- 1International Food Policy Research Institute, Environment and Production Technology Division, Washington, DC, United States
- 2Argonne National Laboratory, Energy Systems Division, Lemont, IL, United States
- 3International Union for Conservation of Nature, Landscape Restoration Science and Knowledge in the Global Forest and Climate Change Programme, Washington, DC, United States
Existing approaches and methodologies that investigate effects of land degradation on food security vary greatly. Although a relatively rich body of literature that investigates localized experiences, geophysical, and socioeconomic drivers of land degradation, and the costs and benefits of avoiding land degradation already exists, less rigorously explored are the global effects of restoring degraded landscapes for the health of the land, the climate, and world food security. The current scale of land degradation is such that the problem can be meaningfully addressed only if local successes are upscaled and a large number of landowners and land managers implement restoration activities. Significant global efforts to address degradation exist, but studies that evaluate the global benefits of these efforts generally do not account for global market forces and the complex web of relationships that determine the effects of wide-scale restoration on production and food security. This paper provides important insights into how a full integration of crop production in restoration efforts could impact food production levels, food availability, forest carbon stocks, and Greenhouse gas emissions.
Introduction
Land degradation has multiple and complex impacts on the global environment through a range of direct and indirect processes that affect a wide range of ecosystem services and livelihoods (Nkonya et al., 2016). Estimates indicate that the global cost of land degradation due to land-use change and to the use of land-management practices that negatively impact the “health of land” is about US$300 billion annually (Nkonya et al., 2016). Between, 1997; Between, 2001, losses due to global land-use changes were estimated at US$4.3–US$20.2 billion per year (Costanza et al., 2014; Suding et al., 2015). Some estimates indicate that land degradation has reduced productivity on 23% of the global land surface (Díaz et al., 2019) and that more than 3.2 billion people are affected by it (United Nations Convention to Combat Desertification (UNCCD), 2017). It has also been estimated that if the current pace of land degradation were to continue, it could reduce global food production by as much as 12 percent and increase the price of some commodities by as much as 30 percent during the period 2010–2030 (IFPRI, 2012).
Even though land and soil degradation is widespread and occurs globally (Nkonya et al., 2011, 2016), research and projects have focused mostly on arid, semiarid, and dry subhumid areas, with particular attention paid to the susceptibility of such ecosystems to desertification (Lu et al., 2007). Research and on-the-field activities to prevent forest degradation and promote restoration have instead concentrated mostly on tropical forests, where important changes are taking place. The magnitude of these changes is important: ~100 million hectares of tropical forest were converted to farmland between 1980 and 2012 (Gibbs et al., 2010; Hansen et al., 2013), and between 2000 and 2005 selective logging was estimated to have affected about 20 percent of tropical forests (Asner et al., 2005). Only a minority of the forests remains as intact forest landscape1 (Potapov et al., 2008). Hansen et al. (2011) report that across the world, 24 percent of the existing tropical forests are intact, 46 percent are fragmented, and 30 percent are degraded. Tropical deforestation, in addition to biodiversity loss and soil degradation, is also responsible for significant amounts of greenhouse gas (GHG) emissions (Henders et al., 2015; Busch et al., 2019).
The scale of degradation and the magnitude of its effects is such that it can be meaningfully addressed only if large numbers of landowners and land managers become involved in restoration activities. Not surprisingly, the international community has been engaged in halting degradation and pushing forward the landscape restoration agenda for decades. The United Nations Convention to Combat Desertification (UNCCD) established in 1994, has led international efforts that aim at reversing and preventing desertification and land degradation. More recently the Bonn Challenge was launched (September 2011) (Bonn Challenge, 2020). It is a global effort that aims at bringing 150 million hectares of the world's deforested and degraded lands into restoration by 2020. The Bonn Challenge was later extended with the New York Declaration on Forests of the 2014 UN Climate Summit, expanding the goal to 350 million hectares by 2030. These efforts also provide an avenue to meet other existing international commitments and agreements, including the Aichi Targets, the UNFCCC REDD+ goal, the Rio+20 Land Degradation Neutrality (LDN) goal, several of the Sustainable Development Goals (SDGs), and the climate change mitigation goals in the Paris Agreement. Given the significant role that the Bonn Challenge plays in addressing degradation globally, we use its restoration commitment (of bringing 350 million hectares of degraded and deforested land into restoration by 2030) as a benchmark for this study.
Significant forces have impeded the progress and the achievement of land and forest restoration goals even though its benefits are well-documented (Chazdon et al., 2017). Too often large-scale restoration is not practiced by communities living in degraded landscapes, although localized demand-driven success stories do exist (Keil et al., 2005; Brown et al., 2009; Reij et al., 2009; Garrity et al., 2010; Lambert and Ozioma, 2012; Matocha et al., 2012; Calle et al., 2013; Winterbottom et al., 2013; Deichert et al., 2014; Kabwe et al., 2016). It has been observed that many national forest strategies have focused exclusively on how to best manage and protect intact areas of forest (Chazdon, 2018) and that when national forest programs and strategies have recognized restoration as a priority, they have tended to concentrate their restoration activities on the establishment of industrial plantations (Rietbergen-McCracken et al., 2007; Lewis et al., 2019). This focus on forests has alienated local communities (Rietbergen-McCracken et al., 2007) while it is well-known that widespread adoption of restoration practices is possible only if landowners, farmers, and land managers benefit from it and have sufficient stakeholder support (Sengupta et al., 2005).
More holistic methods to promote conservation and restoration have been proposed (Schultz et al., 2012; Chazdon, 2018). The Forest Landscape Restoration (FLR) approach is one of them (IUCN WRI, 2014). FLR promotes sustainable forest management, reforestation and regeneration while creating an active role for agriculture. The key tenet of FLR is that a combination of forest and non-forest ecosystems, land uses, and restoration approaches can be accommodated within a landscape to achieve sustainable food production, improve the provisioning of ecosystem services, and preserve biodiversity. Evidence already shows that landscape-level interventions can enhance the provision of ecosystem services and support the functionality of agriculture landscapes (Harvey et al., 2014). Farmers' managed natural regeneration has shown promising results on many fronts. From the management of trees on farmlands and the conservation of soils and water, to the regeneration of natural vegetation with consequent income diversification and enhancement of the adaptive capacity to droughts (Reij et al., 2005, 2009; Garrity et al., 2010; Harvey et al., 2014). Agroforestry, home gardens and silvopastoral systems in productive landscapes allow stakeholders to increase vegetation cover, protect forest remnants, generate wildlife habitats, and conserve and recover soil at the landscape scale (Calle et al., 2014; Mungai et al., 2016). The active role of agriculture in such restoration efforts is expected to induce direct participation of communities, thereby reducing the observed opposition to large-scale restoration projects (Rietbergen-McCracken et al., 2007). Furthermore, population growth, changing diets, and climate change create serious risks to the capacity of agricultural production systems to satisfy an increased demand for food products. This makes including agricultural land in restoration effort not only desirable but also a necessity.
Given the key role that agricultural land and crop production play in the FLR approach, this study sets out to evaluate the potential global effects of achieving the Bonn Challenge through a full inclusion of crop production in restoration efforts. We are particularly interested in assessing the potential conflicts between the implementation of restoration on a global scale and food security. Because our perspective is global, the modeling approach must account for market forces and the complex web of relationships that determine land allocations, food prices, and food availability. To accomplish this, we focus on some aspects of food-crop production that can be modeled globally with a reasonable level of accuracy given the latest developments in modeling capabilities (Nelson et al., 2014; Rosegrant et al., 2014; Rosenzweig et al., 2014; Islam et al., 2016). Our ex-ante analysis considers a group of agricultural practices (no tillage, integrated soil fertility management, and nitrogen-use efficiency) that have the potential to be adopted widely and are known to restore soil fertility and promote sustainable production. These alternative practices require specific modifications and adjustments on the ground and as a consequence, the modeling work presented here is a stylized representation of a range of many technologies and practices that would have to be identified by farmers to best fit their local conditions.
Nevertheless, in this study we provide important insights into how a full integration of crop production into restoration efforts could impact globally food production, food security and other environmentally significant metrics. While the magnitude of the effects depends on the emphasis that countries put on forest restoration vs. cropland restoration, results indicate positive outcomes in terms of production, reduced number of undernourished people, decreased GHG emissions and increased carbon stock. The countries supporting the Bonn Challenge and other restoration efforts should renew their efforts confident that it is possible to meet their commitments without jeopardizing their food security goals.
The Problem of Adoption of Restoration Practices and Participation in Restoration Efforts
Landscape restoration efforts face challenges in reaching sufficiently large implementation levels to make a difference globally. This is due to the lack of understanding of the operational complexities of dealing with landscapes and the perceived conflicts with the most pressing needs of stakeholders (Chazdon et al., 2017; Reinecke and Blum, 2018). On the one hand, the involvement of agricultural land in restoration efforts facilitates the engagement and active role of communities. On the other hand, restoration of agricultural land faces barriers and limits in uptake, just like forest restoration. For the restoration of degraded cropland, economic factors (e.g., availability of labor force and mechanization) might make restoration a non-viable proposition under some conditions. Furthermore, considerations related to the opportunity costs of locking valuable land into restoration programs may make these activities unattractive options.
The literature on participation in forest restoration activities and on adoption of restoration practices tells similar stories about the difficulties of changing the status quo. Insecure property rights and land and tree tenure in particular make rural households unwilling or unable to invest in potentially risky restoration activities (Bewket, 2007; Enfors and Gordon, 2008; Shiferaw et al., 2009; Nkonya et al., 2011; Teklewold and Kohlin, 2011; Bryan et al., 2013; Mungai et al., 2016). Even in successful cases, such as the ones reported by Lamb et al. (2005), key limitations are found in the high implementation costs and the limited capacity to supply significant volumes of commercially useful goods (Erskine, 2002).
Adoption rates of restoration and conservation practices (whether on agricultural lands or other more complex agroecosystems) can vary substantially according to the local geophysical and socioeconomic conditions. Keil et al. (2005) and Kabwe et al. (2009) reported an adoption rate of improved fallows among experimenting farmers in eastern Zambia of ~75 percent. However, a study on the adoption of improved agroforestry technologies among contract farmers in Imo, Nigeria, reported a mean adoption rate of about 34 percent and a study on multiple agricultural technologies in Nepal (Floyd et al., 2003), the mean adoption rate was 31 percent. Adoption rates of silvopastoral systems have remained relatively low and these systems are still not adopted on a wide scale (Dagan and Nair, 2003; Calle et al., 2009).
Moreover, adoption rates of agricultural practices known to improve soil health, reduce negative externalities and increase yields and profits are also often low and vary widely. Floyd et al. (2003) found that adoption was highest (40–60 percent) for the technologies of improved maize, wheat and grain legume varieties, improved tree fruit crops, and the planting of fodder trees. Intermediate levels of adoption (10–30 percent) were found for alternative technologies implemented on rice, finger millet, potato, and barley varieties; crossbreeding of cattle and buffalo; parasitic drenching of livestock; and improved forage species. Adoption levels were low (3–10 percent) for the technologies that targeted vegetables and vegetable seed production. In a recent study, Rosegrant et al. (2014) identified adoption ceilings for a wide variety of agricultural management practices and technologies ranging from 16 percent to 80 percent.
