- 1Department of Nutrition and Food Sciences and Food Systems Program, University of Vermont, Burlington, VT, United States
- 2Gund Institute for Environment, University of Vermont, Burlington, VT, United States
- 3Department of Environmental Science and Policy, University of California, Davis, Davis, CA, United States
- 4CGIAR Research Program on Climate Change, Agriculture and Food Security (CCAFS), Alliance of Bioversity International and International Center for Tropical Agriculture (CIAT), Nairobi, Kenya
Agricultural adaptation to climate change is critical for ensuring future food security. Social capital is important for climate change adaptation, but institutions and social networks at multiple scales (e.g., household, community, and institution) have been overlooked in studying agricultural climate change adaptation. We combine data from 13 sites in 11 low-income countries in East Africa, West Africa, and South Asia to explore how multiple scales of social capital relate to household food security outcomes among smallholder farmers. Using social network theory, we define three community organizational social network types (fragmented defined by lack of coordination, brokered defined as having a strong central actor, or shared defined by high coordination) and examine household social capital through group memberships. We find community and household social capital are positively related, with higher household group membership more likely in brokered and shared networks. Household group membership is associated with more than a 10% reduction in average months of food insecurity, an effect moderated by community social network type. In communities with fragmented and shared organizational networks, additional household group memberships is associated with consistent decreases in food insecurity, in some cases up to two months; whereas in brokered networks, reductions in food insecurity are only associated with membership in credit groups. These effects are confirmed by hierarchical random effects models, which control for demographic factors. This suggests that multiple scales of social capital—both within and outside the household—are correlated with household food security. This social capital may both be bridging (across groups) and bonding (within groups) with different implications for how social capital structure affects food security. Efforts to improve food security could recognize the potential for both household and community level social networks and collaboration, which further research can capture by analyzing multiple scales of social capital data.
Introduction
Climate change impacts on food and agriculture systems are not only projected in the future, but are already felt at multiple scales (Moore and Lobell, 2015; Ramankutty and Iizumi, 2016; Hoffman et al., 2017; Fuller et al., 2018). Indeed, increasing levels of malnutrition and food insecurity in recent years (a challenge for more than 815 million people globally) are attributed, at least in part, to climate changes and an increase in extreme events (FAO, 2018). A large body of research has explored the potential impacts of climate change on crop yields and other agronomic components both globally (Ramankutty and Iizumi, 2016), and increasingly regionally and locally (Moore and Lobell, 2015; Hoffman et al., 2017; Fuller et al., 2018). Smallholder farmers, estimated to number more than half a billion (Lowder et al., 2016), are particularly reliant on rain-fed agriculture (Rockström et al., 2003), and are expected to be among the most vulnerable to these climate change impacts (Morton, 2007). Studies to examine the impact of such changes on farmers, especially smallholder farmers, and their potential adaptation responses to maintain food security have been steadily increasing [e.g., (Kristjanson et al., 2012; Douxchamps et al., 2016; Niles and Brown, 2017; Ritzema et al., 2017)].
However, recent agricultural adaptation research has largely focused on natural science and farmer surveys, with studies on social networks and institutions much less frequent (Davidson, 2016). While there are many biophysical and agronomic factors that can affect agricultural adaptation and food security (Wheeler and von Braun, 2013; Lipper et al., 2014), social networks and institutions may also be relevant. Here we draw upon network and social capital theories to link measures of community and household social capital to household food security outcomes across more than 1,800 households in 13 sites in 11 countries in Sub-Saharan Africa and Asia. We leverage a unique global dataset from the CGIAR Research Program on Climate Change, Agriculture and Food Security (CCAFS) that has assessed climate change adaptation and agricultural development organizations and households around the world, and build on existing studies that have characterized and quantified multiple levels of social capital in each CCAFS research site (Niles and Brown, 2017; Rudnick et al., 2019). To our knowledge, this is the largest study of its type to collect and link data across multiple regions and villages, at both the individual and community levels, to assess social capital and food security.
Understanding how human actors within agricultural systems will adapt to climate change requires integrating social science theory that examines institutions and individual behavior. Social science theory suggests that social capital is a fundamental component of adaptive capacity and resilience (Adger, 2003; Neil Adger et al., 2005; Wolf et al., 2010). Though the term social capital has evolved over time (Putnam, 2000), it broadly refers to the ways in which people and organizations form connections through social networks and relationships, which can build trust and reciprocity to facilitate cooperation, collective action, and resilience (Dietz et al., 2003; Ostrom and Ahn, 2003, 2009). These relationships can occur at different scales (i.e., between individuals, households, or organizations) and establish pathways for exchange of knowledge, resources and influence. Social capital consists of both bonding (relationships within communities) and bridging (relationships beyond communities and across social boundaries) types. Social capital research and theory posit that higher levels of connectedness within a network of other actors can lead to improved resilience and adaptive capacity (Aldrich and Meyer, 2014). However, while social capital theory and research generally explore the positive relationships resulting from social capital, there is evidence that bonding social capital can also have negative outcomes especially when it concentrates power. For example, as Portes notes about this “dark side” of social capital (p. 15), “the same strong ties that bring benefits to members of a group commonly enable it to bar others from access” (Portes, 1998).
In the context of climate change, social capital has been explored extensively including in response to, or preparation for, extreme events (Allen, 2006; Wolf et al., 2010; Chriest and Niles, 2018), island communities (Petzold and Ratter, 2015), individual resilience (Smith et al., 2012), and migration (Scheffran et al., 2012). Social capital may be important for climate change adaptation for a variety of reasons, including the ability of social networks to serve as lifelines in extreme events (Aldrich and Sawada, 2015), facilitating access to needed resources or information, increasing the capacity for trust, reciprocity, and cooperation to foster communities helping each other as the climate changes (Paul et al., 2016; Chriest and Niles, 2018), and the ability for trust to enable people and organizations to better plan, implement and respond to climate impacts (Kettle and Dow, 2014). Particularly relevant for this work, Adger (2003) suggests that social capital links between society and the state are critical for climate change adaptation. In well-functioning states, networks in the state and society are complementary, whereas in poor functioning states, networks within society may substitute for networks at the state level- i.e., societal networks substitute for governments.
At the level of individual households, social capital research has focused on how social networks catalyze peer-to-peer learning, innovation, adoption of sustainable agriculture practices, and how networks motivate greater participation in resource governance or conservation programs (Bandiera and Rasul, 2006; Lubell et al., 2014; Saint Ville et al., 2016), which may be critical for climate change adaptation. In short, existing research confirms that greater levels of social capital within individual households is related with behavioral changes and outcomes associated with resilience (e.g., sustainable agricultural practice adoption, access to resource programs). Further evidence in both high and low-income countries demonstrates positive relationships between social capital, particularly household participation in community groups or organizations (8, 31), and improved food security outcomes in both urban and rural settings (Walker et al., 2007; Misselhorn, 2009; Dean and Sharkey, 2011). Farmers in agricultural intervention projects have larger social networks and more sources of knowledge and information, produce a greater number of crops and have more diversified livelihood strategies (Cadger et al., 2016). Social networks may reduce climate vulnerability by improving capacity to adapt to stressors and increase household food security through times of hardship (Martin et al., 2004; Cadger et al., 2016; Niles and Brown, 2017). Furthermore, reciprocity among neighbors through exchange or lending of food or resources may explain the positive relationship between household social capital and food security (Martin et al., 2004; Quetulio-Navarra et al., 2017) and the negative relationship between social support and health outcomes (Ahmadi et al., 2017). Pooled resources among informal community groups help mitigate risk and better incorporate poor and vulnerable community members, resulting in better success in livelihood activities and an increased resilience and ability to adapt to climate change (Rodima-Taylor, 2012).
