- 1Soil and Crop Sciences Section, School of Integrative Plant Science, Cornell University, Ithaca, NY, United States
- 2Department of Animal Science, Cornell University, Ithaca, NY, United States
- 3Sustainable Agricultural Systems Lab, Agricultural Research Service, U.S. Department of Agriculture, Beltsville, MD, United States
Volatile feed costs and extreme weather events are contributing to greater economic risk and precarity throughout much of the United States dairy industry. These challenges have prompted dairy farmers to seek ways to reduce feed imports without compromising milk production. For organic dairy farmers, the need to produce more homegrown forage is exacerbated by the high cost and limited supply of organic feed. Integrating winter cereals for forage as part of a double-cropping system is a potential solution, but increasing the amount of forage in dairy cow rations can reduce milk production if the forages are not managed for optimal quality. Organically managed field experiments in Maryland (MD) and New York (NY) were conducted to address two primary objectives: (1) determine the yield and quality of winter cereals—four cultivars each for barley (Hordeum vulgare L.), cereal rye (Secale cereale L.), and triticale (× Triticosecale Wittm. ex A. Camus.)—grown as forage and harvested at different crop growth stages, and (2) evaluate the trade-offs between yield and quality in relation to winter cereal phenology and harvest date. Mean yield at a commonly harvested growth stage, swollen boot (Zadoks 45), was 1.3, 2.2, and 2.2 Mg ha−1 in MD and 1.8, 2.5, and 2.9 Mg ha−1 in NY for barley, cereal rye, and triticale, respectively. Mean relative forage quality (RFQ) at the same growth stage was 180, 158, and 163 in MD and 179, 156, and 157 in NY for the three species. Overall, cereal rye reached swollen boot stage the earliest, barley produced the highest RFQ and retained high quality the longest, and cereal rye and triticale produced the highest yields. Based on these results, farmers should consider barley cultivars if quality is the priority and winter-hardiness is not a concern; cereal rye cultivars if an early harvest is most important; and triticale cultivars if greater harvest schedule flexibility would be most valuable. These findings can be used to better meet the needs of dairy farmers, enhance double-cropping system performance, and improve the synchronization of harvest timing with the specific needs of lactating dairy cows, dry cows, heifers, and calves.
1. Introduction
Over the past 30 years, the dairy sector in the United States (US) has experienced a 16-fold increase in consolidation (MacDonald et al., 2020). This transformational shift toward fewer, yet much larger, dairy farms is also characterized by a general movement of the industry toward the western region of the US (von Keyserlingk et al., 2013). Dairy farms in the northeastern US, including Maryland (MD) and New York (NY), are smaller (as measured by mean number of animals per farm) and tend to produce more feed grain and forage on-farm than dairies in the west (von Keyserlingk et al., 2013). With fluctuating feed costs and milk prices, turning a profit can be elusive for many dairy farmers, especially while using environmentally sustainable soil and crop management practices and providing a high standard of animal welfare (von Keyserlingk et al., 2009).
Operating an organic dairy can be more profitable than conventional management. The higher prices received for organic milk can outweigh the greater production costs (e.g., inputs and labor) on organic farms, although farm size plays an important role (MacDonald et al., 2020). In 2016, for instance, organic dairy farmers who managed 50–199 cows received gross returns that were more than double those received by their conventional counterparts, and the difference was similar for farmers who managed 10–49 cows (MacDonald et al., 2020). Still, many of these relatively small-scale organic dairy farmers lost money (per unit of milk sold), which underscores the need to provide these farmers with technical support that might help them increase on-farm forage production and stay in business.
On dairy farms of all sizes, manure management and limiting non-point source pollution can also be a challenge. Winter cereals, such as cereal rye (Secale cereale L.), have been promoted for use as cover crops to deliver a range of ecosystem services, including improved soil and water quality through the reduction of soil erosion, nitrate leaching, and phosphorus runoff (Snapp et al., 2005; Blanco-Canqui et al., 2015; Daryanto et al., 2018; Thapa et al., 2018; McClelland et al., 2021; Wood and Bowman, 2021). As an alternative to growing winter cereals as cover crops, the same winter-hardy species [e.g., barley (Hordeum vulgare L.), cereal rye, and triticale (× Triticosecale Wittm. ex A. Camus.)] can be planted in the fall and harvested in the spring as forage. When grown in tandem with a summer annual forage crop, such as sorghum [Sorghum bicolor (L.) Moench] or corn silage (Zea mays L.), on the same parcel of land and harvested in the same calendar year, the arrangement comprises a double-cropping system.
Compared with growing a summer annual forage crop alone, double-cropping with winter cereals can increase homegrown forage production (Brown, 2006; Heggenstaller et al., 2008, 2009; Fouli et al., 2012; Jemison et al., 2012; Ketterings et al., 2015), reduce the quantity and cost of imported feed (Kim et al., 2016; Veltman et al., 2018; Ranck et al., 2020), and increase feed inventory when it is typically low (Landry et al., 2019). Producing more homegrown forage can also be particularly valuable when regional extreme weather creates a forage shortage and emergency feed becomes a necessity (Ketterings et al., 2015). Winter cereals have more stable yields under drought conditions than corn, and double-cropping enhances diversification, which reduces production risk (Rotz et al., 2002). Double-cropping systems also provide additional opportunities to spread manure, which can be helpful for high density dairies or those with limited manure storage options. In these ways, double-cropping with winter cereals can improve environmental stewardship, productivity, and profitability.
Challenges to the practice of double-cropping still exist, however, with surveyed dairy farmers in NY reporting concerns about weather impacting both the timely establishment of winter cereal forages in the fall and timely harvest in the spring (Ketterings et al., 2015). In the same survey, more information about forage quality was among the highest ranked topics that farmers indicated would best support adoption or continued use of winter cereal forages (Ketterings et al., 2015). This is an important consideration as increasing the amount of forage in dairy cow rations can decrease milk production if the forages are not carefully managed for optimal quality. Dairy cattle performance is a function of nutrient intake and availability, nutrient concentration, digestibility, and metabolic efficiency (Cherney and Mertens, 1998). Harvest management is the single most critical component of dairy forage management because it controls both yield and forage quality (Cherney et al., 2020). Consequently, dairy farmers will benefit from region-specific information and guidance for precise, yet flexible, crop management that balances yield and quality with harvest timing synchronized to meet livestock nutritional demands. Lactating dairy cows, for example, require high forage quality, which is directly related to harvest timing for grasses. To this end, the objectives of our research were to (1) determine the yield and quality of winter cereals—four cultivars each for barley, cereal rye, and triticale—grown as forage when harvested at different crop growth stages, and (2) evaluate the trade-offs between yield and quality in relation to winter cereal phenology and harvest date.
2. Material and methods
2.1. Site description
Conducted during the winter cereal field season of fall 2014 to spring 2015, our experiment consists of two sites in the Northeast region of the US: Beltsville, MD (39° 1′ N, 76° 55′ W; USDA plant hardiness zone 7a), and Aurora, NY (42° 43′ 54.4" N, 76° 39′ 02.6" W; USDA plant hardiness zone 6a) (United States Department of Agriculture, 2012). At the MD field site, the primary soil type is a Codorus-Hatboro silt loam (fine-loamy, mixed, active, mesic, Fluvaquentic Dystrudepts and Endoaquepts). Soil type at the NY field site is a moderately well-drained, calcareous Lima silt loam (fine-loamy, mixed, semiactive, mesic Oxyaquic Hapludalfs), with partial tile drainage. Soil pH and soil organic matter were lower in MD at 5.7 and 1.7%, respectively, compared with 7.5 and 3.0% in NY.
The non-irrigated field sites in both MD and NY were under long-term conventional management prior to establishing our experiment, but no conventional management practices, such as the application of synthetic herbicides or fertilizers, were used at either site in the establishment year of 2014. Wheat (Triticum aestivum L.) and sorghum were the preceding crops in MD and NY, respectively. The experiment was managed organically at both sites.
Precipitation diverged from the long-term mean at both sites throughout much of 2015, but the variability was particularly notable beyond day 120 (May 1) in NY, fluctuating from dry periods of little or no precipitation to periods of very heavy rainfall (Figure 1). Compared with the long-term mean temperature, daily mean temperature was generally cooler prior to the sampling period and warmer during the sampling period in MD and NY.
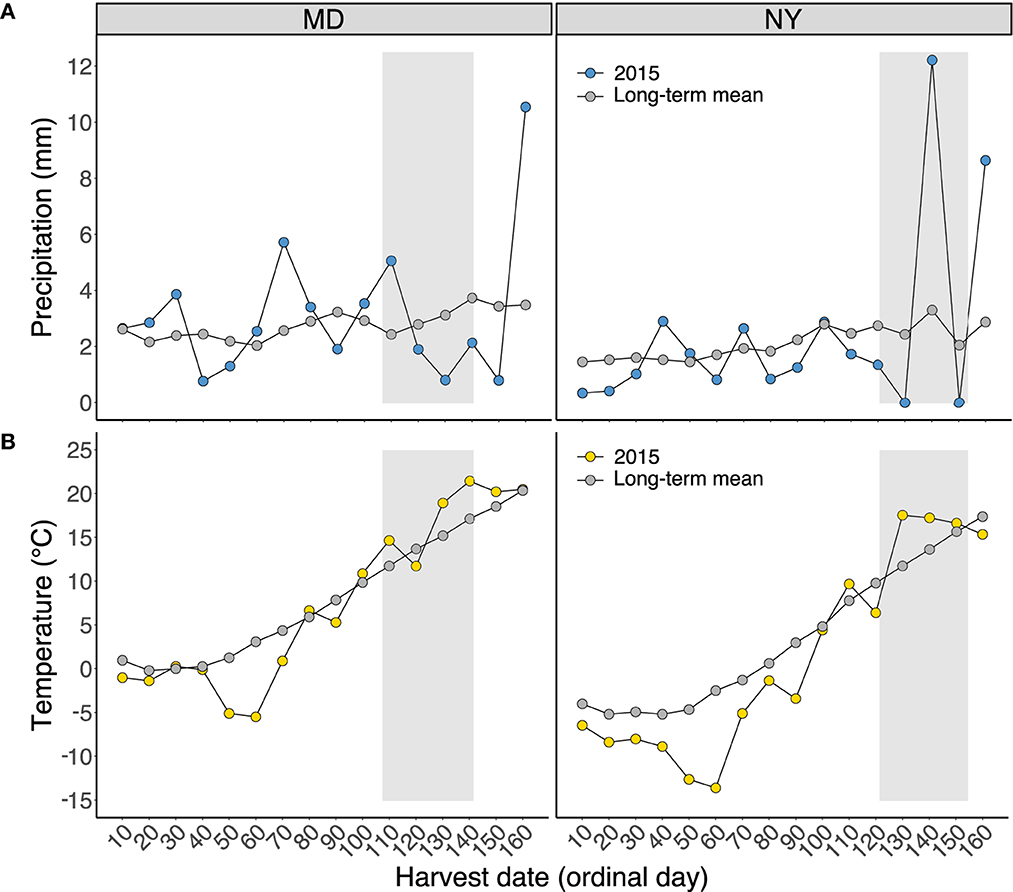
Figure 1. Mean daily (A) precipitation (mm) and (B) temperature (°C) for Beltsville, MD, and Aurora, NY, in 2015 for each 10-day interval beginning January 1 (day 1) through June 9 (day 160). The long-term mean represents the mean daily precipitation or temperature summarized using the earliest year of recorded weather station data associated with each field site: 1941–2014 for MD and 1956–2014 for NY (Northeast Regional Climate Center, 2022). Shaded regions indicate the sampling period, ranging from the first to the last harvest date in each site.
