Bio-Hydrogen Production From Buffalo Waste With Rumen Inoculum and Metagenomic Characterization of Bacterial and Archaeal Community
- CREA Research Center for Animal Production and Aquaculture, Monterotondo, Italy
Biogas production from agricultural and industrial wastes delivers two benefits: on one side, the treatment of organic residues prevents the environmental and economic impact of their disposal; on the other side, methane and/or hydrogen are generated. The aims of this study were both to produce bio-hydrogen from buffalo wastes and to investigate the relationship between biogas production and bacterial and archaeal community composition. Anaerobic codigestion of livestock by-products (buffalo sludge and low protein cheese whey-scotta), with buffalo rumen and buffalo sludge as inoculum, was performed. The microbial community was analyzed using next-generation sequencing of 16S rRNA gene amplicons. Codigestion showed to be positive because of both sludge buffering capability and highly degradable carbohydrates content in scotta. Rumen inoculum proved more efficient compared to sludge during fermentation. In fact, cumulated production was higher (120.8 vs. 65.4 ml H2 g VS−1 respectively) and the average percentage of hydrogen in biogas was 48.1 (v/v) with maximum peak at 64.6. Moreover, rumen bacterial profile showed higher genera richness. Taxonomic classification showed that among the bacteria, Firmicutes, 23.3% of whom Clostridia; Bacteroidetes, and in particular Bacteroidia; Proteobacteria and Tenericutes, accounted for 88.2% of total sequences. Concerning the Clostridia Family XIII, the C. Incertae Sedis was the most represented (6.6%), and its quantity was twice as much in rumen inoculated hydrogen-producing samples than those non-producing. In the archaeal, community predominated the phylum Euryarcheota, with Methanobrevibacter the most represented, which was higher when hydrogen was produced with rumen inoculum. Studies on buffalo rumen as inoculum for hydrogen production are limited and this paper gives a first overview of microbial community composition by NGS in producing and non-producing samples.
Introduction
Biogas production by anaerobic digestion (AD) process from agricultural and industrial wastes delivers two benefits: on one side, the treatment of organic residues prevents the environmental and economic impact of their disposal; on the other side, energy carriers (methane and/or hydrogen) are generated. Current interest in the use of hydrogen as fuel, both for industrial applications and for road haulage, is because it has a high-energy density (122–142 kJ/g) and produced pollution is almost nil (Antonopoulou et al., 2008; Venetsaneas et al., 2009). Infact, hydrogen only produces water and traces of N oxides, when used in combustion systems, while producing only water, when used with electrochemical systems (fuel cells). Bio-hydrogen production is depending on various elements such as feedstocks composition, microbial inocula, pretreatments, pH, temperature (Hawkes et al., 2002; Kim et al., 2006; Karadag and Puhakka, 2010; Karadag et al., 2014).
Considerable amount of cheese whey (CW), around 145 mil ton/year (Macwan et al., 2016) are produced worldwide deriving from cheese-making process. CW is rich in lactose and minerals, along with a chemical oxygen demand (COD) concentration up to 80 g L−1, therefore, it was considered an valid ingredient for bioenergy production (Sansonetti et al., 2009; Hublin and Zelic, 2013; Perna et al., 2013; Karadag et al., 2014). In Italy, a large amount of CW is produced yearly, also deriving from buffalo mozzarella cheese (4th DOP cheese as production), together with “scotta”, a deproteinized by-product, obtained after the production of both cheese and “ricotta” (similar to cottage cheese product, widespread in Anglo-saxon country) (Vasmara and Marchetti, 2017). The scotta has still a high organic load, environmentally pollutant if improperly managed, so cheese manufacturers would be extremely interested to make “scotta” an economic value by-product, also avoiding costly disposal solutions.
Nevertheless, some difficulties in AD of CW have been reported, such as low alkalinity content, rapid acidification and subsequent reactor failure (Ergüder et al., 2001). To address such difficulties, the codigestion with dairy manure has proven effective (Kavacik and Topaloglu, 2010), because of its high suspended solids content and fibrous material, sufficient alkalinity level, and higher buffer capability, so representing a complementary combination with CW (Rico et al., 2015).
AD processes for biogas production are divided in four major phases: hydrolysis, acidogenesis, acetogenesis, and methanogenesis (Batstone et al., 2002; Thauer et al., 2008; Angelidaki et al., 2011). Different bacterial groups accomplish the first three, but methanogenesis is exclusively performed by methanogenic Archaea. So microbial consortia belonging to different environments, such as marine and fresh water sediments, soils, and animal gut, can produce biogas, but they their taxonomic abundance and diversity relies on physical and chemical conditions in addition to substrate availability and kind (Gudelj et al., 2010; Kittelmann et al., 2013; Tapio et al., 2017). According to Johnson et al. (2012), “metabolic specialization provides one plausible explanation for how diversity could be promoted, and is therefore a likely general organizing principle that shapes the assembly of microbial communities.”
The potential of application of rumen microorganisms as inocula has been explored so far, mainly for the conversion of lignocellulosic biomass (Hu and Yu, 2005; Sutherland and Varela, 2014; Sawatdeenarunat et al., 2015; Li et al., 2017). Rumen is a well-adapted microbial community, on which ruminants rely on to convert feed into energy-yielding products, such as VFAs, used by the host as an energy source. Rumen contains two groups of prokaryotes (bacteria, dominated by the phyla Firmicutes and Bacteroidetes, and Archaea) and two groups of eukaryotes (protists and fungi) and theirs strong metabolic interactions characterize its environment. Microbial community in the rumen demonstrates according to Weimer (2015), both redundancy (overlap of physiological capabilities across species) and resilience (resistence to, capacity to spring back to shape after perturbation).This flexibility and consequent resilience would make it suitable for application to different industrial contexts such as energy production (Henderson et al., 2015; Weimer and Kohn, 2016).
Although complexity of microbial communities have been studied for a long time, recent advances in metagenomic approach by next generation sequencing (NGS) offer a new tools because of both their molecular detail and their accessibility to a broad scientific community, supplementing culture-based approaches (Schmidt et al., 1991; Tringe and Hugenholtz, 2008). Metagenomic studies have explored a number of environments, including cow rumen (Li et al., 2017; Tapio et al., 2017), human intestinal tract (Qin et al., 2010) and oral cavity (Dewhirst et al., 2010), marine (Venter et al., 2004), freshwater (Roux et al., 2012), soil (De Angelis et al., 2010), and air (Tringe et al., 2008). The sequencing of 16S rRNA gene is the most efficient approach to assess the phylogeny and diversity of a community, especially if the studied environment contains a large fraction of uncharacterized microorganisms.