At the community level, social capital and networks research has focused on how the structure of collaborative networks influence levels of cooperation, learning, and integration among organizations and between households. Since community can have many definitions, we focus primarily here on administratively defined villages and those organizations working within those villages and in the surrounding geographical locality. These organizations typically include non-governmental organizations, international development groups, and national, regional and local government ministries or offices. A major focus has compared the effects of centralized versus decentralized organizational networks and network leadership on activities and coordination across organizational networks (Provan and Kenis, 2008). Decentralized networks (e.g., shared networks) that distribute leadership and decision-making power across many actors may allow more local knowledge integration and promote specialization among organizations, both of which may be important to effective food security initiatives. Conversely, decentralized networks may be inefficient and have high transaction costs required to maintain many collaborative relationships across organizations, which may reduce what limited resources are available from going directly toward food security and climate resilience initiatives (Johanson and Mattsson, 1987; Ramirez et al., 2018). In comparison, centralized networks (e.g., brokered networks) often are hierarchically structured with a single or few organizations acting as the key leader or decision-maker who directs coordinated network activities. In low-income settings, some organizations may lack the capacity or resources to take on leadership or collaborative relationships; thus, a centralized network leader offers an efficient and lower cost structure to organize network activities (Mitlin et al., 2007; Ramirez et al., 2018). Centralized networks however concentrate political and resource power into a single organizational actor, and thereby become vulnerable to corruption or organizational selfishness, both of which can threaten the effectiveness of the entire network's activities (Gisselquist, 2012; Hoogesteger, 2016). International development organizations have been associated with greater network coordination overall, but local and regional organizations fill network leadership positions most frequently. This may mean that resources are needed for network coordination, but that social capital, in the form of trust and influence, is most important for effective network leadership (Rudnick et al., 2019).
Where current research lacks understanding is in the relationship between community-level (i.e., between organizations) and individual-level (i.e., between individuals or households) social capital, and how these relationships may vary across communities to affect resilience. There is less empirical work investigating how social capital at various scales (i.e., individuals, households, and community) interact and are related (Paul et al., 2016); for example, theory suggests that multi-level networks are necessary to develop social capital (Dietz et al., 2003), but few studies have empirically examined multiple scales of social capital for climate change adaptation using network analysis (Ingold et al., 2010). This is critical because it suggests that very little is known about how community and individual-level social capital and networks relate to each other, which may be vitally important for determining effective climate adaptation interventions that are complementary across network scales in a particular context. Existing theories would suggest that high levels of social capital lead to more resilient outcomes; but is this true across scales? Does household-level social capital relate to levels of social capital within communities? Do these multiple-scales of social capital relate to household resilience?
To understand these questions through a multi-level social capital lens, we explore how community social capital (measured as organizational network structures among non-governmental organizations, government institutions, and other organizations operating within the community; see Table 1 below and Rudnick et al., 2019) is related to individual household social capital (measured as households' group memberships across 13 total types of locally organized production and savings-oriented groups). Drawing on network and social capital theory, we suggest that community-level social capital may be possible to categorize into three types based on the structure of organizational coordination networks; fragmented communities which have the least social capital (fewest network connections), followed by brokered communities (higher connectivity, centralized through a core actor) and finally shared communities (highest density of network connections). Using this framework, we hypothesize that (H1) in communities where social capital is low (i.e., little coordinated activity and collaboration, resulting in fragmented community networks) household social capital will also be low (fewer group memberships). We then evaluate the impact of multi-level social capital on food security outcomes (a type of resilience), controlling for demographic factors. We hypothesize that (H2) the structure of community social capital will mediate the relationship between household social capital and food security, such that group membership has the strongest effect on food security in communities with higher social capital.
Methods
Sites and Context
The original data from both the household and community levels were collected by the Climate Change, Agriculture, and Food Security (CCAFS) Research Program, part of the CGIAR. The CCAFS program selected core sites within their focus regions of East Africa, West Africa and South Asia [see (Förch et al., 2013) for additional methodological details on site selection]. Baseline data was collected between 2010 and 2012 in 15 sites including East Africa: Makueni and Nyando, Kenya; Rakai and Hoima, Uganda; Lushoto, Tanzania; Borana, Ethiopia; West Africa: Yatenga-Tougou, Burkina Faso; Lawra-Jirapa, Ghana; Segou, Mali; Kollo, Niger; Kaffrine, Senegal; South Asia: Bagerhat, Bangladesh; Karnal and Vaishali, India; Rupandehi, Nepal. In this analysis, data from Karnal, India and Yatenga-Yougou, Burkina Faso are excluded because CCAFS has advised that there may be large potential errors as a result of inconsistent data collected in these regions (Sites shown in Figure 1).
CCAFS has detailed their methodology and site selection, as well as key site-specific details around geography, farming systems, and culture (Förch et al., 2013), which we detail briefly here for the sites. The three regions and sites of focus were chosen based on criteria including: high vulnerability to climate change, poor and vulnerable populations, complementary social, cultural and institutional contexts, complementary climatic contexts, significant but contrasting climate problems, and security, governance and institutional capacity to favor the potential to generate transferable results. In East Africa, the sites are characterized by mixed rainfed crop-livestock, pastoral, and subsistence farming systems. In Uganda, the sites also includes agroforestry including coffee and tea. In Rakai, Uganda tobacco and sugar cane are other cash crops. In West Africa, the sites are characterized by small-scale mixed crop-livestock or pastoralist farming systems and agriculture is the dominant economic activity. In South Asia, the agricultural systems are characterized by rice, as well as wheat, sugarcane, banana, and fruits in Bangladesh and India. In Bangladesh, shrimp culture is also prevalent. In India and Nepal, rice is also dominant, along with wheat, pulses and oilseeds and cash crops are grown with irrigated agriculture. Crops are typically integrated with livestock, and most farmers produce food for mainly home consumption. We have included additional information as it relates to farming products, inputs and management by site in Supplementary Table 1 to enable a better understanding of these systems.
Organization Surveys and Network Structures
Organizational data were collected through the CCAFS Organizational Baseline Survey (OBS), in which local research teams visited locally relevant organizations working on issues of climate change and food security within the region. Organizations were nominated for inclusion by village resident focus groups indicating they were among the most important organizations within their communities. Organizations spanned NGOs (e.g., development groups, indigenous organizations, religious organizations, women's organizations, government (local, regional and national)AQ agencies, and private sector organizations ranging in scale from local to international, all of which had work focused, at least in part, on agriculture, climate change and food security. The size of the organizations varied widely, since some were local and some international development organizations. The OBS included questions that asked organizations to name other organizations that they work or collaborate with, which formed the basis for a social network matrix, which included all organizations that were surveyed, as well as additional organizations named as partners, but who were not surveyed themselves. In total, CCAFS surveyed 145 organizations and an additional 270 organizations were named as collaborators in the survey. The network analysis represents a binary relationship between two organizations, and surveys also contributed data about organization type, scale, and work focal area, which were coded as attributes. A network analysis was conducted using R Statistical Software version 3.2.2 and the Statnet package (Hancock et al., 2003). Additional details on the network analysis methodology can be found in (Rudnick et al., 2019). Previous analysis determined that three distinct network types exist within these organizational structures-shared, brokered, and fragmented (differences illuminated in Table 1).
Household Data
Household data were collected through the CCAFS baseline household survey, conducted between 2010 and 2012 across the aforementioned sites within a 10 by 10 km sampling frame. Villages were chosen at random within this sampling frame, and CCAFS worked with village elders to identify households within the villages. Additional information on data collection can be found in (Förch et al., 2013). A total of 1,815 smallholder farmer households are represented in this dataset across 13 villages. The key dependent variable at the household level included in our analysis was the number of months food insecure (range 0–12), which was asked, “in which months if any do you tend to find you do not have enough food to eat for your family.” This metric is does not capture longitudinal data over time, instead only reflects an understanding of food insecurity at one time point, asked in reflection of the previous year. The key independent variable included was the number of community groups the household belonged to (“household group membership”), which consisted of 13 different groups that respondents could indicate membership in (range 0–13). These organizations included savings/credit, productivity, tree nursery/planting, marketing, vegetable, irrigation, crop, forest, soil improvement, water management, seed, fishing, and fish/shrimp pond groups (Supplementary Table 2 for more information about group types). This question was phrased, “Do you, or any other household member, belong to a group or groups doing the following activities?” This variable was utilized in multiple formats (e.g., any group membership, total number of absolute group memberships, and membership to a specific type of group). It is worth noting that the social capital of these group memberships may also facilitate other types of capital- e.g., financial capital through credit groups, or natural resource capital through irrigation groups. These household membership groups were likely distinct from the organizations surveyed in the OBS, and tended to be smaller village-based organizations operating at the hyper-local scale. It is would not be uncommon however, that these organizations may be affiliated or related. For example, local irrigation groups with household members may interact with local governments or state agencies. We also utilize demographic data including female-headed household status, number of people living in the household (household size), and the highest education level of any member of the household (range 0–3). We report average and standard deviations for these demographic controls in Supplementary Table 3 for each site.