When the objective of a program is to implement restoration on a given amount of land area, such as the case of the Bonn Challenge, the rate of adoption of restoration practices and actual number of farmers participating in restoration programs has important implications. The lower the number of people participating in restoration activities per unit of area, the larger the area targeted by a program must be. This has direct effects on implementation costs but also on the type of services provided (e.g., food crop production, carbon stock, and other ecosystem services) these being dependent on the characteristics of the land that is ultimately restored. In addition, countries have different endowments of forested, degraded, and productive agricultural land and it is difficult to decide a priori how and where governments will decide to meet their restoration targets. These uncertainties determine the approach followed in this study.
Materials and Methods
Our analysis focuses on three widely grown crops: maize (Zea mays), wheat (Triticum aestivum), and rice (Oryza sativa). These three crops represent about 41% of the global harvested area and ~64% of the estimated 2–3 Gt CO2e per year emitted by crop production globally (Carlson et al., 2017). Although our focus is on crop production, we must acknowledge the important role of livestock in people's livelihoods. The reason the livestock sector is not included in this study is because at this time we do not have access to a global modeling tool that provides the same level of accuracy as the one we can reach in representing crop production.
The analysis is based on a comparison of a business-as-usual (BAU) scenario without restoration, with a series of alternative scenarios that include restoration of degraded land. The latter require that the tenets of the FLR approach are satisfied, i.e., restoration must occur on a mix of land uses which must include forest and agricultural land. All scenarios, including BAU, share the same assumptions regarding GDP, population, and intrinsic country and crop specific agricultural productivity growth (see Robinson et al., 2015 for details on these assumptions). Specifically, scenarios use the population and income growth assumptions that underly the middle of the road Shared Socioeconomic Pathway (O'Neill et al., 2014; SSP2) from the Intergovernmental Panel on Climate Change Fifth Assessment Report (IPCC AR5; Clarke et al., 2014). Under the SSP2 narrative, global population will reach over 9 billion people by 2050 at an average annual growth rate of 0.6% and per capita GDP grows at just below 2% per year.
All scenarios are simulated under climate change conditions. Climate change projections are generated through two global circulation models—GFDL-ESM2M (Dunne et al., 2012) and HadGEM2-ES (Jones et al., 2011)—under the Representative Concentration Pathway 8.5 (RCP 8.5; Meinshausen et al., 2011). The GFDL climate model can be considered as drier and cooler than HadGEM (See Supplementary Material Section 2 and Table S1).
Modeling Steps
To characterize the involvement of agriculture in restoration efforts and to assess the impact on production and food security of restoring degraded cropland, four datasets and two models were used. The datasets are: the global estimates of land allocations to crop production by You et al. (2014) (Spatial Production Allocation Model, SPAM), the Moderate Resolution Imaging Spectroradiometer (MODIS) global land cover data (Friedl et al., 2010; Channan et al., 2014), the global assessment of degradation hotspots (Bao et al., 2016), and the global assessment of forest aboveground biomass by Hu et al. (2016). The models used are: the Decision Support System for Agrotechnology Transfer (DSSAT; Jones et al., 2003) and the International Model for Policy Analysis of Agricultural Commodities and Trade (IMPACT, Robinson et al., 2015, see Supplementary Material Sections 1, 3 for details on the models and on the calibration steps).
At the center of our analysis is the IMPACT system of models (Robinson et al., 2015; Islam et al., 2016) which links crop and climate models to the economic model that provides a representation of agricultural production across 320 sub-national regions called “food production units” (FPUs, see Supplementary Material Section 1). Because of the linkages among these models, IMPACT's outputs reflect the interactions between biophysical, economic and population trends, the combination of which represents the functioning of the global food market.
The modeling develops as follows and it is applied to both climate models. First, a BAU scenario is generated. This scenario assumes that farmers retain current agricultural practices and do not use any restoration measures on their land. Location and classification of current cropland areas are given by the SPAM model and, based on these areas, the DSSAT model generates estimates for current yields. These are passed to the IMPACT model, which then generates projections for agricultural production, commodity prices and number of undernourished people (See Supplementary Material Section 1 for a description of the model, and of the estimation of people at risk of hunger). After the BAU, the alternative scenarios are created. The candidate areas for FLR and the amount of cropland involved are first identified using maps of degradation hotspots (Bao et al., 2016) and crop allocations (SPAM). Then, DSSAT is used to compute the yield effects of adopting restoration practices within these areas. The new yields are used in the IMPACT model to assess the global impact on production, commodity prices, and number of undernourished people all else being equal. Finally, the results are compared with BAU to assess the effects of adopting the alternative practices. A stylized representation of the modeling steps and of the information flow is provided in Figure 1. The process represented in the figure shows the comparison between the BAU and one alternative scenario. The same process is followed for all alternative scenarios.
The effects of restoration activities on forested areas are estimated separately by assessing the potential gains in biomass attributable to healthy forest after restoration.
Given the uncertainty regarding how and where governments will decide to meet their restoration targets, we assume that restoration can proceed following two different pathways, one that prioritize cropland restoration and the other that favors forest restoration. In addition, we are aware that the rate of participation in restoration efforts by farmers can profoundly affect the results. Therefore, the impact of alternative participation rates is simulated. All scenarios were evaluated for the period 2010–2030 and in additions to production and prices, the effects on carbon stock and GHG emissions were also assessed.
What follows describes in more detail the scenarios, each component of our modeling approach and its function.
The Location of Degraded Land and Candidate Areas for Forest Landscape Restoration
Given the extent of land degradation, the damages caused by it and the potential benefits that derive from restoring degraded land (Nkonya et al., 2016), we concentrated our analysis on agro- and forest ecosystems that are deemed to be degrading2. Bao et al. (2016) used long-term trends of biomass productivity as a proxy for land degradation and provided a global assessment of the areas subjected to a high degree of degradation. These long-term trends are based on changes in the Normalized Difference Vegetation Index (NDVI) derived from satellite images for 1982–2006. In a recent review of the prominent databases and methodologies used to estimate global degraded land undertaken, Gibbs and Salmon (2015) found that all approaches come with strengths and weaknesses; however, methods based on satellite observations have the advantage of being globally consistent, including all land uses and land covers, and being based on observed (rather than estimated or potential) changes in land productivity. Bao et al. (2016) correct for several factors that could potentially confound the relationship between the remotely sensed vegetation index and land-based biomass productivity and find that degradation is widespread in all agroecological zones and that it affects about 2.6 billion hectares, or about 29 percent of global land area.
Although substantial amounts of degradation are detected in forests (~798 million hectares), 638 million hectares of degraded land are estimated to be in areas where crop production takes place. Table 1 reports the 10 most represented crops present in the area that Bao et al. (2016) identified as significantly degraded. Most of them are annual crops (wheat, rice, and maize represent ~42 percent of the cropland present in degraded areas); tree crops are present in <4 percent of the area assessed as degraded.
It is worth noting that a significant amount of degradation is also estimated to be occurring on grasslands and shrublands (a total of 670 million hectares), with potentially significant negative impacts on the livelihoods and well-being of pastoralist communities. Because Bao et al. (2016) identified areas with high magnitude and extent of degradation (hotspots), they likely underestimated the full extent of degradation.
In our model, the areas targeted for the FLR efforts must be classified as degraded and contain both forests and cropland. This is accomplished by intersecting the Bao et al. degradation hotspots, the MODIS data to identify the location of forests, the SPAM data for the location of crop production. Our unit of analysis is the SPAM grid-cell which is of size 0.5 degrees (a square of ~50 km by 50 km at the equator, See Supplementary Material Section 1, the SPAM model). MODIS data has the same resolution as SPAM while the Bao et al. data, originally with cells of size 0.07 degrees, is aggregated to obtain the same resolution. Areas that are too remote3 or that do not have recorded agricultural activities are not considered to be candidates for the FLR approach.
Crop Production and Changes in GHG Emissions
The ex-ante assessment of crop yields under different scenarios (BAU and alternatives) is based on the use of DSSAT (Jones et al., 2003). DSSAT integrates the effects of biophysical elements of the crop systems (interaction among soil, weather, and crop) and management options (the type of tillage, nutrient application, and water availability) to simulate production outcomes originating from interactions among the components of the cropping system. High-resolution data about climate scenarios, irrigation type, and soil properties were geo-linked to each SPAM grid-cell. Therefore, crop growth and yields are driven by spatially explicit climate scenarios, soil properties, and management practice, simulated for the entire globe at the grid-cell level.
After completion of a calibration step (See Supplementary Material Section 3, DSSAT Calibration) these yield responses were aggregated to evaluate agriculture production across IMPACT's FPUs. Yield responses together with harvested areas calculated in IMPACT were used to compute GHG emissions (See Supplementary Material Section 4, Assessment of GHG emissions).
Restoration of Forested Areas
Assessing the impacts of restoration requires a way of estimating what the forest would look like after the activities have taken hold. Specifically of interest is the potential amount of biomass that could be accumulated in a healthy forest after restoration. We assume that suitably healthy forests can be found in the vicinity of each degraded forest, and that the higher levels of recorded biomass in non-degraded areas are a reasonable proxy for the biomass gain that can be attained after restoration. This is operationalized by defining a neighborhood of 40 Km in diameter around each degraded location, and by choosing from this neighborhood the 87.5-th percentile value of biomass recorded by Hu et al. (2016).
Restoration Activities on Cropland—Alternative Agricultural Practices
In order to evaluate the impact on yields and GHG emissions of restoration on areas cultivated with maize, rice and wheat, a set of agricultural practices that are known to restore soil fertility and promote sustainable production under changing climate regimes were simulated in DSSAT. These practices are also part of a new proposed approach to agriculture called climate-smart agriculture (CSA, FAO., 2017). The technologies considered for maize and wheat are no-till, and integrated soil fertility management; nitrogen-use efficiency was simulated for rice.
No-till is characterized by minimum or no soil disturbance, in combination with residue retention, crop rotation, and use of cover crops. The existing literature on conservation agriculture, of which no-till is an essential component, points to a generally positive effect on yields from adopting this practice. However, the effects are variable, as they depend on a range of location-specific exogenous—e.g., climate and learning processes—and endogenous conditions—e.g., soil type (Erenstein et al., 2012; Lal, 2015; Pittelkow et al., 2015). In some conditions, short-term productivity may even decrease under conservation agriculture (Pittelkow et al., 2015), while yields are more stable and often increase with time, especially under dry or drought-stressed conditions (Corbeels et al., 2014; Pittelkow et al., 2015). No-till was simulated in DSSAT by minimizing soil disturbance, by means of a seed-planting stick as the planting method, and a deep injection system for fertilizer applications. Because no-till is already widely adopted in six countries (Argentina, Australia, Brazil, New Zealand, Paraguay, and Uruguay; Derpsch and Friedrich, 2009 and Derpsch et al., 2010), this technology is considered part of the business as usual scenario for those countries.
