- 1Centre for Crop Sciences, Queensland Alliance for Agriculture and Food Innovation (QAAFI), The University of Queensland, Gatton, QLD, Australia
- 2International Maize and Wheat Improvement Centre (CIMMYT), Addis Ababa, Ethiopia
- 3Department of Plant, Soil and Microbial Science, Michigan State University, East Lansing, MI, United States
An adequate food supply is widely recognized as a necessary condition for social development as well as a basic human right. Food deficits are especially common among semi-subsistence farming households in eastern and southern Africa and farm productivity is widely regarded as the locus for enhancing household food outcomes. However, knowledge gaps surrounding benefits associated with climate smart, productivity-enhancing technologies require attention. This study evaluates benefits associated with sustainable intensification farm management practices (crop residue retention, minimum tillage, manure application and use of herbicides, pesticides, fertilizer, and improved seeds) for household calorie and protein supplies and demonstrates their scope across households with high-, moderate- and low- likelihoods of calorie and protein deficits. Household-level calorie and protein deficits were estimated from survey data on food production, acquisition and consumption for households in Ethiopia and Mozambique. Multinomial logistic models were used to identify drivers of household food deficit status and logistic model trees established “rules of thumb” to classify households by food deficit status as low, moderate or high likelihood. In Ethiopia, especially wet seasons were associated with a high likelihood of a food deficit while especially dry seasons were associated with a high likelihood of food deficit in Mozambique. The practices associated with sustainable intensification and related technologies substantially enhanced food outcomes in groups with a high- and a low-likelihood of food deficit, and associated benefits were high for the best-off households. Benefits associated with sustainable intensification technologies were not observed for households with a moderate likelihood of a food deficit and some technologies even increased risk. The sustainable intensification practices assessed here were associated with improved food outcomes yet benefits were limited in scope for households of intermediate status. Thus, there is a need to expand the technical options available to reduce food deficit.
Introduction
Food shortages in Ethiopia and Mozambique remain widespread and erratic despite long term positive food availability trends from 1990–92 to 2014–16 (FAOSTAT, 2020). Although food security indicators are rarely reported in Ethiopia, optimistic government-led projections of food availability estimated that 5.6 million individuals (i.e. 13% of the population) were food insecure following an above-average rainy season from July-September of 2016 (FSIN, 2017). A subsequent drought during the Meher season of 2017 started a prolonged dry spell with crop losses of 50–90% leaving an estimated 9.7 million people in need of urgent food assistance (FAO et al., 2019). Recent food crises in Mozambique resulted from a resurgence of political disputes and armed conflict in central and southern Mozambique (WFP, 2018), a severe drought during the 2015/2016 El Niño years and the occurrence of extreme weather events including cyclone Idai, known as “the worst climate-related disaster to hit Mozambique this millennium” (WFP, 2019). Access and reliability of food supplies were especially precarious for semi-subsistence households, where food availability was especially sensitive to crop failure and high-food prices (FSIN, 2017). The number of undernourished people rose rapidly with resultant food shortages and elevated food prices from 2.7 million in 2010–12 to an estimated 8.8 million in 2015–17 (FAO et al., 2019). The number of moderately or severely food insecure people increased from a three year average of 19.4 million in 2015–2017 to 20.4 million in 2016–2018 (FAOSTAT, 2020). This establishes an urgent need to identify mechanisms that enhance household food-related outcomes in these regions.
Increased household production is widely considered the main mechanism for overcoming food shortages among semi-subsistence household farmers, where an estimated 60% of the household food supply is produced by the household and own production provides the major income source for purchasing food (Frelat et al., 2015; Marenya et al., 2018). Here, sustainable intensification is understood to encompass the wide range of practices including, where appropriate but not limited to conservation agriculture, with potential to produce more food from the same area of land in a variable and changing environment, while maintaining or improving the resource base. This definition, fully consistent with definitions of Pretty (2009), requires potential for increased production in a region, rather than absolute certainty which is justified given the highly temporal and spatial variability of growing conditions that a region can encompass. Sustainable intensification (SI) practices, including fertilizer and herbicide application, crop residue retention, reduced tillage and improved seeds, have potential in many regions to enhance production outcomes. They have been associated with increased yield and reduced risk of crop failure among semi-subsistence households in Ethiopia (Abebe et al., 2014) and Mozambique (Nyagumbo et al., 2016, 2017). In addition to direct production benefits, SI has resulted in income gains and poverty reduction (Teklewold et al., 2013; Zeng et al., 2017). Although the substantial contribution of on-farm production to the household food basket is clear, literature relating SI to household food-related outcomes and their scope across diverse production systems is less established (Webb et al., 2006; Qaim, 2014; Webb and Kennedy, 2014).
Various studies have demonstrated food- and nutrition- related benefits associated with a single SI technology (Jones et al., 2014). Cross-sectional data in eastern Zambia showed that adoption of improved maize varieties significantly reduced child malnutrition as proxied by stunting (Manda et al., 2016). Observational panel data from farm households in India showed that adoption of Bt cotton technology significantly increased calorie and micro-nutrient (zinc, iron, vitamin A) consumption per adult equivalent (Qaim and Kouser, 2013). The few studies that consider impacts of multiple technologies suggest that benefits of agricultural technologies are greater when they are applied jointly, rather than individually. In Ethiopia, joint application of maize-legume cropping diversification and improved maize varieties had a greater impact on child stunting, per capita consumption of calories, protein and iron; and dietary diversity compared to benefits from crop diversification or use of improved maize varieties when used alone (Marenya et al., 2018).
The benefits associated with SI technologies have been inconsistent across semi-subsistence production systems of eastern and southern Africa (Giller et al., 2009). Within Ethiopia, substantial variation in benefits have been reported, where soil and water conservation practices have enhanced productivity (Zikhali, 2008; Adgo et al., 2013; Yenealem et al., 2013; Tesfaye et al., 2016), reduced technical efficiency (Oduol et al., 2011) and yielded very low returns (Kassie and Holden, 2006; Kassie et al., 2009). Benefits have displayed a common phenomenon of relatively low returns under high-risk, resource constrained conditions (Stephens et al., 2012). This distribution of benefits have produced low-level stagnation (Tittonell and Giller, 2013). The combined low-return and high-risk conditions act as a self-reinforcing mechanism or poverty trap, where current poverty is itself a direct cause of poverty in the future (Azariadis and Stachurski, 2005). These relationships have generated chronic poverty and food shortages across a large share of the world's population (Carter and Barrett, 2006; Barrett, 2010; Stephens et al., 2012).
There is a growing body of evidence showing a low-return to high-risk relationship when SI technologies are applied across diverse semi-subsistence farmers in ESA. Households that produced on highly degraded (low soil organic matter) soils in western Kenya experienced lower fertilizer use efficiency and returns to fertilizer use than households that produced on rich soils, resulting in persistent yields declines and poverty and food insecurity (Marenya and Barrett, 2009a,b). Farmers in Zimbabwe provide evidence of constraints imposed on productivity by poverty, where some groups of farmers had a productivity advantage over other groups not only because they used more fertilizer per hectare, but because they attained a higher rate of return from its use (Zikhali, 2008). In Kenya, female-headed households experienced lower returns from increasing land area under cultivation compared to their male counterparts putting them at higher-risk of a food deficit than their male-headed household counterparts (Kassie et al., 2014).
A critical step in understanding the consequences of SI for household food supplies, is to understand who, among semi-subsistence household farmers, is positioned to take advantage of those technologies (Adato et al., 2006; Barrett et al., 2006). This study spans a demographically diverse set of households and agroecological regions to assess the scope of benefits associated with SI technologies. We hypothesize that (i) SI technologies are associated with reduced household food deficit, (ii) and the magnitude of the benefits depends on the baseline likelihood of a food deficit. We characterize differences in the demographic composition and structural characteristics of households with a high-, moderate- and low-likelihood of food deficit in Ethiopia and Mozambique. We then present evidence that these differences have consequences for the benefits (reduced food deficit) of SI technologies.
Materials and Methods
Household Selection
The analysis used three waves (2010, 2013 and 2016) of household survey data collected across all the maize-growing agro-ecological zones of Ethiopia (n = 1940) and Mozambique (n = 1145) under the Sustainable Intensification of Maize-Legume Cropping Systems for Food Security in Eastern and Southern Africa (SIMLESA) Program. The SIMLESA Program was a regional agricultural Research for Development (R4D) program anchored on the collaboration and support from the national agricultural research institutes (NARIs) and many partners, institutions, and farmers. Since 2010, all households included in the study were exposed to SI technology through demonstration plots and through these outreach activities, encouraged to adopt SI practices namely, crop residue retention, minimum tillage, manure application and use of herbicides, pesticides, fertilizer, and improved seeds. All households in the sampled population were included in under the SIMLESA Program and thereby had equal exposure to technologies and information.
Purposive and stratified sampling methods were used to select semi-subsistence households, for which household production played a major role in supporting household food supply and where households had equal exposure to the SIMLESA Program initiatives. Household selection was stratified across agroecologies with distinct maize production potentials. In Ethiopia, households were selected from the Southern (SNNP) region and western parts of Oromiya, where maize–legume-based farming systems were common. In the first stage of household selection, nine districts were purposely selected (five from Oromiya, three from SNNP and one from Benishangul-Gumuz): Bako Tibe, Gubuesyo, Shalla, Dudga, Adami Tullu, Mesrak Badawacho, Meskan, Hawassa Zuriya and Pawe. Seven of these districts (Shalla, Dudga, Adami Tullu, Mesrak Badawacho, Meskan and Hawassa Zuriya) represent the low-potential agroecological zone, where rainfall is generally low and erratic; while the remaining districts represent the high-potential zone with adequate rainfall. In the second stage, households were randomly selected from 69 farmer associations with populations proportional to the size of the association. These regions spanned a wide range of household food supply conditions. Food insecurity indices for these regions have been reported at levels as low as 10 to 0 food insecure people per km2 and as high at 1000 to 100 food insecure people per km2 (Potgieter et al., 2013).