Furthermore, we develop a measurement of household assets to control for wealth within a given community. The survey included questions about absence/presence of 25 different household assets including: radio, television, cell phone, bicycle, motorcycle, car or truck, computer, solar panel, tractor, mechanical plow, mill, improved stove, generator, large battery, water pump/treadle pump, biogas digester, refrigerator, air conditioning, electrical fan, thresher, liquified petroleum gas (LPG), internet access, boat, fishing nets, and bank accounts. Such questions are often utilized in low-income context to measure aspects of wealth (Filmer and Pritchett, 2001), and have been typically developed into asset indexes (Howe et al., 2012), which have proven effective in measuring poverty [e.g. (Sahn and Stifel, 2003)]. We developed an asset index, a count variable from 0 to 25, in which the presence of each asset for a given household was aggregated, the simplest form of an asset index (Howe et al., 2012). However, since we are using data across multiple regions and household assets in Nepal, for instance, may be significantly different than household assets in Kenya, and thus influence food security outcomes, we further normalized the asset scale to a given community. Supplementary Table 4 shows the mean asset scale, standard deviation, and cut-off points for one standard deviation above and below the asset mean in a given community. Households that had an asset score one standard deviation or more below their community mean was categorized as a “poorest” household. Conversely, households that had an asset score of one standard deviation or more above their community mean was categorized as a “wealthiest” household. Thus, the binary categorization of poorest or wealthiest households in our models are normalized to the given community and region in which a household lives. Means and standard deviations of these poorest and wealthiest community members by site are shown in Supplementary Table 4.
Statistical Analysis and Multivariate Models
We ran a series of statistical tests and multivariate models to test our hypotheses. An analysis of variance (ANOVA) was used to test H1, the relationship of household group membership to network type and group type differentials among network types. To test H2, we used an ANOVA across the entire population to look at the relationship of group membership at the household level to food insecurity and to explore food security outcomes within different community network types and household group membership types. Multivariate generalized linear models with random effects (Garson, 2013) were used to explore the relationship of food insecurity to both total group membership and group membership in credit groups, which were the most frequent type of group membership. Models included a binomial logit link, since food insecurity was measured in presence/absence across each month from 0 to 12. Random effects are included at the household and village level, to account for variability at these levels. We also include five control variables at the household level, which have been shown in other studies to be related to food security outcomes (Niles and Brown, 2017; Niles and Salerno, 2018) including female-headed household status, household size, household education and poorest and wealthiest households for a given region. Given the potential importance of wealth in affecting both food security outcomes and group membership variables of interest, we ran our models both with and without poorest and wealthiest household controls to assess omitted variable bias (Supplementary Tables 5–7). We find no differences in the coefficients statistical significance or direction between the two models, suggesting that our results are likely not being driven by omitted variable bias (Chiappori et al., 2012). We recognize that food insecurity is a function of many diverse factors not only including demographics, assets, and social capital. However, our intention is not to conduct a comprehensive assessment of food insecurity, but instead to explore this outcome in a complex multi-scale assessment of social capital. We have explicitly included variables (e.g., wealth) that likely reflect farming system outcomes such as subsistence production, livestock or cash crops through inclusion of asset variables. Previous research has more clearly explored how farming systems, farm and crop characteristics influence food security [e.g., (Kristjanson et al., 2012; Wood et al., 2014; Silvestri et al., 2015)], which we acknowledge is critical, but outside the scope of this work.
Data Availability
Data utilized in this analysis is available in several places. The original organizational data is available in Harvard Dataverse [Climate Change Agriculture and Food Security (CCAFS)], as well as the original household datasets from CCAFS (CCAFS, 2016).
Results
Household and Community Social Capital
In support of H1, we find that higher community social capital is correlated with higher individual household social capital. Specifically, we find that mean household group membership is lowest in fragmented network communities, which have the least organizational network connections, followed by shared and then brokered network communities. On average, households in fragmented network communities have 0.33 group memberships, while households in shared networks have 1.07 group memberships, and household in brokered networks have 1.13 group memberships (p < 0.000). The distribution of household group memberships across the three network types also shows marked differences with 78.6% of households in fragmented network communities having no group memberships, compared to 20.6 and 42.3% of households in brokered and shared network communities, respectively. In brokered network communities, it is most common for a household to have membership with only one group (55.6%), as compared with households in fragmented (15.1%) or shared (32.2%) network communities. Shared network communities had the highest frequency of households in three or more groups (11.0%) (Figure 2).
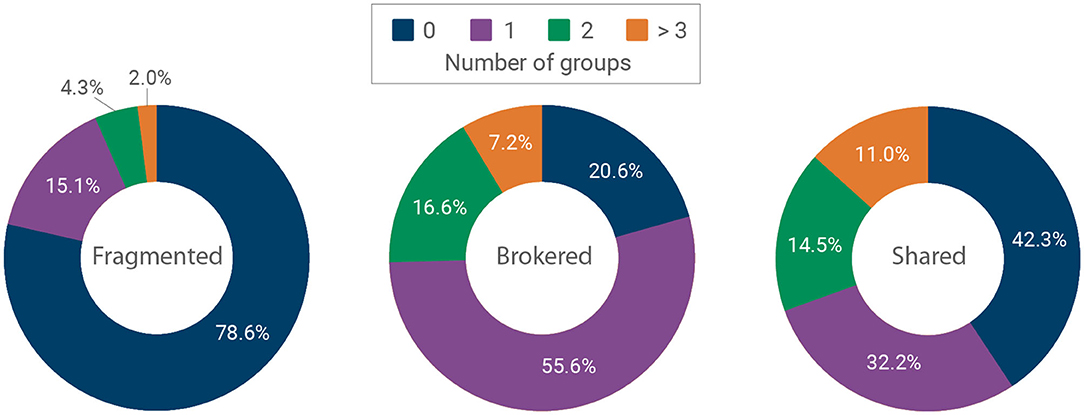
Figure 2. Relationship of community social capital (community network types) to individual household social capital (household group membership).
When we evaluate what specific types of groups households were most involved in, we find the top five types of groups across all community sites include those focused on savings and/or credit (generally, not agriculture specific), marketing of agricultural products, productivity enhancement, tree nursery/tree planting, and vegetable production (Supplementary Material, Figure 2). Household membership in these five types of groups account for 80% of all group membership in fragmented and shared network communities and 91% of all group membership in brokered network communities. Credit groups are the single most common type of group membership across all network types. In brokered network communities, where membership in one group was most common across households, credit group membership was significantly higher (65%) than in other communities (p = 0.0001) (Figure 3).