Integrated soil fertility management (ISFM) is a set of locally adapted practices that use a combination of synthetic fertilizers and locally available soil amendments (such as lime and phosphate rock) and organic inputs (crop residues, compost, and green manure) to replenish lost soil nutrients. The aim is to increase productivity through the efficient and sustainable use of nutrients (Vanlauwe et al., 2011). In DSSAT, ISFM was implemented by applying organic amendment in addition to the inorganic fertilizer applications defined in the baseline management scenario. The site-specific organic manure rate was based on Potter et al. (2010) and was applied monthly during the fallow period (after harvesting–before planting). The rate of inorganic fertilizer application is the same as the BAU; however, the application scheduling is optimized based on the growth stage of each crop to minimize nitrogen stress during flowering and grain filling.
Nitrogen-use efficiency is an approach that aims to reduce the use of nitrogen, nitrogen leaching and pollution, by providing it to the plant root system in more efficient manners. There are various methods of application but in general, they lead to both increased production and reduced emissions (FAO., 2017). To represent this technology, we used urea deep placement as a representative technology, under both rainfed and irrigated conditions. The effects were simulated by setting the in-built option for N fertilizer application method in DSSAT—deep placement of urea supergranules at a depth of 10 centimeters beneath the surface soil.
Restoration Pathways and Participation in Restoration Activities
The realities of each country's geographical features and political economy make it impossible to determine a priori if the emphasis of restoration projects will be on forest or agricultural land and how much cropland will ultimately be part of FLR efforts. Therefore, we consider two alternative restoration pathways to meet the Bonn Challenge. The first one prioritizes forest restoration (henceforth identified as the lower-bound scenario) while the second gives the priority to cropland restoration (henceforth called the upper-bound scenario). The purpose of this approach is to identify two extremes for the effects of restoration knowing that reality is somewhere in between. Each area unit (SPAM grid-cell) contains both tree cover and agricultural land and can be characterized by the ratio of agricultural land by forested area—the lower the ratio, the smaller the amount of agricultural land. In the lower-bound scenario, the grid-cells are arranged in ascending order (from smallest share to largest share of agricultural land) and as many grid-cells are included in the simulation as necessary to reach the 350 million hectares of land under restoration required by the Bonn Challenge. For the upper-bound scenario, the grid-cells are arranged in descending order and similarly, a sufficient amount is selected to meet the goal of the Bonn Challenge. In so doing, the lower-bound scenario maximizes the amount of forest involved in FLR efforts while the upper-bound scenario maximizes restoration over agricultural land.
Furthermore, given the lack of information regarding implementation costs and uptake of restoration activities by local populations, we heuristically account for these barriers by generating five sub-scenarios for both the lower- and the upper-bound scenario. These are constructed by assuming different levels of households' participation in restoration efforts: 40, 50, 70, 90, 100 percent4. The rate of participation has important implications on the overall effects of meeting the Bonn Challenge. This is assuming that the goal is to have restoration efforts actually undertaken over 350 million hectares. To understand this, let us take for example the scenario that emphasize restoration over forested areas. As the uptake of restoration practices decreases, reaching the 350 million hectares requires that restoration activities increasingly target land with a lower presence of forests and with a higher incidence of mix uses, including agriculture (see Figure 2 for a stylized representation). The resulting effect is that as the participation in restoration activities decreases, the benefits from restoring forest are expected to decrease while those from restoring agricultural land increase. The opposite is true for different participation rates for the upper-bound scenario.
To explore the relationship between restoration and food security, the alternative scenarios were constructed by assuming that all farmers who produce maize, wheat, and rice and are located in the areas targeted for restoration adopt the alternative practices (i.e., restoration practices). In our modeling settings therefore, a poor yield performance of the alternative practices would result in lower levels of production compared to BAU. If these were the results of our analysis, they would point to potential compatibility issues between restoration and food security objectives. They would indicate that if restoration practices were widely utilized, they would replace the existing and more productive production systems.
Market and Food Security Effects of Restoration
All scenarios are evaluated using the IMPACT system of models (see Supplementary Material Section 1). IMPACT uses a partial equilibrium approach to model the behavior of a globally competitive agricultural market and generate projections for supply, demand, and prices of agricultural commodities at the country level. Crop yields with current and alternative practices obtained using DSSAT are used as an input for the simulations implemented in IMPACT. The model returns production and yields, areas allocated to crops to satisfy the demand for agricultural products, and commodity prices, as well as estimates of the number of undernourished children and people at risk of hunger (see Supplementary Material Section 1).
Results
The combination of two restoration pathways, each one simulated under two alternative climate models, and the five participation-level sub-scenarios generates a total of twenty different sets of results to be compared with BAU (Table 2). To facilitate their interpretation, results for each scenario are reported as an average across climate models followed in parenthesis by the range across climate models and levels of participation (lowest—highest value). The results are provided in full in the Supplementary Material Section 6.
Business-As-Usual Scenario
Results for the BAU scenario (Figure 3) indicate that production of maize, rice, and wheat is expected to increase by 26 percent (21–31 percent), 14.5 percent (14–15 percent), and 22.5 percent (22–23) percent by 2030. Despite production growth, which should drive prices down, because of population and economic growth and changes in people's diets, commodity prices are projected to increase. Prices of maize, rice, and wheat are projected to grow by 34 percent (24–44 percent), 24 percent (21–27 percent), and 16 percent (13–19 percent), respectively. The price of maize is also affected by a growing demand for animal proteins and the use of this crop as feed for livestock. These changes, together with rising incomes, affect the overall availability and accessibility of food for millions of people and thus their general nutritional status. In 2030, the number of undernourished children is projected to decrease by 15.5 percent (15–16 percent) and the population at risk of hunger is projected to decrease by 31.5 percent (29–34 percent), compared to 2010.
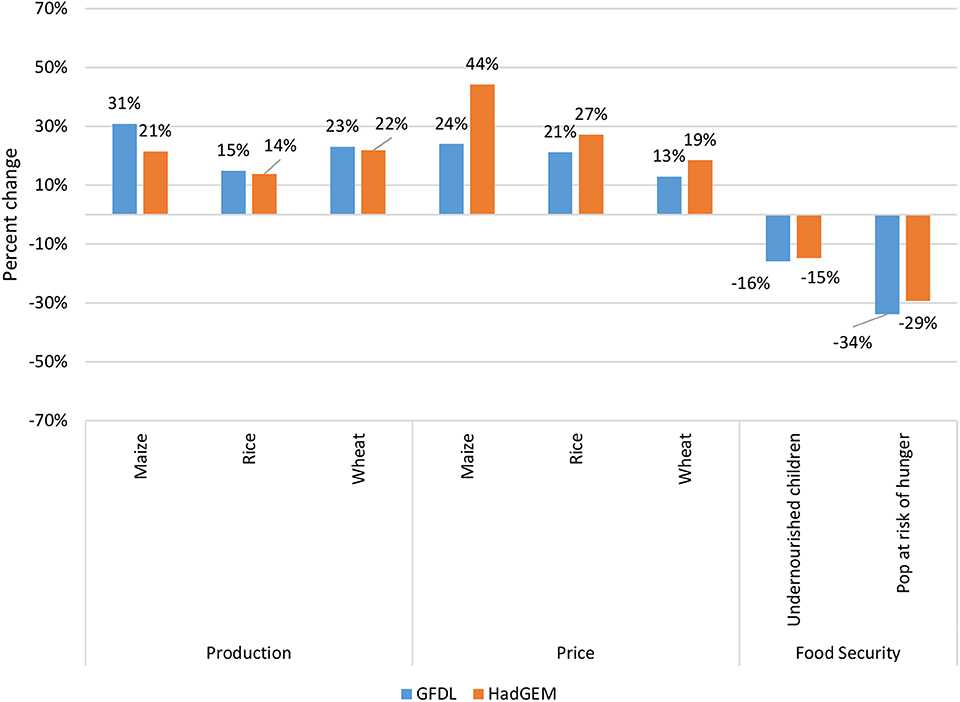
Figure 3. BAU scenario: 2010–2030—Changes in production, prices, undernourished children, and population at risk of hunger.
Achieving the Bonn Challenge With Minimum Involvement of Agriculture (Lower-Bound Scenario)
Figure 4, left column, displays the areas targeted for restoration in the cases of 100 and 40 percent participation level. A comparison of the two images shows how the location of restoration interventions changes with changing participation rates and how cropland becomes increasingly part of restoration as the participation decreases. The 100 percent participation scenario includes, by construction, the largest amount of forest. The total amount present in targeted areas is 167.4 million hectares (5.9 percent of global forests), 104 million hectares of which is classified as tropical forest (7.6 percent of global tropical forests). Results also indicate that about 22 million hectares of cropland (~3 percent of the global harvested area) would be present in areas targeted for restoration. The remaining 161 million hectares are allocated to other land categories such as grassland and shrubland. Although restoration is spread globally, the areas targeted seem more concentrated in Africa south of the Sahara, Southeast Asia, and North and South America.
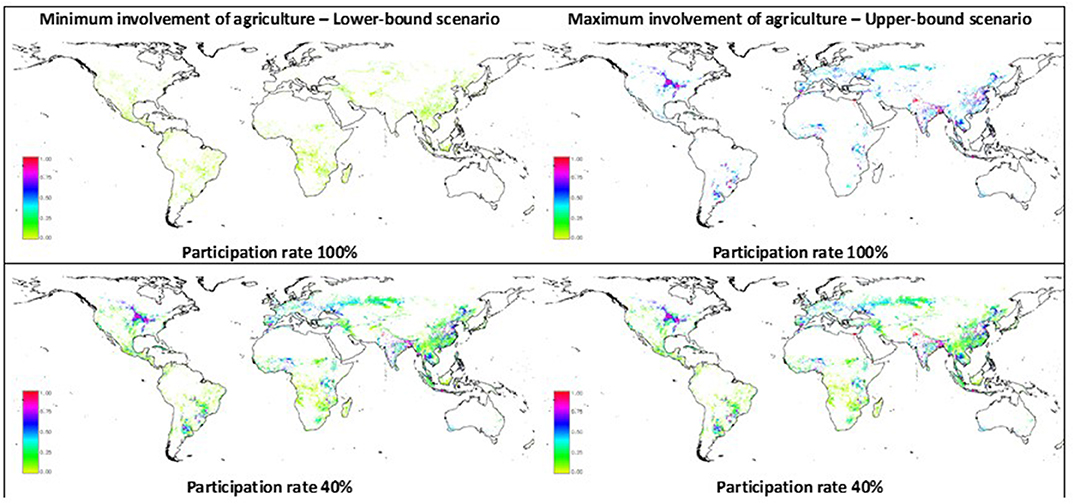
Figure 4. Location and density of cropland in areas targeted by restoration efforts. The legend reports the fraction of cropland area in each grid cell.
