A Physical Model Suggests That Hip-Localized Balance Sense in Birds Improves State Estimation in Perching: Implications for Bipedal Robots
- 1Department of Biomedical Engineering, University of Southern California, Los Angeles, CA, United States
- 2School of Engineering, National Autonomous University of Mexico, Mexico City, Mexico
- 3Division of Biokinesiology and Physical Therapy, University of Southern California, Los Angeles, CA, United States
- 4Comparative Biomedical Sciences, Royal Veterinary College, London, United Kingdom
In addition to a vestibular system, birds uniquely have a balance-sensing organ within the pelvis, called the lumbosacral organ (LSO). The LSO is well developed in terrestrial birds, possibly to facilitate balance control in perching and terrestrial locomotion. No previous studies have quantified the functional benefits of the LSO for balance. We suggest two main benefits of hip-localized balance sense: reduced sensorimotor delay and improved estimation of foot-ground acceleration. We used system identification to test the hypothesis that hip-localized balance sense improves estimates of foot acceleration compared to a head-localized sense, due to closer proximity to the feet. We built a physical model of a standing guinea fowl perched on a platform, and used 3D accelerometers at the hip and head to replicate balance sense by the LSO and vestibular systems. The horizontal platform was attached to the end effector of a 6 DOF robotic arm, allowing us to apply perturbations to the platform analogous to motions of a compliant branch. We also compared state estimation between models with low and high neck stiffness. Cross-correlations revealed that foot-to-hip sensing delays were shorter than foot-to-head, as expected. We used multi-variable output error state-space (MOESP) system identification to estimate foot-ground acceleration as a function of hip- and head-localized sensing, individually and combined. Hip-localized sensors alone provided the best state estimates, which were not improved when fused with head-localized sensors. However, estimates from head-localized sensors improved with higher neck stiffness. Our findings support the hypothesis that hip-localized balance sense improves the speed and accuracy of foot state estimation compared to head-localized sense. The findings also suggest a role of neck muscles for active sensing for balance control: increased neck stiffness through muscle co-contraction can improve the utility of vestibular signals. Our engineering approach provides, to our knowledge, the first quantitative evidence for functional benefits of the LSO balance sense in birds. The findings support notions of control modularity in birds, with preferential vestibular sense for head stability and gaze, and LSO for body balance control,respectively. The findings also suggest advantages for distributed and active sensing for agile locomotion in compliant bipedal robots.
1. Introduction
All terrestrial vertebrates have linear and angular acceleration sense localized to the vestibular system of the inner ear. It is well-known that birds use a variety of reflexes mediated by internal signals to stabilize their head during walking and flying (Maurice et al., 2006). Uniquely among living animals, birds appear to have two specialized balance-sensing organs: the vestibular system of the inner ear and an additional balance sensor located between the hips called the lumbosacral organ (LSO) (Necker, 2006) which has been proposed to be especially useful for terrestrial locomotion (Necker, 2005, 2006). Birds have long flexible necks, with head motions tightly coupled to gaze control (Necker, 2007; McArthur and Dickman, 2011; Pete et al., 2015). Consequently, the vestibular system is not closely nor tightly coupled to the torso. In contrast, the LSO is located in the sacrum between the hips, near the CoM. Having a balance organ at the torso is likely to be beneficial to legged locomotion and balance because the hip joint plays an important role on controlling the position of the CoM of the whole body with respect to the foot (Abourachid et al., 2011). Here we consider and contrast the functional implications of hip-localized (LSO) and head-localized (vestibular) balance-sense.
Generally speaking, keeping balance is a task that many legged-animals perform to prevent falling or rotating about the foot point after perturbations (Vukobratovic et al., 2012). Specifically, a balance-sensing organ produces afferent signals to detect current body posture and motion to determine the movements required to achieve or maintain a desired posture and motion. In birds, direct neurophysiological evidence has clearly established that they must possess balance sense that is independent of the vestibular system (Abourachid et al., 2011). They retain the ability to reflexively compensate for body rotations even after labyrinthectomy and spinal cord transection to eliminate descending inputs influenced by the vision and vestibular senses (Abourachid et al., 2011). This neurophysiological evidence, along with particular anatomical features of avian lumbosacral region (below), suggests a balance sensing function of the LSO that complements proprioceptive information from the vestibular system, as well as mechanoreceptors in the skin, joints and muscles.
Anatomically, the LSO is located within an enlargement of the lumbosacral region of the spinal column, between the 27 and 38th segments (Streeter, 1904). The LSO presents a suite of features unique to the spinal column of birds, including bilateral protrusions of neural tissue identified as mechanosensors (accessory lobe (AL) neurons), located adjacent to ligaments supporting the spinal cord (Schroeder and Murray, 1987; Necker, 2005, 2006; Yamanaka et al., 2008). The spinal cord is dorsally bifurcated in this region and supports a “glycogen body” (GB) centered on top. The entire region is enclosed by bony canals with a distinct concentric ring structure (Necker, 2006). The arrangement of the canals, AL, ligaments, and GB is reminiscent of the vestibular system (Necker, 2006) and invites functional analogy to an accelerometer. Each AL contains mechanoreceptors (Schroeder and Murray, 1987; Necker, 2006; Yamanaka et al., 2008), with commissural axons projecting to last-order premotor interneurons in the spinal pattern generating network (Eide and Glover, 1996; Necker, 2006). The AL neurons within the LSO exhibit spontaneous firing and phase-coupled firing in response to vibrational stimulation between 75 and 100 Hz, and ablation of these neurons disrupts standing balance (Necker, 2006). Thus, multiple lines of anatomical and neurophysiological evidence suggest balance-sensing function of the LSO.
Despite evidence of LSO hip-localized balance-sense in birds, no previous studies have provided quantitative evidence for the functional benefits of LSO as an adaptation for posture balance sensing of posture-relevant information. We hypothesize that hip-localized balance sense provides two main functional advantages compared to head-only balance-sense: (1) reduced sensorimotor delay and (2) more accurate state estimation of foot-ground acceleration due to closer proximity to the feet. Here we use a physical model of a perching guinea fowl subject to foot-ground perturbations to test the hypothesis that hip-localized balance sense enables more rapid sensing and accurate state estimation compared to only a head-localized balance sense.
