- 1Department of Food System Sciences, Research Institute of Organic Agriculture–FiBL, Frick, Switzerland
- 2Department of International Cooperation, Research Institute of Organic Agriculture-FiBL, Frick, Switzerland
- 3Université Cheikh Anta Diop–UCAD, Dakar, Senegal
- 4International Institute of Tropical Agriculture–IITA, Bamako, Mali
Introduction: Agroforestry plays a vital role in maintaining and developing the resilience and productivity of farms and landscapes. Scientific evidence from the Sahel region suggests that integration of trees and shrubs has the potential to improve temperature and moisture levels whilst providing bio-based fertilizer that contributes to increased yields of annual crops. However, little is known about the factors that influence the diffusion of agroforestry. This study examines joint decisions on the use of agroforestry alongside other complementary agricultural practices and disentangles agroforestry awareness from adoption and disadoption decisions.
Methods: Our analysis is based on a comprehensive farm-level dataset covering almost 3,000 farm households in Mali and Senegal. A large number of adoption determinants are utilized, with a special focus on information access, information flows and social groups.
Results: The findings suggest that extension access and training participation boost awareness of agroforestry-based soil fertility management, while information provided by public extension, NGOs and community members is strongly associated with higher adoption intensity. In the analysis of disadoption, farmer-to-farmers exchange in the community was found to be a key factor in the decision to maintain agroforestry use. Membership in cooperatives and youth groups appear to have a favorable effect on awareness and adoption in Mali, but less so in the Senegalese case. Similarly, only results from Mali show that adoption of agroforestry is accompanied by the adoption of other sustainable intensification practices and lower use of synthetic pesticides.
Discussion: We conclude that in order to support the transition to more widespread agroforestry-based soil fertility management, it is essential to strengthen public and NGO-based advisory systems that fully engage with local knowledge networks.
1. Introduction
Across the globe, food systems face the triple challenge of ensuring food and nutrition security for a growing population, providing livelihoods for farmers and others in the food chain, and improving environmental sustainability (FAO, 2018; HLPE, 2019; Barrett et al., 2020; Gerten et al., 2020). When it comes to crop production, sustainable intensification has been widely promoted in many low and middle-income countries to reconcile economic and environmental objectives in agricultural development (Pretty et al., 2011; Kuyper and Struik, 2014; Rockström et al., 2017; Cassman and Grassini, 2020). Agroforestry, as one of the key sustainable intensification strategies, facilitates the enhancement of resilience and productivity at both the farm and landscape scale (Nair and Garrity, 2012; Mbow et al., 2014; Catacutan et al., 2018; Kay et al., 2019; Muchane et al., 2020). Policy-makers have put agroforestry firmly on the agenda for food system transformation and climate-smart agriculture (FAO, 2013, 2016; HLPE, 2019). It is also key to redesigning production systems along agroecological principles (Pretty et al., 2011). As in other parts of the globe, agroforestry helps to provide benefits for the environment, farmer livelihoods and food sovereignty in Sub-Saharan Africa (SSA) (Mbow et al., 2014; Bogie et al., 2018; Leroux et al., 2022).
The Sahel region is particularly vulnerable to climate and other shocks, where frequent droughts hamper efforts to eliminate malnutrition and alleviate rural poverty (Läderach et al., 2021). The integration of shrubs and trees into cropping systems has a particularly promising potential in this context. Underlying factors for realizing the benefits of agroforestry are hydraulic redistribution of water among the trees or shrubs and annual crops, availability of tree and shrub residues to improve soil health, and diversification of nutrition and income sources (Sinare and Gordon, 2015; Kuyah et al., 2019; Miller et al., 2020; Leroux et al., 2022). In Niger, long-term research has demonstrated the positive livelihood impacts of farmer-managed natural regeneration of existing tree crops (Tougiani et al., 2008). Scientific evidence from Senegal suggests that the integration of trees and crops has great potential to positively affect ecosystem services, such as temperature and moisture levels, and to ultimately increase yields (Bayala et al., 2015; Bright et al., 2017; Bogie et al., 2018). Harnessing these factors is essential to help meet the future challenges in food security and sustainable development in the Sahel and elsewhere.
Despite these benefits and decades of promotion of agroforestry practices by national and international organizations, the adoption and practice of agroforestry remain under-documented and poorly understood. Improving the effectiveness of agroforestry diffusion requires a deeper understanding of the farmers' decision-making around agroforestry practices. A substantial literature exists on the determinants of adoption of agricultural practices, with a focus mostly on agronomic aspects and supporting interventions, such as improved varieties, new crop protection sprays or mineral fertilizer subsidies (Pannell and Zilberman, 2020; Piñeiro et al., 2020; e.g. Arslan et al., 2020; Ruzzante et al., 2021). Less is known about the factors that influence the diffusion and adoption dynamics of agroforestry or similar agroecological approaches (Arslan et al., 2020). Regression models often only capture farmers' binary adoption decision, however, to analyse technological change context-specific processes must also be accounted for (Glover et al., 2019). The concept of technology adoption (along with the related concepts of diffusion and scaling) is commonly used to design development interventions, frame impact evaluations and inform decision-making about new investments in development-oriented agricultural research. In all but the simplest cases, binary adoption is likely to be inadequate to capture the complex reconfiguration of social and technical components of an innovation. While some simplification is needed in the quantitative estimation of adoption models, it is important to consider not only a yes/no adoption decision, but rather to understand the process and context of farm-level choices (Pannell and Zilberman, 2020). Therefore, our study combines the analysis of joint adoption decisions with the analysis of factors that influence awareness, adoption intensity and disadoption.
The analysis in this paper is based on the premise that agroforestry provides tangible socio-economic returns and, under the right circumstances, adoption is a rational choice. For the purpose of our research, we refer to agroforestry as the active management of shrubs and trees for improved soil fertility and crop productivity. This is reflected in the literature on ecosystem services from agroforestry in the Sahel (Dossa et al., 2012; Sinare and Gordon, 2015; Leroux et al., 2022) and also emerged as the dominant agroforestry benefit perceived by farmers included in our survey. In the context of this study, application of agroforestry thus involves assisted natural regeneration of shrubs and trees and the active utilization of their residues as fertilizer. While there are multiple other benefits of agroforestry, for example the provision of nutrient-dense foods and livestock fodder, their exhaustive inclusion is beyond the scope of this study. Based on the relevance of training, extension and knowledge in recent adoption reviews (Arslan et al., 2020; Piñeiro et al., 2020; Ruzzante et al., 2021), we focus in particular on the influence of information access and information flows, alongside aspects of social networks and other characteristics of the farmer and farm. Overall, the study aims (i) to examine which agricultural practices are jointly adopted with agroforestry and, (ii) to assess the determinants of agroforestry awareness, adoption intensity and disadoption decisions.
2. Materials and methods
2.1. Study area and data collection
Our adoption research focuses on five sites, namely Fatick in Senegal as well as Kayes, Koulikoro, Segou and Sikasso in Mali. These sites represent cropping and integrated crop-tree-shrub systems in the semi-arid zone of the Sahel with annual rainfall between 500 and 900 mm, with the exception of Sikasso, which has slightly higher precipitation levels (Millennium Ecosystem Assessment, 2005). The main soil types in the study area are regosols, arenosols and podsols, as well as ferric and plinthic luvisols (Bationo et al., 2012). Among others, important trees at the study sites include Faidherbia albida, Vitellaria paradoxa, Parkia biglobosa, while important shrubs at the study sites are Guiera senegalensis and Piliostigma reticulatum. The sites correspond roughly to administrative units, covering one whole region in Senegal and large parts of four distinct regions in Mali (Figure 1). To account for the specific country contexts, we split the sampling, data collection and analysis by country. Results are representative of the five sites we included, and indicative of trends in the wider semi-arid Sahel of West Africa. However, they are not representative of the entire countries, nor the entire Sahel region.