The laboratory trial reported in this paper was preparatory to the application in a two-stage pilot plant for combined production of hydrogen and methane operating with liquid and semiliquid feedstocks (CREA-ENEA Italian patent n° 1416926). The aims of this study were both to produce bio-hydrogen from buffalo wastes (sludge—a mix of manure and urine—and scotta) and to investigate the bacterial and archaeal community structure related to gas production.
The first step was to verify if buffalo rumen fluid (BU) were a better inoculum than buffalo sludge itself (BS) to be used in bio-hydrogen production and if scotta could be a valid substitute for raw CW as well as sludge for manure. The microbial community (bacterial and archaeal) was analyzed using NGS of 16S rRNA gene amplicons, to identify core groups and specific microbes involved in the dark fermentation of these buffalo wastes.
Materials and Methods
Experimental Design and Animals
Anaerobic codigestion experiments using buffalo by-products (sludge and scotta) inoculated with BU and BS were performed. Feedstocks were fresh BS, a mixture of manure and urine taken from our farm sewage tank, strained (two layers of cheesecloth) before use, and fresh scotta from a local cheese factory used within an hour.
Inocula were fresh BS (the same as feedstock) and buffalo rumen. The latter (1 L) was taken from three cannulated Mediterranean Buffalo cows fitted with rumen cannula (#3C, 100 mm, Bar-Diamond Inc., Parma, USA) before the morning feeding, strained (three layers of cheesecloth) and pooled.
The 60:40 BS/Scotta ratio (for a total of 37 g L−1 Volatile Solids (VS)) was used, pH was adjusted at 7 and temperature maintained at mesophilic condition (39°C) throughout both trials, using inoculum at 15%. The 120 ml batch reactors (70 ml of working volume, in three replicates) were flushed with nitrogen gas to establish anaerobic conditions and maintained in the dark at orbital shaking (40 rpm/min). The trials lasted until the end of hydrogen production (i.e., 12 days).
Because BS inoculum gave very low hydrogen production in first trial (T1), a subsequent trial (T2) was carried out using a matured BS inoculum (BS2), maintained for 3 weeks at room temperature.
Substrate composition is reported in Table S1.
An ethics approval for all experimental procedures of this research was obtained from the Italian Welfare Ministry (DGSAF, 588/2017-PR), in accordance with the guidelines established by the European Community Council Directives 86/609/EEC.
Samples Collection
Samples from every replicate and each sampling time (0, 5, 7, 10, 12 days) were taken for VFAs and lactic acid analysis (0.5 mL) and pH determination. VS (70 mL) were determined at the beginning and end of the experiment. Samples for DNA extraction (1 mL) were taken from each replicates at the beginning of the experiment and at day 7, 10, 12 to compare the two trials.
Chemical Analysis
The metabolic products of fermentation (VFAs, lactic acid) were analyzed through high-performance liquid chromatography (HPLC) (Waters 2695, HPLC and detector Waters 2487) equipped with an UV-vis detector (λ = 220 nm) and a refractive index detector. The analytical column was Aminex 85 HPX-87H (300 × 7.8 mm and 9 μm particle size) of BIO-RAD (California, USA) with a 4 × 30 mm security guard cartridge Carbo-H (Phenomenex, California, USA). Operating conditions were 40°C, under isocratic conditions, using a solution of 0.008N H2SO4 as mobile phase (flow rate, 0.6 ml/min filtered through a 0.45 μm Millipore Teflon membrane and degassed). The liquid samples were diluted 1:1 (v/v) in H2SO4 (0.1N) and filtered with 0.45 μm Teflon membrane before injection of 20 μL into the HPLC. The external standard analytical curves were prepared at three different concentrations for each acid (lactic, acetic, propionic, i-butyric, butyric, i-valeric, and valeric, pure standards from Sigma, USA) and fitted with weighted least-square regression.
Total biogas volume was measured using a water displacement system (Kalia et al., 1994) and cumulated gas production (ml g VS−1) was calculated. Biogas composition (% v/v) in reactors headspace was analyzed using a gas chromatograph (GC, MPS)(Pollution, Bologna, Italy) equipped with a Molsieve column (L10m; i.d. 320 μm; film 30 μm) + pre-column (L 3 m; i.d. 320 μm; film 30 μm) with backflush injector for di H2, CH4,O2, N2 determination.
DNA Extraction and Sequencing
DNA was extracted from 26 samples, including the inocula, 15 from trial T1 (the BS non-producing samples were pooled) and 11 from trial T2. The samples were centrifuged at 5,000 g for 5 min and the pellet re-suspended in 400 μl of PBS (Sigma, USA) before extraction, using Maxwell® 16 tissue DNA purification kit (Promega, Madison, USA), in Maxwell® 16 Instrument according to manufacturer guidelines. The quality and the quantity of DNA was determined using NanoPhotometer™ Pearl (Implen GmbH, München, Germany) and Quantity-one fluorimeter (Promega, Madison, USA). The 16S rRNA gene amplicons were generated with the U341F (CCTAYGGGRBGCASCAG) and U806R (GGACTACNNGGGTATCTAAT) primers targeting the hypervariable V3–V4 regions for both bacteria and archaea. Amplicons from individual samples were pooled at equal molar ratios and purified. About 100 ng of each pool was used to construct sequencing libraries using the Ovation Rapid DR Multiplex System 1-96 (NuGEN). The sequencing libraries were pooled and size selected by preparative gel electrophoresis and directly sequenced at 300 bp paired-end reads by using the Illumina MiSeq V3 sequencer (Illumina, S.Diego, USA) for 2.5 million read pairs.
Statistical Analysis
The chemical data were analyzed using the SAS general linear models procedure (SAS Inst., vers. 9.4; Cary, NC, USA) (SAS Statistical Analysis System Institute, 2012). Least square means (LSM) and pooled standard error of means were obtained.
The analysis of metagenomic data included Krustal-Wallis statistical test (Bonferroni adjusted) on OTU abundance in the different sample groups [initial composition (T0) and producing and non-producing samples (Y,N) according to trial (T1, T2)]. On alpha diversity (Chao1 and Observed species indexes), comparison tests using a two-sided Student's two-sample t-test were undertaken. Beta diversity using Unifrac weighted and unweighted distance metrics was calculated and significance tests were performed using a two-sided Student's two-sample t-test (parametric) and nonparametric p-values, calculated using 999 Monte Carlo permutations to underline statistical differences between the above mentioned groups. A hierarchical clustering was obtained by Unweighted Pair Group Method with Arithmetic mean (UPGMA) (Sneath and Sokal, 1973). Jackknifed beta diversity analyses measured robustness of UPGMA clusters.
