to 27 points, which comprises an immediate and a delayed 10-noun free recall test (0–20 points), a serial sevens subtraction test (0–5 points), and a counting backward test (0–2 points) (20). The LWCCF is a performance measurement to determine if participants have cognitive impairments, which is derived from the Aging, Demographics and Memory Study (ADAMS), a sub-study of HRS (20). The LWCCF categorized participants who scored 0–6 points as “demented,” 7–11 as “cognitively impaired but not demented (CIND),” and 12–27 as “normal.” Given that in the ADAMS, the CIND is defined the same as MCI (20), participants with normal cognition who developed only MCI without dementia at any wave of the follow-up were classified as having the early detection of dementia, while those who had developed dementia at any wave of the follow-up were classified as having the late detection of dementia.
Potential associated factors measured in this study included demographic and socioeconomic factors, physical health factors, and health behaviors. Demographic factors included: age, gender, race/ethnicity, census regions, and educational attainment. Socioeconomic factors included total household income, public health insurance status, private health insurance status, and pension status. Physical health factors included self-reported health status, body mass index (BMI), and comorbidities (hypertension, cancer, lung diseases, arthritis diseases, diabetes, heart problems, and stroke). Health behaviors included physical activities (vigorous, moderate, and light physical activities), preventative health service use, alcohol assumption, and currently smoking. All of the potential associated factors were identified based on the HRS surveys. Specifically, physical activities were classified on a survey question about the frequency of activities of participants. Participants were identified as having vigorous, moderate, and light physical activities if they had at least 1–3 times of each activity every month. Preventative health service was based on yearly preventive health care services, including flu shots, breast cancer, mammogram, pap smear, and prostate cancer tests. Alcohol users were identified if they drank at least 1 day each week, and smokers were identified if they were currently smoking.
Statistical analyses
The prevalence of MCI was measured as the number of participants with MCI divided by the number of participants who finished TICS in each wave. Logistic regression models controlling for the effect of survey year were used to examine gender and/or racial/ethnic disparities in the prevalence of MCI and dementia. We used two-tailed t-tests for continuous variables and two-tailed Chi-square tests for categorical variables to compare the baseline characteristics between the participants having an early detection of dementia and those having a late detection of dementia. We estimated a multivariate logistic regression model to identify associated factors for the early detection of dementia. All tests were based on an α of 0.05 to assess statistical significance. Data cleaning and analysis were conducted between May 2020 and June 2021, and performed using SAS Software version 9.4 (Statistical Analysis Systems, Cary, NC). Survey sampling weights were used to generate national estimates for both prevalence and regression. Specifically, variables per weight, strata, and psu in the RAND HRS dataset and coding statements “proc surveyfreq” and “proc surveylogistic” in SAS were used to conduct the weighted analysis. Since the HRS is publicly available and all participants were de-identified, this study was reviewed and exempted by the University of South Carolina Institutional Review Board (IRB).
Results
To estimate the national prevalence of MCI in each wave, 15,178 participants in the 8th wave, 14,935 in the 9th wave, 16,115 in the 10th wave, 16,245 in the 11th wave, 15,941 in the 12th wave, and 15,929 in the 13th wave were, respectively, included. For identifying associated factors of the early detection of dementia, 3,729 individuals who had developed MCI or dementia during the follow-up were identified out of 15,795 participants aged 55 years or older with normal cognition at the baseline (Figure 1). Participants aged 65 years or older accounted for 77.9%, and the male participants accounted for 37.2%. Among the participants included, a total of 3,072 (82.4%) were classified as having an early detection of dementia and 657 (17.6%) as having a late detection of dementia.
Figures 2A, 3A present the overall estimated trend in the prevalence of MCI and dementia from 2006 to 2016. The 10-year prevalence of MCI and dementia was 14.5% and 6.6%, respectively. The prevalence of MCI ranged from 13.6 to 15.1%, and it was lower in 2016 (13.6%) than in 2006 (14.9%). The prevalence of dementia was decreasing over the decade, specifically, from 7.4% in 2006 to 6.0% in 2016. Figures 2B, 3B demonstrate trends in the prevalence of MCI and dementia by race/ethnicity. Using logistic regression after controlling for the year, non-Hispanic black (MCI: OR = 2.83, P < 0.001; dementia: OR = 2.53, P < 0.001), Hispanic (MCI: OR = 2.52, P < 0.001; dementia: OR = 2.62, P < 0.001), and other racial participants (MCI: OR = 1.63, P = 0.244; dementia: OR = 1.77, P = 0.677) had a higher prevalence of MCI and dementia than non-Hispanic white participants. Figures 2C, 3C show trends in the prevalence of MCI and dementia by gender. Although there was no difference in MCI, men had a lower prevalence of MCI (OR = 0.94, P = 0.035) and dementia compared to women (OR = 0.84, P < 0.001).
