- 1Department of Economics, Monash Business School, Monash University, Clayton, VIC, Australia
- 2One Health Unit, The Nossal Institute for Global Health, The University of Melbourne, Melbourne, VIC, Australia
- 3Impact Evaluation, Policy and Foresight Unit, International Rice Research Institute, Los Baños, Philippines
Vietnam faces several adverse climatic stresses such as increases in temperature, drought, flooding, saltwater intrusion, and sea-level rise. Past research on climate change adaptation in Vietnam has highlighted that climatic stresses and challenges faced by populations vary across the country. In this study, we are interested to know if autonomous responses also vary, depending on which stress individuals are responding to. To answer this question, we use primary-collected data of 1,306 individuals from the Mekong River Delta, Central Vietnam, and the Red River Delta. Adaptation choices of these individuals are analyzed at two levels: the household-level and the agricultural-level. We estimate multivariate probit models by Geweke-Hajivassilou-Keane (GHK) simulated maximum likelihood methods. Our results show that climate change adaptations vary depending on which stresses individuals are responding to. At the household level, droughts and floods have the strongest effect on climate change adaptation. However, adaptations at the agricultural level depend more on the impacts of the stress and less so on the climatic strss itself. Understanding what climatic stresses are already eliciting a response, and what adaptations are being used by individuals, is invaluable for designing successful climate change policies. This understanding can also help policymakers identify where gaps exist in individual climate change adaptations and fill these gaps with a public response.
Introduction
The overwhelming consensus of experts is that the climate is changing, and humans are responsible (Oreskes, 2004; Doran and Zimmerman, 2009). Climate change refers to changes in the mean or variability of climate that persists over an extended period, typically of at least a decade, such as global warming (IPCC, 2018). Increased global temperatures bring unprecedented risks to vulnerable populations as a result of disrupting natural systems—examples are increases in the frequency and severity of droughts, floods and other extreme weather events; increased global sea-level rise; and biodiversity loss (IPCC, 2012, 2014; Mysiak et al., 2016).
Vietnam is especially vulnerable to the effect of climate change because of its geography and population demographics. A report from the Asian Development Bank (ADB, 2009) concluded that many of the countries of Southeast Asia are especially vulnerable to the impacts of climate change because of their long coastlines, high concentration of human and economic activity in coastal regions, large and growing populations, and the importance of agriculture as a source of employment and income. Vietnam's vulnerability is high because of its large cities, coastal regions, and high mountain ranges (Albert et al., 2018). Additionally, low-lying river deltas add to its vulnerability and make it one of the most affected countries from adverse climatic stresses, such as flooding, saltwater intrusion, and drought (Dasgupta et al., 2007, 2011). Rural communities that rely on agriculture are some of the most vulnerable populations to climate change because they often have a vulnerable livelihood, reduced adaptive abilities, and live in high-risk areas (Dung and Sharma, 2017). Seventy percent of Vietnam's population lives in rural areas, and around 60% of the rural population relies on agriculture for their incomes (Bergstedt, 2015). The effects of climate change are felt disproportionately by poor households because their livelihoods are more dependent on agriculture than wealthier households (Davies et al., 2009), and climate change decreases agricultural productivity and food security (Iglesias et al., 2011). For example, Jiang et al. (2018) estimate that rice production in Vietnam may see yield reduction of as much as 23% and over 50% over the next two decades for irrigated and rainfed rice, respectively. Increased climate variability will most threaten communities that rely on resources because of their increased vulnerabilities and risk exposure; this is especially true in rural development and agricultural sectors (IPCC, 2012). Climatic stresses will be felt especially hard by agricultural households. There is evidence that these communities are already feeling these effects. A recent study by Trinh et al. (2018), found that farmers in their study are losing 20% of their annual income from agriculture as a result of climate change.
Weather and climate, including rainfall and its timing, the day-to-day high and low temperatures, the frequency, length, and severity of droughts, and basic growing conditions are expected to become more variable for Vietnamese farmers in the near- and long-term future. Studies have forecast increasing average temperatures, sea-level rise, changing precipitation, and increasing drought in regions of Vietnam (Cuong, 2008; IPCC, 2014). IPSONRE (2009) forecast regional climate change, including the three regions covered in our study, the Red River Delta, Central Vietnam, and the Mekong River Delta. Some of these regional forecasts are the same, such as increasing temperature, frequency and intensity of storms, and drought. Other forecasts are variable over regions, Central Vietnam is forecast to have increased rainfall in addition to their seasonal drought, and the Mekong River Delta is forecast to be impacted by increased sea-level rise and salinity intrusion. Sea-level rise of one meter is anticipated to cause severe impacts to the inhabitants of the Mekong River Delta, Red River Delta, and Ho Chi Minh City (MONRE, 2009). In total, between 11 and 25% of the country's population could be directly affected, and GDP losses are estimated to be between 10 and 25% with a one-meter and three-meter increase in sea level, respectively (Dasgupta et al., 2007). Declining agricultural production is anticipated in Vietnam because of direct effects (changes in carbon dioxide availability, precipitation, and temperatures) and indirect effects (reductions in water availability, the transformation of organic matter in soil, increased pest and diseases prevalence, and loss of arable land resulting from the submergence of coastal lands and soil erosion) (World Bank Group and Asian Development Bank, 2020).
With the presence of all these stresses, the Vietnamese government has been proactive in developing climate change policy development. Vietnam, particularly the agricultural and rural development sectors, have developed comprehensive climate change policies with consideration given to adaptation and mitigation (Dung and Sharma, 2017). Examples include the creation of the National Climate Change Strategy in 2011, which lays out strategic objectives to be accomplished by 2050, or the creation of the National Committee on Climate Change created in 2012 (McKinley et al., 2015)1. However, there are opportunities for improvement by bringing in more local stakeholders. Dung and Sharma (2017) state that while the Ministry of Agriculture and Rural Development is ahead in developing policy frameworks for climate change adaptation, current systems do not adequately address the private sector, and local community involvement in responses. Similarly, a key informant interview from McKinley et al. (2015) finds that policy still follows a top-down approach, with almost no consultation with local communities or organizations. Phuong et al. (2018) find that when working in this hierarchical governance system, any effort to support smallholders must be expanded to also engage in policy capacity to ensure efforts are successful. However, Christoplos et al. (2017), bring attention to transformations in climate change adaption in Vietnam that sees local governments playing more important roles in climate change risk reduction—arguing that the role of local government is changing and becoming more responsive facing climate risks. Comprehensive policies with guiding rules to increase the participation of local communities and mechanisms to incentivize them to take part in climate change mitigation and adaptation are essential (Dung and Sharma, 2017). Vietnam has several mitigation options available in agriculture, such as coffee intercropping, use of biochar in maize or rice, and the irrigation technique of alternate wetting and drying in rice production (Escobar Carbonari et al., 2019). Still, climate change is already occurring regardless of how much mitigation is achieved, and moving toward climate change adaptation is urgent and necessary (Owen, 2020). Adaptation and mitigation are not alternatives and must both be pursued, but the costs will influence the choice of policies (Mendelsohn, 2012).