Most birds “perch” (balance with the feet attached to the substrate) when they alight on elevated objects such as branches; therefore we focus on perching as a conveniently simple and ecologically relevant balancing behavior. We built a simple physical model of a standing guinea fowl perched on a horizontal platform (i.e., feet attached to the platform). The horizontal platform was attached to the end effector of a 6 DOF robotic arm, allowing us to apply perturbations analogous to motions of a compliant branch. The physical model provides a first approximation of the muscle-tendon viscoelastic properties that provide leg compliance. We approximated LSO and vestibular balance sensors using 3-D accelerometers located at hips and at the head, respectively. We used system identification to estimate foot-ground acceleration as a function of hip- and head-localized sensing, individually and combined.
2. Materials and Methods
2.1. Physical Model of a Guinea Fowl
A skeletal model of a guinea fowl was built by interconnected and hinged aluminum bars (Figures 1, 2). Friction was reduced by using bearings at the hip, knee, ankle, and foot. The general body size, limb segment lengths and configuration were based on guinea fowl anatomy from the literature (Gatesy and Biewener, 1991; Daley et al., 2007; Gordon et al., 2015), with a hip height of 20 cm.
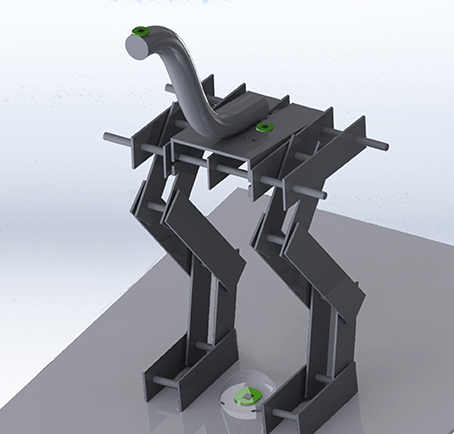
Figure 1. Physical model of the skeleton of the guinea fowl made of articulated aluminum plates and an elastic tube for a neck. The location of the sensors can be seen on the floor between the model's feet, on its pelvis between the hips, and on its head. The joints of the model are, starting from the pelvis: the hip, knee, ankle and metatarsal joints. The transparent sphere around the accelerometer between the feet indicates the scale of random displacements 20 mm in radius.
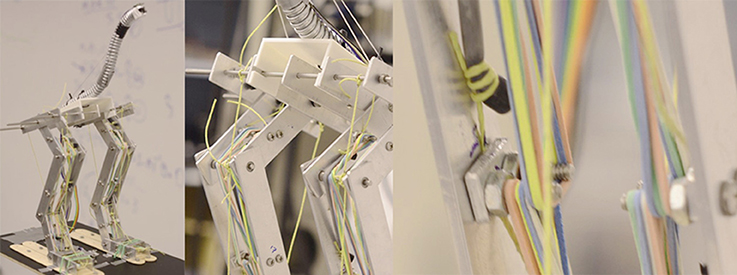
Figure 2. Photographs of the physical model of the skeleton of the guinea fowl. On the left the complete model is shown, on the middle and right sections details of the elastic linkages that are required for the robot to maintain a standing posture can be seen.
This physical model focused on approximating the guinea fowl's (i) LSO (hip) and vestibular (head) balance sensing systems location, (ii) body center of mass location and limb configuration in a standing posture and (iii) visco-elastic mechanical properties of the muscle-tendon-driven limbs. This model was meant as a first approximation of the key physical features, to allow a quantitative comparison of the information available at hip- and head-localized balance sensors. It was not meant to be an exhaustive exploration of the effects of posture, material properties, and muscle-tendon actions. Such considerations could be the subject of future work.
The toes of the model were firmly attached to a platform. Thus, the guinea fowl model “perched” while maintaining an upright standing posture. This posture was maintained by the passive tensions in rubber bands that cross the hip, knee, ankle and metatarsal joints without further assistance or active support (Figure 2). We pre-tensioned rubber bands across joints to represent the tendon-driven functional anatomy of a guinea fowl. These rubber bands also have viscoelastic properties that approximate the passive mechanical properties of “muscles” held at a constant activation level when holding the standing posture. The origins and insertions of the rubber bands were adjusted to have large enough moment arms at each joint to overcome gravity and maintain posture even when perturbed by the moving platform. Our focus was not to explore effects of varying muscle activation patterns for standing postures, but instead to find a set of tensions in the rubber bands sufficient to maintain standing posture and propagate the perturbations from the platform through the skeletal anatomy.
We used two interchangeable necks, each with different stiffness to test the effects of muscle coactivation on balance sensing at the head. Each neck was 25 cm long and curved as shown in Figures 1, 2. The first neck was made of 12.7 mm diameter Ultra-Flex Corrugated Steel Sleeving (McMaster-Carr, part 54885K21). The second was 19.05 mm diameter Abrasion-Resistant Polyurethane Rubber Rod (McMaster-Carr, part 8695K155).
2.2. Instrumentation
The end-effector of a 6 degrees of freedom (DOF) AdeptSix 300 robotic arm (Omron Adept Technologies, Inc, San Ramón, CA) hold the platform where the model perched (Figure 3). We used 3-D accelerometers at the following locations on the model: (i) head to represent the vestibular system; (ii) hip to represent the LSO sensor, and (iii) between the feet to record the reference perturbations or “foot acceleration” (Figure 1). All accelerometers were MEMS inertial sensors Model LIS344ALH (ST Microelectronics, Geneva, Switzerland).
