- 1Department of Civil and Environmental Engineering, Princeton University, Princeton, NJ, United States
- 2Department of Soil, Water and Climate, University of Minnesota, Minneapolis, MN, United States
Urban food action plans seek quantitative data on household agriculture gardening, traditionally difficult to quantify rapidly, as well as data on inequality to explore the potential to improve equitable access to fresh vegetables through household agriculture. This article presents a novel hybrid field survey (HFS) method, combining ground surveys with satellite imagery to quantify the prevalence and area of household agriculture gardens, as well as inequality by neighborhood income. We test the method in Minneapolis and St. Paul, Minnesota, (Twin Cities), USA, analyzing the presence/absence (prevalence) and size of agriculture gardens across a total of ~17,500 households in 2017 and repeated in 2020 (during COVID-19). In 2017, the overall mean frequency of household agricultural gardening was 5.0%, with significant differences (2.7 vs. 7.0%) across low- vs. high-income neighborhoods. The city-wide median area per agriculture garden size was 14.6 m2 with greater size (19.8 vs. 11.6 m2) in low- vs. high-income neighborhoods, respectively. Across all income groups, the gardening area was a small fraction of the yard area, suggesting little land availability constraints. Measurements in the summer of 2020 during COVID-19 found the method sensitive over time, showing an overall 60% increase in the prevalence of household agriculture with low-income neighborhoods increasing rates from 3 to 5%. Overall, the method can inform aggregate production potential and inequality in household agriculture.
Introduction
Urban areas are currently home to over half of the world's population, with this percentage predicted to increase to over two-thirds by 2050 (United Nations, 2016). Urban areas also host substantial economic activity, generating 80% of the global gross domestic product (GDP) (The World Bank, 2020). With such concentrations of both population and activity, urban areas are key focus areas to address global environmental (i.e., greenhouse gas emissions, water use, pollution), human health, and wellbeing outcomes (Ramaswami et al., 2016; ACERE, 2018; Ramaswami, 2020).
In recent years, cities are increasingly interested in implementing actions that utilize the food system as a lever to achieve multiple sustainability outcomes, including health, environment, resilience, and economy (Coppo et al., 2017; Sonnino et al., 2019). For example, to date, over 200 cities globally have signed the Milan Urban Food Policy Pact (MUFPP), committing to work toward improving their city's food system (Milan Urban Food Policy Pact, 2015; Candel, 2020). This is in addition to initiatives put forth by prominent city policy organizations, such as the Food and Agriculture Organization's Urban Food Agenda and C40's Food Network to advance city food actions (C40 Cities, 2022; FAO, 2019). The goals of these initiatives are to provide environmental gains by reducing food miles traveled and provide nutritional security for the underserved, while stimulating the local economy. To initiate action, several cities in the US and internationally are creating food action plans to chart pathways toward these potential benefits (e.g., City of Toronto, 2000; City of Seattle Food Interdepartmental Team, 2012).
Within food action plans, urban agriculture, i.e., the growing of food within cities (USDA, 2022), consistently emerges as a key area of focus (Poulsen et al., 2017). The popularity of urban agriculture is widespread, promoted globally by multi-city organizations and agreements (i.e., Milan UFPP) and encouraged through plans and policies at the individual city level, such as the City of Minneapolis's “Urban Agriculture Policy Plan” (Lang, 2014). This trend toward urban agriculture across multiple forms (household gardens, community gardens, larger commercial farms) is motivated by a wide range of assumed benefits (Raja et al., 2017). The Milan UFPP states urban agriculture has the opportunity to “protect biodiversity… and [address] food and nutrition security, ecosystem services and human wellbeing” (Milan Urban Food Policy Pact, 2015). Other proposed benefits include reducing food miles traveled and hence GHG emissions, mitigating urban flooding and heat island impacts, improving wellbeing and economic development, and addressing social inequality (Alaimo et al., 2008; Kulak et al., 2013; Daigger et al., 2015; Miller, 2015).
Despite the breadth of urban agriculture's assumed benefits, there are few empirical studies to quantify these many presumed benefits (Hamilton et al., 2014; Mok et al., 2014; Santo et al., 2016; Siegner et al., 2018). A key part of the challenge is the lack of data on the area and spatial prevalence of agriculture within the city. For example, assessing urban agriculture's contribution to community-wide food demand requires knowing the total growing area under agriculture today combined with yields and types of crops grown. For cities that are trying to improve food security through household gardening education programs (Sickler, 2018), data on the socio-economic inequality in household gardening are an important parameter. This is particularly important because health data indicate higher food insecurity and disease burden in lower-income areas (CDC, 2022). Thus, replicable methods are needed to measure both the prevalence of urban agriculture and its relation to other intra-city features such as income, race, and ethnicity across neighborhoods. Quantitative parameters on household gardening in the current time can also inform indicators to track the progress of local commitments to the Milan Urban Food Policy Pact (2015; Candel, 2020).
However, such quantitative data are lacking particularly for household urban gardens and is the focus of this article. In the context of household urban agriculture, many studies take a more theoretically aspirational approach—estimating the amount of urban agriculture that could exist, based on maps of all yard space, vacant lots, open space, and/or rooftops (e.g., McClintock et al., 2013; Saha and Eckelman, 2017; Goldstein et al., 2017). While these studies can inform the potential for scaling urban agriculture within a city, they do not quantify agriculture as it currently exists, particularly household agriculture gardening which is an important baseline for any policy intervention.
In terms of tracking agriculture as it happens in the present time, the USDA agricultural census predominantly characterizes rural farms and only those urban farms that sell > $1,000 USD in produce per year (USDA, 2019); food grown in homes and community gardens has to date not been accounted for. The USDA is calling for new ways to better track agriculture within urban areas (Young et al., 2018), specifically, addressing community and household gardens that grow for personal consumption. Other proposed methods for quantifying urban agricultural include remote sensing; however, methods reliant solely on satellite imagery also faced challenges, with a classification accuracy of about 67% in Hanoi (Forster et al., 2009). Similarly, Brown and McCarty (2017) found that relying on high-resolution satellite imagery alone was not effective in identifying urban farms in four cities of Detroit (USA), Ho Chi Minh City (Vietnam), Harare (Zimbabwe), and Dakar (Senegal), as did Young et al. (2018) in Baltimore (Brown and McCarty, 2017; Young et al., 2018). The challenge of detection is particularly significant for household agriculture gardens due to their generally small area. For example, one study in Chicago manually tagged urban gardens through Google Earth Satellite imagery (Taylor and Lovell, 2012), with the authors noting greater success with larger community gardens than small household gardens.