There are numerous climate change adaptations2, falling into different categories and at varying costs. A recent study by Christoplos et al. (2017) finds that Vietnamese farmers' adaptations are increasingly autonomous and less capital intensive. Autonomous adaptations3 are not conscious adaptations to climatic stimuli, but spontaneous responses triggered by changes in natural, market, or human systems (IPCC, 2018). Autonomous adaptations are widely considered to be reactive and undertaken by private actors instead of governments (Malik et al., 2010). Individuals only adopt private adaptations when they are efficient, i.e., when the benefits outweigh the costs, because all of the costs and benefits go to the individual who is making the decision (Mendelsohn, 2000, 2012). Autonomous responses are more often short-run adaptations because the impacts are less uncertain, and benefits are more predictable (Stern, 2007). Long-run are less common than short-run adaptations because of the uncertainty and more substantial capital investments involved in long-term investments (Stern, 2007).
Individuals will make efficient adaptations if they have the resources to do so, but unexpected stress can lead to disruptions in livelihoods, resulting in increased vulnerability from reduced access to social, political, and economic resources (Adger, 1999). Microfinance has the potential to play a significant role in autonomous adaptations by providing households with access to necessary resources (Malik et al., 2010). Agrawala and Carraro (2010) note in their review that the nature of microfinance lending, large volume, and low-value loans is consistent with the needs for adaptation—providing large volumes of decentralized loans. Their review found that significant overlaps already exist between climate change adaptation and microfinance lending. Hammill et al. (2008) argue that microfinance builds resiliency in households by making them less vulnerable to shocks from climatic stresses and more capable of coping with the impacts; as they put it, “the logic here is simple—the more assets and capabilities people have, the less vulnerable they are.”
We are not the first researchers interested in how agricultural households are adapting to climate change in Vietnam. There have been numerous studies about varying topics within climate change responses in Vietnam. Using two villages in central Vietnam, Nguyen et al. (2013) developed a framework to assess rural smallholders' vulnerability and argued rice and other rainfed crops were much more affected by extreme drought or floods compared to tree-based systems and argue for increasing resiliency through adding tree crops. Le Dang et al. (2014), interviewed farmers in the Mekong River Delta, using psychological variables to determine adaptations. They find that farmers are more likely to have an adaptive intention if they perceive higher risks and greater effectiveness of adaption measures. McElwee et al. (2017) looked at perceptions of flooding and flood risk reduction measures across income classes of smallholder farmers in the Red River Delta. They find that poor houses were not less proactive in taking flood risk mitigation measures. Trinh et al. (2018) used a multivariate probit model to investigate determinants of farmer adaptation in Ha Tinh Province. They found that attending agricultural production training, gender, and access to credit (and other socioeconomic variables) were the largest determinants. They also find that some of the most common responses were changing crop varieties, monitoring weather forecasts, and adjusting planting times were some of the most common adaptations in central Vietnam. Waibel et al. (2018) conduct a cross-country study in Vietnam and Thailand to determine if perceptions of climate change are linked to farmers taking adaptive measures. While the answer was yes for both countries, the results for Vietnam are more convincing. Likely a result of more frequent climate-related weather shocks in Vietnam. Lastly, Ylipaa et al. (2019) used focus group discussion in Thai Binh Province to investigate gender inequality in climate change adaption.
Additionally, there have been two studies published that used subsets of the same dataset that we use in our analysis. Mishra and Pede (2017) investigated intra-household gender differences in adaptions to climatic stress for farming households in the Mekong River Delta. They conclude that there are intra-household disparities in responses but leave it to future studies to offer a detailed explanation of these differences. Duffy et al. (2020) use data from the Mekong and Red River Deltas and look primarily at the impacts of farm size and the total number of observed climatic stresses on adaptations.
The intentions of previous publications are generally the same as ours, to provide insights to policymakers in an effort to strengthen climate change policy in Vietnam. However, methods and research questions vary widely across studies, with the most similar study to our own coming from Trinh et al. (2018). While the previous literature adds to the discussion in a meaningful way, we believe that gaps in knowledge still exist and that our novel study fills some of these voids. Namely, we are the first to compare autonomous adaptations across three distinct regions in Vietnam, north, south, and central, using the same survey instrument. Furthermore, while most previous work has investigated a single stress, or climatic stress more generally, we are the first to use a robust dataset to investigate multiple stresses and multiple responses simultaneously. This approach allows us to identify which stresses elicit specific responses from individuals, where gaps exist in responses, and how these responses vary across regions. The purpose of this study is to investigate how climate change adaptation in rice-producing households of Vietnam vary, depending on the primary climate stresses and resulting impacts observed by individuals, where gaps exist in responses, and how these responses vary across regions. These results help policymakers in Vietnam design more targeted and effective government responses by describing what farmers are already doing in response to climatic stress, which stress(es) farmers are most responsive to, and how this varies by location. Modeling multiple responses to numerous climatic stresses is a complicated procedure, but it more realistically captures the decision-making process of smallholder farmers in Vietnam. They experience numerous climatic stimuli and make a series of autonomous adaptation decisions in response to those stimuli.
Methods and Data
Data Collection
Data for this study come from household interviews conducted by the International Rice Research Institute with their local partners in Vietnam; the Institute of Policy and Strategy for Agriculture and Rural Development (IPSARD), and the Vietnam National University of Agriculture (VNAU) as part of the Consultative Group on International Agricultural Research Program on Climate Change, Agriculture, and Food Security. The data collection occurred in three rounds of surveys, with IPSARD overseeing the collection occurring in the Mekong River Delta in early 2015 (succeeding the main rice season), and VNAU overseeing the collecting in the Red River Delta and central Vietnam in mid-2016 (succeeding the winter-spring rice season). These three survey rounds are inclusive of seven provinces of Vietnam; An Giang (n = 180), Bac Lieu (n = 128), Ha Tinh (n = 224), Nam Dinh (n = 210), Quang Ngai (n = 226), Thai Binh (n = 218), and Tra Vinh (n = 120). The survey resulted in a total of 1,306 unique respondents, comprised of husbands and wives from 653 rice-producing households. Missing responses for the key choice variables reduced the number of observations used to 1,290 for the household choice model and 1,244 for the agricultural choice model.