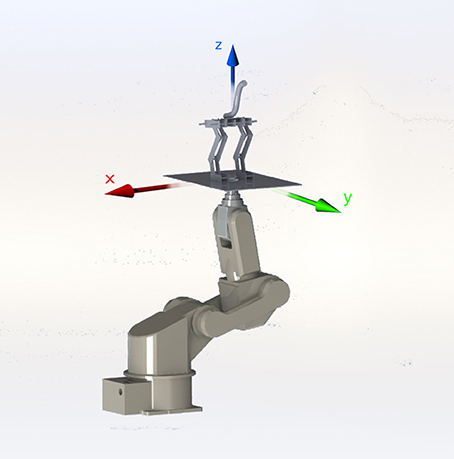
Figure 3. Generating 3D movements with the 6-DOF AdeptSix 300 robotic arm enabled us to apply repeatable and specific type of perturbations to our model.
2.3. Trials
Each trial replicated a scenario that a guinea fowl might face while perching on a tree branch which is subject to perturbations from weather and other animals. Our goal was not to replicate natural perturbation exactly, but to provide a general test of our hypothesis that the LSO has benefits over the vestibular system for rapid and accurate state estimation for balance.
Each trial consisted of a series of 3,000 random, uncorrelated displacements generated by the robotic arm. Each displacement was a center-out-and-back movement in a random direction to the surface of spheres with 2, 5, 10, and 20 mm in radius. Trials were block-randomized across sphere sizes. We recorded a total of eight trials (4 sphere sizes × 2 necks stiffnesses) (Table 1).
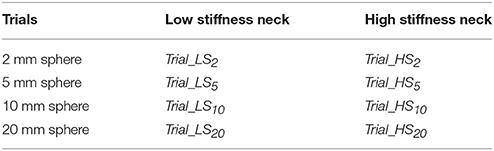
Table 1. Each trial consisted of 3,000 random center-out-and-back displacements (center-surface of a sphere).
2.4. Data Acquisition
We used a high-performance National Instruments (NI) PXI-8108 computer, upgraded with 4 GB DDR2 RAM and a 500 GB SSD. An NI PXI-6254 ADC card recorded the accelerations signals. The data acquisition hardware was housed in the NI PXI-1042 chassis. We acquired data at the sampling rate of 1 kHz.
2.5. Data Analysis
2.5.1. Estimation of Neck Stiffness
To estimate the effective neck stiffness, we performed a boot-strap analysis of 1,000 trials by randomly selecting 30 s segments from each trial. We then found the resonant frequency (the frequency with maximal power) of accelerations at the head. The effective muscle stiffness was estimated from:
where i is the neck number, mi the mass and fi the resonant frequency.
2.5.2. Estimation of Sensory Delay at the Hip and Head
We calculated cross-correlation of foot acceleration against that recorded from hip or head to estimate the propagation delays of the applied mechanical perturbations. The delay was taken as the lag where the cross-correlation was maximal.
2.5.3. Estimation of the Time History of Foot Acceleration
We used a data-driven modeling approach to estimate the time history of the foot acceleration given the time history of signals recorded at the sensory sites (hip and head). To this end, we trained state-space models (in the least-squares sense) to predict foot acceleration from the hip or head accelerations. We used MOESP state-space identification (Verhaegen and Dewilde, 1992; Verhaegen and Verdult, 2007) implemented in the State-space Model Identification (SMI) MATLAB toolbox (Haverkamp and Verhaegen, 1997). The state-space model is represented as follows:
where accsensor(k) is the input signal (acceleration signal recorded from the hip or neck) and accfoot(k) is the measured foot acceleration. x(k) is the state variable, and A, B, C, D are the unknown state-space matrices. We set the model order to three after inspecting the singular values of the extended observability matrix as described in the previous work (Haverkamp, 2000). The model order of three resulted in 21 parameters that was significantly less than the number of 4,000 available training data points for each training run. Since the number of free parameters was much <10% of the training data, the model is not over-parameterized and cannot learn noise and the stochastic behavior.
We assessed the performance of the model in predicting the foot acceleration (). By running the identified models in the prediction mode, we compared the predictions to the actual measured signals, accfoot. We quantified the difference using the identification Variance Accounted For (VAF) expressed as:
where 100% indicates a perfect prediction of all the variability in the measured signals, and 0% means no meaningful prediction.
2.5.4. Boot-Strap Analysis and Statistics
To estimate the robustness of the analyses (cross-correlation, system identification, etc.), we performed a 100 trials boot-strap study (random sampling with replacement) (Efron and Tibshirani, 1994). For each trial, we randomly chose 40 s windows from the measured data, performed the cross-correlation and system identification analyses, and then calculated summary statistics across the 100 measures. We performed student's t-test for statistically significant differences between conditions. Values for central tendency and variance are reported as medians (interquartile range) unless stated otherwise.
3. Results
We first present the differences in neck stiffness, then the effect of sensor location and neck stiffness on (i) sensing delay, and (ii) estimation of foot acceleration.
The necks made from two different materials have different bending stiffnesses whose estimates are shown in Figure 4. Since we measured the dynamical response of the entire physical model (see section 4), each direction and magnitude of perturbation induced a different dynamical response that resulted in a different acceleration measured at the head. This led to different resonant frequencies to be multiplied by the mass of the neck (Equation 1). Note that we would obtain different estimates of neck stiffnesses if the square of the resonant frequency at the head were multiplied by the mass of the whole model. Doing this would have given us an approximation of the stiffness of the whole body, which besides the neck, has a fixed stiffness. Also, if the complete body mass were considered, mass differences between trials with different necks would have been smaller, resulting in a constant bias that would not change the statistical differences between the estimates of neck stiffness. The median neck stiffnesses were 0.67 (0.26–1.05) N/m and 1.25 (0.56–1.55) N/m for the low and high stiffness necks, respectively. Student t-test shows the average neck stiffness are significantly different (p < 0.05).
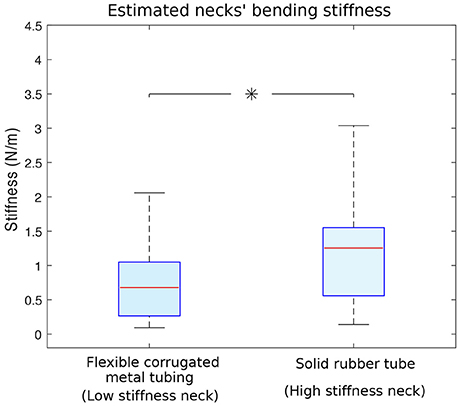
Figure 4. Estimated bending stiffness of the two necks. Neck stiffnesses were calculated using data from 1,000 different trials and the simple lumped-parameter model in Equation 1. Left: stiffness calculated for the flexible corrugated metal tubing (i.e., low stiffness neck). Right: stiffness calculated for the solid rubber tube (i.e., high stiffness neck) Flexible corrugated metal tubing and solid rubber tube data were statistically different (with p < 0.05 and indicated with an asterisk), their respective medians are 0.67 and 1.25 N/m.