Simulations across all participation levels indicate that alternative agricultural practices would have an appreciable positive effect on production and prices compared to BAU. Figure 5 shows the effects on the cumulative production of maize, rice, and wheat computed as fresh harvest weight across the different climate models and participation rates. These effects increase as the land on which restoration is undertaken contains increasingly mixed uses including cropland (i.e., the uptake of restoration practices on mostly forested areas decreases). The results for the lower-bound scenario show that by 2030 the projected cumulative output gain for the three cereals ranges from 0.2 to 0.8 percent. Specifically, maize production increases by 0.5 percent (0.3−0.8 percent), rice production by 0.6 percent (0.2−1.4 percent,) and wheat by 0.2 percent (0.1−0.5 percent) (see Supplementary Material for detailed results). Just like for the BAU scenario, commodity prices are projected to increase but higher production slows down price growth by 1 percent (0.6–1.6 percent) for maize, by 2 percent (0.9–4.8 percent) for rice, and by 0.7 percent (0.3–1.5 percent) for wheat (see Supplementary Material, Table S6 for details). The compounding effect of higher production and lower prices increases the availability and accessibility of these food staples, with a consequent projected reduction in the number of undernourished children and the population at risk of hunger compared with BAU. The number of undernourished children is reduced by an additional 0.2 percent (0.1–0.5 percent) corresponding to 100,000–500,000 children, and the population at risk of hunger by an additional 0.9 percent (0.4–2.0 percent) equivalent to 2,400,000–11,980,000 people.
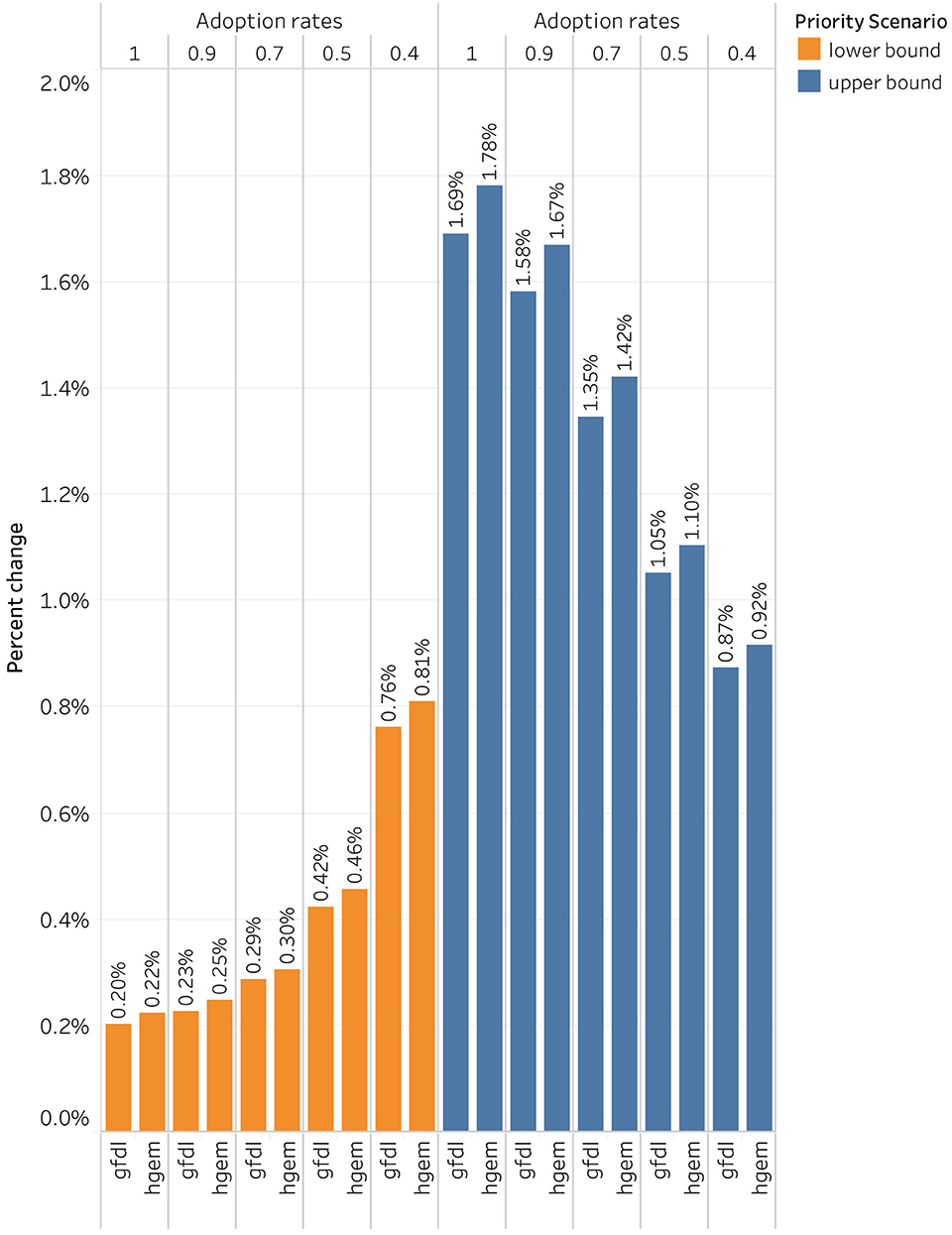
Figure 5. Changes in cumulative fresh harvest weight of maize, rice and wheat across climate models and participation rates compared to BAU.
Achieving the Bonn Challenge With Maximum Involvement of Agriculture (Upper-Bound Scenario)
Figure 4, right column, shows the areas targeted for restoration in the cases of 100 and 40 percent participation level. As in the previous case, a comparison of these two images shows how the location of restoration interventions changes with changing participation rates. By construction, this scenario includes more cropland than the previous. In the case of 100 percent participation, about 190 million hectares of cropland (~27 percent of global harvested area) are involved in restoration efforts. About 63 million hectares of forest (about 2.2 percent of all forests) are also present in this area, 30 million hectares of which are classified as tropical forest (also roughly 2.2 percent of global tropical forests). The remaining 97 million hectares are allocated to other land categories. It should be noted how, in this scenario, the restoration on cropland concentrates in areas different from in the previous one, such as the American Midwest, Iran, Bangladesh, and Indonesia. Some locations are common to both scenarios (Kenya, Zambia, Tanzania, Zimbabwe, Madagascar, China, and Thailand, to name a few).
Results indicate that a wider uptake of conservation practices on degraded land would have a greater positive effect on production (right side of Figure 5) and consequently on prices. The cumulative output gain for the three cereals ranges from 0.9 to 1.8 percent. Specifically, compared to BAU production increases by 1.2 percent (0.8–1.5 percent) for maize, by 2.6 percent (1.6–3.5 percent) for rice, and by 0.8 percent (0.5–1.1 percent) for wheat. Compared to the BAU, price growth is reduced by 2.2 percent (1.6–2.6 percent) for maize, by 8.8 percent (5.6–11.8 percent) for rice, and by 2.4 percent (1.6–3.3 percent) for wheat. The combined effect of higher production and lower prices increases the availability and accessibility of maize, rice, and wheat, with a consequent reduction in the number of undernourished children and in the population at risk of hunger. A direct comparison with BAU shows that the number of undernourished children is reduced by an additional 0.7 percent (0.4–0.9 percent) equivalent to 500,000–1,100,000 children and the population at risk of hunger by an additional 3.6 percent (2.3–4.8 percent) corresponding to 12,700,000–28,170,000 people.
It is important to note how the two scenarios (upper and lower-bound) become similar as the participation rate decreases. This is because the land involved in restoration efforts become increasingly similar in terms of presence of mix uses regardless if one starts with a high concentration of forest or of cropland. These similarities can be appreciated both visually in Figure 4 and numerically in Figure 5.
Effects on GHG Emissions
Adoption of alternative agricultural practices has an effect on soil organic carbon concentration and on GHG emissions. There is however a significant variation in the performance of these practices due to local soil and weather conditions and due to our limits in modeling farmers' tailoring of the alternative practices to local realities. This variation is however expected and is well reported in the literature (See Supplementary Material 5 and Table S4). Table 3 reports the average, and standard deviation, in emissions reductions by crop and climate scenario as calculated by DSSAT prior to the making of all scenarios. Alternative practices are most effective in rice production followed by maize and wheat. The standard deviation shows that there are locations where emissions are higher than BAU. This is an indication that the practices considered can increase yields but can also increase emissions (results by country are made available in the Supplementary Material Section 7).
Considering the global average abatements across all scenarios, GHG emissions are projected to decrease on average by 0.3 Mg CO2 e ha−1 yr−1 (0.2–0.3 Mg CO2 e ha−1 yr−1) which amounts to a total yearly average reduction of GHG emissions of ~58 million Mg CO2 e (17–84 million Mg CO2 e). On average, soil organic carbon is estimated to grow by ~0.1 Mg ha−1 yr−1 (0.08–0.12 Mg ha−1 yr−1) over the area that adopts the alternative practices. These changes, albeit relatively small, are important because they represent increases in soil fertility and in soil water retention and have positive implications for the overall resilience of production systems.
Effects on Carbon Stock
As explained in section Restoration of forested areas, we have used highly simplifying assumptions to compute the potential gains obtainable through restoration efforts in forested areas. Therefore, these results must be considered only as indicative of the impacts on carbon stock. Nevertheless, as expected (Li et al., 2015), this is where the largest impacts of restoration can be found. They vary significantly according to how much emphasis is placed on forest restoration. They are estimated to be 595 Tg C (423–715 Tg C) for the lower-bound scenario and 243 Tg C (127–414 Tg C) for the upper-bound scenario5. To these increases in aboveground biomass, changes in belowground biomass should be added6. Using an indicative ratio below- to above-ground biomass of 0.3, these range from 38 to 207 Tg C. Studies have reported contradictory findings regarding the effects of afforestation on soil carbon stock. Afforestation resulted either in an increase (Lemma et al., 2006; Wei et al., 2014) or a decrease (Farley et al., 2004; Deng et al., 2016) and at times has had a negligible effect (Smal and Olszewska, 2008) on soil carbon stock. It is therefore possible that under some conditions and in some areas, losses in soil carbon stock could partially offset the gains attributable to above- and below-ground biomass. Nonetheless, the gains in the forest carbon stock appear to be large enough to make a meaningful contribution to the global fight against climate change. Using as a benchmark the 1.6 Gt CO2 e yr−1 emissions deriving from land use change IPCC WG1 (Solomon et al., 2007), the gains in carbon estimated correspond to yearly reduction ranging from 2 to 10 percent7.
Figure 6 shows the changes in carbon compared to BAU as the uptake of restoration activities decreases. The gains in carbon stock decrease for the lower-bound scenario as the land involved in restoration activities increasingly contains more mixed uses. The opposite is true for the upper-bound scenario as cropland yields to mixed uses including forest.