2.2. Experimental design and field operations
Arranged in a randomized complete block design, the yield and quality of barley, cereal rye, and triticale cultivars were compared across eight sampling dates. Four cultivars per species were assessed: “P-919,” “Thoroughbred,” “Valor,” and “Verdant” barley; “Aroostook,” “Huron,” “Lakeview VNS,” and “Spooner” cereal rye; and “NE426GT,” “TriCal 718,” “TriCal 815,” and “TriMark 099” triticale. The variety-not-stated (VNS) cereal rye cultivar was from Lakeview Organic Grains in Penn Yan, NY, and will be referred to as “Lakeview VNS.” The eight sampling dates occurred at 4–6-day intervals: day 107, 111, 117, 121, 125, 131, 135, and 141 in MD (i.e., April 17, 21, 27, and May 1, 5, 11, 15, and 21); and day 121, 125, 131, 135, 139, 144, 149, and 154 in NY (i.e., May 1, 5, 11, 15, 19, 24, 29, and June 3). Each site had 48 plots (12 cultivars × 4 blocks), which were 24.4 by 6.1 m in both MD and NY.
Based on soil tests from each site, the soil nutrient status was adequate for winter cereal planting, except for plant-available nitrogen in NY. Using a box-spreader, poultry litter (5–4–3, N–P2O5-K2O) was applied on the surface at a rate of 67 kg total N ha−1 prior to winter cereal planting in NY because the preceding sorghum crop showed signs of nitrogen deficiency. In MD, poultry litter was not applied as a nitrogen deficiency was not observed. The soil was prepared for planting with primary (disking) and secondary (cultipacking) tillage. Winter cereals were drill-planted at a depth of 2.5–3.8 cm with 19-cm row spacing on September 19, 2014, in NY and October 2, 2014, in MD. Although some farmers do not prioritize early winter cereal planting dates, these dates were selected due to the importance of fall growing degree days for biomass production in the spring (Mirsky et al., 2009). Earlier fall planting dates are also compatible with the typical harvest timing for shorter-season summer annual crops, such as sorghum or corn silage.
Seed size can vary widely across species and even cultivars, leading to potentially large differences in plant populations when using mass-based seeding rates (Lounsbury et al., 2022). To address this issue, all twelve winter cereals were seeded at an equivalent rate based on seed density, with “Aroostook” cereal rye as the reference cultivar. “Aroostook” was selected as the reference because it is very winter-hardy, matures early in the spring, and produces high yields (Mirsky et al., 2009), which are all valuable traits for a winter cereal in a double-cropping system. Setting the reference seeding rate at 126 kg ha−1 of “Aroostook” cereal rye, farm-scale seed drills were calibrated to plant an equivalent number of seeds per hectare for the other eleven cultivars, thereby accounting for differences in seed mass among the cultivars (Table 1). This approach resulted in density-based seeding rates that ranged from 1% lower to 86% higher (kg ha−1) than the “Aroostook” reference (Table 1).
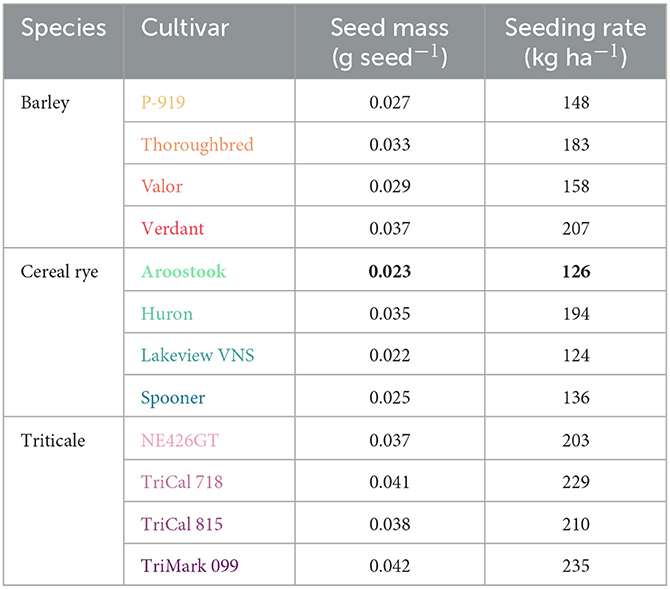
Table 1. Seed mass (g seed−1) and the density-based seeding rate (kg ha−1, relative to the reference cultivar, “Aroostook” cereal rye) for all twelve winter cereal cultivars.
2.3. Sampling protocols and lab procedures
Winter cereal biomass was determined by hand-clipping the plants 10.2 cm above the soil surface, mimicking typical machine harvest of winter cereal forages, within 20 by 100-cm sampling frames. Arranged perpendicular to the direction of winter cereal planting, the sampling frames spanned five crop rows. Biomass samples were collected at eight dates in the spring of 2015 between April and May in MD and May and June in NY. Immediately prior to biomass collection, the growth stage of the winter cereals was visually assessed and identified according to the Zadoks scale (Zadoks et al., 1974). The first sampling date at both sites was based on the formation and visibility of the second node (Zadoks 32) for the reference cultivar, “Aroostook” cereal rye. Due to the difference in latitude (and associated climatic and environmental factors) between the two sites, “Aroostook” cereal rye reached Zadoks 32 earlier in MD (day 107, April 17) than in NY (day 121, May 1). The sampling events, which occurred at 4–6-day intervals following the first sampling date, were selected to encompass a wide range of growth stages, including growth stages both earlier and later than the recommended harvest timing of swollen boot (Zadoks 45) for most winter cereals (Coblentz et al., 2018). These samples were obtained from eight locations within the randomly arranged plots. The sampling locations were systematically assigned during the experimental design phase to maximize the space between the samples and avoid both in-field sampling bias (e.g., avoiding areas of poor growth) and edge effects from sampling too close to plot borders. Following each sampling event, the biomass samples were dried in forced-air ovens at 60°C for ~1 week and then weighed so that dry matter (DM) yield could be calculated.
The dried biomass samples were ground to 1-mm particle size in preparation for the forage quality analyses. The samples were then submitted to Dairyland Laboratories in Arcadia, WI, where Near Infrared Spectroscopy (NIR), supported by a robust database of cool-season grass forages, was used to analyze the winter cereals for crude protein, CP; acid detergent fiber, ADF; neutral detergent fiber, NDF; and 48 h in vitro NDF digestibility, NDFD48 (Marten et al., 1985; Association of Official Agricultural Chemists, 1990). For estimating NDFD, a single point in time (or endpoint) of 48 h was selected to reflect NDF passage kinetics from the rumen (Coblentz et al., 2019).
2.4. Statistical analyses
All data were analyzed using R version 4.0.5 (R Core Team, 2021). Regression diagnostics were performed (“car” package: Fox and Weisberg, 2019; “influence.ME” package: Nieuwenhuis et al., 2012; “redres” package: Goode et al., 2019) to ensure that the residuals and random effect coefficients were homogenously distributed and independent (Schielzeth et al., 2020). Model diagnosis included an assessment of outliers and influential observations (e.g., Cleveland dotplots and Cook's distance); homogeneity of the residuals and linearity between the predictors and the response (e.g., plotting conditional raw residuals against fitted values); and distribution of the residuals and random effects (e.g., quantile plots).
2.4.1. Yield
A linear mixed-effects model (“lme4” package: Bates et al., 2015) was used to predict yield (Mg DM ha−1) as a function of three fixed effects: growth stage (Zadoks, 0–100, continuous), cultivar (12 levels), and site (2 levels). This model included the three-way and all two-way interactions among the fixed effects, along with each predictor as a main effect. Random effects included block (eight levels) and plot (96 levels). With unique designations for each factor level of block and plot, the random effects are equivalent to block and plot individually crossed with site (i.e., 4 blocks site−1 and 48 plots site−1). The fixed effect associated with each subsample (collected in each plot at the eight sampling dates) is represented by growth stage in this model. To address the heteroscedasticity and non-normality of the residuals for yield, the response was square root transformed (McCune et al., 2002).
Analysis of variance (“lmerTest” package: Kuznetsova et al., 2017) was performed on the linear mixed-effects model. The marginal and conditional coefficients of determination (R2) were also assessed (“MuMIn” package: Bartoń, 2020), which represent variance explained by the fixed effects and by both fixed and random effects, respectively (Nakagawa and Schielzeth, 2013). The difference between marginal slopes for each cultivar in the linear regression (i.e., pairwise comparisons or contrasts) was assessed with the Tukey Method to test the interactions (“emmeans” package: Lenth, 2021).