As expected, an accelerometer at the hip generally detected foot acceleration with shorter delays than the accelerometer at the head. Foot-to-hip median delays were 0.02 (0–0.03) s and 0.03 (0.005–0.065) s, respectively for the low and high stiffness necks. Foot-to-head median delays were longer, measured at 0.095 (0.06–0.135) s for the low stiffness neck, and 0.055 (0.02–0.07) s for the high stiffness one (Figure 5). The variability was quite large as the shown information collapses data across different acceleration axes and different sphere experiments (perturbation magnitudes).
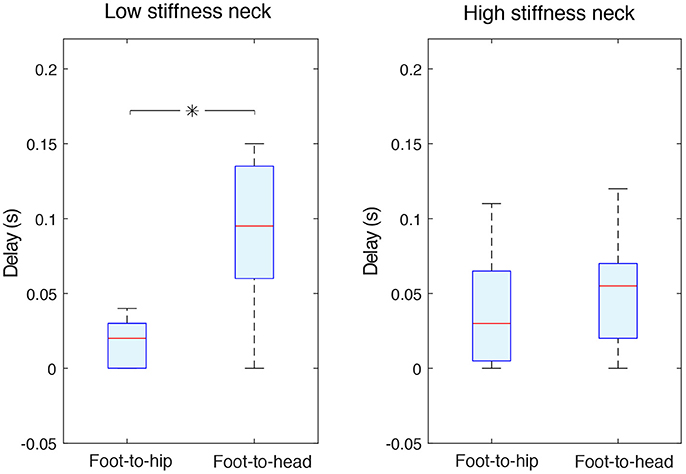
Figure 5. Independently of the neck stiffness, foot-to-hip delays were shorter than foot-to-head ones. The two data groups in the panel corresponding to the low stiffness neck (left panel) were statistically different (with p < 0.05 and indicated with an asterisk); this is not the case for high stiffness neck data (right panel). Foot-to-head median delays were longer, measured at 0.095 s for the low stiffness neck, and 0.055 s for the high stiffness one. Foot-to-hip median delays were 0.02 and 0.03 s respectively for the low stiffness and high stiffness necks.
Foot-to-hip delays were significantly shorter than foot-to-head delays (p < 0.05) for the low stiffness neck, but not significantly different for the high stiffness neck (Figure 5). A stiffer neck reduced the delays for information sensed at the head. This resulted in hip and neck delays that were very similar with no statistical difference.
Estimates of acceleration at the feet are more accurate when using signals from the hip-mounted accelerometers than from the head-mounted accelerometers. Figure 6 shows an example where acceleration at the feet is estimated from the hip- and head- mounted accelerometer, overlaid with the ground-truth signal measured at the feet.
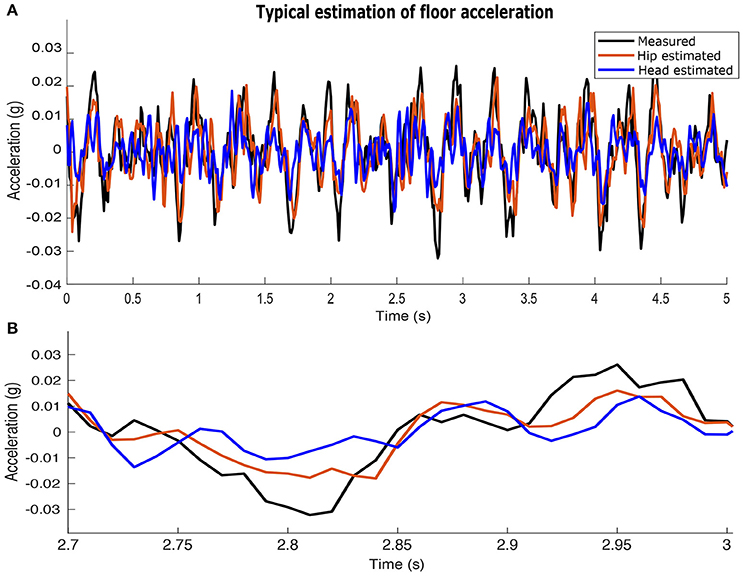
Figure 6. Example of the acceleration at the feet in the sagittal plane estimated from the measured accelerations at the hip and head. The acceleration at the hip yields more accurate estimates of acceleration at the feet. (A) a 5 (s) time window. (B) a 300 (ms) time window.
Hip-localized estimates of the foot acceleration accounted for 30.81–48.96% of variance (% VAF as defined in Equation 3) against 15.59–22.19% of head-localized estimates (Figure 7). This figure summarizes the estimation results by pooling together data from both neck stiffnesses. Prediction of foot acceleration as a function of neck type is shown in Figure 8. Particularly, Figure 7 shows data separated as a function of perturbation magnitude. It demonstrates that independently of the perturbation magnitude, the estimate of foot acceleration from the hip was always more accurate than that from the head sensor. Moreover, sensory fusion (combining info from both sensors) did not significantly improve the foot acceleration estimation. Therefore, sensory fusion did not provide additional benefits beyond hip-only sensing.
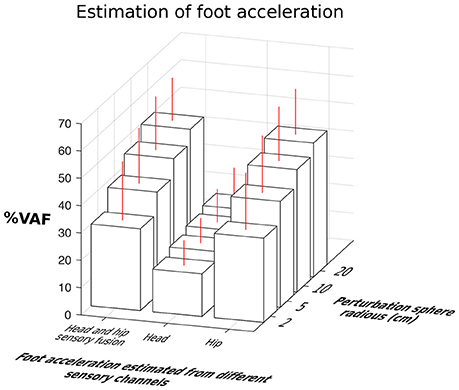
Figure 7. Comparison of estimation accuracy of foot acceleration from the hip, head and their fusion as a function of perturbation magnitudes. Hip-to-feet compared to head-to-foot acceleration estimation was more accurate (p < 0.05). Fusion of the hip and head information did not improve estimation of the foot acceleration beyond that obtained with hip information along.