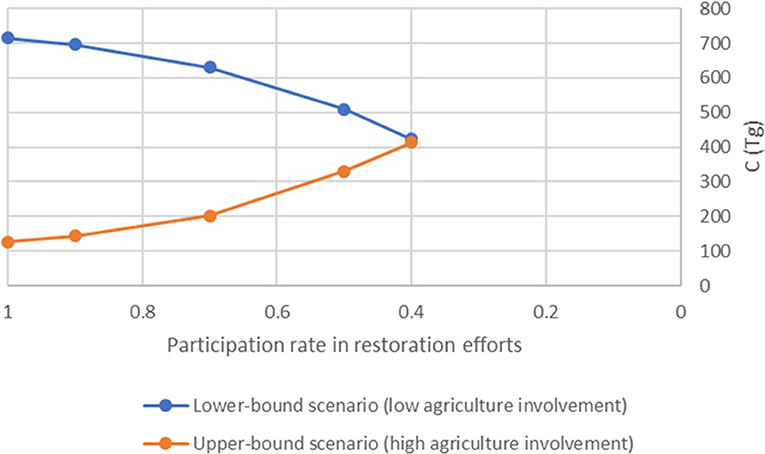
Figure 6. Changes in above-ground biomass with adoption of restoration practices at different participation rate levels.
In reference to climate change, it must also be noted that forest restoration can have opposite effects on global warming depending on the latitude at which it takes place. An increase in forest cover in the boreal zones is expected to induce a net warming because the reduction of albedo is the dominating effect (Brovkin et al., 2013; de Wit et al., 2014). In tropical regions, the net impact is typically a net cooling due to the dominant influence of evapotranspiration and surface roughness (Bala et al., 2007; Jackson et al., 2008).
Effects on Land use
In addition to the effects on GHG emissions and carbon stock, restoration activities on agricultural land have other potentially positive benefits. The combination of higher production and lower prices reduces producers' incentives to expand production areas for wheat and rice. Considering the upper-bound scenario which by construction has the largest effects, the harvested area for wheat is projected to decrease by a maximum of 0.4 percent (0.2–0.5 percent) and for rice by 1.6 percent (1.0–2.2 percent). Area allocated to maize can increase by 0.3 percent (0.1–0.4 percent), due to the increase in the demand for feed for livestock. Despite this increase, when the three crops are considered together, the total harvested area is projected to decrease, with significantly different results from one region of the world to the other (see Figures S4–S8). Although the reduced demand for harvested area could potentially reduce the encroachment of cropland into environmentally sensitive and carbon-rich areas like forests, additional research is necessary to evaluate the specific regional changes vis-à-vis the presence of environmentally sensitive areas.
Important Limits and Future Extensions of This Study
This analysis does not consider the gains that could be obtained by undertaking restoration activities on all different types of degraded agricultural land. This is due to the current modeling limitation which prevent the representation of agroforestry, silvopastoral, and agrosilvopastoral systems at the global level with the same level of accuracy of crop production (Luedeling et al., 2014, 2016). Therefore, the role of these systems on a global scale remains here unexplored.
However, an ad-hoc and partial analysis can reveal the significant contribution that these alternative production systems could provide in restoration projects. Consider for example parklands, which are relatively well-established in some regions of Africa. Parklands occur in various latitudes, and while the most well-known examples are located in semiarid or subhumid zones (Bourlière and Hadley, 1983), production systems with scattered trees are also widespread in southern Africa (Campbell et al., 1991; Maghembe and Seyani, 1991). Extensive intercropping systems which include widely-spaced trees, as is typical of parkland systems, are considered to deliver benefits of limited magnitude (Nair, 2012). However, if the practices we considered in our simulations were to be used in such systems the benefits would compound. Figure 4 shows the degraded area in eastern and southern Africa where restoration activities should be undertaken. Considering the 100% participation rate in the upper-bound scenario, this area totals ~4.5 million hectares. Our simulations indicate that the average annual gains in yield are of some importance (1.3 Mg ha−1 yr−1 for maize, 0.4 Mg ha−1 yr−1 for wheat, and 1.1 Mg ha−1 yr−1 for rice) but the reduction in GHG emissions is rather modest (approximately 1.4 million Mg CO2 e yr−1, or about 0.3 Mg CO2 e ha−1 yr−1)8. Mbow et al. (2014) report a carbon sequestration potential for parkland agroforestry systems with Faidherbia albida to be in the range of 0.2–0.8 Mg C ha−1 yr−1. Using the authors' suggested average value of 0.5 Mg C ha−1 yr−1, we can calculate that the contribution of this system to mitigation would be about 8.3 million Mg CO2 e yr−1, which is approximately six times the greenhouse gas reduction obtained by changing only agricultural practices9. Therefore, the combination of agroforestry and alternative crop production practices promotes greater food production, increased revenues, reduced pressure for cropland expansion, healthier soils, and increased carbon sequestration 10.
Similarly, important but left to future research, is the role of livestock and pastureland as components of silvopasture and agrosilvopasture systems. Existing models cannot capture the complexity and diversity of these systems due to the wide range of climatic and environmental settings. The literature shows that silvopastoral systems can increase productivity, sequester significant amounts of carbon, and increase soil organic carbon (Dulormne et al., 2003; Mosquera-Losada et al., 2011; Udawatta and Jose, 2011). Given the important benefits expected to accrue from agroforestry and silvopasture and agrosilvopasture systems, additional work on identifying locally viable options and scalable innovations, as well as developing models that accurately represent these systems, is of paramount importance.
Discussion and Conclusion
Healthy ecosystems, productive land, and fertile soils are essential to food production and human existence, their degradation poses significant challenges for the well-being of all people around the world. Land and soil degradation produce substantial challenges to meeting global food needs and it generates significant risks to people, particularly those in rural and poor areas who are heavily dependent on natural resources. Given its magnitude, the problem of degradation must be addressed globally; as such, the international community is engaged in several initiatives to address degradation. The most recent is the Bonn Challenge, which aims to bring 350 million hectares of the world's deforested and degraded land into restoration by 2030.
It is in this environment that the FLR approach has gained relevance. FLR underpins the Bonn challenge and operates at the nexus of several competing forces, environmental and developmental, and attempts to resolve this tension by fully integrating other land uses in restoration efforts. The role of agricultural land is particularly important because it is expected to promote the active role of communities and thus a broad implementation of restoration plans.
This paper provides insights into the potential effects of involving crop production in restoration efforts. Our results show that the benefits are multiple, potentially significant in size, and relevant for several international commitments, goals, and programs. Among these are an increase in agricultural production and lower commodity prices which lead to improvements in food security through a substantial reduction in the number of undernourished children and people at risk of hunger (SDG 2). Furthermore, increased productivity reduces the demand for cropland, potentially curbing its encroachment into environmentally sensitive areas (LDN, SDG 15.3, Aichi target 5 and 7). Simulations also indicate that increased productivity is compatible with improvements in soil fertility and reduction of greenhouse gas emissions on a global scale (Paris agreement, SDG 13 and 15). The benefits—not only to farmers but to the broader population—strongly suggest that an approach that meaningfully integrates agriculture into forest restoration efforts can facilitate the implementation of restoration plans on large amounts of land. The fact that current modeling capabilities prevent us from including the use of agroforestry or agrosilvopastoral systems in the simulated scenarios and that we cannot fully represent farmers' tailoring of practices to local conditions indicates that our results are likely to be an underestimation of the full benefits of restoration practices on agricultural land.
It is important to recall that our model does not produce forecasts but rather scenarios of what the future might look like given the assumptions and conditions underlying our modeling environment. Because of the global scope of our analysis, there is a necessary tradeoff between formulating a manageable depiction of myriad individual decisions and their market interactions and incorporating in the model the full complexity of local conditions. Hence, our results—the magnitude of the effects in particular—must be considered as indicative and need to be investigated at the local level. This is not only because of the uncertainty around the results' (GHG emission reductions are an example), but also because of the many intervening forces that can prevent reaching the projected outcomes for 2030. We have implicitly assumed that farmers, once they have adopted restoration practices, continue using them through time. This is far from certain, and gains in soil organic carbon or forests' carbon stock can be lost more quickly than they are acquired. It is therefore necessary to keep supporting research into which type of instruments and incentives may ensure that farmers stay engaged in restoration efforts. This work is already abundant but still finds conflicting evidence on how to move forward (Janssen et al., 2013; Gatiso et al., 2015). We have also assumed that farmers are equally adept at implementing the practices considered, but we know from the literature that this is a simplification. In reality, transferring knowledge on how to switch to new agricultural practices is a complex endeavor that requires institutional support.
These considerations and our findings point to the fundamental role that policies and investments can play in restoration efforts. Reaching the high impacts indicated by our results requires finding and promoting solutions to long-standing problems, such as the need for well-functioning extension services, land and tree rights, access to good-quality information for farmers, and reliable and trustworthy institutions. Without addressing these barriers, farmers will continue to maximize their private short-term benefits, which might conflict with long-term societal goals. Policies and instruments that allow for the proper accounting of social benefits and costs also must be in place to generate an equitable competition between the use of chemical inputs and alternative solutions to increasing land productivity such as agroforestry systems.
The role of governments is also essential to make sure that the productivity gains on agricultural land do not lead to increased deforestation. The projected effect of higher yields and lower agricultural commodity prices is to reduce the need for additional harvested area to fulfill the increasing demand of maize, wheat, and rice. This suggests a reduced pressure to expand cropland and potential land-sparing effects. While the idea that agricultural intensification reduces agricultural land's encroachment into other natural areas is not new, it was famously put forward by Borlaug (1983), whether this happens in reality is the subject of some controversy (Barbier and Burgess, 1997; Rudel et al., 2009; Wiebe et al., 2019). It is worth noting that the uncertainties around the Borlaug hypothesis disappear when agricultural output increases globally, as in the case of our modeling. However, the adoption processes and the ensuing market adjustments are not instantaneous and therefore might not be uniform across farmers and regions. During the transition to more productive practices, some early adopters might gain a competitive advantage and have an incentive to expand their cultivation area—potentially at the expense of environmentally sensitive land. Hence, yield-increasing innovations should be accompanied by careful monitoring and regulation of land conversion.
In conclusion, our results confirm the findings of the many studies that have investigated the benefits of restoration in localized settings. These findings should provide enough confidence to governments and policymakers that they can invest in wide-scale restoration projects without jeopardizing their food security goals.
Data Availability Statement
The datasets generated for this study are available on request to the corresponding author.
Author Contributions
AD, NC, H-YK, RR, TT, SB, and CK developed the outline and provided input to the writing of the manuscript. AD, NC, RR, H-YK, and TT performed the analysis of results. AD, RR, H-YK, JK, and TT developed the methodology for the analysis. NC was responsible for the IMPACT modeling. All authors read and approved the final manuscript.