Metagenomic Data Analysis
Samples were processed and analyzed with the following procedure: (1) pre-processing of raw sequence reads for quality control (QC). Demultiplexing of all libraries for each sequencing lane using the Illumina bcl2fastq 1.8.4 software; clipping of sequencing adapter from 3′ ends of reads; Combination of forward and reverse reads using BBMerge 34.48; FastQC reports for all FASTQ files; (2) processing of the combined reads for the community analysis. Sequences were filtered using Mothur 1.35.1, aligned against the Mothur-Silva16S SEED r119 reference alignment, and subsampled to 80,000 sequences per each sample. Chimeras sequences were removed with Uchime algorithm and taxonomical classification performed against the Silva reference classification. OTUs were picked at a 97% identity level using the average neighbor method. Both OTU diversity and statistical analyses (rarefaction curves, alpha and beta-diversity estimates) were conducted using the Quantitative Insights Into Microbial Ecology (QIIME 1.9.0) open-source pipeline (Caporaso et al., 2010).
Results and Discussion
Batch Hydrogen Production Performances
In T1 trial, biogas production started from day 5 and day 7 when BS and BU inoculum were employed respectively, as reported in Table 1. This could be due to the presence of a richer Archea population, which used hydrogen to produce methane, in buffalo rumen inoculum (I-BU) compared to BS inoculum (I-BS) samples (Figure 1). As reported in Table 1 cumulated production was higher with BU inoculum than with BS (120.8 vs. 23.61 ml H2 g VS−1, respectively), nevertheless, VS reduction at the end of the trial was similar (11% approximately). The hydrogen percentage obtained on average was 48.1 (v/v) with BU at day 7, against a 23.7 of BS.
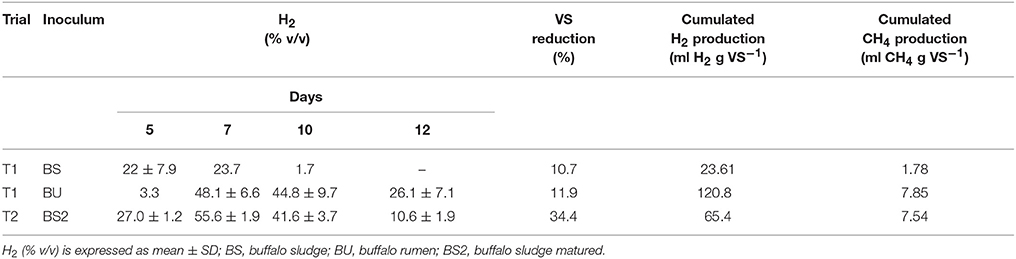
Table 1. H2 average percentage in gas mixture according to sampling times (day 5, 7, 10, and 12), Volatile solids (VS) average reduction, H2 and CH4 cumulated production during trial T1 and T2.
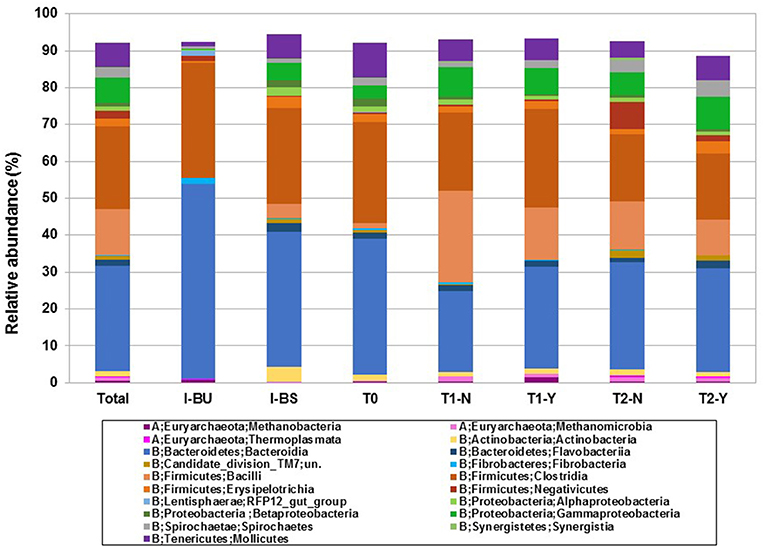
Figure 1. Taxonomic analysis of microbial community at class level according to inocula (I-BU, I-BS), beginning of trial (T0) and hydrogen producing (Y) and non-producing (N) samples in T1 and T2 trials.
In trial T2, the hydrogen production started at day 5 with 27% (v/v in biogas) and increased to 55.6% at day 7 so being higher in BS2 than BU in trial T1, and resulted in 65.4 cumulated production (ml H2 g VS−1). In this case, an inoculum, matured for 3 weeks, was used, confirming that aging can improve performances (Kavacik and Topaloglu, 2010; Di Cristofaro et al., 2014). The hydrogen percentage in biogas was higher in T2 trial compared to T1 (for BU samples) and both results were interesting if compared to what reported in literature from livestock manure and wastewater (Tang et al., 2008; Xing et al., 2010; Di Cristofaro et al., 2014) but nonetheless BU inoculum gave the highest overall yield. This could be due to the higher content of total VFAs (46.2 vs. 44.7 g L−1, T1, and T2 respectively), and particularly acetic acid (P < 0.01, data shown in Tables 2, 3) which suggest a more efficient fermentation process.
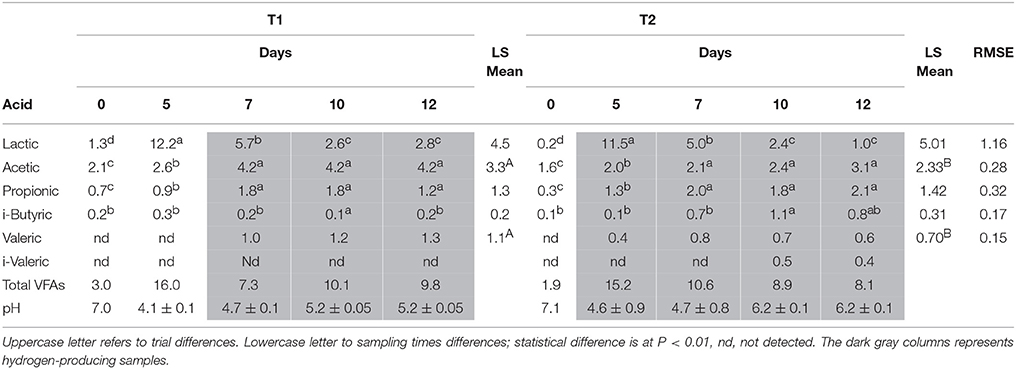
Table 2. Statistical analysis of VFAs and lactic acid concentration (g L−1) during trial T1 and T2 according to sampling times (day 0, 5, 7, 10, and 12).