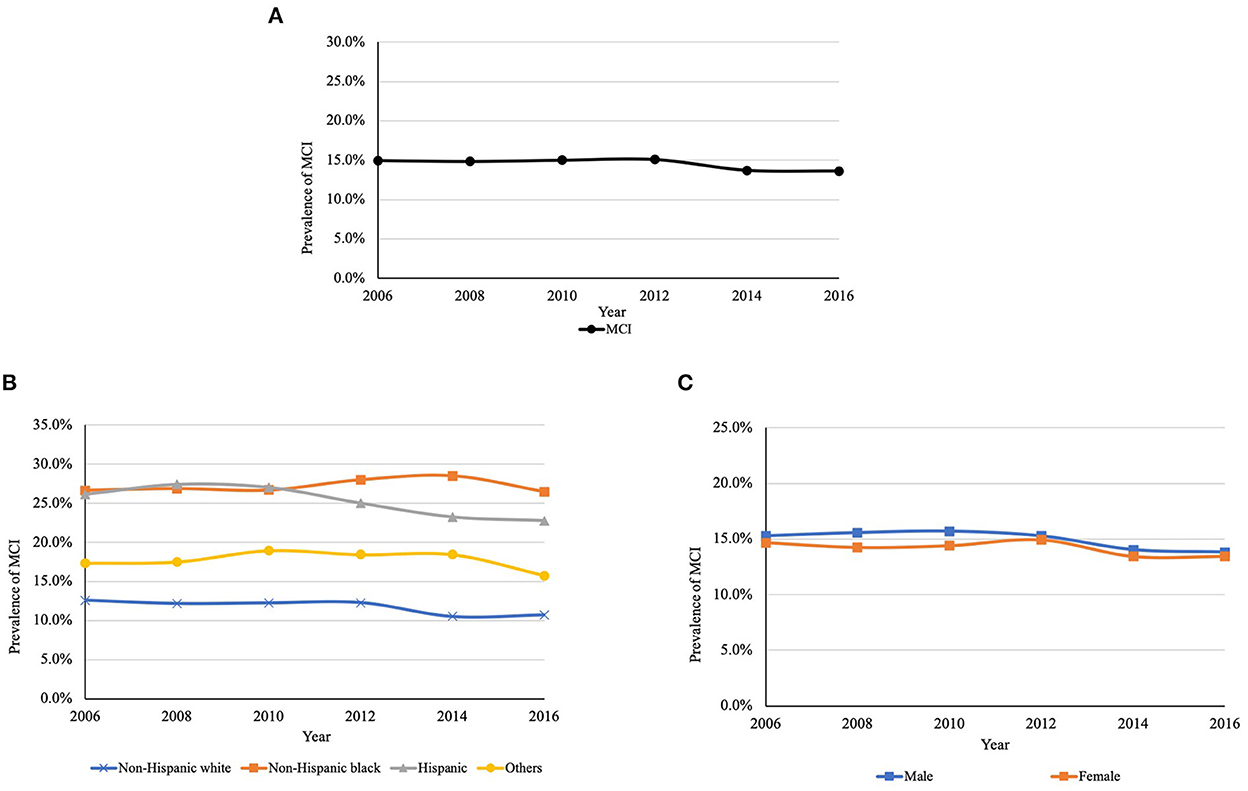
Figure 2. (A) Trends in the prevalence of MCI between 2006 and 2016. (B) Trends in the prevalence of MCI between 2006 and 2016 by race/ethnicitya. (C) Trends in the prevalence of MCI between 2006 and 2016 by genderb. MCI, mild cognitive impairment. aP < 0.001; bP = 0.448. P-values are for the type 3 test for the difference in the prevalence among race and gender.
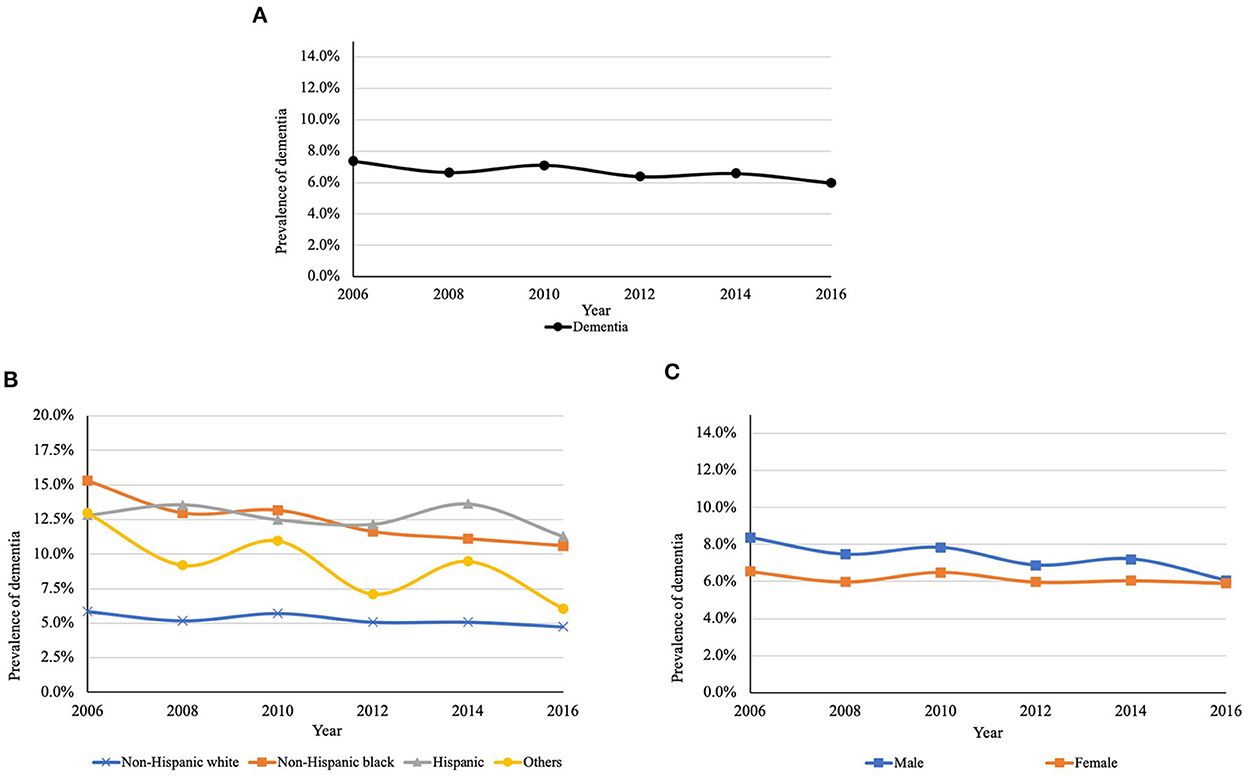
Figure 3. (A) Trends in the prevalence of dementia between 2006 and 2016. (B) Trends in the prevalence of dementia between 2006 and 2016 by race/ethnicitya. (C) Trends in the prevalence of dementia between 2006 and 2016 by genderb. aP < 0.001; bP < 0.001. P-values are for the type 3 test for the difference in the prevalence among race and gender.
