- 1Cumming School of Medicine, University of Calgary, Calgary, AB, Canada
- 2Faculty of Kinesiology, University of Calgary, Calgary, AB, Canada
- 3School of Architecture, Planning and Landscape, University of Calgary, Calgary, AB, Canada
- 4School of Knowledge Science, Japan Advanced Institute of Science and Technology, Nomi, Japan
- 5Faculty of Sport Sciences, Waseda University, Tokorozawa, Japan
- 6Cancer Care Alberta, Alberta Health Services, Calgary, AB, Canada
- 7Graduate School of Environmental Studies, Tohoku University, Sendai, Japan
- 8Public Space and Mobility Policy, Planning and Development Services Department, Calgary, AB, Canada
- 9Toole Design Group, Calgary, AB, Canada
- 10Mental Health Promotion and Illness Prevention, Alberta Health Services, Calgary, AB, Canada
Introduction: Cross-sectional studies consistently find that the neighborhood built environment (e.g., walkability) is associated with walking. However, findings from the few existing longitudinal residential relocation studies that have estimated associations between changes in neighborhood built characteristics and walking are equivocal. The study objective was to estimate whether changes in neighborhood walkability resulting from residential relocation were associated with leisure, transportation, and total walking levels among adults.
Methods: This study included longitudinal data from the “Alberta's Tomorrow Project”—a province-wide cohort study (Alberta, Canada). The analysis included data collected at two time points (i.e., baseline and follow-up) from 5,977 urban adults. The International Physical Activity Questionnaire (IPAQ) captured self-reported walking. We estimated neighborhood walkability, an index capturing intersection, destination, and population counts for the 400 m Euclidean buffer around participants' homes. Using household postal codes reported at baseline and follow-up, we categorized participants into three groups reflecting residential relocation (“non-movers:” n = 5,679; “movers to less walkability:” n = 164, and; “movers to more walkability:” n = 134). We used Inverse-Probability-Weighted Regression Adjustment to estimate differences [i.e., average treatment effects in the treated (ATET)] in weekly minutes of leisure, transportation, and total walking at follow-up between residential relocation groups, adjusting for baseline walking, sociodemographic characteristics, and walkability. The median time between baseline and follow-up was 2-years.
Results: The three residential relocation groups mainly included women (61.6–67.2%) and had a mean age of between 52.2 and 55.7 years. Compared to “non-movers” (reference group), weekly minutes of transportation walking at follow-up was significantly lower among adults who moved to less walkable neighborhoods (ATET: −41.34, 95 CI: −68.30, −14.39; p < 0.01). We found no other statistically significant differences in walking between the groups.
Discussion: Our findings suggest that relocating to less walkable neighborhoods could have detrimental effects on transportation walking to the extent of adversely affecting health. Public health strategies that counteract the negative impacts of low walkable neighborhoods and leverage the supportiveness of high walkable neighborhoods might promote more walking.
Introduction
Encouraging adults to participate in regular physical activity is important as it can protect against numerous modifiable chronic health conditions (e.g., cardiovascular disease, type II diabetes, hypertension, metabolic syndrome, overweight and obesity, cancer, and depression) (1, 2) and premature mortality (3, 4). There is a dose-response relationship between physical activity and health, meaning that even small amounts of moderate-intensity physical activity daily (e.g., walking) can provide health benefits (5). From a public health perspective, walking is a prime intervention target for encouraging the accumulation of physical activity because walking is a safe and innate behavior that most adults can efficiently perform as part of their everyday activities within various physical settings (6). Furthermore, walking is one of the most commonly undertaken physical activities (7, 8) and an essential contributor to the accumulation of total physical activity (9, 10). However, in Canada, less than one-third of adults walk regularly (≥4 times per week) (10), and on average, they accumulate < 5,000 steps per day (11). There is an urgent need to identify effective population-level interventions that encourage more walking among Canadian adults.
Neighborhoods are popular settings where adults walk (12–15). The creation of walkable neighborhood built environments is an intervention strategy that can increase walking and increase physical activity (16–18), social connectedness (19), and improve health (20, 21). Walkable neighborhoods include several built attributes such as connected street layouts, pedestrian infrastructure, amenities, and safety, making walking an easy and convenient option (22). Cross-sectional evidence demonstrates that neighborhoods with higher walkability are associated with more walking (23, 24). However, cross-sectional studies provide no evidence with regard to temporality, limiting their ability to assess causality. It remains unclear whether associations between neighborhood walkability and walking result from neighborhood self-selection (seeking out walkable neighborhoods to fulfill walking preferences) or if exposure to a walkable neighborhood changes walking behavior. Recognizing this limitation, longitudinal studies (retrospective and prospective residential relocation studies and natural experiments) estimating the associations between changes in built environment exposure and physical activity have recently emerged (17, 18, 25, 26). Residential relocation studies, in particular, offer an opportunity to estimate the temporal relations between the built environment and physical activity and can account for residual confounding because participants serve as their controls (26). Moreover, residential relocation interrupts normal or habitual behavioral patterns, of which some of this behavior change might be due to exposure to a different built environment (26, 27).
Findings from residential relocation studies provide only modest and often mixed evidence for an association between the built environment and physical activity (26). Most residential relocation studies to date have been undertaken in the US, Australia, and in European countries, with few studies conducted in Canada (26). The geographical differences in physical activity and the built environment strengthens the need for more Canadian specific studies investigating associations between neighborhood walkability and walking (18). Moreover, prospective residential relocation studies undertaken to date have mostly focussed on associations and found consistent results in relation to the associations between land uses, destinations, and transportation and walking (26, 28) with fewer studies examining changes in exposure to overall neighborhood walkability and walking (26). In a residential relocation study in Canada (average follow-up period of 10 months), Adhikari et al. (29) found that an increase in walkability (residential density, commercial floor area ratio, land use mix, and intersection density combined) was associated with an increase in non-work transport-related walking trips adjusting for changes in neighborhood and travel preferences and life events. In another prospective residential relocation study (12 year follow-up) undertaken in Canada, Wasfi et al. (30) found that relocation to a more walkable neighborhood (measured using Walk Score®) was associated with an increase in the likelihood of participating in transportation walking. In a residential relocation study in the UK (2 year follow-up), Clary et al. (31) found that one standard deviation increase in walkability (i.e., connectivity, land use mix, and residential density combined) was associated with an increase of ~300 steps and 1.7 min of MVPA per day. In contrast, in a prospective residential relocation study in the US (6 year follow-up), Braun et al. (32) found no significant associations between changes in walkability (population density, connectivity, and food and physical activity resources combined) and participation and frequency of overall walking among movers. A residential relocation study (“RESIDE”) undertaken in Western Australia, which spanned 10-years found adults exposed to neighborhoods that incorporated liveable urban design features (i.e., safe, convenient pedestrian-friendly, access to shops, transit, and parkland) in general undertook more local walking (in particular transportation walking), and had a stronger sense of community and improved mental health (28).
Residential relocation studies to date have generated mixed findings regarding associations between the built environment and physical activity, few have investigated associations specifically between neighborhood walkability and walking, and the extent to which walkability and walking for different purposes are temporally related remains unclear (26). Further, there is a need for context specific evidence (i.e., Canadian data) to better inform local urban planning and public policy and practice decision-making. Therefore, the aim of our study was to investigate whether changes in neighborhood walkability resulting from residential relocation were associated with leisure, transportation, and total walking among adults in the Canadian context.
