Effects of agroforestry on grain yield of maize (Zea mays L.)—A global meta-analysis
- 1Institute of Agricultural and Nutritional Sciences, Soil Biogeochemistry, Martin Luther University Halle-Wittenberg, Halle, Germany
- 2German Agency for International Cooperation (GIZ), Bonn, Germany
Maize cropping systems, the world's most important cropping systems in terms of production volume, are responsible for many environmental problems, such as soil degradation and erosion. This calls for sustainable practises that enable environment-friendly production while generating sufficient output, as farmers and consumers worldwide depend on adequate yields. Agroforestry can offer solutions to both of these pressing concerns—food insecurity and adverse environmental impacts of intensive maize cultivation. This meta-analysis investigated the effects of agroforestry on maize (Zea mays L.) grain yield under different environmental and management regimes on a global scale. It is based on 1,215 pairwise data entries from 95 peer-reviewed studies. Overall, agroforestry increased median maize yields by 0.24 Mg ha−1 (7%) compared to tree/hedgerow-free maize monocultures. In subtropical and tropical regions, the median yield increment under agroforestry was 0.30 Mg ha−1 (+16%), and the best results were achieved using broadleaved trees (+0.42 Mg ha−1, +53%), especially N-fixing tree species (+0.56 Mg ha−1, +60%). Maize yields responded very well to the addition of tree prunings to the soil of the maize crop (+0.48 Mg ha−1, +24%). Rotating maize with other crops was beneficial if the intermediate crop is able to fix atmospheric N (+0.28 Mg ha−1, +13%). We found that hedgerow planting densities of at least 5,000 woody perennials ha−1 are required for the positive impacts of agroforestry on maize yields to take effect, and these effects increased with both tree age (+1.17 Mg ha−1, +48% under trees 11–15 years of age) and time under agroforestry (+0.85 Mg ha−1, 81% in response to >15 continuous maize seasons under agroforestry). In addition, they were particularly pronounced on sandy soils (+0.46 Mg ha−1, +23%), moderately acidic soils of pH 5–6 (+0.67 Mg ha−1, +35%), soils with <0.5 g kg−1 N (+1.19 Mg ha−1, +81%), soils with a plant-available water holding capacity <10 mm dm−1 (+1.10 Mg ha−1, +44%), and hyper-arid conditions (+0.60 Mg ha−1, +35%). These findings prove that if designed with due regard for local conditions and crop/tree species characteristics, agroforestry can be a powerful tool to increase food security and the sustainability of maize production systems.
1. Introduction
As a global society, we currently face several urgent crises, such as environmental degradation, loss of biodiversity, climate change, food insecurity, and persisting poverty as a whole—all of which at least partly need to be addressed through agriculture (Schulte et al., 2022). These crises prevent the Sustainable Development Goals (SDGs) from being achieved by 2030. Offsetting previous achievements (Osgood-Zimmerman et al., 2018), the COVID-19 pandemic increased the number of people facing hunger by about 150 million between 2019 and 2021, representing a rise in the prevalence of undernourishment of 1.8% (FAO et al., 2022). This development brings the world off track to achieve SDG 2 (zero hunger) and highlights the need to reform our agrifood-systems (FAO, 2017; El Bilali et al., 2019; FAO et al., 2022), especially considering that the natural resources for human nutrition are diminishing (Cole et al., 2018).
Crucially, our food systems both suffer from and are drivers of resource scarcity, ecosystem degradation, and climate change (El Bilali et al., 2019). The global food system is today's second largest greenhouse gas-emitting sector (IPCC, 2014): Recent estimates attribute about a third of all greenhouse gas (GHG) emissions to worldwide food systems (Crippa et al., 2021; Development Initiatives, 2021), 71% of which are contributed by agriculture and land use/land use change activities (Crippa et al., 2021). Global food production also causes by far the most biodiversity loss, terrestrial ecosystem destruction, freshwater consumption, and water pollution resulting from overuse of N and P. At the same time, food production is the first victim of the environmental pressures associated with the Anthropocene (Rockström et al., 2020). El Bilali et al. (2019) stressed that food sustainability and food security are inextricably linked yet too often discussed as separate concepts in attempts to solve the problems currently generated by conventional food systems. In fact, long-term food and nutrition security are among the main outcomes of sustainable food systems (El Bilali et al., 2019).
A key requirement to achieve food sustainability and thus achieve SDG 2 is a multi-functional agricultural system that is not only increasingly productive, but also contributes to environmental and social targets (Waldron et al., 2017; Nair et al., 2021) and thereby helps achieving other SDGs as well, such as SDG 15 (Life on Land) and 17 (Partnerships to achieve the Goal). One of the most multifunctional forms of agriculture is agroforestry (Waldron et al., 2017)—the purposeful combination of trees, agricultural crops, and sometimes livestock on the same piece of land. This includes the integration of trees and woody perennials into farms and agricultural landscapes, farming in forests and along forest margins, and the agricultural use of trees, e.g., cocoa (Theobroma cacao), coffee (mainly Coffea arabica and Coffea canephora), rubber (Hevea brasiliensis), or oil palm (Elaeis guineensis) (Waldron et al., 2017; World Agroforestry, 2021). Besides the use of individual trees that are spaced widely enough to develop discernible crowns, agroforestry also extends to densely planted hedgerows kept at low height.
Agroforestry can be instrumental in counteracting land degradation/desertification, promoting biodiversity, alleviating poverty and associated injustices, providing ecosystem services, and mitigating the impact of climate change (Dagar and Tewari, 2017; Udawatta and Jose, 2021). It has also been proven to be able to increase crop yields (Kuyah et al., 2019; Piato et al., 2020; Koutouleas et al., 2022). However, generalisations of yield responses to tree-crop-soil-interactions within agroforestry systems are difficult to formulate and can easily be misleading, as these interactions are “inherently location-specific and management-sensitive” (Nair et al., 2021). Major avenues that may produce both positive and negative effects on crop yields include interactions related to soil fertility, competition for resources such as sunlight, water, and nutrients with trees and weeds, microclimate, pests, and diseases (Rao et al., 1997).
While several meta-analyses have investigated agroforestry effects on yields and quality of high-value crops that mainly proceed to be manufactured into luxury goods in the Global North, such as coffee or cocoa (Niether et al., 2020; Koutouleas et al., 2022; Mokondoko et al., 2022), insights on staple crops that play a major role in diets of the Global South are less comprehensively summarised. Addressing this gap, our meta-analysis investigated the effects of agroforestry on grain yields of maize (Zea mays L.) at a global scale. Maize, along with rice (Oryza sativa) and wheat (Triticum), is one of the world's three most important staple crops in human diets. What distinguishes maize from wheat and rice is its versatility as a multi-purpose crop (Nuss and Tanumihardjo, 2010; Erenstein et al., 2022). While it contributes more than 20% of all calories to human diets in Sub-Saharan Africa (SSA), Latin America, and parts of Asia (Shiferaw et al., 2011), its popularity is also a result of its suitability as a livestock feed crop as well as an industrial and energy crop (Nuss and Tanumihardjo, 2010; Erenstein et al., 2022). Notably, maize is the preferred staple to more than 900 million people suffering from poverty in the Global South (Shiferaw et al., 2011). It is also essential to the livelihoods of millions of rural farmers who are considered poor (Shiferaw et al., 2011). Especially in Africa and Latin America, maize is largely produced by smallholder farms (Tanumihardjo et al., 2020). At the same time, the demand for maize in the Global South has been projected to rise as a result of human population growth (Shiferaw et al., 2011). According to current projections, SSA, for instance, will need to increase maize outputs up to 4 fold to self-sufficiently meet its demand by 2050 (Berge et al., 2019). In temperate regions, adoption of agroforestry practises is mainly motivated by environmental benefits such as protection of soil and water quality or promotion of biodiversity in agricultural landscapes (Nair et al., 2021)—particularly with view to the considerable environmental impact of common farming practises, e.g., related to organic fertiliser application in intensive maize production systems (Kim et al., 2009). Another major environmental disadvantage of maize production is an increased risk of soil erosion, a result of low vegetative cover due to the linear structure and wide spacing of the maize rows (Vogel et al., 2016).
Our analysis aimed to provide a quantification of how maize grain yields responded to differently designed agroforestry trials across a wide range of environments. To this end, the analysis presents the response ratio of maize grain yields influenced by agroforestry and the absolute difference in maize grain yields between agroforestry and sole cropping (hereinafter control), both of which are entirely based on data extracted from peer-reviewed studies (ISI Web of Science) published between 1990 and 2021. To gain insights into the impact of a set of additional environmental factors and experiment characteristics, yields were grouped and analysed by a variety of factors related to climate, soil fertility, and trial design. The overall objective was to provide decision-makers with scientifically substantiated recommendations regarding maize cultivation in agroforestry settings.
2. Materials and methods
2.1. Data source and selection
The process of literature screening is visualised in Figure 1. Studies qualified for this analysis if they performed growth experiments under field conditions, reported maize yields in weight per unit area obtained in both an agroforestry and a valid control setting, and if the agroforestry component consisted of living trees or woody plants. Control settings were considered valid if they consisted purely of maize cultivation (no trees or intercrop) and received the exact same agronomic treatment as the agroforestry plot(s). If studies did not mention any differences in management either, they were considered suitable.