The surveyed provinces in this study were selected based on previous knowledge of climate change issues in each location. The same criteria were used to then select communes, districts, and villages. Once a final selection of villages was determined, the village head (or similar) provided a list of farmers with a household head with at least 10 years of rice-farming experience to the enumerators. Survey participants were then selected from the line lists provided for each village using a stratified random sampling procedure with equal numbers of respondents from each village. Enumerators conducted face-to-face interviews at the respondents' households. Informed verbal consent was obtained from each participant, and then husbands and wives of each household were interviewed privately while their spouses waited in a location in which they could not hear the interview. The survey collected socioeconomic data for each household before moving on to specific questions related to climate stress, impacts of this stress, and individual responses to climatic stress.
Data Description
We are primarily interested in how individual responses vary, depending on which climatic stress most affects each respondent and which impacts are brought on by the reported stress. Enumerators asked the respondents to consider changes in climatic stress and resulting impacts and adaptations from the previous 10-year period, hence why only farming households with at least one household member with 10 or more years of experience were interviewed. We look at two levels of autonomous adaptations from this period. For the household level, we use responses to the question, “What coping strategies do you do in response to the negative impacts of this stress?” and for the agricultural level, we use responses to the question, “What changes in your farming activities did you do during this stress?” We argue for causality in these responses because of the structure of the survey. The questionnaire asks respondents to identify all climatic stresses that are present in their area and then identify the one that most affects them from a list of stresses, previously identified to be present in Vietnam. The definition of these stresses and their material impact on rice production are:
▪ Flooding is extended periods of excessive rainfall, beyond the normal limits for a region. Rice crops exposed to flooding for prolonged periods can fail.
▪ Storms are disturbances in the atmosphere that result in periods of strong winds and heavy rainfall. Heavy winds can destroy rice plants in the paddy through lodging, and sudden rainfall from storms can erode soil and destroy crops.
▪ Salinity intrusion is the movement of seawater inland into freshwater aquifers and rice paddies. When soils become too saline from saltwater intrusion, they are no longer suitable to grow rice.
▪ Sea-level rise is an increase in global sea level, which encroaches into low-lying coastal lands. Suitable agricultural land can be lost to the encroaching sea, or farmers may be forced to invest in expensive infrastructure to protect low-lying coastal lands.
▪ Drought is a shortage of water resulting from an extended period of low rainfall. Periods of drought can increase rice farmers' irrigation costs or even result in total crop failure when irrigation is either not available or too costly.
▪ Heat is extended periods of above-average temperatures. High temperatures, particularly during the flowering period, can cause low yields or total crop failure in rice plants, as a result of spikelet sterility.
All succeeding questions refer to the response for stress that most affects them, including the resulting impacts and autonomous adaptations. Respondents reported which impacts they experienced as a result of the climatic stress by answering a series of binary yes-no questions to signify that the stress caused any of the following impacts—decreases in rice paddy yield, or increases in rice crop loss (e.g., crop destroyed from lodging), food insecurity, indebtedness, or detrimental health impacts.
We model the causal structure of decision making as follows:
Perceived climatic stress → resulting impacts/outcomes → reported autonomous adaptations
Because respondents could have multiple reported responses to climate change, we model their choices using a multivariate probit model. This model allows us to jointly estimate several correlated outcomes simultaneously, and we expect that responses to changing climatic stimuli are correlated. To make the use of this model feasible, we clustered the original responses for the household and the agricultural models into aggregate groups. The group aggregates and the corresponding disaggregate responses are in Appendix 1 and 2 in Supplementary Material for the household and agricultural models, respectively. This step is necessary because multivariate probit models produce 2n choice regimes, where n= the number of dependent variables jointly modeled. There were 14 possible original options (i.e., dependent variables) for the household model, which results in an unmanageable problem where there are 214 or 16,384 choice regimes.
Multivariate Probit Estimation
The applications in this study estimate a set of multivariate probit choice models. Unlike other discrete choice models, such as multinomial logit and generalized extreme value distributions, multivariate probit models allow random preferences across agents, general correlations across simultaneous choices, and unrestricted substitution patterns between those choices (Train, 2009). These are all relevant and important properties of the choice framework for this study.
Any multivariate discrete choice model presents several complications with respect to robust and precise estimation of the unknown model parameters. This is especially true for problems with multiple choice dimensions. These kinds of problems inevitably result in multiple integrals over a probability space, generally without any closed form expression or simple mechanism to evaluate or approximate these integrals. This leads to a need for repeated calculations of approximations to these integrals at each iteration while one estimates the unknown structural parameters of the model. This study employs a fully-developed approach that is well-understood and well-accepted in the econometrics of limited dependent variable models that is known to be computationally efficient (i.e., requires a minimum number of calculations), is accurate (unbiased and consistent), and precise (efficient).
The current industry standard for estimating multivariate limited dependent variable (LDV) models such as the multivariate probit proceeds in two important steps. The first reduces the modeling problem through a set of mathematical transformations to one that is bounded on the multivariate unit interval, [0, 1] × ··· × [0, 1] = [0, 1]N The canonical derivation of these reductions is presented in Genz (1993). We reproduce and briefly discuss these mathematical steps for the case of N = 3. The statistical model is based on the system of latent variables,
and the associated observable indicator variables,
The multivariate probit model estimates the probability that each respondent's choices fall in the appropriately associated regime. The probit model estimates the correlation matrix, R, and normalized slope coefficients, βj, as necessary and sufficient conditions for identification.
For N = 3, let be the set of choice regimes and associate each as follows with the percent corresponding to each regime in parentheses.
Household model:
Agricultural model:
The estimation problem is to find values of (β1, ⋯ , βN, R) to maximize the joint likelihood, or probability, of the survey respondents' falling in the associated reported regimes. Genz's (1993) procedure follows a sequence of recursive changes in variables to the associated probability integrals compactly and conveniently for each respondent. First, define the lower triangular Cholesky factorization of the correlation matrix by R = LL′, where L is a lower triangular matrix with strictly positive main diagonal elements, i.e., for the case of N = 3,
Second, define the i.i.d. standard normal random variables, zijiidN(03, I3), by the system of linear equations, εij = Lzij, so that (εij) = LE(zij) = 03, and (εijεij′) = LE(zijzij′)L′ = R, ∀ i = 1, …, I. This implies the recursive structure for the standard normal random variables:
This gives the probability that the ith survey respondent chooses regime in terms of a recursive set of standard normal integrals, with the limits of integration functions of the lower indexed levels of the standard normal random variates. For example, for ri = 0 we have
where is the standard normal probability density function (pdf). The other seven regimes have analogous probability statements with the upper (lower) limits of integration defined by if yij = 0, (1), for each j = 1, 2, 3. If yij = 0, then the lower limit of integration is −∞, while if yij = 1, then the upper limit of integration is + ∞.
These unbounded limits of integration in all cases lead to difficulties in approximating these multivariate probability statements, whether this is done through quadrature or some other means of estimation. Consequently, Genz (1993) and others (Geweke, 1989, 1991; Hajivassiliou, 1993; Keane, 1993, 1994; Hajivassiliou and Ruud, 1994; McFadden and Ruud, 1994; Hajivassiliou et al., 1996; Hajivassiliou and McFadden, 1998) recursively transform the standard normal random variables to the uniform distribution by,
where is the cumulative distribution function (cdf) of the standard normal random variable.