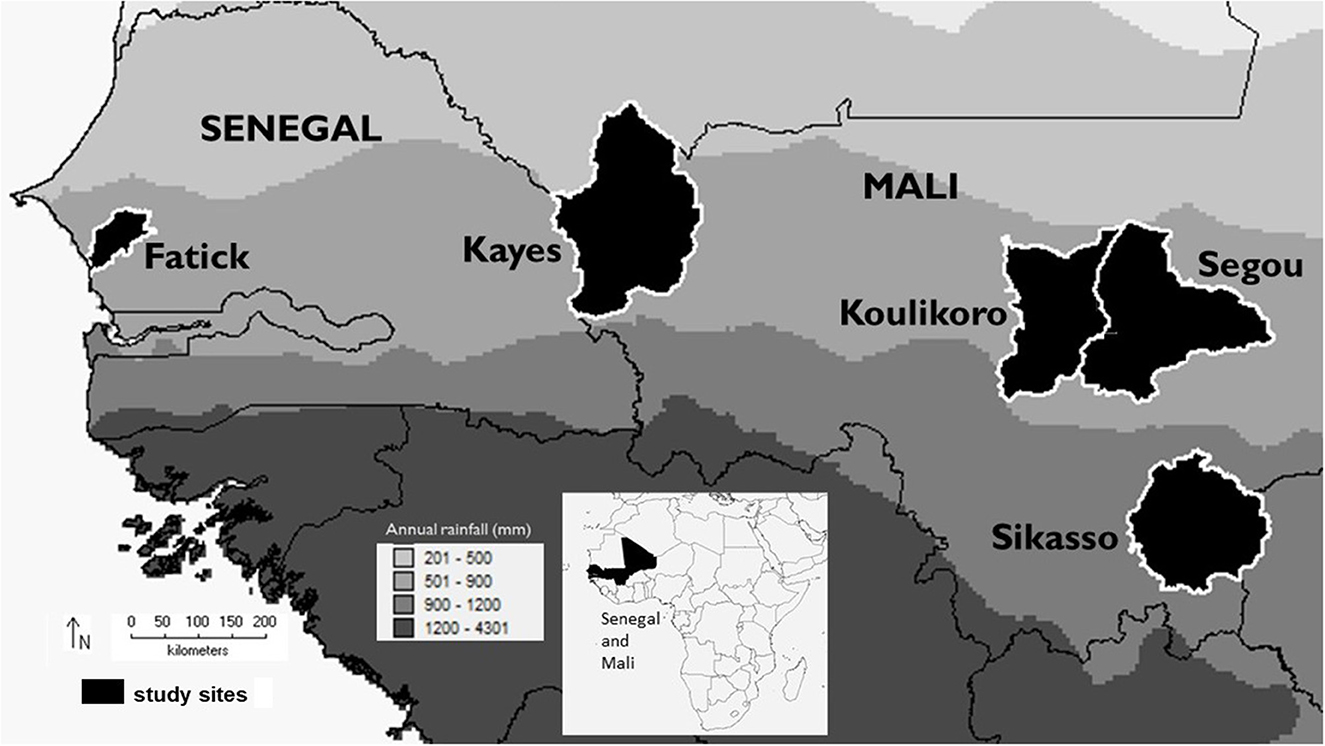
Figure 1. Map of the study area with 5 sites (source: all map layers were available in DIVA-GIS: www.diva-gis.org).
To select farm households for data collection in both countries, a two-stage cluster sampling procedure was applied. In the first stage, we randomly selected 80 villages from a sampling list of villages at each of the five sites. The inclusion of a village in the sampling list was contingent on the farmers in the village having agriculture as the main source of livelihood (including cereal and legume production). Once the villages had been chosen, between six and nine households per village were randomly selected to participate in the survey, depending on the overall farm household population at each site. This resulted in a dataset covering 2,168 farm households across the Kayes, Koulikoro, Segou and Sikasso sites in Mali and 720 farm households across 80 villages in the Fatick site of Senegal.
For the data collection, a structured questionnaire was designed, consisting of ~250 questions covering plot, household, and village-level characteristics (see section A3 in the Appendix). The questionnaire was administered in the local languages to the head of the household. All enumerators and supervisors were trained for ~2 days to ensure that they were sufficiently familiar with the questionnaire and process before the field survey. We conducted a pre-test of the survey instrument before the actual data collection process, whereby two villages in each country were selected for the pre-testing. The pre-test experience was used to modify the questionnaire to ensure that the questionnaire was well-structured, easily understood by the enumerators, and devoid of ambiguity regarding the definition of the questions. The pre-test was also useful in determining the average time it takes to complete one questionnaire, which was ~2 h. Data collection was carried out in 2019 in Mali and 2020 in Senegal, with field surveys taking place in both years between June and August.1
2.2. Response variables
To derive a comprehensive picture of adoption drivers and impacts of the diffusion process, response variables for agroforestry include awareness (whether farmers have heard of the practice), adoption (whether farmers have adopted the practice), adoption intensity (proportion of farm area, where the practice is used) and disadoption (whether farmers have discontinued the use of the practice after initial adoption).2 The primary deciding factor for inclusion of practices, either complementary or competing with agroforestry, was related to the general level of use of that practice, with five percent sample adoption, in at least one of the two countries, as minimum cut off for practice inclusion. This allows focusing on more widely known and thus relevant practices, while also ensuring that our model will solve. When including practices with a low number of adopters in the analysis of joint adoption decisions, it was otherwise difficult to achieve convergence in the maximum likelihood estimation due to the few adopters. Consequently, we included four agroecological practices (in addition to agroforestry) and three conventional practices, as shown in Table 1. The first group of practices include mulching and composting, intercropping, improved rotations and the use of animal manure. These practices are commonly referred to as ecological intensification or climate-smart agricultural strategies in the literature (Arslan et al., 2020; Pannell and Zilberman, 2020; Piñeiro et al., 2020; Ruzzante et al., 2021). The second group is comprised of improved crop varieties, mineral fertilizers and synthetic pesticides. To various extents, the selected practices are part of intensification and natural resource management strategies in the Sahel (Aune and Bationo, 2008; Asfaw et al., 2016; Nord et al., 2022). For all practices, we constructed awareness and use (adoption) measures from our data. These were coded as binary variables, whereby 1 indicates that a farmer is using the practice and 0 would indicate the reverse (Table 1).
For agroforestry, between 51 and 55 percent of farmers at the five sites have heard of the practice in Mali and Senegal, respectively, but only 21 and 40 percent actually use it (Table 1). The higher adoption rate in Senegal may be a result of long-term efforts to disseminate agroforestry-based soil fertility management at the Fatick site3 Disadoption rates are relatively low, ranging from seven to two percent of farmers in Mali and Senegal, respectively.
2.3. Adoption determinants
Farmers' adoption decision of the different practices is often influenced by a host of socio-economic factors (e.g., education, access to and ownership to assets), market and institutional factors (e.g., access to extension and credit) as well as environmental factors (e.g., soil types, rainfall patterns). Ruzzante et al. (2021) identified farmer education, household size, land area, access to credit, land tenure, access to extension services, and organization membership as positive correlates for the adoption of many agricultural technologies in low- and middle-income countries. For agroforestry in Africa more specifically, Arslan et al.'s (2020) review highlighted information, tenure, social groups and distance to roads and markets as important adoption determinants. We cover these factors in our analysis, with a particular focus on extension and information, which we considered the most crucial leverage points in the context of the SustainSahel project and in the current policy discourse4 Peer learning and co-creation are integral parts of an agroecological transition and thus of special interest when analyzing agroforestry adoption dynamics (FAO, 2013). We categorized variables according to relevance and use in the regression models into information access, information flows and remaining farmer and household characteristics (Table 2). To better specify the models and provide relevant insights into potential action points for policy makers, we decided to focus the analysis on information access (through extension, mobility, media or training) and information flows (in terms of information on sustainable agricultural practices from public extension, NGOs, farmer organizations, the community or the media). The extensive list of remaining variables has been primarily implemented to control for other possible determinants from the existing adoption literature and to improve the accuracy and descriptive power of our models. We thus included further variables relating to the farmer and the farm household, such as education, financial conditions, market integration and shock perception (Table 2). The location dummies are not shown in the table, but nine control districts (“cercles”) were included in the estimation of the Mali models (Banamba, Kadiolo, Sikasso, Bla, Segou, Baraouli, Yelimane, Nioro du Sahel, Diema), with Koulikoro district as the base. For estimating the models based on the data from Fatick in Senegal, two districts (“arrondissements”) were used as location dummies (Niakhar and Tattaguine), while Diakhao district served as the base district.
Prior to use in this study, the raw data on all these variables were assessed for outliers. Observations were classed as outliers if they were greater than the 99th percentile for that particular variable. These outliers were then removed and replaced with a missing value. Subsequently, if missing values made up ~1% of the total observations, the missing values were replaced through imputation using selected variables as references. Only households that contained a full complement of observations were kept for the analysis. This resulted in the number of observations being slightly reduced from 2,138 to 2,070 for the Malian and from 720 to 695 for the Senegalese datasets.
