- 1Department of Food Science and Technology, Virginia Tech, Blacksburg, VA, United States
- 2Department of Civil and Environmental Engineering, Virginia Tech, Blacksburg, VA, United States
- 3Department of Biological Systems Engineering, Virginia Tech, Blacksburg, VA, United States
Cattle are commonly administered antibiotics, resulting in excretion of antibiotics, antibiotic-resistant bacteria (ARB), and antibiotic resistance genes (ARGs). The aim of this study was to determine if the use of dairy manure collected during antibiotic administration influences the bacterial microbiota of lettuce and radishes, including carriage of ARB and ARGs, when applied as a soil amendment and if composting mitigates the effects. Lettuce and radishes were grown in field-plots amended with raw manure from antibiotic-treated (cephapirin, pirlimycin) cows, composted manure from antibiotic-treated cows, composted manure from antibiotic-free cows, or an inorganic chemical fertilizer (control; 12 plots, n = 3). Surficial vegetable bacteria and antibiotic resistomes (i.e., total ARGs) were characterized using heterotrophic plate counts (HPCs) on antibiotic-containing media, 16S rRNA gene amplicon sequencing, quantitative polymerase chain reaction (qPCR), and shot-gun metagenomics. The different manure and compost amendments did not result in significant changes to the surficial vegetable bacteria at the phylum level; however, some minor changes at the class and family level were observed. Beta-diversities of the ARGs detected by shotgun metagenomic sequencing were distinctly different between vegetable type (R = 0.30, p = 0.04), with small separations between the resistomes associated with amendment type in unrarefied analysis (R = 0.27, p = 0.02), but not rarefied analysis, of the data. Network analysis highlighted that multi-drug ARG classes commonly co-occurred with plasmid-associated genes and could be a driver of co-and cross-selection of ARGs in the different conditions. Carriage of sul1 and tet(W) ARGs on vegetables quantified by qPCR were strong indicators of manure-based amendment relative to chemical fertilizer, with some reduction incurred via composting (p < 0.05). Also, increased HPCs resistant/tolerant to clindamycin, a class of antibiotics administered to cattle, were on lettuce grown in biological soil amendments relative to chemical fertilizer (p < 0.05). This study demonstrates that amending soil with raw manure collected from dairy cows during antibiotic administration may affect the composition of microbiota and resistomes associated with vegetable surfaces. Composting may be an important strategy to reduce some ARGs on fresh produce, but differences in the resistomes of lettuce and radishes suggest the extent of soil contact should be considered.
Introduction
There is growing awareness of the need to harmonize requirements for increased food production to feed a growing human population with other environmental and public health concerns (Reganold and Wachter, 2016). With the rise in antibiotic resistance expected to result in more deaths due to antibiotic treatment failure than due to cancer by the year 2050 (O'Neill, 2014), linkages between antibiotic use in livestock production and the spread of resistant bacteria to human populations is one such concern (Center for Disease Control (CDC), 2013). Notably, in the United States and many other countries, the majority of antibiotics used are administered to livestock (Van Boeckel et al., 2015), which helps to maintain healthy herds and optimize production (Lhermie et al., 2017). In the absence of alternatives such as vaccines, some level of antibiotic use is necessary at a minimum to treat and prevent illness. In the case of dairy cattle, antibiotics are only used to treat and prevent major infections and cannot be used during milking for human consumption. However, the antibiotic classes used, 1st and 3rd generation cephalosporins and lincosamides, overlap with classes used in human medicine. To combat antibiotic resistance strategies—including reduced use of antibiotics in livestock and avoiding the use of clinically-important antibiotics for human medicine in livestock—should be complemented with manure management strategies (Meek et al., 2015). The potential for tailored manure management strategies to mitigate the spread of antibiotic resistance is gaining attention (Arikan et al., 2009; Tien et al., 2017).
It is well-established that antibiotics administered to livestock, and associated metabolites, are subsequently excreted and can alter levels and types of antibiotic resistant bacteria (ARB) and antibiotic resistance genes (ARGs) in manure (Chambers et al., 2015; Chiesa et al., 2015). Application of manure has been shown to increase ARGs detected in soil (Heuer et al., 2011; Marti et al., 2013; Udikovic-Kolic et al., 2014; Wepking et al., 2017), which brings to question whether the corresponding vegetable-associated microbiota are also affected. The application of manure-based amendments to soils (croplands and greenhouse studies) has been associated with increased detection of ARB and ARGs on lettuce and root vegetables; however, this phenomenon has not been associated uniformly with all crops or ARGs (Marti et al., 2013; Rahube et al., 2014; Wang et al., 2015).
In the United States, the Food Safety Modernization Act (FSMA) recommends that animal manure be composted by acceptable treatment processes, as defined in § 112.54, to achieve pathogen reduction, as defined in § 112.55, before it can be applied to land used for vegetable production [Food Drug Administration (FDA), 2015]. While composting has established benefits for pathogen reduction, the effect of composting on ARGs is unclear. Recent studies have demonstrated that composting can reduce detectable levels of parent antibiotic compounds in the manure (Dolliver et al., 2005; Kim et al., 2012; Ray et al., 2017), but effects on markers of antibiotic resistance (ARBs and ARGs) have only been investigated to a limited extent and with mixed results (Dolliver et al., 2005; Gou et al., 2018) In a controlled microcosm experiment using HT-qPCR, cattle-derived compost-amended soils had significantly lower levels of ARG relative abundance compared to the same raw manure amended soils (Gou et al., 2018). Subsequent effects of composting on vegetable-borne microbiota and carriage of ARBs and ARGs are unclear.
In this field-scale study, culture-dependent and independent analyses were conducted to determine whether raw manure or compost influences the bacterial microbiota and associated resistomes [total ARGs as defined by Wright (2007)] of vegetables cultivated in soils amended with those cattle-derived treatments. Dairy manure served as a good model, as the antibiotics used in this study, pirlimycin and cephapirin, a lincosamide and a 1st generation cephalosporin, respectively, represent classes that are important to human use [World Health Organization (WHO), 2011]. Further, examining both lettuce and radishes, i.e., a leafy vs. a root vegetable, provided insight into the extent to which low vs. high soil contact might influence the effects of the soil amendments. These vegetables are commonly consumed raw, and thus are representative for examining how agricultural practices could ultimately affect human exposure. Field plot manure-derived amendments included the following: raw manure from antibiotic-treated cows, composted manure from antibiotic-treated cows, composted manure from antibiotic-free cows, and an inorganic-chemical fertilizer control applied to a clay loam soil that had not received manure-derived amendments or antibiotics for at least a decade. Lettuce and radishes were grown to maturity, harvested, and a suite of culture-based and molecular-based analyses were applied to characterize the surficial microbiota and measures of antibiotic resistance including sequencing of 16S rRNA gene amplicons, enumeration of antibiotic-resistant/tolerant heterotrophic plate count (HPC) bacteria, quantitative polymerase chain reaction (qPCR) of sul1 and tet(W) as key “indicator” ARGs, and shot-gun metagenomic sequencing for comprehensive profiling of ARGs. The findings provide insight into the relative influences of antibiotic use and manure management in controlling the vegetable-borne resistome as a potential source of spread of antibiotic resistance and exposure to humans.
Materials and Methods
Field Operations
Experiments were performed during the Spring 2016 growing season at the Virginia Tech's Urban Horticulture Center (UHC) in Blacksburg, Virginia (37.2162° N, 80.4637° W) as recently described in a companion study on the effects of the manure-based amendments on culture-based indicators of antibiotic resistance in the soil (Wind et al., 2018). The soil was a clay loam to which manure-derived amendments or antibiotics had not been intentionally applied within the past decade prior to this project. The land was divided into 24 (3 × 3 m) plots, each bordered with steel siding to reduce cross contamination of the soil amendments. The plots were treated with one of four soil amendments: raw manure from antibiotic-treated cows (“antibiotic manure”), composted manure from antibiotic-treated cows (“antibiotic compost”), composted manure from antibiotic-free cows (“antibiotic-free compost”), and an inorganic chemical fertilizer control (“chemical fertilizer”) (Southern States, Richmond, VA; Supplemental Figure S1). Soil was pretreated with Roundup (glyphosate) and Inorganic Nitrogen-Phosphorous-Potassium (N-P-K), as it was determined that the background soil nutrients were not sufficient to support the growth of vegetables. Natural rainfall was sufficient to grow the crops for this study and irrigation was not necessary.
Manure was generated from two groups of three dairy cows that received intramammary administration of either pirlimycin (2 and 50 mg doses 24 h apart) or cephapirin (1 dose, 300 mg) and mixed to form “antibiotic” manure, in the same manner described previously (Ray et al., 2017). The cows had not received antibiotics their previous lactation cycle. Manure was collected over a period of peak antibiotic excretion as determined by Ray et al. (2014a,b). Manure was also collected from “antibiotic-free” cows not administered antibiotics their previous lactation cycle. Administration of antibiotics for the dairy manure generated in this study was approved by Virginia Tech IACUC 13-145 to Dr. Katharine F. Knowlton. Additionally, all members of this project completed Responsible Conduct of Research and Conflict of Interest Trainings, as required by Virginia Tech Office of Research Integrity according to guidelines for individuals conducting federally-funded research.
Manure for composting was combined with alfalfa hay (4:1) and sawdust (4:3) to achieve a Carbon: Nitrogen ratio of 25–30:1 and a moisture content of 55–65%. The same additions and ratios were reached with generate composted manure from cows administered antibiotics (antibiotic compost) or not administered antibiotics (antibiotic-free compost). Both composts were developed using a forced aeration static composting approach following FSMA guidelines, reaching an internal temperature >55°C by day 2 of composting. The core temperature of the compost pile remained thermophilic (>55°C) for 21 days.
Manure and compost were applied at a rate of 6.72 Mg/ha to designated field plots. Manure was stockpiled for 57d (in parallel with the composting) before application. N-P-K was added to the fertilizer control plots at rates recommended for optimal growth for radish (50 N-50P-50K) and lettuce (125N−100P−100K;Virginia Cooperative Extension, 2015). Because the manure/compost was not nutritionally sufficient alone to meet the optimal growth levels, supplemental inorganic N-P-K was also applied to the plots at rates of 50-50-20 for radish and 100-100-75 for lettuce (Wind et al., 2018). N-P-K, manure, and compost(s) were applied to the plots on Day 0 of the comprehensive study.
Lettuce transplants and radish seeds were planted in triplicate plots based on guidance for commercial growers (Virginia Cooperative Extension, 2015). Raphanus sativus cv. Crunchy Royal radish (Johnny's Selected Seeds, Fairfield, Maine) seeds were sown 30d after application of soil amendments (n = 3 per amendment). Seeds were planted 1/8 to 1/4-inch-deep in rows that were roughly 2–3 inches apart (Virginia Cooperative Extension, 2015). Lactuca sativa cv. Organic Nancy lettuce seedlings were transplanted into 12 field plots (3 replicates per amendment, Supplemental Figure S1) at a stock rate of roughly 54 plants per plot. Transplanting occurred 30d after application of soil amendments. Seedlings had been grown in horticulture vermiculite, hand-watered and fertilized with inorganic N-P-K solution for 8 weeks before transplant.
Samples of the edible portions of crops were harvested when deemed market ready: taproots protruding for radishes and lettuce heads of 12 inches in diameter. Lettuce and radish were harvested 68d and 75d after amendment application, respectively. Six heads of lettuce and ten radishes were selected and harvested while wearing gloves and shoe covers; to minimize cross contamination gloves and shoe covers were changed between each plot. Crops that showed visible signs of decay or plant disease were not selected for analysis. The lettuce heads were removed from the base just above soil level using ethanol-sterilized pruning shears. The bottom-most leaves (4–6) in direct contact with the soil were removed from the base and discarded. Crops were placed individually into large collection bags and kept in a cooler for immediate transport to the lab. Samples were processed for culturing and DNA extraction within two hours of harvest.