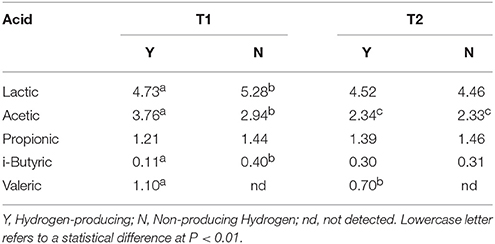
Table 3. Statistical analysis of VFAs and lactic acid concentration (g L−1) according to hydrogen-producing or non-producing samples in trial T1 (BU inoculum) and T2 (BS2 inoculum).
In Table 2, pH and VFAs values, measured during trials T1 and T2, are reported. Only BU (T1) and BS2 (T2) inocula were compared, because hydrogen production from BS was too low. The accumulation of VFAs and especially lactic acid, during the first 5 days, caused an intense reduction of pH, which dropped to 4.1 in T1 and to 4.6 in T2. Nevertheless, the buffering capability of buffalo sludge as feedstock allowed the increase and maintenance of pH at an optimum range (5–6) throughout the fermentation process as reported also by different authors (Antonopoulou et al., 2008; Weiland, 2010; Tenca et al., 2011). The rapid increase in lactic acid during the first 5 days (1.33 to 12.16 in T1 and 0.25 to 11.49 g L−1 in T2, P < 0.01), due to the scotta used as feedstock (Ergüder et al., 2001; Rico et al., 2015), showed statistically significant differences between sampling times but not between trials. As soon as lactic acid concentration started to decrease, hydrogen production began. Acetic acid concentration was the highest among all VFAs, and revealed a significant difference between trials (3.35 vs. 2.33 g L−1 on average for T1 and T2 respectively, P < 0.01). This is in agreement both with Vasmara and Marchetti (2017) results obtained using scotta permeate and Li et al. (2017) data using rumen fluid inoculated reactors for methane production. Propionic acid concentration showed no statistical difference between trials but within the trials. Butyric acid concentration showed a statistical difference only between day 10 and the other sampling times in T1 trial and between day 10 and 12 in T2. Valeric acid was detectable only when hydrogen was produced and the quantity was significantly higher in T1 compared to T2 (1.1 vs. 0.7 g L−1 on average, P < 0.01). i-Valeric was detectable in T2 day 10 and 12 samples. Hu and Yu (2005) using rumen microbes for biogas production reported that “by inhibiting methane formation, a potential hydrogen sink was eliminated, and the reducing power was used to produce higher carboxylic acids. This might be responsible for the production of longer chain fatty acids, such as valerate.” This observation is in agreement with our hypothesis about a more efficient fermentation in BU samples, which resulted in a higher hydrogen production, some of which could be transformed in valeric acid.
In Table 3 the results of the statistical analysis between producing (Y) and non-producing samples (N) in trial T1 (buffalo inoculated) and T2 (BS2 inoculated) are reported. Lactic acid samples were different only in T1 trial, where N samples had the highest value because included day 5 samples (12.2 g L−1, Table 3).
We hypothesized the day 5 samples in T1 did not produce hydrogen, because it included a high number of methanogens, which consumed H2 to produce methane (data reported in Table 1). Acetic acid concentration between Y and N in T1 was also statistically significant, and BU inoculum produced 62% more than BS2 during hydrogen production (3.76 vs. 2.34 g L−1 on average, P < 0.01). i-butyric acid concentration was statistically different in T1 (0.11 vs. 0.40 g L−1, Y, and N respectively) where the quantity decreased when hydrogen production started. It is interesting to note that valeric acid is detectable only when hydrogen is produced and the quantity is significantly higher in T1 samples.
Microbial Community Analysis and Composition
After the pre-processing, 2,003,102 combined reads were retained for the following analysis. Sequences were deposited in the EMBL-EBI (European Nucloeotide Archive) under the study accession PRJEB25602. The 16S rDNA amplicon sequencing depth of the 26 analyzed samples ranged from 31,826 to 140,676 paired reads. Singleton OTUs were removed and the data from each sample were rarefied at 19,769 to keep as many samples as possible. 10,779 OTUs were obtained from all the samples. Krustal-Wallis test did not show any statistically significant differences between the OTUs abundance in the different sample groups.
The first step of our analysis was to obtain a general description of the microbial community composition found in all samples. The majority of the sequences were members of 4 phyla: Firmicutes (39.73%), and in particular Clostridia (23.31%) and Bacilli (12.06%); Bacteroidetes (31.98%), 28.80% of whom Bacteroidia; Proteobacteria (9.92%) and Tenericutes (6.59%), which all together accounted for 88.22% as reported in Table S2. Aboundance of minor bacterial phyla (Actinobacteria, Spirochaetae, Flavobacteria) were below 3%.
The first three main phyla are reported as part of a core group by numerous authors (Abendroth et al., 2015; Bassani et al., 2015; Duda et al., 2015; Goux et al., 2015). In AD of cattle manure and sludge, some authors (Rivière et al., 2009; St-Pierre and Wright, 2014; Campanaro et al., 2016) reported, as part of the core group, also Cloroflexi, which, on the contrary, we found at 0.03% only. Maus et al. (2017) also reported Firmicutes, Bacteroidetes as the main bacteria phyla in mesophyllic conditions plants with cow manure as inoculum. The Firmicutes are important because they are implicated in several metabolic processes including carbohydrates degradation, fatty acids utilization, Wood–Ljungdahl pathway (WLP) (homoacetogenesis) or syntrophic acetate oxidation (SAO) as reported by Campanaro et al. (2016).
Six orders have been found, among Firmicutes: Bacillales (5 families, 20 genera), Lactobacillales (5 families, 18 genera), Clostridiales (18 families, 88 genera), Erysipelotrichiales (1 family, 9 genera), Selemononadales (2 families, 17 genera), OPB54 (1 family, 1 genus).
The Bacteroidetes which are known to be proteolytic bacteria, were the second most abundant phylum, including Bacteroidales (20 families, 41 genera), Cytophagales (3 families, 9 genera), Order III (2 families, 2 genera), Flavobacteriales (3 families, 24 genera), Sphingobacteriales (7 families, 16 genera).
The Proteobacteria included Alphaproteobacteria (1.15%), Betaproteobacteria (0.92%), Deltaproteobacteria (0.41%), Epsilonbacteria (0.5%) and Gammaproteobacteria (6.84%). The Tenericutes include Mollicutes and in particular Acholeplasmatales (5.70%).