The baseline characteristics of participants with detection of MCI or dementia during the follow-up are shown in Table 1. Compared to participants with late detection of dementia, those with early detection were more likely to be younger (p < 0.001), male (p < 0.001), Non-Hispanic white (p = 0.020), married/partnered (p = 0.015), with higher education (p < 0.001), with higher income (p < 0.001), without strokes (p = 0.002), alcohol consumers (p = 0.002), without public insurance (p < 0.001), and with private insurance (p = 0.027).
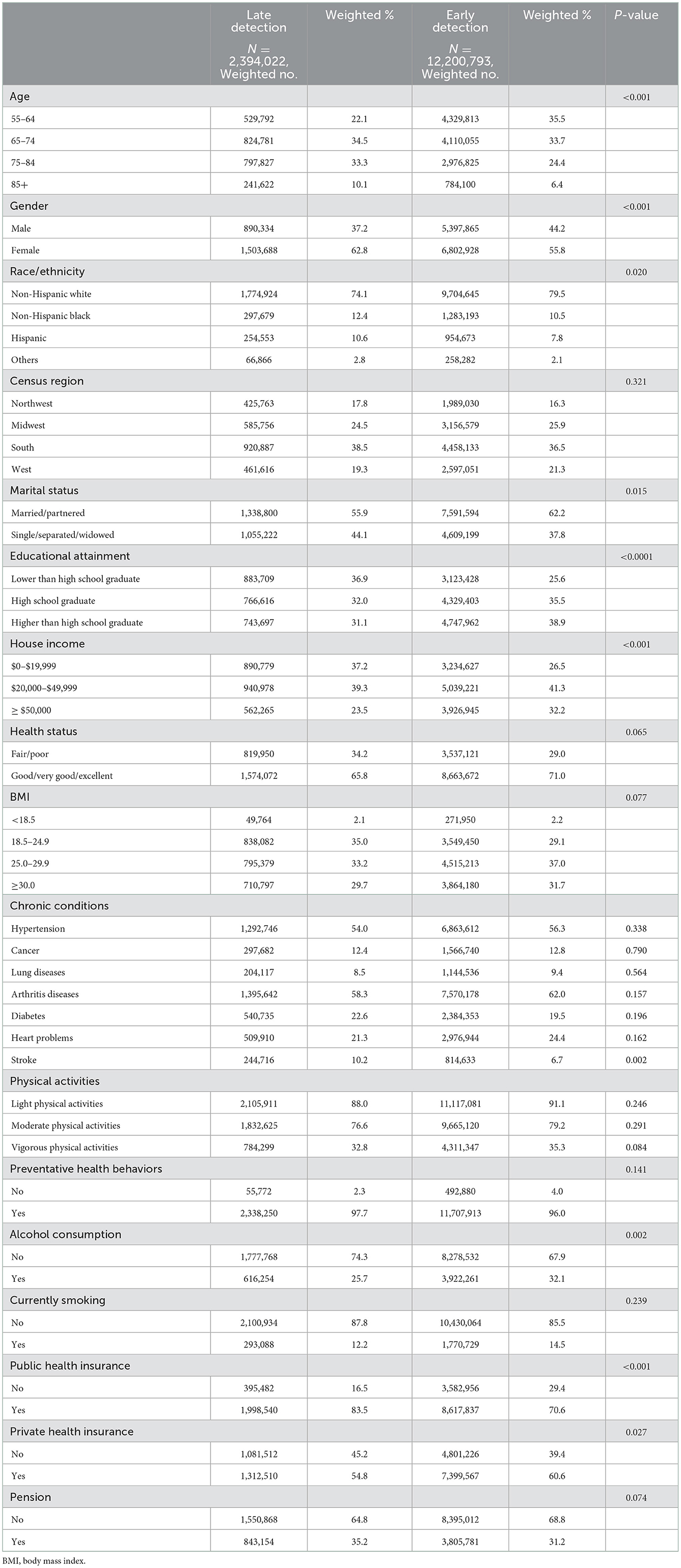
Table 1. Descriptive characteristics of baseline study sample between late and early detection of dementia.