To begin to address this knowledge gap, this article develops and tests a hybrid field survey (HFS) methodology that combines remote sensing with transect walks (i.e., in-person surveying of the study area) in city streets to assess the prevalence of household agriculture and its inequality across neighborhoods by socio-economic strata, particularly by income. An example route for a transect walk is shown in the Supplementary material: the unit of analysis is an individual home where field workers identify whether an agricultural garden is present (yes or no), akin to Bernoulli Trial. We define agriculture gardens as distinct areas dedicated to growing edible plants, excluding ornamental gardens and individual fruiting trees located outside of dedicated areas for agricultural production. Combining on the ground field surveys (i.e., transect walks) with satellite imagery to confirm and delineate the area of agricultural gardens can provide a robust approach to quantifying the prevalence of household agriculture. We refer to this combined method as the hybrid field survey (HFS) method. Transect walks have been previously used to quantify waste burning at a fine scale in cities as well as habitat classification (Nagpure et al., 2015; Templ et al., 2019). A secondary goal is to obtain a first-order estimate of the spatial area of household urban agriculture. The specific objectives of this article are to:
1. Develop a hybrid method to identify the frequency and area of household agriculture gardens comparing neighborhoods by income to assess inequality;
2. Develop a first estimate of the overall contribution of household agriculture to meet city-wide food demand;
3. Apply the method in the Minneapolis-St. Paul metropolitan area (Minnesota, USA) during the summer of 2017 and 2020 (summer of COVID-19) to assess the sensitivity of the method over time.
Methods
Methods overview
The hybrid field survey (HFS) methodology combines transect walks (i.e., in-person surveying of the study area) in city streets with aerial imagery to assess the prevalence and size of household agricultural plots and their inequality across neighborhoods by socio-economic strata, particularly by income.
We clarify that the HFS method is not intended to tag every garden in every household across cities, but rather it can be employed to quantify the statistical features of household agricultural gardening, i.e., frequency (prevalence) as well as the average household agricultural garden area. HFS results can then be scaled up to estimate the overall contribution of household agriculture to city-wide food demand that can inform potential environmental benefits, in addition to quantifying socio-economic inequality in the context of income. Thus, our article evaluates the frequency in size of household gardening across neighborhoods by income, given that income is an important aspect of nutritional inequality in cities.
We also apply the method across 2 years to assess how COVID has impacted urban gardening—with several anecdotal reports suggesting an increase in gardening (Evans and Davies, 2020; Gbadegesin and Olajiire-Ajayi, 2020; Pulighe and Lupia, 2020). Thus, the method can inform aggregate production to address environmental sustainability and quantify social inequality in household agriculture over time. There is potential for this method to be readily replicated in other cities.
The HFS method for urban agriculture garden identification has five main steps: (1) selection of sample areas across the city for transect walks to represent neighborhoods of varying income, important for informing food insecurity and inequality; (2) field implementation of the HFS method, combining transect walks with aerial imagery; (3) data analysis to quantify the frequency and area of household agriculture for transect sites, along with uncertainty analysis; (4) estimating accuracy of HFS Method; and (5) scaling up to determine household urban food production's contribution to city-wide food demand. Each of these steps is described in the following subsections.
Survey sites selection
We developed and tested our method in the cities of St. Paul and Minneapolis, Minnesota, USA, together referred to as the Twin Cities, in summer of 2017 (with subsequent replication in 2020). The Twin Cities have a reported population density of 2,140 people/km2 and 2,880 population/km2, respectively (United States Census Bureau, 2020). The cities are known for harsh winters which constrain the typical agriculture gardening season from mid to late May to early September (University of Minnesota Extension, 2020), limiting the in-person survey period to the Summer season.
The Twin Cities of Minneapolis and St. Paul together comprise a total of 628 Census Block Groups (CBGs) with areas of low (<$43,942), middle ($43,942–$67,500), and high (>$67,500) incomes, classified on a per household basis (United States Census Bureau, 2012). Of these, we selected 46 CBGs to implement the HFS method, choosing CBGs across income categories and based on the ease of viewing gardens during the street transect walks, i.e., CBGs with alleyways, where backyards (and the existence of potential agriculture gardens) can be viewed more easily. Figure 1 shows the distribution of 46 CBGs in our study, distributed across the city encompassing 13 low-, nine middle-, and 24- high-income CBGs with suitable alley access to view the backyards from the street, a total area of 17 km2 (total Twin Cities area = 294 km2).
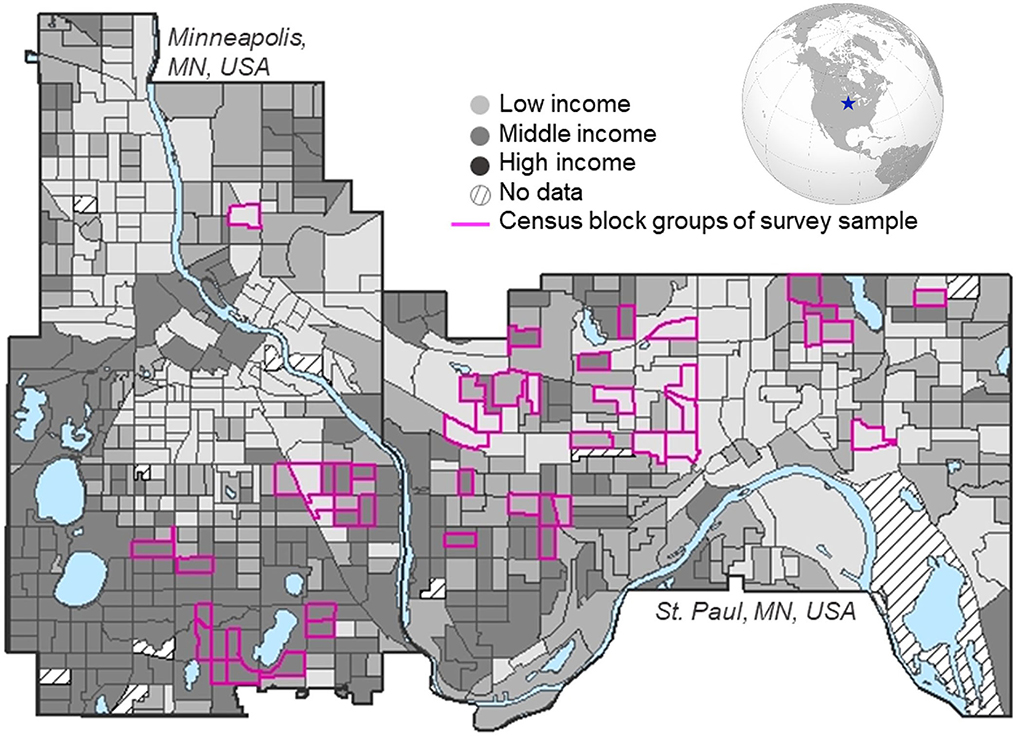
Figure 1. Map of Minneapolis (left) and St. Paul (right), together referred to as the Twin Cities, showing the distribution of high—(dark gray), middle—(medium gray), and low—(light gray) income by household. The pink outlines illustrate the census block groups included in the ground survey. Geographic location of the Twin Cities in reference to North America starred in the top right of the figure.