Households in Mozambique span four districts (Sussundenga, Manica, Gorongosa and Angonia) and 154 villages. Two of the districts (Sussundenga and Manica) are situated in the province of Manica, while Gorongosa and Angonia are found in the provinces of Sofala and Tete, respectively. A multistage random sampling procedure was used to select households from each district. Proportionate household sampling, using census data for the selected villages, identified survey households. All households in Ethiopia and Mozambique provided oral consent to participate in the study prior to their involvement and households were de-identified to ensure confidentiality, using a password secured file that linked household identifiers to a separate file containing contact information.
Households were georeferenced with the aim of sampling identical households over time. However, the surveys were not treated as a panel dataset because the survey data did not satisfy a key assumption of fixed and random effect regression models for analysis of panel data (i.e. unique attributes of individuals which, may or may not be correlated with individual dependent variables, are constant across time). Multiple household characteristics changed from one survey period to the next so that too few household characteristics were consistent enough to be considered the same house. So household surveys are statistically independent across the three years of sampling. In contrast to a longitudinal study, the year was removed from the analysis, while major covariates including climate data were included. Attrition due to changes in household composition, out-migration and deaths likely explained significant changes in households over time in this region as well as high levels of both adoption and dis-adoption (Marenya et al., 2018).
Climate Data
The study spans the severe drought of the 2015/2016 El Niño years. Rainfall data from a gridded (0.5 ×0.5 degree) CRU TS4.01 dataset spanning 1901–2016, (Harris and Jones, 2017), were assigned to each surveyed household based on household GPS coordinates. Two sets of rainfall indicators were derived to capture two timescales that cover the production, harvest and post-harvest periods captured in the household surveys: the entire calendar year and a country-specific growing season. In Mozambique, annual rainfall data covers the 12 months of rainfall from January to December (thus including maize establishment), while seasonal rainfall in Mozambique includes rainfall from October to May. The surveys were conducted between June and October in Mozambique. In Ethiopia, annual rainfall was calculated for the year prior to the survey year and seasonal rainfall is the cumulative rainfall between March and November. The surveys in Ethiopia were conducted between October and December.
Absolute and relative indicators of water stress were derived and included in the analysis to identify predictors of household food supply. In absolute terms, rainfall is expressed as; total rainfall (mm) within the annual or seasonal period and the range (mm) and median (mm) of historical (1901:2016) averages, where range is the difference between the smallest and largest observations. An anomaly ratio, calculated as the difference between the specified timeframe and the long-term average divided by the averaged difference between the specified months and the long-term average provides a relative measure of water-related stress. A large positive anomaly ratio reflects especially wet conditions for the region at the time (e.g. flooding) while a large negative anomaly ratio reflects especially dry conditions for the region at the time (e.g. drought). The rainfall percentile was also reported in the descriptive analysis. The percentile is determined by ranking all historic observations in order, from driest to wettest. These observations are divided into 100 equal groups where the 0th and 100th percentiles are the lowest and highest on record.
Household Surveys
The same structured questionnaire was used in all three years and the surveyed respondents were interviewed using trained and experienced enumerators with knowledge of the local language. The surveys were carried out by the International Wheat and Maize Improvement Center (CIMMYT) in collaboration with the Ethiopian Institute of Agricultural Research (EIAR) in Ethiopia and the Instituto de Investigação Agrária de Moçambique (IIAM) in Mozambique. Each survey was conducted with the member of the household who was nominated by household members to represent the household. This was typically the household head or the spouse. Enumerators were instructed to ensure that any household representative nominated was thoroughly familiar with the production, consumption and other socio-economic activities of the household. While it is possible that no single person was responsible for all food sources, all the nominated household members were heavily involved in production, purchase and consumption decisions. Household representatives were asked to recall household demographics, productive assets (land, labor and livestock), management practices used, crop production levels, and quantities of food acquired, stored and consumed over the most recent production year (Wilkus et al., 2019). This survey was designed and implemented in a way to minimize recall bias, or the discrepancy between natural systems data and a participants' account of their experiences. The survey period did not exceed an hour and participants were asked to recall the most recent harvest season, which had occurred within months of administering the survey. The head of household was asked if the following crop management practices were used on any subplot in the previous season, reporting yes or no to indicate if: 1. Crop residues were left on the sub-plot the previous season, 2. Minimum tillage was practiced on any subplot, 3. Herbicide was applied, 4. Pesticide was applied 5. Fertilizer was applied, 6. Manure was applied and, 7. Improved seed was purchased and sown.
The survey captured household food items from three sources: household livestock production, off-farm purchases and household crop production (Figure 1). Household livestock production, off-farm purchases and household crop production were reported separately for each livestock or meat product and for each crop and produce item. Crop production questions were crop specific and included crop aggregate production per crop (kg) during separate seasons while also accounting for stocks going into each season. In Ethiopia this included the following estimates, stock before the Meher season harvest (kg), Belg season harvest (kg) which amounted to the total available stock after the Belg season harvest (kg). The head of household estimated the quantity consumed (kg) from the total available stock after the season harvest. The survey captured the following to determine the ending stock [stock before the next season's harvest (kg)] from total available stock after the Belg season harvest (kg): the quantity sold (kg), quantity used for seed (kg), gift, tithe, donations given (kg) and post-harvest losses. In addition to estimates from household production, households estimated the amount bought (kg) and food aid/borrowed/gifts received (kg). Specific food products were reported in kilograms and converted into energy (kilocalories) and protein (grams) using product-specific conversion factors (Daba and Shigeta, 2016; US Department of Agriculture et al., 2018), (Supplementary Table 1).
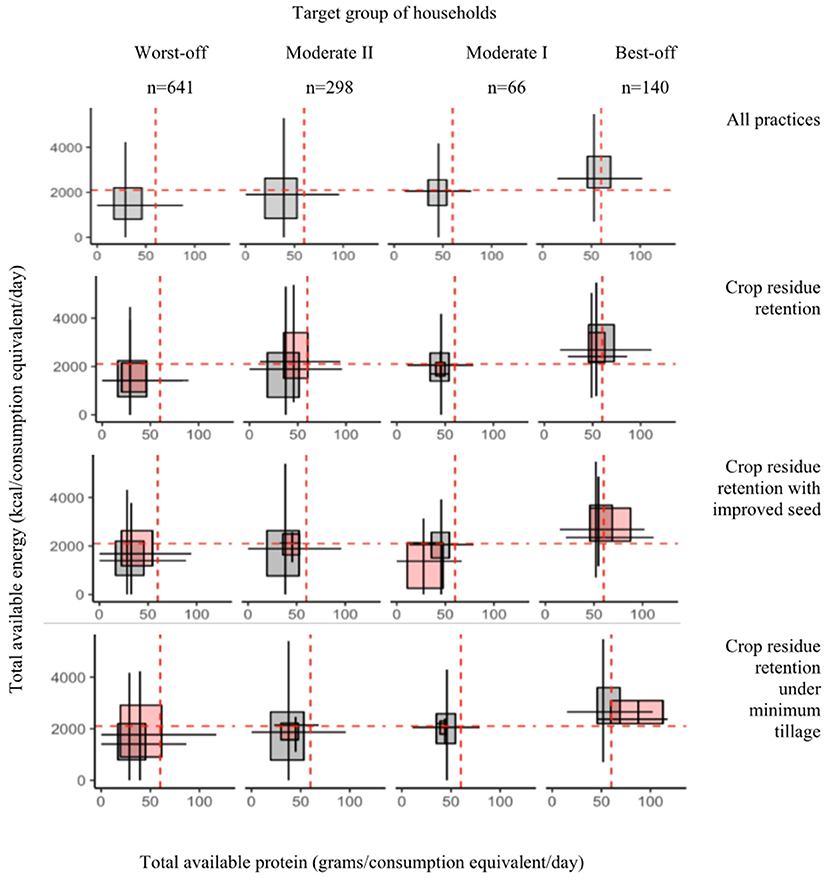
Figure 1. Model for the estimation of food sufficiency showing three sources of food; Household livestock production, food purchase from off-farm activities and on-farm crop production.
Food Sufficiency Estimation
The minimum household food requirement was estimated based on 2100 kcal and 60 g protein per day per consumption equivalent (CE), adjusted using conventional age- and gender-based requirement assumptions (FAO et al., 2015; FAOSTAT, 2020). This adjustment was used in the absence of more accurate data on the health and lifestyle of household members. The minimum food requirement was subtracted from the available food supply to determine the food surplus or deficit, where sufficiency is defined as having consumed and stored at least the minimum energy and protein requirement (Figure 1). Household food status outcomes were; 1. Sufficient energy and protein; 2. Sufficient energy and insufficient protein, 3. Insufficient energy and sufficient protein; and 4. Insufficient energy and protein.
Analytical Approach
The analysis combined standard econometric endogenous switching regressions (Di Falco et al., 2011) and predictive public health modeling to identify and compare sub-populations of surveyed households. Consistent with the first step of the endogenous switching regression approach, the analysis began with the estimate of the multinomial logit model to account for any selection bias on household characteristics that impact both the probability of using a SI practice and the probability of a certain household food status outcome. The second component of standard econometric approaches accounts for any selection bias on household characteristics that impact household participation in an agricultural promotion activity and is relevant from impact assessments where a subset of the population is exposed to an intervention. This study assessed a population with equal exposure to SI promotion efforts and required methods outside of the standard econometric approaches for structuring and comparing surveyed households.
The second step of the analysis established comparison groups. The factors identified in the multinomial logit model were used to develop logistic model trees that divided households into homogenous groups within Ethiopia and Mozambique. This allowed for unbiased within group comparisons of food deficit with and without a management practice or set of practices. The method accounts for important interactions in the data and has been used to explain variation of a single response variable, using combinations of exploratory variables displaying non-linear relationships and high-order interaction (Lemon et al., 2003). The approach has been applied extensively on survey data in ecology, socio-economic analyses and agricultural sciences. The method represents the most widely accepted approach for predicting the probability of an adverse outcome in the medical literature and is widely used to establishes clinical subgroups of subjects at very high or very low risk of health-related outcomes (Demir, 2014). Previous work in the same region and on similar survey data applied classification and regression tree analyses (Tittonell et al., 2006). Given the complexity of the data set, Tittonell et al. (2007) used classification and regression trees (CART) to relate biophysical variables (including food production) with management factors. This approach was similarly used here.