2.4. Joint practice decision model
Consideration of joint adoption decisions is vital to identify combinations of practices that are appropriate to a farm's own context.5 It highlights that improved agricultural practices are seldom adopted in isolation, since the complementarity between particular sets of practices makes adopting them together advantageous for farmers. For instance, farmers in the Sahel face multiple production risks, which often leads to joint adoption of bundles of risk-reducing agricultural practices (Feder et al., 1985). Farmers' “bundle” selection can be considered an expected utility maximization problem (Dorfman, 1996), in that certain factors, such as practice compatibility, influence farmers' observed adoption decisions. For instance, some practices may be viewed as complements, while others are regarded as substitutes for each other. In our case, a farmer may for instance be more or less likely to uptake agroforestry if they already use a different practice. The use of shrubs and trees for soil fertility management is perhaps less prevalent if mineral fertilizers are widely used, while agroforestry use is possibly combined with the application of accumulated arboreal mulch to cultivated land. Kassie et al. (2015) found for instance some complementarity between farm diversification and mineral fertilizer use. The different practices reported in Table 1 are promoted to farmers frequently in combinations by governments and other stakeholders. In this regard, understanding the complex interrelated behavioral patterns underlying farmers' adoption decisions of these practices is expected to inform policies aimed at improving farmers' resilience to climate-related shocks.
We employed a Multivariate probit (MVP) model to account for the interdependent nature of farmers' adoption decisions of the eight different practices outlined in Table 1. That is, since farmers may choose to adopt a combination of practices jointly, failure to account for such interrelationships in the analysis of decision-making behavior could lead to biased estimates and wrong policy conclusions (Greene, 2008). Within an MVP framework, we assume farmers to be rational decision-makers and hence they are expected to choose a combination of agricultural practices that maximizes their expected profits. In that sense, they will only adopt practices either individually or jointly if the net benefit from adoption is positive (Kpadonou et al., 2017). Thus, we consider farmers' joint adoption decision of the following vector (P) of agricultural practices: agroforestry (A); mulching (M), intercropping (I), crop rotation (R), manure (N), improved crop varieties (C), mineral fertilizer (F) and synthetic pesticides (S) within an MVP framework. Let farmer i's latent (unobserved) net benefit from the adoption of any given agricultural practice p (where p∈P) be denoted by (). The estimated model implies that a particular farm household would consider adopting a given practice if the net benefit from adoption is positive. Hence, we relate farmers' observed adoption decisions with their latent net benefit in the following manner:
where yip is a farmer's observed adoption decision of agricultural practice (p); τi indicates district-level control variables, which accounts for regional variations in terms of infrastructure, institutional support and agroecology. We also control for a vector of household and plot level exogenous factors (Xi) concerning access to information and information flows, just like farmer and household characteristics that affect farmers' adoption decisions (see variables 1 to 37 reported in Table 2). Finally, the error term (ϵip) is assumed to be identically and independently distributed across farmers but not across the adoption equations of a given farmer (Teklewold et al., 2013; Kpadonou et al., 2017). That is, the error term (ϵip) of the adoption equations representing unobserved characteristics that affect farmers' choices of the different practices is assumed to have a multivariate normal distribution with the following covariance matrix (Ω) structure
The diagonal elements in the above matrix are normalized to 1 (for identification purposes), which allows the off-diagonal elements of the covariance matrix to be interpreted as the correlation between the stochastic components of the different practices. In particular, a positive (negative) and statistically significant coefficient in the off-diagonal covariance matrix suggests complementarity (substitutability) of the different agricultural practices. In our appilication, the corresponding χ2 test statistic indicated a good fit for the estimated models for Mali and Senegal respectively, both with p > χ2 = 0.000 (see Tables A1, A2 in the Appendix). The null hypothesis that all predicted regression coefficients are jointly equal to zero is strongly rejected in both models.
2.5. Agroforestry adoption models
While the MVP framework described above considers farmers' joint adoption decision of agroforestry and the different practices outlined in Table 1 at the extensive margin (i.e. whether farmers have adopted the practices or not), it doesn't consider adoption at the intensive margin (i.e. the proportion of farmland under agroforestry). From a policy perspective, it is key to identify the major factors that lead to greater intensity of adoption. Therefore, in the next step, we examined drivers of agroforestry uptake at the intensive margin. However, our data shows that only about 50% of the farmers were aware of agroforestry, suggesting the presence of sample selection related to differences in exposure and awareness about the active use of agroforestry-based soil fertility management. As such, non-adoption may either reflect the fact that farmers were unaware of the practice and hence were unable to decide on its uptake, or they were aware and decided to not adopt. This is important since farmers' adoption decision of agroforestry may involve a sequence of steps and endogenous choices. In our setup, this involves learning about agroforestry and then deciding about its use together with the allocation of farmland. Therefore, to capture the sequential nature of farmers' adoption decisions for agroforestry practices, we employed a two-step sample-selection model.
Our two-stage estimation strategy permits agroforestry exposure vs. non-exposure and adoption vs. non-adoption to be generated by different selection processes. More specifically, the selection variable in the first stage captures whether farmers have heard of agroforestry or not, whereas the response variable in the second stage captures the extent to which farmers have actively implemented agroforestry for soil fertility and crop productivity management. Empirically, our estimation strategy involves modeling farmers' awareness of agroforestry via a probit model and then estimating parameters of the adoption intensity via a generalized linear model (GLM). As our response variable is defined as adoption proportion which is bounded between zero and unity, a GLM with a binomial family for the error distribution and a logit link was deemed to be more suitable compared to the standard Heckman model (Papke and Wooldridge, 1996).6 A GLM model with sample selection bias has been implemented by Miranda and Rabe-Hesketh (2006) in Stata through the ssm routine. This allows for consistent estimation of a joint model of the response and selection variables through maximum likelihood estimation. The decision of implementing agroforestry across the farm however hinges on a first-stage selection equation expressed as
Where Ti is a binary awareness variable (yes/no) for farmer i, τi indicates district level control variables and ϵ1i is the error term of the selection equation. Zi captures farmer and household characteristics, but not information (see variables 10 to 37 in Table 2), while Wi defines awareness-relevant information access variables (see variables 1 to 4 in Table 2). These variables include access to extension, ownership of a motorbike, ownership of TV or/and radio through which agroforestry information can be acquired and finally whether farmers have attended any form of training in the last 5 years. Based on the result from the MVP models and logic of innovation diffusion, we postulate that these variables are predominantly drivers of awareness.
The second stage GLM equation is specified as
where yi is the observed adoption extent by farmer i, is the first stage predicted probability of agroforestry awareness (see Eq. 3 above). Vi includes controls for adoption-relevant information sources and flows via different channels (see variables 5–9 in Table 2) and ϵ2i is the error term of the adoption equation. In the presence of non-zero correlation between ϵ1i and ϵ2i, a single-stage GLM regression based on equation (4) would yield biased results. In this case, the GLM model with sample selection (SSM model) provides consistent estimates for all parameters. In our study, the Likelihood Ratio Test produced by the estimated SSM models resulted in a χ2 statistic of 530.15 for the Mali model and 38.55 for the Senegal model, both with a corresponding p > χ2 = 0.000 (see Table 5). This provides strong evidence of issues relating to sample selection. Therefore, the coefficient estimates in the uncorrected GLM model for the extent of use of agroforestry (Model 2, Table 5 and Model 4, Table 6) can be considered biased. The SSM model (Model 3, Table 5 and Model 6, Table 6) is a more appropriate model, as the sample selection bias is factored into the estimation.
2.6. Disadoption model
A simple probit model was used to model disadoption of agroforestry. The sample was restricted to only include farms that had ever used agroforestry, so for the four sites in Mali, this meant a sample of 584 observations and correspondingly a sample of 298 observations for Fatick in Senegal. However, when the disadoption equation was calculated for Fatick there proved to be too few disadopters (13 out of the full sample of 695). This resulted in non-convergence of the probit model and therefore we omitted the disadoption results for Fatick.
3. Results
3.1. Joint adoption decisions
The models for both country samples returned significant Wald tests, indicating that the models provide a good fit to the data. Our results provide an indication that the adoption choice of a certain practice is likely to be related to the choices made regarding the adoption of other practices. Results reported in Table 3 show that agroforestry adoption is complementary to the adoption of mulch composting, intercropping, improved rotation, manure application and the use of improved varieties. That is, farmers who utilize agroforestry are more likely to use one or more of these practices. On the other hand, the coefficient for agroforestry and the use of pesticides indicates that the active use of trees and shrubs is associated with a lower likelihood of using pesticides. Moreover, for the Mali sites, we find that adopting intercropping and the use of manure or improved varieties seem to be significantly positively correlated. Conversely, the use of mineral fertilizer and intercropping returns a significant negative correlation. Lastly, the use of either pesticides or mineral fertilizer is seen to mutually reinforce the likelihood of adoption of one another.