Vegetable Processing
Bacterial cells were recovered from the surface of radish taproots (75 g) or lettuce leaves (25 g, 2–3 inner and outer leaves) by gently shaking at 220 rpm on a multi-purpose rotator (Fisher Scientific, Waltham, MA) for 5 min while submersed in a solution of sterile 0.1% peptone (Difco, Becton Dickinson and Company, Franklin Lakes, NJ) with 0.1% Tween 80 (Fisher Scientific). Each sample was then hand massaged for an additional 2 min after shaking. This approach aimed to gently remove bacteria from the vegetables to minimize disruption of plant cells and release of plant DNA. For each plot (n = 12 lettuce, 12 radish), collected vegetables were processed separately and the resulting diluents pooled to create one composite sample for which a portion was used for enumeration and DNA extracted from the remaining diluent.
Enumeration of Aerobic Heterotrophic Plate Count (HPC) Bacteria
Vegetable diluents were serially diluted to enumerate HPC bacteria by plating 100 μl in duplicate onto suite of R2A media (Difco, Becton Dickinson and Company Franklin Lakes, NJ) containing different antibiotics (3 μg/ ml tetracycline, 10 μg/ ml ceftazidime, 25 μg/ ml, erythromycin, 25 μg/ ml clindamycin, 25 μg/ ml sulfamethoxazole, and 11 μg/ ml vancomycin) and a non-antibiotic control. Antibiotic concentrations were selected based on trial enumerations of bacterial colonies from compost from dairy cattle treated with antibiotics on R2A of differing antibiotic concentrations. Concentrations were chosen by an observed decrease in CFU from the lowest antibiotic concentration tested. Concentrations were chosen by at least one-log decrease in colony forming unit (CFU) from the lowest antibiotic concentration tested. Plates were incubated at 37°C for 24 h prior to enumeration.
DNA Extraction
Immediately after enumeration, the remaining diluent containing the surface vegetable microorganisms was aseptically filtered through 0.22-μm pore size/47-mm diameter mixed cellulose esters membranes (EMD Millipore, Merck Group, Darmstadt, Germany) to collect bacterial cells. Filters were stored at −80°C until DNA extraction. Diluent from each of the 12 lettuce and 12 radish samples was processed independently.
The frozen filters were transferred to Lysing Matrix E tubes from the FastDNA Spin Kit for Soil (MP Biomedicals, Solon, OH) according to manufacturer protocol and homogenizing using a FastPrep® (MP Biomedicals, Solon, OH) speed setting of 6.0 m/s for 40 s. The protocol was modified with an additional bead beating step and 2 h incubation at room temperature, to enhance cell lysis. The freshly eluted DNA was cleaned up using the OneStep PCR Inhibitor Removal Kit (Zymo Research Corporation, Irvine, CA) per manufacturer's directions before storing at −80°C in DNase-free, O-ring screw cap tubes. DNA concentrations were measured using the NanoDrop ND100 (NanODrop Technologies, Wilmington, DE). One radish sample grown in antibiotic-free compost was lost during the DNA extraction process; thus n = 2 for antibiotic-free compost throughout this experiment.
Quantification of Antibiotic Resistance Genes
qPCR was used to enumerate 16S rRNA gene copy numbers as an indicator of “total bacteria” and tet(W) (Aminov et al., 2001) and sul1 (Pei et al., 2006) copies as indicators of anthropogenic sources of antibiotic resistance (Storteboom et al., 2010a,b). DNA extracts from the surface of the field grown lettuce and radishes were diluted 1:10 to reduce PCR inhibition. The 10 μl reaction mixture consisted of 2x SsoFast EVAgreen Supermix (BioRad Laboratories, Hercules, CA), 20 ng of DNA template, and 400 nM primers. Triplicate technical replicates of each sample were amplified along with triplicate standard curves and a negative control (molecular grade water). qPCR was carried out on a CFX Connect TM Touch Real-Time PCR Detection System (BioRad Laboratories Hercules, CA). The protocol consisted of 1 cycle of 98°C for 2 min, 40 cycles of 98°C for 5 s and annealed at various temperatures and times depending on the gene target. 16S rRNA gene targets were annealed at 55°C, 5 s, tet(W) at 61°C, 7 s and sul1 at 71°C, 7 s followed by a melt curve. The standard curves were comprised of seven 10-fold dilutions and ranged from 108 to 102 gene copies/μl for 16S rRNA gene and 107 to 101 gene copies/μl for tet(W) and sul1.
16S rRNA Amplicon Sequencing and Analysis
16S rRNA gene amplicon sequencing was performed on DNA extracts following the Earth Microbiome Project 16S Amplification Protocol version 4_13 (Walters et al., 2016). DNA samples from each vegetable plot were amplified via PCR using unique barcoded bacteria-archaeal primers 515FB and 926R. The amount of DNA used for amplification was normalized to equivalent 16S rRNA gene copy numbers between all samples before barcoded PCR amplification. PCR products were measured using Qubit 2.0 Fluorometer (Thermo Fisher Scientific, Waltham, MA) and pooled equally at 200 ng each. Products were then purified using the QIAquick PCR Purification Kit (QIAGEN, Valencia, CA). Libraries were prepped by the Biocomplexity Institute of Virginia Tech using the MiSeq® Reagent Kit v3 (Illumina, Inc., San Diego, CA) and 2 × 300-bp paired-end reads were produced using MiSeq (Illumina, Inc., San Diego, CA). Paired ends were stitched at 372–375 bp and score ≥0.90 using PANDASeq (Masella et al., 2012) and OTUs were picked de novo using QIIME (Caporaso et al., 2011) from the GreenGenes database at 97% cutoff. Chimera sequences were discarded using ChimeraSlayer with QIIME (Haas et al., 2011). Chloroplast and mitochondrial sequences were also discarded using the filter_taxa_from_otu_table.py function. Raw reads were uploaded to the NCBI BioProject PRJNA506850 with relevant metadata and Short Read Accessions SRX5431847 through SRX 5431869.
Metagenomic Analysis
DNA extracts of biological replicates of each lettuce (n = 12) and radish (n = 11) grown in the four amendments were subject to shotgun metagenomic sequencing at the Biocomplexity Institute of Virginia Tech (Blacksburg, VA). Libraries were prepared from 3 ng DNA extract using the Accel-NGS 2S DNA kit (SwiftBio, Ann Arbor, MI) incorporating 11 cycles of PCR and subject to high throughput sequencing on an Illumina HiSeq 2500 in High Output mode and paired-end 2 × 100 read length protocol. The paired-end sequence files were uploaded and analyzed via MetaStorm (Arango-Argoty et al., 2016). The Comprehensive Antibiotic Resistance Database (CARD v1.2.1) was used for functional annotation of ARGs for the read matched samples (Jia et al., 2017). The gene counts derived from MetaStorm were normalized to the abundance of 16S rRNA gene copy number, accounting for variable read and gene length, to determine the relative abundances of the total ARGs detected as described by Li et al. (2015). The trimmomatic default setting was used in MetaStorm, providing 80% nucleotide coverage of each read. To account for chloroplast contamination of DNA extracts, which can confound analyses because chloroplasts contain 16S rRNA genes and can otherwise be annotated as “bacteria” and also result in highly variable extraction efficiency from true bacteria, two different data analysis approaches were taken. The first was to identify 16S chloroplast sequences obtained from Greengenes (DeSantis et al., 2006) and remove them using Bowtie2 (Langmead and Salzberg, 2012), and then rarefy the data to the same sequencing depth of 11,800,000 reads using Vsearch (Rognes et al., 2016). Scripts can be found at the following web address: https://github.com/gaarangoa/genomic-scripts. The second was to analyze the data unrarefied and without chloroplast removal, representing a more typical approach and avoiding loss of data in the analysis. Raw reads were uploaded to the NCBI BioProject PRJNA506850 with relevant metadata and Short Read Accessions SRX 5255057 through SRX 5255079.
Statistical Analysis
JMP® Pro 13 (SAS Institute, Cary, NC) was utilized for all statistical analyses; p ≤ 0.05 indicated statistical significance for all parametric and non-parametric tests. Plate counts between 25 and 250 CFU/plate were considered countable and log-transformed to approximate normal distribution. A one-way ANOVA analysis with a Tukey's post-hoc analysis was used to test for differences in the average log CFU/g of antibiotic resistant HPCs recovered from lettuce and radish surfaces grown in soils with the different amendments.
Due to non-normal distribution, the non-parametric Wilcoxon coupled with a post hoc Steel-Dwass All Pairs test was used to compare the qPCR gene copy numbers/16S rRNA gene copies from vegetables grown in the different treatments. Significance between samples was defined at p ≤ 0.05.
The α-diversity estimates acquired from the 16S rRNA gene amplicon sequencing (relative abundance of observed species, Shannon index and Chao1 values; Shannon and Weaver, 1949; Chao, 1984; Lozupone and Knight, 2005) were compared by using Wilcoxon coupled with a Steel-Dwass All Pairs test. Unweighted and Weighted Unifrac distances derived from the β-diversity estimates were plotted in Non-metric Multidimensional Scaling (NMDS) plots in PRIMER-E (version 6.1.13). β-diversity estimates were compared in PRIMER-E using analysis of similarities (ANOSIM) (p ≤ 0.10), which is a common cut-off for microbial community analysis. R-value cutoffs as defined by Clarke and Warwick (2001) were used (R > 0.75, well-separated; 0.75 < R>0.25, separated but overlapping; R < 0.25, barely separated). The relative abundances of total ARGs from shotgun metagenomics were compared by using the Kruskal-Wallis test (p < 0.05).
ANOSIM and NMDS plots of total ARGs detected were performed using PRIMER-E (Version 6.1.13) to compare ARG profiles (type and relative abundance; Bray-Curtis). R-value cutoffs as defined by Clarke and Warwick (2001) were used (R > 0.75, well-separated; 0.75<R>0.25, separated but overlapping; R < 0.25, barely separated). Due to lack of normal distribution, one-way comparisons of the relative abundances for each ARG class were determined using R functions for Wilcoxon test with pairwise comparisons. Fold differences in relative abundances were determined using geometric means of the treatment pairs significantly different from each other. JMP® Pro 13 was used for Spearman's correlation and for assembling stacked bar graphs.
Network analysis of the vegetable microbiomes were done via de novo assembled scaffolds to visualize the co-occurrence (similarity of location) of ARGs and mobile genetic elements located on the DNA scaffolds assembled via MetaStorm following methods of Garner et al. (2016). Assembled scaffolds averaged 908 bp and the associated assembled reads ranged between 1,841,022 and 9,578,473 for all samples (n = 22; 2 samples were not able to be assembled). Known housekeeping genes from the CARD v1.6.0 (Jia et al., 2017) were extracted from this analysis. R version 3.3.2 (R Core Team, 2016) and Cytoscape version 3.7.1 (Shannon et al., 2003) were used to visualize co-occurrence of ARGs within the vegetable microbiome.
Results
Effect of Soil Amendment on Antibiotic-Resistant HPCs
Enumerating HPCs served as a culture-based means of assessing whether the amendments influenced the levels of viable and culturable general aerobic heterotrophic bacteria on the vegetable surfaces. The total HPCs recovered from lettuce and radishes (log CFU/g) grown in soil receiving each of manure and compost amendments were comparable to those when grown in plots only receiving inorganic chemical fertilizer (Table 1). HPCs were generally reduced on R2A plates containing antibiotics. HPCs represent a range of aerobic heterotrophic bacteria, most of which have unknown MICs; the HPCs growing on R2A with antibiotics represent the sum of both “tolerant” (i.e., survive due to intrinsic traits, i.e., not targeted by the antibiotics) and truly “resistant” (i.e., contain ARGs that enable otherwise susceptible bacteria to survive in presence of antibiotics) bacteria. For brevity, we hereafter refer to these as “resistant” HPCs. In general, the number of resistant HPCs was not significantly different for either vegetable type when grown with the manure or compost amendments relative to the chemical fertilizer control (p > 0.05; Table 1). The only exception was that lettuce grown in the three compost/manure amendments yielded ~1.5–2.0 log CFU/g greater clindamycin-resistant HPCs compared to lettuce grown with chemical fertilizer only (p < 0.05; Table 1).