Data collection (transect walk)
Researchers with horticultural knowledge conducted a transect survey by walking, bicycling, or driving along every street and alleyway in the 46 CBGs, visually inspecting the front and back yards (treated identically) for the presence or absence of agriculture gardens, and delineating the boundaries of identified gardens in a satellite imagery app GPS Fields Area Measure, Rento UAB (n.d.). Fruiting trees and other types of household agriculture, such as vegetables grown in single pots were too small to be detectable. Each individual household property in the neighborhood was studied and characterized into three categories: (a) households where the entire front or backyards were not visible due to fencing; (b) households with visible yards where an agriculture garden was noted to be present, or (c) households with visible yards where an agriculture garden was noted to be absent. The period of field observation was from June to August, bound by the time span of the local growing season. On average, a team of two to three took ~1–1.5 h to complete a survey of a single CBG. Supplementary Figure 1 shows an example transect walk and resulting data for a single CBG.
Data analysis methods
Overall, we covered 18,188 households on the transect walk across the 46 CBGs, which is akin to a Bernoulli trial, measuring the presence or absence of gardens among the visible yards, and identifying 1,184 gardens. The prevalence rate of gardens was computed as the number of household where agriculture gardens were identified, divided by the total number of households with visible yards. CBGs with more than 10% of yards not visible due to fencing were excluded from subsequent analysis. Of the 46 CBGs surveyed, 38 (83%) met the conditions of minimum visibility (>90% of households' yard area was visible) and were included in subsequent statistical and scaling analyses. This corresponds to a total of 11,196 residential parcel lots as recorded by the cities, corresponding with 17,501 households (United States Census Bureau, 2012). Since the unit of analysis is each home for the prevalence (yes or no) of household agricultural gardening, this represents a substantially large sample size for statistically estimating the prevalence (Bartlett et al., 2001; e.g., much greater than the minimum sample size of 650 estimated for a margin of error of ±5% for categorical variables). Even after delineating the sampled homes into three groups (low-, medium-, and high-income), this represents a very large sample size of individual homes.
When sample sizes are large, theory indicates that the frequency (prevalence rate) in a Bernoulli trial is binomially distributed (Sinharay, 2010) with:
Mean p = nominal value of frequency of observation of an agricultural garden in a sample of n visible households (1)
And
These parameters were used for the statistical analysis of the differences in the mean frequency of gardens in the low-, middle-, and high-income areas. Because we have a three-way comparison, we conducted an ANOVA followed by a post-hoc Tukey test to test if the means are different.
The agriculture garden areas outlined during the transect walks were found to follow a log-normal distribution. We, therefore, use the log-transformed data (Limpert et al., 2001) to analyze statistical differences between the median garden sizes across income groups, using ANOVA and a post-hoc Tukey's honest significant difference (HSD) test on the log-transformed data to determine statistical differences across the three means. In addition, we compared garden size to total available yard size for a set of 100 households to determine the potential correlation of available space to the size of the agriculture garden.
Estimating accuracy of the HFS method
We assessed the accuracy of garden identification (and garden area) during transect walks with two different tests. To estimate the accuracy of garden identification during the transect walk, we independently selected a test sample of 63 households that were known to have agriculture gardens based on their enrollment in a separate soil and life-cycle assessment study (led by co-author Jelinski). Of the households in our test sample where the front and back yards were visible (45/63 homes), the presence or absence of an agriculture garden was correctly identified in 42 households by the field transect walk with 93% accuracy. An approach of no household contact was taken to avoid knocking on doors for verification, which was a sensitive issue during the 2020 and 2021 growing seasons, due to concerns with COVID-19.
To estimate the error in quantifying garden area through marking garden boundaries on satellite imagery, we manually measured 20 public test plots on the ground (in addition to 63 plots in the test homes to determine frequency accuracy) and compare the resulting area by the area as determined by marking the garden boundary on satellite imagery. The root mean square error between the two approaches was 30%.
Based on the accuracy assessments noted above, we determined that our study methods were robust, and the HFS approach offers an improvement to garden detection compared to methods reliant solely on satellite imagery.
Scaling and contextualizing results
To assess the relative contribution of household agriculture production to city-wide food demand, we scale the results of the HFS method to the whole of the Twin Cities. We multiply the mean household agricultural garden area for each income bracket (high, middle, low) and frequency of households with gardens by the total number of households city-wide within that bracket, to estimate the total area of household agriculture garden production area within the whole of the Twin Cities. The arithmetic mean is used for scaling up across households and is appropriate based on the mathematical definition of the (arithmetic) average as the sum of all agriculture garden areas divided by the number of households having agriculture gardens. We subsequently estimated the production quantity of these areas by multiplying city-wide production area by an approximate yield of 1.33 kg of fresh produce per square meter [an average from multiple field studies (Cleveland, 1997; Duchemin et al., 2009; CoDyre et al., 2015)].
To quantify the contribution of urban agriculture gardens to local food demand, we also compare the annual garden yields with the annual residential demand for fresh vegetables. We determine city-wide residential fresh vegetable demand, using the USDA estimated 85 kg/capita per year of fresh vegetable availability (at the retail level) on (Nixon and Ramaswami, 2018; USDA Economic Research Service, 2019). This per capita demand is multiplied by the total population of Minneapolis and St. Paul of 415,622 and 303,637, respectively (United States Census Bureau, 2012).
Results
Analysis of overall frequency and area per agriculture garden
Within the 38 CBGs with suitable yard visibility, through on the ground field surveys, we manually identified a total of 179,174, and 528 agriculture gardens in low-, middle-, and high-income areas, respectively, out of 6,885, 3,283, and 7,333 total homes, respectively. Table 1 shows a summary of surveyed CBGs by income group, the number of total households, as well as the frequency of garden. The overall mean frequency across the entire population of homes was 5.0%. The mean frequency of household agriculture gardens shows statistical differences across income brackets (see Figure 2). On average, 2.6, 5.3, and 7.2% of low-, middle-, and high-income households, respectively, have an agriculture garden within their yard. An ANOVA followed by a post-hoc Tukey HSD test illustrates a significant difference between all pairwise groupings (low-medium, medium-high, low-high, p < 0.001).
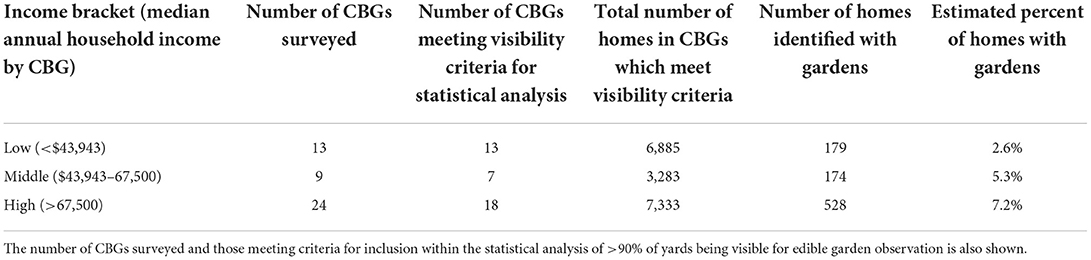
Table 1. Census block groups (CBGs), the number of homes surveyed, and those identified with gardens across areas of low-, middle-, and high-income CBGs.