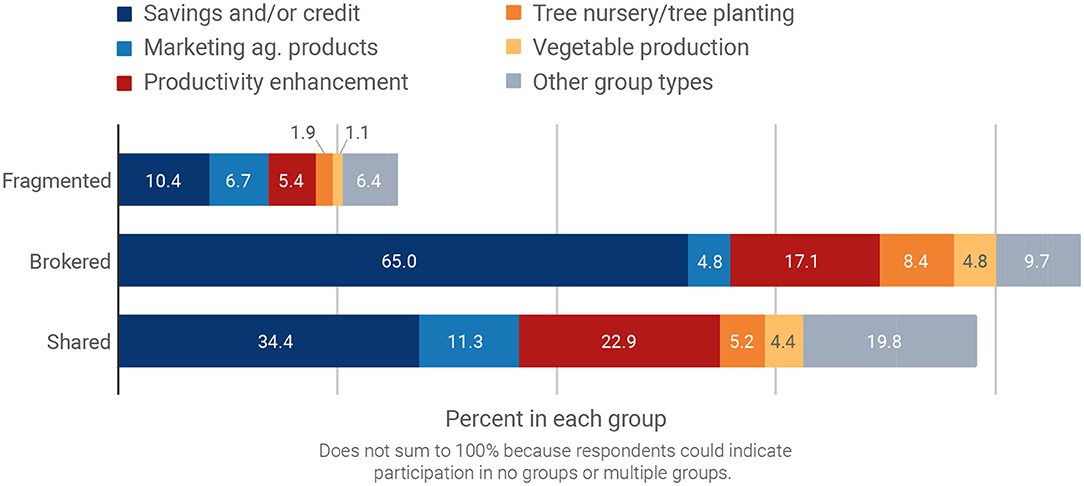
Figure 3. Group prevalence across community network types. These five groups represent the majority of household group memberships. Full details of all group memberships in Supplementary Table 1.
Multiple Scales of Social Capital and Food Security
Households participating in at least one group (of any type) experienced on average 10% fewer months of food insecurity than those without any group membership (mean months food insecurity 3.60 for no group membership compared to 3.22 for any group membership, p = 0.005). This supports part of our H2 that higher social capital at household levels correlates with better food security outcomes at the household level.
Comparing the average number of months of household food insecurity across community network types, we also find significant differences. Households in shared network communities (highest social capital) have the lowest average rates of food insecurity (3.08 months), followed by households in fragmented network communities (least social capital) (3.48 months) and then households in brokered network communities (mid-range social capital) (3.85 months) (p = 0.0001). Further evaluation of both household group membership and community network type together, clarifies these results in alignment with H2. For both fragmented (p < 0.000) and shared networks (p < 0.000), increases in household group membership are associated with a consistent decrease in months of food insecurity (Figure 4). This supports our hypothesis (H2) that increases in household social capital are associated with better food security outcomes at the household level, but suggests community social capital may mediate this relationship. For brokered network types however, the effect of household group membership is more nuanced. In these communities, households who participate in only one group experience a decrease in the average months of food insecurity. Conversely, in brokered network communities, participation in zero groups or participation in two or more groups is correlated with increased months of food insecurity (p = 0.023). This suggests potential interactions occurring between household and community-level social capital, rather than consistent effects of household social capital across all types of communities.
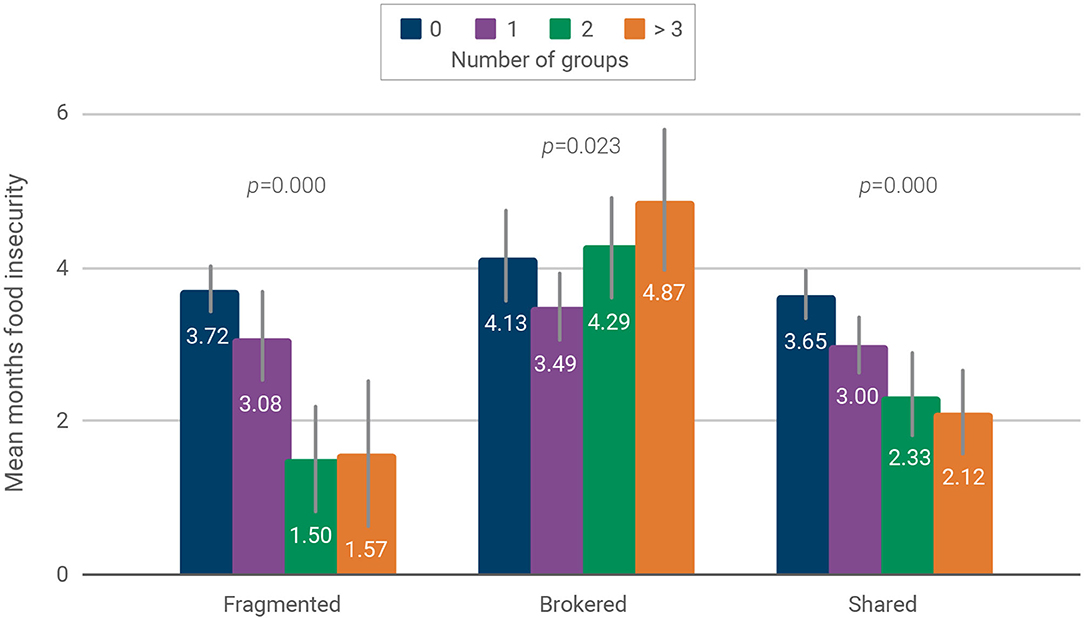
Figure 4. Average months of food insecurity across household group membership by community network type. Lower numbers indicate reductions in food insecurity (i.e., greater food security).
Further, when examining what types of groups households participate in, we find that only credit groups are consistently associated with reduced food insecurity at the household level across all community network types (Table 2). Within brokered networks, household membership in credit groups is associated with 3.65 months food insecurity on average, compared to 4.23 months for those households who are not participating in a credit group (p = 0.05). All other groups in brokered communities except vegetable production groups (p = 0.06) are associated with higher rates of food insecurity (p < 0.001), although some of these groups (i.e., marketing) are not statistically significant. Conversely, in shared network communities, household memberships in any type of group, except marketing groups, are associated with lower rates of food insecurity (p < 0.05). Likewise in fragmented network communities, household membership in any type of group is associated with lower rates of food insecurity, though only membership in credit, productivity, and marketing groups are statistically significant (p < 0.05). This suggests that both the number and type of group memberships may have varying effects across different community network types.
Hierarchical Models Exploring Group Membership and Food Security
Lastly, we utilize generalized linear models with random intercepts to explore how household social capital and demographic controls in different community network structures correlate with food security. Since we are specifically testing hypotheses related to social capital at household and community levels in relation to household food security outcomes, we include credit group membership (since only credit group membership was associated with lower average months of food insecurity across all network types) and total number of groups that households participate in (excluding credit) as two separate measures of social capital. We also include five demographic controls (household size, household education, female-headed households, poorest and wealthiest households) across network types. Since including many control variables does not necessarily lead to better model estimates (Clarke, 2005), we limit ourselves to socioeconomic and demographic controls that have been previously found to affect food security in similar smallholder populations [e.g., (Hadley et al., 2007; Silvestri et al., 2015; Niles and Brown, 2017; Niles and Salerno, 2018)]. We were particularly concerned with controlling for confounding variables such as wealth, which could jointly predict both the capacity to access group memberships (especially credit) and food security, and thus creates the risk that the estimated relationship between group memberships and food security would be biased due to endogeneity.
Models confirm our descriptive findings that credit group membership, across all network types, is consistently associated with reductions in the number of months of food insecurity, though it is only statistically significant for brokered (b = −0.349, p = 0.019) and shared networks (b = −0.454, p < 0.001) (Table 3). Conversely, total number of other group memberships (not including credit membership) is associated with reductions in the number of months of food insecurity in fragmented (b = −0.425, p = 0.000) and shared networks (b = −0.099, p = 0.018), but is associated with increases in the number of months of food insecurity (b = 0.271, p = 0.003) in brokered networks.