In a second step we were focused on the analysis of microbiological community structure in hydrogen producing (Y) and non-producing groups (N) both in trial T1 and T2. In Figure 1 we report the taxonomic analysis at Class level, taking into account frequency above 0.3%, according to: I-BU and I-BS inocula, T0 (beginning of trial) and T1 and T2, Y, and N samples.
The Firmicutes were higher in N samples, in both trial, in particular Bacilli and Negativicutes. Bacilli numbers (97% of which are Lactobacillales) increased markedly from 1.3% in T0 group to 24.9% in T1-N, in accordance to lactic acid increase that we previously discussed (see Table 2). Whitin the trials they decreased in Y samples (24.9% to 14.1% in T1-N and T1-Y and 13.0% to 9.5% in T2-N and T2-Y, respectively) (see Table S3). This was favored by pH increase due to sludge buffering capacity. Moreover, some researchers observed that the excretion of bacteriocins by Lactobacilli and Prevotella resulted in inhibitory effects of hydrogen production (Castelló et al., 2011). Negativicutes (7.3%), Candidate_division_TM7 (2.2%), and Synergistia (0.8%) are more abundant in T2 and in particular N samples, even though the latter are reported to ferment amino acids to acetate and propionate together with other species (Campanaro et al., 2016).
On the contrary, Clostridia were higher in T1 hydrogen producing samples (see Figure 1 and Table S3) as reported in literature (Davila Vazquez et al., 2009; Cheng et al., 2011; Maus et al., 2017). Among the Clostridia, Family XIII, the C. Incertae Sedis was the most represented (6.5% in our trial), in particular in T1-Y, the quantity was twice as much as T1-N; in T2 trial we observed the opposite trend. Matsumoto et al. (2017) reported a positive correlation between the Incertae Sedis bacteria and hydrogen production in human gut.
Clostridium Senso Stricto 1 (1.7%), among Clostridiaceae_1, reported by Maus et al. (2017) as the most represented, in our trial was the second more abundant genus and we observed higher quantity in T1-N than T1-Y, and lower quantity in T2-N than T2-Y.
Among Bacteroidales the most represented was Prevotella (4.2% on average), particularly high in T2-N (8.8%), nevertheless we observed three fold more bacteria in T1-Y samples (5.9%) compared to T1-N (1.7%). On the contrary, RC9 gut group (2.1%) was more abundant in T1 trial compared to T2, and in Y samples compared to N. BS11 gut group was present in T1 only, which had buffalo rumen inoculum, and in higher quantity in hydrogen-producing samples.
The alpha diversity analysis between groups (initial composition (T0) and producing and non-producing samples (Y,N) according to trial (T1, T2)) using Chao1 index is reported as box-plot in Figure 2. A statistically significant difference (P < 0.05) was revealed between groups considering T0 vs. T2-Y, T1-Y vs. T2-N, T1-Y vs. T2-Y. The higher species richness observed in T1 vs. T2 and T0 group, are probably due to the presence of rumen inoculum, to be noticed that its richness is not evident in Figure 1, where only frequencies above 0.3% were included.
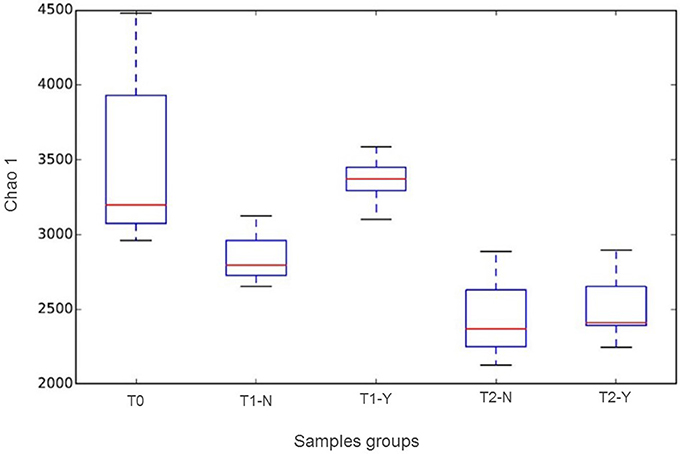
Figure 2. Chao 1 boxplots according to beginning of trial (T0) and hydrogen producing (Y) and non-producing (N) samples in T1 and T2 trials.
Statistical significant difference according to beta diversity was obtained only between T1-Y Vs T2-Y with parametric test (data not shown). The result of beta diversity between all samples calculated as hierarchical clustering by UPGMA is presented in Figure 3. The Figure shows clearly the clustering of the BU inoculated samples separated from the BS samples with a good jackknife support (> 75%). Moreover the BU inoculum is separated from all the other samples.
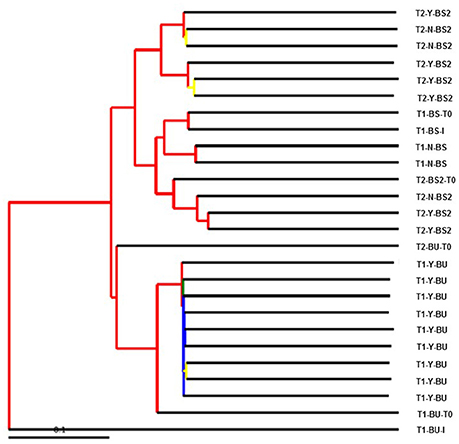
Figure 3. A visualization of bootstrap-supported hierarchical clustering (UPGMA) of the 26 sample's microbial communities. Internal tree nodes color represent jackknifed support (red for 75–100%, yellow for 50–75%, green for 25–50%, and blue < 25%).
All the Archea identified species obtained in this study were attributed predominantly to phylum Euryarcheota (1.74%) as reported in Table S2. Considering the groups analysis, Euryarcheota included 5 orders: Halobacteriales, Methanobacteriales, Methanomicrobiales, Methanosarcinales, and Thermoplasmatales as found in previous studies (Rivière et al., 2009; Sun et al., 2015). The most abundant were Methanocorpusculaceae (0.5%) with predominance of genus Methanocorpusculum, and Methanobacteriaceae (0.5%) with three genera Methanobacterium, Methanosphaera, and Methanobrevibacter (Table S3). The latter was particularly higher when hydrogen was produced with rumen inoculum (1.5%, Table S3). As reported in bovine rumen there is a prevalence of this hydrogenotrophic Archaea, which uses H2-CO2 as substrate to produce methane (Li et al., 2017).