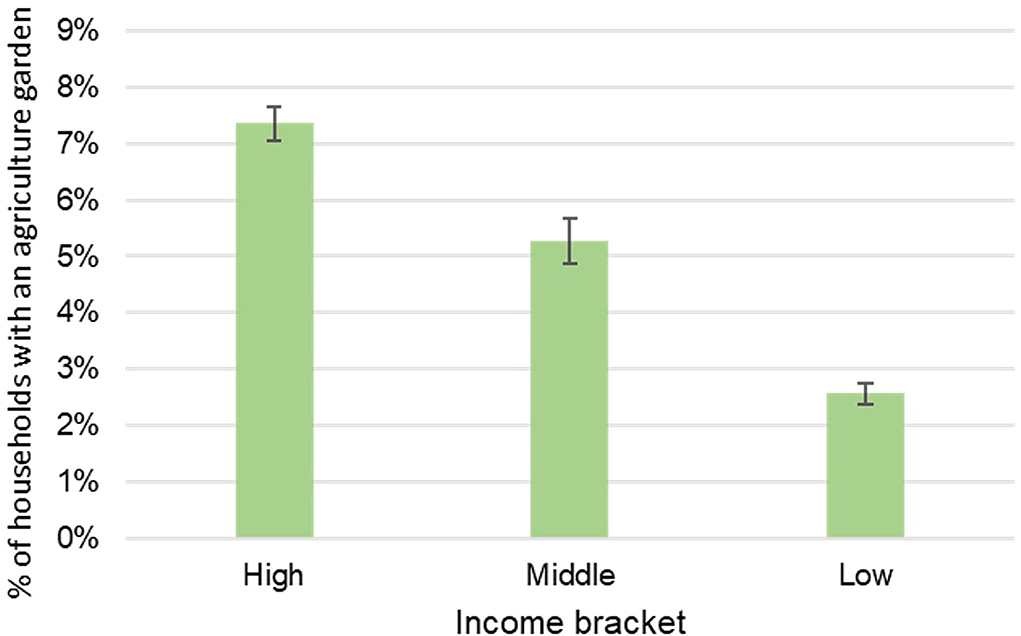
Figure 2. Frequency of households with an agriculture garden across three income brackets (high, middle, low) in the Twin Cities. Error bars represent the 95% confidence interval of the mean.
The average garden area was 14.6 m2 for the overall population of homes that had a garden. The data on per agriculture garden area followed a log-normal distribution, which is a key finding of our study. We, therefore, evaluated differences in garden areas across income levels with the use of natural log-transformed data (see Limpert et al., 2001). The mean of the log-transformed data corresponds with the back-transformed median agriculture garden areas of 19.8, 19.2, and 11.6 m2, for low-, middle-, and high-income areas, respectively. An ANOVA test followed by a post-hoc Tukey HSD test determines a significant difference between low vs. high-income (p-value < 0.001) and middle- vs. high-income areas (p-value < 0.001) (Figure 3).
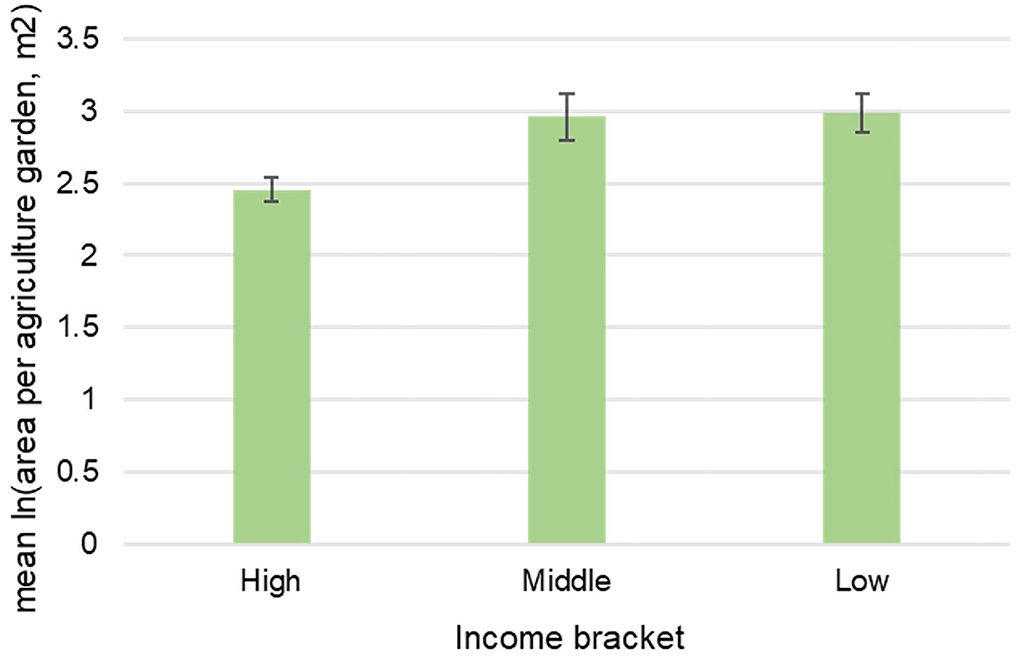
Figure 3. Natural log-transformed mean area per agriculture garden by income bracket (low, middle, high) in the Twin Cities. Error bars represent the 95% confidence interval of the displayed mean.
Comparison of available yard space (individual parcel area minus all built structures and paving) vs. the area of agriculture garden within the corresponding parcel of 100 agriculture gardens across all three income groups, finds a weak correlation (R2 = 0.2, p = 0.000) and small slope (0.089) between area dedicated to an agriculture garden and the total physical land available to the household. The median percentage of agriculture garden to yard space was 10% (range of 1–47%). This suggests that the availability of yard space itself does not appear to limit the agricultural gardening area.
First-order estimate of city-wide production
The study-wide average frequency of household agriculture gardening [5.0% (±0.002%)] was multiplied by the city-wide population and the study-wide estimate of the mean per garden area of 26 m2 (±30%) to compute the contribution of household gardening to be 39 ha (±30%), and corresponding to 316 tons of fresh produce. Scaling up by the population in various income strata in Figures 2, 3, found the current potential to be 36 ha and corresponding to 290 tons of fresh vegetables, an estimate within 10% of the earlier estimate (that was not income stratified by income).
The overall annual fresh vegetable demand of residents at the retail level within the Twin Cities is 60,942 tons. Thus, the HFS method enables the first assessment of current household agriculture production as contributing nominally 290–316 tons annually, which is ~0.5% of fresh vegetable demand. These estimates provide an important baseline upon which city policies can be measured. This result may potentially be representative of other U.S. cities with cold climates. Replication of this method in other cities will provide further information on the variation of agricultural gardening practices by city.