If yij = 0, then set the lower limit of integration for uij to Uij = 0 and the upper limit of integration for uij to . On the other hand, if yij = 1, then set the lower limit of integration to and the upper limit of integration to be Ūij = 1. Accurate and fast algorithms are available to evaluate the standard normal cdf (Hastings, 1995) and its inverse (Acklam, 2010).
In each individual survey response and at every level of integration, dependence of the sequential limits of integration on and uniform random variables [ui1 … ui,j−1]′ is taken into account explicitly to evaluate and update the likelihood function. However, there remains the additional complication of repeatedly, accurately, and quickly evaluating the multidimensional probability integrals in the multivariate probit model. This is the focus of the second major step in the estimation process, developed and analyzed independently by Geweke (1989, 1991), Hajivassiliou (1993), and Keane (1993, 1994). This step uses unbiased simulations of the unknown probabilities and their derivatives with respect to the estimated parameters in each regime. By construction, these probability simulations are unbiased in each replication. Letting the number of simulations be denoted by S and the probability of a given regime be denoted by Pr, the simple arithmetic average of S independent simulations also is unbiased and has variance Pr(1 − Pr)/S, a small number for reasonably large values of S, since , i.e., 100 simulations for each multivariate probability integral has a variance that is bounded from above by 1/400.
This particular simulation method is commonly known as the GHK “importance sampling” algorithm, to denote the developers Geweke, Hajivassiliou, and Keane. This and many other simulation methods have received a great deal of detailed theoretical and empirical analysis, with noteworthy studies by McFadden (1989); Hajivassiliou and Ruud (1994); McFadden and Ruud (1994); Hajivassiliou et al. (1996); Hajivassiliou and McFadden (1998); Train (2009). The overarching conclusion of these studies is that the GHK algorithm is the most accurate and computationally efficient method to estimate a wide range of LDV models. From the unbiased and precise simulated probability estimates, the invariance principle for maximum likelihood estimators is invoked to generate consistent and asymptotically normal estimators of the structural parameters, B,Σ, and their asymptotic (i.e., large sample) standard errors.
For our study, the individual choice probability, or likelihood function, is given by
Each joint integral is over a proper subset of the 3-dimensional unit cube, [0, 1] × [0, 1] × [0, 1], so that this can be evaluated quickly and precisely with any number of methods. The current industry standard is simulation methods. There is no limit to the number of discrete choices, in principle. However, the curse of dimensionality increases computational time rapidly as the dimension of a problem grows, even with modern computing speeds and power. The full likelihood function for all survey respondents is
The method simulates the likelihood function for each given (xi, β, L) to approximate the integrals on the right-hand-side, and searches of the parameters (β, L) to find the simulated maximum likelihood estimators.
A complete list of independent variables with summary statistics is in Table 1, and descriptions of the variables are in Appendix 3 in Supplementary Material.
Results and Discussion
Climatic Stresses
The provinces surveyed in this study face varied and unique climatic stress. Of those mapped in Figure 1, the most notable result is that very few farmers reported no stress present in their areas. Provinces in the Red River Delta had the highest percentage of no-stress-present responses with 10 and 13% for Thai Binh and Nam Dinh, respectively. However, all other provinces only reported between 1 and 2% that there is no stress present in their area. Climatic stresses are observed widely across the entire country. These results provide empirical evidence in support of climate change vulnerability mapping done by Yusuf and Francisco (2009), which forecast high vulnerability to climate change in all three regions of our study.
Some stresses are reported more homogenously across the country, while others impact individual provinces much more than others. Heat stress is reported more uniformly across provinces by anywhere from one-half to three-quarters of respondents in each province. Drought is frequently reported in all provinces as well, although less frequently in the Red River Delta, where only one-quarter of all respondents report its presence. Other surveyed provinces report drought more frequently, between 41 and 89% of the time. Individuals report the remaining stresses more heterogeneously. Respondents commonly cite flooding in Central Vietnam and An Giang Province, but less so in the Red River Delta and the coastal provinces of the Mekong River Delta. They also report storms least frequently in the Mekong River Delta compared to other locations. Finally, salinity and sea-level rise are more common in low-lying coastal regions. For example, An Giang province is comfortably inland, and nobody from this province reported the presence of either sea-level rise or salinity. Some climatic stresses are felt homogenously across Vietnam, but others vary significantly from one province or region to another.
Household Adaptations
We begin by looking at the autonomous responses to climatic stress at the household level to determine if specific climatic stresses and their impacts are eliciting stronger or more varied responses from individuals. The results of the multivariate probit model for household adaptations are available in Table 24.
We find variations in the type of responses and likelihoods of individuals choosing a specific adaptation depending on the stress that most affected them. Flood and drought stresses elicit the strongest responses. Drought is a significant factor in selecting both financial and lifestyle changes. Flood stress is only a significant factor for financial change, but it has the largest coefficient and highest level of significance among all the stresses. Storm and salinity stresses are also significant factors for individuals choosing a financial change, but only at the 10% level of significance. Individuals responded the least to heat stress in their adaptation decisions. Heat stress is only a significant factor for a lifestyle change adaptation, and it reduced the likelihood of an individual choosing that option. Whether an individual has an autonomous response varies by the type of stress that most affects them.
A financial response is the most common autonomous adaptation selected as a result of stress. The likelihood of a financial response increased for all stresses, except for drought. Additionally, increased debt as an impact of stress correlates with financial response. This unsurprising result is likely from individuals borrowing money as an adaptation strategy; the adaptation is worsening the impact. The popularity of financial adaptations shows the importance of providing affordable credit schemes, such as microfinance, to support the autonomous responses of individuals. Hammill et al. (2008) argue that access to microfinance builds resiliency in households. Furthermore, Mendelsohn (2000, 2012) argues that individuals only make efficient adaptations where the benefits outweigh the costs. Supporting these financial adaptations can improve the efficiency of autonomous responses because it decentralizes decisions to make them more site- and individual-specific.
Drought and heat stress also significantly affected whether or not an individual chose a lifestyle adjustment, albeit only at the 10% level of significance. Drought made an individual more likely to make a lifestyle adjustment, and heat made an individual less likely to make a lifestyle adjustment. Lifestyle adjustments are understandably less common than financial adaptations because these short-run adaptations can have long-lasting consequences. For example, the most detrimental (and thankfully least frequently reported) lifestyle adjustment, taking a child out of school, can burden the child with reduced earnings over their entire lifetime. The other two reported lifestyle adjustments, reducing consumption and working more, are more limited than financial responses because of their explicit binding constraints. There is a ceiling on how many hours a person can work per day and a floor on how little they can consume and still survive.