Associated factors for early vs. late detection of dementia are shown in Table 2. Compared with individuals aged 55–64 years, those aged between 75 and 84 years [Odds ratio (OR) = 0.55, 95% CI Confidence interval (CI), 0.31–0.98], and 85 and older (OR = 0.49, 95% CI, 0.26–0.92) were less likely to be associated with the early detection of dementia rather than the late detection. Women were less likely to have an early detection compared to men (OR = 0.79, 95% CI, 0.65–0.96). Compared to non-Hispanic white participants, non-Hispanic participants were less likely to have an early detection of dementia other than a late detection (OR = 0.71, 95% CI, 0.54–0.94). Compared to participants with less than a high school education, those with a high school education (OR = 1.58, 95% CI, 1.22–2.05) or higher (OR = 1.74, 95% CI, 1.35–2.24) were more likely to have an early detection of dementia other than a late detection. In addition, individuals who had arthritis diseases (OR = 1.34, 95% CI, 1.06–1.70), heart problems (OR = 1.37, 95% CI, 1.05–1.78) were more likely, while those with stroke (OR = 0.69, 95% CI, 0.48–0.99) were less likely to have an early detection of dementia. Also, compared to individuals without pensions, those who receive pensions were less likely to have an early detection of dementia (OR = 0.80, 95% CI, 0.65–0.98).
Discussion
We examined a cluster of associated factors of the early detection of dementia and reported updated trends in the prevalence of MCI and dementia using a nationally representative longitudinal dataset from 2006 to 2016. The prevalence of MCI and dementia declined from 2006 to 2016 in the U.S. which is similar to previous years or other regions (27–32). According to a review of global trends in the prevalence and incidence of dementia, although studies on trends in the prevalence are not clear, the incidence of dementia may be declining in high-income countries (27). The decline in prevalence in the U.S. between 2006 and 2016 might be the long-term result of the decline in the incidence (33). Similar to our results, a study using HRS based on previous years in the U.S. found that the prevalence of dementia declined from 1993 to 2002, and declined in 2021 compared with 2000 in the U.S. (28, 32).
Our results are also consistent with other studies conducted during a similar period of study time (29–31). Using the 2011–2015 National Health and Aging Trends Study, Freedman et al. found that there was a decline in the prevalence of probable dementia over this period, from 10.6% in 2011 to 9.9% in 2015 (29). Similarly, a study of the population in aged care services in Australia found that the age- and sex-standardized prevalence of dementia declined from 2005 to 2014 (30). In addition, Taudorf et al., using national registry-based data which included over 2 million people in Demark, showed a reduction in the incidence of dementia from 2004 to 2015 (31).
We also found that non-Hispanic black and Hispanic participants have a higher prevalence of MCI and dementia than non-Hispanic white participants. These results indicate that potential health inequities might exist among different racial and ethnic groups (34–37). Similarly, a recent study using three different algorithms for identifying dementia found that black and Hispanic participants in HRS had a higher prevalence of dementia compared to black participants (38). Policies and strategies for cognitive impairment care should be developed to ensure high-risk groups have access to healthcare resources for MCI and dementia and to reduce potential inequities (39, 40). In addition, we found that there were gender disparities in the prevalence of MCI and dementia. These findings are consistent with previous studies in different groups of individuals. Sachdev et al. found that there is no significant gender difference in the prevalence of MCI among adults aged 60 years or older, and a systematic review found that the prevalence was greater in women than in men (41, 42). This might be because MCI occurs early, while dementia comes later in age, and because women have a longer life span than men, they thus have a higher prevalence of dementia (43–45). A study by Petersen et al. also found that among adults aged between 70 and 89 years, the prevalence of MCI was higher in males than in females among older adults (46).