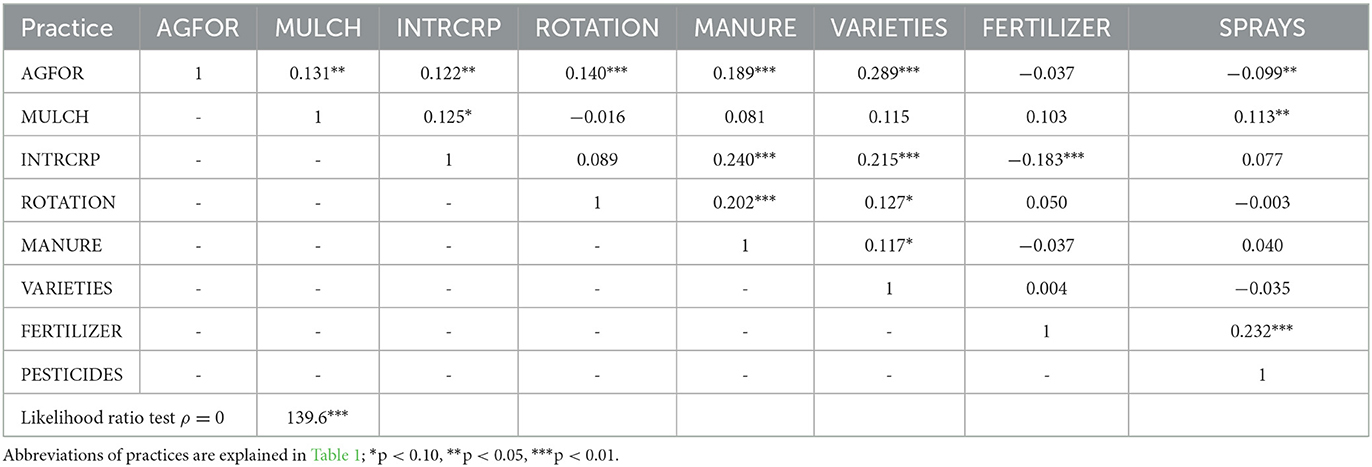
Table 3. Correlation matrix of the multivariate probit model for joint decisions on practice adoption in the four Mali sites.
Evidence for joint decision-making was also found for the Fatick site in Senegal, with some noticeable differences between the results in Mali and Senegal. We found no relationship between the decision to adopt agroforestry and any of the other practices apart from intercropping. However, some similarities can be found, with the decisions to use mulch composting and intercropping appearing to be correlated in both samples (Table 4). Moreover, as was seen for the sites in Mali, the decision to implement the use of mineral fertilizers and synthetic pesticides could be regarded as complementary. Interestingly, in the Senegalese case, the decision for adoption of mulch composting appears to rather substitute for the use of manure, mineral fertilizer or synthetic pesticides.
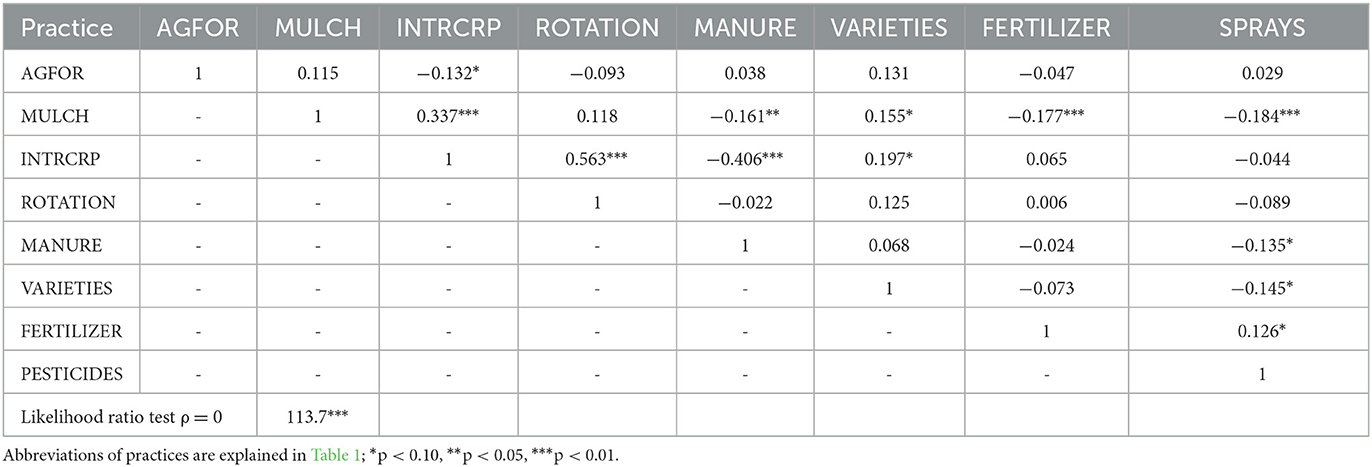
Table 4. Correlation matrix of the multivariate probit model for joint decisions on practice adoption at the Fatick site, Senegal.
In general, access to extension agents, ownership of motorized transport, ownership of a TV or radio and receipt of training in the preceding 5 years had no important associations with the use of any of the eight practices (Tables A1, A2 in the Appendix). These four variables were subsequently used as instruments for awareness in the sample selection models. Receiving information on sustainable agricultural practices from community exchange was consistently seen to have significant positive associations with the use decision of all agroecological practices for both countries. Information from government or NGO extension services was also consistently associated with a greater likelihood of practice adoption. It should be noted that MVP models made no sample selection correction as the estimations focus only on joint decisions.
3.2. Agroforestry awareness and adoption extent across the Mali sites
All three models used to understand awareness and adoption extent of agroforestry, as explained in Section 2.5, had significant Wald tests, which indicates that the models provide a good fit to the data. As explained in Section 2.5, the analysis is affected by sample selection bias. Therefore, the results described are mainly those of Model 3 in Table 5.
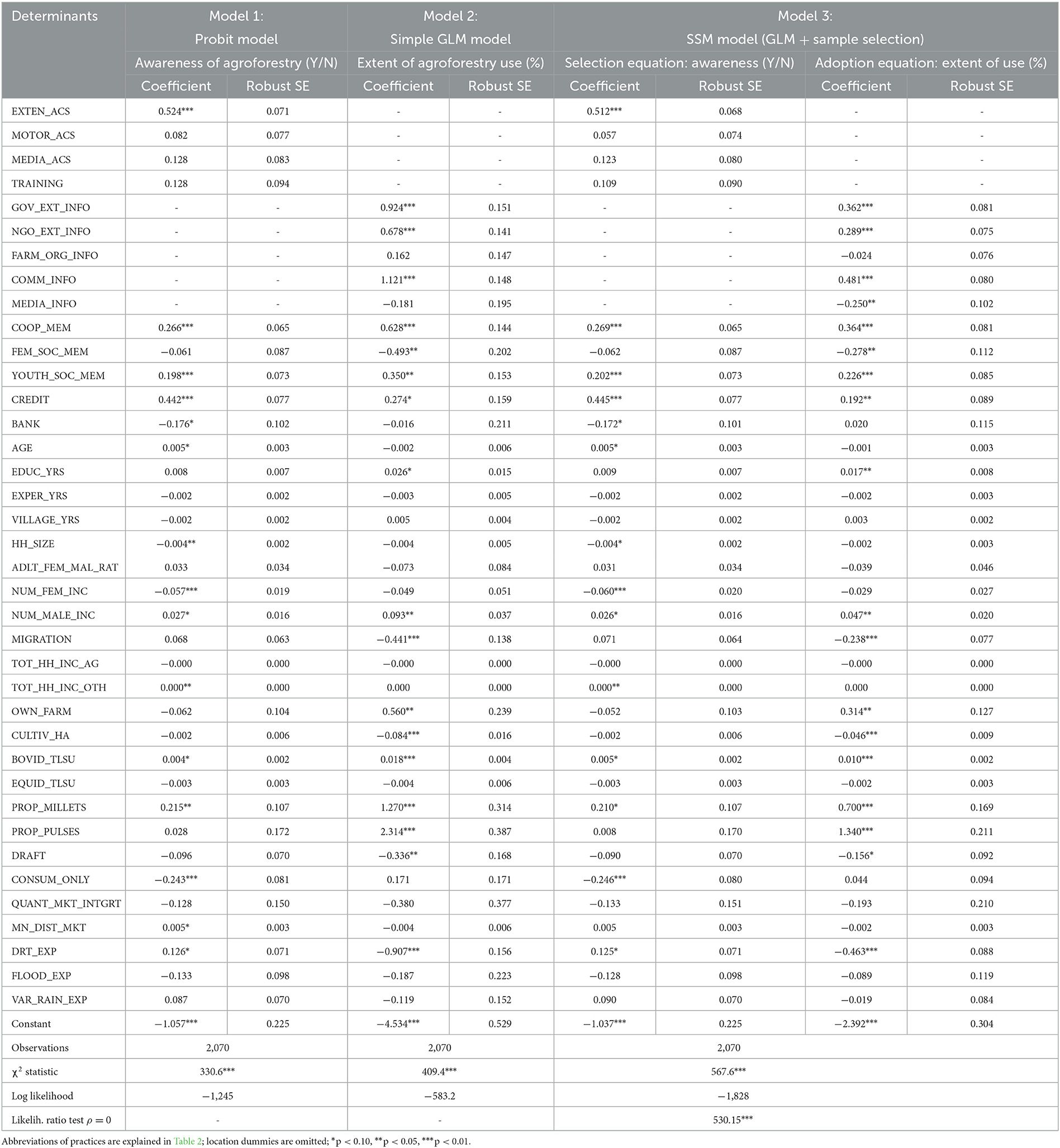
Table 5. Maximum likelihood estimates of models explaining agroforestry awareness and adoption across the four Mali sites, with and without sample selection.