Food Sufficiency Models
Food sufficiency models were developed using independent variables that accounted for the largest proportion of the observed variability in the data (Osborne and Costello, 2009). A Principal Component Analysis (PCA) was initially conducted for each country separately to reduce the dimensionality of the analysis and minimize collinearity between predictors of food sufficiency. Variables with the highest loading weights within each principal component having an eigenvalue greater than 1 were retained to model (Supplementary Tables 2, 3).
Multinomial logit models (function “multinom”, R Core Team, 2017) were developed for each country separately to control from differences in institutional settings that could influence the likelihood of a food deficit. Household food status outcomes were modeled as a linear combination of predictor variables. The method did not assume normality, linearity or homoscedasticity, which would be required with alternative approaches like discriminant function or canonical analyses. The food sufficiency models were further simplified by iteratively removing variables through backward stepwise AIC analysis. Energy and protein sufficiency is treated as the reference category and is expressed as Equation 1.
The probability of experiencing one household food status outcome was compared to the likelihood of energy and protein sufficiency, so an increase in the probability reflects an increased likelihood of a food deficit. This required the calculation of three equations (Equation 2), one for each category relative to the reference category (energy and protein sufficiency). Three predicted log odds – or logit coefficients (β), were generated with one for each food sufficiency outcome relative to the reference category.
Classification of Households Into Best-, Moderate and Worst-Off Groups
The variables that predicted household food status were used to classify households into groups with a low-, moderate and high-likelihood of a food deficit. The group with a low likelihood of a food deficit was considered “best-off” while the group with a high likelihood of a food deficit was considered “worst-off”. This step of classifying farms is necessary for further analysis of distinct subpopulations, rather than a pooled dataset. Through this method of disaggregating the dataset, the analysis controls for any bias distribution of household characteristics that impact household food status. Threshold levels of the food sufficiency predictors were calculated from the food sufficiency models using recursive partitioning trees (Package rpart in R software, Therneau and Atkinson, 1997). The rpart function identified the variable which discriminated most between household groups, partitioning the data on this variable, and then identified splitting criteria which maximized the separation in the data between distinguishable sub-groups. This process was repeated until all groups were distinct. The minimal number of terminal nodes or household groups, was selected to ensure ease of interpretation while maintaining a sufficient number of households (at least three) to represent SI technologies for subsequent analysis.
Benefits of Sustainable Intensification Practices
Similar to the first iteration of food sufficiency models, multinomial logit models were used to predict food sufficiency outcomes from the on-farm application of SI technologies (function “multinom”, R Core Team, 2017). These models were developed separately for groups with a low-, moderate- and high-likelihood of a food deficit. Within group comparisons thereby accounted for any selection bias on household characteristics that impacted both the probability of adopting sustainable intensification practices and household food status outcomes.
Results
Climatic Conditions
Total seasonal rainfall levels, relative to the long-term seasonal average, varied across the surveyed households in Ethiopia. In the 2010 main growing season, the majority (75%) of households experienced dryer than normal conditions (below 50% of historical rainfall levels). However, by 2013, the majority (75%) experienced wetter conditions and in 2016, most households (83%) experienced normal conditions (Figure 2). Overall, 42% of households reflect normal conditions, 26% reflect dry and the remaining 31% represent wetter than normal conditions. The majority of the dry observations fell within the 20–40th percentile range and the wet observations fell in the 60–80th percentile range. The wettest conditions observed in Ethiopia represent the 90th percentile of historic rainfall levels and the driest conditions represent the 4th percentile.
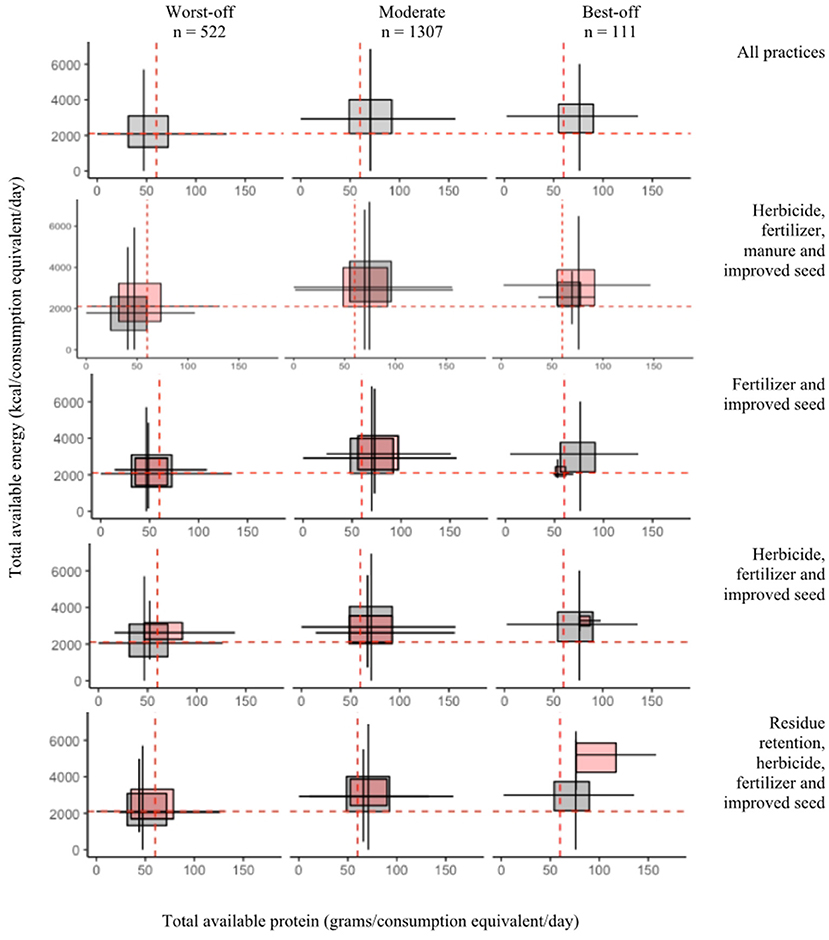
Figure 2. Spatial distribution of the seasonal rainfall anomaly ratio across the sampled households in Ethiopia and Mozambique. The anomaly ratio is calculated as the difference between the survey period and the long-term average, divided by the average difference between the specified months and the long-term average. Seasonal anomaly ratios are shown for each main growing season prior to the survey and demonstrate the range of conditions experienced over the survey years. The histograms plot the rainfall percentile received over the survey period.
In the 2010 main growing season in Mozambique, 51% of surveyed households experienced normal conditions and 42% experienced a relatively dry season. In 2013, 95% of households experienced normal conditions and in 2015, 96% had a dry season, reflecting the severe drought of the 2015/2016 El Niño years. The majority of the dry observations fell within the 5–10th percentile range while the few households that experienced a wet year fell within the 80th percentile. The wettest conditions observed in Mozambique represent the 88th percentile of historical rainfall levels and the driest conditions represent the 8th percentile.
Household Food Status Outcomes
Just over half (52%) of surveyed households in Ethiopia had sufficient energy and protein, while 30% of the surveyed households were deficient in energy and protein, 16% were deficient in protein only and 1% was deficient in energy only. In Mozambique, 19% of the surveyed households had sufficient energy and protein, and 59% were deficient in energy and protein, while the remaining 22% were deficient in protein only.
Predictors of Food Deficit
The variables retained from the PCA analysis and evaluated as potential predictors of food deficit in Ethiopia were; age of the head of household, land area under cultivation in the ‘short rain' Belg season, tropical livestock units (TLUs), distance from the household to the nearest market, median annual rainfall and the seasonal rainfall anomaly ratio (Supplementary Table 2). Out of this set of variables, the likelihood of a household food deficit in Ethiopia increased the seasonal rainfall anomaly ratio (wetter than average years) and the age of the household head and decreased with rainfall level (seasonal rainfall median) and the number of livestock owned (Table 1).
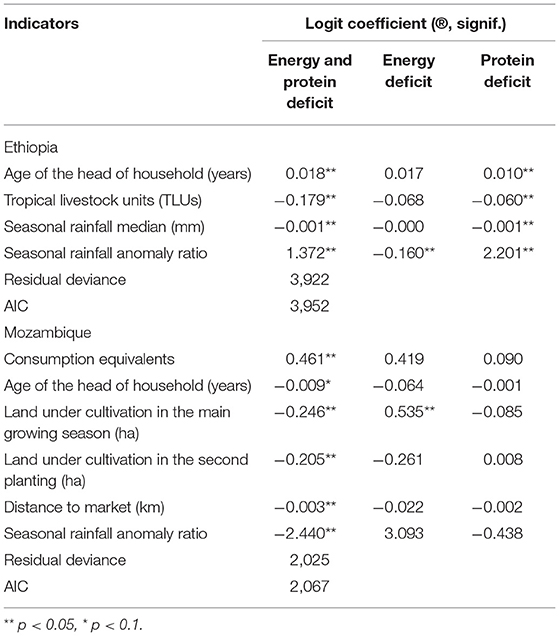
Table 1. Change in the log odds of a food deficit with household- and environment-level factors in Ethiopia and Mozambique.
The variables retained from the PCA analysis and evaluated as predictors of food deficit in Mozambique were; household consumption equivalents (CE, i.e. family size adjusted for age and gender), age of the head of household, education of the head of household, land area under cultivation in the main growing season, land area under cultivation in the second planting, distance from the household to the nearest market, total seasonal rainfall and the seasonal rainfall anomaly ratio (Supplementary Table 3). The likelihood of a food deficit decreased with the seasonal rainfall anomaly ratio (wetter than average years) and the age of the head of household in Mozambique (Table 1). The likelihood of a food deficit also decreased with land area under cultivation in the main growing season, land area under cultivation in the second planting and with distance to the market.
Best-, Moderate and Worst-Off Groups
The logistic model tree identified “rules of thumb” for establishing groups with distinct likelihoods of food deficit. The number of tropical livestock units (TLUs) provided a first simple rule of thumb to classify households in Ethiopia (Figure 3). 60% of the households with at least three TLUs had sufficient energy and protein, whereas only 38% of the households with less than three TLUs had sufficient energy and protein. A rainfall anomaly ratio value of less than −0.1 (where the lowest reported value was −0.37) provided the next rule of thumb to predict food availability status among the households with less than three TLUs. 64% of the households with an anomaly ratio of less than −0.1 had sufficient energy and protein while only 32% of the households with an anomaly ratio of greater than −0.1 had sufficient energy and protein. This group, had the lowest likelihood of a food deficit and constituted only 6% of the surveyed population or 111 households (Table 2). The moderate group in Ethiopia had more TLUs (TLU ≥ 3) than the best- and worst-off groups and constituted 66% of the surveyed households (n = 1307).