Comparison with 2020
Repeating the HFS during summer of 2020, surveying 36 of the CBGs in the original sample (inclusive of 15,559 households) provides the first quantification of potential changes in gardening prevalence during COVID-19. City-wide, we find a 60% increase in the percentage of households with an agriculture garden from 5% in 2017 to 8% in 2020. Figure 4 shows a sample area of the city, delineating gardens pre (blue polygons) and during COVID-19 (red dots) illustrating this increase in garden prevalence.
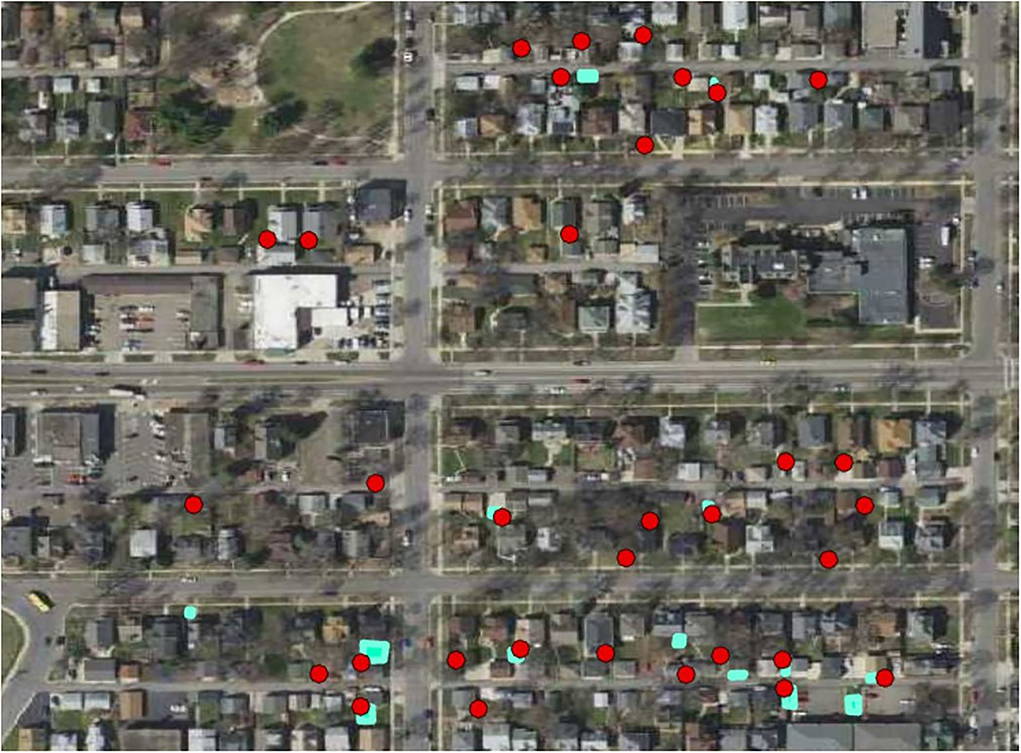
Figure 4. A sample area in the Twin Cities (Minneapolis and St. Paul, MN, USA) illustrates an increased prevalence in households with an agriculture garden. Household agriculture gardens identified via the hybrid field survey method in the summer of 2017 are marked with blue polygons vs. 2020 as red dots.
While there was a substantial increase in the prevalence of household agriculture gardens city-wide, differences exist across income groups. In low-income areas, the prevalence of households with an agriculture garden nearly doubled, increasing from 3% in 2017 to 5% in 2020. Middle-income areas saw a similarly substantial increase, increasing from 6 to 10% between 2017 and 2020. High-income areas saw a more modest increase from 7% in 2017 to 10% in 2020 (Figure 5).
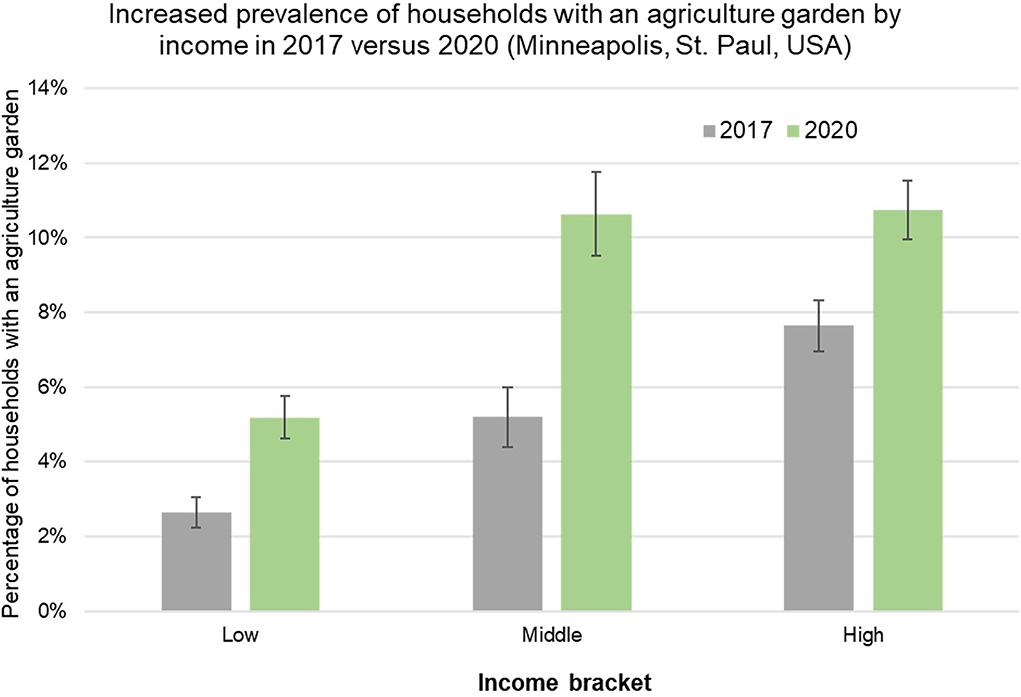
Figure 5. Prevalence of households with an agriculture garden by income in the Twin Cities (Minneapolis and St. Paul, MN, USA) in the summer of 2017 (gray) and 2020 (green). Error bars illustrate the 95% confidence interval of the mean.
Discussion
Prior methods reliant exclusively on satellite imagery have reported challenges in identifying households that practice agricultural gardening, which include the presence of tree canopies, diversity of garden crops and form, the small size of many household gardens, and the temporal and seasonal variation of gardens. For example, Taylor and Lovell (2012) could identify only 0.4% of households as participated in agricultural garden in Chicago, which they acknowledge as a likely underestimate, due to the difficulties in identifying gardens via aerial imagery. In contrast, our HFS method is successful in identifying households with agriculture gardens with an estimated accuracy of 93%. Further, we computed an overall frequency of gardening at a single point in time that far exceeds prior remote sensing estimates—in the Twin Cities 5% in 2017 to 8% in 2020 of households city-wide. These numbers are an order of magnitude higher than what remote sensing has previously been able to detect (e.g., 0.4% in Taylor and Lovell, 2012). However, this figure still appears to be less than reported by surveys, e.g., ~20% frequency (Das and Ramaswami, 2022; National Gardening Association Research Division, 2021). However, this could be due to a difference in surveys not asking about people gardening in that specific year. Moreover, the HFS method, as practiced in this study, did not quantify fruiting trees and single potted plants.