The magnitude of coefficients, signs and significance levels for the determinant variables in both the awareness probit model (Model 1, Table 5) and the selection equation of the SSM model (Model 3, Table 5) were found to be very similar. The estimates however show some minor differences, which is due to the fact that, in Model 3, both the selection and outcome equation are estimated using the full maximum likelihood method. The only difference observed between Model 1 and the Model 3 selection equation was for the distance to market, with a greater distance being slightly positively significant in Model 1 (p = 0.088 in Model 1 vs. 0.102 in the selection equation of Model 3). Access to extension services is positive and highly significant across both awareness estimations. Farm cooperative membership and credit are also significantly correlated with awareness of agroforestry (p = 0.000). Having a household member taking part in a youth association was also associated with a greater likelihood of awareness in both models (p = 0.006 respectively). Growing millet over a greater proportion of farmland and having higher off-farm income was related to a greater likelihood of awareness (p = 0.051 and 0.013 respectively). Conversely, a higher number of females who have a regular income and a larger household size was found to be associated with a lower likelihood of awareness (p = 0.002 and 0.057 respectively). Furthermore, if a farm is a subsistence farm, the model indicated a lower likelihood of agroforestry awareness (p = 0.002).
When the adoption intensity equations in Model 2 and Model 3 are compared, we see some large differences in the magnitude of the coefficients. This is due to the fact that the SSM model takes the sample selection bias into account. For instance, obtaining information on sustainable practices from government extension, NGO extension and community exchange are highly significant in both models but the magnitude of coefficients are approximately half to one-third in the SSM model. Accessing sustainability information through the media is found to be significantly negatively related to adoption extent only in the SSM model (p = 0.015). Being a member of a farmer cooperative or having a youth association member in the household are found to be also highly positively related to adoption extent. However, having a household member who is a member of a female organization was seen to be negatively associated with adoption extent (p = 0.013). Furthermore, having household members migrate, having experienced drought on the farm and having a larger cultivated area were all found to be significantly negatively related to agroforestry adoption extent (p = 0.002, 0.000 and 0.000 respectively). However, devoting a greater proportion of the farm to the production of millets or to pulses was highly significantly related to adoption extent (p = 0.000 for both). Having access to credit, a greater number of years of education, a larger number of male income earners and having ownership of the farm were all found to have a positive and significant relationship with agroforestry adoption below the 5% significance level.
These models were tested with and without the inclusion of data from the region of Sikasso. This was due to the difference in climate and ecosystem compared with the other regions. The results were robust for the two different samples, with only the results for credit access and farm ownership changing, becoming insignificant for adoption extent. In the full SSM presented in Table 5, both districts (Kadiolo and Sikasso) from the Sikasso site are included within the list of location control variables. These variables capture some of the geographic and climactic differences, and therefore, analysis of all four Malian regions in conjunction is not drastically affected by uncaptured local differences.
3.3. Agroforestry awareness and adoption extent in Fatick, Senegal
When modeling awareness and extent of agroforestry adoption in Senegal, there was a great degree of similarity with the results obtained for Mali, but also some clear differences. All three Senegalese models had significant Wald tests, which again show that these models fit the data well. Due to the presence of sample selection bias, the SSM model (Model 6, Table 6) is thus the focus of the following paragraphs.
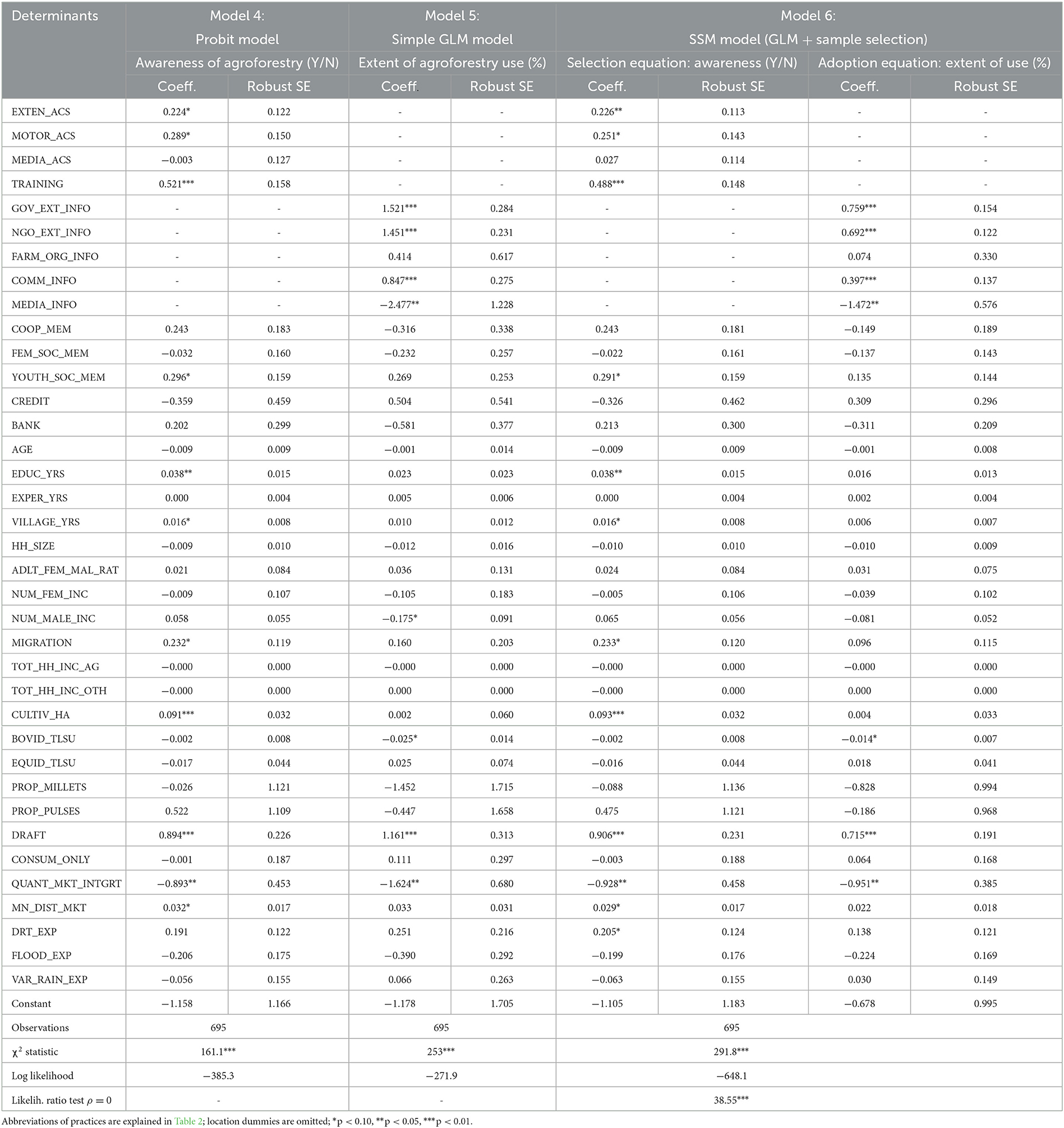
Table 6. Model estimates for agroforestry awareness and adoption in Fatick, Senegal, with and without sample selection.
In terms of the probit and selection equation for awareness of agroforestry (Models 4 and 6, Table 6), again both are very similar with only some minor differences associated with the different estimation techniques, discussed in the previous section. There are also relatively fewer significant coefficients for the Senegalese model compared to that of Mali, which in part is due to the fact the sample size is more limited. Extension access in Senegal appears to be marginally significant and positive for awareness, whilst having attended training in the last 5 years is highly significant and positively associated with awareness of agroforestry. We find also that having attained a higher level of education and also owning a greater amount of farmland is positively associated with awareness (p = 0.014 and 0.004 respectively). Moreover, ownership of draft animals for cultivation is seen to relate positively to awareness whilst a greater degree of market orientation was found to be negatively associated with agroforestry awareness (p = 0.000 and 0.043 respectively). Youth society membership, a greater number of years living in the same village, family migration, a greater distance from the local market and experience of drought were all minorly positively associated with agroforestry awareness in Senegal.