The effects of the impacts brought on by stress vary by reported adaptation. All three responses significantly correlate with increased debt. Reporting a financial change or receiving outside assistance also significantly correlates with experiencing crop loss. None of the other impacts were significant factors in selecting household responses, likely because most of the data collected in the survey are agricultural impacts and not general impacts that the household may experience from climate change.
The map in Figure 2 provides a spatial representation of where adaptations are happening (or not happening) in Vietnam. The map shows some apparent differences in how individuals in different provinces are adapting to climate change. Generally, fewer adaptations occurred in the Red River Delta and reported adaptations increased farther south in Vietnam. In the Red River Delta, provinces report no change as an adaptation to climate change most frequently, 70 and 71% for Thai Binh and Nam Dinh, respectively. This response is substantially higher than any of the other adaptation options for the region Respondents from the Red River Delta are using fewer autonomous responses than the other regions of the study.
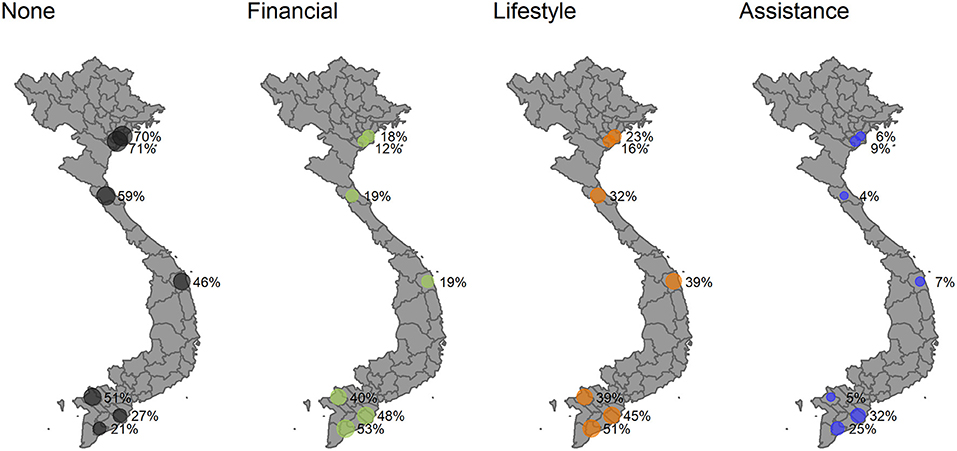
Figure 2. Map showing the percent of respondents practicing household adaptations in each surveyed province.
The Mekong River Delta, and especially the coastal provinces, were the most responsive to climate change. These results from Figure 2, in concert with results from Figure 1, provides some empirical support to Le Dang et al. (2014) that perception of risk drives adaptive intentions. The provinces reporting the most stresses also report the most adaptations. Bac Lieu and Tra Vinh reported the highest percentage of respondents who practice financial changes, lifestyle changes and receive outside assistance. An Giang reported similar values for financial and lifestyle changes but reported receiving assistance much less than the coastal provinces of the Mekong River Delta. The results show that even within the same region, adaptation strategies can vary considerably.
Agricultural Adaptations
A multivariate probit model is also used to analyze autonomous responses to climatic stress through agricultural adaptations. This level of adaptation is similar to the study from Trinh et al. (2018) conducted in Ha Tinh province. The results of this multivariate probit model are in Table 35. Agricultural adaptations are much less responsive to specific climatic stresses than household responses were in the previous section. Instead, individuals are more responsive to the impacts resulting from stress than which stress caused the impact. Only heat elicits a response at the agricultural level. Individuals who report heat stress are more likely to adapt using a crop change on their farm and less likely to adapt by moving resources into livestock production and away from rice production. While moving into livestock was a popular response in some cases, the negative coefficient signals that livestock is also susceptible to heat stress and therefore not a suitable response to this climatic stress.
Adaptations vary, depending on which impact of stress individuals are responding to. Individuals change their rice variety when the resulting impact of the stress is either lower yields or increased debt. Low yield also made individuals more likely to make a crop change. Additionally, individuals made a crop change if they experienced crop loss. Individuals who report food insecurity and increased debt, are both more likely to make a livestock change in which they move away from rice production and into raising livestock. Low yields, crop loss, food insecurity, and increased debt all produce climate change adaptations, but the adaptations vary across the range of impacts.
The three responses to climatic stress estimated in our agricultural model take varying levels of effort from farmers to adopt. For instance, changing from one rice variety to another takes the least effort, as the benefits are embedded in the seed technology, and all other aspects of producing rice remain the same. However, this convenience comes at a price; purchasing rice seed can be expensive compared to other responses. Farmers likely exert more effort with the crop change response because they may need to learn how to grow an unfamiliar crop or invest time to learn about cropping calendars in their regions. Farmers may also face additional costs (e.g., new crops can require new infrastructure) or loss of revenue if they leave lands fallow. Switching from rice to livestock production, particularly for farmers with no previous livestock experience, requires the most effort (e.g., learning about animal health, nutrition, husbandry, etc.) and can also be costly. Not only do farmers have to invest in the livestock, but they may also incur infrastructure costs to accommodate the livestock. The effort and costs associated with these responses help explain the frequency in which farmers report using them (e.g., why switching to livestock is the least common response).
Like the previous section, the map in Figure 3 provides a spatial representation of where agricultural adaptations are happening, or not happening, in Vietnam. Unlike what we find in the household model, the range of individuals that report taking no action to climatic stress is more homogenous across provinces. Taking no action ranged from 32% in Nam Dinh to 42% in An Giang. Most individuals in all provinces already use an autonomous response; however, the adaptation they use varies by location. Reporting a crop change response such as diversifying crops, adjusting the cropping pattern, or leaving land fallow, is the most commonly cited climate change adaptation. Anywhere from 47 to 61% of respondents from each province reported making one of the abovementioned changes to their cropping practices.
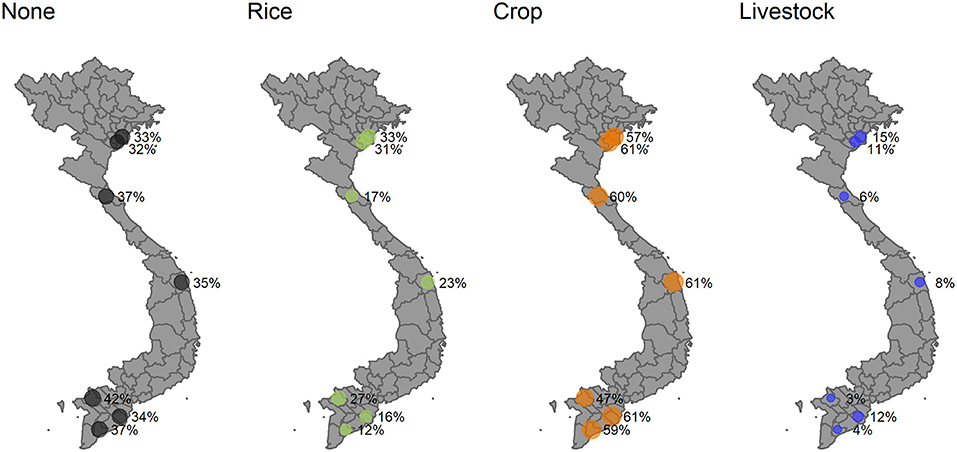
Figure 3. Map showing the percent of respondents practicing agricultural adaptations in each surveyed province.