Methods
Study and sample design
Previous articles have described the methodological details of the Alberta's Tomorrow Project (ATP) (33, 34). Briefly, ATP is a longitudinal, province-wide study that began in Alberta (Canada) in 2000. From 2000 to 2008, a random sample of adults aged 35–69 years (n = 63,486) were invited to complete a health and lifestyle questionnaire (HLQ), of which n = 31,072 responded. In 2008, n = 20,707 participants completed a first follow-up survey and between 2009 and 2015, n = 15,963 completed a second follow-up survey (34). We undertook secondary (longitudinal) analysis of ATP data that were collected in 2008 and from 2009 to 2015 (herein referred to as “baseline” and “follow-up,” respectively) because the walking outcome measures of interest were consistent between these two surveys. Participant's residential addresses were collected at each survey however, to comply with ethics and to maintain participant anonymity only postal codes could be used for analyses (e.g., linking with built environment data). In our analysis, we included participants from urban areas only, identified from the Forward Sortation Area (FSA) information contained in the first 3-digits of their 6-digit residential postal codes. Rural postal codes include a zero in the second position of the FSA and denotes an area where there are no letter carriers (mail delivered to a post office or postal box). We included only participants from urban areas only because there are urban-rural differences in land use and transportation planning processes, built characteristics may exert different effects on walking in urban vs. rural neighborhoods (35), and in Canadian there are urban-rural differences in the prevalence physical inactivity (36).
Further, we included data from participants that provided complete data on the variables of interest for both the baseline and follow-up surveys, with which built environment data were available and linkable to their survey data (n = 5,977). The median follow-up time between the baseline and follow-up surveys was 2 years. The University of Calgary Conjoint Health Research Ethics Board approved the acquisition and analysis of ATP data for this study (REB17-1466).
Variables
Self-reported physical activity
The International Physical Activity Questionnaire (IPAQ) (37), captured leisure and transportation walking at baseline and follow-up. Leisure walking included walking undertaken for recreation, sport or leisure and transportation walking included walking undertaken to go from place to place. Participants reported the number of days in the past week they undertook at least 10-min of leisure or transportation walking. Participants also reported time spent undertaking walking during a typical day. We multiplied reported days by reported daily minutes to estimate weekly minutes of leisure, transportation, and total walking (i.e., leisure plus transportation walking). As recommended (38), we truncated each walking outcome to 180-min per day to remove outliers and adjust for over-reporting.
Neighborhood walkability
The walkability index used in our analysis has been described elsewhere (39). We geocoded all Alberta 6-digit urban postal codes for 2008–2015 (n = 77,602–84,115) to create points. Canadian urban postal codes provide a reasonable approximation of household location, when complete street address information is not available (e.g., 50% of postal codes are located within a 69 m, and 88% of postal codes are located within 200 m, of the true household address location) (40). Using Geographical Information Systems, we created 400 m Euclidean buffers (polygons) around each geocoded postal code point. Neighborhoods were defined by a 400 m buffer boundary that captured neighborhood built characteristics located within about a 5–10 min walking distance from home (41). Overlaid with a street network file (CanMap Streetfiles and Route Logistics, DMTI Spatial Inc.), we calculated the count of 3-way and 4-way intersections within each buffer. Using the Enhanced Points of Interest (DMTI Spatial Inc.) and available Standard Industry Codes, we calculated the count of business destinations (e.g., hardware stores, department stores, grocery stores, restaurants, banks, libraries, laundry stores, stationery stores, liquor stores, jewelry stores, barbershops, museums, schools, colleges, and universities) within each buffer. To calculate population counts, we overlaid dissemination area census data from Statistics Canada (years 2006, 2011, and 2016) onto the buffers. Dissemination areas (DA) are the smallest standard geographical unit available for research purposes from Statistics Canada (each DA includes ~400–700 persons) (42). DAs have irregular-shaped boundaries that often follow roads or other features (e.g., railways and water features); therefore, they did not match the geographical shape of the circular neighborhood buffers. Thus, the total population count assigned to each buffer was based on a weighted sum that reflected the proportion of geometric overlap between each DA boundary and the buffer (i.e., multiple DAs could overlap a single buffer). For non-census years, we imputed population counts using the averages for years in which census data were available.
For each year (2008–2015) across all buffers, we converted raw counts for intersections (3-way and 4-way), destinations, and population to z-scores. The z-scores were summed to derive a walkability score (WS) for each buffer [WS = [0.5 × z (3-way intersections)] + z (4-way intersections) + z (destinations) + z (population)]. Higher positive scores reflected more walkability, while higher negative scores reflected less walkability. Relative to 4-way intersections, 3-way intersections contribute less to street connectivity and may offer less support for walking (43–45). Therefore, we down-weighted the contribution of 3-way intersections to the WS. The walkability score (estimated for 77,597 postal codes using 2,008 values) was positively correlated with each of the individual built environment variables (i.e., 3-way intersections r = 0.400; 4-way intersections r = 0.753; destinations r = 0.794, and; population count r = 0.605). We also found the WS to have concurrent validity compared against Walk Score®, a valid, widely-used, measure of walkability (46, 47) (r = 0.648; estimate for 81,114 postal codes using 2012 values). The intra-class correlation (ICC) for WSs estimated for postal codes available for all years from 2008 to 2015 (ICC = 0.974) showed that the estimated WS were relatively stable during this period.
We temporally matched participant survey data (baseline and follow-up) with buffer WSs using 6-digit postal codes and created three residential relocation groups. Among movers, the direction of the absolute difference between baseline (origin neighborhood) and follow-up (destination neighborhood) walkability scores, regardless of magnitude, was used to categorize participants as having relocated to either a: (1) less walkable neighborhood or; (2) more walkable neighborhood. Non-movers constituted the third category, regardless of the direction of change in walkability estimated between the baseline and follow-up surveys. Including a non-mover control group is vital for accounting for changes in walking that might have occurred had those moving not relocated neighborhood (i.e., the counterfactual) and for isolating the effect of a change in walkability (e.g., increase or decrease in walkability) on walking.
Sociodemographic characteristics
Sociodemographic variables from the baseline survey included sex, age, children under 18 years of age at home, highest educational attainment, annual gross household income, marital status, employment status, and dwelling type in origin neighborhood (i.e., single dwelling, duplex or row housing, apartment, and other). The season of survey completion was captured.
Statistical analysis
We used descriptive statistics (mean, standard deviation, and frequencies) and inferential statistics (One-way Analysis of Variance—ANOVA, and Pearson's chi-square) to estimate the differences in baseline sociodemographic and walking variables between the three residential relocation groups (i.e., non-movers, moved to less walkability, and moved to more walkability). ANOVA (with Tukey-Kramer post hoc tests) estimated between-group differences in the walkability of the origin and destination neighborhoods (or change over time in the origin neighborhood walkability only for non-movers) for the three residential relocation groups. Dependent (paired) t-tests estimated within-group differences between the walkability of origin and destination neighborhoods for each residential relocation group. Using pooled data (ignoring residential relocation groupings), we performed generalized linear regression (Gaussian distribution with identity link) to estimate the slope coefficients (b) for the baseline cross-sectional associations between walking outcomes and WS, adjusting for covariates (i.e., sex, age, number of children under 18 years of age at home, highest educational attainment, annual gross household income, marital status, employment status, and dwelling type).
We undertook analysis to estimate the average treatment effect on the treated (ATET)—i.e., the estimated difference in leisure, transportation, and total walking between those who moved to less walkability and those who moved to more walkability compared with non-movers. For analytical purposes, we regarded non-movers as a “control” group and those relocating to less or more walkability as the “treatment” groups. Estimating these differences relative to non-movers (control group) accounted for the change in walking behavior among the treatment groups that resulted from factors other than residential relocation and change in walkability exposure.
We used treatment effects models to estimate the ATET. We used the teffects and ipwra Stata commands (48) to derive inverse-probability-weighted regression adjusted estimates to optimize the balance in baseline covariates between the three residential relocation groups and to compute the average treatment-level predicted outcomes (leisure, transportation, and total walking). This approach was taken to make the three residential relocation groups conditionally exchangeable (49), allowing for the average causal effect of relocating to either a less or more walkable neighborhood to be estimated from the contrasts in the average predicted outcomes between these two (treatment) groups relative to non-movers (control group). To compute the inverse-probability-weights, a treatment model (multinomial logit) was first estimated in which the residential relocation group was regressed onto the baseline covariates (i.e., sex, age, number of children under 18 years of age at home, highest educational attainment, annual gross household income, marital status, employment status, dwelling type, WS in origin neighborhood, and total walking minutes). We applied the inverse-probability weights to regression (outcome) models and estimated treatment-specific predicted follow-up walking outcomes for each participant. To control for the influence of seasonality on physical activity (50) and, in particular, walking within the Canadian context (51), we included the season during which the participant returned the follow-up survey as a covariate in the regression models. The mean walking outcomes were calculated for each residential relocation group (treatment-specific predicted outcomes), and contrasts were performed to calculate the differences in the means between the two treatment groups relative to non-movers (control group) to provide the treatment-specific ATET estimates.