In terms of associated factors, variables significantly associated with the early detection of dementia compared with the late detection included younger age, male gender, non-Hispanic whites, higher educational attainment, no history of stroke, and not yet receiving pensions. The prevalence and the risk of dementia increase with aging, leading to a decrease in the probability of detecting dementia at an early stage (47, 48). Therefore, screening in the younger population for cognitive impairment is critical to avoid detecting dementia at a late stage when it cannot be reversed. Consistent with the disparities found in the trends of MCI and dementia, there were racial/ethnic and gender disparities in detecting dementia at different stages, which might be related to possible physiologically-based differences in the development of dementia (49). We also found that adults with higher educational attainment were more likely to have the early detection of dementia instead of the late detection. Tailored educational sessions might be needed for the less educated middle-aged and older adults to facilitate the early detection of cognitive impairment. The results of our study also demonstrated that several chronic diseases, including arthritis diseases and heart problems, were associated factors of the early detection of dementia other than the late detection of dementia among U.S. middle-aged and older adults. Compared with those without these chronic conditions, adults with these chronic conditions are more likely to use more healthcare services and therefore making it more likely to detect dementia at an early stage (50, 51). Therefore, for middle-aged and older adults who do not frequently use health care services, policies or measures might be tailored to encourage them to undergo regular screening or annual wellness visits for early detection of cognitive impairment. Given that routine screening for older adults has not yet been fully implemented in the U.S., our results may support the significance of this initiative. In addition to arthritis and heart problems, we found that stroke was associated with a lower possibility of the early detection of dementia, which might be related to reduced consciousness and thinking ability among participants with stroke (52). Therefore, clinical care for participants with stroke should be intensified to prevent them from the onset of dementia or reduce their potential loss caused by the onset of dementia. We also found that middle-aged and older adults with pensions were less likely to have the early detection of dementia. This is consistent with previous research showing that paid work as a productive activity reduces the risk of cognitive decline although it is also possible that this relationship is due to reverse causation that one may retire later due to stellar cognitive health or earlier because of the occurrence of MCI or dementia (53).
Our study has several important strengths. First, by using a nationally representative survey, we estimated the national prevalence of MCI in 10 years and found racial/ethnic disparities. Second, we used a longitudinal design to identify associated factors of the early detection compared with late detection of dementia, which could imply a possible causal relationship between associated factors and the early detection.
However, there are still several limitations in this study. First, although we excluded participants with MCI or participants with dementia at the baseline, we could not exclude participants who had MCI before 2006 but converted back to normal cognition in 2006, which might cause a latency bias to a certain degree. In addition, because participants with missing information on predictors and weight were excluded in the data cleaning process, there might be non-differential classification bias, which might lead the effects being underestimated. However, since only about 100 observations were excluded, it would not substantially affect the results. Second, we only included information about the living region. Due to the lack of related data, we did not include information on whether participants lived in rural or urban areas, which might be a potentially associated factor for the detection of MCI (25). Third, as we used survey data as the data source, which did not contain specific diagnostic information, we classified cognitive status based on the LWCCF and derived the total cognitive scores from telephone interviews instead of clinical diagnosis. The mapping methods that LWCCF used to categorize cognitive status and telephone interviews might lead to some biases, such as differential misclassification and response bias (20). However, as the Langa-Weir classification has been validated with HRS participants and our results are consistent with previously published studies using other data sources, such as registries (31, 54), we believe that the Langa-Weir classification was valid in determining dementia and MCI in our study. Yet, studies using specific diagnostic information are still needed to provide a more rigorous national estimate of the prevalence of dementia in the United States. Furthermore, the influence of other life events (lack of sleep, medications, drugs, etc.) on cognitive performance at the time of TIC administration could not be assessed because of a lack of data. Finally, since we used a survey-based dataset that did not include the specific diseases of each participant, we were not able to use a comprehensive comorbidity indicator to control for comorbidities. However, we have included the main comorbidities based on previously published literature, which could minimize the impact of comorbidities on other associated factors (54–57).
Conclusion
Using a nationally representative sample of U.S. middle-aged and older adults, we found racial/ethnic disparities in the prevalence of MCI and dementia, gender disparities in the prevalence of dementia, and associated factors of an early detection compared with a late detection of dementia. Healthcare policies and strategies may be needed to address health disparities in the prevalence of MCI and dementia, as well as to take the associated factors of early detection of dementia into account in the clinical settings to reduce possible disease and economic burden due to late detection of dementia.
Data availability statement
Publicly available datasets were analyzed in this study. The data can be found and accessed at: https://hrs.isr.umich.edu/about.
Ethics statement
The studies involving human participants were reviewed and approved by University of South Carolina Institutional Review Board (IRB). Written informed consent for participation was not required for this study in accordance with the national legislation and the institutional requirements.
Author contributions
KL had full access to all of the data in the study, takes responsibility for the integrity of the data, the accuracy of the data analysis, and contributed to concept and design. KL, XX, ML, JY, YL, and DF contributed to acquisition, analysis, or interpretation of data, drafting of the manuscript, and critical revision of the manuscript for important intellectual content. KL and XX contributed to statistical analysis. All authors contributed to the article and approved the submitted version.
Funding
This work was supported by the National Institutes of Health, National Institute on Aging (Grant Number: P30AGO59294). The funders had no role in the design and conduct of the study; collection, management, analysis, and interpretation of the data; preparation, review, or approval of the manuscript; and decision to submit the manuscript for publication.
Conflict of interest
The authors declare that the research was conducted in the absence of any commercial or financial relationships that could be construed as a potential conflict of interest.
Publisher's note
All claims expressed in this article are solely those of the authors and do not necessarily represent those of their affiliated organizations, or those of the publisher, the editors and the reviewers. Any product that may be evaluated in this article, or claim that may be made by its manufacturer, is not guaranteed or endorsed by the publisher.