The differences between the GLM and SSM for the extent of agroforestry adoption are more obvious, comparing Model 5 and Model 6 in Table 6, with the magnitude of coefficients tending to be approximately half once sample selection is corrected for. As was seen in Mali, gaining information on sustainable practices from government extension, NGO extension and community exchange were found to have a highly positive and highly significant relationship with the extent of agroforestry adoption. Learning about sustainable agricultural practices using these sources is therefore linked with a greater area of adoption relative to farm size. Conversely, hearing about sustainable agricultural practices from media sources is found to correlate negatively with the extent of agroforestry adoption (p = 0.011). Like in the awareness and selection equations of Model 4 and 6, ownership of draft animals is positively correlated and higher degrees of market orientation is negatively correlated with the extent of agroforestry adoption (p = 0.000 and 0.013). Lastly, having a greater number of cattle, sheep or goats (BOVID_TLSU) was minorly significantly related to a lower degree of agroforestry adoption. District dummies not shown in Table 6 captured some of the effects of different geographies, climates and cultures that are not explicitly included in these models.
3.4. Agroforestry disadoption across the Mali sites
The last model examined the agroforestry disadoption decisions of farmers. Firstly, having received sustainable practice information from community exchange predicted a significantly reduced likelihood of disadoption (p = 0.010). Additionally, farmers with more years of education were seen to be less likely to stop using agroforestry (p = 0.004). On the other hand, receiving sustainability information from a farm organization or having a member of the household take part in a female association was found to be correlated with a significantly larger chance of disadoption being observed (p = 0.034 and 0.003). Furthermore, having a member of the family take part in a youth association, a higher number of females or males with a regular income, owning draft animals and having experienced flooding on the farm were all significantly related to a lower chance of disadoption. Conversely, a higher ratio of females to males in the household, having experienced migration and having experienced drought were all linked with a greater likelihood below the 10% significance level (Table 7).
4. Discussion
In agricultural innovation research, the concept of adoption is sometimes deemed inappropriate for its lack of temporal and spatial complexity (Douthwaite et al., 2003; Glover et al., 2019), so qualitative approaches are often suggested as the preferable method of analysis. While these approaches are key to an in-depth understanding of innovation processes, it is more difficult to obtain representative results and detect relationships in multivariate data. Precision and nuance have been added to the study of adoption through econometric estimation techniques that concentrate on evaluating the intensity of adoption (Arslan et al., 2020). Moreover, technological change involving multiple components can be somewhat captured by econometric models that analyse joint adoption decisions (Kassie et al., 2015; Glover et al., 2019). The present study tried to address shortcomings in the quantitative analysis of the adoption of agricultural practices by incorporating two perspectives into one analytical framework: decision-making on the joint use of eight agricultural practices as well as adoption dynamics covering awareness, intensity of use and abandonment of agroforestry. Thereby, we aim to understand the interaction effects of agroforestry with other practices together with its diffusion. While our study goes well beyond a binary adoption decision, it cannot shed full light on the experimentation and reconfiguration processes that happen along the way. This would require more specific tracing of innovation trajectories (e.g., Schut et al., 2020) as well as the collection of representative panel data sets, which, whilst outside the scope of the analysis, is a key area for future research on this topic.
Whereas panel data could more completely uncover the dynamics of farmers' choices to adopt, adapt and possibly give up agroforestry-based soil fertility management, a shortcoming of our study is the reliance on cross-sectional data. This implies that interpretation of results is based on one time period and correlations rather than causal relations. The dataset nevertheless provides a comprehensive picture of the status-quo of the knowledge about and the use of shrubs and trees for improving soil fertility and crop productivity across different contexts in Senegal and Mali.
Overall, our results are in line with the recent literature on adoption, stressing the importance of public as well as NGO advisory services and of farmer networks and community exchange for innovation diffusion (Arslan et al., 2020; Pannell and Zilberman, 2020; Piñeiro et al., 2020; Ruzzante et al., 2021). The negative association between membership in women's groups and the intensity of agroforestry adoption seems counterintuitive, but is likely linked to the fact that these groups have been more focused on issues outside of agriculture and have limited influence on adoption decisions (Ragasa, 2012). By distinguishing between access to information, different information flows and social groups, we can better understand the diverse drivers of awareness, adoption and disadoption. It becomes apparent that agroforestry promotion is a multi-factorial challenge. For both the Malian and Senegalese contexts, our findings imply that investments in public or NGO extension support the dissemination of agroforestry-based soil fertility management. External advice needs to be coupled with promoting farmer-to-farmer exchanges in the community as well as efforts to encourage and nurture agriculturally orientated group participation. It is well known that the scaling of agroecological approaches has been shown to rely on horizontal peer learning. In India, the Andhra Pradesh Natural Farming programme plans to roll out agroecology to 6 million farmers in the state through a combination of farmer-based extension, government and NGO support and women groups (Bharucha et al., 2020). Participatory extension programmes are widely used to promote agricultural innovations and are found to be effective if properly implemented (Knook et al., 2020).
Our results capturing information flows and social networks are similar in the models estimated for the four Mali sites and for Fatick, which underscores their general validity. Meta-analysis performed by Ruzzante et al. (2021) highlights extension advice and organized interests as crucial determinants for the uptake of natural resource management practices. Arslan et al. (2020) have shown in their review that these variables are often positive and significant in agroforestry adoption studies in the SSA context. They also found that land tenure and distance to markets are key drivers. Our results, just like those of Ruzzante et al. (2021), confirm the first, but not the latter. In fact, proximity to markets may increase farmers' use of external inputs and thus decrease agroforestry-based soil fertility management. This could also be linked with the fact that better market access may predispose a farm toward more commercially-oriented monocropping. Distance to markets is also difficult to influence for decision-makers, while access to credit along with extension is one of the most likely variables to be shaped by policy (Ruzzante et al., 2021). Implementing agroforestry measures on the farm often requires medium to long-term investment decisions. Tenure and credit are considered key for farmers to invest into agroforestry (Ali et al., 2011). In this regard, evidence from Mali suggests that improved credit access should be combined with fostering knowledge and learning if these agroecological practices are to be scaled. In the case of Senegal credit access is also a positive, but not significant, driver of adoption.
It is not only a combination of adoption drivers that shape the diffusion of agroforestry in the Sahel. The combined decision-making on adoption also plays a part. Kassie et al. (2015) found that farmers located in eastern and southern Africa often take joint decision on the adoption of a set of sustainable intensification practices. In the Mali model, we also ascertained that agroforestry adoption is accompanied by the uptake of other sustainable intensification practices, such as mulching, rotations, use of manure and improved varieties, but by a reduction in synthetic pesticides. No clear pattern of joint decisions emerges from the results from Fatick, Senegal. This might be due to the higher overall level of commercialization there and potentially due to a more limited geographical scope. All in all, agroforestry seems either complementary or relatively unrelated to other practices, except in the case of synthetic pesticides, where the relationship is clearly negative.
Our results provide key insights for future policy-making on the promotion of sustainable agricultural practices. Namely, they imply that it is essential to strengthen the capacity of public and NGO-based advisory systems. Importantly, these need to fully engage with local knowledge networks. Improved informations flows on sustainable agriculture from these sources are key to supporting the transition to more widespread agroforestry-based soil fertility management. Furthermore, solutions for agroforestry microfinance are needed to back up knowledge gains with the financial means for implementation. Instead of disseminating stand-alone solutions, our results suggest that a package of agroforestry, mulching, rotations, use of manure, improved varieties and intercropping can be jointly promoted.
5. Conclusions
Agroforestry-based crop management is an important strategy for ecological intensification in the semi-arid Sahel and evidence is needed on dissemination strategies. To obtain insights on innovation drivers in agroforestry, awareness, adoption intensity, disadoption and joint decisions should be considered. Our study illustrates how extension access, together with participation in training, enhance awareness of agroforestry in the case of Fatick. Information on sustainable agricultural practices provided by public extension services and private NGOs, alongside community information flows and membership in social networks, are key drivers of the extent of agroforestry use. For disadoption, which we could only analyse with the Mali data, information exchange on sustainable agricultural practices in the community appears an important lever to encourage continued use of the practice. Youth and cooperative membership appear to have a favorable relationship with agroforestry use, albeit not significant for Fatick, Senegal. In terms of joint decisions, we conclude that agroforestry uptake is generally complementary or unrelated to the use of other practices, with some indications of substitution, in that farmers who use synthetic pesticides are less likely to make use of agroforestry and vice versa. To support the transition to more widespread agroforestry-based soil fertility management, it is essential to strengthen advisory systems that fully engage with local knowledge networks. For capacity development purposes, agroforestry can be packaged together with most other practices found in the study area.