STATA's tebalance summarize and teffects overlap commands facilitated covariate balance checking between the three residential relocation groups. We assessed the balance of each baseline covariate using the average standardized absolute mean difference (SMD) and variance ratio (VR) and assessed the visual overlap in the group covariate distributions using box plots and cumulative distribution functions (52). Covariates of perfectly balanced groups have an SMD = 0 and VR = 1; however, we considered groups to be sufficiently balanced if the SMD was | < 0.1| and the VR was 0.5–2 for all covariates (53, 54). We estimated bootstrapped standard errors (1,000 repetitions with replacement) and 95% confidence intervals (95 CI) for the generalized linear and treatment effect models. We considered p-values < 0.05 as statistically significant. We undertook the analysis using Stata/SE 15.1 (StataCorp LLC, College Station, Texas, USA).
Results
Sample characteristics
The sample (n = 5,977) consisted mostly of participants that were female, married, employed and residing in single-dwelling homes and had completed post-secondary education (Table 1). Approximately 5% (n = 298) of our sample relocated neighborhood between the baseline and follow-up surveys. Non-movers, movers to less walkability, and movers to more walkability significantly differed (p < 0.05) concerning their baseline age, marital status, employment status, and dwelling type. Mean weekly minutes of leisure, transportation, and total walking at baseline and follow-up did not significantly differ by residential relocation group, except for follow-up transportation walking (i.e., movers = 119.5, movers to less walkability = 83.5, and movers to more walkability = 128.6; p < 0.05; Table 1). Among all participants from baseline to follow-up (pooled), we found, on average, significant decreases in weekly minutes of leisure walking (Δ = −9.28, 95 CI: −4.26, −14.29, p < 0.001) and total walking (Δ = −8.77, 95 CI: −0.94, −16.59, p = 0.028) but not transportation walking (Δ = 0.83, 95 CI: −4.56, 6.22, p = 0.764).
Among the entire sample, mean (SD; median; minimum; maximum) walkability was −0.09 (2.15; −0.31; −4.32; 11.84) at baseline and −0.07 (2.19; −0.29; −4.37; 12.04) at follow-up. Mean walkability at baseline was significantly different between the residential relocation groups (Table 2). Notably, participants who moved to neighborhoods with less walkability came from origin neighborhoods that were significantly (p < 0.01) more walkable (WS = 0.86) compared with those who moved to more walkability (WS = −0.95) or non-movers (WS = −0.10). Among non-movers, change in walkability between the baseline and follow-up significantly improved (Δ = 0.03; 95 CI: 0.02, 0.05, p < 0.001), albeit by a smaller magnitude relative to the absolute changes in walkability observed among those who moved to less (Δ = −2.14; 95 CI: −2.43, −1.85) and more walkable neighborhoods (Δ = 2.40; 95 CI: 2.01, 2.78; Table 2).
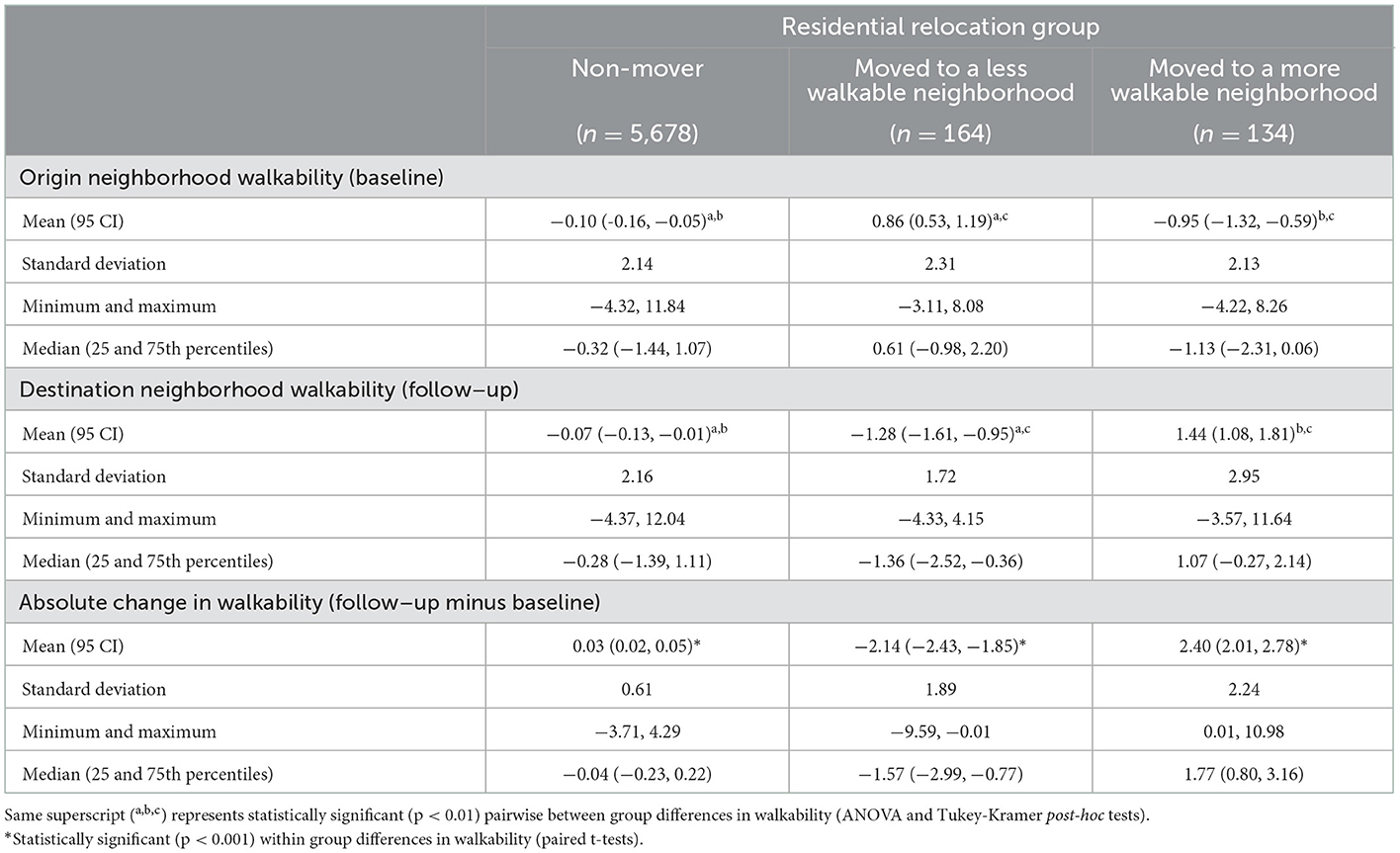
Table 2. Walkability scores for origin and destination neighborhoods by residential relocation group.
Baseline cross-sectional associations between walkability and walking outcomes
Adjusting for all covariates, WS was positively associated with baseline weekly minutes of transportation walking (b = 3.17; 95 CI: 0.82, 5.53; p = 0.008), but not with baseline minutes of leisure walking (b = −1.33; 95 CI: −3.59, 0.94; p = 0.251) or total walking (b = 1.89; 95 CI: −1.60, 5.38; p = 0.288).