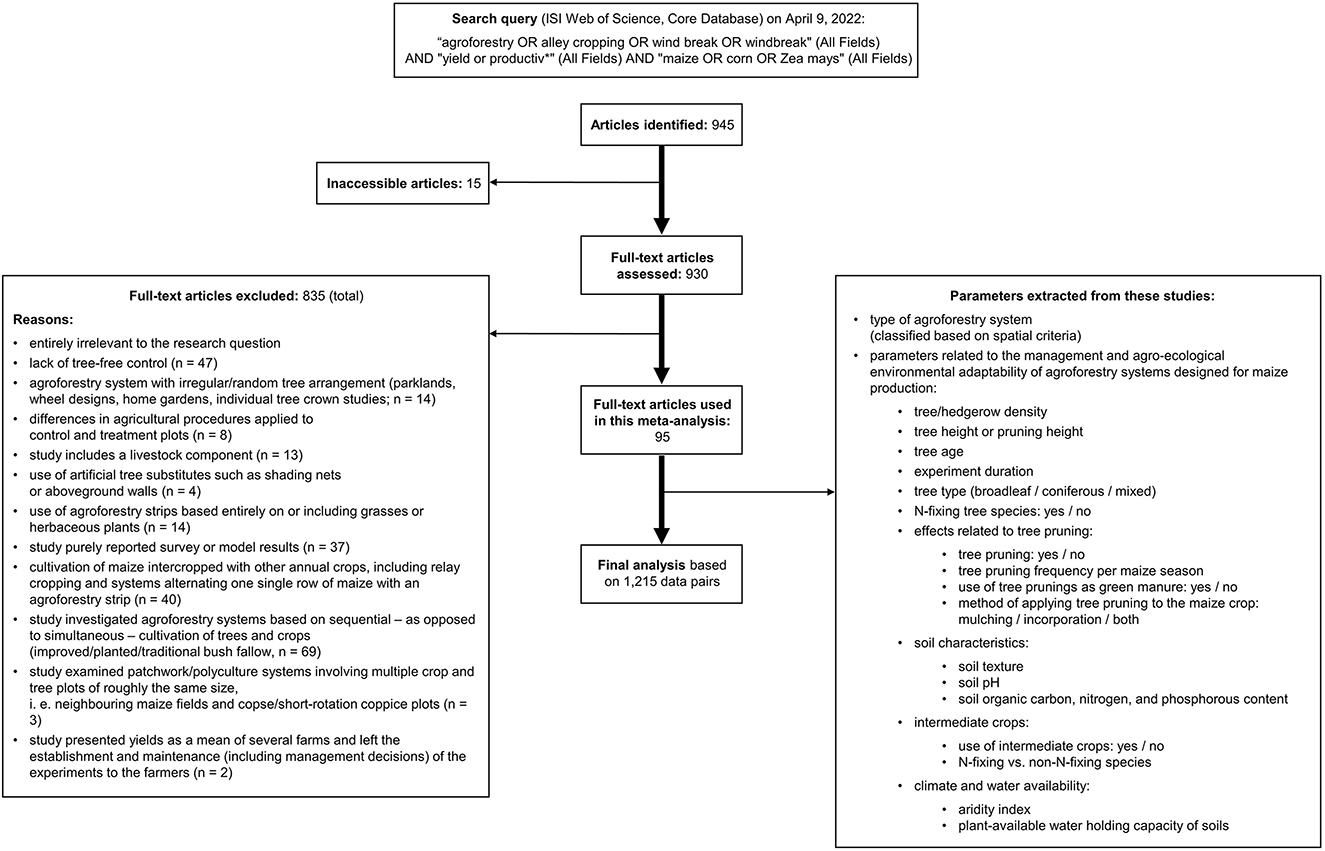
Figure 1. PRISMA diagramme—graphic description of the literature search and article selection process.
Not included were studies that lack a tree-free control (n = 47); applied different agricultural procedures to control and treatment plots (n = 8); include a livestock component (n = 13); used artificial tree substitutes such as shading nets or aboveground walls (n = 4), used agroforestry strips based entirely on or including grasses or herbaceous plants (n = 14); purely reported survey or model results (n = 37); cultivated maize intercropped with other annual crops, including relay cropping and systems alternating one single row of maize with an agroforestry strip (n = 40); investigated separate—as opposed to intermittent—agroforestry systems (improved/planted/traditional bush fallow, n = 69); or examined patchwork/polyculture systems involving multiple crop and tree plots of roughly the same size, i.e., neighbouring maize fields and copse/short-rotation coppice plots (n = 3).
Also excluded were studies that presented yields as a mean of several farms and that left the establishment and maintenance of the experiments to the farmers. In these cases, the unquantifiable risks of spatial heterogeneity in the environmental factors influencing yields as well as in the management decisions and implementation was considered too high.
Furthermore, studies were required to feature an agroforestry setting characterised by a certain degree of regularity in the planting pattern of the agroforestry component. More specifically, agroforestry systems such as parklands (trees that are randomly scattered within agricultural fields), wheel designs, home-gardens, or investigations into the effects of individual tree crowns are not represented in this analysis. For a complete list of the studies included in this analysis, see the Supplementary Table S1.
The majority (87%) of studies included in this meta-analysis reported maize grain yields per area, which were extracted and used to quantify both absolute and relative effects. In the absence of yields per area, only relative changes in yield were extracted. This also applies to studies that reported yields per area, but did not include grain yields (Supplementary Table S1).
If data on yields or site/experiment characteristics were only presented in graphs, WebPlotDigitizer version 4.6 (Rohatgi, 2020) was used to extract precise values.
2.2. Data categorisation
2.2.1. Experiment characteristics
Our analysis included several variables related to the design and implementation of the experiments. As for agroforestry systems, this study distinguished between three different types: alley cropping (strip arrangement) on level to near-level fields, alley cropping (strip arrangement) on sloping fields, and single-row systems (boundary arrangement). Alley cropping includes all systems containing a minimum of two tree rows, which were divided into two spatial categories based on whether they were established on (near-) planar fields or slopes. The category “single-row” combines all systems characterised by one single row of trees—many, but not all of which were designed as windbreaks. This category also included studies that used the term “alley cropping” for systems containing only one row.
Tree and hedgerow density were expressed as trees ha−1 and woody perennials ha−1, respectively. We used the term “tree” only in systems containing <5,000 trees ha−1, whereas all densities exceeding this threshold were classified as “hedgerows”. Densely planted hedgerows, which were often kept at low height, were particularly common in tropical agroforestry. See the Supplementary material for more details on this classification.
2.2.2. Soil and climate characteristics
Furthermore, various variables related to soil physical and chemical properties were extracted from the studies. These include soil texture, sand and clay content in %, bulk density in g cm−3, soil pH, soil organic carbon (SOC) and total N in g kg−1, and extractable P in mg kg−1. Where more than one option was given, we always used the values relating to the control site and/or at the start of the experiment to characterise the initial situation rather than the influence of agroforestry on soil quality (the latter was of value for the discussion of our results). If the naturally occurring initial situation was significantly altered prior to the start of the experiment, e.g., by applying substantial amounts of lime, we used the values obtained after this operation. Out of 46 studies that provided information on soil sampling depth, the majority (39 studies) reported soil properties in relation to one particular sampling depth, which amounted to 26 cm on average. The remaining studies (n = 7) sampled at several depth intervals. In these cases, mean values were extracted for the depth range closest to the mean sampling depth of the remaining studies.
Soil texture was classified by dominant particle size class (sand, clay, silt) and the intermediate category “loam,” added to represent the classes “sandy loam” and “clay loam,” using the triplet coordinate system of the World Reference Base (IUSS Working Group WRB, 2015). Soil pH, SOC content, total N content, and extractable P content were grouped as follows:
• pH: <5, 5–6, >6–7, >7.
• SOC [g kg−1]: <5, 5–10, >10–20, >20–30, >30.
• Total N [g kg−1]: <0.5, 0.5–1.0, >1.0–5.0, >5.0.
• Plant-available P [mg kg−1]: <5, 5–15, >15–30, >30.
Plant-available water holding capacity θAWHC was determined using four pedotransfer functions (Equations 4–9) developed by Bagnall et al. (2022). Details on this procedure are provided in the Supplementary material.
The aridity index AI of the study locations was extracted from a global dataset provided by Zomer et al. (2022) using QGIS 3.16.0 (QGIS Development Team, 2020). The index expresses the ratio of precipitation over evapotranspiration:
where MAPrec is the mean annual precipitation [mm] and MAET0 is the mean annual FAO Reference Evapotranspiration after Penman-Monteith [mm]. By relating precipitation (incoming moisture) to atmospheric water content (outgoing moisture), the index quantifies water availability for plant growth (Zomer et al., 2022). The resulting values were classified based on a scheme provided by the United Nations Environment Programme (1997) as detailed in Table 1.
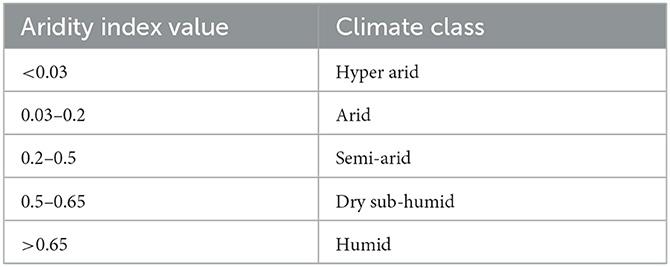
Table 1. Climate classification scheme for aridity index values used in this analysis, provided by the United Nations Environment Programme (1997) and cited in Zomer et al. (2022).