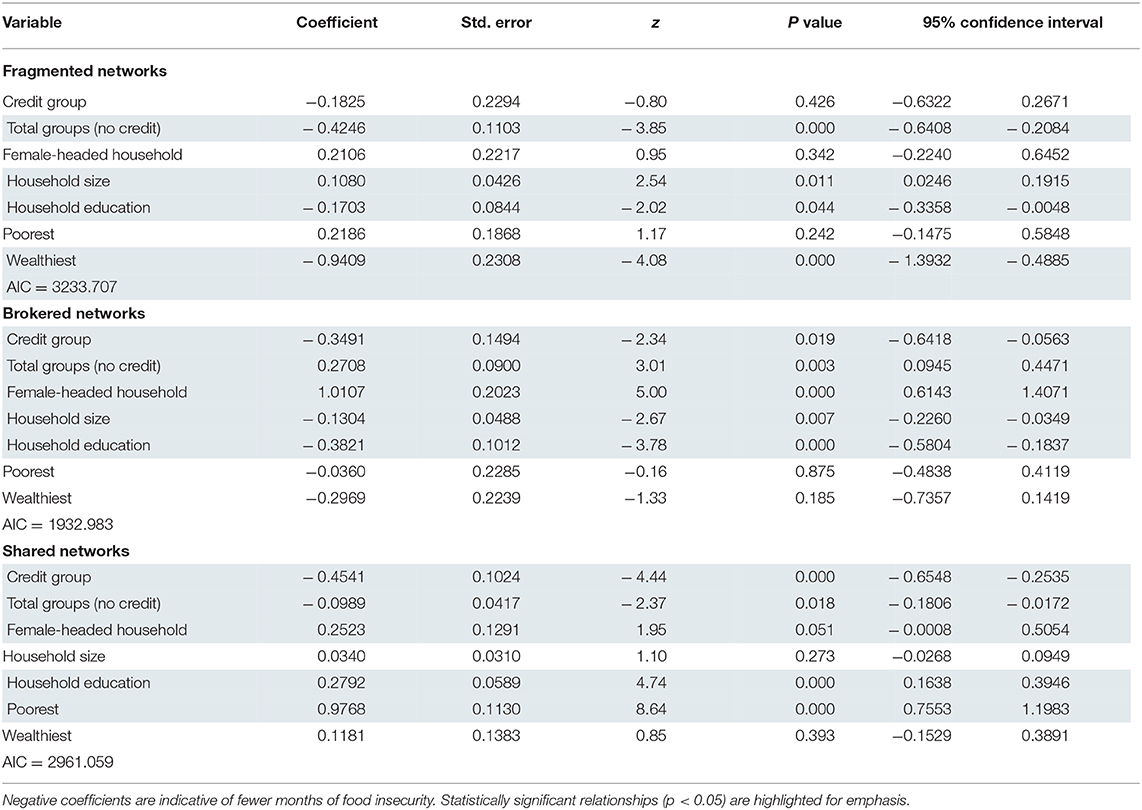
Table 3. Generalized linear model results of food insecurity as a function of credit group membership and total group membership.
Models also demonstrate female-headed households are associated with higher rates of food insecurity, which is statistically significant in brokered (b = 1.01, p < 0.001) and shared networks (b = 0.252, p = 0.051). Larger households are significantly associated with reduced food insecurity in brokered networks (b = −0.130, p = 0.007), but associated with higher food insecurity in fragmented networks (b = 0.108, p = 0.011). Higher rates of formal education in the household are positively associated with reductions in food insecurity in fragmented (b = −0.170, p = 0.044) and brokered networks (b = −0.382, p < 0.001) but positively associated with food insecurity in shared networks (b = 0.279, p < 0.001). Finally, wealth is also associated with reduced food insecurity, but only significantly in some communities. Being in the poorest households in a shared network is positively associated with higher rates of food insecurity (b = 0.977, p < 0.001) such that poorest households have almost one additional month of food insecurity. Conversely, being in the richest households in fragmented networks is negatively associated with food insecurity (b = −0.941, p < 0.001) in that these households have almost one month reduction in food insecurity.
Discussion
We find significant associations between household and community-level social capital and their relationship to household food security outcomes. Fragmented network community types, which have the lowest community-level social capital (i.e., fewest number of connections among organizations), have the lowest average household group membership. Theoretically, our work suggests that there are clear relationships between multiple scales of social capital; when social capital is low among households, it is also more likely to be low among organizations working in those same communities (or vice versa, the directionality of the relationship remains to be understood). Additionally, there appear to be five dominant types of groups (out of 13 considered, see Supplementary Table 1) that make up more than 80% of all household group memberships across all community types, with household credit groups being the most common group membership, and significantly more common than all other group types in brokered communities. Households have the most diverse group memberships in shared networks, where there are higher levels of collaboration among the organizations, or higher community-level social capital. Overall, the rate of group membership is consistent with other studies from similar regions, finding smallholder farmer participation groups ranging from 32% (Gyau et al., 2016) to the mid 40% of surveyed farmers (Abdul-Rahaman and Abdulai, 2018; Sinyolo and Mudhara, 2018).
We also find evidence that the relationship between group membership and food security is mediated by the structure of community-level social capital. The results are consistent with H2, especially in the case of membership in credit groups, which was positively associated with food security across all community types. This basic finding corroborates the argument that credit access is a critical resource for food security (Wossen and Berger, 2015; Niles and Brown, 2017), as well as improved adaptation to climate change (Bryan et al., 2009). However, the positive effect of credit group membership is strongest in shared networks, followed by brokered networks, and with a very small estimate in fragmented networks. This suggests that credit groups may be more effective when embedded in well-connected community development networks, such as those with a shared network structure. This structure may allow for more collaborative organizational structures, enabling credit groups to be better connected to other organizations and to households. Such community-level social capital may be especially important in communities where the capacity for reciprocity or food lending among other community members may be limited or households lack resources (Paul et al., 2016).
Intriguingly, the effect of memberships in other types of groups, other than credit groups, is not consistent with our simple hypothesis (H2) that household social capital is more effective as community-level social capital increases. Other group memberships are positively associated with food security in fragmented and shared network communities, but negatively associated with food security in brokered communities. The mixed findings suggest that broader types of social capital may be operating via different mechanisms in different types of communities, which stimulates some intriguing possibilities for future research, including how social capital may relate to or interact with other types of capitals (e.g., natural, human) within a given place.
Specifically, we speculate, drawing upon Adger (2003) that the positive correlation of other group memberships in fragmented network communities to household food security, may represent a mechanism where group membership is a substitute for community-level social capital. Absent organized community networks, additional household group memberships may expand trust, reciprocity and access to resources among households, where there may otherwise be less organized activity or aid [e.g., (Martin et al., 2004; Quetulio-Navarra et al., 2017)]. Conversely, in shared networks it may be that connected community organizations are a complement to individual-level social capital especially by providing bridging social capital that goes beyond the community and connects to more types of organizations (Karlsson and Hovelsrud, 2015). This is supported by the fact that shared, more densely connected community networks have a higher presence of international actors, which may bring additional resources to a community and enable relationship and capacity building through bridging capital (Rudnick et al., 2019). Indeed, communities that organize and cooperate have been shown to better access external support for climate change adaptation (Karlsson and Hovelsrud, 2015) and active participation of local organizations results in better food security outcomes among households in those communities (Thornton et al., 2018).
The negative effect of other group membership in brokered communities is more puzzling, but may be a clue to the potential negative consequences of concentrating power into a single or few actors (i.e., the central organization or “network broker”) and accentuating the influence of bonding social capital (Portes, 1998). Brokered community networks may concentrate power in one or two key actors, which then have outsized authority over the distribution of resources between more peripheral organizations (Panda, 2015). Beyond these powerful central actors, the other groups may be less organized or even become a source of group conflict, such that household connections to those groups are a drain on food security and other social and economic outcomes. Such power differentials may result in stratification among households in the community, where only a subset of households have access and connection to the key actors (Chaudhury et al., 2017). Previous research has found that strong bonding social capital, which may be present among connections to the highly centralized actor within a brokered network, can foster in-group favoritism and conformity, which is also associated with corruption (Wachs et al., 2019). In the case of this work, it may be that the network structures in brokered communities lead to a concentration in critical resources only among credit groups, and actually reduce the effectiveness of the other groups. The central actors in such communities may in fact have “captured” or gained a “policy monopoly” on social capital, which reinforces their central status over time.