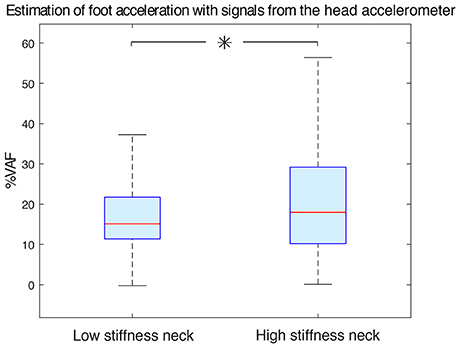
Figure 8. Estimation accuracy of foot acceleration as a function of neck type. Increasing neck stiffness improved estimation of foot acceleration from acceleration measured at the head. Low stiffness and high stiffness neck data were statistically different (with p < 0.05 and indicated with an asterisk). The median %VAF was 15.11 and 17.95 for the low stiffness neck and high stiffness neck respectively.
We have found that when only head-localized accelerometers were available, the high stiffness neck improved estimates of foot acceleration compared to the low stiffness neck (Figure 8). With the low stiffness neck, the median VAF was 15.11 (11.38–21.74)%, while it was 17.95 (10.18–29.19)% for the high stiffness one. These data groups were statistically different (p < 0.05).
4. Discussion
To validate the anatomical and neurophysiological evidence of LSO balance sensing function in birds, we present a quantitative investigation of the functional benefits of hip-localized balance sense. Here we investigated the perturbation sensing dynamics of a physical model of a guineafowl perched in a standing posture. We explored two proposed functional advantages of hip-localized compared to head-localized balance sense: minimization of sensorimotor delay and improved estimation of foot-ground acceleration, due to closer proximity of the sensor to the feet. To our knowledge, this is the first study to quantitatively analyze the practical benefits of hip-localized sensing of accelerating for balance control. We find that a hip-localized acceleration sensor—analogous to the LSO—provides shorter delays and improved state estimation of feet acceleration during substrate perturbations.
In particular, our experimental paradigm applied displacements at the feet, where we also measured the “ground truth” acceleration of the moving substrate on which the bird is perched. We then compared the ability to sense and reconstruct that ground truth acceleration on the basis of accelerations measured at the hip and head. We find that the location of these simulated balance sense organs has important consequences to how a bird (a model of a guinea fowl, in this case) could use acceleration information from hip-localized balance sense for bipedal perching, standing and locomotion. A second level of analysis focused on the material properties of the neck of the physical model. One was (less stiff) corrugated tubing, and the other (more stiff) solid rubber tubing. These material differences were designed to explore the effect of muscle co-contraction at the neck as a means of active sensing, or at least modulation of the utility of head-localized balance sense.
Before discussing the results in detail, it is important to clarify some features of our experimental approach to balance sense. A salient feature of our experimental results is the variability in our results, as in Figure 4. Shouldn't the bending stiffness of each neck be thought as a single number? Similarly, shouldn't the foot-to-hip delays be constant and the same independently of neck stiffness (Figure 5)? Recall that the stiffness of the system is inferred from the resonant frequency of the acceleration measured at the head. The acceleration at the head is a function of the the dynamical response of the entire guinea fowl model to input perturbations. In fact, we are measuring the frequency response and delays of the coupled oscillations of the legs held in a standing position by rubber bands, plus the pelvis and neck. Given that this physical structure is only symmetric in the sagittal plane, its dynamical response will depend on the direction of the 3D perturbations—which naturally results in variability in our results. Nevertheless, the corrugated tubing condition (“low stiffness neck”) leads to perturbation responses at the head that, in general and on average, reflect a lower stiffness for this lumped-parameter analysis. Similarly, foot-to-hip delays were, in general and on average, shorter than the feet-to-head delays. In a sense, instead of “neck stiffness,” the results in Figure 4 may be better called the “apparent stiffness lumped at the head.” But given that the purpose of this analysis is to test for the effect of the material properties of the neck on time delays and estimation accuracy, we chose not to belabor this point and simply call it “neck stiffness.” After all, (i) the neck is the only body part that was swapped, and (ii) changes in material properties only at the neck better reflect the potential effects of muscle co-contraction at the neck in the guinea fowl.
There are limitations to our approach that, while worth mentioning, we believe do not challenge the validity of our results. Importantly, our physical model can only approximate the anatomy and muscle mechanics of the guinea fowl. Our multi-link articulated structure approximates only the general link-segment arrangement and length proportions of the animal skeleton, and the viscoelastic rubber bands only roughly approximate the properties of muscle-tendon linkages. Similarly, we did not consider the proprioceptive signals coming from the joints, skin and muscles that could also contribute to state estimates of foot acceleration. While these limitations prevent us from claiming that our results are direct parallels of how a guinea fowl would respond neuro-mechanically to perturbations, it is nevertheless a valid means to test for differences in sensory signals as a function of sensor location and neck stiffness. Moreover, we explicitly avoided making the assumption that the skeleton of the guinea fowl was simply a set of links rigidly fused at a given posture. Rather, we used rotating hinges at the joints, where the posture of the model was achieved by appropriately setting the lengths and tensions of the rubber bands to approximate muscle-tendon actions to maintain posture at rest. This mechanical structure—as a first approximation—provides a biomechanically realistic instantaneous response to a perturbation at the feet, and avoids other multiple assumptions associated with a computational model (Martins et al., 2009). The results we present here are an analysis of the aggregate acceleration responses to a sequence of center-out 3-dimensional perturbations. As such, we consider the details of each response only implicitly. Future research could explore the moment-to-moment details of the responses within an individual perturbation.