Funding
The authors acknowledged the generous support of the International Union for the Conservation of Nature as part of the KNOWFOR program funded by UK aid from the UK government, the Trees on Farms project funded by BMU-IKI and the support of the CGIAR Research Program on policies, Institutions, and Markets (PIM) funded by CGIAR Fund Donors and the CGIAR Research Program on Climate Change, Agriculture, and Food Security, which is carried out with support from CGIAR Fund Donors and through bilateral funding agreements.
Conflict of Interest
The authors declare that the research was conducted in the absence of any commercial or financial relationships that could be construed as a potential conflict of interest.
Acknowledgments
We would like to thank Frank Place, Ephraim Nkonya, and Dennis Garrity for their useful comments. The authors take sole responsibility for the opinions expressed within this article.
Supplementary Material
The Supplementary Material for this article can be found online at: https://www.frontiersin.org/articles/10.3389/fsufs.2020.00061/full#supplementary-material
Footnotes
1. ^Intact forest landscape is defined as areas between 500 hectares and 10 square kilometers wide with no settlements or industrial logging.
2. ^It would be natural to use the global data set generated by the Atlas of Forest Landscape Restoration Opportunities (World Resource Institute (WRI), 2017) which in part informed the commitments for the Bonn Challenge. However, this dataset was created by contrasting potential and actual tree cover and identifies land with restoration potential which is not necessarily degraded.
3. ^As suggested by the Atlas of Forest and Landscape Restoration Opportunities, there are areas that are practically too far to be considered for sustained restoration practices. We set this distance to be no greater than 2 days of travel form population centers of 20,000 inhabitants (Nelson, 2008).
4. ^Take for example an area of one hundred hectares of mixed uses forest-agriculture that is targeted by a restoration project. Under the 50 percent participation assumption, restoration efforts will be undertaken only on 50 hectares.
5. ^Carbon content is calculated by multiplying the biomass gains by 0.47 given that biomass is approximately 50% of dry weight (IPCC, 2006).
6. ^The ratio below-ground biomass to above-ground biomass of 0.3 is an average of the ratios across ecological zones listed in IPCC (2006).
7. ^This is calculated by converting the estimated gains in carbon stock into CO2e and by assuming a 20-year period to obtain those gains. For example, 715 Tg C + 207 Tg C = 3,384 Tg CO2e which correspond to an average annual gain of 169 Tg CO2e.
8. ^Soil organic carbon is estimated to increase by 0.15 Mg C ha−1 yr−1, but greenhouse gas emissions are expected to be reduced by 0.3 Mg CO2 e ha−1 yr−1.
9. ^1.00 Mg of carbon equals 3.67 Mg of CO2.
10. ^It is also documented that in some areas and conditions, nitrogen-fixing trees on crop fields offer yield increases between 50 and 300 percent in associated cereal crops (Sileshi et al., 2008); that they also promote restoration by replenishing the soil stock of organic carbon (Nair, 2012).
References
Asner, G. P., Knapp, D. E., Broadbent, E. N., Oliveira, P. J., Keller, M., Silva, J., et al. (2005). Selective logging in the Brazilian amazon. Science 310, 480–482. doi: 10.1126/science.1118051
Bala, G., Caldeira, K., Wickett, M., Phillips, T. J., Lobell, D. B., Delire, C., et al. (2007). Combined climate and carbon-cycle effects of large-scale deforestation. Proc. Natl. Acad. Sci. U.S.A. 104, 6550–6555. doi: 10.1073/pnas.0608998104
Bao, Q. L., Nkonya, E., and Mirzabaev, A. (2016). “Biomass productivity-based mapping of global land degradation hotspots” in Economics of Land Degradation and Improvement: A Global Assessment for Sustainable Development, eds E. Nkonya, A. Mirzabaev, and J. von Braun (Cham: Springer), 55–84. doi: 10.1007/978-3-319-19168-3_4
Barbier, E. B., and Burgess, J. C. (1997). The economics of tropical forest land use options land. Land Econ.73, 174–95. doi: 10.2307/3147281
Bewket, W. (2007). Soil and water conservation intervention with conventional technologies in northwestern highlands of ethiopia: acceptance and adoption by farmers. Land Use Policy 24, 404–416. doi: 10.1016/j.landusepol.2006.05.004
Bonn Challenge (2020). Available online at: https://www.bonnchallenge.org/content/challenge (accessed March 2020).
Borlaug, N. E. (1983). Contributions of conventional plant breeding to food production. Science 80, 689–693. doi: 10.1126/science.219.4585.689
Bourlière, F., and Hadley, M. (1983). “Present-day savannas: an overview,” in Tropical Savannas Ecosystems of the World, ed F. Bourlière (Amsterdam: Elsevier), vol. 13, 1–17.
Brovkin, V., Boysen, L., Arora, V. K., Boisier, J. P., Cadule, P., Chini, L., et al. (2013). Effect of anthropogenic land-use and land-cover changes on climate and land carbon storage in CMIP5 projections for the twenty-first century. J. Clim. 26, 6859–6881. doi: 10.1175/JCLI-D-12-00623.1
Brown, D. R., Dettmann, P., Rinaudo, T., Tefera, H., and Tofu, A. (2009). Poverty alleviation and environmental restoration using the clean development mechanism: a case study from Humbo, Ethiopia. Environ. Manage. 48, 322–333. doi: 10.1007/s00267-010-9590-3
Bryan, E., Ringler, C., Okoba, B., Roncoli, C., Silvestri, S., and Herrero, M. (2013). Adapting agriculture to climate change in Kenya: household strategies and determinants. J. Environ. Manage. 114, 26–35. doi: 10.1016/j.jenvman.2012.10.036
Busch, J., Engelmann, J., Cook-Patton, S. C., Griscom, B. W., Kroeger, T., Possingham, H., et al. (2019). Potential for low-cost carbon dioxide removal through tropical reforestation. Nat. Clim. Chang. 9, 463–466. doi: 10.1038/s41558-019-0485-x
Calle, A., Calle, Z., Garen, E., and Del Cid-Liccardi, A., (eds) (2014). Symposium on Ecological Restoration and Sustainable Agricultural Landscapes. Environmental Leadership and Training Initiative. New Haven, CT: Yale University; Panama City: Smithsonian Tropical Research Institute.
Calle, A., Montagnini, F., and Zuluaga, A. F. (2009). Farmer's perceptions of silvopastoral system promotion in Quindío, Colombia. Bois Forets Trop. 300, 79–94.
Calle, Z., Murgueitio, E., Chará, J., Molina, C. H., Zuluaga, A. F., and Calle, A. (2013). A strategy for scaling-up intensive silvopastoral systems in Colombia. J. Sustain. For. 32, 677–693. doi: 10.1080/10549811.2013.817338
Campbell, B. M., Clarke, J. M., and Gumbo, D. J. (1991). Traditional agroforestry practices in Zimbabwe. Agrofor. Syst. 14, 99–111. doi: 10.1007/BF00045726
Carlson, K. M., Gerber, J. S., Mueller, N. D., Herrero, M., MacDonald, G. K., Brauman, K. A., et al. (2017). Greenhouse gas emissions intensity of global croplands. Nat. Clim. Chang. 7, 63–68. doi: 10.1038/nclimate3158
Channan, S., Collins, K., and Emanuel, W. R. (2014). Global Mosaics of the Standard MODIS Land Cover Type Data. College Park, MD: University of Maryland and the Pacific Northwest National Laboratory.
Chazdon, R. L. (2018). Protecting intact forests requires holistic approaches. Nat. Ecol. Evol. 2:915. doi: 10.1038/s41559-018-0546-y
Chazdon, R. L., Brancalion, P. H. S., Lamb, D., Laestadius, L., Calmon, M., and Kumar, C. (2017). A policy-driven knowledge agenda for global forest and landscape restoration. Conserv. Lett. 10, 125–132. doi: 10.1111/conl.12220
Clarke, L, Jiang, K., Akimoto, K., Babiker, M., Blanford, G., Fisher-Vanden, K., et al. (2014). “Assessing transformation pathways,” in Climate Change 2014: Mitigation of Climate Change. Contribution of Working Group III to the Fifth Assessment Report of the Intergovernmental Panel on Climate Change, eds O. Edenhofer, R. Pichs-Madruga, Y. Sokona, E. Farahani, S. Kadner, K. Seyboth, A. Adler, I. Baum, S. Brunner, P. Eickemeier, B. Kriemann, J. Savolainen, S. Schlömer, C. von Stechow, T. Zwickel, and J. C. Minx (Cambridge; New York, NY: Cambridge University Press).
Corbeels, M., de Graaff, J., Ndah, T. H., Penot, E., Baudron, F., Naudin, K., et al. (2014). Understanding the impact and adoption of conservation agriculture in africa: a multiscale analysis. Agric. Ecosyst. Environ. 187, 155–170. doi: 10.1016/j.agee.2013.10.011
Costanza, R., de Groot, R., Sutton, P., van der Ploeg, S., Anderson, S. J., Kubiszewski, I., et al. (2014). Changes in the global value of ecosystem services. Glob. Environ. Chang. 26, 152–158. doi: 10.1016/j.gloenvcha.2014.04.002
Dagan, A. B. K., and Nair, P. K. R. (2003). Silvopastoral research and adoption in Central America: recent findings and recommendations for future directions. Agrofor. Syst. 59, 149–155 doi: 10.1023/A:1026394019808
de Wit, H. A., Bryn, A., Hofgaard, A., Karstensen, J., Kvalevåg, M. M., and Peters, G. P. (2014). Climate warming feedback from mountain birch forest expansion: reduced albedo dominates carbon uptake. Glob. Chang. Biol. 20, 2344–2355. doi: 10.1111/gcb.12483
Deichert, G., Krämer, F., and Schöning, A. (2014). Turning degraded land into productive landscapes, Ethiopian highlands. ETFRN News 56, 82–87.
Deng, L., Zhu, G., Tang, Z., and Shangguan, Z. (2016). Global patterns of the effects of land-use changes on soil carbon stocks. Glob. Ecol. Conserv. 5, 127–138. doi: 10.1016/j.gecco.2015.12.004
Derpsch, R., and Friedrich, T. (2009). “Global overview of conservation agriculture adoption,” in Proceedings Lead Papers (New Delhi).
Derpsch, R., Friedrich, T., Kassam, A., and Hongwen, L. (2010). Current status of adoption of no-till farming in the world and some of its main benefits. Int J Agric Biol Eng. 3, 1–25. doi: 10.3965/j.issn.1934-6344.2010.01.0-0
Díaz, S., Settele, J., Brondízio, E., Ngo, H. T., Guèze, M., Agard, J., et al. (2019). Summary for Policymakers of the Global Assessment Report on Biodiversity and Ecosystem Services of the Intergovernmental Science-Policy Platform on Biodiversity and Ecosystem Services. Secretariat of the Intergovernmental Science-Policy Platform on Biodiversity and Ecosystem Services. Bonn: IPBES.