Importantly, the estimated frequency of household agriculture gardens via the HFS method does not require seeing every garden in every home. Our frequency estimate derives from CBGs where >90% of yards did not have fencing that obscured the view of yards and contained >15,000 households. For such a large sample, the study is synonymous with a Bernoulli trial and yielded robust estimates of the mean percentage of households with gardens and enabled comparison across neighborhoods by the income level. Nominal prevalence rates are 5% across the income groups and statistically different rates of 2.6, 5.3, and 7.2% in low-, middle-, and high-income areas, with significant differences between the prevalence of all three groupings.
Application of the HFS method during COVID-19 illustrates the method's sensitivity to changes in agriculture prevalence over time and its utility as a tool to assess changes in gardening due to events or city intervention. The results illustrate a 60% increase in the prevalence of household agriculture gardening community-wide, with differences in the magnitude of increase across high, middle, and low-income areas in a medium-sized U.S. city. While all three income categories exhibited an increase in gardening, further research might explore potential differing motivations for this increase between income categories, e.g., gardening as a means of food security in lower-income areas vs. serving as a leisure activity in higher incomes areas.
Conclusion
Hybrid Field Survey methods offer a rapid, robust, and ground-truthed alternative to remote sensing methods in estimating the scale and other characteristics of urban household agricultural gardening that can be utilized in other cities to evaluate differences across city types and further develop the methodology. The HFS methodology offers a number of advantages when compared to methodologies that solely utilize satellite imagery, especially regarding the small size of household gardens. When compared to independent data on household gardening, the current methodology had an estimated accuracy of 93%; this should be evaluated in additional field studies in other cities, which is feasible given the method's ease of replication.
One of the potential barriers to replication is the visibility of yards for the transect walk, which had relatively high visibility in the Twin Cities area but may be different in other cities.
The HFS methods revealed statistically significant differences in the frequency and size of agricultural gardens across income strata in the Twin Cities. Such data can be useful for equitable urban agriculture planning, particularly given that case studies have shown that household gardening in underserved neighborhoods has the potential for beneficial nutrition outcomes (Sickler, 2018). Our study reveals interesting results that homes in low-income CBGs have a lower frequency of agricultural gardens but larger garden areas.
Future use of HFS methods could look at other demographic factors reflected in census data (e.g., race and ethnicity, education, etc.) which could further inform equity. A temporal comparison noted an overall 60% increase in the prevalence of gardens between 2017 and 2020, during the beginning of the COVID pandemic and lockdowns. From an equity perspective, it is notable that the low- and middle-income groups had higher increase in garden prevalence than the high-income group. Our results are in line with recent surveys of pre-, during-, and post-pandemic gardening behaviors (including turf grass, etc., San Fratello et al., 2022). The HFS approach has the advantage of focusing on agricultural gardening rather than greenery in general, which is important for agrifood security.
Our scaling-up results show that household gardening contributes little in aggregate, <0.05% of household fresh vegetable demand city-wide in the Twin Cities. This suggests that urban agriculture as is currently practiced at this scale will have very little impact on many presumed environmental benefits such as resource circularity or avoided food miles. Indeed, unless it is significantly more efficient than large-scale vegetable production, urban agriculture may actually increase environmental impacts (McDougall et al., 2019). However, our study shows that urban agriculture can have benefits to equity, particularly during the pandemic. Indeed, these equity benefits may be the best reason to pursue urban agriculture as has been suggested by others (Alaimo et al., 2008; Litt et al., 2011; Santo et al., 2016; Ambrose et al., 2020), for which the HFS method developed in this article can offer a robust methodology for analysis and monitoring.
Data availability statement
The raw data supporting the conclusions of this article will be made available by the authors, without undue reservation.
Author contributions
DB and AR equally contributed to the study design, analytical method, writing of the article, and design of graphics. Additionally, AR coordinated and conducted multiple rounds of revisions of the article. PN conducted fieldwork for the article and contributed to the subsequent analysis and writing of the paper. NJ contributed to the science of gardens, analysis, and estimating the accuracy of garden identification. All authors contributed to the article and approved the submitted version.
Funding
This study was funded by an Agriculture and Food Research Initiative (AFRI) grant from the National Institute of Food and Agriculture at the National Science Foundation/United States Department of Agriculture - Innovations at the Nexus of Food, Energy, and Water Systems (INFEWS) (Grant No. 2019-67019-30463) and the National Science Foundation's Sustainable Research Network (SRN) (Award # 1444745).
Acknowledgments
The authors would like to thank students that contributed at various stages of the project, including Rachel Kosse, Graham Ambrose, Meghna Subha, Koehl Simmons, Rafi Barash, Morgan Schultz, and Camila Merino Franco. Base maps used in Figure 1 include maps of census block groups of the Twin Cities downloaded from the US Census Tigerfiles cache, and a globe map of the United States used in Figure 1 was downloaded from Wikipedia Commons.
Conflict of interest
Author DB was affiliated with Princeton University and the University of Minnesota while the research was taking place, and after the conclusion of the research is now employed by Cargill.
The remaining authors declare that the research was conducted in the absence of any commercial or financial relationships that could be construed as a potential conflict of interest.
Publisher's note
All claims expressed in this article are solely those of the authors and do not necessarily represent those of their affiliated organizations, or those of the publisher, the editors and the reviewers. Any product that may be evaluated in this article, or claim that may be made by its manufacturer, is not guaranteed or endorsed by the publisher.
Supplementary material
The Supplementary Material for this article can be found online at: https://www.frontiersin.org/articles/10.3389/fsufs.2022.997081/full#supplementary-material
References
ACERE (2018). Sustainable Urban Systems: Articulating a Long-Term Convergence Research Agenda. National Science Foundation (Alexandria, VA, USA). Advisory Committee for Environmental Research & Education.
Alaimo, K., Packnett, E., Miles, R. A., and Kruger, D. J. (2008). Fruit and vegetable intake among urban community gardeners. J. Nutr. Educ. Behav. 40, 94–101. doi: 10.1016/j.jneb.2006.12.003
Ambrose, G., Das, K., Fan, Y., and Ramaswami, A. (2020). Is gardening associated with greater happiness of urban residents? A multi-activity, dynamic assessment in the Twin-Cities region, USA. Landscape Urban Plan. 198, 103776. doi: 10.1016/j.landurbplan.2020.103776
Bartlett, J. E., Kotrlik, J. W., and Higgins, C. C. (2001). Organizational research: determining appropriate sample size in survey research. Inform. Technol. Learn. Performance J. 19, 43–50. Available online at: https://scholar.google.com/scholar?cluster=6309593362850930082&hl=en&as_sdt=0,31&as_vis=1
Brown, M. E., and McCarty, J. L. (2017). Is remote sensing useful for finding and monitoring urban farms? Appl. Geogr. 80, 23–33. doi: 10.1016/j.apgeog.2017.01.008
C40 Cities (2022). The Food System Network. C40 Cities Climate Leadership Group. Available online at: https://www.c40.org/networks/food-systems-network/ (accessed 2022).