References
1. Iqbal K, Liu F, Gong CX, Grundke-Iqbal I. Tau in Alzheimer disease and related tauopathies. Curr Alzheimer Res. (2010) 7:656–64. doi: 10.2174/156720510793611592
2. National Institute on Aging. Basics of Alzheimer's Disease and Dementia. What is Alzheimer's Disease? (2021). Available online at: https://www.nia.nih.gov/health/what-alzheimers-disease (accessed November 25, 2019).
3. U.S. Department of Health & Human Services. What is Alzheimer's Disease and Related Dementias. Available online at: https://aspe.hhs.gov/what-alzheimers-disease-and-related-dementias (accessed November 25, 2019).
4. Barnes DE, Yaffe K. Predicting dementia: role of dementia risk indices. Future Neurol. (2009) 4:555–60. doi: 10.2217/fnl.09.43
5. Alzheimer's Association. 2019 Alzheimer's disease facts and figures. Alzheimers Dement. (2019). 15:321–87. doi: 10.1016/j.jalz.2019.01.010
6. Zekry D, Hauw JJ, Gold G. Mixed dementia: epidemiology, diagnosis, and treatment. J Am Geriatr Soc. (2002) 50:1431–8. doi: 10.1046/j.1532-5415.2002.50367.x
7. Mangialasche F, Kivipelto M, Solomon A, Fratiglioni L. Dementia prevention: current epidemiological evidence and future perspective. Alzheimers Res Ther. (2012) 4:6. doi: 10.1186/alzrt104
8. DeCarli C. Mild cognitive impairment: prevalence, prognosis, aetiology, and treatment. Lancet Neurol. (2003) 2:15–21. doi: 10.1016/S1474-4422(03)00262-X
9. Morley JE. Mild cognitive impairment-a treatable condition. J Am Med Dir Assoc. (2014) 15:1–5. doi: 10.1016/j.jamda.2013.11.001
10. Malek-Ahmadi M. Reversion from mild cognitive impairment to normal cognition: a meta-analysis. Alzheimer Dis Assoc Disord. (2016) 30:324–30. doi: 10.1097/WAD.0000000000000145
11. Blumenthal JA, Smith PJ, Mabe S, Hinderliter A, Lin PH, Liao L, et al. Lifestyle and neurocognition in older adults with cognitive impairments: a randomized trial. Neurology. (2019) 92:e212–23. doi: 10.1212/WNL.0000000000006784
12. Koepsell TD, Monsell SE. Reversion from mild cognitive impairment to normal or near-normal cognition: risk factors and prognosis. Neurology. (2012) 79:1591–8. doi: 10.1212/WNL.0b013e31826e26b7
13. Langa KM, Levine DA. The diagnosis and management of mild cognitive impairment: a clinical review. JAMA. (2014) 312:2551–61. doi: 10.1001/jama.2014.13806
14. Gauthier S, Reisberg B, Zaudig M, Petersen RC, Ritchie K, Broich K, et al. Mild cognitive impairment. Lancet. (2006) 367:1262–70. doi: 10.1016/S0140-6736(06)68542-5
15. Lunsford R, Heeman PA. Using linguistic indicators of difficulty to identify mild cognitive impairment. In: Sixteenth Annual Conference of the International Speech Communication Association. Dresden (2015). doi: 10.21437/Interspeech.2015-235
16. Frisoni GB, Boccardi M, Barkhof F, Blennow K, Cappa S, Chiotis K, et al. Strategic roadmap for an early diagnosis of Alzheimer's disease based on biomarkers. Lancet Neurol. (2017) 16:661–76. doi: 10.1016/S1474-4422(17)30159-X
17. Teipel S, Kilimann I, Thyrian JR, Kloppel S, Hoffmann W. Potential role of neuroimaging markers for early diagnosis of dementia in primary care. Curr Alzheimer Res. (2018) 15:18–27. doi: 10.2174/1567205014666170908093846
18. Lewczuk P, Kornhuber J, Vanmechelen E, Peters O, Heuser I, Maier W, et al. Amyloid beta peptides in plasma in early diagnosis of Alzheimer's disease: a multicenter study with multiplexing. Exp Neurol. (2010) 223:366–70. doi: 10.1016/j.expneurol.2009.07.024
19. Juster FT, Suzman R. An overview of the health and retirement study. J Hum Resour. (1995) 30:S7–56. doi: 10.2307/146277
20. Langa KM, Plassman BL, Wallace RB, Herzog AR, Heeringa SG, Ofstedal MB, et al. The aging, demographics, and memory study: study design and methods. Neuroepidemiology. (2005) 25:181–91. doi: 10.1159/000087448
21. Sonnega A, Faul JD, Ofstedal MB, Langa KM, Phillips JW, Weir DR. Cohort profile: the health and retirement study (HRS). Int J Epidemiol. (2014) 43:576–85. doi: 10.1093/ije/dyu067
22. Cheshire H, Ofstedal MB, Scholes S, Schroeder M. A comparison of response rates in the English longitudinal study of ageing and the health and retirement study. Longit Life Course Stud. (2011) 2:127–44. doi: 10.14301/llcs.v2i2.118
23. Choi M, Lohman MC, Mezuk B. Trajectories of cognitive decline by driving mobility: evidence from the health and retirement study. Int J Geriatr Psychiatry. (2014) 29:447–53. doi: 10.1002/gps.4024
24. Health and Retirement Study (HRS). Sampling Weights. (2016). Available online at: https://hrs.isr.umich.edu/sites/default/files/biblio/HRS2016SamplingWeights.pdf (accessed November 8, 2022).