2.4.2. Forage quality
The University of Wisconsin Alfalfa/Grass Evaluation System (also known as MILK 2016) was used to calculate relative forage quality (RFQ), which is described as
where DMIgrass is the dry matter intake of a cool season grass as a percentage of body weight (Moore et al., 1999); TDNgrass is the total digestible nutrients for a cool season grass as a percentage of dry matter (Moore and Undersander, 2002); and the divisor, 1.23, is used to adjust the equation so that the mean and range are similar to Relative Feed Value (Undersander et al., 2010). The effects of growth stage, cultivar, and site on RFQ were analyzed with the same linear mixed-effects model structure and analytical approach described for yield, except no transformation of the response was required. Although RFQ was evaluated as the primary measure of nutritive value for each winter cereal cultivar, supplemental analyses for the constituents of the summative RFQ metric—CP (% DM), ADF (% DM), NDF (% DM), and NDFD48 (% NDF)—were also conducted in the same way as RFQ.
2.4.3. Crop phenology
Winter cereal phenological development was predicted with self-starting logistic models (“nlme” package: Pinheiro et al., 2021). The data were fit to a four-parameter sigmoidal (or “S-shaped”) function, which can be described as
where, given ø4 > 0, ø1 is the horizontal asymptote as x (harvest date) approaches ∞; ø2 is the horizontal asymptote as x approaches –∞; ø3 is the value of x at the inflection point (i.e., the growth stage response, y, is midway between the asymptotes at this x value); and ø4 is a scale parameter on the x-axis (i.e., when x = ø3 + ø4 the response is ~three-quarters of the distance from ø1 to ø2) (Pinheiro and Bates, 2000). As a four-parameter model did not fit the “Aroostook” cereal rye data in MD, phenological development for this cultivar was predicted with a three-parameter sigmoidal function. The three-parameter logistic model is a special case of the four-parameter model in which one of the horizontal asymptotes (ø1 or ø2) is zero (Pinheiro and Bates, 2000).
2.4.4. Trade-offs
Farmers commonly use swollen boot stage (Zadoks 45) as a visual phenological indicator to time winter cereal harvest for forage. Using this growth stage as a baseline, percent change in yield and RFQ was calculated for growth stages that occur before and after Zadoks 45. Using the day associated with the earlier (Zadoks 39) and later (Zadoks, 51, 57, and 63) growth stages, the number of days earlier or later—relative to Zadoks 45—that a farmer would need to harvest to obtain forage at these specific growth stages was also determined. The trade-offs between yield and RFQ, and the timing (and length) of these phenology-based harvest dates, were all calculated using the estimated marginal means drawn from the previously described statistical models for yield, RFQ, and harvest date (day).
3. Results and discussion
3.1. Winter cereal yield
A three-way interaction among cultivar, growth stage, and site was observed (p = 0.005) for yield (Table 2), and all slopes were different from zero (p < 0.0001). As growth stage advanced, yield increased across all winter cereal cultivars. However, the rate of yield gain varied by cultivar, and these differences depended on site.
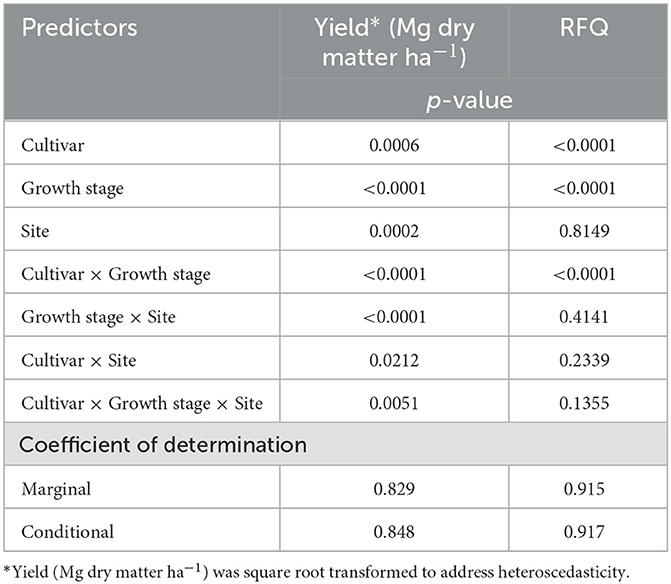
Table 2. Analysis of variance for yield (Mg dry matter ha−1) and relative forage quality (RFQ) as affected by winter cereal cultivar (all twelve), growth stage (Zadoks), and site (MD and NY).
In MD, yield ranged across sampling dates from 0.5 to 5.9 Mg ha−1 among barley cultivars, 0.8–7.4 Mg ha−1 among cereal rye cultivars, and 0.8–7.1 Mg ha−1 among triticale cultivars (Figure 2). As growth stage increased in MD, all barley cultivars produced lower yields than “TriCal 718” triticale (p ≤ 0.03). “Valor” barley also gained less yield across growth stages (i.e., the slope was less steep) than all cultivars except “Verdant” barley and “TriCal 815” triticale. No slope differences were observed between any cereal rye and triticale cultivars in MD.
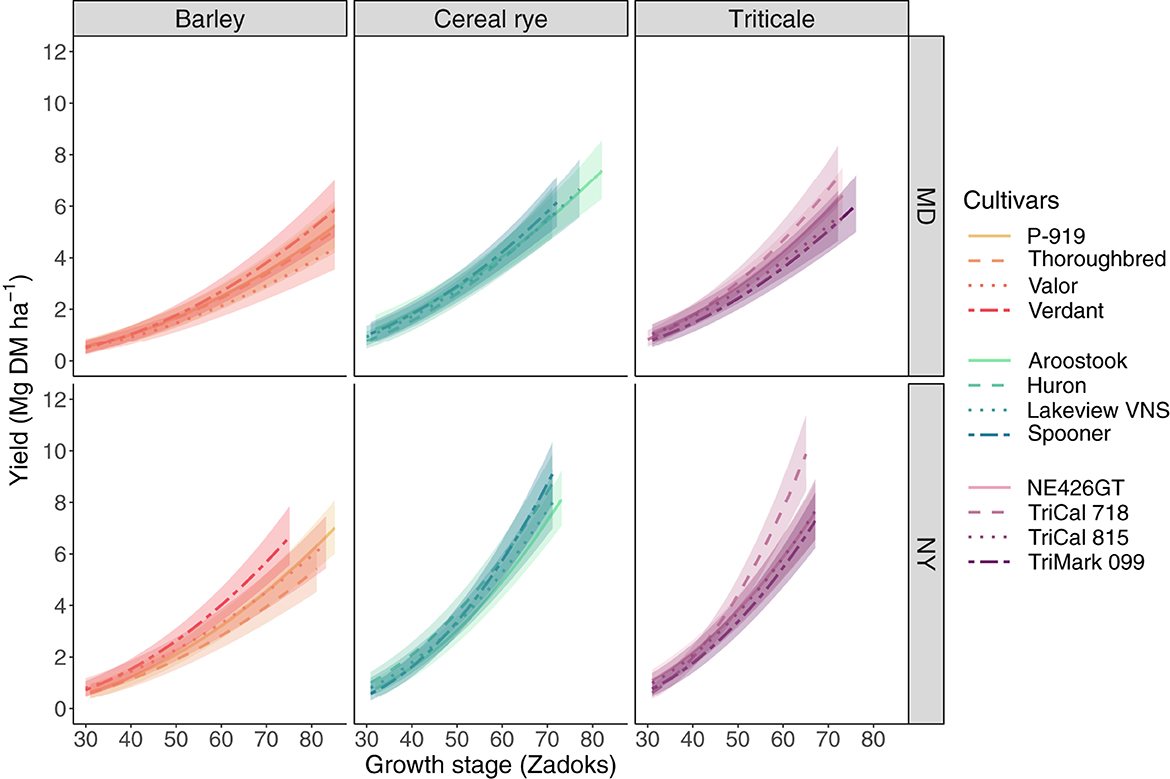
Figure 2. The effect of winter cereal cultivar (four per species), growth stage (Zadoks), and site (MD and NY) on yield (Mg dry matter ha−1). Yield has been back- transformed from the square root transformation. The shaded “ribbon” accompanying each cultivar slope (line) represents a 95% confidence interval. Analysis of variance and coefficients of determination for this linear mixed-effects model are presented in Table 2.
In NY, yield ranged across sampling dates from 0.6 to 7.0 Mg ha−1 among barley cultivars, 0.6–9.1 Mg ha−1 among cereal rye cultivars, and 0.6–9.9 Mg ha−1 among triticale cultivars (Figure 2). Yield gain differences across growth stage were also largely limited to barley cultivars in NY. “P-919,” “Thoroughbred,” and “Valor” barley produced lower yield gains (p ≤ 0.003) as growth stage advanced when compared with cereal rye and triticale cultivars. “Verdant” barley, in contrast, yielded more similarly to cereal rye and triticale cultivars, although “Spooner” cereal rye and “NE426GT” triticale produced greater yield gains (p ≤ 0.009) across growth stages than “Verdant.” Intraspecific comparisons of yield gains exhibited no differences among the four barley cultivars. “TriCal 718” triticale also emerged as a high-yielding cultivar in NY, producing greater yield gains (p ≤ 0.04) than all other cultivars except “Spooner” cereal rye (p = 0.2) and “TriCal 815” triticale (p = 0.06).
After a period of vernalization and growth has advanced beyond the formation of a pseudostem (Zadoks 30), winter cereals make a transition from the vegetative to reproductive phase of development. The reproductive phase includes stem elongation (Zadoks 30–39), booting (Zadoks 40–49), spike (i.e., head or ear) emergence (Zadoks 50–59), anthesis (Zadoks 60–69), milk development (Zadoks 70–79), and dough development (Zadoks 80–89). The alignment of developmental stages with seasonal conditions (temperature, solar radiation, and water availability) during the reproductive phase is essential for optimizing winter cereal yields (Hyles et al., 2020). Genotypic response and region-specific factors, such as latitude and day length (photoperiod), also interact with abiotic conditions and soil and crop management (including planting and harvest timing) to influence yield in relation to growth stage (Slafer and Rawson, 1994). The addition of poultry litter (5–4–3) at fall planting in NY, for instance, might have contributed to the higher overall yields and CP content compared with MD (Supplementary Figure 1).
Among species, cereal rye is known to produce greater yields than triticale (Brown and Almodares, 1976; Helsel and Thomas, 1987; Kaspar and Bakker, 2015), which in turn is known to out-yield barley (Jemison et al., 2012). However, some research has reported that triticale produces greater yields than cereal rye (Tumbalam et al., 2016; Landry et al., 2019), which was observed in our experiment for “TriCal 718” when compared with three of the four cereal rye cultivars. Lower barley yields are frequently reported in experiments from regions with low winter temperatures, due in part to its inferior winter hardiness. Winterkill was not observed in our experiment, but barley does not survive winters as consistently as cereal rye or triticale in NY.