Responding with a change specific to rice production (i.e., changing rice variety) is the second most commonly cited response behind crop change. However, adopting a new rice variety is the most common response when compared to the individual components of the aggregated crop change response variable. This finding is similar to Trinh et al. (2018), who found changing rice variety was the most popular adaptive practice in their study area. While this is a popular option in our study, there are considerable differences across locations for changing rice varieties. This variation is likely a result of the types of stress present in each of the provinces. The popularity and availability of stress-tolerant rice varieties differ with each of the stresses. The provinces that report changing their rice variety least often are the same provinces that report salinity stress most frequently. While this result may indicate a lack of interest or availability in saline-tolerant varieties at the time of our data collection, a study conducted after our survey by Paik et al. (2020) suggests that salinity-tolerant varieties in the Mekong River Delta are now widely adopted.
Changing from rice to livestock is the least common adaptation selected for all provinces. The rice and crop changes we previously discussed are all short-run adaptations in which inputs to production are varied (Stern, 2007). Livestock is a form of capital (Jarvis, 1974), and capital adjustments are long-run adaptations that are more difficult for individuals to use because of increased uncertainty (Stern, 2007). Individuals from the Red River Delta report livestock investments most commonly, but only 15% of individuals in Thai Binh and 11% of individuals in Nam Dinh report this option. Outside of the Red River Delta, adapting to climate change through livestock is sparsely reported, with all other provinces reporting in the single digits, except for Tra Vinh, where 12% of individuals reported using making a livestock change.
Conclusions
This article set out to better understand if some climatic stresses or impacts from climatic stress elicited stronger climate change adaptations from individuals. The answer to this question is a resounding yes. At the individual adaptation level, drought, flooding, and to a lesser extent, storms and salinity intrusion, elicited the strongest autonomous adaptations from individuals. The most common autonomous response at the household level is to have a financial adaptation, such as selling assets, borrowing money, or using savings. Households using a financial response may provide an opportunity for microfinance lending in Vietnam as a way to build capacity and reduce vulnerability in households as they adapt to climate change. Autonomous adaptations taken in the private market are generally understood to be efficient. Microfinance is a way for poorer households to access the additional resources necessary to carry out efficient autonomous responses to climate change.
Compared to the household level, sources of climatic stress are less critical for adaptation decisions at the agricultural level. At this level, impacts brought on by climatic stress elicited stronger adaptation responses from individuals than the sources of the stress. Farmers who experienced low yields as a result of stress are more likely to adapt their rice-farming practices through changing the variety of rice that they grow. Our results provide field-level evidence that the sources of stress vary across landscapes in Vietnam. These results show the necessity for location-specific adaptation policies in Vietnam, which have been called for in previous publications.
Furthermore, this study provides policymakers with evidence of which stresses, and where, are already causing autonomous adaptations among individuals and the different responses individuals are using. Equally important as climate change action is climate change inaction. We did not find climate change adaptations resulting from specific stresses, such as sea-level rise and saltwater intrusion. This leaves room for a government response to those stresses where private adaptations are presently absent. All the while, the government can financially support private autonomous adaptations, through channels such as microfinance lending. Of course, autonomous adaptations alone are not enough. Instead, it should be seen as a way to help individuals help themselves in the short run, while other planned adaptations and mitigation options are established as part of a comprehensive climate change policy.
Data Availability Statement
The raw data supporting the conclusions of this article will be made available by the authors, without undue reservation.
Ethics Statement
Ethical review and approval was not required for the study on human participants in accordance with the local legislation and institutional requirements. Written informed consent for participation was not required for this study in accordance with the national legislation and the institutional requirements. Approval for data collection was granted by all necessary levels of government in Vietnam and verbal consent was obtained by all participants.
Author Contributions
All authors contributed to the study conception and design. Material preparation and data collection were performed by VP. Data processing and analysis were performed by JM and JL. The first draft of the manuscript was written by JM and all authors provided revisions to the manuscript.
Funding
This research was implemented as part of the CGIAR Research Programs Climate Change, Agriculture, and Food Security (CCAFS), and RICE which are carried out with support from CGIAR Fund Donors and through bilateral funding agreements. For details please visit https://ccafs.cgiar.org/donors. The views expressed in this document cannot be taken to reflect the official opinions of any of these organizations. The study was initiated as part of the Policy Information and Response Platform on Climate Change and Rice in ASEAN and its Member Countries (PIRRCA) Project under CCAFS.
Conflict of Interest
The authors declare that the research was conducted in the absence of any commercial or financial relationships that could be construed as a potential conflict of interest.
Publisher's Note
All claims expressed in this article are solely those of the authors and do not necessarily represent those of their affiliated organizations, or those of the publisher, the editors and the reviewers. Any product that may be evaluated in this article, or claim that may be made by its manufacturer, is not guaranteed or endorsed by the publisher.
Acknowledgments
The authors would like to thank the Institute of Policy and Strategy for Agriculture and Rural Development (IPSARD) and the Vietnam National University of Agriculture (VNUA) for assisting in conducting the survey. Lastly, we thank all the households who participated in the survey.
Supplementary Material
The Supplementary Material for this article can be found online at: https://www.frontiersin.org/articles/10.3389/fsufs.2021.762650/full#supplementary-material
Footnotes
1. ^See McKinley et al. (2015) and Dung and Sharma (2017) for recent reviews of climate change policies in Vietnam.
2. ^Climate change adaptation is commonly defined as an adjustment in natural or human systems in response to actual or expected climate stimuli or their effects, which moderates harm or exploits beneficial opportunities (IPCC, 2018; UNFCCC, 2020).
3. ^See Malik et al. (2010) for a review of autonomous adaptations.
4. ^Some responses at the household level of adaptation were ambiguous. Table 2 includes these ambiguous responses as part of the outside option. An alternative specification that omits ambiguous responses is in Appendix 4 in Supplementary Materials. Similar results are obtained in both specifications of the model.
5. ^Some responses at the agricultural level of adaptation were ambiguous. Table 3 includes these ambiguous responses are part of the outside option. An alternative specification that omits ambiguous responses is in Appendix 5 in Supplementary Materials. Similar results are obtained in both specifications of the model.