The Methanomicrobiales included 5 families The Methanosarcinales were represented by Metanosarcinaceae and in particular Methanimicrococcus (0.4%) and Thermoplasmatales by family Incertae Sedis and genus Candidatus Methanomethylophilus (0.2%).
Conclusions
This paper deals with the bio-hydrogen production from livestock by-products (buffalo sludge and scotta) with buffalo rumen and buffalo sludge as inocula as well as the microbial community composition. Codigestion was positive due both to sludge buffering capability and scotta's content in high degradable carbohydrates. Buffalo rumen has a number of appealing features as lignocellulose degradation and community resilience, which could be exploited in industrial application such as gas production. It proved more efficient compared to buffalo sludge as inoculum when hydrogen cumulated production is concerned, showing also higher VFAs production, particularly acetic acid and confirming bacterial species richness. However, the maturation of BS inoculum, allowed to improve its performances.
The majority of the bacterial identified genera belonged to Firmicutes—in particular Clostridia—, Bacteroidetes, Proteobacteria, and Tenericutes. Clostridia were higher in hydrogen producing samples confirming what is generally reported in literature. In our study the Family XIII, C. Incertae Sedis was the most represented when rumen inoculated samples were tested.
Most of the archaeal sequences belonged to Euryarcheota and the most abundant was Methanobrevibacter, particularly when hydrogen is produced with buffalo inoculum.
Studies on buffalo rumen employed as inoculum for hydrogen production are limited and this paper gives a first overview of the microbial community structure in dark fermentation processes by next generation sequencing.
Author Contributions
AnC, contributed to the study conception and design of the study and performed the dark fermentation analysis; AlC contributed to the metagenomic analysis. Both authors have contributed to the analysis and interpretation of data, drafting of manuscript as well as critical revision. Both authors have read and approved the submitted version.
Conflict of Interest Statement
The authors declare that the research was conducted in the absence of any commercial or financial relationships that could be construed as a potential conflict of interest.
The reviewer BG and handling Editor declared their shared affiliation.
Acknowledgments
This work was supported by the Italian Ministry of Agriculture (MiPAAF) under the AGROENER project (D.D. n. 26329, 1 April 2016) - http://agroener.crea.gov.it/. We are also grateful to farm workers for animal care and Emanuela Rossi for literature review.
Supplementary Material
The Supplementary Material for this article can be found online at: https://www.frontiersin.org/articles/10.3389/fsufs.2018.00013/full#supplementary-material
Table S1. Substrate chemical composition.
Table S2. Taxonomy-binned sequences counts per samples. The levels correspond to taxonomic classification (from Phylum to Class).
Table S3. Bacterial and archaeal taxa abundances according to beginning of trial (T0) and T1 and T2 trials, hydrogen producing (Y) and non-producing (N) samples. The levels correspond to taxonomic classification (from Phylum to Genus)
References
Abendroth, C., Vilanova, C., Günther, T., Luschnig, O., and Porcar, M. (2015). Eubacteria and archaea communities in seven mesophilic anaerobic digester plants in Germany. Biotechnol. Biofuels 8:87. doi: 10.1186/s13068-015-0271-6
Angelidaki, I., Karakashev, D., Batstone, D. J., Plugge, C. M., and Stams, A. J. (2011). Biomethanation and its potential. Meth. Enzymol. 494, 327–335. doi: 10.1016/B978-0-12-385112-3.00016-0
Antonopoulou, G., Gavala, H. N., Skiadas, I. V., Angelopoulos, K., and Lyberatos, G. (2008). Biofuels generation from sweet sorghum: fermentative hydrogen production and anaerobic digestion of the remaining biomass. Bioresour. Technol. 99, 110–119. doi: 10.1016/j.biortech.2006.11.048
Bassani, I., Kougias, P. G., Treu, L., and Angelidaki, I. (2015). Biogas upgrading via hydrogenotrophic methanogenesis in two-stage continuous stirred tank reactors at mesophilic and thermophilic conditions. Environ. Sci. Technol. 49, 12585–12593. doi: 10.1021/acs.est.5b03451
Batstone, D. J., Keller, J., Angelidaki, I., Kalyuzhnyi, S. V., Pavlostathis, S. G., Rozzi, A., et al. (2002). The IWA anaerobic digestion model No 1 (ADM1). Water Sci. Technol. 45, 65–73.
Campanaro, S., Treu, L., Kougias, P. G., De Francisci, D., Valle, G., and Angelidaki, I. (2016). Metagenomic analysis and functional characterization of the biogas microbiome using high throughput shotgun sequencing and a novel binning strategy. Biotechnol. Biofuels 9:26. doi: 10.1186/s13068-016-0441-1
Caporaso, J. G., Kuczynski, J., Stombaugh, J., Bittinger, K., Bushman, F. D., Costello, E. K., et al. (2010). QIIME allows analysis of high-throughput community sequencing data. Nat. Methods 7, 335–336. doi: 10.1038/nmeth.f.303
Castelló, E., Perna, V., Wenzel, J., Borzacconi, L., and Etchebehere, C. (2011). Microbial community composition and reactor performance during hydrogen production in a UASB reactor fed with raw cheese whey inoculated with compost. Water Sci. Technol. 64, 2265–2273. doi: 10.2166/wst.2011.706
Cheng, C.-H., Hsu, S.-H., Wu, C.-H., Chang, P.-W., and Hung, C.-H. (2011). Quantitative analysis of microorganism composition in a pilot-scale fermentative biohydrogen production system. I. J. Hydrog. Energy. 36, 14153–14161. doi: 10.1016/j.ijhydene.2011.05.023
Davila Vazquez, G., Cota-Navarro, C. B., de León-Rodríguez, A., and Razo-Floresa, E. (2009). Continuous biohydrogen production using cheese whey: improving the hydrogen production rate. I. J. Hydrog. Energy 34, 4296–4304. doi: 10.1016/j.ijhydene.2009.02.063
De Angelis, K. M., Gladden, J. M., and Allgaier, M. (2010). Strategies for enhancing the effectiveness of metagenomic-based enzyme discovery in lignocellulolytic microbial communities. Bio Energy Res. 3, 146–158. doi: 10.1007/s12155-010-9089-z
Dewhirst, F. E., Chen, T., Izard, J., Paster, B. J., Tanner, A. C., Yu, W. H., et al. (2010). The human oral microbiome. J. Bacteriol. 192, 5002–5017. doi: 10.1128/JB.00542-10