We also distinguished between values from tropical and temperate studies using the exact locations and climate specifications provided by the studies. As there were only three studies with a total of six values from subtropical locations, these entries were classified as tropical rather than assigned to a distinct category.
2.3. Data analysis
Two different indices were used to estimate the effect of agroforestry on maize yields—one for relative and one for absolute changes. Relative effects are represented by the dimensionless response ratio R:
where XE is the maize yield [Mg ha−1] obtained in an experimental agroforestry setting, XC is the maize yield [Mg ha−1] of the experimental control group, and the resulting ratio of both is subtracted by 1 for the purpose of facilitated interpretation.
The absolute yield difference yield [Mg ha−1] was calculated using the following equation:
where XE is the maize yield [Mg ha−1] under agroforestry and XC is the maize yield [Mg ha−1] of the experimental control.
The results of these calculations are presented as notched boxplots that visualise the 95% confidence interval (CI) of the median, represented by the extent of the notches, as follows:
where R is the dimensionless median response ratio, yield is the absolute difference in maize yields in Mg ha−1, 1.57 is the confidence coefficient, IQR is the interquartile range, and n is the number of individual values (McGill et al., 1978).
The vertical grey solid line in all boxplots represents R or yield = 0, which translates into no relative/absolute effect of agroforestry on maize grain yields, being equal to the “zero-effect-line.” Positive effects (increase in maize yields under the influence of agroforestry) are achieved if R>0, while R < 0 is indicative of negative effects (decrease in maize yields under the influence of agroforestry). If the range of the upper and lower 95% confidence interval, i.e., the notch, crosses the grey zero-effect-line, the result can be interpreted as statistically insignificant. The median effect size of each group is considered to be significantly different from each other at p < 0.05, if the notches do not overlap. The number in parentheses denotes the number of data entries this value is based on. For the purpose of enhanced readability, we visually removed all data points that exceeded the whiskers (i.e., the lower and upper quartile of the data, limited to 1.5 times the interquartile range) from the boxplots. This modification was purely visual; the underlying dataset and, thereby, the statistics provided were not altered in any way. The Supplementary material contains the original plots including all outliers (Supplementary Figures S4–S10).
We tested the yield data for normal distribution via Shapiro-Wilk Normality Test. It was not normally distributed (p < 2.2 × 10−16), which led us to use the Kruskal-Wallis-Test (α = 0.05) to establish whether there are significant differences between groups. The results of this test were visualised in the boxplots (***for p < 0.001, **for p < 0.01, and *for p < 0.05). The lack of a normal distribution of the yield data is the reason why we consistently use the median instead of the mean to visualise and compare both absolute and relative yield responses. We also did not transform our data because in that case, results would be only valid for this transformed data but not for the original dataset, especially not when back-transformed for visualisation reasons as done in most meta-analysis studies. This meta-analysis does not apply a differentiating weighting factor, which is an approach that allows us to include as many data entries as possible.
All calculations were performed using Microsoft Excel (2021) (Microsoft Corporation, 2021). The results were statistically analysed and visualised using R version 4.1.2 (RStudio Team, 2021).
3. Results and discussion
This meta-analysis systematically summarised the findings of 95 studies with a total of 1,215 treatment-control data pairs. The individual study sites were in North America (n = 6), Middle and South America (n = 15), Europe and the Middle East (n = 3), Sub-Saharan Africa (n = 58), and Asia (n = 13) (Figure 2).
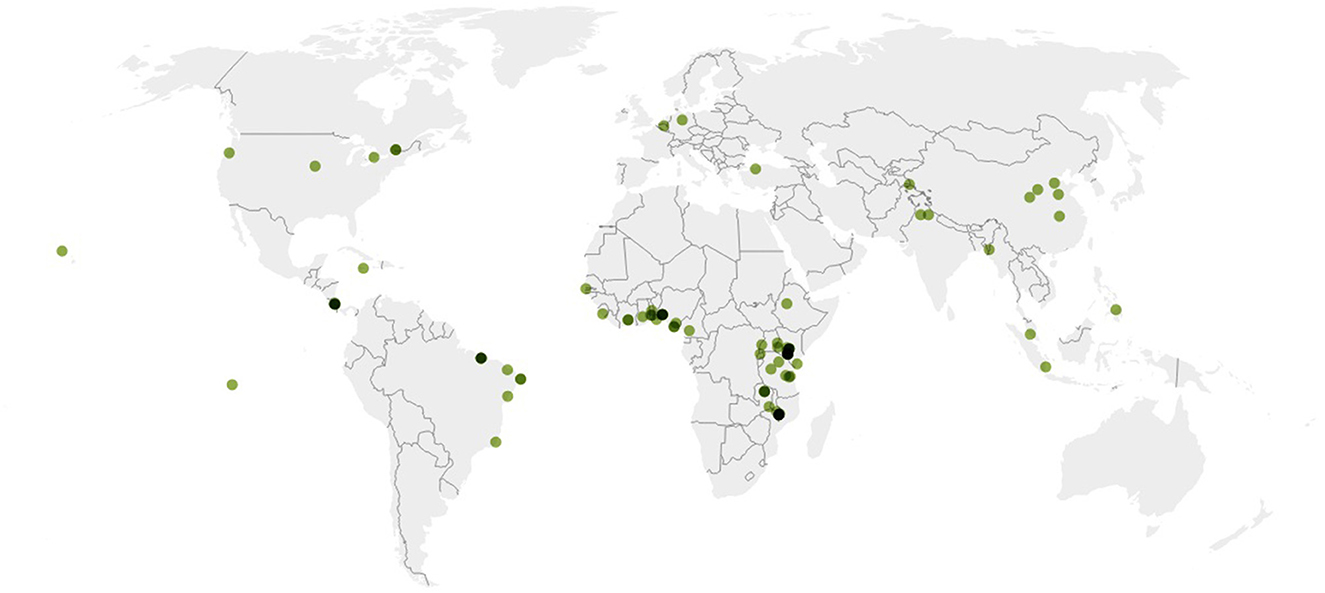
Figure 2. Global distribution of study sites represented in this meta-analysis (map created using Datawrapper).
The agroforestry plots produced a median maize grain yield of 2.6 Mg ha−1, which is significantly more than the median yield of 1.9 Mg ha−1 achieved by the control plots (Figure 3). Regarding the response of maize grain yields to agroforestry, the grand median amounted to an absolute yield difference of 0.24 Mg ha−1 and a response ratio of 7%.
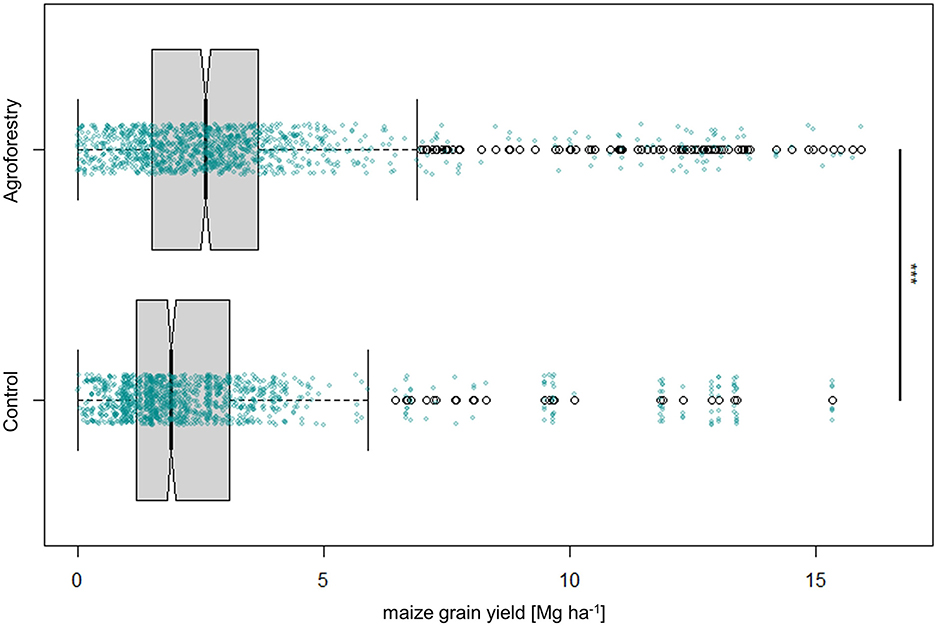
Figure 3. Maize grain yield [Mg ha−1] of control (maize) and agroforestry plots. Each box contains the middle 50% of the data of a category. The median of the data is shown as a vertical solid line within the box. Notches within boxes represent the 95% confidence interval of the median. The whiskers indicate the lower and upper quartile of the data, respectively, and are limited to 1.5 times the interquartile range. Green dots represent each data point that was included in the calculation, whereas white dots represent outliers that exceed the whiskers. The solid black line annotated with stars to the right of the plot indicates a statistically significant difference between groups according to the Kruskal-Wallis-Test (***for p < 0.001).
3.1. Effects of agroforestry systems
As visualised in Figure 4, alley cropping on sloping fields outperformed the other systems at a median yield increase of 0.30 Mg ha−1, closely followed by alley cropping on (near-)level fields at 0.25 Mg ha−1. Single-row systems also produced a positive result at 0.09 Mg ha−1. Alley cropping on (near-)level and sloping fields increased maize yields by 12 and 9%, respectively, in contrast to an almost imperceptible 0.5% in single-row systems. Overall, only alley cropping on (near-)level fields showed a statistically significant effect on maize yields, and the ecologically relevant differences between systems were very small.