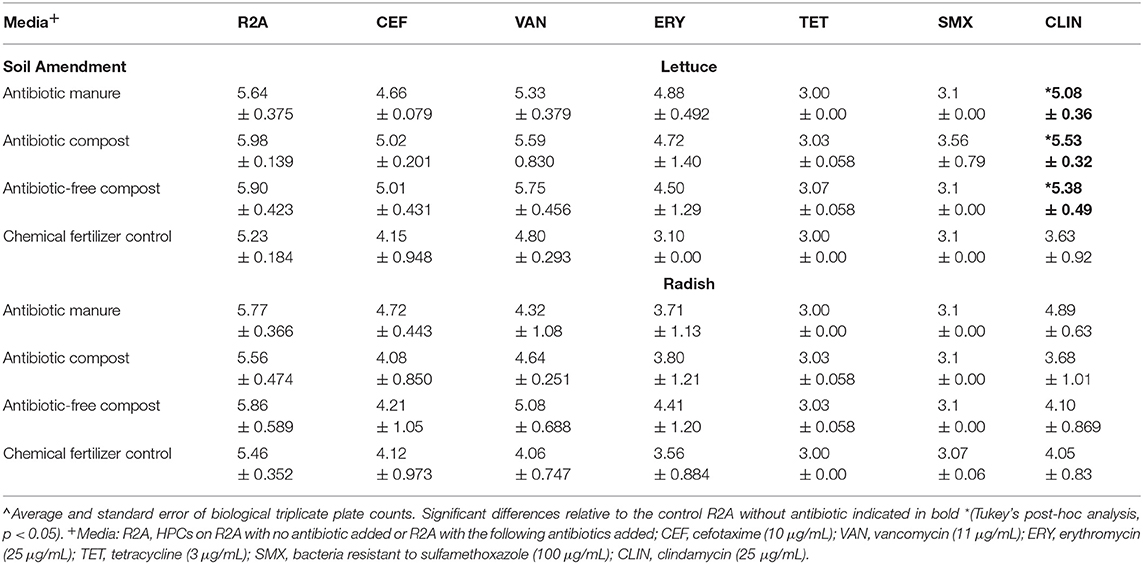
Table 1. Effect of amendments on lettuce and radish heterotrophic plate counts (HPCs) determined on R2A media with and without indicated antibiotics∧.
Effect of Soil Amendments on the Vegetable-Associated Bacterial Microbiota
Sequencing of 16S rRNA genes served to assess whether the various amendment conditions influenced the bacterial microbiota profiles of the vegetable surfaces. A total of 273,429, and 149,010 bacterial sequence reads were obtained from the lettuce and radishes, respectively. Rarefaction curves indicated that coverage of lettuce samples was nearly saturated and greater than coverage of radish samples (Supplemental Figures S2, S3). Sequence depth ranged between 6,127 and 40,750 per sample, with chloroplast sequences removed from all samples and rarefied to 6,127 for further comparison.
The Unweighted Unifrac distances, reflecting the β-diversity of the lettuce and radish bacterial communities, were significantly different (p < 0.001) and strongly separated (Global R = 0.77) based on vegetable type (Figure 1). Further, growth in different amendments created a significant difference in β-diversity for both vegetable types (p = 0.02), however, the compositions of the bacterial microbiota were only weakly separated (Global R < 0.25). Pairwise tests determined that vegetables grown in antibiotic manure were significantly different than those grown in control plots (p = 0.02) and were distinctly separated (R = 0.48; Figure 1). Vegetables grown in antibiotic compost were also found to be significantly different than those samples grown in antibiotic manure (p = 0.02) and were distinctly separated (R = 0.68). The Weighted Unifrac distances were significantly different (p < 0.001) and strongly separated based on vegetable type (Global R = 0.76), but not amendment type (p > 0.05, Global R < 0.25; Supplemental Figure S4).
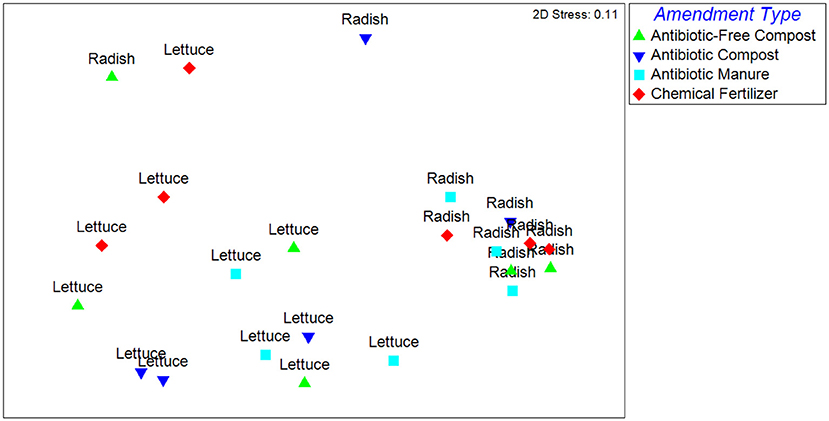
Figure 1. NMDS analysis of Unweighted Unifrac distance matrix of the bacterial microbiota represented by 16S rRNA gene amplicon sequences recovered from field-grown lettuce and radish samples. Each point indicates vegetables harvested from separate replicated plots described in Supplemental Figure S1. ANOSIM indicated that samples were significantly different based on vegetable type (p < 0.001) and amendment type (p = 0.02). Samples were strongly separated based on vegetable type (Global R = 0.77).
The same phyla and bacterial classes dominated the surfaces of the lettuce and radish samples in varying relative abundances. Proteobacteria were the most abundant phylum for both lettuce and radishes (>80%) followed by Actinobacteria (>4%) and Firmicutes (>6%; Figure 2, Supplemental Tables S1, S2). Gammaproteobacteria (57.3%) was the dominant class among lettuce samples while Alphaproteobacteria (34.6%) dominated the radish surfaces (Figure 2, Supplemental Tables S1, S2).
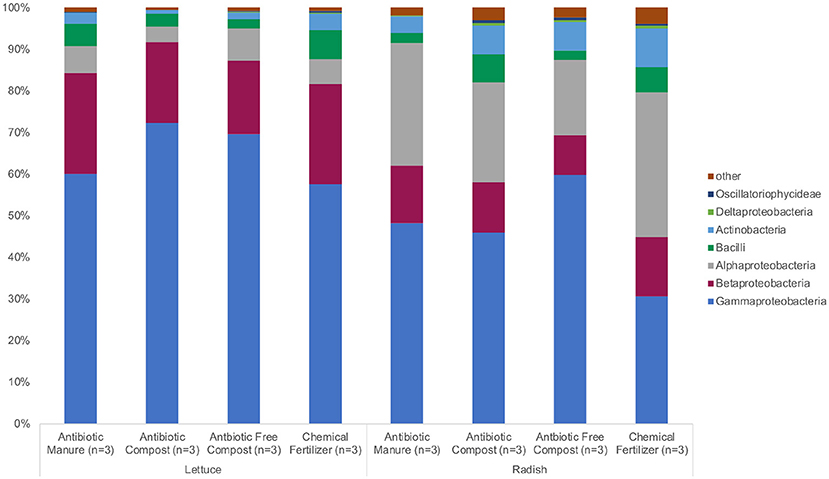
Figure 2. Relative abundance of microbiota represented by 16S rRNA gene amplicon sequences recovered from field-grown lettuce and radish samples. No significant differences in relative abundance were detected using Wilcoxon with Steele-Dwass post-hoc adjustment.
Effect of Soil Amendment on Lettuce-Associated Bacterial Microbiota
The α-diversity (Shannon index, Chao1, and Evenness) of the lettuce phyllosphere bacterial microbiota were minimally affected by the use of biological soil amendment (p > 0.05; Supplemental Table S3). Lettuce grown in antibiotic manure yielded the highest mean Chao1 value, implying that the samples were more diverse in their bacterial composition than the three other treatment types.
The Unweighted Unifrac distances of the lettuce phyllosphere amplicons were significantly different (p = 0.01) and slightly separated (Global R = 0.35) based on amendment type (Supplemental Figure S5). In particular, bacterial microbiota of lettuce grown in antibiotic manure or antibiotic compost were significantly different (p = 0.10) and clustered separately (R = 0.70 and 0.96, respectively) from those grown with chemical fertilizer only (Supplemental Figure S5). ANOSIM of the Weighted Unifrac distances of the lettuce samples grown in the field did not define amendment type as an overall separation factor (p > 0.10, Global R < 0.25; Supplemental Figure S6). However, pairwise testing revealed that lettuce grown in antibiotic compost indicated significant differences, being significantly different (p = 0.10) and slightly separated (R = 0.33) from lettuce grown in chemical fertilizer only (Supplemental Figure S6).
The bacterial sequences classified for lettuce samples were assigned to 13 phyla; chiefly Proteobacteria (93.9%), Firmicutes (4.38%), and Actinobacteria (2.39%; Figure 2, Supplemental Table S1). There were no significant differences between the relative abundances of detected phyla based on amendment type. Bacteria were identified from 44 different classes; Gammaproteobacteria had the highest relative abundance among lettuce samples (64.8%). Additionally, Betaproteobacteria (21.5%), Actinobacteria (2.30%), Bacilli (4.33%), and Alphaproteobacteria (5.89%) belonged to the most represented classes (representing more than 1% relative abundance) identified from the lettuce samples. There were no significant differences between the relative abundances of detected classes and amendment type. The most abundant bacterial order identified from the lettuce sequences belonged to Pseudomonadales (49.1%); Burkholderiales (20.1%); and Eneterobacteriales (14.6%). Methylophilales (1.37%) were significantly more abundant on lettuce samples grown in the two composted amendments (p = 0.045; Supplemental Table S1). Of the 11 most represented families of bacteria, Oxalobacteraceae (19.5%), Moraxellaceae (21.5%), and Pseudomonadaceae (27.5%) proved to be most abundant. Methylophilaceae were roughly 40 times more abundant (p = 0.044) on lettuce samples grown in the two composted amendments compared to samples grown in antibiotic manure or chemical fertilizer plots.
Effect of Soil Amendment on Radish-Associated Bacterial Microbiota
The α-diversity indices (Shannon index, Chao1, and Evenness) were highly similar among radish samples, regardless of amendment type (p > 0.05; Supplemental Table S3). In addition, the β-diversity of the radish bacterial microbiota (unweighted Unifrac distances) were not significantly different when grown in different amendment types (p > 0.10, Global R < 0.25; Figure 1, Supplemental Figure S5). ANOSIM of the weighted Unifrac distances did result in a significant difference among radish amendment conditions (p = 0.56) and almost created separation (Global R = 0.24; Supplemental Figure S6). Bacterial microbiota of radishes grown in antibiotic manure were significantly different and separated from radishes grown in antibiotic compost or chemical fertilizer only plots (p = 0.10; R = 0.50, and R = 0.59, respectively; Supplemental Figure S6).