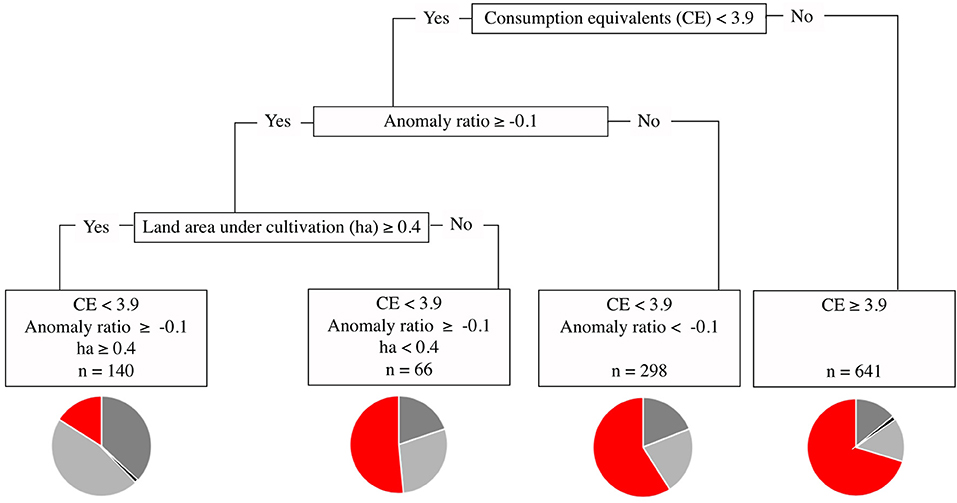
Figure 3. Logistic model tree for households in Ethiopia household. Three groups of households are represented as terminal nodes. Tropical livestock units and the anomaly ratio of the last seasons' rainfall predict food availability status.
Households in Mozambique were classified into groups with distinct likelihoods of a food deficit based on household consumption equivalents (CE), the anomaly ratio of the last seasons' rainfall and the area of land under cultivation over the last major growing season (Figure 4). A consumption equivalent (CE) threshold of 3.9 provided the first simple rule of thumb to predict the risk of an energy or protein deficit (Figure 4). 14% of the households with 3.9 CE or greater had sufficient energy and protein, whereas 25% of the households with less than 3.9 CE had sufficient energy and protein (Table 3). A rainfall anomaly ratio value of −0.1 provided the second rule of thumb to predict food availability status among the households with less than 3.9 CE. 20% of the households with an anomaly ratio of less than −0.1 had sufficient energy and protein while 31% of the households with an anomaly ratio of at least −0.1 had sufficient energy and protein. A land area under cultivation of 0.4 ha provided the final criterion for predicting food deficits in households with less than 3.9 CE and an anomaly ratio of at least −0.1. 38% of households with greater than or equal to 0.4 ha had sufficient energy and protein while only 20% of households on less than 0.4ha had sufficient energy and protein. Households with at least 3.9 CE were most likely to have an energy and protein deficit (Table 3). Two moderate household groups were identified, both of which had smaller households (CE <3), than the worst-off group. One moderate group (Moderate I) had a smaller household size (<3.9) than the high-risk group and experienced less variable rainfall (Anomaly ratio < −0.1) than the best-off group and the other moderate-risk group. The other moderate-risk group (Moderate II) was distinguished from the best-off group by cultivated land area, where the moderate group had less land under cultivation (ha <0.4) than the best-off group.
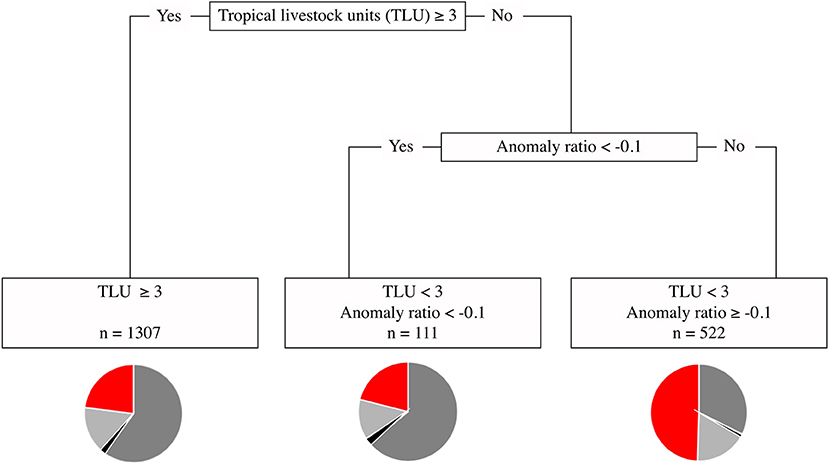
Figure 4. Logistic model tree for households in Mozambique. The tree produces four groups of households, represented as terminal nodes. Household consumption equivalents (CE), the anomaly ratio of the last reasons' rainfall and the area of land under cultivation over the last major growing season predict food availability status.
Food Sufficiency With Sustainable Intensification Technologies
The most common practice observed in Ethiopia was the joint use of herbicide, fertilizer, manure and improved seed (Table 4). The joint use of fertilizer, manure and improved seed (without herbicide) was the second most common practice. In Ethiopia, the joint use of herbicide, fertilizer and improved seed were associated with a slight reduction in energy and protein deficit in the worst-off group (Figure 5, Table 5). Energy deficit levels were significantly lower in the worst-off group with the addition of manure to this set of practices. In the best-off group (i.e. TLU <3, Anomaly ratio < −0.1), the combined use of residue retention, herbicide, fertilizer and improved seed was the only set of SI practices associated with a reduced energy and protein deficit. However, the use of these practices by the best-off group, was associated with the most substantial reduction in food deficit levels of all the practices, across all groups. Among the largest, moderate-risk group (TLU ≥ 3), the SI technologies either had no observable benefit or increased risk. The use of herbicide, fertilizer, manure and improved seed even increased the risk of an energy deficit in this group.
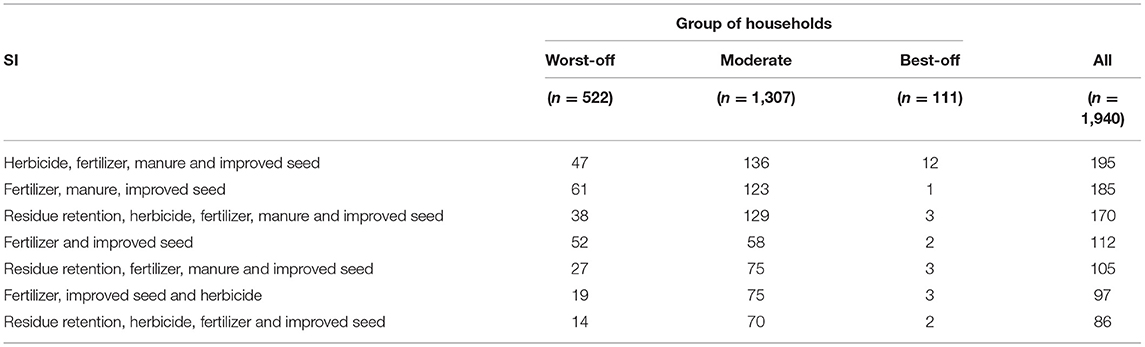
Table 4. The number of households using management practices across target groups in Ethiopia. Sustainable intensification (SI) technologies in grey are excluded from further analysis.
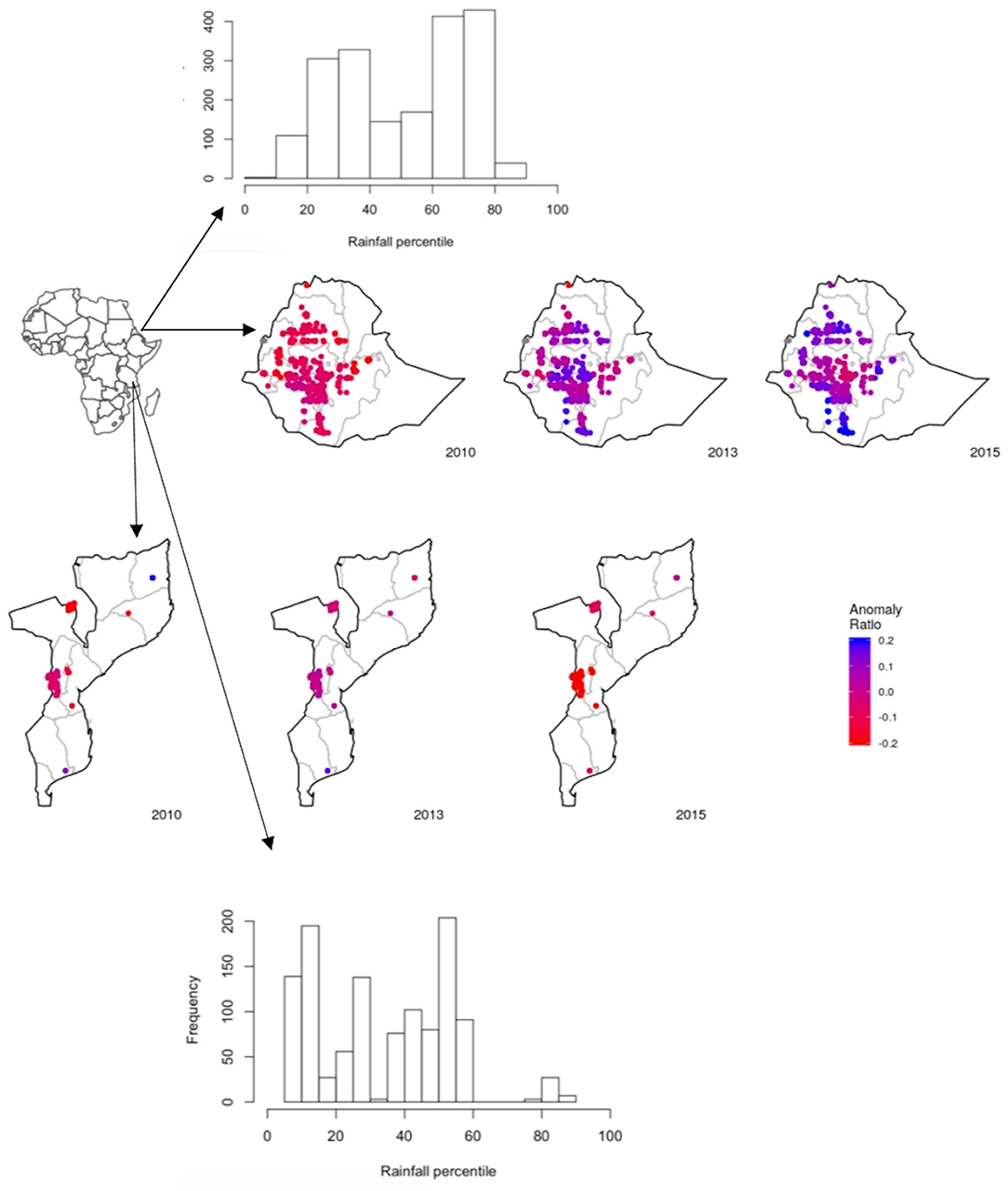
Figure 5. Box plots of food sufficiency with (red) and without (grey) conservation agriculture (CA) practices across target groups in Ethiopia. The food requirement threshold for energetic needs (2100 kcal/CE/day) is indicated with a horizontal line. The food requirement threshold for protein needs (60 g/CE/day) is indicated with the vertical line.