25. Weden MM, Shih RA, Kabeto MU, Langa KM. Secular trends in dementia and cognitive impairment of U.S. rural and urban older adults. Am J Prev Med. (2018) 54:164–72. doi: 10.1016/j.amepre.2017.10.021
26. Richardson RA, Keyes KM, Medina JT, Calvo E. Sociodemographic inequalities in depression among older adults: cross-sectional evidence from 18 countries. Lancet Psychiatry. (2020) 7:673–81. doi: 10.1016/S2215-0366(20)30151-6
27. Roehr S, Pabst A, Luck T, Riedel-Heller SG. Is dementia incidence declining in high-income countries? A systematic review and meta-analysis. Clin Epidemiol. (2018) 10:1233–47. doi: 10.2147/CLEP.S163649
28. Langa KM, Larson EB, Crimmins EM, Faul JD, Levine DA, Kabeto MU, et al. A comparison of the prevalence of dementia in the United States in 2000 and 2012. JAMA Intern Med. (2017) 177:51–8. doi: 10.1001/jamainternmed.2016.6807
29. Freedman VA, Kasper JD, Cornman JC, Agree EM, Bandeen-Roche K, Mor V, et al. Validation of new measures of disability and functioning in the National Health and Aging Trends Study. J Gerontol A Biol Sci Med Sci. (2011) 66:1013–21. doi: 10.1093/gerona/glr087
30. Harrison SL, Lang C, Whitehead C, Crotty M, Ratcliffe J, Wesselingh S, et al. Trends in prevalence of dementia for people accessing aged care services in Australia. J Gerontol Ser A. (2020) 75:318–25. doi: 10.1093/gerona/glz032
31. Taudorf L, Nørgaard A, Islamoska S, Jørgensen K, Laursen TM, Waldemar G. Declining incidence of dementia: a national registry-based study over 20 years. Alzheimers Dement. (2019) 15:1383–91. doi: 10.1016/j.jalz.2019.07.006
32. Langa KM, Larson EB, Karlawish JH, Cutler DM, Kabeto MU, Kim SY, et al. Trends in the prevalence and mortality of cognitive impairment in the United States: is there evidence of a compression of cognitive morbidity? Alzheimers Dement. (2008) 4:134–44. doi: 10.1016/j.jalz.2008.01.001
33. Luck T, Luppa M, Briel S, Riedel-Heller SG. Incidence of mild cognitive impairment: a systematic review. Dement Geriatr Cogn Disord. (2010) 29:164–75. doi: 10.1159/000272424
34. Katz MJ, Lipton RB, Hall CB, Zimmerman ME, Sanders AE, Verghese J, et al. Age-specific and sex-specific prevalence and incidence of mild cognitive impairment, dementia, and Alzheimer dementia in blacks and whites: a report from the Einstein Aging Study. Alzheimer Dis Assoc Disord. (2012) 26:335–43. doi: 10.1097/WAD.0b013e31823dbcfc
35. O'Bryant SE, Johnson L, Reisch J, Edwards M, Hall J, Barber R, et al. Risk factors for mild cognitive impairment among Mexican Americans. Alzheimers Dement. (2013) 9:622–31. doi: 10.1016/j.jalz.2012.12.007
36. Zuckerman IH, Ryder PT, Simoni-Wastila L, Shaffer T, Sato M, Zhao L, et al. Racial and ethnic disparities in the treatment of dementia among Medicare beneficiaries. J Gerontol B Psychol Sci Soc Sci. (2008) 63:S328–S33. doi: 10.1093/geronb/63.5.S328
37. Cogburn CD. Culture, race, and health: Implications for racial inequities and population health. Milbank Q. (2019) 97:736–61. doi: 10.1111/1468-0009.12411
38. Power MC, Bennett EE, Turner RW, Dowling NM, Ciarleglio A, Glymour MM, et al. Trends in relative incidence and prevalence of dementia across non-Hispanic Black and White individuals in the United States, 2000-2016. JAMA Neurol. (2021) 78:275–84. doi: 10.1001/jamaneurol.2020.4471
39. Raphael D. Health inequities in the United States: prospects and solutions. J Public Health Policy. (2000) 21:394–427. doi: 10.2307/3343281
40. Assari S. Health disparities due to diminished return among black Americans: Public policy solutions. Soc Issues Policy Rev. (2018) 12:112–45. doi: 10.1111/sipr.12042
41. Sachdev PS, Lipnicki DM, Kochan NA, Crawford JD, Thalamuthu A, Andrews G, et al. The prevalence of mild cognitive impairment in diverse geographical and ethnocultural regions: the COSMIC collaboration. PLoS One. (2015) 10:e0142388. doi: 10.1371/journal.pone.0142388
42. Cao Q, Tan CC, Xu W, Hu H, Cao X-P, Dong Q, et al. The prevalence of dementia: a systematic review and meta-analysis. J Alzheimers Dis. (2020) 73:1157–66. doi: 10.3233/JAD-191092
43. Viña J, Borrás C, Gambini J, Sastre J, Pallardó FV. Why females live longer than males? Importance of the upregulation of longevity-associated genes by oestrogenic compounds. FEBS Lett. (2005) 579:2541–5. doi: 10.1016/j.febslet.2005.03.090