Our work has important limitations that point the way for additional research. First, we cannot make a strong causal claim about the relationship between household social capital and food security. Our analyses account for some of the main threats to the identification of a causal effect, in particular by controlling for household wealth and using random effects models, but still does not allow for causal inference. Longitudinal data collection that could enable difference in difference approaches, or other causal statistical techniques could improve this understanding. Second, while we have nearly 2,000 households in our dataset, there are only 13 sites across which to assess and compare community-level social capital, which limits our ability to estimate how the effect of individual social capital varies across sites. Expanding the scale of this type of analysis to more regions and sites within regions would allow a more precise estimation of how the direction and magnitude of the relationship between household social capital and food security varies across community context including the structure of development networks. Third, our measures of household social capital are not explicitly connected to specific organizational nodes in the community-level networks. For example, the manner in which our data was collected does not allow us to know if the household tree-planting group in a given site is connected to or the result of the international NGO working on natural resource management in the same given site. To address this problem, we recommend the collection of multi-level network data that explicitly ties households to identifiable organizations in the community-level networks. This would enable the estimation of more sophisticated statistical models of multi-level networks that link different types of community-level network structures to household outcomes (Bodin et al., 2019), for example the dynamics of bonding versus bridging social capital under different community network structures. Finally, we acknowledge there are other factors that influence food security, including farm systems and agricultural markets and outputs. The focus of this work was to explicitly consider relationships between social capital at varying scales and food security outcomes, while controlling for demographic factors that have previously been shown to affect food security. While we believe that these demographic controls account for some of the farm system and agricultural factors that could influence food security, (e.g., controlling for wealth could capture market access, cash crop growth, and farm size), we certainly have not included all possible predictors of food security in our model.
Future research with this scale and depth of data could certainly explore a rich network analysis to further test our hypotheses and understand multi-level social capital in the context of food security and climate change adaptation. Furthermore, we suggest that this methodological approach and its implications could have important relevance for exploring other important development goals and outcomes at household and community levels within low-income country contexts. Utilizing network analysis and explicitly gathering data in this way, thus may provide important insights for researchers and policymakers at the interface of achieving the Sustainable Development Goals.
Conclusion
Our analysis is the first attempt to link multiple scales of social capital in the context of food security and climate change adaptation across multiple low-income countries. We find evidence that both household and community social capital are related to household food security, but that there are important interactions between social capital at these different scales. Overall household group membership is correlated with better food security outcomes, but the type of group and the way that organizations within that community collaborate appear to correlate with these outcomes. In shared and fragmented networks, there are additive effects of additional household group memberships on food security outcomes, albeit with potentially different mechanisms at play (i.e., substitution vs. complementarity). Conversely, in brokered networks, participation in credit groups is the only type of household membership that correlates with better food security outcomes, which we theorize may be explained by the possible negative consequences of bonding social capital in networks where power is concentrated in a few central actors. Importantly, all outcomes control for household demographics and socioeconomic factors, which may also influence food security outcomes, including gender, household education, size, and wealth. As such, we suggest that research and development efforts aimed to improve food security outcomes need to consider not only household factors, but also the structures of community organizations in the sites that they work, in order to secure effective outcomes.
These results suggest that social capital at multiple scales and the interactions between these scales are critically important for food security in a changing climate and beyond. Research that explores social capital on only one scale may miss the ways in which households are embedded within communities where organizations work and coordinate in different ways, which as we have shown, may shift different types of social capital. Programs and policies aimed to build social capital should consider these multiple scales and their interactions for most effective outcomes. However, our understanding of these complex interactions is nascent and requires a much larger effort for data collection using networks across households and institutions. As such, we suggest that it is imperative to not only improve data collection and analysis using network methods, but to ensure that such methods work across communities and scales. These new data collection and research efforts have great potential to help achieve important community and household goals far beyond food security, including many other Sustainable Development Goals.
Data Availability Statement
The original contributions presented in the study are included in the article/Supplementary Material, further inquiries can be directed to the corresponding author. CCAFS data are available through Harvard Dataverse: https://dataverse.harvard.edu/dataverse/CCAFSbaseline.
Ethics Statement
The studies involving human participants were reviewed and approved by CGIAR CCAFS. The patients/participants provided their written informed consent to participate in this study.
Author Contributions
MN conceived the research, analyzed data, wrote and revised the manuscript, and developed visualizations. JR conceived the research, analyzed data, and revised the manuscript. ML and LC conceived the research and revised the manuscript. All authors contributed to the article and approved the submitted version.
Funding
We thank CCAFS, the University of Vermont, and the University of California Davis for their support funding the personnel on this project.
Conflict of Interest
The authors declare that the research was conducted in the absence of any commercial or financial relationships that could be construed as a potential conflict of interest.
Acknowledgments
The baseline data presented in this paper was collected by the CGIAR CCAFS program, which is carried out with support from the CGIAR Trust Fund and through bilateral funding agreements (for details please visit https://ccafs.cgiar.org/donors). The views expressed in this document cannot be taken to reflect the official opinions of these organizations. Additionally, the authors thank the staff at CCAFS for their support on the OBS data to be accessible for our network analysis. We thank Thomas Wentworth for his assistance in graphic design of the figures. We thank CCAFS, the University of Vermont, and the University of California Davis for their support funding the personnel on this project.
Supplementary Material
The Supplementary Material for this article can be found online at: https://www.frontiersin.org/articles/10.3389/fsufs.2021.583353/full#supplementary-material
References
Abdul-Rahaman, A., and Abdulai, A. (2018). Do farmer groups impact on farm yield and efficiency of smallholder farmers? Evidence from rice farmers in northern Ghana. Food Policy 81, 95–105. doi: 10.1016/j.foodpol.2018.10.007
Adger, W. N. (2003). Social capital, collective action, and adaptation to climate change. Econ. Geogr. 79, 387–404. doi: 10.1111/j.1944-8287.2003.tb00220.x
Ahmadi, D., Sinclair, K., Melgar-Quinonez, H., Helal, G., Ebadi, N., and Monteith, H. (2017). Personal health index, food security status and social capital items: a study on women with young children (under 5) living in households with different levels of food security. FASEB J. 31, 791.4–791.4. doi: 10.1096/fasebj.31.1_supplement.791.4
Aldrich, D. P., and Meyer, M. A. (2014). Social capital and community resilience. Am. Behav. Sci. 59, 254–269. doi: 10.1177/0002764214550299
Aldrich, D. P., and Sawada, Y. (2015). The physical and social determinants of mortality in the 3.11 tsunami. Soc. Sci. Med. 124, 66–75. doi: 10.1016/j.socscimed.2014.11.025
Allen, K. M. (2006). Community-based disaster preparedness and climate adaptation: local capacity-building in the Philippines. Disasters 30, 81–101. doi: 10.1111/j.1467-9523.2006.00308.x
Bandiera, O., and Rasul, I. (2006). Social networks and technology adoption in Northern Mozambique. Econ. J. 116, 869–902. Available online at: http://www.jstor.org/stable/4121936 (accessed December 15, 2020).
Bodin, Ö., Alexander, S. M., Baggio, J., Barnes, M. L., Berardo, R., Cumming, G. S., et al. (2019). Improving network approaches to the study of complex social–ecological interdependencies. Nat. Sustain. 2, 551–559. doi: 10.1038/s41893-019-0308-0
Bryan, E., Deressa, T. T., Gbetibouo, G. A., and Ringler, C. (2009). Adaptation to climate change in Ethiopia and South Africa: options and constraints. Environ. Sci. Policy 12, 413–426. Available online at: http://www.sciencedirect.com/science/article/B6VP6-4V995Y6-2/2/acc29834a1d072508bae3636d20dcc6d (accessed December 15, 2020).