Covariate balance between the residential relocation groups
The inverse-probability-weights generated from the treatment model improved covariate balance between the three residential relocation groups concerning the SMDs (unweighted: range = −0.473 to 0.433 vs. weighted: range = −0.117 to 0.247) and VRs (unweighted: range = 0.598 to 2.737 vs. weighted: range = 0.738–1.298; Table 3; Figure 1). Although improved after weighting, the SMD for baseline walkability (SMD from 0.433 to 0.247) did not meet the criteria for establishing covariate balance (i.e., SMD = | < 0.1| and the VR = 0.5–2; Table 3). As a result, we doubly-adjusted for baseline walkability by including it as covariate in the treatment effect model.
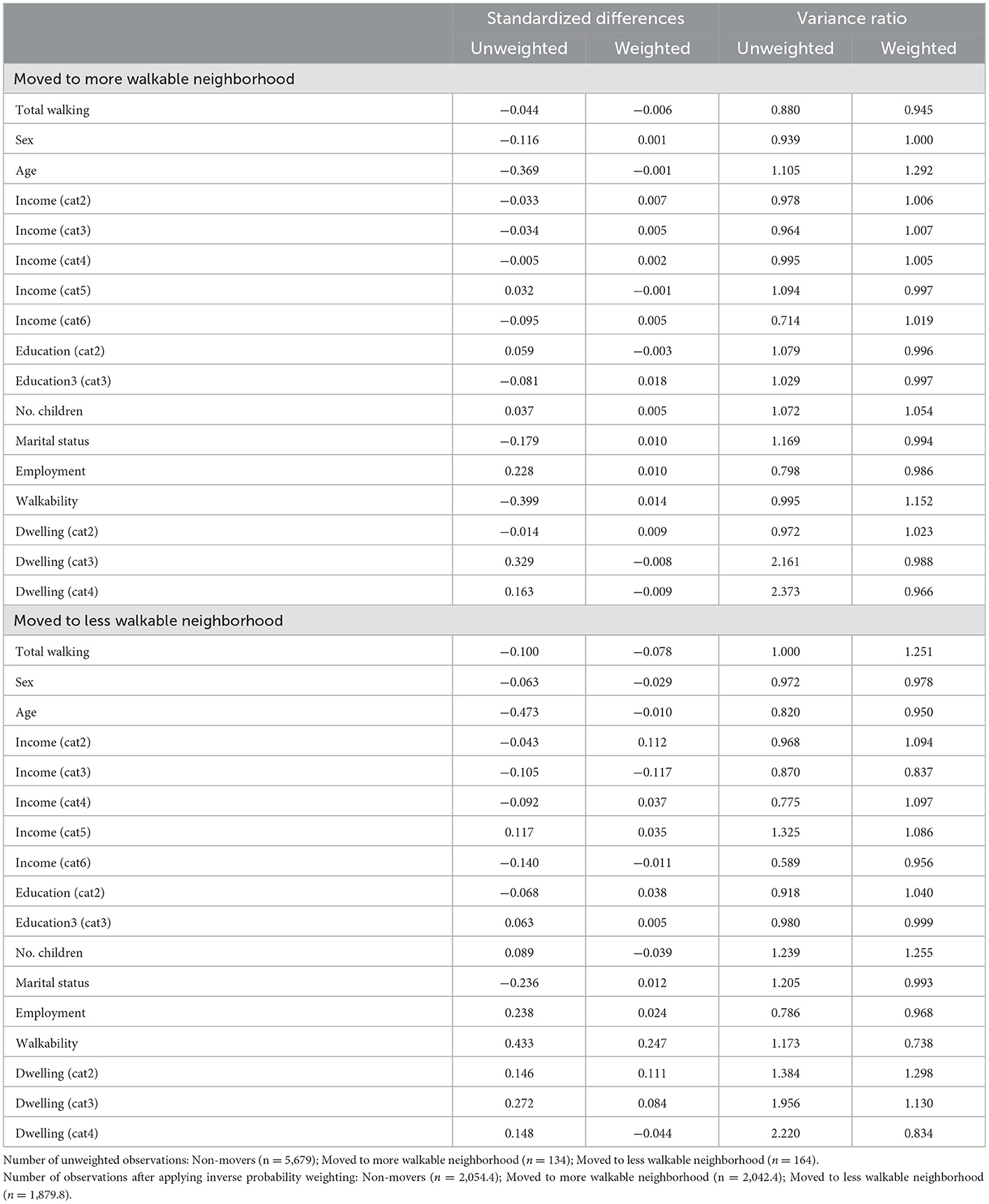
Table 3. Covariate balance of baseline covariates before and after weighting between residential relocation groups.
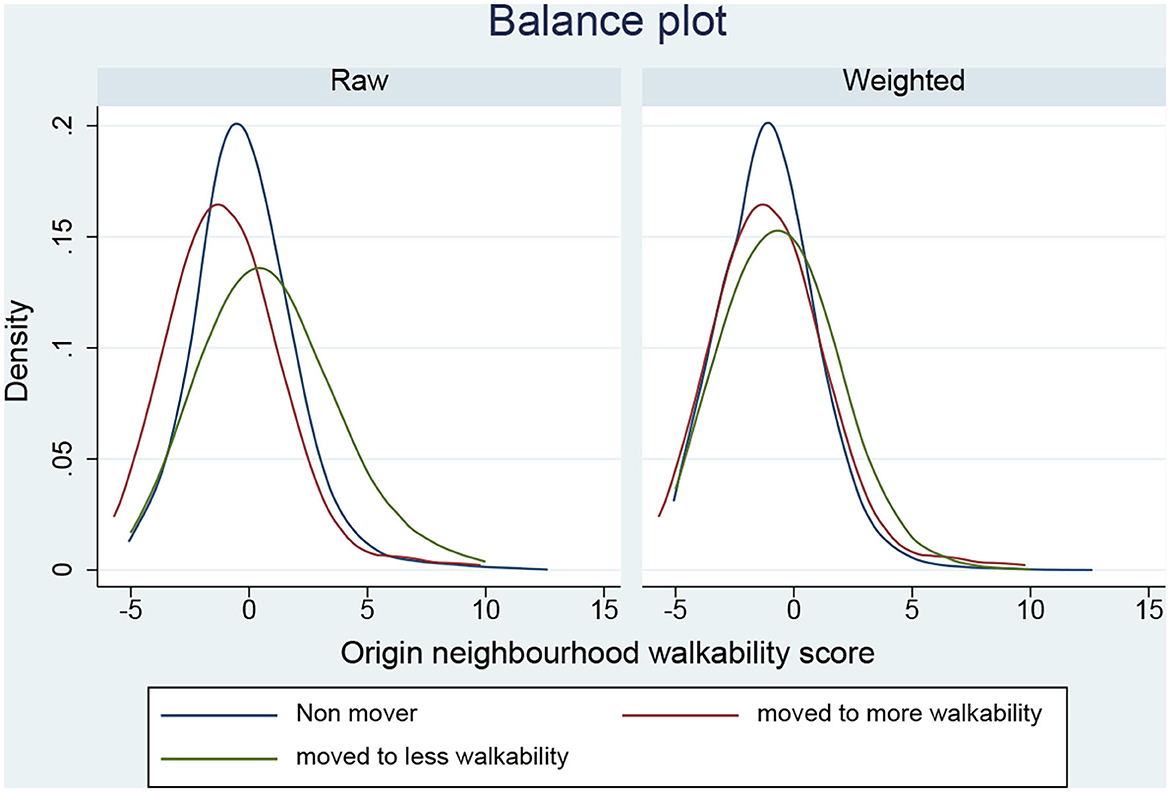
Figure 1. Balance in origin neighborhood walkability scores between residential relocation status groups before and after weighting.
Differences in walking behavior by residential relocation group (ATET)
Compared to non-movers, weekly minutes of transportation walking was significantly lower at follow-up among those who moved to a less walkable neighborhood (−43.48 min/week; 95 CI: −68.17, 17.78, p < 0.01; Table 4). Furthermore, the difference in weekly minutes of total walking at follow-up between non-movers and those who moved to a less walkable neighborhood approached statistical significance (−50.86; 95 CI: −102.67, 0.99, p = 0.054). We found no other statistically significant between-group differences in walking.