The wide array of factors that affect winter cereal yield can make cultivar selection within a species an important decision. In our experiment, intraspecific yield differences were equivalent to, or larger than, interspecific differences (Figure 2). In other research comparing multiple cultivars per winter cereal species, yield differences among cultivars have been reported (Juskiw et al., 2000; Harmoney and Thompson, 2010; Kaspar and Bakker, 2015), though not consistently (Carr et al., 2004). Depending on the narrowness of the germplasm pool that cultivars are selected from, substantial differences may or may not be expected (Lyu et al., 2018).
Although this experiment did not compare the combined yields of a winter cereal and summer annual with a summer annual alone, numerous studies in the US (Brown, 2006; Heggenstaller et al., 2008, 2009; Fouli et al., 2012; Jemison et al., 2012; Ketterings et al., 2015; West et al., 2020) and abroad (Graß et al., 2013; Wannasek et al., 2019) have found that double-cropping systems tend to provide more biomass than single (or “sole”) cropping systems, while also providing additional ecosystem services. It should be noted that some research has shown that growing a winter cereal prior to planting a summer annual crop can suppress the second crop in a double-cropping system. Most of this research has focused on cereal rye and corn silage double-cropping systems, with yield reductions in corn attributed to a reduction in soil nitrate or soil moisture (Krueger et al., 2011, 2012), negative effects of allelopathy (Raimbault et al., 1990; Tollenaar et al., 1992; Acharya et al., 2021), increased incidence of seedling disease (Acharya et al., 2017, 2020), or delayed planting (Darby and Lauer, 2002).
3.2. Forage quality
Although yields are important, dairy farmers must also consider the quality, or nutritive value, of a forage when making cropping system decisions. A three-way interaction among cultivar, growth stage, and site was not detected (p = 0.1) for RFQ (Table 2). Assessing the two-way interactions, site did not interact with cultivar (p = 0.2) or growth stage (p = 0.4), but an interaction was observed between cultivar and growth stage (p < 0.0001). All marginal slopes were different from zero (p < 0.0001). Key components of RFQ, such as CP, ADF, NDF, and NDFD48, all followed expected trends (Khorasani et al., 1997) in relation to advancing crop maturity: CP decreased, ADF increased, NDF increased, and NDFD48 decreased (Supplementary Figures 1–4, Supplementary Table 1). Winter cereal RFQ, however, will be the focus here as it represents a summative estimation of nutritive value.
As growth stage advanced, RFQ declined (Figure 3). In MD, RFQ decreased across sampling dates from 200 to 129 among barley cultivars, 216 to 41 among cereal rye cultivars, and 202 to 81 among triticale cultivars. In NY, RFQ decreased across sampling dates from 200 to 136 among barley cultivars, 205 to 64 among cereal rye cultivars, and 200 to 82 among triticale cultivars. At both sites, no differences in the slopes describing RFQ across growth stages were observed among the four barley cultivars (p ≥ 0.4), but each barley cultivar maintained greater RFQ (p < 0.0001) as the plants matured compared with all cereal rye and triticale cultivars (i.e., the negative slopes were less steep for barley cultivars).
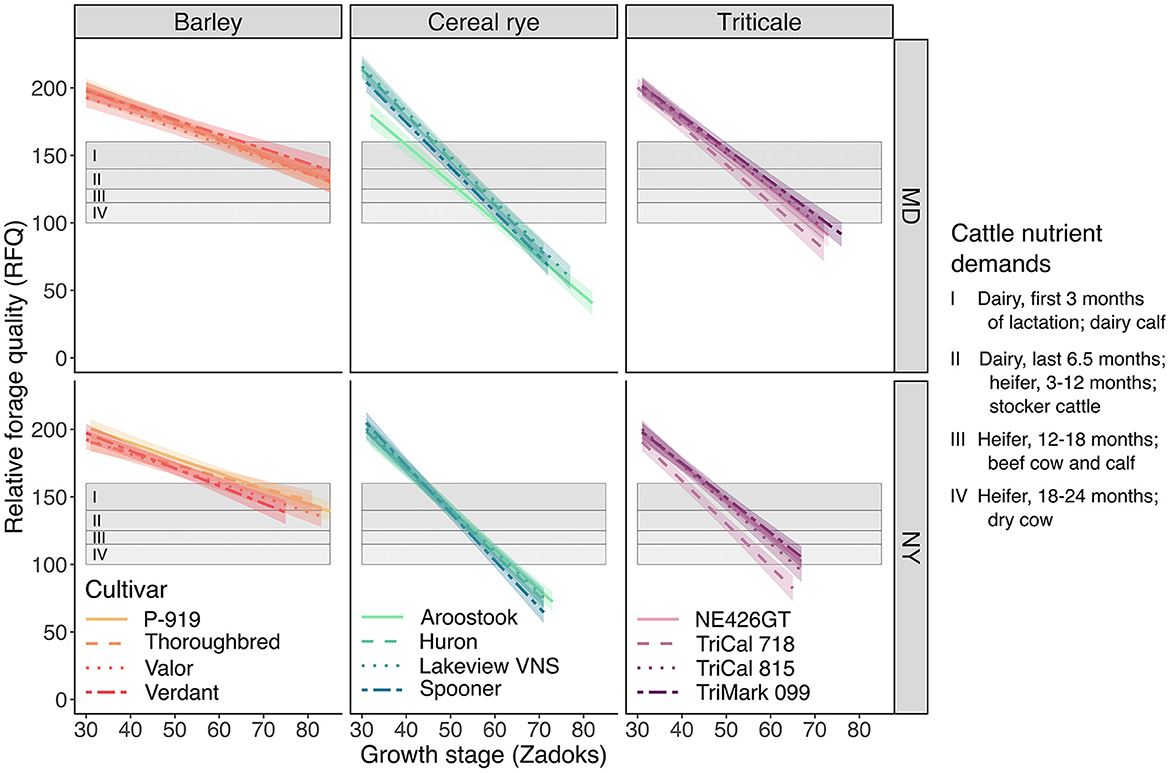
Figure 3. The effect of winter cereal cultivar (four per species), growth stage (Zadoks), and site (MD and NY) on relative forage quality. The shaded “ribbon” accompanying each cultivar slope (line) represents a 95% confidence interval. Shaded rectangular areas indicate the recommended forage quality as it relates to the specific nutrient demands of an animal: (I) dairy cow (first 3 months of lactation) or dairy calf, RFQ 140–160; (II) dairy (last 6.5 months), heifer (3–12 months), or stocker cattle, RFQ 125–150; (III) heifer (12–18 months) or beef cow and calf, RFQ 115–130; and (IV) heifer (18–24 months) or dry cow, RFQ 100–120 (adapted from Ball et al., 2008). The ranges presented here show the minimum RFQ for each group (I–IV) to the minimum of the next group (with the exception of group I, as there is no group that has higher quality demands); the maximum values, which overlap with the next group (with the exception of group I), are not depicted in the shaded areas. Analysis of variance and coefficients of determination for this linear mixed-effects model are presented in Table 2.
In MD, the slope associated with “Aroostook” cereal rye (−2.8 RFQ growth stage−1) was not different from the other cereal rye (p ≥ 0.4) and triticale (p ≥ 0.9) cultivars, whereas “Huron,” “Lakeview VNS,” and “Spooner” cereal rye exhibited a greater decline in RFQ (−3.3 RFQ growth stage−1 for all three cultivars) compared with “NE426GT,” “TriCal 718,” “TriCal 815,” and “TriMark 099” triticale (−2.4 to −2.9 RFQ growth stage−1, p ≤ 0.02). Among the four triticale cultivars, no differences were detected in MD (p ≥ 0.7).
In NY, no differences between slopes were observed among cereal rye (−3.0 to −3.1 RFQ growth stage−1) and triticale cultivars (−2.6 to −3.2 RFQ growth stage−1), with two exceptions: “Spooner” cereal rye exhibited greater RFQ decline (-3.5 RFQ growth stage−1) compared with “NE426GT” (−2.7 RFQ growth stage−1, p = 0.02) and “TriMark 099” (−2.6 RFQ growth stage−1, p = 0.008) triticale. Although differences were detected when conducting pairwise comparisons of the slopes among cultivars at each site separately, it should be noted that interactions with site, and site as a main effect, did not help explain the variance for RFQ in our model (Table 2).
Compared with cereal rye and triticale cultivars, barley cultivars exhibited a notably smaller decline in RFQ as the crops advanced in maturity. For barley cultivars, this decrease ranged from 0.9 to 1.2 RFQ growth stage−1, whereas cereal rye and triticale exhibited a 2–4-fold greater decline in RFQ growth stage−1.
As a cultivar bred for grain, “Thoroughbred” barley was of surprisingly similar quality to the other three barley cultivars, which were bred as a dual-purpose grain and forage cultivar (“P-919”) or explicitly for forage or grazing (“Valor” and “Verdant”). Previous research has determined that barley has higher in vitro digestibility and lower acid detergent fiber, acid detergent lignin, and cell wall constituents than triticale (Cherney and Marten, 1982a). By separately analyzing each whole-plant yield component (e.g., leaf blade, leaf sheath, stem, and inflorescence) in winter cereal forages, greater digestibility of barley was found primarily as a result of highly digestible inflorescence comprising a greater proportion of the total dry matter across growth stages (Cherney and Marten, 1982b).
Particularly at more advanced growth stages, other research has also found higher forage quality for barley compared with triticale (Khorasani et al., 1997; Lyu et al., 2018) or cereal rye (Helsel and Thomas, 1987), and triticale has been found to exhibit higher quality than cereal rye (Brown and Almodares, 1976). Although differences among cultivars within each species were not consistently detected in our experiment, intraspecific differences have been frequently reported when comparing the nutritive value of forage cultivars (Juskiw et al., 2000; Kim et al., 2016; Lyu et al., 2018). Such differences represent a key component of livestock management decisions.
Livestock have specific nutrient demands at different ages and reproductive stages. For example, dairy calves and dairy cows during the first 3 months of lactation have the highest nutritional requirements among cattle on a dairy farm (Ball et al., 2008). Meeting these high demands is a priority for farmers. High quality feed rations that fulfill such requirements, however, are typically the most expensive to purchase, as well as the most time-sensitive and challenging to manage and produce on-farm.