Data availability statement
The raw data supporting the conclusions of this article will be made available by the authors, without undue reservation.
Author contributions
CG: coordination of manuscript, conceptualization of analysis, supervision, and write-up. CR: main analysis and drafting methods sections. AB: data collection, contributions to analysis, and feedback manuscript draft. TW: contributions to analysis and editing manuscript. TA: data collection and feedback on draft manuscript. HC: overall project coordination, stakeholder consultation, and feedback on draft manuscript. All authors contributed to the article and approved the submitted version.
Funding
The research has been funded under the Horizon 2020 Programme of the European Union (Project No: 861974) and has received data through the Norway-funded Climate Smart Agriculture Technologies (CSAT) project.
Acknowledgments
The authors would like to acknowledge the financial support for this research under the EU-funded Horizon 2020 SustainSahel project and under the Norway-funded Climate Smart Agriculture Technologies (CSAT) project, the latter providing the full dataset for the adoption analysis in Mali.
Conflict of interest
The authors declare that the research was conducted in the absence of any commercial or financial relationships that could be construed as a potential conflict of interest.
Publisher's note
All claims expressed in this article are solely those of the authors and do not necessarily represent those of their affiliated organizations, or those of the publisher, the editors and the reviewers. Any product that may be evaluated in this article, or claim that may be made by its manufacturer, is not guaranteed or endorsed by the publisher.
Supplementary material
The Supplementary Material for this article can be found online at: https://www.frontiersin.org/articles/10.3389/fsufs.2023.1042551/full#supplementary-material
Footnotes
1. ^More details on data collection can be found in Deliverable 3.1 of the Horizon 2020 SustainSahel project: https://cordis.europa.eu/project/id/861974.
2. ^As discussed in the introduction, adoption of “Agroforestry” involves the active management of shrubs and trees for improved soil fertility and crop productivity as well as keeping trees, including economic and fruit trees, on the field for natural regeneration, an activity commonly supported by NGOs, development projects and government initiatives to engage farmers in combating desertification in the Sahel.
3. ^For example the Regreening Africa Project, run by World Vision and the World Agroforestry Center, is active in Fatick: https://regreeningafrica.org/.
4. ^For more information, please see Deliverable 2.1 of the Horizon 2020 SustainSahel project: https://cordis.europa.eu/project/id/861974.
5. ^If practices are only considered in isolation, the analysis may fail to account for the fact that adoption decisions are often simultaneous decisions (Feder et al., 1985). Omission of such interrelations may therefore lead to biased estimates (Dorfman, 1996; Bekele and Drake, 2003; Kpadonou et al., 2017).
6. ^The standard Heckman model uses OLS regression in the second stage.
References
Ali, D. A., Dercon, S., and Gautam, M. (2011). Property rights in a very poor country: tenure insecurity and investment in Ethiopia. Agric. Econ. 42, 75–86. doi: 10.1111/j.1574-0862.2010.00482.x
Arslan, A., Floress, K., Lamanna, C., Lipper, L., Asfaw, S., and Rosenstock, T. (2020). “The adoption of improved agricultural technologies—A meta-analysis for Africa,” in IFAD Research Series Issue 63. Rome: International Fund for Agricultural Development. Available online at: https://www.ifad.org/en/web/knowledge/publication/asset/42041675 (accessed August 28, 2020).
Asfaw, S., Di Battista, F., and Lipper, L. (2016). Agricultural technology adoption under climate change in the sahel: micro-evidence from Niger. J. Afr. Econ. 25, 637–669. doi: 10.1093/jae/ejw005
Aune, J. B., and Bationo, A. (2008). Agricultural intensification in the Sahel – the ladder approach. Agric. Syst. 98, 119–125. doi: 10.1016/j.agsy.2008.05.002
Barrett, C. B., Reardon, T., Swinnen, J., and Zilberman, D. (2020). Agri-food value chain revolutions in low- and middle-income countries. J. Econ. Lit.
Bationo, A., Hartemink, A., Lungu, O., Naimi, M., Okoth, P., Smaling, E., et al. (2012). “Knowing the African Soils to Improve Fertilizer Recommendations,” in Improving Soil Fertility Recommendations in Africa using the Decision Support System for Agrotechnology Transfer (DSSAT), Kihara, J., Fatondji, D., Jones, J. W., Hoogenboom, G., Tabo, R., and Bationo, A. (Dordrecht: Springer Netherlands) p. 19–42. doi: 10.1007/978-94-007-2960-5_3
Bayala, J., Sanou, J., Teklehaimanot, Z., Ouedraogo, S. J., Kalinganire, A., Coe, R., et al. (2015). Advances in knowledge of processes in soil–tree–crop interactions in parkland systems in the West African Sahel: a review. Agric. Ecosyst. Environ. 205, 25–35. doi: 10.1016/j.agee.2015.02.018
Bekele, W., and Drake, L. (2003). Soil and water conservation decision behavior of subsistence farmers in the Eastern Highlands of Ethiopia: a case study of the Hunde-Lafto area. Ecol. Econ. 46, 437–451. doi: 10.1016/S0921-8009(03)00166-6
Bharucha, Z. P., Mitjans, S. B., and Pretty, J. (2020). Towards redesign at scale through zero budget natural farming in Andhra Pradesh, India. Int. J. Agric. Sustain. 18, 1–20. doi: 10.1080/14735903.2019.1694465
Bogie, N. A., Bayala, R., Diedhiou, I., Conklin, M. H., Fogel, M. L., Dick, R. P., et al. (2018). Hydraulic redistribution by native sahelian shrubs: bioirrigation to resist in-season drought. Front. Environ. Sci. 6, 98. doi: 10.3389/fenvs.2018.00098
Bright, M. B. H., Diedhiou, I., Bayala, R., Assigbetse, K., Chapuis-Lardy, L., Ndour, Y., et al. (2017). Long-term Piliostigma reticulatum intercropping in the Sahel: crop productivity, carbon sequestration, nutrient cycling, and soil quality. Agric. Ecosyst. Environ. 242, 9–22. doi: 10.1016/j.agee.2017.03.007
Cassman, K. G., and Grassini, P. (2020). A global perspective on sustainable intensification research. Nat. Sustain. 3, 262–268. doi: 10.1038/s41893-020-0507-8
Catacutan, D., Finlayson, R., Perdana, A., Lusiana, B., Gassner, A., Leimona, B., et al. (2018). The ASEAN Guidelines for Agroforestry Development. Jakarta: Association of South East Asian Nations Secretariat.
Dorfman, J. H. (1996). Modeling multiple adoption decisions in a joint framework. Am. J. Agric. Econ. 78, 547–557. doi: 10.2307/1243273
Dossa, E. L., Diedhiou, I., Khouma, M., Sene, M., Lufafa, A., Kizito, F., et al. (2012). Crop Productivity and Nutrient Dynamics in a Shrub (Guiera senegalensis)–based farming system of the Sahel. Agron. J. 104, 1255–1264. doi: 10.2134/agronj2011.0399
Douthwaite, B., Kuby, T., van de Fliert, E., and Schulz, S. (2003). Impact pathway evaluation: an approach for achieving and attributing impact in complex systems. Agric. Syst. 78, 243–265. doi: 10.1016/S0308-521X(03)00128-8
FAO (2013). The 10 Elements of Agroecology. Rome: Food and Agriculture Organization of the United Nations.
FAO (2016). Climate Change, Agriculture and Food Security. Rome: Food and Agriculture Organization of the United Nations.
FAO (2018). The Future of Food and Agriculture–Alternative Pathways to 2050. Rome: Food and Agriculture Organization of the United Nations.
Feder, G., Just, R. E., and Zilberman, D. (1985). Adoption of agricultural innovations in developing countries: a survey. Econ. Dev. Cult. Change. 33, 255–298. doi: 10.1086/451461
Gerten, D., Heck, V., Jägermeyr, J., Bodirsky, B. L., Fetzer, I., Jalava, M., et al. (2020). Feeding ten billion people is possible within four terrestrial planetary boundaries. Nat. Sustain. 3, 200–208. doi: 10.1038/s41893-019-0465-1
Glover, D., Sumberg, J., Ton, G., Andersson, J., and Badstue, L. (2019). Rethinking technological change in smallholder agriculture. Outlook Agric. 48, 169–180. doi: 10.1177/0030727019864978
Greene, W. (2008). Functional forms for the negative binomial model for count data. Econ. Lett. 99, 585–590. doi: 10.1016/j.econlet.2007.10.015
HLPE (2019). HLPE Report #14–Agroecological and Other Innovative Approaches for Sustainable Agriculture and Food Systems that Enhance Food Security and Nutrition. Rome: A report by the High Level Panel of Experts on Food Security and Nutrition of the Committee on World Food Security.