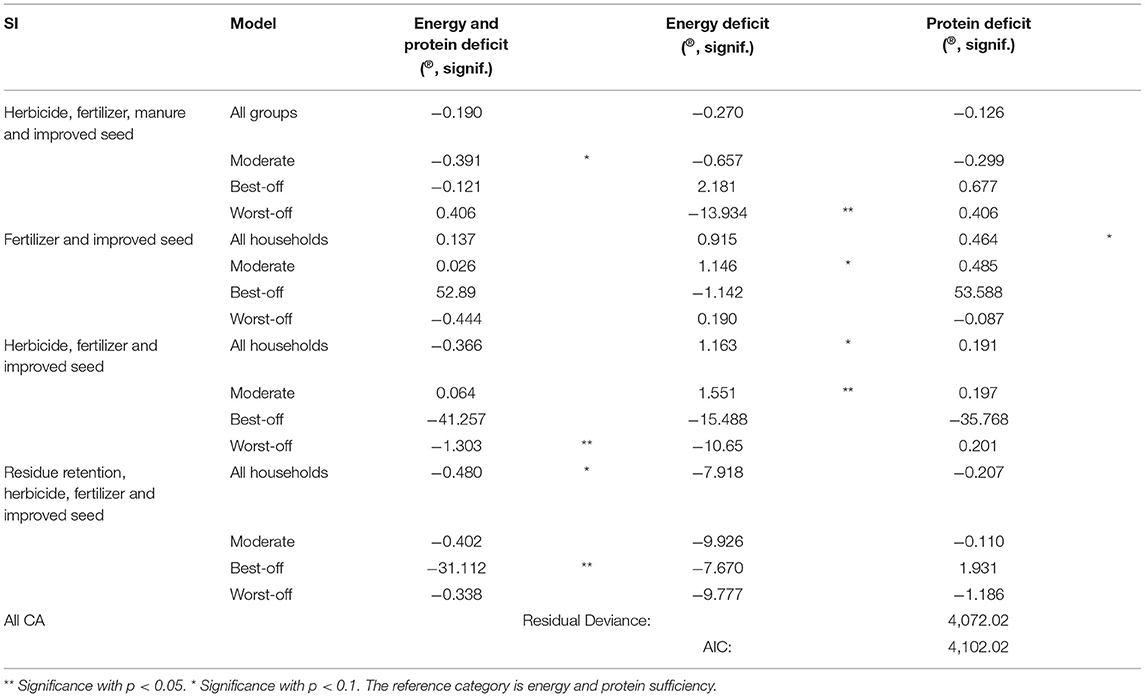
Table 5. Multinomial logistic regression table showing sustainable intensification (SI) management practices associated with food availability in Ethiopia.
In Mozambique, the most common practice was crop residue retention only and the second most common practice was minimum tillage only (Table 6). Residue retention with minimum tillage reduced the energy and protein deficit levels in the best- and worst-off groups (Figure 6, Table 7). Residue retention with improved seeds reduced the energy and protein deficit in the worst-off group only. The benefits of crop reside retention with minimum tillage were substantially greater for the best-off group than those found in the worst-off group. Food deficit levels did not differ significantly between SI users and non-users in the moderate groups.
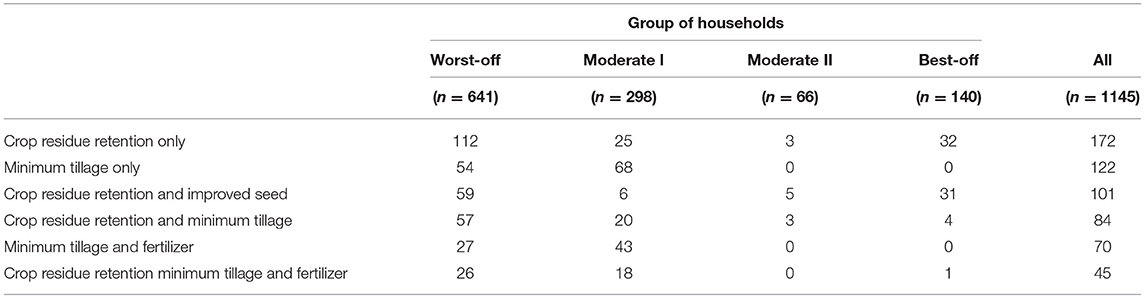
Table 6. The number of households using management practices across target groups in Mozambique. sustainable intensification (SI) technologies in grey are excluded from further analysis.
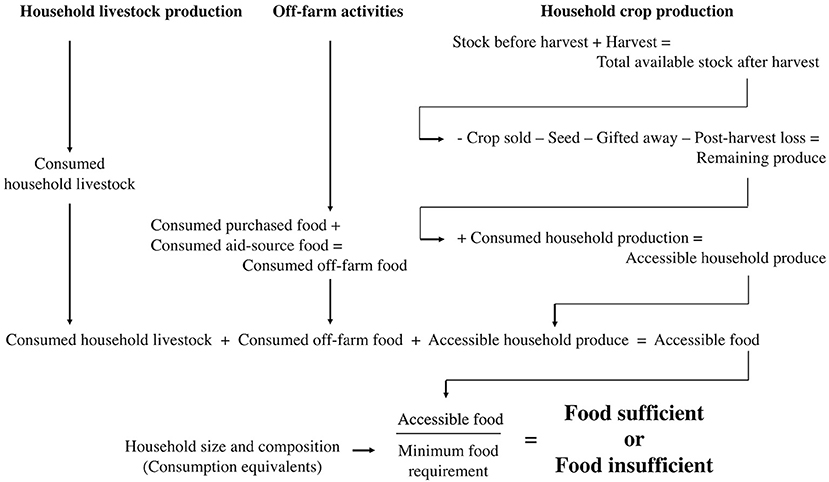
Figure 6. Box plots of food sufficiency outcomes with (red) and without (grey) conservation agriculture (CA) practices across target groups in Mozambique. The food requirement threshold for energetic needs (2100 kcal/CE/day) is indicated with a horizontal line. The food requirement threshold for protein needs (60 g/CE/day) is indicated with the vertical line.
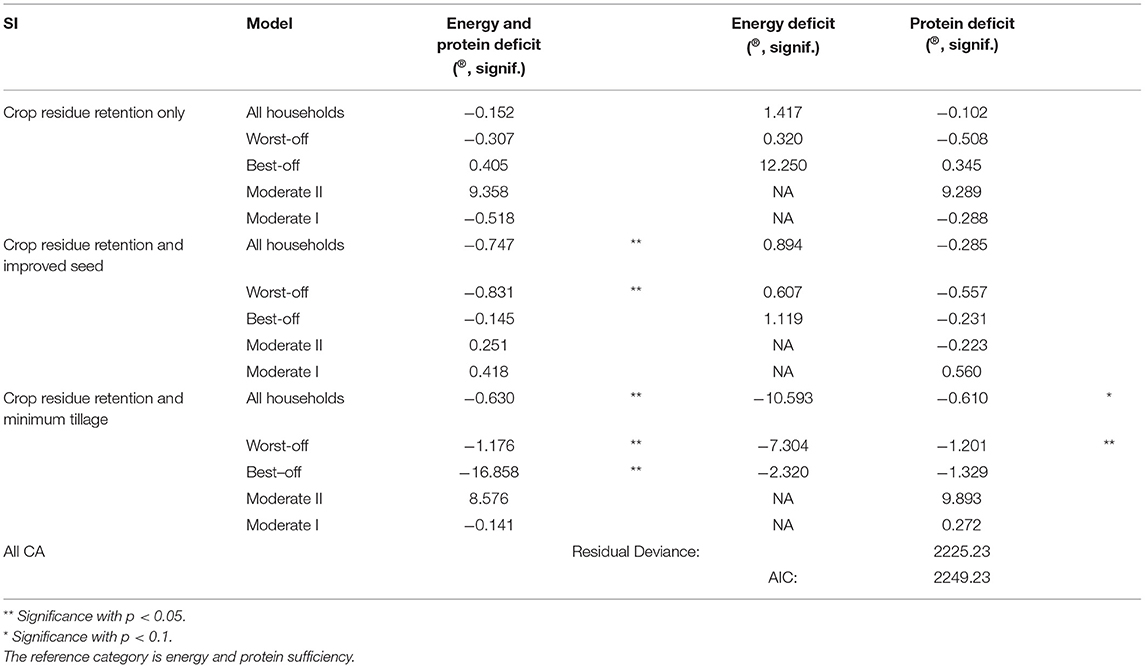
Table 7. Multinomial logistic regression table showing sustainable intensification(SI) management practices associated with food availability in Mozambique.