Harvesting winter cereals for forage at swollen boot stage is common among dairy producers (Coblentz et al., 2018) because the nutritive value (e.g., RFQ) is typically high enough to feed to the most nutrient-demanding cattle on the farm. Also, swollen boot stage is reached relatively early in the growing season, thereby minimizing any delay in preparing the soil and planting the following crop. At swollen boot stage, RFQ ranged from 144 to 185 across cultivars and sites in our experiment (Figure 3). Based on previously defined thresholds (Ball et al., 2008), all cultivars maintained high enough RFQ to meet the nutrient requirements of the most demanding cattle (group I, Figure 3). However, not all cultivars performed equivalently when considering yield (Figure 2), the date at which swollen boot stage is reached, and length of the harvest window. These considerations are equally important if a farmer is seeking to produce forage for other cattle (groups II–IV) as well (Figure 3).
3.3. Phenological development and harvest windows
Located further south than NY, the MD site is in USDA plant hardiness zone 7a (minimum temperature = −17.8°C). This hardiness zone has a greater minimum temperature threshold than plant hardiness zone 6a (minimum temperature = −23.3°C), which is associated with the NY site location (United States Department of Agriculture, 2012). Accordingly, all cultivars across the three species in MD—as described by three- and four-parameter logistic models (Supplementary Table 2)—reached swollen boot stage earlier in the year than in NY (Figure 4). The earliest and latest cultivars to reach swollen boot stage, however, were the same in both MD and NY: “Aroostook” cereal rye reached swollen boot stage in 113 days (April 23) in MD and 128 days (May 8) in NY, while “TriCal 718” triticale reached the same growth stage in 125 days (May 5) in MD and 141 days (May 21) in NY, which was 12 and 13 days later than “Aroostook,” respectively.
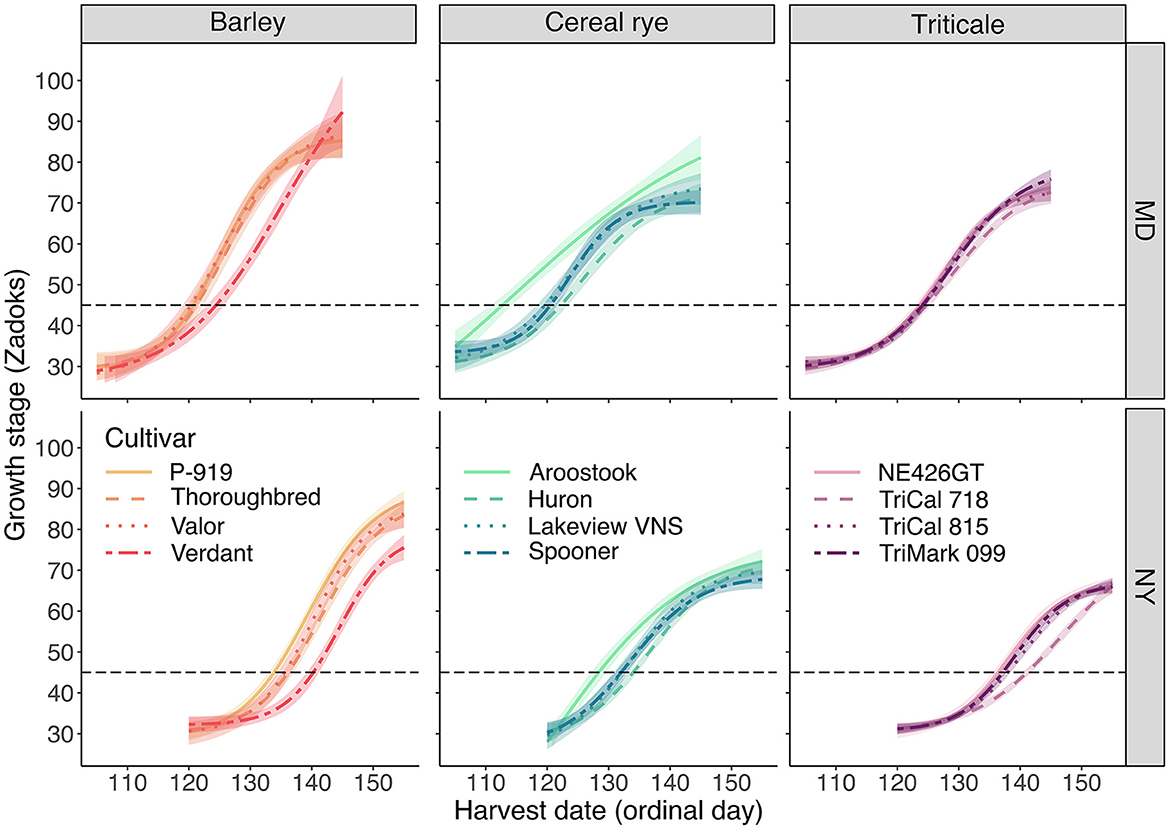
Figure 4. Phenological development for barley, cereal rye, and triticale cultivars as described by the logistic models for the relationship between growth stage (Zadoks) and harvest date (ordinal day) in MD and NY. The shaded “ribbon” for each sigmoidal curve represents a 95% confidence interval. The horizontal dashed line indicates a common phenology-based harvest timing, swollen boot stage (Zadoks 45), for winter cereals grown for forage.
Within species, the mean number of days to reach swollen boot stage for barley, cereal rye, and triticale was 122, 119, and 124 in MD and 136, 132, and 138 in NY, respectively (Figure 4). Among the barley cultivars, “P-919,” “Thoroughbred,” and “Valor” reached swollen boot stage ~ 4–5 and 4–6 days earlier than “Verdant” in MD and NY, respectively. Among the cereal rye cultivars, “Aroostook” reached swollen boot stage ~6–8 and 3–5 days earlier than “Huron,” “Lakeview VNS,” and “Spooner” in MD and NY, respectively. The four-parameter logistic model was modified to accommodate the particularly rapid transition from vegetative to reproductive growth observed in “Aroostook” cereal rye in MD (Supplementary Table 2). And, among the triticale cultivars, no notable maturation differences were observed in MD, whereas “NE426GT,” “TriCal 815,” and “TriMark 099” reached swollen boot stage ~3–5 days earlier than “TriCal 718” in NY.
Early maturity is a valuable characteristic for a winter cereal forage, particularly in double-cropping systems. Using swollen boot stage as a baseline for comparisons across species, the average cereal rye cultivar reached the target growth stage 3–4 days earlier than the average barley cultivar, which in turn reached swollen boot stage ~2 days earlier than the average triticale cultivar. Similar outcomes were found by other researchers who compared at least two of the three species assessed in our experiment. For example, research conducted in Ontario, Canada, found that cereal rye reached swollen boot stage 4–8 days earlier than triticale (Landry et al., 2019), whereas a 5–6-day difference was observed, on average, in our experiment in the northeastern US. However, a much larger difference of 12–13 days is observed in our experiment if the earliest-maturing cereal rye cultivar (“Aroostook”) is compared with the latest-maturing triticale cultivar (“TriCal 718”). This discrepancy between the maximum and minimum day values at swollen boot stage and the mean day values highlights the potential advantages, or disadvantages, associated with both species and cultivar selection.
As some dairy farmers might harvest a winter cereal that has matured beyond swollen boot stage, either to feed a heifer or dry cow or because timely harvest at swollen boot stage was not possible, it is important to consider winter cereal phenology past that target growth stage. The sigmoidal function of the logistic models (Figure 4) indicates that the barley cultivars exhibit more rapid maturation than cereal rye and triticale cultivars following booting (Zadoks 40–49) and continuing through anthesis (Zadoks 60–69) or milk development (Zadoks 70–79). For example, the barley cultivars reached quarter-complete anthesis (Zadoks 63) 0–1 day earlier than the average cereal rye cultivar despite arriving at swollen boot (Zadoks 45) 3–4 days later than the cereal rye cultivars. Similarly, barley cultivars reached quarter-complete anthesis 4–6 days earlier than triticale cultivars despite arriving at swollen boot stage just 2 days before the triticale cultivars.
3.4. Harvest timing and the trade-offs between yield and quality
Although the non-linear models provide biologically realistic representations of winter cereal phenological development, applying these findings requires synthesis with yield and quality outcomes. Consequently, an evaluation of the trade-offs among yield, quality, and timing is an essential component of designing and managing the winter cereal forage component within a double-cropping system. Relativizing the increases and decreases in yield and RFQ as percent change across growth stages provides a simple approach for comparing the outcomes of phenology-based harvest timing (Table 3). With swollen boot stage as the baseline, percent change in yield and RFQ—relative to this reference growth stage—are shown for multiple identifiable growth stages at 6-stage intervals (Table 3): when the flag leaf ligule just becomes visible (Zadoks 39), when the tip of the spike just becomes visible (Zadoks 51), when the spike is three-quarters emerged from the boot (Zadoks 57), and when anthesis is one-quarter complete (Zadoks 63). To increase the practical relevance of these changes, the trade-offs between yield and quality should also be considered in relation to the number of days that separate the growth stage differences (Table 3).