Candel, J. J. (2020). What's on the menu? A global assessment of MUFPP signatory cities' food strategies. Agroecol. Sustain. Food Syst. 44, 919–946. doi: 10.1080/21683565.2019.1648357
CDC (2022). Social Determinants of Health. U.S. Centers for Disease Controls & Prevention. Available online at: https://www.cdc.gov/socialdeterminants/ (accessed 2022).
City of Seattle Food Interdepartmental Team (2012). City of Seattle Food Action Plan. City of Seattle. Available online at: https://www.seattle.gov/environment/sustainable-communities/food-access/food-action-plan (accessed 2022).
City of Toronto (2000). Toronto's Food Charter. City of Toronto. Available online at: https://www.toronto.ca/legdocs/mmis/2018/hl/bgrd/backgroundfile-118057.pdf (accessed 2022).
Cleveland, D. (1997). Are urban gardens an efficient use of resources. Arid Lands Newsletter 42, 1–5.
CoDyre, M., Fraser, E. D., and Landman, K. (2015). How does your garden grow? An empirical evaluation of the costs and potential of urban gardening. Urban Forestry Urban Greening 14, 72–79. doi: 10.1016/j.ufug.2014.11.001
Coppo, G., Stempfle, S., and Reho, M. (2017). Urban food strategies and plans: considerations on the assessment construction. City Territory Architecture 4, 1–7. doi: 10.1186/s40410-017-0064-2
Daigger, G. T., Newell, J. P., Love, N. G., McClintock, N., Gardiner, M., Mohareb, E., et al. (2015). “Scaling up agriculture in city-regions to mitigate FEW system impacts,” in Proceedings of the FEW Workshop: “Scaling Up” Urban Agriculture to Mitigate Food-Energy-Water Impacts. Ann Arbor, MI: University of Michigan, 5–6.
Das, K., and Ramaswami, A. (2022). Who gardens and how in urban USA: informing social equity in urban agriculture plans. Front. Sustain. Food Syst. 6:923079. doi: 10.3389/fsufs.2022.923079
Duchemin, É., Wegmuller, F., and Legault, A.-M. (2009). Urban agriculture: Multi-dimensional tools for social development in poor neighbourhoods. Field Act. Sci. Rep. 2, 1–8. doi: 10.5194/facts-2-1-2009
Evans, D., and Davies, J. (2020). 4 reasons why the world needs more urban farming post-pandemic. World Economic Forum. Available online at: https://cityfarmer.info/4-reasons-why-the-world-needs-more-urban-farming-post-pandemic/ (accessed 2022).
FAO (2019). FAO Framework for the Urban Food Agenda. Rome: Food and Agriculture Organization of the United Nations. Available online at: https://www.fao.org/3/ca3151en/CA3151EN.pdf (accessed 2022).
Forster, D., Buehler, Y., and Kellenberger, T. (2009). Mapping urban and peri-urban agriculture using high spatial resolution satellite data. J. Appl. Remote Sens. 3, 033523. doi: 10.1117/1.3122364
Gbadegesin, A., and Olajiire-Ajayi, B. (2020). Beyond COVID-19: turning crisis to opportunity in Nigeria through urban agriculture. J. Agriculture Food Syst. Commun. Dev. 9, 171–174. doi: 10.5304/jafscd.2020.094.033
Goldstein, B. P., Hauschild, M. Z., Fernández, J. E., and Birkved, M. (2017). Contributions of local farming to urban sustainability in the Northeast United States. Environ. Sci. Technol. 51, 7340–7349. doi: 10.1021/acs.est.7b01011
Hamilton, A. J., Burry, K., Mok, H. F., Barker, S. F., Grove, J. R., and Williamson, V. G. (2014). Give peas a chance? Urban agriculture in developing countries. A review. Agron. Sustain. Dev. 34, 45–73. doi: 10.1007/s13593-013-0155-8
Kulak, M., Graves, A., and Chatterton, J. (2013). Reducing greenhouse gas emissions with urban agriculture: a life cycle assessment perspective. Landscape Urban Plan. 111, 68–78. doi: 10.1016/j.landurbplan.2012.11.007
Lang, U. (2014). Cultivating the sustainable city: urban agriculture policies and gardening projects in Minneapolis, Minnesota. Urban Geogr. 35, 477–485. doi: 10.1080/02723638.2014.916142
Limpert, E., Stahel, W. A., and Abbt, M. (2001). Log-normal distributions across the sciences: keys and clues: on the charms of statistics, and how mechanical models resembling gambling machines offer a link to a handy way to characterize log-normal distributions, which can provide deeper insight into variability and probability—normal or log-normal: that is the question. BioScience 51, 341–352. doi: 10.1641/0006-3568(2001)0510341:LNDATS2.0.CO;2
Litt, J. S., Soobader, M. J., Turbin, M. S., Hale, J. W., Buchenau, M., and Marshall, J. A. (2011). The influence of social involvement, neighborhood aesthetics, and community garden participation on fruit and vegetable consumption. Am. J. Public Health 101, 1466–1473. doi: 10.2105/AJPH.2010.300111
McClintock, N., Cooper, J., and Khandeshi, S. (2013). Assessing the potential contribution of vacant land to urban vegetable production and consumption in Oakland, California. Landscape Urban Plann. 111, 46–58. doi: 10.1016/j.landurbplan.2012.12.009
McDougall, R., Kristiansen, P., and Rader, R. (2019). Small-scale urban agriculture results in high yields but requires judicious management of inputs to achieve sustainability. Proc. Natl. Acad. Sci. 116, 129–134. doi: 10.1073/pnas.1809707115
Milan Urban Food Policy Pact (2015). Milan Urban Food Policy Pact. Available online at: https://www.milanurbanfoodpolicypact.org/wp-content/uploads/2020/12/Milan-Urban-Food-Policy-Pact-EN.pdf (accessed 2018).
Miller, W. M. (2015). UK allotments and urban food initiatives:(limited?) potential for reducing inequalities. Local Environ. 20, 1194–1214. doi: 10.1080/13549839.2015.1035239
Mok, H. F., Williamson, V. G., Grove, J. R., Burry, K., Barker, S. F., and Hamilton, A. J. (2014). Strawberry fields forever? Urban agriculture in developed countries: a review. Agron. Sustain. Dev. 34, 21–43. doi: 10.1007/s13593-013-0156-7
Nagpure, A. S., Ramaswami, A., and Russell, A. (2015). Characterizing the spatial and temporal patterns of open burning of municipal solid waste (MSW) in Indian cities. Environ. Sci. Technol. 49, 12904–12912. doi: 10.1021/acs.est.5b03243
National Gardening Association Research Division (2021). National Gardening Survey 411 2021. Available at: https://gardenresearch.com/view/national-gardening-survey-2021-412 edition/ (accessed 2022).