Discussion
This study demonstrated variation in the likelihood of food deficit and benefits from sustainable intensification technologies across diverse populations of semi-subsistence households in Ethiopia and Mozambique. Previous work has demonstrated the need to go beyond calculations of total food available in a population to understand the distribution of food. For instance Marenya et al. (2018) found that 50% of the farm households in Ethiopia consumed fewer calories than the recommended daily intake of 2,100 kcal even when the average calorie consumption (2,200 kcal) was sufficient to support the population (Marenya et al., 2018). This study found that substantial portions of the populations experienced energy and protein deficits, reaching 30 and 59% of the households, in Ethiopia and Mozambique respectively. These figures are consistent with previous work showing that an estimated 40% of the population in Ethiopia consumed less than the recommended daily calories and average protein consumption was 14 grams less that the recommended daily intake of 56 grams per person per day (von Grebmer et al., 2015). These figures are critical for ongoing efforts to understand and overcome food shortages and are especially valuable for Mozambique where data on food security indicators is scarce.
Predictors of Food Deficit
The study identifies climate- and household-level factors to predict food deficits and demonstrates that these factors influence benefits associated with from sustainable intensification technologies. Predictors of household food status (i.e. insufficient energy and protein, insufficient energy only and insufficient protein only) were generally consistent with previous studies. Consistent with broader trends observed across 17 countries in sub-Saharan Africa (Frelat et al., 2015), household consumption equivalents was associated with an increased likelihood of a food deficit. Also consistent with Frelat et al. (2015), tropical livestock units and land area under cultivation was associated with a decreased likelihood of a food deficit. While increases in family size can be beneficial, providing various forms of support to the family through on- and off-farm activities, household members may increase household food requirements, necessitating innovations for ensuring food sufficiency for the entire household (for examples refer to Devereux, 2003).
The anomaly ratio, an indication of water stress impacted household food outcomes in different ways in Ethiopia and Mozambique. In Ethiopia, wetter seasons increased the likelihood of a food deficit while drier seasons increased the likelihood of a food deficit in Mozambique. The finding that the rainfall anomaly ratio (an indication of how especially wet or dry the season was for that location) influenced the risk of a household food deficit is expected as rainfall is one of the most highly cited climatic factors influencing food availability in these rainfed production systems (Afifi et al., 2013). The anomaly ratio is especially relevant for understanding production risks and is supported by previous studies and theory surrounding climate uncertainty, which considers challenges identifying appropriate management practices under uncertain growing conditions and predicts a decline in food security with erratic climate behaviour, through declines in on-farm production (McCarthy et al., 2001; Parry et al., 2004, 2005; Lewis, 2017). Increased rainfall under generally wet and humid conditions may have been associated with an increased risk of mould and other crop damage in the field or in storage in Ethiopia. Dry seasons in Mozambique included the severe drought of the 2015/2016 El Niño years when 96% of the surveyed households experienced drier than normal conditions. These dry conditions may have been especially detrimental to household food status given the extent of the community effected. In contrast to crop failure events that are isolated to one household, broad scale drought events effect the entire community and limit opportunities for individual households to appeal to neighbours for support (Wilkus, 2016). A limitation of this study is that it does not account for the role of social networks in maintaining household food supplies.
An unexpected finding from Mozambique was that the households located closer to markets were less likely to meet their food needs than households further from markets (Table 1). Reduced transaction costs associated with proximity to markets along with greater access to accurate market information suggest that household food status would improve with greater access to markets (Gabre-Madhin, 1999; Frelat et al., 2015). Participation in livestock and grain markets have also been treated as a means of securing access to food through different forms of market exchange (Beyene, 2015; Beyene and Kassie, 2015). It is possible that poverty may have the propensity to deny households access to market-sourced food (Mbajiorgu, 2019) or farmers may require additional skills to operate in commercial markets (Hendriks, 2014). Households located close to markets may typically allocate resources to off-farm enterprises that provide inconsistent income. Households located close to markets may therefore, be especially vulnerable to unpredictable and fluctuating markets. Further analysis can evaluate market imperfections in Mozambique that may undermine market-related benefits and compare the stability of on- and off-farm income sources. Off-farm income may be stable relative to the seasonal agricultural income, which was subject to vagaries of weather, as this study shows.
Unequal Benefits From Sustainable Intensification Across Best-, Moderate- and Worst-Off Households
Adoption of SI practices in the surveyed populations was low to moderate. Multiple practices used in concert were most common in Ethiopia where the joint use of herbicide, fertilizer, manure and improved seed (10% of the population) and joint use of fertilizer, manure and improved seed (10% of the population) were most frequently observed. The occurrence of sustainable intensification practices in Ethiopia are consistent with recent adoption studies showing 8 and 29% of the a surveyed population in Ethiopia that jointly adopting sustainable intensification practices in 2010 and 2013, respectively (Marenya et al., 2018). This study identified few household farms that used a single management practice in Ethiopia, while Marenya et al. (2018) found evidence for high levels of adoption of improved seeds only, reaching 46 and 36% of the population in 2010 and 2013, respectively. In contrast to the adoption patterns observed in Ethiopia, the most common practices in Mozambique involved the use of a single SI technology where 15% of the population in Mozambique practiced crop residue retention alone and 11% of the population practiced minimum tillage alone. Joint adoption of multiple practices may be rare in Mozambique because basic agronomic conditions have limited potential benefits of SI practices. Improved agronomic practice represented the largest return on investment for farmers in this region (van Ittersum et al., 2013). For instance, improved planting density explained half of maize yield gains observed over six seasons from 2010–2016 in Mozambique, overshadowing any benefits from SI practices (Nyagumbo et al., 2018).
The benefits associated with of SI technologies and the most beneficial technologies varied across best-, moderate- and worst-off groups. Overall, the benefits associated with SI technologies were greatest for the best-off groups in both Ethiopia and Mozambique. For the worst-off group in Ethiopia, a reduced energy and protein deficit was only observed with joint use of herbicide, fertilizer and improved seed and the reduction was minor. The energy deficit was lowest among the worst-off households that added manure to herbicide, fertilizer and improved seed. These findings may reflect a process by which the worst-off households incrementally improving operations to enhance food outcomes. By primarily incorporating herbicide, fertilizer and improved seed into household management practice might explain the reduced energy and protein deficit and the subsequen addition of manure might explain the reduced energy deficit. Although the associated benefits were minor, this gradual adoption process offers a promising strategy for the worst-off households, with limited investment options to escape poverty traps (Sime and Aune, 2016). Future research research can better determine causality and investigate specific pathways by which these practices enhance household food outcomes would greatly assist in formulating recommendations for strategic and gradual adoption processes. The only management practice associated with a reduced energy and protein deficit for the best-off group in Ethiopia was the joint use of residue retention, herbicide, fertilizer and improved seed. In contrast to the benefits associated with the most promising practice for the worst-off group, the benefits from the most promising practice were substantial for the best-off group.
The study shows that predictors of food deficit can be valuable criteria for identifying groups that respond differently to SI practices. Uneven benefits associated with SI technologies in Ethiopia may reflect different processes and constraints operating in the best- and worst-off groups. The main distinction between the best- and worst-off groups in Ethiopia was in the rainfall patterns observed between them where the worst-off group experienced especially wet conditions and the best-off group experienced especially dry conditions. Evidence from previous studies showing that manure decomposition rates were positively correlated with cumulative precipitation (Zhu et al., 2020), would suggest that benefits associated with manure would be greater for the worst-off households, however this was not the case. While both groups had relatively few livestock and limited access to manure as a consequence, worst-off households may have applied less manure overall compared to the best-off group based on differences in their market participation. Market developments have made maize stover a valuable feed resource, creating an additional competing use for residues that may preclude use as mulch (Tittonell et al., 2007). Worst-off households, experiencing acute food shortage may opt to allocate a portion of manure towards market sales and purchase food with that income. The application rates of 0.5–2t ha-1 of manure is considered necessary to sustain crop yields, yet this level may be unrealistic for the worst-off group. The alternative, application levels of 0–0.5 t ha-1, forego benefits of the practice (Wezel and Rath, 2002). This study does not account for differences in the quantities of manure applied, so it is possible that the best-off group applied larger quantities overall compared to the worst-off group.
Two sets of SI options were associated with benefits for the worst off household in Mozambique: joint use of crop residue retention and improved seed and joint use of crop residue retention with minimum tillage. A slight reduction in energy and protein deficits was associated with these practises for the worst-off group. The only observed benefits associated with SI practices for the best-off group of households were found with the use of crop residue retention with minimum tillage. The main difference between the worst-off and best-off group was family size, where the worst-off group had larger families. While it is possible that production benefits associated with crop residue retention with minimum tillage were equal for the best- and worst-off groups, those associated benefits are diffused in the worst-off households, where household consumption requirements are larger.
Benefits associated with SI technologies were not observed for moderate households in Ethiopia or Mozambique. The moderate households in Ethiopia had more tropical livestock units (TLU ≥3) and thus greater demand for crop residue as livestock feed than the best- and worst-off groups and constituted 66% of the surveyed households (n = 1307). Tropical livestock units may also operate to stabilize access to food. The SI technologies that were evaluated were either associated with an increased likelihood of a food deficit or had no observable benefit for the largest group. In addition to the smaller household size (CE <3.9), the best-off group was distinguished from one of the moderate groups (II) in Ethiopia by cultivated land area, where the moderate group had less land under cultivation (ha <0.4) than the best-off group. This moderate risk group (II) may benefit from the same SI technologies (residue retention with minimum tillage) as the best-off group however, benefits may not be observable or consequential under the smaller scale of production when those benefits are slight, however impactful to the household. Two moderate groups were identified in Mozambique, both of which had smaller households (CE <3), than the worst-off group. Although smaller households have lower consumption requirements, they may also have limited labour capacity, resulting in less intensive management practice. The second, moderate group (I) may have relied less on the use of the SI technologies under less variable climate conditions, which allowed household members to better anticipate food sources and availability.
Conclusions
Sustainable intensification was associated with enhanced food outcomes but also limited in scope. The number of households that experienced benefits associated with SI technologies were large in Mozambique, where the worst-off group represented 56% of the sampled population, compared to only 27% of households in Ethiopia. There was no evidence of risk reduction associated with CA technologies in moderate households, which represented the majority of households in Ethiopia. In both Ethiopia and Mozambique, associated benefits from “best-bet” SI practices were lower for the group of worsts-off households than the group of best-off households. Alternative approaches were necessary to better support households with a high- and moderate- likelihood of a food deficit and ensure equal opportunities for enhancing household food-related outcomes. These findings support a substantial body of evidence on self-reinforcing mechanisms underlying poverty-traps (Barrett, 2010; Naschold, 2012; Stephens et al., 2012; Tittonell and Giller, 2013) and advance our understanding of the role that sustainable intensification plays in supporting household food-related outcomes across diverse populations.