Di Cristofaro, F., Carotenuto, C., Carillo, P., Wooddrow, P., Morrone, B., and Minale, M. (2014). Evaluation of CH4 and H2 Yield with different mixture of digested and fresh buffalo manure. Chem. Eng. Trans. 37, 283–288. doi: 10.3303/CET1437048
Duda, R. M., da Silva Vantini, J., Martins, S. L., de Mello Varani, A., Lemons, M. V. F., Ferro, M. I. T., et al. (2015). A balanced microbiota efficiently produces methane in a novel high-rate horizontal anaerobic reactor for the treatment of swine wastewater. Biores. Technol. 197, 152–160. doi: 10.1016/j.biortech.2015.08.004
Ergüder, T., Tezel, U., Güven, E., and Demirer, G. N. (2001). Anaerobic biotransformation and methane generation potential of cheese whey in batch and UASB reactors. Waste Manag. 21, 643–650. doi: 10.1016/S0956-053X(00)00114-8
Goux, X., Calusinska, M., Lemaigre, S., Marynowska, M., Klocke, M., Udelhoven, T., et al. (2015). Microbial community dynamics in replicate anaerobic digester exposed sequentially to increasing organic loading rate, acidosis and process recovery. Biotechnol. Biofuels 8:122. doi: 10.1186/s13068-015-0309-9
Gudelj, I., Weitz, J. S., Ferenci, T., Horner-Devine, M. C., Marx, C. J., Meyer, J. R., et al. (2010). An integrative approach to understanding microbial diversity: from intracellular mechanisms to community structure. Ecol. Lett. 13, 1073–1084. doi: 10.1111/j.1461-0248.2010.01507.x
Hawkes, F. R., Dinsdale, R., Hawkes, D. L., and Hussy, L. (2002). Sustainable fermentative hydrogen production: challenges for process optimization. Int. J. Hydrogen Energy 27, 1339–1347. doi: 10.1016/S0360-3199(02)00090-3
Henderson, G., Cox, F., Ganesh, S., Jonker, A., Young, W., et al. (2015). Rumen community composition varies with diet and host, but a core microbiome is found across a wide geographic range. Sci. Reports 5:14567. doi: 10.1038/srep14567
Hu, Z. H., and Yu, H. Q. (2005). Application of rumen microorganisms for enhanced anaerobic fermentation of corn stover. Process Biochem. 40, 2371–2377 doi: 10.1016/j.procbio.2004.09.021
Hublin, A., and Zelic, B. (2013). Modelling of the whey and cow manure co-digestion process. Waste Manag. Res. 3, 353–360. doi: 10.1177/0734242X12455088
Johnson, D. R., Goldschmidt, F., Lilja, E. E., and Ackermann, M. (2012). Metabolic specialization and the assembly of microbial communities. ISME 6, 1985–1991. doi: 10.1038/ismej.2012.46
Kalia, V. C., Jain, S. R., Kumar, A., and Joshi, A. P. (1994). Fermentation of biowaste to H2 by Bacillus licheniformis. World J. Microbiol. Biotechnol. 10, 224–227. doi: 10.1007/BF00360893
Karadag, D., Köroglu, O. E., Ozkaya, B., Cakmakci, M., Heaven, S., and Banks, C. (2014). A review on fermentative hydrogen production from dairy industry wastewater. J. Chem. Technol. Biotechnol. 89, 1627–1636. doi: 10.1002/jctb.4490
Karadag, D., and Puhakka, J. A. (2010). Effect of changing temperature on anaerobic hydrogen production and microbial community composition in an open-mixed culture bioreactor. Int. J. Hydr. Ener. 35, 10954–10959. doi: 10.1016/j.ijhydene.2010.07.070
Kavacik, B., and Topaloglu, B. (2010). Biogas production from co-digestion of a mixture of cheese whey and dairy manure. Biomass Bioenergy 34, 1321–1329. doi: 10.1016/j.biombioe.2010.04.006
Kim, S. H., Han, S. H., and Shin, H. S. (2006). Effect of substrate concentration on hydrogen production and 16S rDNA-based analysis of the microbial community in a continuous fermenter. Process Biochem. 41, 199–207. doi: 10.1016/j.procbio.2005.06.013
Kittelmann, S., Seedorf, H., Walters, W. A., Clemente, J. C., Knight, R., Gordon, J. I., et al. (2013). Simultaneous amplicon sequencing to explore co-occurrence patterns of bacterial, archaeal and eukaryotic microorganisms in rumen microbial communities. PLoS ONE 8:e47879. doi: 10.1371/journal.pone.0047879
Li, K., Zhu, H., Zhang, Y., and Zhang, H. (2017). Characterization of the microbial communities in rumen fluid inoculated reactors for the biogas digestion of wheat straw. Sustainability 9:243. doi: 10.3390/su9020243
Macwan, S. R., Dabhi, B. K., Parmar, S. C., and Aparnathi, K. D. (2016). Whey and its utilization. Int. J. Curr. Microbiol. App. Sci. 5, 134–155. doi: 10.20546/ijcmas.2016.508.016
Matsumoto, M., Fujita, A., Yamashita, A., Kameoka, S., Shimomura, Y., Kitada, Y., et al. (2017). Effects of functional milk containing galacto-oligosaccharide, maltitol and glucomannan on the production of hydrogen gas in the human intestine. J. Func. Foods 35, 13–23. doi: 10.1016/j.jff.2017.05.013
Maus, I., Kim, Y. S., Wibberg, D., Stolze, Y., Off, S., Antonczyk, S., et al. (2017). Biphasic study to characterize agricultural biogas plants by high-throughput 16S rRNA gene amplicon sequencing and microscopic analysis. J. Microbiol. Biotechnol. 27, 321–334. doi: 10.4014/jmb.1605.05083
Perna, V., Castello, E., Wenzel, J., Zampol, C., Lima, D. M. F., Borzacconi, L., et al. (2013). Hydrogen production in an upflow anaerobic packed bed reactor used to treat cheese whey. Int. J. Hydr. Energy 38, 54–62. doi: 10.1016/j.ijhydene.2012.10.022
Qin, J., Li, R., Raes, J., Arumugam, M., Burgdorf, K. S., Manichanh, C., et al. (2010). A human gut microbial gene catalogue established by metagenomic sequencing. Nature 464, 59–70. doi: 10.1038/nature08821
Rico, C., Munoz, N., and Rico, J. L. (2015). Anaerobic co-digestion of cheese whey and the screened liquid fraction of diary manure in a single continuously stirred tank reactor process: limits in co-substrate ratios and organic loading rate. Biores. Tech. 189, 327–333. doi: 10.1016/j.biortech.2015.04.032
Rivière, D., Desvignes, V., Pelletier, E., Chaussonnerie, S., Guermazi, S., Weissenbach, J., et al. (2009). Towards the definition of a core of microorganisms involved in anaerobic digestion of sludge. ISME J. 3, 700–714. doi: 10.1038/ismej.2009.2
Roux, S., Enault, F., Robin, A., Ravet, V., and Personnic, S. (2012). Assessing the diversity and specificity of two freshwater viral communities through metagenomics. PLoS ONE 7:e33641. doi: 10.1371/journal.pone.0033641
Sansonetti, S., Curcio, S., Calabrò, V., and Iorio, G. (2009). Bio-ethanol production by fermentation of ricotta cheese whey as an effective alternative non-vegetable source. Biomass Bioenergy 33, 1687–1692. doi: 10.1016/j.biombioe.2009.09.002
SAS Statistical Analysis System Institute. (2012). 2002–2012. Vers 9.4. SAS User's Guide, Statistics. Cary, NC:SAS Institute Inc.