Bacterial sequences classified across all radish samples were assigned to 21 phyla. Most sequences were assigned to Proteobacteria (85.7%), Actinobacteria (7.06%), and Firmicutes (4.42%) (Figure 2, Supplemental Table S2). Significant differences were not detected between the relative abundances of the detected phyla. Sixty-four classes of bacteria were identified from the radish sequences; Gammaproteobacteria had the highest relative abundance (46.2%). The most represented sequences belong to the classes Alphaproteobacteria (26.5%), Betaproteobacteria (12.4%), Actinobacteria (6.75%), and Bacilli (4.31%) (Figure 2). There were no significant differences between the relative abundances of detected classes. The most abundant order of bacteria identified from the radish sequences belonged to Pseudomonadales (38.5%); Rhizobiales (21.4%), and Burkholderiales (9.70 %). Seven additional bacterial orders were largely represented among radish samples. Of the 12 most represented families of bacteria, Pseudomonadaceae (27.5%), Rhizobiaceae (17.5%), and Moraxellaceae (15.8%) proved to be most abundant. Pseudomonadaceae were up to 10 times more abundant on radishes grown in manure (p = 0.032; Supplemental Table S2) than any other amendment type.
Shotgun Metagenomic Profiling of ARGs
Shotgun metagenomic sequencing served as a comprehensive means to profile the resistomes associated with the vegetable surfaces, in terms of total ARGs detected, classes of antibiotics to which they encode resistance, and relative abundances of individual classes and types. A total of 333,843,560 paired-end reads were generated after quality filtering from 23 DNA extracts, with an average of 14,514,937 reads per sample. 16S rRNA gene sequences obtained from the lettuce and radish shotgun libraries were found to be 4.1–67.1% and 0.3–17.2% chloroplast-derived, respectively (Supplemental Table S4). Twenty different antibiotic resistance classes were identified. While all 20 classes were detected in all radish amendment conditions, 17 of the 20 classes were identified across all 4 lettuce amendment conditions (Supplemental Tables S5, S6). Sulfonamide ARGs were only detected on lettuce amended with antibiotic manure. Elfamycin and tetracenomycin ARGs were also not detected on lettuce amended with antibiotic-free compost. Because DNA extractions targeting vegetable-associated bacterial microbiota tend to be highly variable in yield and are susceptible to chloroplast contamination, analysis was carried out both on unrarefied data without chloroplast removal and on data rarefied to 11,800,000 reads per sample with chloroplast 16S rRNA genes removed. The unrarefied method represented a traditional approach and avoided losing information, while the rarefied method facilitated statistical comparison on more comparable data sets. A total of 640 and 622 ARGs were identified prior to and after rarefication, respectively, with the same 20 antibiotic resistance classes detected in both datasets (Figure 3).
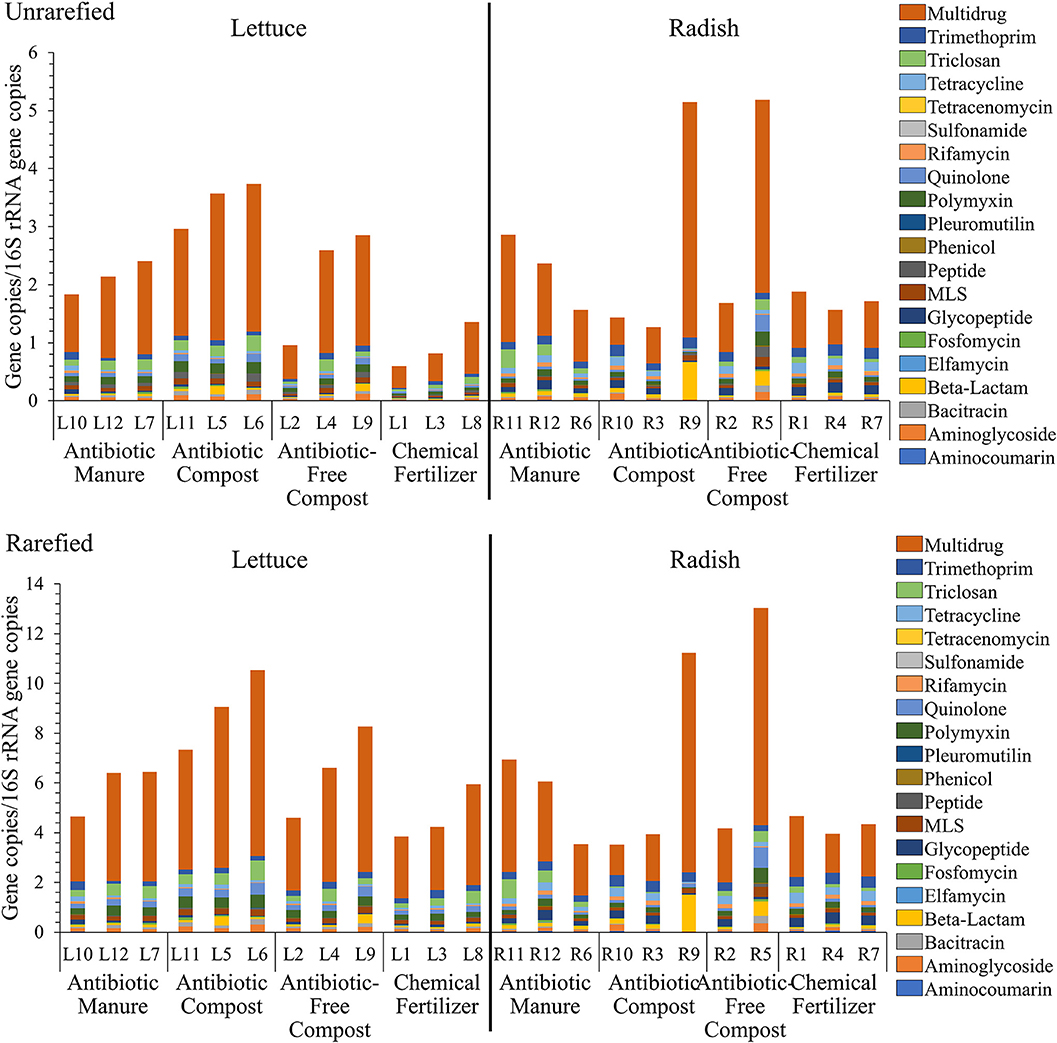
Figure 3. Relative abundance of total DNA sequence reads annotated as ARGs, as aligned to CARD v1.2.1 (e-value < 1e−10, 80% identity, ≥25 amino acids), and normalized to 16S rRNA reads, as described by Li et al. (2015). (top) Unrarefied, no chloroplast removal: 20 different antibiotic resistance classes were represented, normalized to the number of 16S rRNA gene copies. Kruskal-Wallis indicated significant effects of amendments for lettuce in terms of total ARGs (p = 0.0329); Fosfomycin (p = 0.0415); Multidrug (p = 0.0434); Peptide (p = 0.0455); Polymyxin (p = 0.0237); and Quinolone (p = 0.0455) classes. No differences across radishes noted except the Tricloson class (p = 0.049). (bottom) Rarefied to 11,800,000 reads per sample and excluding chloroplast 16S rRNA genes. Twenty different antibiotic resistance classes were represented across lettuce treatments. Kruskal-Wallis indicated no significant effects across amendment conditions, except Fosfomycin (p = 0.043) and Polymyxin (p = 0.043) for lettuce and Triclosan (p = 0.049) for radish.
Figure 3 provides a comparison of total ARGs/16S rRNA genes detected on the lettuce and radish across the four conditions, classified according to the class of antibiotic to which they encode resistance. There were only significant differences in total ARGs/16SrRNA copies detected across the four amendment conditions when considering the unrarefied lettuce data set (Kruskal-Wallis p = 0.03); no differences across radishes amendment conditions were noted. In the rarefied dataset, Kruskal-Wallis indicated no significant effects across amendment conditions, except fosfomycin (p = 0.04) and polymyxin (p = 0.04) for lettuce and triclosan (p = 0.05) for radish.
An NMDS plot of the Bray-Curtis dissimilarities produced from the relative abundances of individual ARG types/16S rRNA genes demonstrated distinct patterns between the lettuce vs. radish, as indicated by two distinct clusters for both unrarefied and rarefied data sets (Figure 4). ANOSIM of the Bray-Curtis dissimilarity matrix indicated distinct separation of the lettuce and radishes resistomes (Unrarefied Global R = 0.22, p = 0.09; Rarefied Global R = 0.30, p = 0.04). In the unrarefied dataset, amendment type across vegetables was significant (Global R = 0.27; p = 0.02), as were pairwise comparisons of antibiotic manure vs. chemical fertilizer (R = 0.48, p = 0.03), antibiotic compost vs. chemical fertilizer (R = 0.56, p = 0.02), and antibiotic manure vs. antibiotic compost (R = 0.46, p = 0.03). There were no significant differences in ARG profiles of vegetables grown in the different amendment types in the rarefied datasets (ANOSIM Global R = 0.11, p = 0.18).
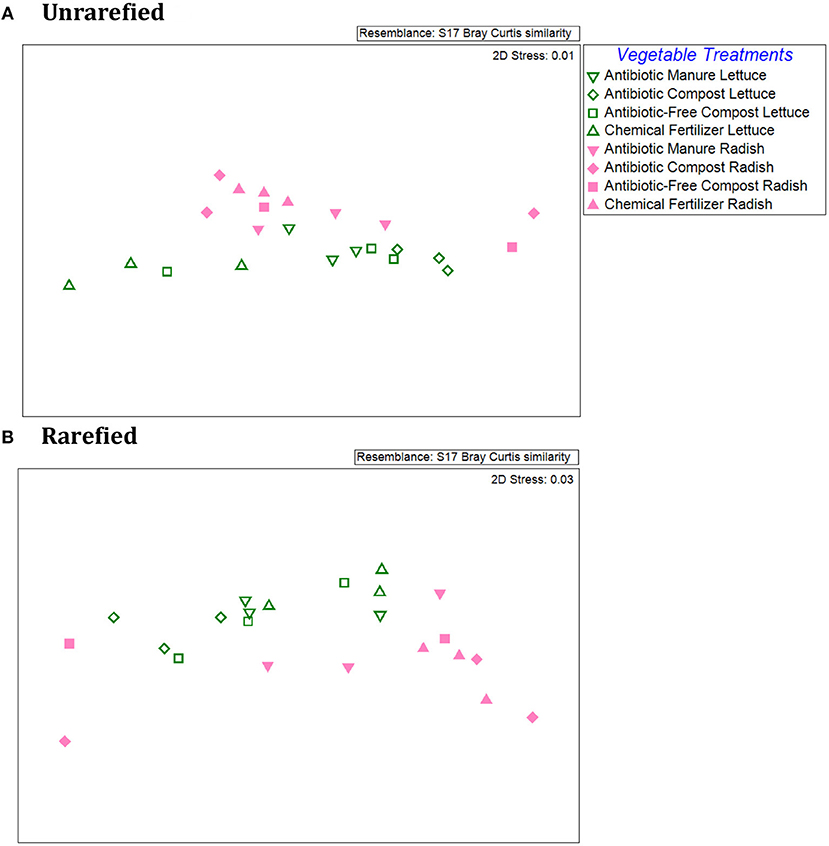
Figure 4. Comparison of lettuce and radish resistomes across amendment conditions via non-metric multidimensional scaling (NMDS) analysis of Bray Curtis similarities of the relative abundances of ARGs detected via shotgun metagenomic sequencing and comparison to CARD 1.2.1. (A) Unrarefied data without chloroplast 16S rRNA gene sequences removed, where vegetable types were weakly separated (R = 0.218; p = 0.087) and amendment conditions across both vegetable types were separated (R = 0.266; p = 0.022) (2-Way ANOSIM). Pairwise comparisons indicated separation between chemical fertilizer and antibiotic manure (R = 0.556, p = 0.02), chemical fertilizer and antibiotic compost (R = 0.418, p = 0.03), and antibiotic compost and antibiotic manure (R = 0.463, p = 0.03). (B) Rarefied data with chloroplast 16S rRNA removal. Rarefied resistomes were separable based on vegetable type (R = 0.30, p = 0.036), but lettuce or radish resistomes were not separable based on different amendment types (R = 0.1, p = 0.18).