Data Availability Statement
The original contributions presented in the study are included in the article/Supplementary Material, further inquiries can be directed to the corresponding authors.
Ethics Statement
Ethical review and approval was not required for the study on human participants in accordance with the local legislation and institutional requirements. Written informed consent from the participants' legal guardian/next of kin was not required to participate in this study in accordance with the national legislation and the institutional requirements.
Author Contributions
SS, JD, and PM provided critical feedback and helped shape the research, analysis and manuscript. EW conceived of the presented idea, developed the theory, performed the analysis, and wrote the manuscript. PdeV prepared the data and images. DR supervised the project. All authors contributed to the article and approved the submitted version.
Funding
The research was conducted under the Sustainable Intensification of Maize-Legume Cropping Systems for Food Security in Eastern and Southern Africa (SIMLESA) I (Project code: CSE/2009/024) and II (Project code: CSE/2013/008) Program with funding from The Australian Centre for International Agricultural Research (ACIAR).
Conflict of Interest
The authors declare that the research was conducted in the absence of any commercial or financial relationships that could be construed as a potential conflict of interest.
Publisher's Note
All claims expressed in this article are solely those of the authors and do not necessarily represent those of their affiliated organizations, or those of the publisher, the editors and the reviewers. Any product that may be evaluated in this article, or claim that may be made by its manufacturer, is not guaranteed or endorsed by the publisher.
Acknowledgments
Household surveys were conducted with the collaborative cooperation of country partners and the International Maize and Wheat Improvement Center (CIMMYT). Country partners were the Ethiopian Agricultural Research Institute (EARI) and the Instituto de Investigação Agrária de Moçambique (IIAM). Socioeconomics who supervised the collection of household surveys include Adam Bekele in Ethiopia and Isabelle Cachomba and Maria da Luz in Mozambique. The work would not be possible without the enumerators who administered the surveys.
Supplementary Material
The Supplementary Material for this article can be found online at: https://www.frontiersin.org/articles/10.3389/fsufs.2021.649218/full#supplementary-material
References
Abebe, Z., Birhanu, T., Tadesse, S., and Degefa, K. (2014). Conervation agriculture: maize-legume intensification for yield, profitability and soil fertility improvement in maize belt areas of western Ethiopia. Int. J. Plant Soil Sci. 3, 969–985. doi: 10.9734/IJPSS/2014/8797
Adato, M., Carter, M. R., and May, J. (2006). Exploring poverty traps and social exclusion in South Africa using qualitative and quantitative data. J. Develop. Stud. 42, 226–247. doi: 10.1080/00220380500405345
Adgo, E., Teshhome, A., and Mati, B. (2013). Impact of long-term soil and water conservation on agriculture al productivity: the case of Anjenie watershed, Ethiopia. Agric. Water Manage. 117, 55–61. doi: 10.1016/j.agwat.2012.10.026
Afifi, T., Liwenga, E., and Kwezi, L. (2013). Rainfall-induced crop failure, food insecurity and out-migration in Same-Kilimanjaro, Tanzania. Clim. Develop. 6, 53–60. doi: 10.1080/17565529.2013.826128
Azariadis, C., and Stachurski, J. (2005). Chapter 5 Poverty Traps. In: Aghion, P., and Durlauf, S. (Eds.), Handbook of Economic Growth, Elsevier B.V, North Holland. pp. 295–384. doi: 10.1016/S1574-0684(05)01005-1
Barrett, C. B.. (2010). Food systems and the escape from poverty and ill-health traps in Sub-Saharan Africa. In: Pinstrup-Andersen, P., (Ed.), The African food system and its interactions with human health and nutrition, Cornell University Press, Ithaca.
Barrett, C. B., Carter, M. R., and Little, P. D. (2006). Understanding and reducing persistent poverty in Africa: introduction to a special issue. J. Develop. Stud. 42, 167–177. doi: 10.1080/00220380500404587
Beyene, A. D., and Kassie, M. (2015). Speed of adoption of improved maize varieties in Tanzania: an application of duration analysis. Technol. Forec. Soc. Change. 96, 298–307. doi: 10.1016/j.techfore.2015.04.007
Beyene, F.. (2015). Determinants of food security under changing land-use systems among pastoral and agro-pastoral households in eastern Ethiopia. Environ. Develop. Sustain. 17, 1163–1182. doi: 10.1007/s10668-014-9596-8
Carter, M. R., and Barrett, C. B. (2006). The economics of poverty traps and persistent poverty: an asset-based approach. J. Develop. Stud. 42, 178–199. doi: 10.1080/00220380500405261
Daba, T., and Shigeta, M. (2016). Enset (Enset Ventricosum) Production in Ethiopia: Its nutritional and socio-cultural values. Agric. Food Sci. Res. 3, 66–74. doi: 10.20448/journal.512/2016.3.2/512.2.66.74
Demir, E.. (2014). A decision support tool for predicting patients at risk of readmission: A comparison of classification trees, logistic regression, generalized additive models, and multivariate adaptive regression splines. Decis. Sci. 45, 849–880. doi: 10.1111/deci.12094
Devereux, S.. (2003). Policy options for increasing the contribution of social protection to food security ODI. In: Forum for Food Security in Southern Africa Theme Paper, Institute of Development Studies, University of Sussex.
Di Falco, S., Veronesi, M., and Yesuf, M. (2011). Does adaptation to climate change provide food security? A micro-perspective from Ethiopia. Am. J. Agric. Econ. 93, 829–846. doi: 10.1093/ajae/aar006
FAO IFAD, UNICEF, and WHO.. (2019). The state of food security and nutrition in the world 2019: Safeguarding against economic slowdowns and downturns. In: FAO (Ed.), FAO, Rome.
FAO IFAD, and WFP.. (2015). FAO IFAD, and WFP. The State of Food insecurity in the World 2015: Meeting the 2015 International Hunger Targets: Taking Stock of Uneven Progress. Rome: FAO, 2015. Adv. Nutr. 6, 623–624. doi: 10.3945/an.115.009936
FAOSTAT (2020). FAO statistics website, Food and Agriculture Organization of the United Nations, Rome, Italy.
Frelat, R., Lopez-Ridaura, S., Giller, K. E., Herrero, M., Douxchamps, S., Djurfeldt, A. A., et al. (2015). Drivers of household food availability in sub-Saharan Africa based on big data from small farms. PNAS 113, 458–463. doi: 10.1073/pnas.1518384112
Gabre-Madhin, E.. (1999). Transaction costs and market institutions: Grain brokers in Ethiopia, MSSD Discussion Paper No. 31, IFPRI, Washington, DC.
Giller, K. E., Witter, E., Corbeels, M., and Tittonell, P. (2009). Conservation agriculture and smallholder farming in Africa: the heretics' view. Field Crops Res. 114, 23–34. doi: 10.1016/j.fcr.2009.06.017
Harris, I. C., and Jones, P. D. (2017). CRU TS4.01: University of East Anglia Climatic Research Unit (CRU) Time-Series (TS) version 4.01 of high-resolution gridded data of month-by-month variation in climate (Jan. 1901- Dec. 2016). Centre for Environmental Data Analysis.
Hendriks, S.. (2014). Food security in South Africa: status quo and policy imperatives. Agrekon. 53, 1–24. doi: 10.1080/03031853.2014.915468
Jones, A. D., Shrinivas, A., and Bezner-Kerr, R. (2014). Farm production diversity is associated with greater household diversity in Malawi: findings from nationally representative data. Food Policy. 46, 1–12. doi: 10.1016/j.foodpol.2014.02.001
Kassie, M., and Holden, S. T. (2006). Parametric and non-parametric estimation of soil conservation impact on productivity in the northwestern Ethiopian highlands. In: International Association of Agricultural Economists Conference, Gold Coast, Australia.
Kassie, M., Ndiritu, S. W., and Stage, J. (2014). What Determines Gender Inequality in Household Food Security in Kenya? Application of exogenous switching treatment regression. World Develop. 56, 153–171. doi: 10.1016/j.worlddev.2013.10.025
Kassie, M., Yesuf, M., and Köhlin, G. (2009). The role of production risk in sustainable land-management technology adoption in the Ethiopian highlands, Working Papers in Economics. In: University of Gothenburg: School of Business, Economics and Law, Göteborg, Sweden.
Lemon, S. C., Roy, J., Clark, M. A., Friedmann, P. D., and Rokowski, W. (2003). Classification and regression tree analysis in public health: Methodological review and comparison with logistic regression. Annals of Behavioral Medicine 26, 172–181. doi: 10.1207/S15324796ABM2603_02
Lewis, K.. (2017). Understanding climate as a driver of food insecurity in Ethiopia. Clim. Change 144, 317–328. doi: 10.1007/s10584-017-2036-7
Manda, J., Gardebroak, C., Khonje, M. G., Alene, A. D., Mutenje, M., and Kassie, M. (2016). Determinants of child nutrition status in the eastern province of Zambia: the role of improved maize varieties. Food Secur. 8, 239–253. doi: 10.1007/s12571-015-0541-y
Marenya, P., and Barrett, C. B. (2009a). Soil quality and fertilizer use among smallholder farmers in Western Kenya. Agric. Econ. 40, 561–572. doi: 10.1111/j.1574-0862.2009.00398.x
Marenya, P., and Barrett, C. B. (2009b). State-condition fertilizer yield response on Western Kenyan farms. Am. J. Agric. Econ. 91, 991–1006. doi: 10.1111/j.1467-8276.2009.01313.x
Marenya, P., Kassie, M., Teklewold, H., Erenstein, O., Qaim, M., and Rahut, D. (2018). Does the adoption of maize-legume cropping diversification and modern seeds affect nutritional security in Ethiopia? Evidence from panel data analysis. In: 30th International Conference of Agricultural Economists IAAE, Vancourver, Canada.