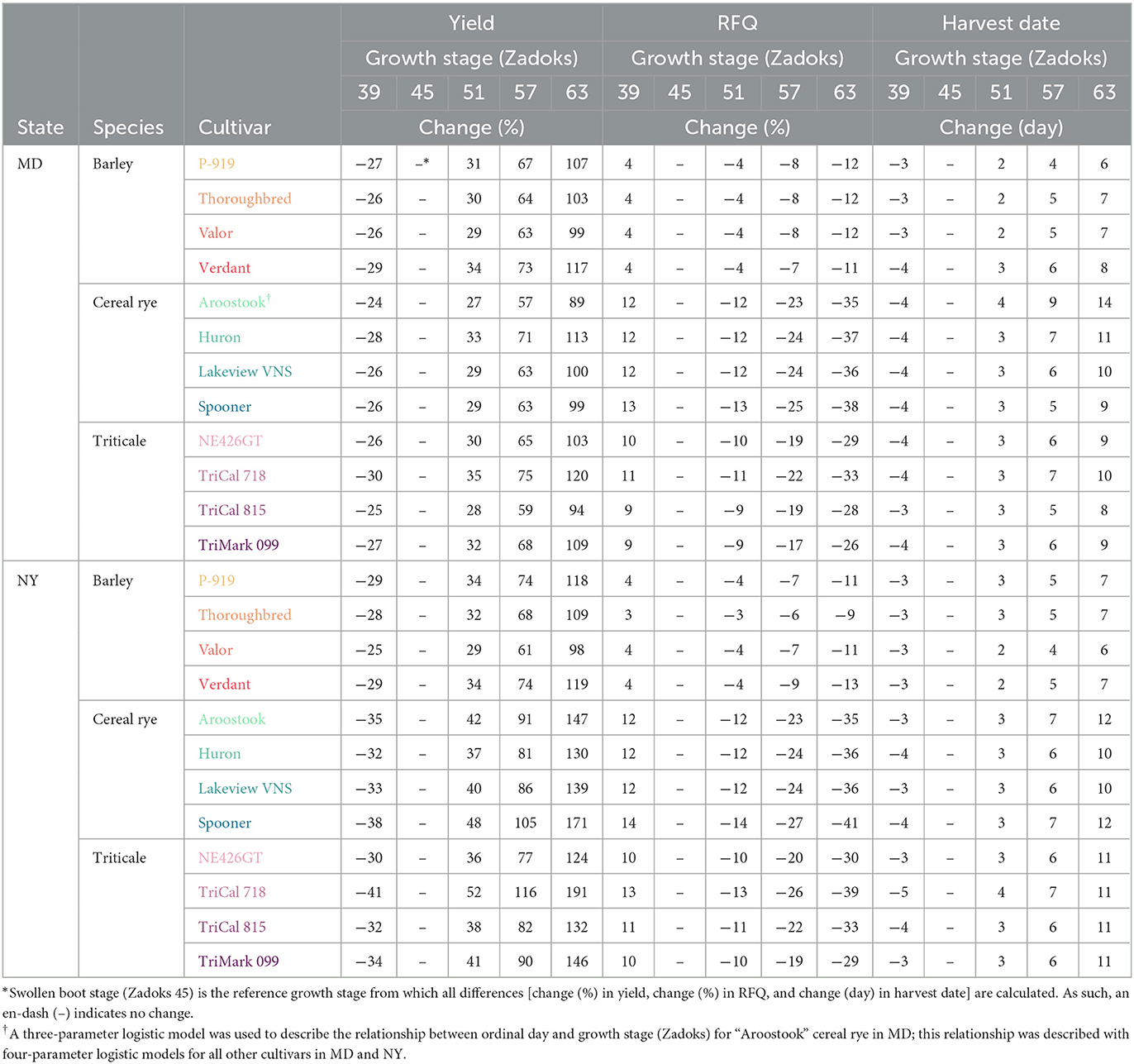
Table 3. Change (%) in dry matter yield, change (%) in relative forage quality (RFQ), and change (number of days) in harvest date in relation to a common winter cereal harvest timing of swollen boot stage (Zadoks 45) for all barley, cereal rye, and triticale cultivars in MD and NY.
Prior to the yield plateau that is reached at advanced maturity, harvesting winter cereals at earlier growth stages resulted in lower yields and higher quality. Harvesting when the flag leaf ligule became visible, which occurred 3–5 days earlier than swollen boot stage, resulted in 27, 30, and 31% lower mean yields and 4, 12, and 10% greater mean RFQ for barley, cereal rye, and triticale cultivars, respectively, across sites. Given that all twelve cultivars at swollen boot stage maintained RFQ in excess of 160, which is the upper value of the required RFQ range for the most demanding cattle (group I, Figure 3), this trade-off between yield and timing might be undesirable for most farmers. However, a 27–31% yield reduction to gain an excessive or unnecessary 4–12% improvement in RFQ and 3–5 days for the following growing season might appear more favorable if using those additional days for the subsequent crop (e.g., corn silage or sorghum) resulted in greater yield and quality gains than the winter cereal yield loss. It is also worth noting that the spring is a particularly busy time of year for dairy farmers, and pre-boot harvesting might be preferred if a farmer is unlikely to harvest the winter cereal crop at swollen boot stage (or shortly thereafter) due to other farm tasks or projected weather impediments.
Delaying harvest 2–4 days until the tip of the ear becomes visible (i.e., a six-stage delay to Zadoks 51) resulted in similar values for gains and losses as harvesting 3–5 days (6 growth stages) earlier, except inverted: at Zadoks 51, mean yields were 32–37% greater and mean RFQ was 4–12% lower across species and sites (Table 3). If harvest is delayed 6–14 days past swollen boot stage until the winter cereal crop reached quarter-complete anthesis (i.e., an 18-stage delay to Zadoks 63), mean yield gains would more than double at 109, 124, and 127% and mean RFQ would decrease by 11, 37, and 31% for barley, cereal rye, and triticale, respectively (Table 3). Across all barley cultivars, RFQ remains high enough to feed lactating cows in their first trimester or dairy calves (cattle group I) at quarter-complete anthesis, making the mean 109% increase in yield particularly attractive. Notably, barley cultivars reach quarter-complete anthesis in 6–8 days after swollen boot stage, which is quicker than cereal rye (9–14 days) and triticale (8–11 days). Still, barley cultivars were the lowest-yielding cultivars in the experiment (Figure 2).
Cereal rye and triticale cultivars exhibited large yield gains of 124 and 127%, respectively, when harvest was delayed until quarter-complete anthesis (Table 3). The trade-off with RFQ, however, was substantial with a 37% decline for cereal rye and a 31% decline for triticale compared with RFQ at swollen boot stage. Unlike the barley cultivars, none of the cereal rye and triticale cultivars maintained high enough RFQ at quarter-complete anthesis to meet the nutritional demands of cattle group I (140–160 RFQ). Instead, the RFQ at this later growth stage ranged from 93 to 106 for the cereal rye cultivars and 89–123 for the triticale cultivars across sites (Figure 3). Only “Lakeview VNS” maintained >100 RFQ in both MD and NY at quarter-complete anthesis (9–14 days post-boot) among the cereal rye cultivars, whereas all triticale cultivars, except “TriCal 718” in NY, met the nutritional demands of cattle group III (115–130 RFQ) or IV (100–120 RFQ) at the same growth stage (8–11 days post-boot).
At the cultivar level, the trade-off between yield and quality across growth stages is demonstrated by “TriCal 718” triticale in NY. This cultivar produced over 9 Mg DM ha−1 at quarter-complete anthesis, which was more than all other cultivars (p ≤ 0.06). At this growth stage, however, “TriCal 718” exhibited a RFQ of 89, which was lower than all other triticale cultivars (p ≤ 0.06), all barley cultivars (p < 0.0001), and no different from the cereal rye cultivars (p ≥ 0.2).
4. Summary and next steps
These experiments have shown that while barley cultivars maintain higher RFQ than cereal rye and triticale, yield was substantially lower. With RFQ ranging from 144 to 166 at swollen boot stage across cultivars and sites, cereal rye was a strong candidate for obtaining a balance between yield and quality early in the season. Although the RFQ of cereal rye decreased quickly at growth stages beyond booting, the rate of decline for cereal rye cultivars (−2.8 to −3.5 RFQ per growth stage) was similar to triticale cultivars (−2.4 to −3.2 RFQ per growth stage). Triticale yield was similar to cereal rye, and the RFQ of triticale also declined more rapidly than barley. At later growth stages, however, triticale generally exhibited greater RFQ than cereal rye. This higher quality in triticale might provide the benefit of a slightly longer “harvest window” to obtain forage that is nutritionally suitable for multiple cattle groups. Overall, our results show that cereal rye cultivars are recommended if early harvest is the priority, triticale cultivars are recommended for farmers who seek greater flexibility in their harvest schedules, and barley cultivars are generally not recommended in regions with low winter temperatures due to lower yields and lower cold tolerance (Table 4).
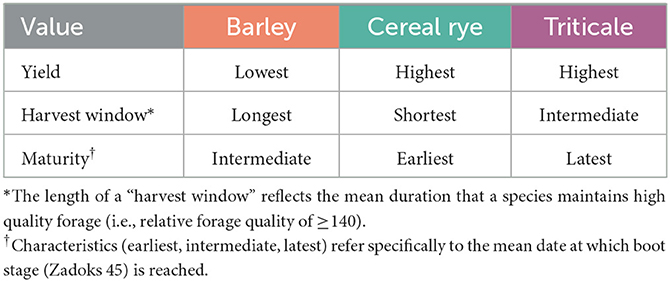
Table 4. Qualitative summary of the trade-offs for yield, harvest window (i.e., forage quality retention), and maturity among barley, cereal rye, and triticale.
As this study is limited by a lack of temporal replication, more multi-year research that assesses the trade-offs among yield, quality, length of harvest window, and earliness of maturity for multiple winter cereal species and cultivars will be valuable for establishing robust, region-specific recommendations for farmers and other practitioners. Due to previous conventional management at both research stations, the organically managed experiments in MD and NY represent cropland under transition to organic certification. Findings from our research should be further investigated with on-farm research in fields with a history of organic management and in close collaboration with farmers who have experience with certified organic production.
To improve the performance of forage double-cropping systems for dairy farmers in the Northeast, research focused on plant breeding, crop diversification, ecosystem services, and whole-system assessment should be simultaneously pursued. Genetic improvements to barley cold tolerance, which could indirectly improve yield potential, would be of great value to dairy farmers in more northern regions of the US given the sustained, high RFQ of barley cultivars. Our results demonstrated that intraspecific differences within a species can be as important as interspecific differences between species, which underscores the importance of breeding for specific environments and co-benefits. More research is needed on fall-planted mixtures of winter cereal species and mixtures of winter cereals and legumes for spring-harvested forage. Continued research on summer annual forages that can serve as alternatives to corn silage will also be critical for providing farmers with more options for diversifying their cropping system. The effect of grazing or harvesting winter cereals, compared with incorporating or creating a mulch with the plant residue, is also under-researched as it relates to the delivery of various ecosystem services. Comparing the combined agronomic, environmental, and economic value—in total yield, quality, and ecosystem services—of double-cropping with single cropping is important for facilitating greater adoption.