Dulormne, M., Sierra, J., Nygren, P., and Cruz, P. (2003). Nitrogen-fixation dynamics in a cut-and-carry silvopastoral system in the subhumid conditions of guadeloupe, French Antilles. Agrofor. Syst. 59, 121–129. doi: 10.1023/A:1026387711571
Dunne, J. P., John, J. G., Adcroft, A. J., Griffies, S. M., Hallberg, R. W., Shevliakova, E., et al. (2012). GFDL's ESM2 global coupled climate–carbon earth system models. Part I: physical formulation and baseline simulation characteristics. J. Clim. 25, 6646–6665. doi: 10.1175/JCLI-D-11-00560.1
Enfors, E. I., and Gordon, L. J. (2008). Dealing with drought: the challenge of using water system technologies to break dryland poverty traps. Glob. Environ. Chang. 18, 607–616 doi: 10.1016/j.gloenvcha.2008.07.006
Erenstein, O., Sayre, K., Wall, P., Hellin, J., and Dixon, J. (2012). Conservation agriculture in maize- and wheat-based systems in the (sub)tropics: lessons from adaptation initiatives in South Asia, Mexico, and Southern Africa. J. Sustain. Agric. 36, 180–206. doi: 10.1080/10440046.2011.620230
Erskine, P. D. (2002). Land clearing and forest rehabilitation in the wet tropics of North Queensland, Australia. Ecol. Manage. Restor. 3:136.
FAO. (2017). Climate Smart Agriculture Sourcebook. Available online at: http://www.fao.org/climate-smart-agriculture-sourcebook/en/ (accessed June 20, 2019).
Farley, K. A., Kelly, E. F., and Hofstede, R. G. M. (2004). Soil organic carbon and water retention following conversion of grasslands to pine plantations in the Ecuadoran andes. Ecosystems 77, 729–739. doi: 10.1007/s10021-004-0047-5
Floyd, C., Harding, A-H., Paudel, K. C., Rasali, D. P., Subedi, K., Subedi, P., et al. (2003). Household adoption and the associated impact of multiple agricultural technologies in the western hills of Nepal. Agric. Syst. 76, 715–738. doi: 10.1016/S0308-521X(02)00152-X
Friedl, M. A., Sulla-Menashe, D., Tan, B., Schneider, A., Ramankutty, N., Sibley, A., et al. (2010). MODIS Collection 5 Global Land Cover: Algorithm Refinements and Characterization of New Datasets, 2001-2012, Collection 5.1 IGBP Land Cover. Boston, MA: Boston University.
Garrity, D. P., Akinnifesi, F. K., Ajayi, O. C., Weldesemayat, S. G., Mowo, J. G., Kalinganire, A., et al. (2010). Evergreen agriculture: a robust approach to sustainable food security in Africa. Food Security 2, 197–214. doi: 10.1007/s12571-010-0070-7
Gatiso, T. T., Vollan, B., and Nuppenau, E. A. (2015). Resource scarcity, and democratic elections in commons dilemmas: an experiment on forest use in Ethiopia. Ecol. Econ. 114, 199–207. doi: 10.1016/j.ecolecon.2015.04.005
Gibbs, H. K., Ruesch, A. S., Achard, F., Clayton, M. K., Holmgren, P., Ramankutty, N., et al. (2010). Tropical forests were the primary sources of new agricultural land in the 1980s and 1990s. Proc. Natl. Acad. Sci. U.S.A. 107, 16732–16737. doi: 10.1073/pnas.0910275107
Gibbs, H. K., and Salmon, J. M. (2015). Mapping the world's degraded lands. Appl. Geogr. 57, 12–21. doi: 10.1016/j.apgeog.2014.11.024
Hansen, M., Egorov, A., Roy, D. P., Potapov, P., Ju, J., Turubanova, S., et al. (2011). Continuous fields of land cover for the conterminous united states using landsat data: first results from the Web-Enabled Landsat Data (WELD) project. Remote Sens. Lett. 2, 279–288. doi: 10.1080/01431161.2010.519002
Hansen, M. C., Potapov, P. V., Moore, R., Hancher, M., Turubanova, S. A., Tyukavina, A., et al. (2013). High-resolution global maps of 21st-century forest cover change. Science 342, 850–853. doi: 10.1126/science.1244693
Harvey, C. A., Chacón, M., Donatti, C. I., Garen, E., Hannah, L., Andrade, A., et al. (2014). Climate-smart landscapes: opportunities and challenges for integrating adaptation and mitigation in tropical agriculture. Conserv. Lett. 7, 77–90. doi: 10.1111/conl.12066
Henders, S., Persson, U. M., and Kastner, T. (2015). Trading forests: land-use change and carbon emissions embodied in production and exports of forest-risk commodities. Environ. Res. Lett. 10:125012. doi: 10.1088/1748-9326/10/12/125012
Hu, T., Su, Y., Xue, B., Liu, J., Zhao, X., Fang, J., et al. (2016). Mapping global forest aboveground biomass with spaceborne LiDAR, optical imagery, and forest inventory data. Remote Sens. 8, 2–27 doi: 10.3390/rs8070565
International Food Policy Research Institute (IFPRI) (2012). 2011 Global Food Policy Report. Washington, DC: International Food Policy Research Institute (IFPRI).
IPCC (2006). “2006 IPCC guidelines for national greenhouse gas inventories,” in Prepared by the National Greenhouse Gas Inventories Programme, eds H. S. Eggleston, L. Buendia, K. Miwa, T. Ngara, and K. Tanabe (Hayama: Institute for Global Environmental Strategies).
Islam, S., Cenacchi, N., Sulser, T. B., Gbegbelegbe, S., Hareau, G., Kleinwechter, U., et al. (2016). Structural approaches to modeling the impact of climate change and adaptation technologies on crop yields and food security. Glob. Food Security 10, 63–70. doi: 10.1016/j.gfs.2016.08.003
IUCN and WRI (2014). A Guide to the Restoration Opportunities Assessment Methodology (ROAM): Assessing Forest Landscape Restoration Opportunities at the National or Sub-National Level. Working Paper (Gland: IUCN), 125.
Jackson, R. B., Randerson, J. T., Canadell, J. G., Anderson, R. G., Avissar, R., Baldocchi, D. D., et al. (2008). Protecting climate with forests. Environ. Res. Lett. 3:044006. doi: 10.1088/1748-9326/3/4/044006
Janssen, M. A., Bousquet, F., Cardenas, J. C., Castillo, D., and Worrapimphong, K. (2013). Breaking the elected rules in a field experiment on forestry resources. Ecol. Econ. 90, 132–139. doi: 10.1016/j.ecolecon.2013.03.012
Jones, C. D., Hughes, J. K., Bellouin, N., Hardiman, S. C. S., Jones, G., Knight, J., et al. (2011). The HadGEM2-ES implementation of CMIP5 centennial simulations. Geosci. Model Dev. 4, 543–570. doi: 10.5194/gmd-4-543-2011
Jones, J. W., Hoogenboom, G., Porter, C. H., Boote, K. J., Batchelor, W. D., Hunt, L. A., et al. (2003). The DSSAT cropping system model. Eur. J. Agron. 18, 235–265. doi: 10.1016/S1161-0301(02)00107-7
Kabwe, G., Bigsby, H., and Cullen, R. (2009). “Factors influencing adoption of agroforestry among smallholder farmers in Zambia,” in NZARES Conference (New Zealand). Available online at: http://ageconsearch.umn.edu/bitstream/97135/2/2009_9_Zambian%20Agroforestry%20Adoption_KabweG.pdf (accessed September 20, 2019).
Kabwe, G., Bigsby, H., and Cullen, R. (2016). Why is adoption of agroforestry stymied in Zambia? perspectives from the ground-up. Afr. J. Agric. Res. 11, 4704–4717. doi: 10.5897/AJAR2016.10952
Keil, A., Zeller, M., and Franzel, S. (2005). Improved fallows in smallholder maize production in zambia: do initial testers adopt the technology? Agrofor. Syst. 64, 225–236. doi: 10.1007/s10457-004-2410-0
Lal, R. (2015). Sequestering carbon and increasing productivity by conservation agriculture. J. Soil Water Conserv. 70, 55A−62A. doi: 10.2489/jswc.70.3.55A
Lamb, D., Erskine, P. D., and Parrotta, J. A. (2005). Restoration of degraded tropical forest landscapes. Science 310, 1628–1632. doi: 10.1126/science.1111773
Lambert, O., and Ozioma, A. F. (2012). Adoption of improved agroforestry technologies among contact farmers in imo state, Nigeria. Asian J. Agric. Rural Dev. 2, 1–9. doi: 10.22004/ag.econ.197935
Lemma, B., Kleja, D. B., Nilsson, I., and Olsson, M. (2006). Soil carbon sequestration under different exotic tree species in the southwestern highlands of Ethiopia geoderma. Asian J. Agric. Rural Dev. 136, 886–898. doi: 10.1016/j.geoderma.2006.06.008
Lewis, S. L., Wheeler, C. E., Mitchard, E. T. A., and Koch, A. (2019). Restoring natural forests is the best way to remove atmospheric carbon. Nature 568, 25–28. doi: 10.1038/d41586-019-01026-8
Li, M., De Pinto, A., Ulimwengo, J., You, L., and Robertson, R. (2015). Modeling land-use allocation with mixed-level data: an econometric analysis for the democratic republic of the Congo. Environ. Resour. Econ. 60, 433–469. doi: 10.1007/s10640-014-9775-y
Lu, D., Batistella, M., Mausel, P., and Moran, E. (2007). Mapping and monitoring land degradation risks in the western Brazilian amazon using multitemporal landsat TM/ETM+ images. Land Degrad. Dev.18, 41–54. doi: 10.1002/ldr.762
Luedeling, E., Huth, N. I., Kindt, R., and König, K. (2014). Agroforestry systems in a changing climate—challenges in projecting future performance. Curr. Opin. Environ. Sustain. 6, 1–7. doi: 10.1016/j.cosust.2013.07.013
Luedeling, E., Smethurst, P. J., Baudron, F., Bayala, J., Huth, N. I., van Noordwijk, M., et al. (2016). Field-scale modeling of tree-crop interactions: challenges and development needs. Agric. Syst. 142, 51–69. doi: 10.1016/j.agsy.2015.11.005
Maghembe, J. A., and Seyani, J. H. (1991). Multipurpose Trees Used by Smallholder Farmers in Malawi: Results of an Ethnobotanical Survey. Agro Forestry Research Network for Africa Report No. 42. Nairobi: International Council for Research in Agroforestry.