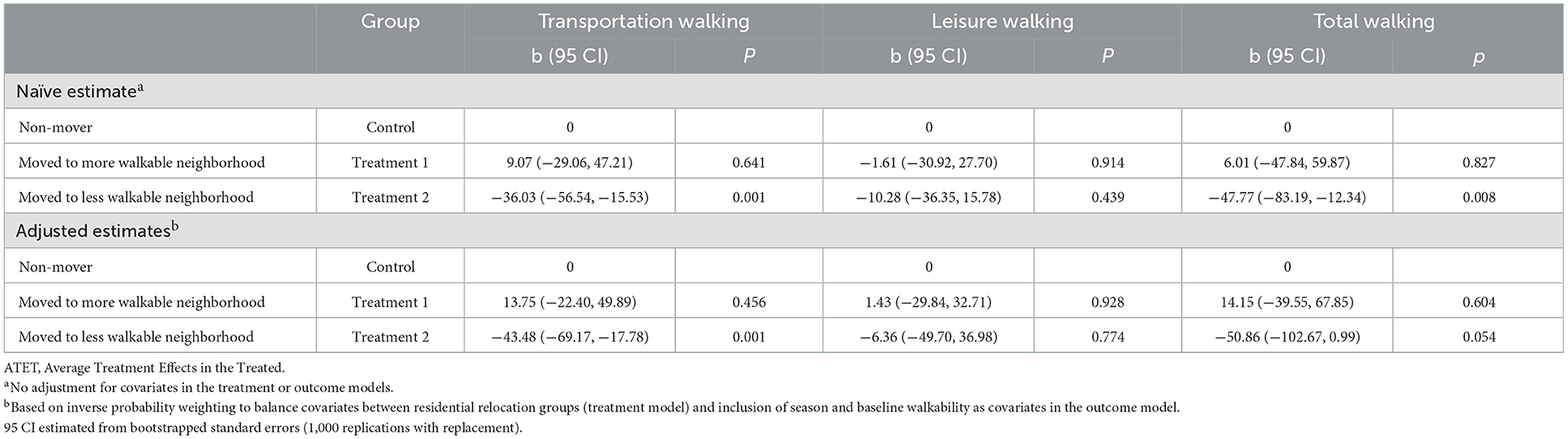
Table 4. Differences (ATET) in weekly minutes per week of walking duration post relocation by relocation group.
Discussion
Residential relocation studies provide a unique opportunity for generating rigorous causal evidence that can inform urban design and public health policy (55, 56). Our study investigated whether a change in neighborhood walkability resulting from residential relocation was associated with leisure, transportation, and total walking levels among adults in the Canadian context. Our findings suggest that relocating to a less walkable neighborhood may have detrimental effects on transport-specific and total walking. Specifically, we found that compared with non-movers, adults moving to less walkable neighborhoods undertook ~41 min less of transportation walking per week. A difference in walking of this magnitude is of clinical relevance, and could have negative physical and mental health consequences (57–60). Our findings are congruent with other studies demonstrating that a change in walkability following residential relocation was associated with transportation walking (29, 30) however; our findings are novel in that we only found a significant difference in transportation walking for those relocating to neighborhoods of less, but not to more walkability. Our cross-sectional and longitudinal findings support the consistent evidence demonstrating that the neighborhood built environment may be more strongly associated with transportation versus leisure walking (18, 23, 24).
A notable finding from this study was that relocating to a less walkable neighborhood may have a greater impact on transportation walking than relocating to a higher walkable neighborhood. The reduction in transportation walking (41 min per week, on average), was in response to an average reduction in walkability (between origin and destination neighborhood) by ~2 standard deviations, independent of the walkability of the origin neighborhood. However, given that walkability was measured as a score which combined three built environment variables (z-scores representing transformed counts of intersections, destinations, and population) translating this score into a description of the change in built environment is difficult. Nevertheless, this finding aligns with the general scientific consensus that higher neighborhood walkability is better for walking, and in particular transportation walking. Our novel finding may reflect the mismatch between walking preferences and built environment characteristics that provide opportunities to walk (61, 62). Specifically, adults preferring to walk for transportation but who relocate to a neighborhood that has an unsupportive built environment (i.e., low walkability) may have no option but to walk less (i.e., a discouraging effect). Alternatively, an adult who relocates to a neighborhood with a built environment that is more supportive (i.e., high walkability) have the option to choose to walk for transportation or not walk according to their preferences. Our finding might also suggest that the transportation walking patterns or habits formed while residing in less walkable neighborhoods remain even after relocating to a more walkable neighborhood. That is, those who did not walk for transport in their origin neighborhoods may be less likely to initiate transportation walking after relocating to a more walkable neighborhood.
Unlike transportation walking, we found that walkability was not associated with leisure walking in either the cross-sectional or longitudinal analysis. Notably, weekly minutes of leisure walking was not impacted by relocating neighborhood (whether it be less or more walkable), which might suggest that preferences for and opportunities to undertake this type of walking is less constrained by the neighborhood built environment. Other studies however, have found changes in leisure walking following neighborhood relocation. For instance, improvements in participation of transportation and leisure walking have been reported among adults who relocated to a mixed-use (e.g., more walkable) neighborhood (63). Nevertheless, leisure walking may be more strongly determined by proximal intrapersonal factors (e.g., self-efficacy, intentions, enjoyment, and perceived barriers) with a smaller influence from the built environment (64, 65). Our lack of significant findings regarding leisure walking may also reflect the built environment characteristics included in our walkability measure. The walkability measure did not include built attributes such as parks and pathways that may be important for supporting leisure walking (66). More research on how changes in exposure to individual built characteristics influences walking undertaken for different purposes is needed (26).
Our findings have important implications. For adults considering relocating neighborhood and wanting to continue to walk for transportation, our findings suggest that these adults should seek out new neighborhoods with at least similar or higher levels of walkability relative to their origin neighborhoods. Publically available tools, such as Walk Score®, Bike Score®, and Transit Score® (available on real estate sites such as Redfin: https://www.redfin.com/) may be useful for identifying new neighborhoods that can support preferences for transport-related physical activity. Moreover, real estate professionals may play an important role in matching home-seekers with neighborhoods that include built characteristics that support their walking preferences (67–69). More importantly, our findings highlight the need for urban development and planning authorities, municipalities, and land developers to increase the supply of newly developed walkable neighborhoods and invest in the redevelopment of existing neighborhoods to increase their supportiveness for transportation walking. Broadening the availability of walkable neighborhoods may reduce the number of people who decrease their transportation walking following relocation as poor design might overshadow people's intentions to walk. Improving the built environment and implementing strategies for improving awareness and education on the importance of walkability among home-seekers is important. Further, our findings lend support for public health strategies (e.g., mass media campaigns and individualized trip planning) that counteract the negative impact of poor urban design in low walkable neighborhoods and capitalize on the supportive pedestrian infrastructure available in walkable neighborhoods.
Our study has several strengths. Our objective-measure of walkability, estimated change in walkability exposure resulting from neighborhood relocation, inclusion of measures of walking for different purposes, statistical adjustment for baseline walking and walkability, inclusion of a control group (“non-movers”), and our two-staged modeling strategy that balanced the observed covariates between the groups prior to treatment effect estimation strengthened internal validity. Non-movers were observed to have a relatively small change in walkability between the baseline and follow-up suggesting that the neighborhood built environment remained relatively stable during the short term (70, 71). Moreover, this temporal stability was also supported by our estimated intra-class correlation for the neighborhood walkability score for years 2008–2015 (ICC = 0.974). Participants included in our study were from urban areas that spanned an entire Canadian province, thus contributing to the representativeness of the sample and our findings.