Within vegetable type, ANOSIM of the Bray Curtis similarities of the rarefied ARG relative abundances associated with lettuce surfaces did not indicate an overall effect of amendment type (Global R = 0.17, p = 0.14; Supplemental Figure S7). However, ARG profiles of lettuce grown in antibiotic compost were significantly different from those of lettuce grown in antibiotic manure or chemical fertilizer only control plots (p = 0.10) and were separate from one another (R = 0.41, R = 0.82). With respect to radishes, ANOSIM of the Bray Curtis similarities similarly did not identify amendment type as an overall separation factor of the ARG profiles (p = 0.40, Global R = 0.02). However, there was a separation between radish samples grown in antibiotic manure vs. chemical fertilizer only control plots (R = 0.30; Supplemental Figure S7). In terms of differences corresponding to different classes of antibiotics, Kruskal-Wallis tests of unrarefied data indicated significant effects of amendments for lettuce for fosfomycin (p = 0.04); multidrug (p = 0.04); peptide (p = 0.04); polymyxin (p = 0.02); and quinolone (p = 0.05) ARG classes. In the rarefied data set, only the abundances of fosfomycin and polymyxin ARGs were significantly different for lettuce as a function of the different soil amendments (Kruskal-Wallis, p = 0.04) (Supplemental Table S5). For radishes, relative abundance of ARGs encoding resistance to triclosan was significantly different in both unrarefied and rarefied data sets (p = 0.05, p = 0.05, respectively; Supplemental Table S6).
Effect of Soil Amendment on sul1 and tet(W) Indicators of Resistance
sul1 and tet(W) gene copies were enumerated by qPCR as these genes have been identified as “indicators” of anthropogenic sources of antibiotic resistance and qPCR provides more sensitive and quantitative detection than metagenomics (Storteboom et al., 2010a,b).
Lettuce
Strikingly, lettuce grown in antibiotic manure-amended plots carried nearly 160-fold greater copies of sul1 per gram than when grown in the inorganic chemical fertilizer control (p = 0.002; Figure 5). Further, lettuce grown in antibiotic compost and antibiotic-free compost carried about 60- and 40-fold greater (p = 0.002), respectively, gene copies than lettuce grown in the chemical fertilizer control (Figure 5). Relative abundance of sul1 (i.e., per 16S rRNA gene copy) was also significantly greater for lettuce grown in antibiotic manure or antibiotic compost compared to when grown in the chemical fertilizer, by 16- and 13-fold, respectively (p = 0.01; Figure 5). Total tet(W) gene copies recovered per gram of lettuce grown in antibiotic manure-amended plots were also significantly higher than when grown in the chemical fertilizer control (p = 0.04; Figure 5). Relative abundance of tet(W) copies was also significant, but the magnitude difference was small (p = 0.024; Figure 5).
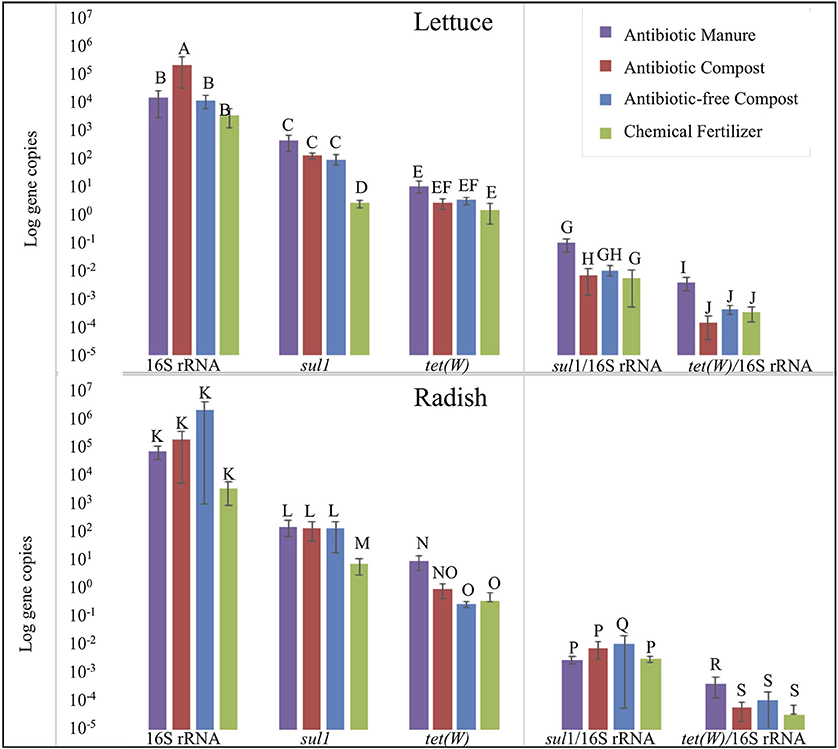
Figure 5. Effect of soil amendment on gene copy numbers recovered from lettuce (top) and radish (bottom) surfaces measured by qPCR. Wilcoxon analysis with Steel-Dwass adjustment were used to determine significance (p < 0.05). Error bars represent 1 standard error from the mean. Letters denote significant differences between soil amendment type (n = 3, except n = 2 for Radish Antibiotic compost due to lost sample).
Radish
Radish trends in carriage of sul1 and tet(W) were similar to those observed for lettuce, but somewhat lower in magnitude. Radishes carried about 22-, 20-, and 15-fold greater gene copies of sul1 per gram when grown in antibiotic manure, antibiotic compost, or antibiotic-free compost, respectively, than when grown in chemical fertilizer control (p = 0.01; Figure 5). Analysis based on relative abundance of sul1 indicated no significant effect of any of these conditions (p > 0.05; Figure 5). Total tet(W) gene copies recovered per gram of radish grown in antibiotic manure and antibiotic compost were roughly 30- (p = 0.002) and 3-fold (p = 0.01) greater, respectively, than when grown in chemical fertilizer-amended plots (Figure 5). Relative abundance of tet(W) gene copies on radishes grown in antibiotic manure-amended plots was 4-fold greater than on radishes grown in the chemical fertilizer control (p = 0.01). Radishes grown in the antibiotic compost carried about double the tet(W) relative abundance compared to the chemical fertilizer plots (p = 0.04; Figure 5).
Network Analysis Comparison of Lettuce and Radish Resistomes
Network analysis provided insight into potential for co-selection and horizontal gene transfer in terms of co-occurrence among ARGs and mobile genetic elements. Overall, 69 plasmid-associated genes, consisting of 13 unique drug classes, were observed (Figure 6). mdtB was the most frequent plasmid-associated gene in the microbial biota, whereas vat(H), a macrolide-lincosamide-streptogramin (MLS) drug, was the least frequently plasmid-associated gene in the network. For both lettuce and radish networks, ARGs corresponding to triclosan and multi-drug resistance, TriC and mdtB, respectively, were the most prominent in terms of co-occurrence with plasmid-associated genes (Figure 6). sul1 and tet(W) genes, which were found to generally be good indicators of use of manure or compost amendments according to qPCR, were not prominent in terms of co-occurrence with other ARGs or plasmid-associated genes. Each ARG and plasmid interaction within the vegetable microbiome has been visualized within Cytoscape v3.7.1 (Shannon et al., 2003) using GeneTools (scripts can be found at https://github.com/gaarangoa/genomic-scripts/tree/master/Gene Tools) via Python v3.6.0 to generate edge and node files from CARD and ACLAME databases (Supplemental Figure S8).
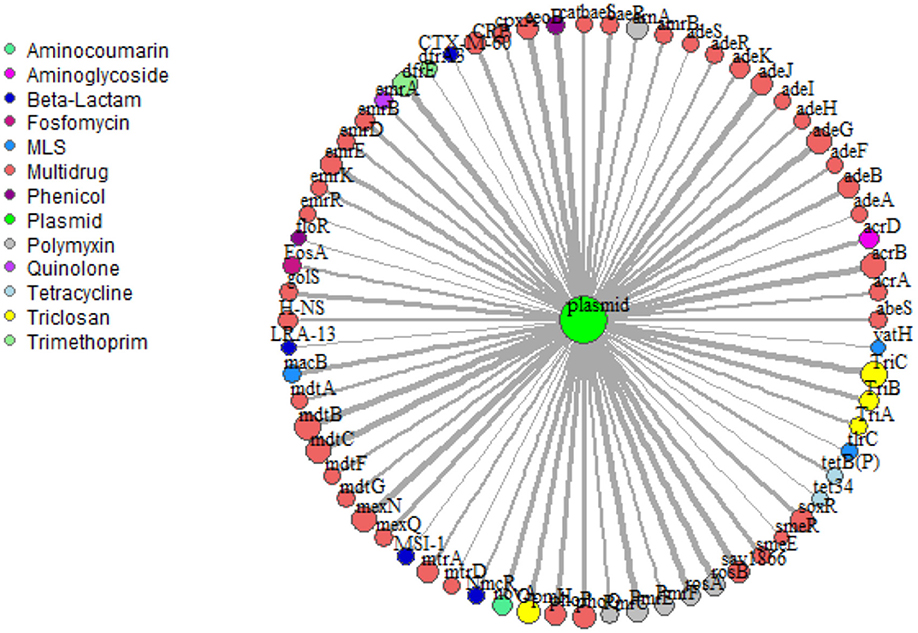
Figure 6. Network analysis predicting frequency of co-occurrence of ARGs (annotated using CARD 1.2.1) with plasmid-associated genes (annotated against ACLAME database) based on assembled metagenomic data. Relative size of node and thickness of the line is scaled according to frequency of co-occurrence. In total there were 69 plasmid-associated genes (nodes) consisting of 13 distinct drug classes. Edges were filtered to <5.
Discussion
Untreated biological soil amendments including cattle manure have been identified as significant sources of produce contamination with enteric disease-causing bacteria [Food Drug Administration (FDA), 2011]. However, concerns about spreading antibiotic resistance via vegetable cultivation and processing are relatively recent. Soil amendments could influence the bacterial microbiota and resistome associated with the vegetables through multiple avenues. First, the bacteria present in the amendments could persist in the soil and be transferred directly to the vegetables during cultivation (Rastogi et al., 2012; Leff and Fierer, 2013; Gorni et al., 2015). However, the rhizosphere and phyllosphere each represent unique environments, and different physiological traits are important in phyllosphere vs. rhizosphere bacteria, selecting for different microbial communities (Knief et al., 2012). For example, plant seeds are known to contribute to the initial population of bacteria of plants as they germinate, but the bacterial members quickly alter post-germination (Lopez-Velasco et al., 2013). The soil itself also serves as a major microbial reservoir; many of the OTUs identified from plant surfaces can be detected in the soil used for cultivation (Badri et al., 2013; Zarraonaindia et al., 2015; Allard et al., 2016). Microbes both in the biological amendments and in the soil could also hypothetically be influenced by residual antibiotics or other selective agents, which in turn influences what is effectively transferred onto the plant. In the present study, the relative abundances of the communities associated with radish (Raphanus sativus) surfaces were dominated by the same three phyla as lettuce samples; Proteobacteria, Actinobacteria, and Firmicutes (Figure 2, Supplemental Tables S1, S2), suggesting that soil could have been a major driver of the dominant populations of bacteria recovered from plant surfaces. The network analysis conducted in this study also emphasizes the likely interconnected roles of selection and horizontal gene transfer, as multi-drug resistance genes (mdtB, mexN, adeG, arcB) most commonly co-occurred with plasmid-associated genes in both lettuce and radish networks.