Mbajiorgu, G.. (2019). Human development and food sovereignty: a step closer to achieving food security in South Africa's rural households. J. Asian Afric. Stud. 55, 330–350. doi: 10.1177/0021909619875757
McCarthy, J., Canziani, O. F., Leary, N. A., Dokken, D. J., and White, C. (2001). Climate Change 2001: Impacts, Adaptation, and Vulnerability Contribution of Working Group II to the Third Assessment Report of the Intergovernmental Panel on Climate Change. University Press, Cambridge, UK.
Naschold, F.. (2012). “The Poor Stay Poor”: household asset poverty traps in rural semi-arid India. World Develop. 40, 2033–2043. doi: 10.1016/j.worlddev.2012.05.006
Nyagumbo, I., Mkuhlani, S., Mupangwa, W., and Rodriguez, D. (2017). Planting date and yield benefits from conservation agriculture practices across Southern Africa. Agric. Syst. 150, 21. doi: 10.1016/j.agsy.2016.09.016
Nyagumbo, I., Mkuhlani, S., Pisa, C., Kamalongo, D., Dias, D., and Mekuria, M. (2016). Maize yield effects of conservation agriculture based maize– legume cropping systems in contrasting agro- ecologies of Malawi and Mozambique. Nutr. Cycl. Agroecosyst. 105, 275–290. doi: 10.1007/s10705-015-9733-2
Nyagumbo, I., Siyeni, D., and Dias, D. (2018). Using improved agronomy to close the yield gap in smallholder farming systems of southern Africa: lessons from the field. In: Network, A. C. T. (Ed.), Second Africa congress on conservation agriculture: making climate-smart agriculture real in Africa with conservation agriculture supporting the Malabo Declaration and agenda 2063 condensed papers book, 2ACCA, Johannesburg, South Africa. pp. 271–274.
Oduol, J. B. A., Binam, J. N., Olarinde, L., Diagne, A., and Adakunle, A. (2011). Impact of adoption of soil and water conservation technologies on technical efficiency: Insight from smallholder farmers in Sub-Saharan Africa. J. Develop. Agric. Econ. 3, 655–669. doi: 10.5897/JDAE11.091
Osborne, J. W., and Costello, A. B. (2009). Best practices in exploratory factor analysis: Four recommendations for getting the most from your analysis. Pan-pacific Manage. Rev. 12, 131–146. doi: 10.7275/jyj1-4868
Parry, M., Rosenzweig, C., and Livermore, M. (2005). Climate change, global food supply and risk of hunger. Phil. Trans. R. Soc. B 360, 2125–2138. doi: 10.1098/rstb.2005.1751
Parry, M., Rozenzweig, C., Iglesias, A., Livermore, M., and Fisher, G. (2004). Effects of climate change on global food production under SRES emisions and socio-economic scenarios. Global Environ. Change. 14, 53–67. doi: 10.1016/j.gloenvcha.2003.10.008
Potgieter, A., Rodriguez, D., Davis, P., and Dimes, J. (2013). From information to actionable knowledge: Hotspots of food insecurity in eastern Africa. In: Gommes, R., and Kayitakire, F. (Eds.), The Challenges of Index-based Insurance for Food Insecurity in Eastern Africa, IRI, Earth Institute, Columbia University, ISPRA, Italy.
Qaim, M.. (2014). Evaluating nutrition and health impacts of agricultural innovations. In: R. T. G. R. “GlobalFood” (Ed.), GlobalFood Discussion Papers, Georg-August-Universität Göttingen, Göttingen.
Qaim, M., and Kouser, S. (2013). Genetically modified crops and food security. PLoS ONE. 8, e64879. doi: 10.1371/journal.pone.0064879
R Core Team (2017). R: A language and environment for statistical computing. Vienna: R Foundation for Statistical Computing. Available online at: https://www.R-project.org/
Sime, G., and Aune, J. B. (2016). Sequential effects of minimum tillage, seed priming, fertilizer microdosing and mulching in maize, semiarid central rift valley Ethiopia. Experim. Agric. 52, 331–345. doi: 10.1017/S0014479715000125
Stephens, E. C., Nicholson, C. F., Brown, D. R., Parsons, D., Barrett, C. B., Lehmann, J., et al. (2012). Modeling the impact of natural resource-based poverty traps on food security in Kenya: The Crops, Livestock and Soils in Smallholder Economic Systems (CLASSES) model. Food Secur. 4, 423–439. doi: 10.1007/s12571-012-0176-1
Teklewold, H., Kassie, M., Shiferaw, B., and Kohlin, G. (2013). Cropping system diversification, conservation tillage and modern seed adoption in Ethiopia: impacts on household income, agrochemical use and demand for labor. Ecol. Econ. 93, 85. doi: 10.1016/j.ecolecon.2013.05.002
Tesfaye, A., Brouwer, R., van der Zaag, P., and Negatu, W. (2016). Assessing the costs and benefits of improved land management practicies in three watershed areas in Ethiopia. Int. Soil Water Conserv. Res. 4, 20–29. doi: 10.1016/j.iswcr.2016.01.003
Therneau, T. M., and Atkinson, E. J. (1997). An introduction to recursive partitioning using the rpart routines. Division of Biostatistics. p. 61.
Tittonell, P., and Giller, K. E. (2013). When yield gaps are poverty traps: the paradigm of ecological intensification in African smallholder agriculture. Field Crops Res. 143, 76–90. doi: 10.1016/j.fcr.2012.10.007
Tittonell, P., Shepherd, K. D., Vanlauwe, B., and Giller, K. E. (2006). Unravelling the effects of soil and crop management on maize productivity in smallholder agricultural systems of western Kenya - An application of classification and regression tree analysis. Agric. Ecosyst. Environ. 123, 137–150 doi: 10.1016/j.agee.2007.05.005
Tittonell, P., van Wijk, M. T., Rufino, M. C., Vrugt, J. A., and Giller, K. E. (2007). Analysing trade- offs in resource and labour allocation by smallholder farmers using inverse modelling techniques: a case-study from Kakamega district, western Kenya. Agric. Syst. 95, 76–95. doi: 10.1016/j.agsy.2007.04.002
US Department of Agriculture Agricultural Research, Service Laboratory ND. (2018). USDA National Nutrient Database for Standard Reference, Legacy, Version Current: 2018.
van Ittersum, M. K., Cassman, K. G., Grassini, P., Wolf, J., Tittonell, P., and Hochman, Z. (2013). Yield gap analysis with local to global relevance—A review. Field Crops Res. 143, 4–17. doi: 10.1016/j.fcr.2012.09.009
von Grebmer, K., Bernstein, J., de Waal, A., Prasai, N., Yin, S., and Yohannes, Y. (2015). 2015 Global Hunger Index: Armed Conflict and the Challenge of Hunger. Welthungerhilfe International Food Policy Research Institute (IFPRI) and Concern Worldwide, Washington, D.C. and Dublin, Ireland.
Webb, P., Coates, J., Frongillo, E. A., Rogers, B. L., Swindale, A., and Bilinsky, P. (2006). Measuring household food insecurity: why it's so important and yet so difficult to do. J. Nutr. 136, 1404S. doi: 10.1093/jn/136.5.1404S
Webb, P., and Kennedy, E. (2014). Impacts of agricultural nutrition: nature of the evidence and research gaps. Food Nutr Bull. 35, 126–132. doi: 10.1177/156482651403500113
Wezel, A., and Rath, T. (2002). Resource conservation strategies in agro-ecosystems of semi-arid West Africa. J. Arid Environ. 51, 383–400. doi: 10.1006/jare.2001.0968
WFP (2018). Mozambique | World Food Program, World Food Program, Via C. G. Viola 68 Parco dei Medici - 00148 Rome, Italy.
WFP (2019). Working round the clock to save lives in the wake of cyclone Idai: US$ 140 million needed as World Food Programme (WFP) steps up assistance in Mozambique. in: W. F. Programme (Ed.), World Food Programme Insight.
Wilkus, E. L.. (2016). Seed Exchange Among Common Bean Producers in Uganda: Examples of Networks That Stimulate Adoption and Market Participation Ecology Graduate Group. University of California, Davis, Davis, CA. pp. 177.
Wilkus, E. L., Roxburgh, C., and Rodriguez, D. (2019). Understanding household diversity in rural eastern and southern Africa. ACIAR, Canberra, ACT.
Yenealem, K., Fekadu, B., Jema, H., and Belaineh, L. (2013). Impact of integrated soil and water conservation program on crop production and income in west Harerghe zone, Ethiopia. IJEMA. 1, 111–120. doi: 10.11648/j.ijema.20130104.11
Zeng, D., Alwang, J., Norton, G. W., Shiferaw, B., Jaleta, M., and Yirga, C. (2017). Ex post impacts of improved maize varieties on poverty in rural Ethiopia. Agric. Econ. 46, 515–526. doi: 10.1111/agec.12178
Zhu, Y., Merbold, L., Leitner, S., Pelster, D. E., Okoma, S. A., Ngetich, F., et al. (2020). The effects of climate on decomposition of cattle, sheep and goat manure in Kenyan tropical pastures. Plant Soil 451, 325–343. doi: 10.1007/s11104-020-04528-x
Keywords: Eastern Africa, poverty-trap, semi-subsistence, Southern Africa, sustainable intensification
Citation: Wilkus EL, deVoil P, Marenya P, Snapp S, Dixon J and Rodriguez D (2022) Sustainable Intensification Practices Reduce Food Deficit for the Best- and Worst-Off Households in Ethiopia and Mozambique. Front. Sustain. Food Syst. 5:649218. doi: 10.3389/fsufs.2021.649218
Received: 04 January 2021; Accepted: 09 December 2021;
Published: 10 January 2022.
Edited by:
Philip Dobie, World Agroforestry Centre (Kenya), KenyaReviewed by:
Polly Joanna Ericksen, International Livestock Research Institute (ILRI), KenyaHeru Irianto, Sebelas Maret University, Indonesia
Copyright © 2022 Wilkus, deVoil, Marenya, Snapp, Dixon and Rodriguez. This is an open-access article distributed under the terms of the Creative Commons Attribution License (CC BY). The use, distribution or reproduction in other forums is permitted, provided the original author(s) and the copyright owner(s) are credited and that the original publication in this journal is cited, in accordance with accepted academic practice. No use, distribution or reproduction is permitted which does not comply with these terms.
*Correspondence: Erin Lynn Wilkus, d2lsa3VzZXJpbkBnbWFpbC5jb20=