Nixon, P. A., and Ramaswami, A. (2018). Assessing current local capacity for agrifood production to meet household demand: Analyzing select food commodities across 377 US metropolitan areas. Environ. Sci. Technol. 52, 10511–10521. doi: 10.1021/acs.est.7b06462
Poulsen, M. N., Neff, R. A., and Winch, P. J. (2017). The multifunctionality of urban farming: perceived benefits for neighbourhood improvement. Local Environ. 22, 1411–1427. doi: 10.1080/13549839.2017.1357686
Pulighe, G., and Lupia, F. (2020). Food first: COVID-19 outbreak and cities lockdown a booster for a wider vision on urban agriculture. Sustainability 12, 5012. doi: 10.3390/su12125012
Raja, S., Morgan, K., and Hall, E. (2017). Planning for equitable urban and regional food systems. Built Environ. 43, pp.309–314. doi: 10.2148/benv.43.3.309
Ramaswami, A. (2020). Unpacking the urban infrastructure nexus with environment, health, livability, well-being, and equity. One Earth. 2, 120–124. doi: 10.1016/j.oneear.2020.02.003
Ramaswami, A., Russell, A. G., Culligan, P. J., Sharma, K. R., and Kumar, E. (2016). Meta-principles for developing smart, sustainable, and healthy cities. Science 352, 940–943. doi: 10.1126/science.aaf7160
Rento UAB (n.d.). GPS Fields Area Measure. Available online at: https://play.google.com/store/apps/details?id=lt.noframe.fieldsareameasure&hl=en_US&gl=US (accessed 2022).
Saha, M., and Eckelman, M. J. (2017). Growing fresh fruits and vegetables in an urban landscape: a geospatial assessment of ground level and rooftop urban agriculture potential in Boston, USA. Landscape Urban Plan. 165, 130–141. doi: 10.1016/j.landurbplan.2017.04.015
San Fratello, D., Campbell, B. L., Secor, W. G., and Campbell, J. H. (2022). Impact of the COVID-19 pandemic on gardening in the United States: postpandemic expectations. HortTechnology 32, 32–38. doi: 10.21273/HORTTECH04911-21
Santo, R., Palmer, A., and Kim, B. (2016). Vacant Lots to Vibrant Plots: A Review of the Benefits and Limitations of Urban Agriculture. Baltimore, MD: Johns Hopkins Center for a Livable Future.
Sickler, J. (2018). Homegrown Program Evaluation Results: 2015-2017. Pittsburgh, Pennsylvania. Available online at: https://static1.squarespace.com/static/619e8565e81f2234b8e5c20f/t/61b7c33f54fb6f75e579f9ce/1639433024233/Phipps+-+Homegrown+Final+Evaluation+Report+2017+-+FINAL.pdf (accessed 2022).
Siegner, A., Sowerwine, J., and Acey, C. (2018). Does urban agriculture improve food security? Examining the nexus of food access and distribution of urban produced foods in the United States: a systematic review. Sustainability 10, 2988. doi: 10.3390/su10092988
Sinharay, S. (2010). “Discrete probability distributions,” in International Encyclopedia of Education. Elsevier Ltd., 132–134.
Sonnino, R., Tegoni, C. L., and De Cunto, A. (2019). The challenge of systemic food change: Insights from cities. Cities 85, 110–116. doi: 10.1016/j.cities.2018.08.008
Taylor, J. R., and Lovell, S. T. (2012). Mapping public and private spaces of urban agriculture in Chicago through the analysis of high-resolution aerial images in Google Earth. Landscape Urban Plan. 108, 57–70. doi: 10.1016/j.landurbplan.2012.08.001
Templ, B., Mózes, E., Templ, M., Földesi, R., Szirák, Á., Báldi, A., et al. (2019). Habitat-dependency of transect walk and pan trap methods for bee sampling in farmlands. J. Apicult. Sci. 63, 93–115. doi: 10.2478/jas-2019-0014
The World Bank (2020). Urban Development Overview. Available at: https://www.worldbank.org/en/topic/urbandevelopment/overview (accessed 2018).
United Nations (2016). The World's Cities in 2016. Available at: https://www.un.org/en/development/desa/population/publications/pdf/urbanization/the_worlds_cities_in_2016_data_booklet.pdf (accessed 2022). doi: 10.18356/8519891f-en
United States Census Bureau (2012). 2012-2016 ACS 5-Year Estimates. Available online at: https://www.census.gov/programs-surveys/acs/technical-documentation/table-and-geography-changes/2016/5-year.html (accessed 2022).
United States Census Bureau (2020). QuickFacts: St. Paul City. Minnesota; Minneapolis City, Minnesota. Available online at: https://www.census.gov/quickfacts (accessed 2022).
University of Minnesota Extension (2020). Extending the Growing Season: Start Early, End Later How to Use Plastic Mulch. Available online at: https://extension.umn.edu/planting-and-growing-guides/extending-growing-season (accessed 2018).
USDA (2019). CropScape - Cropland Data. National Agricultural Statistics Service. United States Department of Agriculture. Available online at: https://www.nass.usda.gov/Research_and_Science/Cropland/SARS1a.php (accessed 2018).
USDA (2022). National Agricultural Library: Urban Agriculture. United States Department of Agriculture. Available online at: https://www.nal.usda.gov/legacy/afsic/urban-agriculture (accessed 2018).
USDA Economic Research Service (2019). Food Availability (Per Capita) Data System. United States Department of Agriculture. Available online at: https://www.ers.usda.gov/data-products/food-availability-per-capita-data-system/ (accessed 2022).
Keywords: urban agriculture, Milan Urban Food Policy Pact, urban food systems, household gardening, urban food action planning, food access, food equity
Citation: Ramaswami A, Boyer D, Nixon P and Jelinski N (2022) A hybrid method to quantify household urban agriculture gardening: Implications for sustainable and equitable food action planning. Front. Sustain. Food Syst. 6:997081. doi: 10.3389/fsufs.2022.997081
Received: 18 July 2022; Accepted: 23 September 2022;
Published: 28 October 2022.
Edited by:
Charlie Shackleton, Rhodes University, South AfricaReviewed by:
Patrick Likongwe, Leadership for Environment and Development Southern and Eastern Africa (LEAD SEA), MalawiSumita Ghosh, University of Technology Sydney, Australia
Copyright © 2022 Ramaswami, Boyer, Nixon and Jelinski. This is an open-access article distributed under the terms of the Creative Commons Attribution License (CC BY). The use, distribution or reproduction in other forums is permitted, provided the original author(s) and the copyright owner(s) are credited and that the original publication in this journal is cited, in accordance with accepted academic practice. No use, distribution or reproduction is permitted which does not comply with these terms.
*Correspondence: Anu Ramaswami, YW51LnJhbWFzd2FtaUBwcmluY2V0b24uZWR1
†These authors have contributed equally to this work and share first authorship