Matocha, J., Schroth, G., Hills, T., and Hole, D. (2012). “Integrating climate change adaptation and mitigation through agroforestry and ecosystem conservation,” in Agroforestry: The Future of Global Land Use, Vol. 9. eds P. K. Ramachandran Nair, and D. Garrity (Dordrecht: Springer) 105–126. doi: 10.1007/978-94-007-4676-3_9
Mbow, C., Smith, P., Skole, D., Duguma, L., and Bustamante, M. (2014). Achieving mitigation and adaptation to climate change through sustainable agroforestry practices in Africa. Curr. Opin. Environ. Sustain. 6, 8–14. doi: 10.1016/j.cosust.2013.09.002
Meinshausen, M., Smith, S. J., Calvin, K., Daniel, J. S., Kainuma, M. L. T., Lamarque, J. F., et al. (2011). The RCP greenhouse gas concentrations and their extensions from 1765 to 2300. Clim. Chang. 109, 213–241. doi: 10.1007/s10584-011-0156-z
Mosquera-Losada, M. R., Freese, D., and Rigueiro-Rodríguez, A. (2011). “Carbon sequestration in european agroforestry systems,” in Carbon Sequestration Potential of Agroforestry Systems: Opportunities and Challenges, Vol. 8, eds B. M. Kumar, and P. K. R. Nair (New York, NY: Springer), 43–59. doi: 10.1007/978-94-007-1630-8_3
Mungai, L. M., Snapp, S., Messina, J. P., Chikowo, R., Smith, A., Anders, E., et al. (2016). Smallholder farms and the potential for sustainable intensification. Front. Plant Sci. 7:1720. doi: 10.3389/fpls.2016.01720
Nair, P. K. R. (2012). “Climate change mitigation: a low-hanging fruit of agroforestry,” in Agroforestry—The Future of Global Land Use, eds P. Nair, and D. Garrity (Dordrecht: Springer), 9, 31–67. doi: 10.1007/978-94-007-4676-3_7
Nelson, A. (2008). Global 1 Km Accessibility (CostDistance) Model Using Publicly Available Data. Washington, DC: World Bank.
Nelson, G. C., van der Mensbrugghe, D., Ahammad, H., Blanc, E., Calvin, K., Hasegawa, T., et al. (2014). Agriculture and climate change in global scenarios: why don't the models agree. Agric. Econ. 45, 85–101. doi: 10.1111/agec.12091
Nkonya, E., Gerber, N., Baumgartner, P., von Braun, J., De Pinto, A., Graw, V., et al. (2011). The Economics of Land Degradation: Toward an Integrated Global Assessment. Development Economics and Policy. Vol. 66. Frankfurt am Main: Peter Lang. doi: 10.2139/ssrn.1890668
Nkonya, E., Mirzabaev, A., and von Braun, J, . (eds). (2016). Economics of Land Degradation and Improvement: A Global Assessment for Sustainable Development. Cham: Springer. doi: 10.1007/978-3-319-19168-3
O'Neill, B. C., Kriegler, E., Riahi, K., Ebi, K. L., Hallegatte, S., Carter, T. R., et al. (2014). A new scenario framework for climate change research: the concept of shared socioeconomic pathways. Clim. Chang. 122, 387–400. doi: 10.1007/s10584-013-0905-2
Pittelkow, C. M., Liang, X. Q., Linquist, B. A., van Groenigen, K. J., Lee, J., Lundy, M. E., et al. (2015). Productivity limits and potentials of the principles of conservation agriculture. Nature 517, 365–482. doi: 10.1038/nature13809
Potapov, P., Yaroshenko, A., Turubanova, S., Dubinin, M., Laestadius, L., Thies, C., et al. (2008). Mapping the world's intact forest landscapes by remote sensing. Ecol. Soc. 13:51. doi: 10.5751/ES-02670-130251
Potter, P., Ramankutty, N., Bennett, E. M., and Donner, S. D. (2010). Characterizing the spatial patterns of global fertilizer application and manure production. Earth Interact. 14, 1–22. doi: 10.1175/2009EI288.1
Reij, C., Tappan, G., and Belemvire, A. (2005). Changing land management practices and vegetation on the central plateau of Burkina Faso (1968–2002). J. Arid Environ. 63, 642–659. doi: 10.1016/j.jaridenv.2005.03.010
Reij, C., Tappan, G., and Smale, M. (2009). Agroenvironmental Transformation in the Sahel: Another Kind ofGreen Revolution. IFPRI Discussion Paper 00914. Washington, DC: International Food Policy Research Institute.
Reinecke, S., and Blum, M. (2018). Discourses across scales on forest landscape restoration. Sustainability 10, 1–19 doi: 10.3390/su10030613
Rietbergen-McCracken, J., Maginnis, S., and Sarre, A., (eds). (2007). The Forest Landscape Restoration Handbook. London: The Earthscan Forestry Library.
Robinson, S., Daniel, M-D, Islam, S., Sulser, T. B., Robertson, R., Zhu, T., et al. (2015). The International Model for Policy Analysis of Agricultural Commodities and Trade (IMPACT): Model Description for Version 3. IFPRI Discussion Paper 1483. Washington, DC: International Food Policy Research Institute.
Rosegrant, M. W., Koo, J., Cenacchi, N., Ringler, C., Robertson, R. M., et al. (2014). Food Security in a World of Natural Resource Scarcity—The Role of Agricultural Technologies. Washington, DC: International Food Policy Research Institute.
Rosenzweig, C., Elliott, J., Deryng, D., Ruane, A. C., Müller, C., Arneth, A., et al. (2014). Assessing agricultural risks of climate change in the 21st century in a global gridded crop model intercomparison. Proc. Natl. Acad. Sci. U.S.A. 111, 3268–3273 doi: 10.1073/pnas.1222463110
Rudel, T. K., Schneider, L., Uriarte, M., Turner, B. L., DeFries, R., Lawrence, D., et al. (2009). Agricultural intensification and changes in cultivated areas, 1970–2005. Proc. Natl. Acad. Sci. U.S.A. 106:20675–20680. doi: 10.1073/pnas.0812540106
Schultz, C. A., Jedd, T., and Beam, R. D. (2012). The collaborative forest landscape restoration program: a history and overview of the first projects. J. For. 110, 381–391. doi: 10.5849/jof.11-082
Sengupta, S., Maginnis, S., and Jackson, W. (2005). “Site-level strategies for restoring forest functions on agricultural land,” in Restoring Forest Landscapes: An Introduction to the Art and Science of Forest Landscape Restoration. ITTO Technical Series 23 (Tokyo: International Tropical Timber Organization and International Union for Conservation of Nature) 109–116.
Shiferaw, B. A., Okello, J., and Reddy, R. V. (2009). Adoption and adaptation of natural resource management innovations in smallholder agriculture: reflections on key lessons and best practices. Environ. Dev. Sustain. 11, 601–619. doi: 10.1007/s10668-007-9132-1
Sileshi, G., Akinnifesi, F. K., Ajayi, O. C., and Place, F. (2008). Meta-analysis of maize yield response to planted fallow and green manure legumes in sub-Saharan Africa. Plant Soil 307, 1–19. doi: 10.1007/s11104-008-9547-y
Smal, H., and Olszewska, M. (2008). The effect of afforestation with scots pine (Pinus silvestris L.) of sandy post-arable soils on their selected properties. Plant Soil 305, 171–187. doi: 10.1007/s11104-008-9538-z
Solomon, S., Qin, D., Manning, M., Chen, Z., Marquis, M., Avery, K. B., (eds.). (2007). Climate Change 2007: The Physical Science Basis. Cambridge; New York, NY: Cambridge University Press, 996.
Suding, K., Higgs, E., Palmer, M., Callicott, J. B., Anderson, C. B., Baker, M., et al. (2015). Committing to ecological restoration. Science 348, 638–640. doi: 10.1126/science.aaa4216
Teklewold, H., and Kohlin, G. (2011). Risk preferences as determinants of soil conservation decisions in Ethiopia. J. Soil Water Conserv. 66, 87–96. doi: 10.2489/jswc.66.2.87
Udawatta, R. P., and Jose, S. (2011). “Carbon sequestration potential of agroforestry practices in Temperate North America,” in Carbon Sequestration Potential of Agroforestry Systems: Opportunities and Challenges, eds B. M. Kumar, and P. K. R. Nair (New York, NY: Springer), 8, 17–42. doi: 10.1007/978-94-007-1630-8_2
United Nations Convention to Combat Desertification (UNCCD) (2017). The Global Land Outlook, 1st ed. Bonn: UNCCD.
Vanlauwe, B., Kihara, J., Chivenge, P., Pypers, P., Coe, R., and Six, J. (2011). Agronomic use efficiency of N fertilizer in maize-based systems in sub-Saharan Africa within the context of integrated soil fertility management. Plant Soil 339, 35–50. doi: 10.1007/s11104-010-0462-7
Wei, X., Shao, M., Gale, W., and Li, L. (2014). Global pattern of soil carbon losses due to the conversion of forests to agricultural land. Sci. Rep. 4:4062. doi: 10.1038/srep04062
Wiebe, K. D., Sulser, T. B., Pacheco, P., De Pinto, A., Mason-D'Croz, D., Dermawan, A., et al. (2019). The Palm Oil Dilemma: Policy Tensions Among Higher Productivity, Rising Demand, and Deforestation. Washington, DC: International Food Policy Research Institute (IFPRI).
Winterbottom, R., Reij, C., Garrity, D., Glover, J., Hellums, D., McGahuey, M., et al. (2013). Improving Land and Water Management. WRI Working Paper, Installment 4 of Creating a Sustainable Food Future. Washington, DC: World Resources Institute. Available online at: http://www.worldresourcesreport.org.
World Resource Institute (WRI) (2017). Atlas of Forest and Landscape Restoration Opportunities—FLR Key Characteristics. Available online at: http://www.wri.org/resources/maps/atlas-forest-and-landscaperestoration-Opportunities (accessed August 8, 2019).
Keywords: forest landscape restoration, land degradation, bonn challenge, food security, climate change
Citation: De Pinto A, Cenacchi N, Robertson R, Kwon H-Y, Thomas T, Koo J, Begeladze S and Kumar C (2020) The Role of Crop Production in the Forest Landscape Restoration Approach—Assessing the Potential Benefits of Meeting the Bonn Challenge. Front. Sustain. Food Syst. 4:61. doi: 10.3389/fsufs.2020.00061
Received: 30 September 2019; Accepted: 15 April 2020;
Published: 12 May 2020.
Edited by:
Robert Martin Rees, Scotland's Rural College, United KingdomReviewed by:
Mark Van Wijk, International Livestock Research Institute, KenyaDave Reay, University of Edinburgh, United Kingdom
Copyright © 2020 De Pinto, Cenacchi, Robertson, Kwon, Thomas, Koo, Begeladze and Kumar. This is an open-access article distributed under the terms of the Creative Commons Attribution License (CC BY). The use, distribution or reproduction in other forums is permitted, provided the original author(s) and the copyright owner(s) are credited and that the original publication in this journal is cited, in accordance with accepted academic practice. No use, distribution or reproduction is permitted which does not comply with these terms.
*Correspondence: Alessandro De Pinto, YS5kZXBpbnRvQGNnaWFyLm9yZw==