References
Acklam, P. J. (2010). An algorithm for computing the inverse normal cumulative distribution function. Peter's Page. Fallbrook, CA: The St. Peter Apostle Catholic School.
ADB (2009). The Economics of Climate Change in Southeast Asia: A Regional Review. Manila: Asian Development Bank.
Adger, W. N. (1999). Social vulnerability to climate change and extremes in coastal Vietnam. World Dev. 27, 249–269. doi: 10.1016/S0305-750X(98)00136-3
Agrawala, S., and Carraro, M. (2010). Assessing the Role of Microfinance in Fostering Adaptation to Climate Change. FEEM Working Paper No. 82., CMCC Research Paper No. 91. Available online at: SSRN:https://ssrn.com/abstract=1646883
Albert, S., Bronen, R., Tooler, N., Leon, J., Yee, D., Ash, J., Boseto, D., and Grinham, A. (2018). Heading for the hills: climate-driven community relocations in the solomon islands and alaska provide insight for a 1.5 C future. Region. Environ. Change 18, 2261–2272. doi: 10.1007/s10113-017-1256-8
Bergstedt, C. (2015). Cultivating Gender: Meanings of Place and Work in Rural Vietnam. Copenhagen K: Nias Press.
Christoplos, I., Le Duc, N., Le Thi, H. S., Nguyen Thi, T. H., and Nguyen, H. (2017). Changing arenas for agricultural climate change adaptation in Vietnam. Dev. Pract. 27, 132–142. doi: 10.1080/09614524.2017.1285272
Cuong, N. (2008). Viet Nam Country Report—A Regional Review on the Economics of Climate Change in Southeast Asia. Report submitted for RETA 6427.
Dasgupta, S., Laplante, B., Meisner, C., Wheeler, D., and Yan, J. (2007). The Impact of Sea Level Rise on Developing Countries: A Comparative Analysis. Washington, DC: The World Bank. doi: 10.1596/1813-9450-4136
Dasgupta, S., Laplante, B., Murray, S., and Wheeler, D. (2011). Exposure of developing countries to sea-level rise and storm surges. Clim. Change 106, 567–579. doi: 10.1007/s10584-010-9959-6
Davies, M., Guenther, B., Leavy, J., Mitchell, T., and Tanner, T. (2009). Climate change adaptation, disaster risk reduction and social protection: complementary roles in agriculture and rural growth? IDS Work. Papers 2009, 1–37. doi: 10.1111/j.2040-0209.2009.00320_2.x
Doran, P. T., and Zimmerman, K. M. (2009). Examining the scientific consensus on climate change. Eos Trans. Amer. Geophys. Union 90, 22–23. doi: 10.1029/2009EO030002
Duffy, C., Pede, V., Toth, G., Kilcline, K., O'Donoghue, C., Ryan, M., and Spillane, C. (2020). Drivers of household and agricultural adaptation to climate change in Vietnam. Clim. Dev. 13, 242–255. doi: 10.1080/17565529.2020.1757397
Dung, P. T., and Sharma, S. (2017). “Responding to climate change in the agriculture and rural development sector in Vietnam,” in Redefining Diversity and Dynamics of Natural Resources Management in Asia, Vol. 2, eds G. Shivakoti, T. N. Thang, N. T. Dung, D. Hulse, and S. Sharma (Hanoi: Elsevier), 13–25. doi: 10.1016/B978-0-12-805453-6.00002-4
Escobar Carbonari, D., Grosjean, G., Läderach, P., Tran, D. N., Sander, B. O., et al. (2019). Reviewing Vietnam's nationally determined contribution: a new perspective using the marginal cost of abatement. Front. Sustain. Food Syst. 3, 14. doi: 10.3389/fsufs.2019.00014
Genz, A. (1993). Comparison of methods for the computation of multivariate normal probabilities. Comput. Sci. Stat. 25, 400–405.
Geweke, J. (1989). Bayesian inference in econometric models using Monte Carlo integration. Econometrica 57, 1317–1339. doi: 10.2307/1913710
Geweke, J. (1991). “Efficient simulation from the multivariate normal and Student-t distribution subject to linear constraints,” in Computer Science and Statistics: Proceedings of the Twenty-Third Symposium on Inference (Seattle, WA: American Statistical Association), 571–578.
Hajivassiliou, V. (1993). “Simulation estimation methods for limited dependent variables models,” in Handbook of Statistics, Vol. 11, Econometrics, eds G. S. Maddala, C. R. Rao, and H. D. Vinod (Amsterdam: North Holland), 519–543. doi: 10.1016/S0169-7161(05)80054-9
Hajivassiliou, V., and McFadden, D. (1998). The method of simulated scores for the estimation of LDV models. Econometrica 66, 863–896. doi: 10.2307/2999576
Hajivassiliou, V., McFadden, D., and Ruud, P. (1996). Simulation of multivariate normal rectangle probabilities and their derivatives theoretical and computational results. J. Econom. 72, 85–134. doi: 10.1016/0304-4076(94)01716-6
Hajivassiliou, V., and Ruud, P. (1994). Classical estimation methods for LDV models using simulation. Handb. Econom. 4, 2383–2441. doi: 10.1016/S1573-4412(05)80009-1
Hammill, A., Matthew, R., and McCarter, E. (2008). Microfinance and climate change adaptation. IDs Bull. 39, 113–122. doi: 10.1111/j.1759-5436.2008.tb00484.x
Hastings, C. (1995). Approximations for Digital Computers. Princeton, NJ: Princeton University Press.
Iglesias, A., Quiroga, S., and Diz, A. (2011). Looking into the future of agriculture in a changing climate. Eur. Rev. Agric. Econ. 38, 427–47. doi: 10.1093/erae/jbr037
IPCC. (2012). Managing the Risks of Extreme Events and Disasters to Advance Climate Change Adaptation–Summary for Policy Makers. 24. Geneva: World Meteorological Organization.
IPCC. (2014). Climate Change 2013: The Physical Science Basis: Working Group I Contribution to the Fifth Assessment Report of the Intergovernmental Panel on Climate Change. Cambridge University Press.
IPCC. (2018). “Annex I: Glossary,” in Global Warming of 1.5°C. An Ipcc Special Report on the Impacts of Global Warming of 1.5°C above Pre-Industrial Levels and Related Global Greenhouse Gas Emission Pathways, in the Context of Strengthening the Global Response to the Threat of Climate Change, Sustainable Development, and Efforts to Eradicate Poverty, eds J. B. R. Matthews (IPCC).