Characterizing Bacterial Microbiota and the Resistome by Multiple Measures
While methods exist for tracking individual antibiotic-resistant bacterial targets, of interest to this study was to more broadly capture environmental sources of antibiotic resistance across the microbial community indicative of antibiotic resistance potential. To this end, HPCs were tracked as a viable indicator of phenotypic resistance, shotgun metagenomic analysis was applied to profile ARGs across multiple classes of antibiotics and to observe their linkages to plasmid-associated genes, and qPCR was applied for more sensitive quantification of two key indicator ARGs, sul1, and tet(W). Further, 16S rRNA gene amplicon sequencing provided insight into the extent to which shifts in bacterial microbiota could be driving the observed patterns. This approach provided multi-dimensional, complimentary information about the bacterial microbiota and the resistome.
Arguably the most striking effects of the biological soil amendments were in terms of the sul1 and tet(W) gene copies. Consistent with expectation, these ARGs generally were highest in the antibiotic manure condition, lowest in the chemical fertilizer condition, and in the middle for the two compost conditions. Others have also observed these genes, particularly sul1 and the associated intI1 gene, to be strong indicators of anthropogenic sources of resistance (Gillings et al., 2015). Another striking result was the elevated numbers of clindamycin-resistant HPCs on leaves of lettuce grown in all three biological amendments, compared to the fertilizer control. Interestingly, HPCs were not elevated on either the media containing sulfamethoxazole or tetracycline. This is not necessarily unexpected given that it has been estimated that <1% of microbes residing in the rhizosphere are readily culturable (Singh et al., 2004; Delmont et al., 2015). Thus, the HPCs that happened to grow well on the R2A media probably represented a small fraction of the total bacteria captured in the 16S rRNA gene profiles and simply may not have happened to carry sul1 or tet(W). Also, the addition of antibiotics is a further selection pressure; Proteobacteria have been enriched by the addition of clindamycin when enumerating culturable bacteria from tomato surfaces (Lee et al., 2016).
sul1 and tet(W) also were not prevalent in the shotgun metagenomic sequence libraries and were not found amongst the dominant ARGs co-occurring with plasmid-associated genes in the network analysis. Metagenomic analysis detected the sul1 gene in only one of 23 field samples while qPCR detected ~ 102 gene copies. Similarly, the tet(W) gene was detected in one sample from metagenomic analyses but in all 23 samples when using qPCR. This is likely a result of the higher detection limit of metagenomics (Besser et al., 2018) and emphasizes that the resistome patterns observed here are mainly indicative of dominant ARGs (Li et al., 2015). Key aspects of metagenomic sequencing to consider include sequencing depth and data processing. While metagenomics is a very powerful tool for broadly profiling ARGs, with 640 total identified across the samples, there are no standard means of analyzing the data. Here it is emphasized that the vegetable samples are particularly challenging because careful processing is necessary to recover the very low populations of bacteria from the vegetable surface without incurring extensive chloroplast contamination from the vegetable itself. Vegetables processed in the present study were shaken and hand massaged in a solution containing a surfactant to promote removal of the cells. Other studies used pure Milli-Q water with sodium phosphate buffer and 1 min of hand massaging, which might not have promoted as much surface removal and may account for smaller number of bacteria detected in those studies (Tien et al., 2017). To account for chloroplast contamination, a rarefication approach was applied prior to analysis and compared to a more commonplace direct unrarefied analysis of the data. Interestingly, the overall trends were remarkably similar using both approaches (Figure 2). However, a number of the comparisons lost statistical significance when using the rarefied data set. Currently, there is debate regarding use of rarefied metagenomic datasets, particularly cautioning against loss of information given the expense of the data collection (McMurdie and Holmes, 2014), but vegetable metagenomics may be a special case where rarefying the data set is justified.
It is clear that there are inherent strengths and limitations for each of the above methods applied in this study for broadly capturing the effects of the cultivation conditions on vegetable resistomes. Ideally, such methods should be able to distinguish effects of different soil amendment conditions, but observed differences were relatively subtle according to all measures applied in this study. This could be because the effects truly were subtle, or because additional measures, such as culturing antibiotic-resistant strains, targeting different genes by qPCR, or deeper metagenomic sequencing would be necessary to identify stronger differences. Little is known about the effects of soil amendments on resistomes and minor effects of factors such as antibiotic administration are not necessarily unexpected, as there are numerous complex factors that contribute to the microbiota ultimately comprising the rhizosphere and phyllosphere.
Effect of Vegetable Type: Lettuce and Radish
For both the microbiota and the resistome data, the vegetable type consistently had the strongest differences among the factors tested. In β-diversity analysis the lettuce and radish samples were strongly separated, and α-diversity of lettuce appeared more sensitive to the soil amendment conditions. Interestingly, trends in carriage of sul1 and tet(W) were similar between the two vegetables and responsive to the biological amendments, suggesting that they were the primary source of these two ARGs. The bacterial communities of aerial vegetable surfaces have been fairly well-characterized (Rastogi et al., 2012; Leff and Fierer, 2013; Gorni et al., 2015), but there is a general void in research on the characterization of the microbial communities associated with the radish taproots, though communities of radish endophytes and sprouts have been studied (Seo et al., 2010; Nuesch-Inderbinem et al., 2015; Rezki et al., 2018). Radish taproots are dominated by OTUs classified in the Proteobacteria phylum; within this phylum the taproot surfaces have higher prevalence OTUs belonging to the order Psuedomonadales (up to 45%), while seeds have been shown to have higher levels of Pantoea (Rezki et al., 2018). Meanwhile, the metagenomics techniques used in this study did not allow discrimination of co-occurring ARGs and 16S rDNA sequences, the dominant group of Pseudomonadacea, comprising up to 44% of radish and 31% lettuce communities, have a high frequency of carrying class 1 integrons, such as those conferring sul1 resistance (Partridge et al., 2009). Pseudomonas spp. have been shown to increase Pseudomonas spp. populations by ten-fold in field soil (Gotz and Smalla, 1997) and to be the largest contributors of class 1 integrons and ARGs to soils after manure slurry application (Partridge et al., 2009; Leclercq et al., 2016). Pseudomonas are of further interest for future study given their relevance to multi-antibiotic resistance in clinical settings (Micek et al., 2015). Overall, lettuce displayed stronger effect of the soil amendments compared to radish, even though it had less contact with soil.
Effect of Biological Soil Amendment (Manure or Compost)
Amendment of soil with manure or compost had a clear effect on bacterial microbiota of the vegetables (e.g., β-diversity and ANOSIM) as well as carriage of sul1 and tet(W), when compared to the chemical fertilizer control. Effects of biological amendments were more subtle in examining the metagenomic data, likely owing to the detection limit. In the unrarefied dataset, amendment type across vegetables resulted in weak separation, while pairwise comparisons of antibiotic manure vs. chemical fertilizer and antibiotic compost vs. chemical fertilizer resulted in the two strongest separations. Notably, the greatest number of classes of ARGs were detected in the antibiotic manure-amended lettuce condition. Further, significant effects of amendments were noted in terms of carriage of Fosfomycin, Multidrug, Peptide, Polymyxin, and Quinolone ARG classes for lettuce and Triclosan ARGs for radish in the unrarefied data set. Interestingly, the triclosan ARG, TriC, was identified as commonly co-occurring with plasmid-associated genes (Figure 6) and thus could be particularly susceptible to horizontal gene transfer in this experiment.
Other studies of sul1 on vegetables grown in raw manure amendment have not produced as strong of a trend (Rahube et al., 2014; Tien et al., 2017). In a similar field study, manure amendment resulted in much higher concentrations (9.52 Log10 copies g) of sul1 in the soil than the vegetables, with a relatively small fraction, 2.03 Log10 copies g, transferred to carrots grown in the same plots (Tien et al., 2017). Interestingly, no sul1 genes were above the limit of detection on lettuce and radish samples grown in the same plots (Tien et al., 2017). Other studies report increases in sul1copies on carrots grown in biological amendments between 1-log−2 log (Rahube et al., 2014). The origin of the manure may also play a role in ARG presence and survival in the soil.
Effect of Composting Manure Amendment Prior to Application
Composting was of interest for its potential to mitigate effects of raw manure amendment on vegetable resistomes. The composting process transforms the bacterial community of manure with elevated heat, inactivating many enteric bacteria, and increasing populations of thermophilic bacteria (Song et al., 2014). As noted above, all biological amendments resulted in significant differences relative to the fertilizer control, but there were differences between the raw manure and compost amendments as well. For example, the bacterial microbiota of vegetables grown in antibiotic compost were distinctly separated relative to that of those grown in the corresponding antibiotic manure. While the radish samples had a significant increase in Psuedomonadaceae when grown in raw-manure amended soils, they carried roughly 40 times more OTUs identified in the order Methylophilales when grown in compost-amended soil. Methylophilales have been identified as important microbes in large-scale aerobic composting processes due to biodegradation properties of the bacteria (Liu et al., 2015). Thus, the results are consistent with the compost having influenced the microbiota of the vegetables.
There were also a few differences in the resistome noted. In the unrarefied dataset, antibiotic manure vs. antibiotic compost resulted in separation across both vegetables. Sulfonamide ARGs were also only detected on lettuce amended with antibiotic manure, not antibiotic compost. In several cases, the qPCR results also indicated greater copies of sul1 and tet(W) in the raw antibiotic manure condition than in the composting condition, overall suggesting that composting could help mitigate transfer of ARGs to vegetable surfaces.
Effects of Antibiotics Administered to the Dairy Cattle
The study design also provided insight into potential downstream effects of standard antibiotic use in dairy cattle. Notably, the dairy cows utilized in this experiment had been administered pirlimycin, a lincosamide class antibiotic that has the same mode of action as clindamycin class drugs. Interestingly, the only group of resistant HPC bacteria to be significantly increased was resistant to clindamycin. This suggests that resistant bacteria could have been selected in the gut and eventually transferred to the vegetable surface. However, it is important to point out that numbers were also elevated in the antibiotic-free compost condition, which could be a result of use in prior lactation cycles or inherited/cross-contaminated cow microbiomes. Other studies have generally noted difficulties detecting effects of biological soil amendments containing antibiotics on fresh produce using culture-based methods (Marti et al., 2013; Rahube et al., 2014; Tien et al., 2017). Consistent with the present study, Wind et al. (2018) also noted elevated clindamycin-resistant coliform bacteria in the soil of these same field plots immediately following biological amendment application.
No significant difference was detected in the overall resistome composition of radishes or lettuce grown in the antibiotic vs. antibiotic-free compost, which provides the most direct comparison of antibiotic effects in this study. Also notable, composting the manure did not reduce total ARGs carried by lettuce in the antibiotic compost condition, which still carried higher total ARGs than the chemical fertilizer condition. In contrast, total ARGs on lettuce grown in the antibiotic-free compost condition were statistically equivalent to the chemical fertilizer condition. Elfamycin and Tetracenomycin ARGs were only detected on lettuce grown soils amended with antibiotic manure and antibiotic compost, not antibiotic-free compost. This observation also brings up the point that the significant effects observed did not always correspond to the classes of antibiotics administered, which was also the situation with sul1. Significant increases in sul1 occurred on vegetables grown in antibiotic-dosed manure even though sulfonamides were not administered to dairy cows during this study, but it is possible these cows were previously treated, and resistant bacteria persisted within the gut. sul1 commonly co-occurs with other ARGs associated with mobile class 1 integrons and thus sul1 may be highly susceptible to co-selection during antibiotic administration (Gillings et al., 2015). Additionally, the co-occurrence of ARGs on plasmids identified by network analysis suggests multidrug-resistant ARGs play a role in cross-selection and co-selection of resistance to antibiotics other than those administered.