The biological interpretation of these results hinges on the assumption that the functional benefits of hip-localized balance sense could translate into selective evolutionary pressure to promote the anatomical specialization of the LSO in evolutionary time. This assumption is supported by two fundamental control-theoretical notions: (i) that delays are detrimental because they make any causal closed-loop controller (biological or engineered) more unstable (Gu et al., 2003) and (ii) that having a more faithful estimate of a perturbation improves the corrective response, and thus improving performance, economy and stability.
The simplest interpretation of the time delays hinges on the notion that a causal feedback controller has knowledge of the past, but not of the present (strictly speaking) or future. Therefore, it cannot execute anticipatory control actions and is thus limited by its closed-loop bandwidth. In contrast, biological systems are well-known to produce anticipatory motor commands (Aruin and Latash, 1995; Westwick and Perreault, 2011), as well as short-latency reflexive responses (Sinkjær et al., 1999; Jalaleddini et al., 2017b,a). Anticipatory strategies are considered to be critical adaptations to mitigate the deleterious effects of large transmission and processing delays inherent to neural systems (Bean, 2007; Faisal et al., 2008). Nevertheless, any voluntary, anticipatory or reflexive action would benefit from shorter delays. This point is supported by the observation of many morphological and physiological adaptations in the nervous systems to reduce time delays such as increased axonal diameter, myelination and saltatory conduction.
The biological relevance of state estimation (Kalman, 1960; Simon, 2006) relates to the fact that physiological sensory signals contain task-relevant information, but not necessarily in the coordinates and units used by the controller. In particular, some version of the “state” of the system is encoded in sensory coordinates and units that are different from those used by the neural controller to select, plan and execute a response. This means that any raw sensory signal (e.g., acceleration at the LSO or vestibular system) must first be interpreted to extract useful information (e.g., the details of the perturbation at the feet). The MOESP state-space identification technique is but one example of a state estimator (Verhaegen and Dewilde, 1992; Verhaegen and Verdult, 2007). To test our hypothesis, it suffices to show that a hip-localized balance sensing organ is better at sensing, estimating, and reconstructing the perturbations at the feet than a head-localized one, Figure 6. On the same figure, we only show forward/backward accelerations (i.e., along the y axis), which are the most destabilizing during locomotion. It has been shown that lateral (i.e., side-to-side) movements are more stable than forward/backward movements because stance width naturally provides a stabilizing effect (Dean et al., 2007). Whether and how the concept of state estimation applies to the nervous system, however, is yet unresolved (Loeb, 2012).
Necker stated in the concluding paragraph of his 2006 paper that “The local organization of the neuronal network [of the LSO] favors rapid and hence effective control,” with no further elaboration (Necker, 2006). We now present what is, to the best of our knowledge, the first concrete evidence that a hip-localized balance sense organ (like the LSO) is an effective source of faster and better sensing of posture-relevant information. Faster sensing is evidenced by the shorter time delays for hip-localized vs. a head-localized accelerometers. Moreover, our results also show that the time delays for head-localized balance sense organs can be shortened by cocontracting neck muscles (i.e., a stiffer neck). From the state estimation point of view, however, we find that hip-localized balance sense organs are superior, and do not benefit from sensory fusion with head-localized acceleration—independently of neck stiffness. Therefore, we conclude that hip-localized balance sense indeed promotes more rapid and effective control.
These results have important implications for how the evolution of hip-localized balance sense by the LSO might have contributed to the unique sensorimotor control features of birds. In particular, it has long been recognized that birds have relatively “modular” function and control of wings, legs and tail compared to other vertebrates (Gatesy and Dial, 1996). The functional dissociation between forelimb (wing) for aerial locomotion and hindlimb (leg) for terrestrial locomotion is paralleled by increased autonomy of their respective sensorimotor control networks (Biederman-Thorson and Thorson, 1973; Jacobson and Hollyday, 1982; Sholomenko and Steeves, 1987; Ho and O'Donovan, 1993; McArthur and Dickman, 2011). The presence of a local and distributed balance sensing organ that is directly integrated with hindlimb spinal networks has likely contributed to this modular control organization. The mechanosensing neurons of the LSO project directly to pre-motor neurons in the spinal cord (Eide, 1996; Necker, 2006). This suggests the balance sense information produced by the LSO is likely to contribute to rapid and effective control because it is processed locally. Such local processing is advantageous because involving the brain in the response could introduce counterproductive time delays.
While our results focus on perching, hip-localized balance sense is likely beneficial for other postural and locomotor tasks. We designed our perturbations to simulate sensory inputs analogous to bird perching on a branch subject to varied 3-D movements such as wind, movements of other animals, etc. During perching, a bird is exposed to 3-D substrate perturbations, for which short-latency reflex responses could suffice, if sufficiently rapid sensing is available. This is similar to the observed knee and ankle strategies in the control of human upright posture (Bingham et al., 2011), or slip-grip mechanisms for human finger manipulation (Cole and Abbs, 1988). Moreover, such rapid and informative sensing is also critical to low-level (distributed, spinal or sub-cortical) sensorimotor processing to control short-latency responses to perturbations (Lawrence et al., 2015a,b) that ultimately supports long-latency control of voluntary function in general. The LSO is directly integrated with the hindlimb spinal motor control networks (Eide, 1996; Necker, 2006), suggesting that hip-localized balance sense is likely relevant to all hindlimb-mediated behaviors, including perching, standing balance, over-ground locomotion and arboreal locomotion. Birds effectively have two distinct balance sensorimotor processing centers: the “cerebral brain,” responsible for executive function and navigation, and the “sacral brain,” responsible for low-level, short latency control of terrestrial perching, standing and locomotion.
Adopting lessons from the millions of years of biological evolution poses intriguing and exciting possibilities for the engineering evolution of robust and versatile bipedal robots. There are well-known forms of morphological control where the structure of the body co-evolves with the nervous system (or controller) to simplify and improve open- or closed-loop control (Lipson and Pollack, 2000; Valero-Cuevas et al., 2007; Pete et al., 2015). At the other extreme we have the classical robotics approach to fully centralized control that depends on algorithms that process sensory information and issue motor commands. The LSO provides support for an intermediate alternative, where one can have the benefits of morphological adaptations and central control—but supplemented by distributed neural control centers informed by distributed balance sense organs like the LSO.