Cadger, K., Quaicoo, K. A., Dawoe, E., and Isaac, E. M. (2016). Development interventions and agriculture adaptation: a social network analysis of farmer knowledge transfer in Ghana. Agric. 6:6030032. doi: 10.3390/agriculture6030032
Chaudhury, A. S., Thornton, T. F., Helfgott, A., Ventresca, M. J., and Sova, C. (2017). Ties that bind: local networks, communities and adaptive capacity in rural Ghana. J. Rural Stud. 53, 214–228. doi: 10.1016/j.jrurstud.2017.05.010
Chiappori, P.-A., Oreffice, S., and Quintana-Domeque, C. (2012). Fatter attraction: anthropometric and socioeconomic matching on the marriage market. J. Polit. Econ. 120, 659–695. doi: 10.1086/667941
Chriest, A., and Niles, M. (2018). The role of community social capital for food security following an extreme weather event. J. Rural Stud. 64, 80–90. doi: 10.1016/j.jrurstud.2018.09.019
Clarke, K. A. (2005). The phantom menace: omitted variable bias in econometric research. Confl. Manag. Peace Sci. 22, 341–352. doi: 10.1080/07388940500339183
Climate Change Agriculture and Food Security (CCAFS) (2012). Organisational Baseline Study 2012. Wageningen: CCAFS. doi: 10.7910/DVN/Q7PKLN
Davidson, D. (2016). Gaps in agricultural climate adaptation research. Nat. Clim. Chang. 6, 433–435. doi: 10.1038/nclimate3007
Dean, W. R., and Sharkey, J. R. (2011). Food insecurity, social capital and perceived personal disparity in a predominantly rural region of Texas: an individual-level analysis. Soc. Sci. Med. 72, 1454–1462. doi: 10.1016/j.socscimed.2011.03.015
Dietz, T., Ostrom, E., and Stern, P. C. (2003). The struggle to govern the commons. Science 302, 1907–1912. doi: 10.1126/science.1091015
Douxchamps, S., Van Wijk, M. T., Silvestri, S., Moussa, A. S., Quiros, C., Ndour, N. Y. B., et al. (2016). Linking agricultural adaptation strategies, food security and vulnerability: evidence from West Africa. Reg. Environ. Chang. 16, 1305–1317. doi: 10.1007/s10113-015-0838-6
FAO (2018). The State of Food Security and Nutrition in the World. Rome. Available online at: http://www.fao.org/state-of-food-security-nutrition/en/ (accessed December 15, 2020).
Filmer, D., and Pritchett, L. H. (2001). Estimating wealth effects without expenditure data- or tears: an application to educational enrollments in states of India. Demography 38, 115–132. doi: 10.1353/dem.2001.0003
Förch, W., Sijmons, K., Mutie, I., Kiplimo, J., Cramer, L., Kristjanson, P., et al. (2013). CCAFS Site Portfolio. East Africa, West Africa and South Asia, Copenhagen: Core Sites in the CCAFS Regions, CGIAR Research Program on Climate Change, Agriculture and Food Security (CCAFS).
Fuller, T. L., Sesink Clee, P. R., Njabo, K. Y., Tróchez, A., Morgan, K., Meñe, D. B., et al. (2018). Climate warming causes declines in crop yields and lowers school attendance rates in Central Africa. Sci. Total Environ. 610–611, 503–510. doi: 10.1016/j.scitotenv.2017.08.041
Garson, G. D. (2013). Hierarchical Linear Modeling: Guide And Applications. Thousand Oaks, CA: Sage.
Gisselquist, R. (2012). Good Governance as a concept, and why this matters for development policy. WIDER Work. Paper No. 2012/30.
Gyau, A., Mbugua, M., and Oduol, J. (2016). Determinants of participation and intensity of participation in collective action: evidence from smallholder avocado farmers in Kenya. J. Chain Netw. Sci. 16, 147–156. doi: 10.3920/JCNS2015.0011
Hadley, C., Mulder, M. B., and Fitzherbert, E. (2007). Seasonal food insecurity and perceived social support in rural Tanzania. Public Health Nutr. 10, 544–551. doi: 10.1017/S1368980007246725
Hancock, M., Hunter, D., Butts, C., Goodreau, S., and Morris, M. (2003). Statnet: Software Tools for the Statistical Modeling of Network Data. J. Stat. Softw. 24:29824. doi: 10.18637/jss.v024.i01
Hoffman, A. L., Kemanian, A. R., and Forest, C. E. (2017). Analysis of climate signals in the crop yield record of sub-Saharan Africa. Glob. Chang. Biol. 24, 143–157. doi: 10.1111/gcb.13901
Hoogesteger, J. (2016). NGOs and the democratization of ecuadorian water governance: insights from the multi-stakeholder platform el Foro de los Recursos Hídricos. Voluntas 27, 166–186. doi: 10.1007/s11266-015-9559-1
Howe, L. D., Galobardes, B., Matijasevich, A., Gordon, D., Johnston, D., Onwujekwe, O., et al. (2012). Measuring socio-economic position for epidemiological studies in low- and middle-income countries: a methods of measurement in epidemiology paper. Int. J. Epidemiol. 41, 871–886. doi: 10.1093/ije/dys037
Ingold, K., Balsiger, J., and Hirschi, C. (2010). Climate change in mountain regions: how local communities adapt to extreme events. Local Environ. 15, 651–661. doi: 10.1080/13549839.2010.498811
Johanson, J., and Mattsson, L.-G. (1987). Interorganizational relations in industrial systems: a network approach compared with the transaction-cost approach. Int. Stud. Manag. Organ. 17, 34–48. doi: 10.1080/00208825.1987.11656444
Karlsson, M., and Hovelsrud, G. K. (2015). Local collective action: adaptation to coastal erosion in the Monkey River Village, Belize. Glob. Environ. Chang. 32, 96–107. doi: 10.1016/j.gloenvcha.2015.03.002
Kettle, N. P., and Dow, K. (2014). The role of perceived risk, uncertainty, and trust on coastal climate change adaptation planning. Environ. Behav. 48, 579–606. doi: 10.1177/0013916514551049
Kristjanson, P., Neufeldt, H., Gassner, A., Mango, J., Kyazze, F., Desta, S., et al. (2012). Are food insecure smallholder households making changes in their farming practices? Evidence from East Africa. Food Secur. 4, 381–397. doi: 10.1007/s12571-012-0194-z
Lipper, L., Thornton, P., Campbell, B. M., Baedeker, T., Braimoh, A., Bwalya, M., et al. (2014). Climate-smart agriculture for food security. Nat. Clim. Chang. 4, 1068–1072. doi: 10.1038/nclimate2437
Lowder, S. K., Skoet, J., and Raney, T. (2016). The number, size, and distribution of farms, smallholder farms, and family farms worldwide. World Dev. 87, 16–29. doi: 10.1016/j.worlddev.2015.10.041
Lubell, M., Niles, M., and Hoffman, M. (2014). Extension 3.0: managing agricultural knowledge systems in the network age. Soc. Nat. Resour. 27, 1089–1103. doi: 10.1080/08941920.2014.933496
Martin, K. S., Rogers, B. L., Cook, J. T., and Joseph, H. M. (2004). Social capital is associated with decreased risk of hunger. Soc. Sci. Med. 58, 2645–2654. doi: 10.1016/j.socscimed.2003.09.026
Misselhorn, A. (2009). Is a focus on social capital useful in considering food security interventions? Insights from KwaZulu-Natal. Dev. South. Afr. 26, 189–208. doi: 10.1080/03768350902899454
Mitlin, D., Hickey, S., and Bebbington, A. (2007). Reclaiming development? NGOs and the challenge of alternatives. World Dev. 35, 1699–1720. doi: 10.1016/j.worlddev.2006.11.005
Moore, F. C., and Lobell, D. B. (2015). The fingerprint of climate trends on European crop yields. Proc. Natl. Acad. Sci. Available online at: http://www.pnas.org/content/early/2015/02/11/1409606112.abstract (accessed December 15, 2020).
Morton, J. F. (2007). The impact of climate change on smallholder and subsistence agriculture. Proc. Natl. Acad. Sci. U.S.A. 104, 19680–196805. doi: 10.1073/pnas.0701855104
Neil Adger, W., Arnell, N. W., and Tompkins, E. L. (2005). Successful adaptation to climate change across scales. Glob. Environ. Chang. 15, 77–86. doi: 10.1016/j.gloenvcha.2004.12.005
Niles, M. T., and Brown, M. E. (2017). A multi-country assessment of factors related to smallholder food security in varying rainfall conditions. Sci. Rep. 7:16277. doi: 10.1038/s41598-017-16282-9
Niles, M. T., and Salerno, J. D. (2018). A cross-country analysis of climate shocks and smallholder food insecurity. PLoS ONE 13:192928. doi: 10.1371/journal.pone.0192928
Ostrom, E., and Ahn, T.-K. (2003). Foundations of Social Capital. Northhampton, MA: Edward Elgar Publishing.