Our study also has several limitations. The median follow-up time of 2 years, limits our ability to infer whether long-term changes in walking might occur as individuals become more aware, accustomed, and exposed to their new neighborhood surroundings. The walkability variable represented the combination of three built environment variables (intersections, destinations, and population), and while these are important characteristics for supporting walking (16–18), they do not represent all neighborhood built characteristics that might facilitate walking. Examining changes in walking resulting from changes in exposure to neighborhood built characteristics such as transit availability and accessibility, green space, pathways, traffic and personal safety, and aesthetics may be important to consider in future research. Moreover, the small sample of movers did not allow us to examine and test different cut-off scores for change in walkability exposure (i.e., sensitivity analysis), thus we are not able to determine the extent to which magnitude of walkability change affected walking or whether a minimum exposure threshold to elicit a change in walking existed. Walking outcomes were self-reported and may not accurately reflect actual walking (72). Moreover, despite most walking being undertaken close to home (12–15), our walking outcomes were not context-specific (73), thus some of the walking reported likely occurred outside the neighborhood (i.e., at locations further than 400 meters from home). We defined neighborhoods using a 400 m Euclidean buffer representing a 5–10 min walking distance from home (41). Relative to network buffers, Euclidean defined boundaries can result in underestimates of associations between the built environment and walking irrespective of boundary size (74, 75). Speculatively, our walking measure and neighborhood boundary definition likely resulted in conservative estimates of the relationships between neighborhood walkability and walking. The sample included middle-aged to older adults and thus the patterns found in relation to walkability and walking may not generalize to younger adults, limiting the study's generalizability.
Our findings demonstrate that adults who relocate to new neighborhoods that are less walkable than their origin neighborhoods undertake less transportation walking. More longitudinal studies need to investigate the short and long-term changes in walking associated with changes in neighborhood walkability exposure following residential relocation.
Data availability statement
Data that support the findings of this study are available from the Alberta's Tomorrow Project (https://myatp.ca/) following data requisition approval. Requests to access these datasets should be directed to Alberta's Tomorrow Project (https://myatp.ca/).
Ethics statement
The studies involving human participants were reviewed and approved by the University of Calgary Conjoint Health Research Ethics Board (REB17-1466). The patients/participants provided their written informed consent to participate in this study.
Author contributions
GRM, JV, GM, JC, and RM were involved in the conception of the study. JV was involved in the study design and data collection for ATP. GRM, MK, KO, and TN advised on the analysis and interpreted the results. GRM drafted the original manuscript. All authors reviewed and approved the final manuscript.
Funding
This research was supported by the Canadian Institutes of Health Research (GRM; FDN-154331 and FRN-152922). TN was supported by the Japan Society for the Promotion of Science (JSPS) Grants-in-Aid for Scientific Research program (grant: 20H00040). KO was supported by the JSPS Grants-in-Aid for Scientific Research program (grant: 20H04113).
Acknowledgments
The authors acknowledge Anita Blackstaffe and Liam Turley for assisting in the preparation, cleaning, and management of the data. Alberta's Tomorrow Project is only possible due to the commitment of its research participants, its staff and its funders: Alberta Health, Alberta Cancer Foundation, Canadian Partnership Against Cancer and Health Canada, and substantial in-kind funding from Alberta Health Services.
Conflict of interest
RM was employed by Toole Design Group.
The remaining authors declare that the research was conducted in the absence of any commercial or financial relationships that could be construed as a potential conflict of interest.
Publisher's note
All claims expressed in this article are solely those of the authors and do not necessarily represent those of their affiliated organizations, or those of the publisher, the editors and the reviewers. Any product that may be evaluated in this article, or claim that may be made by its manufacturer, is not guaranteed or endorsed by the publisher.
Author disclaimer
The views expressed herein represent the views of the authors and not of the ATP or any of its funders.
References
1. Warburton DER, Nicol CW, Bredin SSD. Health benefits of physical activity: The evidence. CMAJ. (2006) 174:801–9. doi: 10.1503/cmaj.051351
2. Warburton DER, Bredin SSD. Health benefits of physical activity: A systematic review of current systematic reviews. Curr Opin Cardiol. (2017) 32:541–56. doi: 10.1097/HCO.0000000000000437
3. Carlson SA, Adams EK, Yang Z, Fulton JE. Percentage of deaths associated with inadequate physical activity in the United States. Prev Chronic Dis. (2018) 15:E38. doi: 10.5888/pcd18.170354
4. Warburton DER, Charlesworth S, Ivey A, Nettlefold L, Bredin SSD. A systematic review of the evidence for Canada's Physical Activity Guidelines for Adults. Int J Behav Nutr Phys Act. (2010) 7:39. doi: 10.1186/1479-5868-7-39
5. Lee IM, Buchner DM. The importance of walking to public health. Med Sci Sports Exerc. (2008) 40:S512–8. doi: 10.1249/MSS.0b013e31817c65d0
6. Morris JN, Hardman AE. Walking to health. Sports Med. (1997) 23:306–32. doi: 10.2165/00007256-199723050-00004
7. Saint-Maurice PF, Berrigan D, Whitfield GP, Watson KB, Patel S, Loftfield E, et al. Amount, type, and timing of domain-specific moderate to vigorous physical activity among US adults. J Phys Activity Health. (2021) 18:S114–22. doi: 10.1123/jpah.2021-0174
8. Hulteen RM, Smith JJ, Morgan PJ, Barnett LM, Hallal PC, Colyvas K, et al. Global participation in sport and leisure-time physical activities: A systematic review and meta-analysis. Prev Med. (2017) 95:14–25. doi: 10.1016/j.ypmed.2016.11.027
9. Simpson ME, Serdula M, Galuska DA. Walking trends among U.S. adults: The behavioral risk factor surveillance system, 1987–2000. Am J Prev Med. (2003) 25:95–100. doi: 10.1016/S0749-3797(03)00112-0
10. Bryan SN, Katzmarzyk PT. Patterns and trends in walking behaviour among Canadian adults. Can J Public Health. (2009) 100:294–8. doi: 10.1007/BF03403950
11. Althoff T, Sosič R, Hicks JL, King AC, Delp SL, Leskovec J, et al. Large-scale physical activity data reveal worldwide activity inequality. Nature. (2017) 547:336–9. doi: 10.1038/nature23018
12. Powell KE, Martin LM, Chowdhury PP. Places to walk: convenience and regular physical activity. Am J Public Health. (2003) 93:1519–21. doi: 10.2105/AJPH.93.9.1519
13. Spinney JEL, Millward H, Scott D. Walking for transport versus recreation: A comparison of participants, timing, and locations. J Phys Activity Health. (2012) 9:153–62. doi: 10.1123/jpah.9.2.153
14. Suminski RR, Carlos Poston WS, Petosa RL, Stevens E, Katzenmoyer LM. Features of the neighborhood environment and walking by U.S. adults. Am J Prev Med. (2005) 28:149–55. doi: 10.1016/j.amepre.2004.09.009