Author Contributions
DU-M designed and constructed the physical model of the guinea fowl, wrote the Title, Abstract, Introduction, did renders of the physical model of the guinea fowl and put together different author ideas and perspectives. KJ guided the NI DAQ implementation and data analysis activities: system identification analysis, bootstrap analysis and statistics. Together, DU-M and KJ implemented the NI DAQ system, programmed the AdeptSix 300 robotic arm, did data analysis on MATLAB, wrote the Methods section created and edited the figures. MD and FV-C provided the initial idea of giving an engineering quantitative analysis for functional benefits of the LSO balance sense in birds. They wrote most of the Discussion section and validated: (i) the design and construction of the physical model of the guinea fowl, (ii) data analysis activities, and (iii) each of the paper sections and figures. All the authors contributed to editing the paper for style, clarity, succinctness and grammar.
Funding
Research reported in this publication was supported by the National Institute of Arthritis and Musculoskeletal and Skin Diseases of the National Institutes of Health under Awards Number R01 AR-050520 and R01 AR-052345, and by the Department of Defense under award number MR150091 to FV-C. The content of this endeavor is solely the responsibility of the authors and does not necessarily represent the official views of the National Institutes of Health or the Department of Defense. This work was also supported by Fonds Québécois de la Recherche sur la Nature et les Technologies to KJ.
Conflict of Interest Statement
The authors declare that the research was conducted in the absence of any commercial or financial relationships that could be construed as a potential conflict of interest.
Acknowledgments
Author MD would like to thank Alexander Spröwitz for discussions on the potential balance sensing function of the lumbosacral organ of birds.
References
Abourachid, A., Hackert, R., Herbin, M., Libourel, P. A., Lambert, F., Gioanni, H., et al. (2011). Bird terrestrial locomotion as revealed by 3D kinematics. Zoology 114, 360–368. doi: 10.1016/j.zool.2011.07.002
Aruin, A. S., and Latash, M. L. (1995). The role of motor action in anticipatory postural adjustments studied with self-induced and externally triggered perturbations. Exp. Brain Res. 106, 291–300.
Bean, B. P. (2007). The action potential in mammalian central neurons. Nat. Rev. Neurosci. 8, 451–465. doi: 10.1038/nrn2148
Biederman-Thorson, M., and Thorson, J. (1973). Rotation-compensating reflexes independent of the labyrinth and the eye. J. Comp. Physiol. 83, 103–122. doi: 10.1007/BF00696890
Bingham, J. T., Choi, J. T., and Ting, L. H. (2011). Stability in a frontal plane model of balance requires coupled changes to postural configuration and neural feedback control. J. Neurophysiol. 106, 437–448. doi: 10.1152/jn.00010.2011
Cole, R. J., and Abbs, J. H. (1988). Grip force adjustments evoked by load force perturbations of a grasped object. J. Neurophysiol. 60, 1513–1522.
Daley, M. A., Felix, G., and Biewener, A. A. (2007). Running stability is enhanced by a proximo-distal gradient in joint neuromechanical control. J. Exp. Biol. 210, 383–394. doi: 10.1242/jeb.02668
Dean, J. C., Alexander, N. B., and Kuo, A. D. (2007). The effect of lateral stabilization on walking in young and old adults. IEEE Trans. Biomed. Eng. 54, 1919–1926. doi: 10.1109/TBME.2007.901031
Efron, B., and Tibshirani, R. J. (1994). An Introduction to the Bootstrap. Boca Raton, FL: CRC Press.
Eide, A. L. (1996). The axonal projections of the hofmann nuclei in the spinal cord of the late stage chicken embryo. Anat. Embryol. 193, 543–557.
Eide, A. L., and Glover, J. C. (1996). Development of an identified spinal commissural interneuron population in an amniote: neurons of the avian hofmann nuclei. J. Neurosci. 16, 5749–5761.
Faisal, A. A., Selen, L. P., and Wolpert, D. M. (2008). Noise in the nervous system. Nat. Rev. Neurosci. 9, 292–303. doi: 10.1038/nrn2258
Gatesy, S., and Biewener, A. (1991). Bipedal locomotion: effects of speed, size and limb posture in birds and humans. J. Zool. 224, 127–147.
Gatesy, S. M., and Dial, K. P. (1996). Locomotor modules and the evolution of avian flight. Evolution 50, 331–340.
Gordon, J. C., Rankin, J. W., and Daley, M. A. (2015). How do treadmill speed and terrain visibility influence neuromuscular control of guinea fowl locomotion? J. Exp. Biol. 218, 3010–3022. doi: 10.1242/jeb.104646
Gu, K., Chen, J., and Kharitonov, V. L. (2003). Stability of Time-Delay Systems. Boston, MA: Springer Science & Business Media.
Haverkamp, B. (2000). Subspace Method Identification, Theory and Practice. Ph.D. thesis, TU Delft, Delft.
Haverkamp, B., and Verhaegen, M. (1997). SMI Toolbox: State Space Model Identification Software for Multivariable Dynamical Systems. Delft University of Technology, Delft.
Ho, S., and O'Donovan, M. J. (1993). Regionalization and intersegmental coordination of rhythm-generating networks in the spinal cord of the chick embryo. J. Neurosci. 13, 1354–1371.
Jacobson, R. D., and Hollyday, M. (1982). Electrically evoked walking and fictive locomotion in the chick. J. Neurophysiol. 48, 257–270.
Jalaleddini, K., Niu, C. M., Raja, S. C., Sohn, W. J., Loeb, G. E., Sanger, T. D., et al. (2017a). Neuromorphic meets neuromechanics, part II: the role of fusimotor drive. J. Neural Eng. 14:025002. doi: 10.1088/1741-2552/aa59bd
Jalaleddini, K., Tehrani, E. S., and Kearney, R. E. (2017b). A subspace approach to the structural decomposition and identification of ankle joint dynamic stiffness. IEEE Trans. Biomed. Eng. 64, 1357–1368.