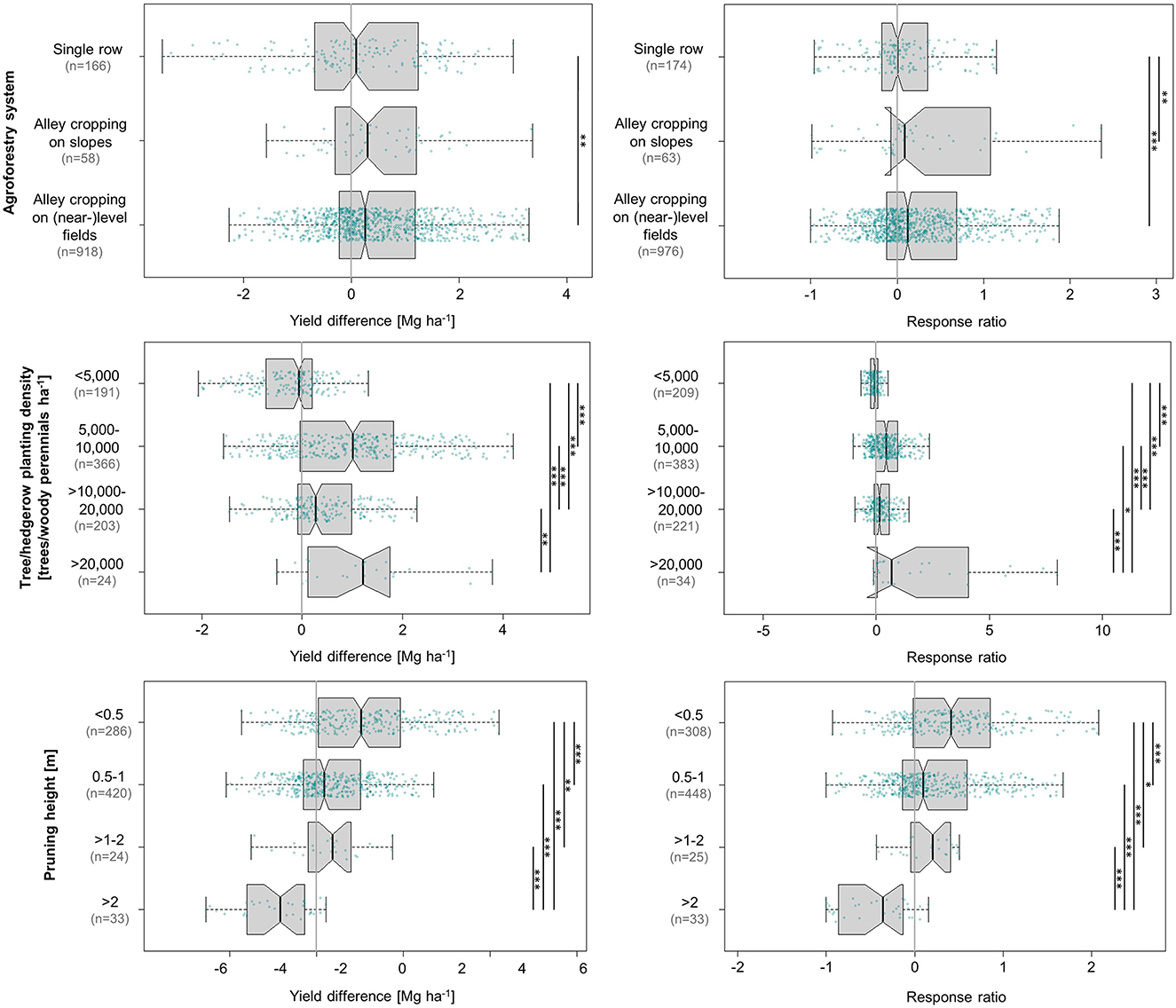
Figure 4. Influence of agroforestry system, tree/hedgerow planting density, and pruning height on absolute (left) and relative (right) differences in maize grain yield achieved under agroforestry as compared to the control plots (only maize). Each box contains the middle 50% of the data of a category. The median of the data is shown as a vertical solid line within the box. Notches represent the 95% confidence interval of the median. The whiskers indicate the lower and upper quartile of the data, respectively, and are limited to 1.5 times the interquartile range. Green dots represent the individual data points that were included in the calculation. Data points exceeding the whiskers were removed visually, without alteration of the underlying dataset. The number of values per group is given in parentheses, with the first one relating to the yield difference in Mg ha−1 and the second one to the response ratio. The solid black lines annotated with stars to the right of the plot indicate statistically significant differences between groups according to the Kruskal-Wallis-Test (***for p < 0.001, **for p < 0.01, and *for p < 0.05).
Alley cropping systems on slopes are common in tropical and subtropical highlands (Nair et al., 2021), such as the East African highlands or the semi-arid ravines of West India, where intensive land use on sloping terrain coupled with high rainfall and population pressure accelerates soil erosion rates, reducing soil fertility and crop productivity (O'Neill et al., 2002; Guto et al., 2011; Jinger et al., 2022). A global review found that relative erosion-induced yield losses are two-six times smaller in North America and Europe than in Africa, Asia, Australia, and Latin America (den Biggelaar et al., 2003). Their higher losses are primarily a result of much lower average yields, i.e., identical amounts of erosion lead to more rapid yield declines in relative terms (den Biggelaar et al., 2003). Agroforestry, however, has been proven to be an effective tool in counteracting erosion: A meta-analysis of 17 tropical studies showed that soil erosion rates were significantly lower under agroforestry than in crop monocultures in all studies. Overall, the trees reduced soil erosion by 50%. Infiltration rates were 75% higher under agroforestry, runoff was reduced by 57%, and soil macroaggregates >0.25 mm were increased by 22% (Muchane et al., 2020). In consequence, higher nutrient availability and enhanced moisture retention in alley cropping systems on slopes could explain the positive yield responses to these systems observed in our analysis.
The positive results of single row systems—many of which were designed as windbreaks or shelterbelts and, thereby, also represent a functional category—are likely a result of improved microclimatic growing conditions for crops. Tree windbreaks or shelterbelts, i.e., single or multiple tree lines established at right angles to the prevailing wind direction or along field boundaries, are a common form of agroforestry in temperate regions, although they are also used in the tropics (Nair et al., 2021). In fact, the single-row studies included in this meta-analysis (n = 8) are a 50/50-mixture of tropical and temperate studies. Historically, shelterbelts originated from the Great Plains of North America (Nair et al., 2021), but they were also strongly promoted, for instance, across the Soviet Union (Thevs et al., 2017). Their primary objective is to enhance crop growth by manipulation of plants and soil microclimate (Takle, 2015). The rule of thumb for windbreaks is that the area protected from wind erosion extends laterally to 10 times the height of the tallest (central) row of trees in the barriers (Nair et al., 2021). Another major benefit of reduced wind speed in water-limited systems is enhanced water productivity of crops, i.e., alleviated moisture stress (Easterling, 1997; Thevs et al., 2017). Thevs et al. (2017) found maize water consumption to be reduced by 6% under the influence of a shelterbelt compared to open-field conditions on a field size of 500 × 500 m in semi-arid Central Asia. This reduction in water consumption corresponds to a literature review-based scenario of a shelterbelt-induced reduction of wind speed by 35%, a decrease in mean air temperature by 1°C, and an increase in air humidity by 5% compared to open field conditions. In a similar study, the water consumption of maize was reduced by 49% in a 50 × 50 m grid of multiple row elm tree windbreaks, while a windbreak grid of 100 × 100 m still reduced maize water consumption by 27%. Furthermore, shelterbelts can increase soil moisture by acting as snow traps (Thevs et al., 2017). These effects may be most important during the maize seedling stage, as severe water stress during this phase in development has a higher impact on plant growth and development than stress that occurs during the later stages of phenological development (jointing, heading, and grain-filling) (Song et al., 2019). With view to global warming, modelling has projected the beneficial microclimate effects of shelterbelts on dryland maize to be greatest for the most severe scenarios of change, i.e., maximum precipitation deficiency and greatest increase in windspeed (Easterling, 1997), highlighting their potential as an adaptive strategy.
3.2. Effects of tree/hedgerow planting density
The success of agroforestry systems aimed at crop yield improvement generally depends on a favourable balance between negative effects related to tree-crop-competition for growth resources and mechanisms by which trees promote crop development. Maize yield responses to varying tree/hedgerow planting densities essentially indicate which of these yield-determining mechanisms prevails at different intensities of integrating woody perennials into the system. This analysis revealed the outcome to be partly system-specific and not entirely intuitive. One would assume that competition effects gain in relevance and, thereby, increasingly diminish crop yields with rising tree/hedgerow density. This is indeed reflected by the fact that densities of >10,000–20,000 woody perennials ha−1 resulted in a distinctly lower median yield increase (0.14 Mg ha−1) than 5,000–10,000 woody perennials ha−1 (0.44 Mg ha−1; Figure 4). The high relative and absolute yield increase registered at a hedgerow density >20,000 woody perennials ha−1 (1.2 Mg ha−1, 68%) was largely (19 out of the total 24 values) based on alley cropping systems on slopes.