In the Northeast, winter cereals can serve as important sources of high-quality forage on organic and non-organic farms when feed inventories are low or when extreme weather events have disrupted feed and forage production in other regions of the US, causing feed shortages. With timely management, dairy farmers can synchronize winter cereal harvest with the specific nutritional requirements of the cattle on their farms. Greater home-grown double-cropping forage production can reduce the environmental and economic costs associated with feed imports, help relocalize nutrient cycles, provide more opportunities for judiciously applying dairy manure, and increase both crop and non-crop biodiversity.
Data availability statement
The raw data supporting the conclusions of this article will be made available by the authors, without undue reservation.
Author contributions
JL, SM, and MR designed and implemented the study with support from JC, QK, and CP. JL, SM, and CP established the field experiments and collected the data. JL analyzed the data and led the writing and revision of the manuscript. JC, QK, SM, CP, and MR provided guidance, comments, and edits. All authors approved the submitted version.
Funding
This research was supported by the USDA National Institute of Food and Agriculture (NIFA) Organic Research and Extension Initiative (OREI) projects 2016-25-8468 and 2020-51300-32183.
Acknowledgments
We would like to thank the staff at the Musgrave Research Farm and USDA Beltsville Agricultural Research Center (BARC) for their assistance with field operations, as well as members of the Sustainable Cropping Systems Lab at Cornell University and Sustainable Agricultural Systems Lab at BARC for their assistance with in-field data collection and processing. JL also thanks the Natural Sciences and Engineering Research Council of Canada for providing additional financial support.
Conflict of interest
The authors declare that the research was conducted in the absence of any commercial or financial relationships that could be construed as a potential conflict of interest.
Publisher's note
All claims expressed in this article are solely those of the authors and do not necessarily represent those of their affiliated organizations, or those of the publisher, the editors and the reviewers. Any product that may be evaluated in this article, or claim that may be made by its manufacturer, is not guaranteed or endorsed by the publisher.
Supplementary material
The Supplementary Material for this article can be found online at: https://www.frontiersin.org/articles/10.3389/fsufs.2023.1067506/full#supplementary-material
References
Acharya, J., Bakker, M. G., Moorman, T. B., Kaspar, T. C., Lenssen, A. W., and Robertson, A. E. (2017). Time interval between cover crop termination and planting influences corn seedling disease, plant growth, and yield. Plant Dis. 101, 591–600. doi: 10.1094/PDIS-07-16-0975-RE
Acharya, J., Kaspar, T. C., and Robertson, A. E. (2021). Effect of 6-Methoxy-2-Benzoxazolinone (MBOA) on Pythium species and corn seedling growth and disease. Plant Dis. 105, 752–757. doi: 10.1094/PDIS-04-20-0824-SC
Acharya, J., Moorman, T. B., Kaspar, T. C., Lenssen, A. W., and Robertson, A. E. (2020). Cover crop rotation effects on growth and development, seedling disease, and yield of corn and soybean. Plant Dis. 104, 677–687. doi: 10.1094/PDIS-09-19-1904-RE
Association of Official Agricultural Chemists (1990). Official Methods of Analysis of AOAC International, 15th Edn. Gaithersburg, MD: AOAC International.
Ball, D., Ballard, E., Kennedy, M., Lacefield, G., and Undersander, D. (2008). Extending Grazing and Reducing Stored Feed Needs. Bryan, TX: USDA NRCS.
Bartoń, K. (2020). MuMIn: Multi-Model Inference. Available online at: https://CRAN.R-project.org/package=MuMIn (accessed April 15, 2022).
Bates, D., Mächler, M., Bolker, B., and Walker, S. (2015). Fitting linear mixed-effects models using lme4. J. Stat. Softw. 67, 1–48. doi: 10.18637/jss.v067.i01
Blanco-Canqui, H., Shaver, T. M., Lindquist, J. L., Shapiro, C. A., Elmore, R. W., Francis, C. A., et al. (2015). Cover crops and ecosystem services: insights from studies in temperate soils. Agron. J. 107, 2449–2474. doi: 10.2134/agronj15.0086
Brown, A. R., and Almodares, A. (1976). Quantity and quality of triticale forage compared to other small grains. Agron. J. 68, 264–266. doi: 10.2134/agronj1976.00021962006800020014x
Brown, B. D. (2006). Winter cereal-corn double crop forage production and phosphorus removal. Soil Sci. Soc. Am. J. 70, 1951–1956. doi: 10.2136/sssaj2005.0288
Carr, P. M., Horsley, R. D., and Poland, W. W. (2004). Barley, oat, and cereal–pea mixtures as dryland forages in the northern Great Plains. Agron. J. 96, 677–684. doi: 10.2134/agronj2004.0677
Cherney, D. J. R., and Mertens, D. R. (1998). “Modelling grass utilization by dairy cattle,” in Grass for Dairy Cattle, eds J. H. Cherney, and D. J. R. Cherney (Wallingford: CAB International), 351–371 doi: 10.1079/9780851992884.0000
Cherney, J. H., Kallenbach, R. L., and Picasso Risso, V. D. (2020). “Systems for temperate humid areas,” in Forages: The Science of Grassland Agriculture, 7th Edn, Vol. 2, eds K. J. Moore, M. Collins, C. J. Nelson, and D. D. Redfearn (Hoboken, NJ: Wiley & Sons), 369–386 doi: 10.1002/9781119436669.ch20
Cherney, J. H., and Marten, G. C. (1982a). Small grain crop forage potential: I. Biological and chemical determinants of quality, and yield. Crop Sci. 22, 227–231. doi: 10.2135/cropsci1982.0011183X002200020007x
Cherney, J. H., and Marten, G. C. (1982b). Small grain crop forage potential: II. Interrelationships among biological, chemical, morphological, and anatomical determinants of quality. Crop Sci. 22, 240–245. doi: 10.2135/cropsci1982.0011183X002200020010x
Coblentz, W. K., Akins, M. S., Kalscheur, K. F., Brink, G. E., and Cavadini, J. S. (2018). Effects of growth stage and growing degree day accumulations on triticale forages: 1. Dry matter yield, nutritive value, and in vitro dry matter disappearance. J. Dairy Sci. 101, 8965–8985. doi: 10.3168/jds.2018-14868
Coblentz, W. K., Akins, M. S., Ogden, R. K., Bauman, L. M., and Stammer, A. J. (2019). Effects of sample size on neutral detergent fiber digestibility of triticale forages using the Ankom DaisyII incubator system. J. Dairy Sci. 102, 6987–6999. doi: 10.3168/jds.2019-16681
Darby, H. M., and Lauer, J. G. (2002). Planting date and hybrid influence on corn forage yield and quality. Agron. J. 94, 281–289. doi: 10.2134/agronj2002.2810
Daryanto, S., Fu, B., Wang, L., Jacinthe, P.-A., and Zhao, W. (2018). Quantitative synthesis on the ecosystem services of cover crops. Earth Sci. Rev. 185, 357–373. doi: 10.1016/j.earscirev.2018.06.013
Fouli, Y., Duiker, S. W., Fritton, D. D., Hall, M. H., Watson, J. E., and Johnson, D. H. (2012). Double-cropping effects on forage yield and the field water balance. Agric. Water Manag. 115, 104–117. doi: 10.1016/j.agwat.2012.08.014
Fox, J., and Weisberg, S. (2019). An R Companion to Applied Regression, 3rd Edn. Thousand Oaks, CA: Sage.
Goode, K., McCleron, K., Zhao, J., Zhang, Y., and Huo, Y. (2019). Redres: Residuals and Diagnostic Plots for Mixed Models. Available online at: https://github.com/goodekat/redres.git (accessed April 22, 2022).
Graß, R., Heuser, F., Stülpnagel, R., Piepho, H.-P., and Wachendorf, M. (2013). Energy crop production in double-cropping systems: results from an experiment at seven sites. Eur. J. Agron. 51, 120–129. doi: 10.1016/j.eja.2013.08.004
Harmoney, K. R., and Thompson, C. A. (2010). Using long-term relative yield and quality to select adapted small grain forages. For. Grazinglands 8, 1–10. doi: 10.1094/FG-2010-0125-01-RS
Heggenstaller, A. H., Anex, R. P., Liebman, M., Sundberg, D. N., and Gibson, L. R. (2008). Productivity and nutrient dynamics in bioenergy double-cropping systems. Agron. J. 100, 1740–1748. doi: 10.2134/agronj2008.0087
Heggenstaller, A. H., Liebman, M., and Anex, R. P. (2009). Growth analysis of biomass production in sole–crop and double–crop corn systems. Crop Sci. 49, 2215–2224. doi: 10.2135/cropsci2008.12.0709
Helsel, Z. R., and Thomas, J. W. (1987). Small grains for forage. J. Dairy Sci. 70, 2330–2338. doi: 10.3168/jds.S0022-0302(87)80293-X
Hyles, J., Bloomfield, M. T., Hunt, J. R., Trethowan, R. M., and Trevaskis, B. (2020). Phenology and related traits for wheat adaptation. Heredity 125, 417–430. doi: 10.1038/s41437-020-0320-1
Jemison, J. M., Darby, H. M., and Reberg-Horton, S. C. (2012). Winter grain-short season corn double crop forage production for New England. Agron. J. 104, 256–264. doi: 10.2134/agronj2011.0275
Juskiw, P. E., Helm, J. H., and Salmon, D. F. (2000). Forage yield and quality for monocrops and mixtures of small grain cereals. Crop Sci. 40, 138–147. doi: 10.2135/cropsci2000.401138x
Kaspar, T. C., and Bakker, M. G. (2015). Biomass production of 12 winter cereal cover crop cultivars and their effect on subsequent no-till corn yield. J. Soil Water Conserv. 70, 353–364. doi: 10.2489/jswc.70.6.353
Ketterings, Q. M., Ort, S., Swink, S. N., Godwin, G., Kilcer, T., Miller, J., et al. (2015). Winter cereals as double crops in corn rotations on New York dairy farms. J. Agric. Sci. 7, 18–25. doi: 10.5539/jas.v7n2p18
Khorasani, G. R., Jedel, P. E., Helm, J. H., and Kennelly, J. J. (1997). Influence of stage of maturity on yield components and chemical composition of cereal grain silages. Can. J. Anim. Sci. 77, 259–267. doi: 10.4141/A96-034
Kim, K.-S., Anderson, J. D., Newell, M. A., and Butler, T. J. (2016). Variations of forage yield and nutritive value in winter rye germplasm. Crop Sci. 56, 1018–1024. doi: 10.2135/cropsci2015.08.0487
Krueger, E. S., Ochsner, T. E., Baker, J. M., Porter, P. M., and Reicosky, D. C. (2012). Rye-corn silage double-cropping reduces corn yield but improves environmental impacts. Agron. J. 104, 888–896. doi: 10.2134/agronj2011.0341
Krueger, E. S., Ochsner, T. E., Porter, P. M., and Baker, J. M. (2011). Winter rye cover crop management influences on soil water, soil nitrate, and corn development. Agron. J. 103, 316–323. doi: 10.2134/agronj2010.0327
Kuznetsova, A., Brockhoff, P. B., and Christensen, R. H. B. (2017). lmerTest package: tests in linear mixed effects models. J. Stat. Soft. 82, 1–26. doi: 10.18637/jss.v082.i13
Landry, E., Janovicek, K., Lee, E. A., and Deen, W. (2019). Winter cereal cover crops for spring forage in temperate climates. Agron. J. 111, 217–223. doi: 10.2134/agronj2018.03.0214
Lenth, R. V. (2021). Emmeans: Estimated Marginal Means, Aka Least-Squares Means. Available online at: https://CRAN.R-project.org/package=emmeans (accessed April 18, 2022).