44. Mathuranath PS, Cherian PJ, Mathew R, Kumar S, George A, Alexander A, et al. Dementia in Kerala, South India: prevalence and influence of age, education and gender. Int. J Geriatr Psychiatry. (2010) 25:290–7. doi: 10.1002/gps.2338
45. Kim KW, Park JH, Kim MH, Kim MD, Kim BJ, Kim SK, et al. A nationwide survey on the prevalence of dementia and mild cognitive impairment in South Korea. J Alzheimers Dis. (2011) 23:281–91. doi: 10.3233/JAD-2010-101221
46. Petersen RC, Roberts RO, Knopman DS, Geda YE, Cha RH, Pankratz VS, et al. Prevalence of mild cognitive impairment is higher in men. The Mayo Clinic Study of Aging. Neurology. (2010) 75:889–97. doi: 10.1212/WNL.0b013e3181f11d85
47. Savva GM, Wharton SB, Ince PG, Forster G, Matthews FE, Brayne C, et al. Age, neuropathology, and dementia. N Engl J Med. (2009) 360:2302–9. doi: 10.1056/NEJMoa0806142
48. Burton CL, Strauss E, Bunce D, Hunter MA, Hultsch DF. Functional abilities in older adults with mild cognitive impairment. Gerontology. (2009) 55:570–81. doi: 10.1159/000228918
49. Mielke MM. Sex and gender differences in Alzheimer's disease dementia. Psychiatr Times. (2018) 35:14–7.
50. Ploeg J, Northwood M, Duggleby W, McAiney CA, Chambers T, Peacock S, et al. Caregivers of older adults with dementia and multiple chronic conditions: exploring their experiences with significant changes. Dementia. (2020) 19:2601–20. doi: 10.1177/1471301219834423
51. Sanders S, Power J. Roles, responsibilities, and relationships among older husbands caring for wives with progressive dementia and other chronic conditions. Health Soc Work. (2009) 34:41–51. doi: 10.1093/hsw/34.1.41
52. Pendlebury ST, Rothwell PM. Prevalence, incidence, and factors associated with pre-stroke and post-stroke dementia: a systematic review and meta-analysis. Lancet Neurol. (2009) 8:1006–18. doi: 10.1016/S1474-4422(09)70236-4
53. Dufouil C, Pereira E, Chêne G, Glymour MM, Alpérovitch A, Saubusse E, et al. Older age at retirement is associated with decreased risk of dementia. Eur J Epidemiol. (2014) 29:353–61. doi: 10.1007/s10654-014-9906-3
54. Crimmins EM, Kim JK, Langa KM, Weir DR. Assessment of cognition using surveys and neuropsychological assessment: the Health and Retirement Study and the Aging, Demographics, and Memory Study. J Gerontol B Psychol Sci Soc Sci. (2011) 66(Suppl 1):i162–71. doi: 10.1093/geronb/gbr048
55. Hreha KP, Downer B, Ehrlich JR, Howrey B, Taglialatela G. Association between vision impairment and cognitive decline in older adults with stroke: Health and Retirement Study. Aging Clin Exp Res. (2021) 33:2605–10. doi: 10.1007/s40520-020-01776-w
56. Iwashyna TJ, Ely EW, Smith DM, Langa KM. Long-term cognitive impairment and functional disability among survivors of severe sepsis. JAMA. (2010) 304:1787–94. doi: 10.1001/jama.2010.1553
57. Andrews JS, Desai U, Kirson NY, Enloe CJ, Ristovska L, King S, et al. Functional limitations and health care resource utilization for individuals with cognitive impairment without dementia: findings from a United States population-based survey. Alzheimers Dement. (2016) 6:65–74. doi: 10.1016/j.dadm.2016.11.005
Keywords: mild cognitive impairment, dementia, Alzheimer's disease, early detection, middle-aged and older populations
Citation: Lu K, Xiong X, Li M, Yuan J, Luo Y and Friedman DB (2023) Trends in prevalence, health disparities, and early detection of dementia: A 10-year nationally representative serial cross-sectional and cohort study. Front. Public Health 10:1021010. doi: 10.3389/fpubh.2022.1021010
Received: 16 August 2022; Accepted: 06 December 2022;
Published: 04 January 2023.
Edited by:
Konstantin G. Arbeev, Duke University, United StatesReviewed by:
Mack Shelley, Iowa State University, United StatesMirjana Ratko Jovanovic, University of Kragujevac, Serbia
Copyright © 2023 Lu, Xiong, Li, Yuan, Luo and Friedman. This is an open-access article distributed under the terms of the Creative Commons Attribution License (CC BY). The use, distribution or reproduction in other forums is permitted, provided the original author(s) and the copyright owner(s) are credited and that the original publication in this journal is cited, in accordance with accepted academic practice. No use, distribution or reproduction is permitted which does not comply with these terms.
*Correspondence: Kevin Lu, bHUzMkBlbWFpbC5zYy5lZHU=