In addition, the values mostly originated from one study-−15 values were extracted from Banda et al. (1994), who achieved consistently high yield increases of up to 3.8 Mg ha−1 compared to the grand median of +0.24 Mg ha−1. This suggests that alley cropping on slopes may support much higher densities of woody perennials than alley cropping on planar areas of land. The well-substantiated (n = 191/209) slightly negative response of −0.05 Mg ha−1 (−0.07%) at <5,000 trees ha−1 may indicate that it takes a certain tree population for any mentionable impact in either direction to come into effect. One reason why very low tree densities can have negative effects on crop yields is that trees planted at wide distances tend to exhibit rapid root growth (Lawson et al., 2019). This leads to their fine roots quickly inhabiting the uppermost soil horizons (Lawson et al., 2019), where they compete with crops for growth resources.
In the context of alley cropping on flat land, one specific trial design used in five of the Makoka studies (Akinnifesi et al., 2006, 2007; Makumba et al., 2006; Mweta et al., 2007; Sileshi et al., 2012) proved to be particularly successful. In these experiments, the tree species gliricidia (Gliricidia sepium) was planted in furrows spaced 0.9 m within and 1.5 m between rows (7,400 woody perennials ha−1). According to the authors, this design “eliminates the hedge-effect” as a constraint to maize productivity. Combined with three prunings per cropping season at 0.3 m height and subsequent incorporation of the pruned materials for approx. 80% of all values, the five trials (98 values) based on this design achieved a comparatively high median absolute yield increase of 2.23 Mg ha−1.
3.3. Effects of tree characteristics
3.3.1. Effects of nitrogen-fixing trees
At a significant median grain yield increase of 0.6 Mg ha−1, the absolute response of maize yields to tree influence was very clearly in favour of trees with N-fixing symbionts, which constituted the majority at 67% of all values (Figure 5). The alternative, trees that do not contribute to biological N-fixation, produced a non-significant yield difference of nearly zero (0.01 Mg ha−1). This pattern was also reflected—albeit less distinctly—in the relative response at 60% response to N-fixing and 30% response to non-N-fixing tree species. The responses to N-fixing and non-N-fixing trees were statistically different from each other, which applies to both absolute and relative effects.
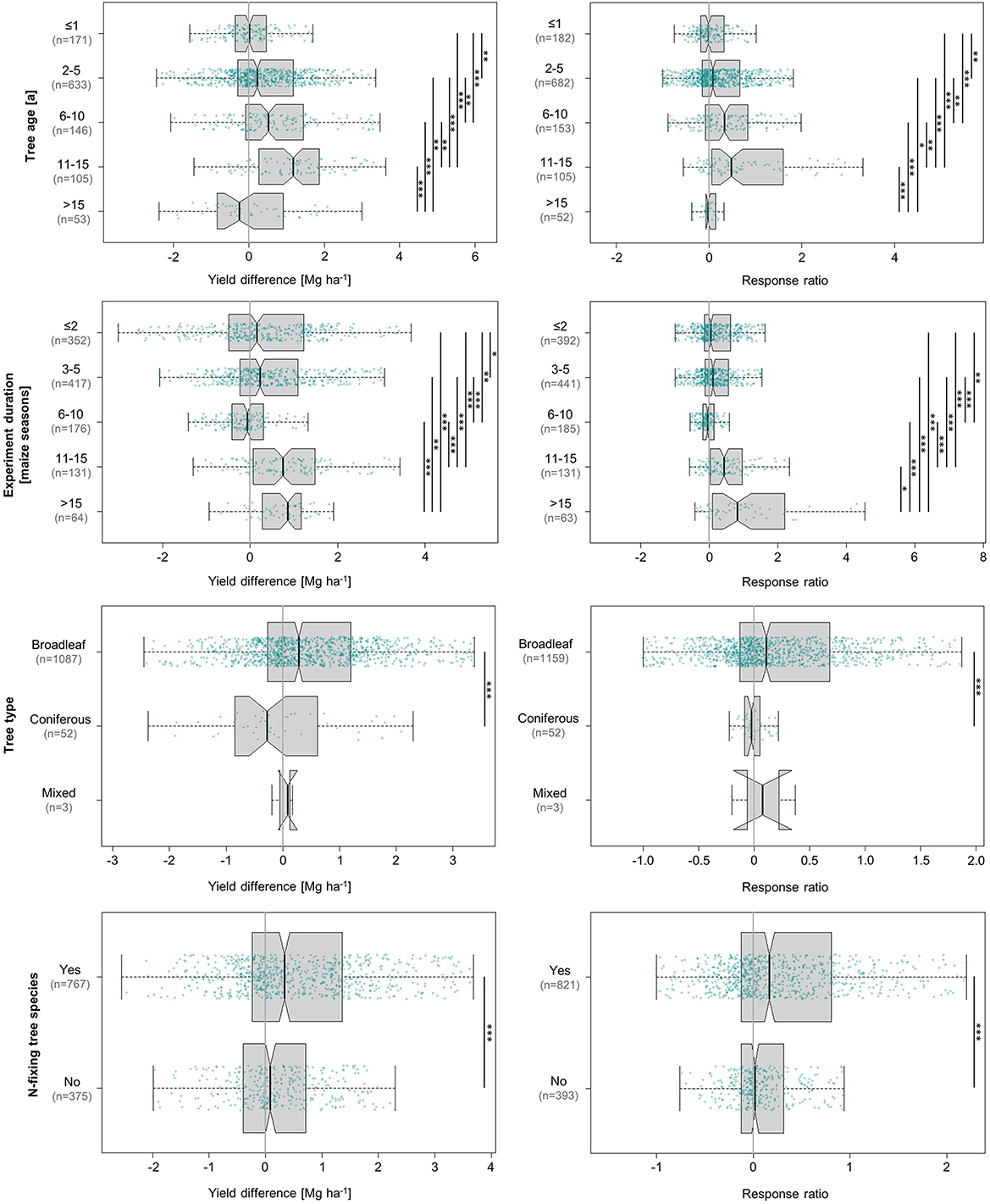
Figure 5. Influence of tree age, experiment duration, tree leaf anatomy, and N-fixing tree species on absolute (left) and relative (right) differences in maize grain yield achieved under agroforestry as compared to the control plots (sole maize). Each box contains the middle 50% of the data of a category. The median of the data is shown as a vertical solid line within the box. Notches represent the 95% confidence interval of the median. The whiskers indicate the lower and upper quartile of the data, respectively, and are limited to 1.5 times the interquartile range. Green dots represent the individual data points that were included in the calculation. Data points exceeding the whiskers were removed visually, without alteration of the underlying dataset. The number of values per group is given in parentheses, with the first one relating to the yield difference in Mg ha−1 and the second one to the response ratio. The solid black lines annotated with stars to the right of the plot indicate statistically significant differences between groups according to the Kruskal-Wallis-Test (***for p < 0.001, **for p < 0.01, and *for p < 0.05).
This quantified relationship consolidates and adds to well-established knowledge, given the long-standing recognition of biologically N-fixing trees as major assets to agrisilvicultural systems. Numerous studies conducted since the 1980s have supported the finding that biologically N-fixing trees predominantly outperform those unable to biologically fix N in terms of improving the yields of the associated crop (Nair et al., 2021). They are commonly referred to as “fertiliser trees” in light of their considerable capacity to increase plant-available N (Sileshi et al., 2014). Importantly, these effects require that pruned tree material be added to the cropped area as fertiliser, as our study revealed.
3.3.2. Effects of tree type: broadleaved vs. coniferous trees
Broadleaved trees, which represented 95% of the total dataset, significantly increased maize grain yields by an average of 0.42 Mg ha−1 (53% response ratio). In contrast, yields influenced by coniferous trees decreased by almost the same amount at−0.44 Mg ha−1, corresponding to a relative loss of 4% (Figure 5). Mixed stands were only represented by three values from one study of a single-row system in Nebraska, USA. They showed an intermediate response of 0.02 Mg ha−1 in yield difference and 8% relative increase.
This positive influence of broadleaved over coniferous trees on maize grain yields can be attributed to several factors, all of which trace back to the quality and amount of litter input. Generally, broadleaved and deciduous litter is known to decompose faster than needle litter, at least in the first one to 2 years, due to its lower lignin content (Prescott et al., 2000, 2005; Lim et al., 2018). Decomposition releases nutrients, and as about half of the tree species represented in this analysis—which contribute 67% of absolute and 68% of relative values (Figure 5)—are able to biologically fix N with the help of their rhizosphere symbionts, the leaves often contain high amounts of N. Fast decomposition makes these nutrients, which are not only limited to N, available for crop uptake. Combined with high rates of tree biomass production, this renders the broadleaved trees suitable for regular pruning followed by addition of these prunings to the soil of the maize crop. In addition to nutrients, tree litter is a major contributor of soil organic matter (SOM) input (Hou et al., 2020; Mayer et al., 2022). SOM, in turn, plays an essential role in multiple functions of fertile soils, such as the capacity for moisture and nutrient retention, and is thereby a key determinant of soil productivity (Oldfield et al., 2019). A common proxy for SOM is SOC (Oldfield et al., 2019), with SOC contributing about half the amount of SOM (SOM = SOC×2) (Amelung et al., 2018). A recent meta-analysis on SOC sequestration in temperate agroforestry systems showed that SOC sequestration rates were higher in systems with broadleaved tree species than under the influence of coniferous trees (Mayer et al., 2022). This is partly due to the aforementioned high amounts of aboveground litter that decomposes relatively fast but is also attributed to the fact that broadleaved trees produce more root-derived organic C input, leading to relatively high SOC sequestration rates in the subsoil (Mayer et al., 2022).