15. McCormack GR. Neighbourhood built environment characteristics associated with different types of physical activity in Canadian adults. Health Promot Chronic Dis Prev Can. (2017) 37:175–85. doi: 10.24095/hpcdp.37.6.01
16. Wang Y, Chau CK, Ng WY, Leung TM. A review on the effects of physical built environment attributes on enhancing walking and cycling activity levels within residential neighborhoods. Cities. (2016) 50:1–15. doi: 10.1016/j.cities.2015.08.004
17. McCormack GR, Shiell A. In search of causality: A systematic review of the relationship between the built environment and physical activity among adults. Int J Behav Nutr Phys Act. (2011) 8:125. doi: 10.1186/1479-5868-8-125
18. Farkas B, Wagner DJ, Nettel-Aguirre A, Friedenreich C, McCormack GR. A systematized literature review on the associations between neighbourhood built characteristics and walking among Canadian adults. Health Promot Chronic Dis Prev Can. (2019) 39:1. doi: 10.24095/hpcdp.39.1.01
19. Glover TD, Todd J, Moyer L. Neighborhood walking and social connectedness. Front Sports Act Living. (2022) 4:825224. doi: 10.3389/fspor.2022.825224
20. McCormack GR, Cabaj J, Orpana H, Lukic R, Blackstaffe A, Goopy S, et al. A scoping review on the relations between urban form and health: A focus on Canadian quantitative evidence. Health Promot Chronic Dis Prev Can. (2019) 39:187–200. doi: 10.24095/hpcdp.39.5.03
21. Stevenson M, Thompson J, de Sá Hérick T, Ewing R, Mohan D, McClure R, et al. Land use, transport, and population health: Estimating the health benefits of compact cities. Lancet. (2016) 388:2925–35. doi: 10.1016/S0140-6736(16)30067-8
22. Forsyth A. What is a walkable place? The walkability debate in urban design. Urban Design Int. (2015) 20:274–92. doi: 10.1057/udi.2015.22
23. Hajna S, Ross NA, Brazeau AS, Bélisle P, Joseph L, Dasgupta K, et al. Associations between neighbourhood walkability and daily steps in adults: A systematic review and meta-analysis. BMC Public Health. (2015) 15:768. doi: 10.1186/s12889-015-2082-x
24. Grasser G, Van Dyck D, Titze S, Stronegger W. Objectively measured walkability and active transport and weight-related outcomes in adults: A systematic review. Int J Public Health. (2013) 58:615–25. doi: 10.1007/s00038-012-0435-0
25. Karmeniemi M, Lankila T, Ikaheimo T, Koivumaa-Honkanen H, Korpelainen R. The built environment as a determinant of physical activity: A systematic review of longitudinal studies and natural experiments. Ann Behav Med. (2018) 52:239–51. doi: 10.1093/abm/kax043
26. Ding D, Nguyen B, Learnihan V, Bauman AE, Davey R, Jalaludin B, et al. Moving to an active lifestyle? A systematic review of the effects of residential relocation on walking, physical activity and travel behaviour. Br J Sports Med. (2018) 52:789–99. doi: 10.1136/bjsports-2017-098833
27. Scheiner J, Holz-Rau C. Changes in travel mode use after residential relocation: A contribution to mobility biographies. Transportation. (2013) 40:431–58. doi: 10.1007/s11116-012-9417-6
28. Hooper P, Foster A, Bull F, Knuiman M, Christian H, Timperio A, et al. Living liveable? RESIDE's evaluation of the “Liveable Neighborhoods” planning policy on the health supportive behaviors and wellbeing of residents in Perth, Western Australia. SSM-Population Health. (2020) 10:100538. doi: 10.1016/j.ssmph.2020.100538
29. Adhikari B, Hong A, Frank LD. Residential relocation, preferences, life events, and travel behavior: A pre-post study. Res Transport Bus Manag. (2020) 36:100483. doi: 10.1016/j.rtbm.2020.100483
30. Wasfi RA, Dasgupta K, Eluru N, Ross NA. Exposure to walkable neighbourhoods in urban areas increases utilitarian walking: Longitudinal study of Canadians. J Transport Health. (2016) 3:440–7. doi: 10.1016/j.jth.2015.08.001
31. Clary C, Lewis D, Limb E, Nightingale CM, Ram B, Page AS, et al. Longitudinal impact of changes in the residential built environment on physical activity: Findings from the ENABLE London cohort study. Int J Behav Nutr Phys Act. (2020) 17:96. doi: 10.1186/s12966-020-01003-9
32. Braun LM, Rodriguez DA, Song Y, Meyer KA, Lewis CE, Reis JP, et al. Changes in walking, body mass index, and cardiometabolic risk factors following residential relocation: Longitudinal results from the CARDIA study. J Transp Health. (2016) 3:426–39. doi: 10.1016/j.jth.2016.08.006
33. Robson PJ, Solbak NM, Haig TR, Whelan HK, Vena JE, Akawung AK, et al. Design, methods and demographics from phase I of Alberta's Tomorrow Project cohort: A prospective cohort profile. CMAJ Open. (2016) 4:E515–27. doi: 10.9778/cmajo.20160005
34. Ye M, Robson PJ, Eurich DT, Vena JE, Xu JY, Johnson JA, et al. Cohort profile: Alberta's tomorrow project. Int J Epidemiol. (2017) 46:1097–8l. doi: 10.1093/ije/dyw256
35. Frost S, Goins R, Hunter R, Hooker S, Bryant L, Kruger J, et al. Effects of the built environment on physical activity of adults living in rural settings. Am J Health Promot. (2010) 24:267–83. doi: 10.4278/ajhp.08040532
36. Kapral MK, Austin PC, Jeyakumar G, Hall R, Chu A, Khan A, et al. Rural-urban differences in stroke risk factors, incidence, and mortality in people with and without prior stroke. Circulation. (2019) 12:e004973. doi: 10.1161/CIRCOUTCOMES.118.004973
37. Craig C, Marshall A, Sjostrom M, Bauman A, Booth M, Ainsworth B, et al. International physical activity questionnaire: 12-country reliability and validity. Med Sci Sports Exerc. (2003) 35:1381–95. doi: 10.1249/01.MSS.0000078924.61453.FB
38. Wolin KY, Heil DP, Askew S, Matthews CE, Bennett GG. Validation of the international physical activity questionnaire-short among blacks. J Phys Act Health. (2008) 5:746–60. doi: 10.1123/jpah.5.5.746
39. Nichani V, Turley L, Vena JE, McCormack GR. Associations between neighbourhood built characteristics and sedentary behaviours among Canadian men and women: Findings from Alberta's Tomorrow Project. Prev Med. (2021) 150:106663. doi: 10.1016/j.ypmed.2021.106663
40. Bow C, Waters N, Faris P, Seidel J, Galbraith P, Knudtson M, et al. Accuracy of city postal code coordinates as a proxy for location of residence. Int J Health Geogr. (2004) 3:5. doi: 10.1186/1476-072X-3-5
41. James P, Berrigan D, Hart JE, Hipp JA, Hoehner CM, Kerr J, et al. Effects of buffer size and shape on associations between the built environment and energy balance. Health Place. (2014) 27:162–70. doi: 10.1016/j.healthplace.2014.02.003
42. Statistics Canada,. Dictionary, Census Of Population, 2016 - Dissemination Area (DA). (2019). Available online at: https://www12.statcan.gc.ca/census-recensement/2016/ref/dict/geo021-eng.cfm (accessed August 3, 2022).
43. Shashank A, Schuurman N. Unpacking walkability indices and their inherent assumptions. Health Place. (2019) 55:145–54. doi: 10.1016/j.healthplace.2018.12.005
44. Cervero R, Duncan M. Walking, bicycling, and urban landscapes: Evidence from the San Francisco Bay Area. Am J Public Health. (2003) 93:1478–83. doi: 10.2105/AJPH.93.9.1478
45. Herrmann T, Gleckner W, Wasfi RA, Thierry B, Kestens Y, Ross NA, et al. A pan-Canadian measure of active living environments using open data. Health Rep. (2019) 30:16–25. doi: 10.25318/82-003-x201900500002-eng
46. Carr LJ, Dunsiger SI, Marcus BH. Walk score as a global estimate of neighborhood walkability. Am J Prev Med. (2010) 39:460–3. doi: 10.1016/j.amepre.2010.07.007
47. Duncan DT, Aldstadt J, Whalen J, Melly SJ, Gortmaker SL. Validation of walk score for estimating neighborhood walkability: An analysis of four US metropolitan areas. Int J Environ Res Public Health. (2011) 8:4160–79. doi: 10.3390/ijerph8114160
48. Stata.Com. teffects ipwra - Inverse-Probability-Weighted Regresson Adjustment. (2022). Available online at: https://www.stata.com/manuals/teteffectsipwra.pdf (accessed August 3, 2022).