Kalman, R. E. (1960). A new approach to linear filtering and prediction problems. J. Basic Eng. 82, 35–45.
Lawrence, E. L., Cesar, G. M., Bromfield, M. R., Peterson, R., Valero-Cuevas, F. J., and Sigward, S. M. (2015a). Strength, multijoint coordination, and sensorimotor processing are independent contributors to overall balance ability. Biomed. Res. Int. 2015:561243. doi: 10.1155/2015/561243
Lawrence, E. L., Dayanidhi, S., Fassola, I., Requejo, P., Leclercq, C., Winstein, C. J., et al. (2015b). Outcome measures for hand function naturally reveal three latent domains in older adults: strength, coordinated upper extremity function, and sensorimotor processing. Front. Aging Neurosci. 7:108. doi: 10.3389/fnagi.2015.00108
Lipson, H., and Pollack, J. B. (2000). Automatic design and manufacture of robotic lifeforms. Nature 406, 974–978. doi: 10.1038/35023115
Loeb, G. E. (2012). Optimal isnt good enough. Biol. Cybernet. 106, 757–765. doi: 10.1007/s00422-012-0514-6
Martins, F. V., Carrano, E. G., Wanner, E. F., Takahashi, R. H., and Mateus, G. R. (2009). “A dynamic multiobjective hybrid approach for designing wireless sensor networks,” in IEEE Congress on Evolutionary Computation, 1145–1152.
Maurice, M., Gioanni, H., and Abourachid, A. (2006). Influence of the behavioural context on the optocollic reflex (OCR) in pigeons (Columba livia). J. Exp. Biol. 209, 292–301. doi: 10.1242/jeb.02005
McArthur, K. L., and Dickman, J. D. (2011). State-dependent sensorimotor processing: gaze and posture stability during simulated flight in birds. J. Neurophysiol. 105, 1689–1700. doi: 10.1152/jn.00981.2010
Necker, R. (2005). The structure and development of avian lumbosacral specializations of the vertebral canal and the spinal cord with special reference to a possible function as a sense organ of equilibrium. Anat. Embryol. 210, 59–74. doi: 10.1007/s00429-005-0016-6
Necker, R. (2006). Specializations in the lumbosacral vertebral canal and spinal cord of birds: evidence of a function as a sense organ which is involved in the control of walking. J. Comp. Physiol. A 192, 439–438. doi: 10.1007/s00359-006-0105-x
Necker, R. (2007). Head-bobbing of walking birds. J. Comp. Physiol. A 193, 1177–1183. doi: 10.1007/s00359-007-0281-3
Pete, A. E., Kress, D., Dimitrov, M. A., and Lentink, D. (2015). The role of passive avian head stabilization in flapping flight. J. R. Soc. Interface 12:20150508. doi: 10.1098/rsif.2015.0508
Schroeder, D., and Murray, R. (1987). Specializations within the lumbosacral spinal cord of the pigeon. J. Morphol. 194, 41–53.
Sholomenko, G. N., and Steeves, J. D. (1987). Effects of selective spinal cord lesions on hind limb locomotion in birds. Exp. Neurol. 95, 403–418.
Simon, D. (2006). Optimal State Estimation: Kalman, H Infinity, and Nonlinear Approaches. New Jersey, NJ: John Wiley & Sons.
Sinkjær, T., Andersen, J. B., Nielsen, J. F., and Hansen, H. J. (1999). Soleus long-latency stretch reflexes during walking in healthy and spastic humans. Clin. Neurophysiol. 110, 951–959.
Streeter, G. L. (1904). The structure of the spinal cord of the ostrich. Developmental Dynamics, 3, 1–27.
Valero-Cuevas, F. J., Yi, J.-W., Brown, D., McNamara, R. V., Paul, C., and Lipson, H. (2007). The tendon network of the fingers performs anatomical computation at a macroscopic scale. IEEE Trans. Biomed. Eng. 54, 1161–1166. doi: 10.1109/TBME.2006.889200
Verhaegen, M., and Dewilde, P. (1992). Subspace model identification part 1. The output-error state-space model identification class of algorithms. Int. J. Control 56, 1187–1210.
Verhaegen, M., and Verdult, V. (2007). Filtering and System Identification: A Least Squares Approach. Cambridge: Cambridge University Press.
Vukobratovic, M., Borovac, B., Surla, D., and Stokic, D. (2012). Biped Locomotion: Dynamics, Stability, Control and Application, Vol. 7. Berlin; Heidelberg: Springer Science & Business Media.
Westwick, D. T., and Perreault, E. J. (2011). Closed-loop identification: application to the estimation of limb impedance in a compliant environment. IEEE Trans. Biomed. Eng. 58, 521–530. doi: 10.1109/TBME.2010.2096424
Keywords: balance, lumbosacral organ, vestibular system, birds, perch, compliant robot, co-localized sensing, distributed sensing
Citation: Urbina-Meléndez D, Jalaleddini K, Daley MA and Valero-Cuevas FJ (2018) A Physical Model Suggests That Hip-Localized Balance Sense in Birds Improves State Estimation in Perching: Implications for Bipedal Robots. Front. Robot. AI 5:38. doi: 10.3389/frobt.2018.00038
Received: 23 December 2017; Accepted: 19 March 2018;
Published: 04 April 2018.
Edited by:
Stéphane Viollet, Aix-Marseille Université, FranceReviewed by:
Anick Abourachid, National Museum of Natural History, FrancePierre Moretto, Université de Toulouse, France
Copyright © 2018 Urbina-Meléndez, Jalaleddini, Daley and Valero-Cuevas. This is an open-access article distributed under the terms of the Creative Commons Attribution License (CC BY). The use, distribution or reproduction in other forums is permitted, provided the original author(s) and the copyright owner are credited and that the original publication in this journal is cited, in accordance with accepted academic practice. No use, distribution or reproduction is permitted which does not comply with these terms.
*Correspondence: Francisco J. Valero-Cuevas, valero@usc.edu
†These authors have contributed equally to this work.