Perspectives on Sustainable Food Systems and Implications for Public Health
This study clearly demonstrates that surficial vegetable microbiota and resistomes are uniquely shaped by whether soils are amended with manure, compost, or chemical fertilizer. With respect to antibiotic resistance and public health concern, the question remains in terms of whether this represents a substantial human health risk. The metagenomic approach applied here provided broad profiling of how these factors influence a broad range of ARGs, but deeper sequencing may be necessary to identify effects on rarer, but potentially clinically important, forms of antibiotic resistance. Appropriate models for translating resistome characterization to measures of human health risk are also needed. The present study provides data that could be useful to making such a determination in the future. Judicious use of antibiotics is key both to ensuring sustainable use in agriculture in the future and protecting public health. Here, few effects of collecting the manure during antibiotic administration were observable, which could be in part because antibiotics have been used for decades in livestock and have fixed traits in the microbiome. Composting manure also had measurable effects on the surficial microbiota and resistomes compared to raw manure, but also appeared minor, with the major effect being biological soil amendment as a whole, vs. chemical fertilizer. This is also an important question for sustainable food production because biological amendments generally incur less of an environmental cost than chemical fertilizer. However, this is assuming appropriate manure management practices are implemented, which could have synergistic benefits not only for reducing pathogen loads, but also potentially as a barrier to antibiotic resistance. Still, the strongest effect was in terms of vegetable type, indicating that degree of contact with soil and potential to be eaten raw might be an important factor to consider in future recommendations.
Author Contributions
Field experiments were designed and implemented by LW, WH, L-AK, and AP. MP, KF, GG, AP, and LS designed the laboratory experiments. KF and GG performed laboratory analysis. KF, GG, LW, and IK performed data analysis. KF, GG, MP, LS, LW, and AP prepared the manuscript. All authors have agreed to the submission.
Funding
This work was supported by the USDA NIFA-AFRI 2014-05280/2015-68003-23050 awards and the Virginia Agricultural Experiment Station and the Hatch Program of the National Institute of Food and Agriculture, U.S. Department of Agriculture. The funders had no role in the study design, collection of data, analysis, or writing of this manuscript.
Conflict of Interest Statement
The authors declare that the research was conducted in the absence of any commercial or financial relationships that could be construed as a potential conflict of interest.
Acknowledgments
The authors thank Dr. Katharine Knowlton, Dr. Partha Ray, and Chrissy Teets for providing the cow-based soil amendments. The authors also thank Kyle Jacobs for the field study design and implementation and Kyle Templeton, Kim Waterman, Nicholas Poe, and Kelsey Trimble for their assistance in the laboratory. Lastly, the authors thank Dr. Pang Du, Dr. Pan Ji, and Dr. Emily Garner for assistance with data analysis.
Supplementary Material
The Supplementary Material for this article can be found online at: https://www.frontiersin.org/articles/10.3389/fsufs.2019.00022/full#supplementary-material
References
Allard, S. M., Walsh, C. S., Wallis, A. E., Ottesen, A. R., Brown, E. W., and Micallef, S. A. (2016). Solanum lycopersicum (tomato) hosts robust phyllosphere and rhizosphere bacterial communities when grown in soil amended with various organic and synthetic fertilizers. Sci. Total Environ. 573, 555–563. doi: 10.1016/j.scitotenv.2016.08.157
Aminov, R. I., Garrigues-Jeannean, N., and Mackie, R. I. (2001). Molecular ecology of tetracycline resistance: development and validation of primers for detection of tetracycline resistance genes encoding ribosomal protection proteins. Appl. Environ. Microbiol. 67, 22–32.
Arango-Argoty, G., Singh, G., Heath, L. S., Pruden, A., Xiao, W., and Zhang, L. (2016). MetaStorm: a public resource for customizable metagenomics annotation. PLoS ONE 11:e0162442. doi: 10.1371/journal.pone.0162442
Arikan, O. A., Mulbry, W., and Rice, C. (2009). Management of antibiotic residues from agricultural sources: use of composting to reduce chlortetracycline residues in beef manure from treated animals. J. Hazard. Mater. 164, 483–489. doi: 10.1016/j.jhazmat.2008.08.019
Badri, D. V., Zolla, G., Bakker, M. G., Manter, D. K., and Vivanco, J. M. (2013). Potential impact of soil microbiomes on the leaf metabolome and on herbivore feeding behavior. New Phytol. 198, 264–273. doi: 10.1111/nph.12124
Besser, J., Carleton, H. A., Gerner-Smidt, P., Lindsey, R. L., and Trees, E. (2018). Next-generation sequencing technologies and their application to the study and control of bacterial infections. Clin. Microbiol. Infect. 24, 335–341. doi: 10.1016/j.cmi.2017.10.013
Caporaso, J. G., Lauber, C. L., Walters, W. A., Berg-Lyons, D., Lozupone, C. A., Turnbaugh, P. J., et al. (2011). Global patterns of 16S rRNA diversity at a depth of millions of sequences per sample. Proc. Natl. Acad. Sci. U.S.A. 108, 4516–4522. doi: 10.1073/pnas.1000080107
Center for Disease Control (CDC) (2013). Antibiotic Resistance Threats in the United States. Available online at: https://www.cdc.gov/drugresistance/biggest_threats.html
Chambers, L., Yang, Y., Littier, H., Ray, P., Zhang, T., Pruden, A., et al. (2015). Metagenomic analysis of antibiotic resistance genes in dairy cow feces following therapeutic administration of third generation cephalosporin. PLoS ONE 10:0133764. doi: 10.1371/journal.pone.0133764
Chao, A. (1984). Non-parametric estimation of the number of classes in a population. Scand. J. Statistics 11, 265–270.
Chiesa, L., Nobile, M., Arioli, F., Britti, D., Trutic, N., Pavlovic, R., et al. (2015). Determination of veterinary antibiotics in bovine urine by liquid chromatography-tandem mass spectrometry. Food Chem. 185, 7–15. doi: 10.1016/j.foodchem.2015.03.098
Clarke, K. R., and Warwick, R. M. (2001). Change in Marine Communities: An Approach to Statistical Analysis and Interpretation. 1st Ed. Plymouth: Plymouth Marine Laboratory.
Delmont, T. O., Eren, A. M., Maccario, L., Prestat, E., Esen, Ö. C., Pelletier, E., et al. (2015). Reconstructing rare soil microbial genomes using in situ enrichments and metagenomics. Front. Microbiol. 6:00358. doi: 10.3389/fmicb.2015.00358
DeSantis, T. Z., Hugenholtz, P., Larsen, N., Rojas, M., Brodie, E. L., Keller, K., et al. (2006). Greengenes, a chimera-checked 16S rRNA gene database and workbench compatible with ARB. Appl. Environ. Microbiol. 72, 5069–5072. doi: 10.1128/AEM.03006-05
Dolliver, H., Gupta, S., and Noll, S. (2005). Antibiotic degradation during manure composting. J. Environ. Qual. 37, 1245–1253. doi: 10.2134/jeq2007.0399
Food and Drug Administration (FDA). (2015). Update on Animal Pharmaceutical Industry Response to Guidance #213. Silver Spring, MD: Food and Drug Administration.
Food and Drug Administration (FDA). (2011). Standards for the Growing, Harvesting, Packing, and Holding of Produce for Human Consumption. Docket: FDA.
Garner, E., Wallace, J. S., Argoty, G. A., Wilkinson, C., Fahrenfeld, N., Heath, L. S., et al. (2016). Metagenomic profiling of historic Colorado Front Range flood impact on distribution of riverine antibiotic resistance genes. Sci. Rep. 6, 1–10. doi: 10.1038/srep38432
Gillings, M. R., Gaze, W. H., Pruden, A., Smalla, K., Tiedje, J. M., and Zhu, Y. G. (2015). Using the class 1 integron-integrase gene as a proxy for anthropogenic pollution. ISME J. 9, 1269–1279. doi: 10.1038/ismej.2014.226
Gorni, C., Allemand, D., Rossi, D., and Mariani, P. (2015). Microbiome profiling in fresh-cut products. Trends Food Sci. Technol. 46, 295–301. doi: 10.1016/j.tifs.2015.10.013
Gotz, A., and Smalla, K. (1997). Manure enhances plasmid mobilization and survival of Pseudomonas putida introduced into field soil. Appl. Environ. Microbiol. 63, 1980–1986. doi: 10.1145/1390156.1390197
Gou, M., Hu, H. W., Zhang, Y. J., Wang, J. T., Hayden, H., Tang, Y. Q., et al. (2018). Aerobic composting reduces antibiotic resistance genes in cattle manure and the resistome dissemination in agricultural soils. Sci. Total Environ. 612, 1300–1310. doi: 10.1016/j.scitotenv.2017.09.028
Haas, B. J., Gevers, D., Earl, A. M., Feldgarden, M., Ward, D. V., Giannoukos, G., et al. (2011). Chimeric 16S rRNA sequence formation and detection in Sanger and 454-pyrosequenced PCR amplicons. Genome Res. 21, 494–504. doi: 10.1101/gr.112730.110
Heuer, H., Schmitt, H., and Smalla, K. (2011). Antibiotic resistance gene spread due to manure application on agricultural fields. Curr. Opin. Microbiol. 14, 236–243. doi: 10.1016/j.mib.2011.04.009
Jia, B., Raphenya, A. R., Alcock, B., Waglechner, N., Guo, P., Tsang, K. K., et al. (2017). CARD 2017: expansion and model-centric curation of the comprehensive antibiotic resistance database. Nucleic Acids Res. 45, D566–D573. doi: 10.1093/nar/gkw1004
Kim, S.-C., Yang, J.-E., Ok, Y.-S., Jung, D.-Y., and Carlson, K. (2012). Degradation kinetics of three veterinary antibiotics in composted and stockpiled manure. Korean J. Soil Sci. Fertil. 45, 43–50. doi: 10.7745/KJSSF.2012.45.1.043
Knief, C., Delmotte, N., Chaffron, S., Stark, M., Innerebner, G., and Wassmann, R. (2012). Metaproteogenomic analysis of microbial communities in the phyllosphere and rhizosphere of rice. ISME J. 6, 1378–1390. doi: 10.1038/ismej.2011.192
Langmead, B., and Salzberg, S. L. (2012). Fast gapped-read alignment with Bowtie 2. Nat. Methods 9, 357–9. doi: 10.1038/nmeth.1923
Leclercq, S. O., Wang, C., Sui, Z., Wu, H., Zhu, B., Deng, Y., et al. (2016). A multiplayer game: species of Clostridium, Acinetobacter, and Pseudomonasare responsible for the persistence of antibiotic resistance genes in manure-treated soils. Environ. Microbi. 8, 3494–3508. doi: 10.1111/1462-2920.13337
Lee, S. A., Park, J., Chu, B., Kim, J. M., Joa, J.-H., and Sang, M. K. (2016). Comparative analysis of bacterial diversity in the rhizosphere of tomato by culture-dependent and -independent approaches. J. Microbiol. 54, 823–831. doi: 10.1007/s12275-016-6410-3
Leff, J. W., and Fierer, N. (2013). Bacterial communities associated with the surfaces of fresh fruits and vegetables. PLoS ONE 8, 1–9. doi: 10.1371/journal.pone.0059310
Lhermie, G., Gröhn, Y. T., and Raboisson, D. (2017). Addressing antimicrobial resistance: an overview of priority actions to prevent suboptimal antimicrobial use in food-animal production. Front. Microbiol. 7, 1–11. doi: 10.3389/fmicb.2016.02114
Li, B., Yang, Y., Ma, L., Ju, F., Guo, F., Tiedje, J. M., et al. (2015). Metagenomic and network analysis reveal wide distribution and co-occurrence of environmental antibiotic resistance genes. ISME J. 9, 2490–2502. doi: 10.1038/ismej.2015.59
Liu, D., Li, M., Xi, B., Zhao, Y., Wei, Z., Song, C., et al. (2015). Metaproteomics reveals major microbial players and their biodegradation functions in a large-scale aerobic composting plant. Microb. Biotechnol. 8, 950–960. doi: 10.1111/1751-7915.12290
Lopez-Velasco, G., Carder, P. A., Welbaum, G. E., and Ponder, MA. (2013). Diversity of the spinach (Spinacia oleracea) spermosphere and phyllopshere bacterial communities. FEMS Microbiol. Lett. 346, 146–54. doi: 10.1111/1574-6968.12216
Lozupone, C., and Knight, R. (2005). UniFrac: a new phylogenetic method for comparing microbial communities UniFrac: a new phylogenetic method for comparing microbial communities. Appl. Environ. Microbiol. 71, 8228–8235. doi: 10.1128/AEM.71.12.8228
Marti, R., Scott, A., Tien, Y. C., Murray, R., Sabourin, L., Zhang, Y., et al. (2013). Impact of manure fertilization on the abundance of antibiotic-resistant bacteria and frequency of detection of antibiotic resistance genes in soil and on vegetables at harvest. Appl. Environ. Microbiol. 79, 5701–5709. doi: 10.1128/AEM.01682-13
Masella, A. P., Bartram, A. K., Truszkowski, J. M., Brown, D. G., and Neufeld, J. D. (2012). PANDAseq: PAired-eND Assembler for Illumina sequences. BMC Bioinformatics 13:31. doi: 10.1186/1471-2105-13-31
McMurdie, P. J., and Holmes, S. (2014). Waste not, want not: why rarefying microbiome data is inadmissible. PLoS Comput. Biol. 10:e1003531. doi: 10.1371/journal.pcbi.1003531
Meek, R. W., Vyas, H., and Piddock, L. J. (2015). Nonmedical uses of antibiotics: time to restrict their use? PLoS Biol. 13, 1–11. doi: 10.1371/journal.pbio.1002266
Micek, S. T., Wunderink, R. G., Kollef, M. H., Chen, C., Rello, J., Chastre, J., et al. (2015). An international multicenter retrospective study of Pseudomonas aeruginosa nosocomial pneumonia: impact of multidrug resistance. Crit. Care 19, 1–8. doi: 10.1186/s13054-015-0926-5
Nuesch-Inderbinem, M., Zurfluh, K., Pheterhans, S., Hachler, H., and Stephan, R. (2015). Assessment of the prevalence of extended-spectrum β-lactamase–producing Enterobacteriaceae in ready-to-eat salads, fresh-cut fruit, and sprouts from the swiss market. J. Food Protect. 78, 1178–1181. doi: 10.4315/0362-028X.JFP-15-018
O'Neill, J. (2014). Antimicrobial Resistance: Tackling a Crisis for the Health and Wealth of Nations. Available online at: http://amrreview.org/Publications (accesed January 19, 2018).