Lounsbury, N. P., Warren, N. D., Hobbie, J., Darby, H., Ryan, M. R., Mortensen, D. A., et al. (2022). Seed size variability has implications for achieving cover cropping goals. Agric. Environ. Lett. 7, e20080. doi: 10.1002/ael2.20080
Lyu, D., Doce, R. R., Juskiw, P., Zhou, G., and Baron, V. S. (2018). Diverse grain-filling dynamics affect harvest management of forage barley and triticale cultivars. Agron. J. 110, 1017–1027. doi: 10.2134/agronj2017.09.0549
MacDonald, J. M., Law, J., and Mosheim, R. (2020). Consolidation in U.S. Dairy Farming. USDA ERS. Available online at: https://www.ers.usda.gov/publications/pub-details/?pubid=98900 (accessed March 23, 2022).
Marten, G. C., Shenk, J. S., and Barton, F. E. II. (eds.). (1985). Near Infrared Reflectance Spectroscopy (NIRS): Analysis of Forage Quality. Agriculture Handbook No. 643. Washington, DC: U.S. Department of Agriculture, Agricultural Research Service.
McClelland, S. C., Paustian, K., and Schipanski, M. E. (2021). Management of cover crops in temperate climates influences soil organic carbon stocks: a meta-analysis. Ecol. Appl. 31, e02278. doi: 10.1002/eap.2278
McCune, B., Grace, J. B., and Urban, D. L. (2002). Analysis of Ecological Communities. Gleneden Beach, OR: MjM software design.
Mirsky, S. B., Curran, W. S., Mortensen, D. A., Ryan, M. R., and Shumway, D. L. (2009). Control of cereal rye with a roller/crimper as influenced by cover crop phenology. Agron. J. 101, 1589–1596. doi: 10.2134/agronj2009.0130
Moore, J. E., Brant, M. H., Kunkle, W. E., and Hopkins, D. I. (1999). Effects of supplementation on voluntary forage intake, diet digestibility, and animal performance. J. Anim. Sci. 77, 122. doi: 10.2527/1999.77suppl_2122x
Moore, J. E., and Undersander, D. J. (2002). “Relative forage quality: an alternative to relative feed value and quality index,” in Proceedings 13th Annual Florida Ruminant Nutrition Symposium (Gainesville, FL), 16–32
Nakagawa, S., and Schielzeth, H. (2013). A general and simple method for obtaining R2 from generalized linear mixed-effects models. Methods Ecol. Evol. 4, 133–142. doi: 10.1111/j.2041-210x.2012.00261.x
Nieuwenhuis, R., Te Grotenhuis, M., and Pelzer, B. (2012). influence.ME: tools for detecting influential data in mixed effects models. R J. 4, 38–47. doi: 10.32614/RJ-2012-011
Northeast Regional Climate Center (2022). Northeast RCC CLIMOD 2. Available online at: http://climod2.nrcc.cornell.edu/ (accessed April 27, 2022).
Pinheiro, J., Bates, D., DebRoy, S., Sarkar, D., and R Core Team (2021). nlme: Linear and Nonlinear Mixed Effects Models. Available online at: https://CRAN.R-project.org/package=nlme (accessed April 17, 2022).
Pinheiro, J. C., and Bates, D. M. (2000). Mixed-effects Models in S and S-PLUS. New York, NY: Springer-Verlag. doi: 10.1007/978-1-4419-0318-1
R Core Team (2021). R: A Language and Environment for Statistical Computing. Vienna: R Foundation for Statistical Computing. Available online at: https://www.R-project.org/ (accessed April 7, 2022).
Raimbault, B. A., Vyn, T. J., and Tollenaar, M. (1990). Corn response to rye cover crop management and spring tillage systems. Agron. J. 82, 1088–1093. doi: 10.2134/agronj1990.00021962008200060012x
Ranck, E. J., Holden, L. A., Dillon, J. A., Rotz, C. A., and Soder, K. J. (2020). Economic and environmental effects of double-cropping winter annuals and corn using the integrated farm system model. J. Dairy Sci. 103, 3804–3815. doi: 10.3168/jds.2019-17525
Rotz, C. A., Roth, G. W., and Stout, W. L. (2002). Economic and environmental implications of small grain production and use on Pennsylvania dairy farms. Appl. Eng. Agric. 18, 12. doi: 10.13031/2013.8752
Schielzeth, H., Dingemanse, N. J., Nakagawa, S., Westneat, D. F., Allegue, H., Teplitsky, C., et al. (2020). Robustness of linear mixed-effects models to violations of distributional assumptions. Methods Ecol. Evol. 11, 1141–1152. doi: 10.1111/2041-210X.13434
Slafer, G., and Rawson, H. (1994). Sensitivity of wheat phasic development to major environmental factors: a re-examination of some assumptions made by physiologists and modellers. Funct. Plant Biol. 21, 393. doi: 10.1071/PP9940393
Snapp, S. S., Swinton, S. M., Labarta, R., Mutch, D., Black, J. R., Leep, R., et al. (2005). Evaluating cover crops for benefits, costs and performance within cropping system niches. Agron. J. 97, 322–332. doi: 10.2134/agronj2005.0322a
Thapa, R., Mirsky, S. B., and Tully, K. L. (2018). Cover crops reduce nitrate leaching in agroecosystems: a global meta-analysis. J. Environ. Qual. 47, 1400–1411. doi: 10.2134/jeq2018.03.0107
Tollenaar, M., Mihajlovic, M., and Vyn, T. J. (1992). Annual phytomass production of a rye-corn double-cropping system in Ontario. Agron. J. 84, 963–967. doi: 10.2134/agronj1992.00021962008400060011x
Tumbalam, P., Hard, K., and Thelen, K. D. (2016). Integrating winter annual cereal rye or triticale into a corn forage biofuel production system. J. Crop Improv. 30, 526–540. doi: 10.1080/15427528.2016.1189472
Undersander, D., Moore, J. E., and Schneider, N. (2010). Relative Forage Quality. Madison, WI: University of Wisconsin.
United States Department of Agriculture, Agricultural Research Service (2012). USDA Plant Hardiness Zone Map. Available online at: https://planthardiness.ars.usda.gov/ (accessed April 20, 2022).
Veltman, K., Rotz, C. A., Chase, L., Cooper, J., Ingraham, P., Izaurralde, R. C., et al. (2018). A quantitative assessment of beneficial management practices to reduce carbon and reactive nitrogen footprints and phosphorus losses on dairy farms in the US Great Lakes region. Agric. Syst. 166, 10–25. doi: 10.1016/j.agsy.2018.07.005
von Keyserlingk, M. A. G., Martin, N. P., Kebreab, E., Knowlton, K. F., Grant, R. J., Stephenson, M., et al. (2013). Invited review: Sustainability of the US dairy industry. J. Dairy Sci. 96, 5405–5425. doi: 10.3168/jds.2012-6354
von Keyserlingk, M. A. G., Rushen, J., de Passillé, A. M., and Weary, D. M. (2009). Invited review: the welfare of dairy cattle—key concepts and the role of science. J. Dairy Sci. 92, 4101–4111. doi: 10.3168/jds.2009-2326
Wannasek, L., Ortner, M., Kaul, H.-P., Amon, B., and Amon, T. (2019). Double-cropping systems based on rye, maize and sorghum: impact of variety and harvesting time on biomass and biogas yield. Eur. J. Agron. 110, 125934. doi: 10.1016/j.eja.2019.125934
West, J. R., Ruark, M. D., and Shelley, K. B. (2020). Sustainable intensification of corn silage cropping systems with winter rye. Agron. Sustain. Dev. 40, 11. doi: 10.1007/s13593-020-00615-6
Wood, S. A., and Bowman, M. (2021). Large-scale farmer-led experiment demonstrates positive impact of cover crops on multiple soil health indicators. Nat. Food 2, 97–103. doi: 10.1038/s43016-021-00222-y
Keywords: barley, cereal rye, double-cropping, harvest management, nutritive value, organic, phenology, triticale
Citation: Liebert J, Cherney JH, Ketterings QM, Mirsky SB, Pelzer CJ and Ryan MR (2023) Winter cereal species, cultivar, and harvest timing affect trade-offs between forage quality and yield. Front. Sustain. Food Syst. 7:1067506. doi: 10.3389/fsufs.2023.1067506
Received: 11 October 2022; Accepted: 09 February 2023;
Published: 21 March 2023.
Edited by:
Carla Pinheiro, New University of Lisbon, PortugalReviewed by:
Marcin Studnicki, Warsaw University of Life Sciences, PolandAmanda Grev, University of Maryland, United States
Copyright © 2023 Liebert, Cherney, Ketterings, Mirsky, Pelzer and Ryan. This is an open-access article distributed under the terms of the Creative Commons Attribution License (CC BY). The use, distribution or reproduction in other forums is permitted, provided the original author(s) and the copyright owner(s) are credited and that the original publication in this journal is cited, in accordance with accepted academic practice. No use, distribution or reproduction is permitted which does not comply with these terms.
*Correspondence: Jeffrey Liebert, amFsNDg1QGNvcm5lbGwuZWR1