Sawatdeenarunat, C., Surendra, K. C., Takara, D., Oechsner, H., and Khanal, S. K. (2015). Anaerobic digestion of lignocellulosic biomass: challenges and opportunities. Bioresour. Technol. 178, 178–186. doi: 10.1016/j.biortech.2014.09.103
Schmidt, T. M., DeLong, E. F., and Pace, N. R. (1991). Analysis of a marine picoplankton community by 16S rRNA gene cloning and sequencing. J. Bacteriol. 173, 4371–4378. doi: 10.1128/jb.173.14.4371-4378.1991
Sneath, P. H. A., and Sokal, R. R. (1973). Numerical Taxonomy: The Principles and Practice of Numerical Classification. San Francisco, CA: W. H. Freeman.
St-Pierre, B., and Wright, A. D. (2014). Comparative metagenomic analysis of bacterial populations in three full-scale mesophilic anaerobic manure digesters. Appl. Microbiol. Biotechnol. 98, 2709–2717. doi: 10.1007/s00253-013-5220-3
Sun, L., Pope, P. B., Eijsink, V. G. H., and Schnürer, A. (2015). Characterization of microbial community structure during continuous anaerobic digestion of straw and cow manure. Microb. Biotechnol. 8, 815–827. doi: 10.1111/1751-7915.12298
Sutherland, A. D., and Varela, J. C. (2014). Comparison of various microbial inocula for the efficient anaerobic digestion of laminaria hyperborean. BMC Biotechnol. 14:7. doi: 10.1186/1472-6750-14-7
Tang, G. L., Huang, J., Sun, Z. J., Tang, Q. Q., Yan, C. H., and Liu, G. Q. (2008). Biohydrogen production from cattle wastewater by enriched anaerobic mixed consortia: influence of fermentation temperature and pH. J. Biosci. Bioeng. 106, 80–87. doi: 10.1263/jbb.106.80
Tapio, I., Fischer, D., Blasco, l., Tapio, M., Wallace, R. J., Bayat, A. R., et al. (2017). Taxon abundance, diversity, co-occurrence and network analysis of the ruminal microbiota in response to dietary changes in dairy cows. PLoS ONE 12:e0180260. doi: 10.1371/journal.pone.0180260
Tenca, A., Schievano, A., Perazzolo, F., Adani, F., and Oberti, R. (2011). Biohydrogen from thermophilic co-fermentation of swine manure with fruit and vegetable waste: maximizing stable production without pH control. Biores. Technol. 102, 8582–8588. doi: 10.1016/j.biortech.2011.03.102
Thauer, R., Kaster, A., Seedorf, H., Buckel, W., and Hedderich, R. (2008). Methanogenic Archaea: ecological relevant differences in energy conservation. Nat. Rev. Microbiol. 6, 579–589. doi: 10.1038/nrmicro1931
Tringe, S. G., and Hugenholtz, P. (2008). A renaissance for the pioneering 16S rRNA gene. Curr. Opin. Microbiol. 11, 442–446. doi: 10.1016/j.mib.2008.09.011
Tringe, S. G., Zhang, T., Liu, X., Yu, Y., Lee, W. H., Yap, J., et al. (2008). The airborne metagenome in an indoor urban environment. PLoS ONE 3:e1862. doi: 10.1371/journal.pone.0001862
Vasmara, C., and Marchetti, R. (2017). Initial pH influences in-batch hydrogen production from scotta permeate. Int. J. Hydr. Energy 42, 14400–14408. doi: 10.1016/j.ijhydene.2017.04.067
Venetsaneas, N., Antonopoulou, G., Stamatelatou, K., Kornaros, M., and Lyberatos, G. (2009). Using cheese whey for hydrogen and methane generation in a two-stage continuous process with alternative pH controlling approaches. Bioresour. Technol. 100, 3713–3717. doi: 10.1016/j.biortech.2009.01.025
Venter, J. C., Remington, K., Heidelberg, J. F., Halpern, A. L., Rusch, D., Eisen, J. A., et al. (2004). Environmental genome shotgun sequencing of the Sargasso Sea. Science 304, 67–74. doi: 10.1126/science.1093857
Weiland, P. (2010). Biogas production: current state and perspective. Appl. Microbiol. Biotechnol. 85, 849–860. doi: 10.1007/s00253-009-2246-7
Weimer, P. J. (2015). Redundancy, resilience, and host specificity of the ruminal microbiota: implications for engineering improved ruminal fermentations. Front. Microbiol. 6:296. doi: 10.3389/fmicb.2015.00296
Weimer, P. J., and Kohn, R. A. (2016). Impact of ruminal microorganisms on the production of fuels: how can we intercede from the outside? Appl. Microbiol. Biotechnol. 100, 3389–3398. doi: 10.1007/s00253-016-7358-2
Keywords: waste, bio-hydrogen, rumen, buffalo sludge, low protein cheese whey (scotta), metagenomic, microbial community
Citation: Chiariotti A and Crisà A (2018) Bio-Hydrogen Production From Buffalo Waste With Rumen Inoculum and Metagenomic Characterization of Bacterial and Archaeal Community. Front. Sustain. Food Syst. 2:13. doi: 10.3389/fsufs.2018.00013
Received: 26 January 2018; Accepted: 10 April 2018;
Published: 02 May 2018.
Edited by:
Claudia Wagner-Riddle, University of Guelph, CanadaCopyright © 2018 Chiariotti and Crisà. This is an open-access article distributed under the terms of the Creative Commons Attribution License (CC BY). The use, distribution or reproduction in other forums is permitted, provided the original author(s) and the copyright owner are credited and that the original publication in this journal is cited, in accordance with accepted academic practice. No use, distribution or reproduction is permitted which does not comply with these terms.
*Correspondence: Antonella Chiariotti, antonella.chiariotti@crea.gov.it