Ostrom, E., and Ahn, T. K. (2009). “The meaning of social capital and its link to collective action,” in Handbook of Social Capital: The Troika of Sociology, Political Science and Economics, eds G. T. Svendsen and G. L. Haase Svendsen (Northhampton, MA: Edward Elgar), 240–241.
Panda, S. (2015). Political connections and elite capture in a poverty alleviation programme in India. J. Dev. Stud. 51, 50–65. doi: 10.1080/00220388.2014.947281
Paul, C. J., Weinthal, E. S., Bellemare, M. F., and Jeuland, M. A. (2016). Social capital, trust, and adaptation to climate change: evidence from rural Ethiopia. Glob. Environ. Chang. 36, 124–138. doi: 10.1016/j.gloenvcha.2015.12.003
Petzold, J., and Ratter, B. M. W. (2015). Climate change adaptation under a social capital approach – An analytical framework for small islands. Ocean Coast. Manag. 112, 36–43. doi: 10.1016/j.ocecoaman.2015.05.003
Portes, A. (1998). Social capital: its origins and applications in modern sociology. Annu. Rev. Sociol. 24, 1–24. doi: 10.1146/annurev.soc.24.1.1
Provan, K. G., and Kenis, P. (2008). Modes of network governance: structure, management, and effectiveness. J. Public Adm. Res. Theory 18, 229–252. doi: 10.1093/jopart/mum015
Putnam, R. (2000). Bowling Alone: The Collapse and Revival of American Community. New York, NY: Simon and Schuster. doi: 10.1145/358916.361990
Quetulio-Navarra, M., Frunt, E., and Niehof, A. (2017). “The role of social capital and institutions in food security and wellbeing of children under five for resettled households in Central Java, Indonesia,” in Diversity and Change in Food Wellbeing (Wageningen: Wageningen Academic Publishers), 115–136.
Ramankutty, N., and Iizumi, T. (2016). Changes in yield variability of major crops for 1981–2010 explained by climate change. Environ. Res. Lett. 11:34003. doi: 10.1088/1748-9326/11/3/034003
Ramirez, M., Bernal, P., Clarke, I., and Hernandez, I. (2018). The role of social networks in the inclusion of small-scale producers in agri- food developing clusters? Food Policy 77, 59–70. doi: 10.1016/j.foodpol.2018.04.005
Ritzema, R. S., Frelat, R., Douxchamps, S., Silvestri, S., Rufino, M. C., Herrero, M., et al. (2017). Is production intensification likely to make farm households food-adequate? A simple food availability analysis across smallholder farming systems from East and West Africa. Food Secur. 9, 115–131. doi: 10.1007/s12571-016-0638-y
Rockström, J., Barron, J., Fox, P., and others (2003). Water productivity in rain-fed agriculture: challenges and opportunities for smallholder farmers in drought-prone tropical agroecosystems. Water Product. Agric. Limits Oppor. Improv. 85199:8. doi: 10.1079/9780851996691.0145
Rodima-Taylor, D. (2012). Social innovation and climate adaptation: local collective action in diversifying Tanzania. Appl. Geogr. 33, 128–134. doi: 10.1016/j.apgeog.2011.10.005
Rudnick, J., Niles, M., Lubell, M., and Cramer, L. (2019). A comparative analysis of governance and leadership in agricultural development policy networks. World Dev. 117, 112–126. doi: 10.1016/j.worlddev.2018.12.015
Sahn, D. E., and Stifel, D. (2003). Exploring Alternative Measures of Welfare in the Absence of Expenditure Data. Rev. Income Wealth 49, 463–489. doi: 10.1111/j.0034-6586.2003.00100.x
Saint Ville, A. S., Hickey, G. M., Locher, U., and Phillip, L. E. (2016). Exploring the role of social capital in influencing knowledge flows and innovation in smallholder farming communities in the Caribbean. Food Secur. 8, 535–549. doi: 10.1007/s12571-016-0581-y
Scheffran, J., Marmer, E., and Sow, P. (2012). Migration as a contribution to resilience and innovation in climate adaptation: Social networks and co-development in Northwest Africa. Appl. Geogr. 33, 119–127. doi: 10.1016/j.apgeog.2011.10.002
Silvestri, S., Sabine, D., Patti, K., Wiebke, F., Maren, R., Ianetta, M., et al. (2015). Households and food security: lessons from food secure households in East Africa. Agric. Food Secur. 4:23. doi: 10.1186/s40066-015-0042-4
Sinyolo, S., and Mudhara, M. (2018). Farmer groups and inorganic fertiliser use among smallholders in rural South Africa. South Afr. J. Sci. 114, 1–9. doi: 10.17159/sajs.2018/20170083
Smith, J. W., Anderson, D. H., and Moore, R. L. (2012). Social capital, place meanings, and perceived resilience to climate change*. Rural Sociol. 77, 380–407. doi: 10.1111/j.1549-0831.2012.00082.x
Thornton, P. K., Kristjanson, P., Förch, W., Barahona, C., Cramer, L., and Pradhan, S. (2018). Is agricultural adaptation to global change in lower-income countries on track to meet the future food production challenge? Glob. Environ. Chang. 52, 37–48. doi: 10.1016/j.gloenvcha.2018.06.003
Wachs, J., Yasseri, T., Lengyel, B., and Kertész, J. (2019). Social capital predicts corruption risk in towns. R. Soc. Open Sci. 6:182103. doi: 10.1098/rsos.182103
Walker, J. L., Holben, D. H., Kropf, M. L., Holcomb, J. P., and Anderson, H. (2007). Household food insecurity is inversely associated with social capital and health in females from special supplemental nutrition program for women, infants, and children households in Appalachian Ohio. J. Am. Diet. Assoc. 107, 1989–1993. doi: 10.1016/j.jada.2007.08.004
Wheeler, T., and von Braun, J. (2013). Climate change impacts on global food security. Science 341, 508 LP−513. Available online at: http://science.sciencemag.org/content/341/6145/508.abstract (accessed December 15, 2020).
Wolf, J., Adger, W. N., Lorenzoni, I., Abrahamson, V., and Raine, R. (2010). Social capital, individual responses to heat waves and climate change adaptation: an empirical study of two UK cities. Glob. Environ. Chang. 20, 44–52. doi: 10.1016/j.gloenvcha.2009.09.004
Wood, S. A., Jina, A. S., Jain, M., Kristjanson, P., and DeFries, R. S. (2014). Smallholder farmer cropping decisions related to climate variability across multiple regions. Glob. Environ. Chang. 25, 163–172. doi: 10.1016/j.gloenvcha.2013.12.011
Keywords: climate change, agriculture, network analysis, social networks, food security, organization
Citation: Niles MT, Rudnick J, Lubell M and Cramer L (2021) Household and Community Social Capital Links to Smallholder Food Security. Front. Sustain. Food Syst. 5:583353. doi: 10.3389/fsufs.2021.583353
Received: 14 July 2020; Accepted: 02 February 2021;
Published: 03 March 2021.
Edited by:
Peter Newton, University of Colorado Boulder, United StatesReviewed by:
Celia A. Harvey, Monteverde Institute, Costa RicaKurt Waldman, Indiana University, United States
Copyright © 2021 Niles, Rudnick, Lubell and Cramer. This is an open-access article distributed under the terms of the Creative Commons Attribution License (CC BY). The use, distribution or reproduction in other forums is permitted, provided the original author(s) and the copyright owner(s) are credited and that the original publication in this journal is cited, in accordance with accepted academic practice. No use, distribution or reproduction is permitted which does not comply with these terms.
*Correspondence: Meredith T. Niles, mtniles@uvm.edu