3.4. Effects of tree age
According to our data, both absolute and relative maize yields showed a predominantly positive response to rising tree age (Figure 5). Our analysis indicated that the more mature the trees were, the higher their positive effect on yields may be. While trees younger than 1 year achieved no significant yield difference of 0.01 Mg ha−1 (−0.5%), trees between the age of 11 and 15 years recorded median gains of 1.2 Mg ha−1 (48% response). The only negative absolute response was observed in trees aged 15 and older, which can be explained by the fact that 48 out of 53 values in this category corresponded to coniferous trees.
While the effects of tree age on the type and magnitude of tree-crop-competition have been reported to vary with planting density (Vyamana et al., 2021) and cannot be fully isolated from the effects of other tree characteristics or tree management practises such as pruning, there are several mechanisms that may account for the trends we observed in our analysis. One of the most important benefits of agroforestry systems is their scientifically verified capacity to improve soil fertility: A meta-analysis by Muchane et al. (2020) revealed that agroforestry in the humid and sub-humid tropics increased SOC and soil N storage by 21 and 13%, made 46% more soil N and 11% more P available to crops, and slightly alleviated soil acidity compared to monocultures. In many cases, these effects became more pronounced as the trees matured, which may account for the growing yield increases observed in this analysis. A study on gum acacia (Acacia senegal), for instance, found that soils under 7-year-old plants exhibited significantly greater total N and SOC contents than soils under 9-months-old stands (Isaac et al., 2011). Furthermore, soil N mineralisation rates were significantly (2–3 times) higher under the 7-year-old trees, which may have been linked to stimulated soil microbial activity (Isaac et al., 2011). The positive effect of agroforestry on SOC stocks has been found to increase with tree age, albeit to varying extent depending on the climate zone (Ma et al., 2020). Tropical agroforestry systems quickly accumulate SOC to peak levels, whereas SOC levels under temperate systems grow at a slower rate but peak at an overall higher level (Ma et al., 2020). Specifically, temperate agroforestry has been shown to take 35 years and arid or boreal agroforestry 13 years to reach an SOC equilibrium, compared to only 5 years in the tropics (Ma et al., 2020). The main benefits of SOC accumulation for maize cultivation also vary between different regions and climates (Oldfield et al., 2019).
In addition, tree roots can influence the pathways of soil water and nutrients in ways that benefit the companion crop. In this context, one important concept is the so-called “safety net hypothesis,” which describes the interception of mobile nutrients leaching from crop root zones. Related to this, the “nutrient pump hypothesis” involves tree root uptake of nutrients from deep soil layers, where they would otherwise be inaccessible to the companion crop. Both mechanisms result in the incorporation of nutrients into the leaves, which are then transferred to upper soil layers—i.e. the rooting zone of the alley crop—through leaf shedding or manual harvest and application, followed by decomposition (Bayala and Prieto, 2020). Moreover, there is the possibility of nutrients being transferred between trees and crops via shared mycelium networks of mycorrhiza symbionts (Jalonen et al., 2013). In an 11-years field trial, Makumba et al. (2006) were able to demonstrate that deep-rooting gliricidia trees took up P, Ca, Mg, and K from subsoil layers and transferred them to the topsoil, and the amount of all four nutrients increased over time. There has also been evidence for temporal variations in root activity of trees and crops, another avenue of reducing competition between roots that occupy the same spheres (Odhiambo et al., 2001).
Furthermore, deep-rooted trees can lift or redistribute water to the upper layers through a mechanism known as hydraulic lift or through shared mycorrhizal networks, potentially supplying adjacent crops with additional water (Bayala and Prieto, 2020; Cardinael et al., 2020). This may be part of the reason why we found that agroforestry particularly benefits maize yields in strongly water-limited surroundings. Increasing age of broadleaved trees, predominantly in tropical and subtropical regions led to an increase in maize yields. This observation suggests that the benefits associated with growing maturity seem to outweigh the negative effects associated with enhanced competition for natural resources between older trees and crops. However, the effects of tree age on the type and magnitude of tree-crop-competition have also been reported to vary with planting density (Vyamana et al., 2021).
3.5. Effects of experiment duration
This category was analysed to gain insights into potential temporal trends that may emerge from continuous cropping trials which were long enough to look past seasonal fluctuations—a relationship that is closely related to, but not entirely the same as the effects of tree age. Nonetheless, the responses to tree age and experiment duration followed a similar pattern. The median absolute response of maize yields to increasing time under the influence of agroforestry (Figure 5) continually increased from 0.2 Mg ha−1 in the first 2 years to 0.9 Mg ha−1 in systems that had existed for 15 or more years. The only exception to this trend was the negative response of −0.1 Mg ha−1 registered between 6–10 years. Relative increases exhibited the same trend.
These effects may, to a large extent, be attributed to the increase in soil fertility over time. At the same time, they relate to the detrimental effects of continuous maize cropping without adequate external inputs on soil fertility (Mugendi et al., 1999) that the monocrop experimental control is subjected to. In a long-term gliricidia-maize alley cropping experiment in Malawi (Makumba et al., 2006), observed that alley cropped maize amended with gliricidia prunings achieved three times the yield of monoculture maize without any soil amendments after 11 years (3.8 and 1.1 Mg ha−1, respectively). After starting at similar initial levels, alley-cropped maize yields increased over time, while those of monoculture maize declined or nearly stagnated. Importantly, the unfertilised control exhibited by far the lowest fluctuations, while fertilised and alley-cropped maize showed considerable inter-annual differences (Makumba et al., 2006). Contrasting stability effects were observed in two other long-term trials (12 consecutive years) in Zambia, where the highest yields were recorded in fully fertilised monoculture maize but yields under gliricidia alley cropping—which were still distinctly higher than those of the unfertilised control—proved to be more stable in the long-term (Sileshi et al., 2012). Given that the de facto practise employed by many farmers is continuous maize cropping without external inputs due to their high costs (Mugendi et al., 1999; Sileshi et al., 2012), the comparison to partially or unfertilised maize often poses the most relevant, reality-oriented one. These insights should discourage decision-makers from abandoning agroforestry practises after 1 or 2 years of declining yields, demonstrating that long-term consistency can be expected to pay off.
3.6. Effects related to tree pruning
3.6.1. Effects of tree pruning
Figure 6 visualises all absolute and relative yield responses related to aspects of tree pruning. Tree pruning significantly increased maize grain yields by 0.5 Mg ha−1, corresponding to a relative response of 62%. Trees that were not pruned, which were mainly found in temperate studies and clearly represented the minority at ~18% of all values, recorded a significant negative effect on maize grain yields at −0.4 Mg ha−1 (+42% relative response). The discrepancy between the negative absolute and positive relative response is likely a result of the fact that the relative response drew on 20 additional values.