IPSONRE. (2009). Viet Nam Assessment Report on Climate Change (Varcc). Hanoi: Institute of Strategy and Policy on Natural Resources and Environment,
Jarvis, L. S. (1974). Cattle as capital goods and ranchers as portfolio managers: an application to the argentine cattle sector. J. Polit. Econ. 82, 489–520. doi: 10.1086/260209
Jiang, Z., Raghavan, S. V., Hur, J., Sun, Y., Liong, S.-Y., Nguyen, V. Q., and Van Pham Dang, T. (2018). Future changes in rice yields over the Mekong River Delta due to climate change—alarming or alerting? Theor. Appl. Climatol. 137, 545–555. doi: 10.1007/s00704-018-2617-z
Keane, M. (1993). “20 Simulation estimation for panel data models with limited dependent variables,” in Handbook of Statistics, Vol. 11, Econometrics, 545–571. doi: 10.1016/S0169-7161(05)80055-0
Keane, M. (1994). A computationally efficient practical simulation estimator for panel data, with applications to estimating temporal dependence in employment and wages. Econometrica 62, 95–116. doi: 10.2307/2951477
Le Dang, H., Li, E., Nuberg, I., and Bruwer, J. (2014). Understanding farmers' adaptation intention to climate change: a structural equation modelling study in the Mekong Delta, Vietnam. Environ. Sci. Policy 41, 11–22. doi: 10.1016/j.envsci.2014.04.002
Malik, A., Qin, X., and Smith, S. C. (2010). Autonomous Adaptation to Climate Change: A Literature Review. Working Paper Series: 1–25. Washington, DC: Institute for International Economic Policy.
McElwee, P., Nghiem, T., Le, H., and Vu, H. (2017). Flood vulnerability among rural households in the red river delta of vietnam: implications for future climate change risk and adaptation. Nat. Hazards 86, 465–92. doi: 10.1007/s11069-016-2701-6
McFadden, D. (1989). A method of simulated moments for estimation of discrete response models without numerical integration. Econometrica 57, 995–1026. doi: 10.2307/1913621
McFadden, D., and Ruud, P. (1994). Estimation by simulation. Rev. Eco. Stat. 76, 591–608. doi: 10.2307/2109765
McKinley, J., Adaro, C., Pede, V., Setiyono, T., Thanh, C. T., et al. (2015). The Current State of Climate Change Perceptions and Policies in Vietnam: 2014 Report. Los Banos: International Rice Research Institute.
Mendelsohn, R. (2000). Efficient adaptation to climate change. Clim. Change 45, 583–600. doi: 10.1023/A:1005507810350
Mendelsohn, R. (2012). The economics of adaptation to climate change in developing countries. Clim. Change Econ. 3, 1250006. doi: 10.1142/S2010007812500066
Mishra, A. K., and Pede, V. O. (2017). Perception of climate change and adaptation strategies in Vietnam. Int. J. Clim. Change Strat. Manage. 9, 501–516. doi: 10.1108/IJCCSM-01-2017-0014
Mysiak, J., Surminski, S., Thieken, A., Mechler, R., and Aerts, J. (2016). Brief Communication: Sendai framework for disaster risk reduction–success or warning sign for Paris? Nat. Hazard. Earth Syst. Sci. 16, 2189–93. doi: 10.5194/nhess-16-2189-2016
Nguyen, Q., Hoang, M. H., Öborn, I., and van Noordwijk, M. (2013). Multipurpose agroforestry as a climate change resiliency option for farmers: an example of local adaptation in Vietnam. Clim. Change 117, 241–57. doi: 10.1007/s10584-012-0550-1
Oreskes, N. (2004). The scientific consensus on climate change. Science 306, 1686. doi: 10.1126/science.1103618
Owen, G. (2020). What makes climate change adaptation effective? A systematic review of the literature. Glob. Environ. Change 62, 102071. doi: 10.1016/j.gloenvcha.2020.102071
Paik, S., Le, D. T. P., Nhu, L. T., and Mills, B. F. (2020). Salt-tolerant rice variety adoption in the mekong river delta: farmer adaptation to sea-level rise. PLoS ONE 15, e0229464. doi: 10.1371/journal.pone.0229464
Phuong, L. T. H., Biesbroek, G. R., and Wals, A. E. (2018). Barriers and enablers to climate change adaptation in hierarchical governance systems: the case of Vietnam. J. Environ. Policy Plan. 20(4):518–532. doi: 10.1080/1523908X.2018.1447366
Stern, N. (2007). The Economics of Climate Change: The Stern Review. Cambridge: Cambridge University Press. doi: 10.1017/CBO9780511817434
Train, K. (2009). Discrete Choice Methods with Simulation, 2nd Edn. Cambridge: Cambridge University Press.
Trinh, T. Q., Rañola, R. F. Jr., Camacho, L. D., and Simelton, E. (2018). Determinants of farmers' adaptation to climate change in agricultural production in the central region of Vietnam. Land Use Policy 70, 224–231. doi: 10.1016/j.landusepol.2017.10.023
UNFCCC (2020). Glossary of Climate Change Acronyms and Terms. United Nations Framework Convention on Climate Change (accessed April 3, 2020).
Waibel, H., Pahlisch, T. H., and Völker, M. (2018). “Farmers' perceptions of and adaptations to climate change in Southeast Asia: the case study from Thailand and Vietnam,” in Climate Smart Agriculture, eds L. Lipper, N. McCarthy, D. Zilberman, S. Asfaw, and G. Branca (Cham: Springer), 137–160. doi: 10.1007/978-3-319-61194-5_7
World Bank Group and Asian Development Bank. (2020). Climate Risk Profile. Vietnam: World Bank Group and Asian Development Bank.
Ylipaa, J., Gabrielsson, S., and Jerneck, A. (2019). Climate change adaptation and gender inequality: insights from rural Vietnam. Sustainability 11, 2805. doi: 10.3390/su11102805
Keywords: adaptation, climate change, maximum likelihood method, multivariate probit, rice, Vietnam, climatic stress
Citation: McKinley JD, LaFrance JT and Pede VO (2021) Climate Change Adaptation Strategies Vary With Climatic Stress: Evidence From Three Regions of Vietnam. Front. Sustain. Food Syst. 5:762650. doi: 10.3389/fsufs.2021.762650
Received: 22 August 2021; Accepted: 27 September 2021;
Published: 26 October 2021.
Edited by:
Enoch Owusu-Sekyere, Swedish University of Agricultural Sciences, SwedenReviewed by:
Yong Sebastian Nyam, University of the Free State, South AfricaHiroshi Takagi, Tokyo Institute of Technology, Japan
Huynh Viet Khai, Can Tho University, Vietnam
Copyright © 2021 McKinley, LaFrance and Pede. This is an open-access article distributed under the terms of the Creative Commons Attribution License (CC BY). The use, distribution or reproduction in other forums is permitted, provided the original author(s) and the copyright owner(s) are credited and that the original publication in this journal is cited, in accordance with accepted academic practice. No use, distribution or reproduction is permitted which does not comply with these terms.
*Correspondence: Justin D. McKinley, justin.mckinley@unimelb.edu.au