Partridge, S. R., Tsafnat, G., Coiera, E., and Iredell, J. R. (2009). Gene cassettes and cassette arrays in mobile resistance integrons: Review article. FEMS Microbiol. Rev. 33, 757–784. doi: 10.1111/j.1574-6976.2009.00175.x
Pei, R., Kim, S.-C., Carlson, K. H., and Pruden, A. (2006). Effect of river landscape on the sediment concentrations of antibiotics and corresponding antibiotic resistance genes (ARG). Water Res. 40, 2427–2435. doi: 10.1016/j.watres.2006.04.017
R Core Team (2016). R: A Language and Environment for Statistical Computing. Vienna: R Foundation for Statistical Computing. Available online at: http://www.R-project.org/
Rahube, T. O., Marti, R., Scott, A., Tien, Y. C., Murray, R., Sabourin, L., et al. (2014). Impact of fertilizing with raw or anaerobically digested sewage sludge on the abundance of antibiotic-resistant coliforms, antibiotic resistance genes, and pathogenic bacteria in soil and on vegetables at harvest. Appl. Env. Microbiol. 80, 6898–6907. doi: 10.1128/AEM.02389-14
Rastogi, G., Sbodio, A., Tech, J. J., Suslow, T. V., Coaker, G. L., and Leveau, J. H. (2012). Leaf microbiota in an agroecosystem: spatiotemporal variation in bacterial community composition on field-grown lettuce. ISME J. 6, 1812–1822. doi: 10.1038/ismej.2012.32
Ray, P., Chen, C., Knowlton, K. F., Pruden, A., and Xia, K. (2017). Fate and Effect of Antibiotics in beef and dairy manure during static and turned composting. J. Environ. Qual. 46:45. doi: 10.2134/jeq2016.07.0269
Ray, P., Knowlton, K. F., Shang, C., and Xia, K. (2014a). Development and validation of a UPLC-MS/MS method to monitor Cephapirin excretion in dairy cows following intramammary infusion. PLoS ONE 9:112343. doi: 10.1371/journal.pone.0112343
Ray, P., Knowlton, K. F., Shang, C., and Xia, K. (2014b). Method development and validation: solid phase extraction-ultra performance liquid chromatography-tandem mass spectrometry quantification of pirlimycin in bovine feces and urine. J. AOAC Int. 97, 1730–1736. doi: 10.5740/jaoacint.14-040
Reganold, J. P., and Wachter, J. M. (2016). Organic agriculture in the twenty-first century. Nat. Plants 2:15221. doi: 10.1038/nplants.2015.221
Rezki, S., Campion, C., Simoneau, P., Jacques, M. A., Shade, A., and Barret, M. (2018). Assembly of seed-associated microbial communities within and across successive plant generations. Plant Soil 422, 67–79. doi: 10.1007/s11104-017-3451-2
Rognes, T., Flouri, T., Nichols, B., Quince, C., and Mahé, F. (2016). VSEARCH: a versatile open source tool for metagenomics. PeerJ 4:e2584. doi: 10.7717/peerj.2584
Seo, W. T., Lim, W. J., Kim, E. J., Yun, H. D., Lee, Y. H., and Cho, K. M. (2010). Endophytic bacterial diversity in the young radish and their antimicrobial activity against pathogens. J. Appl. Biol. Chem. 53, 493–503. doi: 10.3839/jksabc.2010.075
Shannon, C. E., and Weaver, W. (1949). The Mathematical Theory of Communication. Urbana, IL: University of Illinois Press.
Shannon, P., Markiel, A., Ozier, O., Baliga, N. S., Wang, J. T., Ramage, D., et al. (2003). Cytoscape: a software environment for integrated models of biomolecular interaction. Networks 13, 2498–2504. doi: 10.1101/gr.1239303.metabolite
Singh, B. K., Millard, P., Whiteley, A. S., and Murrell, J. C. (2004). Unravelling rhizosphere-microbial interactions: opportunities and limitations. Trends Microbiol. 12, 386–393. doi: 10.1016/j.tim.2004.06.008
Song, C., Li, M., Jia, X., Wei, Z., Zhao, Y., Xi, B., et al. (2014). Comparison of bacterial community structure and dynamics during the thermophilic composting of different types of solid wastes: anaerobic digestion residue, pig manure and chicken manure. Microb. Biotechnol. 7, 424–433. doi: 10.1111/1751-7915.12131
Storteboom, H., Arabi, M., Davis, J. G., Crimi, B., and Pruden, A. (2010a). Identification of antibiotic-resistance-gene molecular signatures suitable as tracers of pristine River, urban, and agricultural sources. Environ. Sci. Technol. 44, 1947–1953. doi: 10.1021/es902893f
Storteboom, H., Arabi, M., Davis, J. G., Crimi, B., and Pruden, A. (2010b). Tracking antibiotic resistance genes in the south platte river basin using molecular signatures of urban, agricultural, and pristine sources. Environ. Sci. Technol. 44, 7397–7404. doi: 10.1021/es101657s
Tien, Y. C., Li, B., Zhang, T., Scott, A., Murray, R., Sabourin, L., et al. (2017). Impact of dairy manure pre-application treatment on manure composition, soil dynamics of antibiotic resistance genes, and abundance of antibiotic-resistance genes on vegetables at harvest. Sci. Total Environ. 581–582, 32–39. doi: 10.1016/j.scitotenv.2016.12.138
Udikovic-Kolic, N., Wichmann, F., Broderick, N. A., and Handelsman, J. (2014). Bloom of resident antibiotic-resistant bacteria in soil following manure fertilization. Proc. Natl. Acad. Sci. U.S.A. 111, 15202–15207. doi: 10.1073/pnas.1409836111
Van Boeckel, T. P., Brower, C., Gilbert, M., Grenfell, B. T., Levin, S. A., Robinson, T. P., et al. (2015). Global trends in antimicrobial use in food animals. Proc. Natl. Acad. Sci. 112, 5649–5654. doi: 10.1073/pnas.1503141112
Virginia Cooperative Extension (2015). Commercial Vegetable Production Recommendations. Blacksburg, VA: Virginia Publications.
Walters, W., Hyde, E. R., Berg-Lyons, D., Ackermann, G., Humphrey, G., Parada, A., et al. (2016). Improved bacterial 16s rrna gene (v4 and v4-5) and fungal internal transcribed spacer marker gene primers for microbial community surveys. mSystems 1, e00009–e00015. doi: 10.1128/mSystems.00009-15
Wang, F. H., Qiao, M., Chen, Z., Su, J. Q., and Zhu, Y. G. (2015). Antibiotic resistance genes in manure-amended soil and vegetables at harvest. J. Hazard. Mater. 299, 215–221. doi: 10.1016/j.jhazmat.2015.05.028
Wepking, C., Avera, B., Badgley, B., Barrett, J. E., Franklin, J., Knowlton, K. F., et al. (2017). Exposure to dairy manure leads to greater antibiotic resistance and increased mass-specific respiration in soil microbial communities. Proc. R. Soc. B Biol. Sci. 284:20162233. doi: 10.1098/rspb.2016.2233
Wind, L., Krometis, L.-A., Hession, W. C., Chen, C., Du, P., Jacobs, K., et al. (2018). Fate of pirlimycin and antibiotic-resistant fecal coliforms in field plots amended with dairy manure or compost during vegetable cultivation. J. Environ. Qual. 47, 436–444. doi: 10.2134/jeq2017.12.0491
World Health Organization (WHO) (2011). Critically Important Antimicrobials for Human Medicine. 3rd Ed. Geneva: World Health Org. Doc. Prod. Serv.
Wright, G. D. (2007). The antibiotic resistome: the nexus of chemical and genetic diversity. Nat. Rev. Microbiol. 5, 175–186. doi: 10.1038/nrmicro1614
Keywords: antibiotic resistance genes, phyllosphere bacteria, metagenomics, vegetable production, network analysis
Citation: Fogler K, Guron GKP, Wind LL, Keenum IM, Hession WC, Krometis L-A, Strawn LK, Pruden A and Ponder MA (2019) Microbiota and Antibiotic Resistome of Lettuce Leaves and Radishes Grown in Soils Receiving Manure-Based Amendments Derived From Antibiotic-Treated Cows. Front. Sustain. Food Syst. 3:22. doi: 10.3389/fsufs.2019.00022
Received: 18 January 2019; Accepted: 18 March 2019;
Published: 10 April 2019.
Edited by:
Ömer Akineden, University of Giessen, GermanyReviewed by:
Jaak Truu, University of Tartu, EstoniaWaleed Abu Al-Soud, Al Jouf University, Saudi Arabia
Copyright © 2019 Fogler, Guron, Wind, Keenum, Hession, Krometis, Strawn, Pruden and Ponder. This is an open-access article distributed under the terms of the Creative Commons Attribution License (CC BY). The use, distribution or reproduction in other forums is permitted, provided the original author(s) and the copyright owner(s) are credited and that the original publication in this journal is cited, in accordance with accepted academic practice. No use, distribution or reproduction is permitted which does not comply with these terms.
*Correspondence: Amy Pruden, YXBydWRlbkB2dC5lZHU=
Monica A. Ponder, bXBvbmRlckB2dC5lZHU=