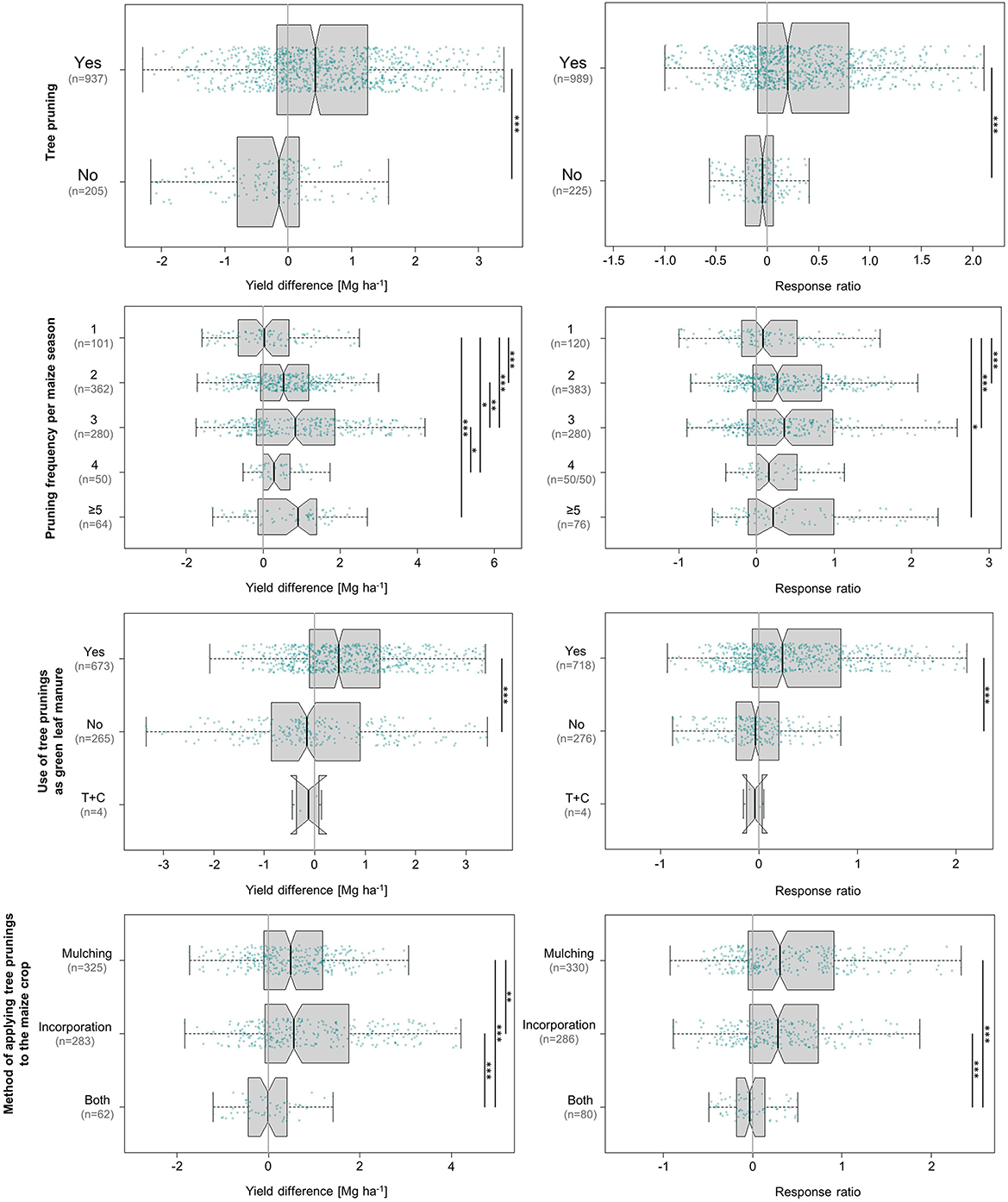
Figure 6. Influence of tree pruning, pruning frequency, utilisation of tree prunings as green leaf manure, and the method of applying tree prunings to the maize crop on absolute (left) and relative (right) differences in maize grain yield achieved under agroforestry as compared to the control plots (sole maize). “T+C” stands for application in treatment and control plot. Each box contains the middle 50% of the data of a category. The median of the data is shown as a vertical solid line within the box. Notches represent the 95% confidence interval of the median. The whiskers indicate the lower and upper quartile of the data, respectively, and are limited to 1.5 times the interquartile range. Green dots represent the individual data points that were included in the calculation. Data points exceeding the whiskers were removed visually, without alteration of the underlying dataset. The number of values per group is given in parentheses, with the first one relating to the yield difference in Mg ha−1 and the second one to the response ratio. The solid black lines annotated with stars to the right of the plot indicate statistically significant differences between groups according to the Kruskal-Wallis-Test (***for p < 0.001, **for p < 0.01, and *for p < 0.05).
In general, pruning of trees in alley cropping systems serves two purposes—to reduce shading of the interplanted crops and to obtain nutrient-rich pruned tree material mainly used for crop fertilisation (Akinnifesi et al., 2007). Importantly, tree pruning has also been shown to reduce root growth in shallow soil layers (i.e., where competition with crops for water and nutrients occurs) (Bayala and Prieto, 2020), which weakens one of the main yield-decreasing influence factors of trees in agroforestry systems, allowing to capitalise on the benefits of deep tree roots instead. At the same time, root water uptake is regulated by the demand of the aboveground parts (Bayala and Prieto, 2020). For this reason, pruning—i.e., removal of a large part of aboveground biomass—can be an effective way of deliberately manipulating the water uptake of shallow-rooted trees from the soil. Several studies observed a decrease in tree root densities in shallow soil resulting from the artificial reduction of aboveground demand. This way, competition for water with the companion crop can be strategically alleviated (Bayala and Prieto, 2020).
3.6.2. Effects of pruning frequency
The analysis of pruning frequency revealed very similar patterns of absolute and relative yield responses, but no clear trend as to an optimum seasonal rate (Figure 6). Maize generally responded positively to pruning, and there was an overall tendency of rising yield increments with increasing pruning frequency. Only one pruning event per maize season did not produce a significant effect, and the response to four prunings (0.3 Mg ha−1, 16%)—which is also the group that drew on the smallest database—was the only one to break with the trend of continuous yield increase.
Whether, when, and how intensively trees are pruned largely determines the level of shading the companion crop is subjected to. As shading is also dependent on tree/hedgerow density, pruning activities should be scheduled in consideration of the tree spacing scheme (Kunhamu et al., 2011). The most intuitive trend in this context would be rising yields in response to decreasing levels of shade, i.e., increasing pruning intensity. If the prunings are applied to the soil of the maize crop—as 57% of studies in this analysis did—pruning intensity also determines the level of nutrient supply to the crop. To some extent, this is reflected by the fact that the lowest pruning intensity produced a negative yield difference. Increasing the level beyond two prunings per season did not generate significantly higher yields (Figure 6). From a practitioners view, this could be considered a positive outcome, as it potentially reduces the requirement for labour-intensive tasks related to tree pruning. The response of varying tree species to different pruning regimes is also an aspect to be considered, as not all tree species support high pruning intensities and may react with lower biomass production (Kiepe, 1996; Neba et al., 2022).
3.6.3. Effects of pruning height
Pruning height, registered by far the most positive response in the category <0.5 m (1.03 Mg ha−1, 41%), but the categories 0.5–1.0 m and >1.0–2.0 m still showed a positive outcome (Figure 4). The distribution of values indicates that in practise trees are preferably pruned at 1 m or lower (only 57 absolute and 58 relative data points in the pruning categories >1 m).
Tree/hedgerow density, tree and pruning height are a mixture between adverse competition for light and favourable effects on the microclimate surrounding the plot. The yield declines observed in response to trees pruned at greater heights are consistent with the well-established notion that shading is one of the key competitive factors limiting maize yields in agroforestry systems (Lawson and Kang, 1990). This is because maize has a C4 photosynthetic pathway and is, therefore, shade-intolerant (Rivest and Vézina, 2015). The negative influence of shade on maize performance has been demonstrated in agroforestry settings (Tiwari et al., 2012; Suryanto et al., 2014; Dilla et al., 2018), but can also be proven with the help of artificial light barriers, as such experiments allow to only change one source of variation and maintain the remaining conditions constant (Cui et al., 2015). Modelling approaches have attempted to isolate the effects of shading by only using data from situations of high water and nutrient availability, which has confirmed the detrimental influence of shade on maize grain yields (Dilla et al., 2018). Looking at the results of our study, it appears that these effects can be outweighed by beneficial factors to a certain extent (e.g., hydraulic lift, nutrient input, SOC build-up, or erosion control).
3.6.4. Effects of utilising pruning material as green manure
Regarding the use of tree pruning material as green manure, our results were highly conclusive, very clearly encouraging the use of tree pruning material as a natural source of nutrients and organic matter (Figure 6). Out of the 937 data pairs recorded in a setting where trees were pruned and the pruned material was used as soil amendment, 719 represented positive effects, leading to a statistically significant median yield increase of 0.5 Mg ha−1. This result consolidates well-established knowledge gained from numerous studies over the last four decades. Depending on the soil type and tree species, decomposed tree pruning material deliver substantial amounts of plant nutrients (Dagar et al., 2020). A review by Akinnifesi et al. (2010) on N-fixing trees in maize-based production systems across East and Southern Africa reached the conclusion that nutrient contributions from N-fixing tree biomass can reduce the need for synthetic fertiliser by 75%. The yield-enhancing effects of tree pruning material as soil amendment on maize growth have been confirmed by cut-and-carry experiments (Kang et al., 1981a; Kormawa et al., 1999; Jama et al., 2000; Vanlauwe et al., 2001; Akintan, 2019), which provide a useful basis for comparison, as they harvest trees that are grown off-site and are thereby able to singularly investigate the influence exerted by the pruning material. Notably, however, application of tree pruning material to the cropped portion of agroforestry systems is a practise limited to the tropical and sub-tropical regions. While trees in temperate systems are pruned as well, their pruning material is not added to the crop due to their high lignin content and slow decomposition rates under temperate conditions (Oelbermann et al., 2004). Instead, they may be taken off-site or chipped and spread within the tree row (Oelbermann et al., 2004). In our analysis, removing the pruned tree material from the site led to a median yield loss of 0.1 Mg ha−1 (3%).
Besides the concerns surrounding N cycling, P availability in agricultural soils is also a major concern—especially in small-scale farming systems of sub-humid and semiarid Africa, where P deficiency is widely considered to be the main abiotic constraint to crop productivity (Sanchez et al., 1997). Tree pruning material releases P during decomposition but is also able to influence P adsorption-desorption dynamics. This includes facilitated complex formation involving ions such as Al and Fe, which otherwise limit P availability, and the release of anions that compete with P for fixation sites, thereby restricting the soil's P sorption capacity and increasing P uptake by maize (Mweta et al., 2007). Based on these mechanisms, gliricidia pruning material has been shown to significantly increase both total P uptake by maize and maize grain yields—either by themselves or coupled with inorganic fertilisers (Mweta et al., 2007). However, as these mechanisms are strongly dependent on soil pH, their effects are site-specific rather than general. Direct P contributions resulting from leaf P contents also bear considerable potential when compared to projections by Berge et al. (2019), who estimated that SSA countries will require minimum long-term P inputs ranging from 6 to 23 kg ha−1 a−1 to meet their maize demand by 2050.