Kassie, M., Teklewold, H., Jaleta, M., Marenya, P., and Erenstein, O. (2015). Understanding the adoption of a portfolio of sustainable intensification practices in eastern and southern Africa. Land Use Policy. 42, 400–411. doi: 10.1016/j.landusepol.2014.08.016
Kay, S., Graves, A., Palma, J. H. N., Moreno, G., Roces-Díaz, J. V., Aviron, S., et al. (2019). Agroforestry is paying off – Economic evaluation of ecosystem services in European landscapes with and without agroforestry systems. Ecosyst. Serv. 36, 100896. doi: 10.1016/j.ecoser.2019.100896
Knook, J., Eory, V., Brander, M., and Moran, D. (2020). The evaluation of a participatory extension programme focused on climate friendly farming. J. Rural Stud. 76, 40–48. doi: 10.1016/j.jrurstud.2020.03.010
Kpadonou, R. A. B., Owiyo, T., Barbier, B., Denton, F., Rutabingwa, F., and Kiema, A. (2017). Advancing climate-smart-agriculture in developing drylands: Joint analysis of the adoption of multiple on-farm soil and water conservation technologies in West African Sahel. Land Use Policy 61, 196–207. doi: 10.1016/j.landusepol.2016.10.050
Kuyah, S., Whitney, C. W., Jonsson, M., Sileshi, G. W., Öborn, I., Muthuri, C. W., et al. (2019). Agroforestry delivers a win-win solution for ecosystem services in sub-Saharan Africa. A meta-analysis. Agron. Sustain. Dev. 39, 47. doi: 10.1007/s13593-019-0589-8
Kuyper, T. W., and Struik, P. C. (2014). Epilogue: global food security, rhetoric, and the sustainable intensification debate. Curr. Opin. Environ. Sustain. 8, 71–79. doi: 10.1016/j.cosust.2014.09.004
Läderach, P., Ramirez-Villegas, J., Prager, S. D., Osorio, D., Krendelsberger, A., Zougmor,é, R. B., et al. (2021). The importance of food systems in a climate crisis for peace and security in the Sahel. Int. Rev. Red Cross 103, 995–1028. doi: 10.1017/S1816383122000170
Leroux, L., Faye, N. F., Jahel, C., Falconnier, G. N., Diouf, A. A., Ndao, B., et al. (2022). Exploring the agricultural landscape diversity-food security nexus: an analysis in two contrasted parklands of Central Senegal. Agric. Syst. 196, 103312. doi: 10.1016/j.agsy.2021.103312
Mbow, C., Smith, P., Skole, D., Duguma, L., and Bustamante, M. (2014). Achieving mitigation and adaptation to climate change through sustainable agroforestry practices in Africa. Curr. Opin. Environ. Sustain. 6, 8–14. doi: 10.1016/j.cosust.2013.09.002
Millennium Ecosystem Assessment (2005). Ecosystems and Human Wellbeing : Desertification Synthesis: a Report of the Millennium Ecosystem Assessment. Washington DC.: World Resources Institute.
Miller, D. C., Ordoñez, P. J., Brown, S. E., Forrest, S., Nava, N. J., Hughes, K., et al. (2020). The impacts of agroforestry on agricultural productivity, ecosystem services, and human well-being in low-and middle-income countries: an evidence and gap map. Campbell Syst. Rev. 16, e1066. doi: 10.1002/cl2.1066
Miranda, A., and Rabe-Hesketh, S. (2006). Maximum likelihood estimation of endogenous switching and sample selection models for binary, ordinal, and count variables. Stata J. 6, 285–308. doi: 10.1177/1536867X0600600301
Muchane, M. N., Sileshi, G. W., Gripenberg, S., Jonsson, M., Pumariño, L., and Barrios, E. (2020). Agroforestry boosts soil health in the humid and sub-humid tropics: a meta-analysis. Agric. Ecosyst. Environ. 295, 106899. doi: 10.1016/j.agee.2020.106899
Nair, P. K. R., and Garrity, D. (2012). Agroforestry–The Future of Global Land Use. Dordrecht: Springer. Available online at: https://link.springer.com/book/10.1007/978-94-007-4676-3 (accessed August 4, 2022).
Nord, A., Snapp, S., and Traore, B. (2022). Current knowledge on practices targeting soil fertility and agricultural land rehabilitation in the Sahel. A review. Agron. Sustain. Dev. 42, 79. doi: 10.1007/s13593-022-00808-1
Pannell, D., and Zilberman, D. (2020). Understanding adoption of innovations and behavior change to improve agricultural policy. Appl. Econ. Perspect. Policy. 42, 3–7. doi: 10.1002/aepp.13013
Papke, L. E., and Wooldridge, J. M. (1996). Econometric methods for fractional response variables with an application to 401(k) plan participation rates. J. Appl. Econom. 11, 619–632. doi: 10.1002/(SICI)1099-1255(199611)11:6<619::AID-JAE418>3.0.CO;2-1
Piñeiro, V., Arias, J., Dürr, J., Elverdin, P., Ibáñez, A. M., Kinengyere, A., et al. (2020). A scoping review on incentives for adoption of sustainable agricultural practices and their outcomes. Nat. Sustain. 3, 809–820. doi: 10.1038/s41893-020-00617-y
Pretty, J., Toulmin, C., and Williams, S. (2011). Sustainable intensification in African agriculture. Int. J. Agric. Sustain. 9, 5–24. doi: 10.3763/ijas.2010.0583
Ragasa, C. (2012). Gender and institutional dimensions of agricultural technology adoption: a review of literature and synthesis of 35 case studies. In: International Association of Agricultural Economists. Available online at: https://econpapers.repec.org/paper/agsiaae12/126747.htm (accessed October 27, 2022).
Rockström, J., Williams, J., Daily, G., Noble, A., Matthews, N., Gordon, L., et al. (2017). Sustainable intensification of agriculture for human prosperity and global sustainability. Ambio 46, 4–17. doi: 10.1007/s13280-016-0793-6
Ruzzante, S., Labarta, R., and Bilton, A. (2021). Adoption of agricultural technology in the developing world: a meta-analysis of the empirical literature. World Dev. 146, 105599. doi: 10.1016/j.worlddev.2021.105599
Schut, M., Leeuwis, C., and Thiele, G. (2020). Science of Scaling: understanding and guiding the scaling of innovation for societal outcomes. Agric. Syst. 184, 102908. doi: 10.1016/j.agsy.2020.102908
Sinare, H., and Gordon, L. J. (2015). Ecosystem services from woody vegetation on agricultural lands in Sudano-Sahelian West Africa. Agric. Ecosyst. Environ. 200, 186–199. doi: 10.1016/j.agee.2014.11.009
Teklewold, H., Kassie, M., and Shiferaw, B. (2013). Adoption of multiple sustainable agricultural practices in rural Ethiopia. J. Agric. Econ. 64, 597–623. doi: 10.1111/1477-9552.12011
Keywords: awareness, disadoption, Senegal, West Africa, Mali, multivariate probit (MVP) model, sample selection (Heckman-type) models
Citation: Grovermann C, Rees C, Beye A, Wossen T, Abdoulaye T and Cicek H (2023) Uptake of agroforestry-based crop management in the semi-arid Sahel – Analysis of joint decisions and adoption determinants. Front. Sustain. Food Syst. 7:1042551. doi: 10.3389/fsufs.2023.1042551
Received: 12 September 2022; Accepted: 06 March 2023;
Published: 23 March 2023.
Edited by:
Maria Conceição Caldeira, University of Lisbon, PortugalReviewed by:
Maria Raimondo, University of Naples Federico II, ItalyFrancisco Javier Rodríguez Rigueiro, University of Santiago de Compostela, Spain
Ana Catarina Sequeira, Centro de Ecologia Aplicada “Prof. Baeta Neves” (CEABN), Portugal
Copyright © 2023 Grovermann, Rees, Beye, Wossen, Abdoulaye and Cicek. This is an open-access article distributed under the terms of the Creative Commons Attribution License (CC BY). The use, distribution or reproduction in other forums is permitted, provided the original author(s) and the copyright owner(s) are credited and that the original publication in this journal is cited, in accordance with accepted academic practice. No use, distribution or reproduction is permitted which does not comply with these terms.
*Correspondence: Christian Grovermann, Y2hyaXN0aWFuLmdyb3Zlcm1hbm5AZmlibC5vcmc=