49. Craig P, Katikireddi SV, Leyland A, Popham F. Natural experiments: An overview of methods, approaches, and contributions to public health intervention research. Annu Rev Public Health. (2017) 38:39–56. doi: 10.1146/annurev-publhealth-031816-044327
50. Garriga A, Sempere-Rubio N, Molina-Prados MJ, Faubel R. Impact of seasonality on physical activity: A systematic review. Int J Environ Res Public Health. (2022) 19:2. doi: 10.3390/ijerph19010002
51. McCormack GR, Friedenreich C, Shiell A, Giles-Corti B, Doyle-Baker PK. Sex- and age-specific seasonal variations in physical activity among adults. J Epidemiol Community Health. (2010) 64:1010–6. doi: 10.1136/jech.2009.092841
52. Austin PC. Balance diagnostics for comparing the distribution of baseline covariates between treatment groups in propensity-score matched samples. Stat Med. (2009) 28:3083–107. doi: 10.1002/sim.3697
53. Zhang Z, Kim HJ, Lonjon G, Zhu Y, Big-Data Clinical Trial Collaborative Group AME. Balance diagnostics after propensity score matching. Ann Transl Med. (2019) 7:16. doi: 10.21037/atm.2018.12.10
54. Kainz K, Greifer N, Givens A, Swietek K, Lombardi BM, Zietz S, et al. Improving causal inference: Recommendations for covariate selection and balance in propensity score methods. J Soc Social Work Res. (2017) 8:279–303. doi: 10.1086/691464
55. Giles-Corti B, Sallis J, Sugiyama T, Frank L, Lowe M, Owen N, et al. Translating active living research into policy and practice: One important pathway to chronic disease prevention. J Public Health Policy. (2015) 36:231–43. doi: 10.1057/jphp.2014.53
56. Ramanathan S, Allison KR, Faulkner G, Dwyer JJM. Challenges in assessing the implementation and effectiveness of physical activity and nutrition policy interventions as natural experiments. Health Promot Int. (2008) 23:290–7. doi: 10.1093/heapro/dan022
57. Kelly P, Kahlmeier S, Götschi T, Orsini N, Richards N, Roberts N, et al. Systematic review and meta-analysis of reduction in all-cause mortality from walking and cycling and shape of dose response relationship. Int J Behav Nutr Phys Act. (2014) 11:132. doi: 10.1186/s12966-014-0132-x
58. Moxley E, Habtzghi D. A systematic review comparing dose response of exercise on cardiovascular and all-cause mortality. Home Health Care Manag Pract. (2019) 31:263–73. doi: 10.1177/1084822319831929
59. Warburton DER, Bredin SSD. Reflections on physical activity and health: What should we recommend? Can J Cardiol. (2016) 32:495–504. doi: 10.1016/j.cjca.2016.01.024
60. Craft LL, Perna FM. The benefits of exercise for the clinically depressed. Prim Care Companion J Clin Psychiatry. (2004) 6:104–11. doi: 10.4088/PCC.v06n0301
61. Schwanen T, Mokhtarian PL. What affects commute mode choice: Neighborhood physical structure or preferences toward neighborhoods? J Transport Geogr. (2005) 13:83–99. doi: 10.1016/j.jtrangeo.2004.11.001
62. Badland HM, Oliver M, Kearns RA, Mavoa S, Witten K, Duncan MJ, et al. Association of neighbourhood residence and preferences with the built environment, work-related travel behaviours, and health implications for employed adults: Findings from the URBAN study. Soc Sci Med. (2012) 75:1469–76. doi: 10.1016/j.socscimed.2012.05.029
63. Mumford KG, Contant CK, Weissman J, Wolf J, Glanz K. Changes in physical activity and travel behaviors in residents of a mixed-use development. Am J Prev Med. (2011) 41:504–7. doi: 10.1016/j.amepre.2011.07.016
64. Van Dyck D, Cerin E, Conway TL, De Bourdeaudhuij I, Owen N, Kerr J, et al. Interacting psychosocial and environmental correlates of leisure-time physical activity: A three-country study. Health Psychology. (2014) 33:699–709. doi: 10.1037/a0033516
65. Rhodes RE, Courneya KS, Blanchard CM, Plotnikoff RC. Prediction of leisure-time walking: An integration of social cognitive, perceived environmental, and personality factors. Int J Behav Nutr Phys Act. (2007) 4:51. doi: 10.1186/1479-5868-4-51
66. Christian H, Knuiman M, Divitini M, Foster S, Hooper P, Boruff B, et al. A longitudinal analysis of the influence of the neighborhood environment on recreational walking within the neighborhood: Results from RESIDE. Environ Health Perspect. (2017) 125:e077009. doi: 10.1289/EHP823
67. Trowbridge MJ, Pickell SG, Pyke CR, Jutte DP. Building healthy communities: Establishing health and wellness metrics for use within the real estate industry. Health Aff. (2014) 33:1923–9. doi: 10.1377/hlthaff.2014.0654
68. Carnoske C, Hoehner C, Ruthmann N, Frank L, Handy S, Hill J, et al. Developer and realtor perspectives on factors that influence development, sale, and perceived demand for activity-friendly communities. J Phys Act Health. (2010) 7(Suppl.1):S48–59. doi: 10.1123/jpah.7.s1.s48
69. McCormack GR, Nesdoly A, Ghoneim D, McHugh TL. Realtors' perceptions of social and physical neighborhood characteristics associated with active living: A Canadian Perspective. Int J Environ Res Public Health. (2020) 17:239150. doi: 10.3390/ijerph17239150
70. Timmermans EJ, Visser M, Wagtendonk AJ, Noordzij JM, Lakerveld J. Associations of changes in neighbourhood walkability with changes in walking activity in older adults: A fixed effects analysis. BMC Public Health. (2021) 21:1323. doi: 10.1186/s12889-021-11368-6
71. Bentley R, Blakely T, Kavanagh A, Aitken Z, King T, McElwee P, et al. A longitudinal study examining changes in street connectivity, land use, and density of dwellings and walking for transport in Brisbane, Australia. Environ Health Perspect. (2018) 126:057003. doi: 10.1289/EHP2080
72. Prince SA, Adamo KB, Hamel ME, Hardt J, Gorber SC, Tremblay M, et al. A comparison of direct versus self-report measures for assessing physical activity in adults: A systematic review. Int J Behav Nutr Phys Act. (2008) 5:56. doi: 10.1186/1479-5868-5-56
73. Giles-Corti B, Timperio A, Bull F, Pikora T. Understanding physical activity environmental correlates: Increased specificity for ecological models. Exerc Sport Sci Rev. (2005) 33:175–81. doi: 10.1097/00003677-200510000-00005
74. Li J, Peterson A, Auchincloss AH, Hirsch JA, Rodriguez DA, Melly SJ, et al. Comparing effects of Euclidean buffers and network buffers on associations between built environment and transport walking: the Multi-Ethnic Study of Atherosclerosis. Int J Health Geogr. (2022) 21:12. doi: 10.1186/s12942-022-00310-7
Keywords: urban design, longitudinal, physical activity, built environment, urban form
Citation: McCormack GR, Koohsari MJ, Vena JE, Oka K, Nakaya T, Chapman J, Martinson R and Matsalla G (2023) Associations between neighborhood walkability and walking following residential relocation: Findings from Alberta's Tomorrow Project. Front. Public Health 10:1116691. doi: 10.3389/fpubh.2022.1116691
Received: 05 December 2022; Accepted: 28 December 2022;
Published: 16 January 2023.
Edited by:
Linchuan Yang, Southwest Jiaotong University, ChinaReviewed by:
Andrew Kaczynski, University of South Carolina, United StatesXiaowei Li, Xi'an University of Architecture and Technology, China
Copyright © 2023 McCormack, Koohsari, Vena, Oka, Nakaya, Chapman, Martinson and Matsalla. This is an open-access article distributed under the terms of the Creative Commons Attribution License (CC BY). The use, distribution or reproduction in other forums is permitted, provided the original author(s) and the copyright owner(s) are credited and that the original publication in this journal is cited, in accordance with accepted academic practice. No use, distribution or reproduction is permitted which does not comply with these terms.
*Correspondence: Gavin R. McCormack, gmccorma@ucalgary.ca