3.6.5. Effects of different methods of applying pruning material
With respect to the best technique of pruning material application, the studies in this analysis differentiated two techniques: (a) superficial mulching, where the pruning material is simply spread across the soil surface and left to decompose, or (b) active incorporation into the soil at varying depths and mixing intensities. Direct comparison revealed incorporation to be significantly more effective in increasing maize yields than mulching (absolute response of 0.6 and 0.5 Mg ha−1, respectively). This is consistent with earlier observations that leucaena (Leucaena leucocephala) pruning material is a more effective N source when incorporated into the soil rather than applied as mulch (Kang et al., 1981a,b). A potential explanation is that incorporated pruning material may be decomposed and mineralised more quickly (Kadiata et al., 1997). In fact, ideal application of leucaena pruning material is likely to require a delicate balance regarding the technique, amount, and time of application, as the finely divided pinnate leaves are known to decompose so quickly that nutrients may be leached before they can be taken up by the maize crop. This is also why leucaena is unsuited for weed suppression (Orwa et al., 2022), an additional benefit associated only with mulching. Other species, such as gliricidia and siamese cassia (Senna siamea), have been observed to be very effective in reducing weed density and biomass when applied as mulch (Kamara et al., 2000). Our study, however, revealed that for agroforestry to develop its positive effects on maize yields, it is important that some kind of mechanical weed control (e.g., manual removal, hoeing) be exercised (data not shown in figures).
While the relative responses of these two treatments were highly similar at 28% for mulching and 31% for incorporation, the combination of both registered nearly no absolute or relative response at −0.02 Mg ha−1 and −3%, respectively—an outcome that is difficult to explain, aside from the fact that it was based on the smallest database at 81 (11%) data entries.
3.7. Effects related to soil properties
3.7.1. Effects of soil texture
Note that all analyses related to soil properties are associated with some uncertainty due to the fact that we did not standardise the soil depth for which the individual characteristics are reported. Sampling depths, which were not provided by all studies, ranged from 15 to 120 cm, with a mean of 26 cm and a median of 20 cm.
As shown in Figure 7, the only soil texture category to produce statistically significant and ecologically relevant yield gains was sand (0.6 Mg ha−1, 35%). Consequently, high sand content appears to provide an environment in which agroforestry influences maize production.
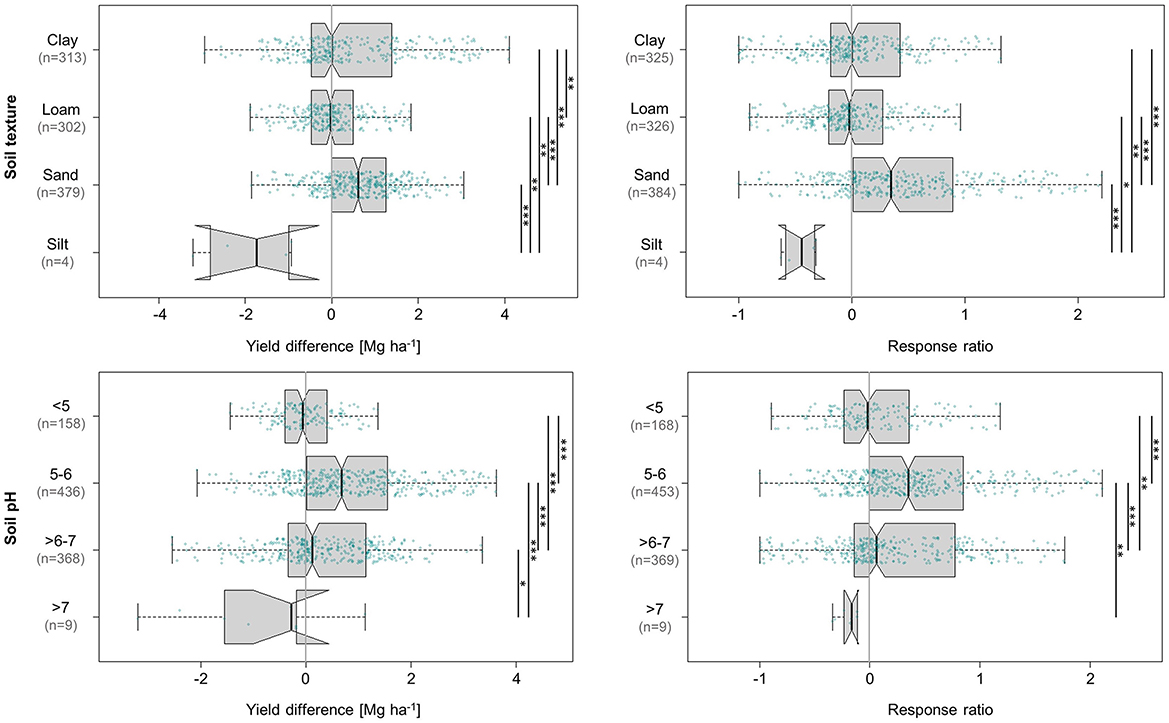
Figure 7. Influence of soil texture and soil pH on absolute (left) and relative (right) differences in maize grain yield achieved under agroforestry as compared to the control plots (sole maize). Each box contains the middle 50% of the data of a category. The median of the data is shown as a vertical solid line within the box. Notches represent the 95% confidence interval of the median. The whiskers indicate the lower and upper quartile of the data, respectively, and are limited to 1.5 times the interquartile range. Green dots represent the individual data points that were included in the calculation. Data points exceeding the whiskers were removed visually, without alteration of the underlying dataset. The number of values per group is given in parentheses, with the first one relating to the yield difference in Mg ha−1 and the second one to the response ratio. The solid black lines annotated with stars to the right of the plot indicate statistically significant differences between groups according to the Kruskal-Wallis-Test (***for p < 0.001, **for p < 0.01, and *for p < 0.05).
Trees have been shown to exert greater influence on soil cation exchange capacity (CEC) in sandy soils than in fine-textured soils. This is attributed to the fact that the key determinant of CEC in sandy soils is SOM, whereas in fine-textured soils, CEC is mainly a function of clay content, at least in temperate soils (Campbell et al., 1994). Elevated levels of SOM input, which positively affect many soil properties related to soil fertility (Oldfield et al., 2019), are a key benefit of broadleaved agroforestry systems. The capacity of SOM to improve the nutrient and water retention capacity of soils is particularly important in sandy soils because these two factors mainly limit crop performance on sandy sites (Liu et al., 2012). In addition, sandy soils are more prone to erosion, the prevention or reduction of which is an important benefit of agroforestry (Muchane et al., 2020).
3.7.2. Effects of soil pH
Regarding the relationship between soil pH and effects of agroforestry on maize grain yields (Figure 7), the only category exhibiting appreciable yield gains was pH 5–6 with a median yield increment of 0.7 Mg ha−1 (35%), which was also the only statistically significant response in absolute terms. Notably, this category also drew on the largest database (436 absolute, 453 relative values), although the categories pH >6–7 (0.1 Mg ha−1, 6%) and pH <5 (−0.1 Mg ha−1, −2%) were also well-substantiated (pH >6–7: 368 relative and 369 absolute values; pH <5: 158 absolute and 168 relative values). Soils with a pH >7 were strongly underrepresented at a mere nine values.
To some extent, our results agree with the narrative that agroforestry is able to promote crop production by alleviating soil acidity (Wong et al., 2000; Muchane et al., 2020), i.e., offers the highest benefits to crops on soils that do not represent ideal growing conditions in terms of pH. A meta-analysis by Muchane et al. (2020) revealed that agroforestry in the humid and sub-humid tropics alleviated soil acidity by 2% compared to monocultures. The pH optimum for maize cultivation has been reported to be in the range of 6.0–6.5 for ultisols (Adeoye and Agboola, 1985), which cover 11% of the tropics (Rowe et al., 2005) and are represented by at least eight studies (109 values) in our analysis. Our analysis showed by far the most positive impact of agroforestry on maize yields in the category just below this range (pH 5–6). Importantly, these benefits are linked to the rate of litterfall (George et al., 2002) or soil incorporation of tree prunings (Wong et al., 2000), a widespread practise in tropical agroforestry that applies to 60% of our values in the pH range of 5–6. The reason is that soil acidity increases the availability of phytotoxic Al species, which—combined with Ca, P, Mg, and N deficiency—are key causes of acidity-related constraints to soil fertility (Wong et al., 2000). One of the studies in our analysis, for instance, was conducted on a soil where Al saturation in the subsoil verged on 60% (Rowe et al., 2005). In many tropical soils, the content of exchangeable Al decreases to nearly zero at pH >5 (Thomas and Hargrove, 1984). Liming by itself, however, does not provide an adequate solution in many cases, as it may require additional nutrient input or can even restrict availability of certain nutrients if applied excessively. At the same time, lime is limited in both market availability and affordability in countries of the Global South. Tree prunings, in turn, constitute an integrated approach to the amelioration of acidic soils (Wong et al., 2000). However, our results suggest that these effects are restricted to conditions of moderate acidity, ceasing to operate in strongly acidic soils of pH <5—which is well substantiated, given that 88% of our values in this range correspond to trials that incorporated tree prunings into the soil.
3.7.3. Effects of soil organic carbon content
Our study revealed no clear trend with respect to maize yield responses to agroforestry at different levels of SOC content (Figure 8). Yields increased the most in soils with 5–10 g kg−1 SOC (0.64 Mg ha−1, 38%), followed by 0.47 Mg ha−1 (24%) in soils with >30 g kg−1 SOC. The remaining categories registered slight increases, with the only negative response in the intermediate range of >10–20 g kg−1 (−0.04 Mg ha−1, −2%).
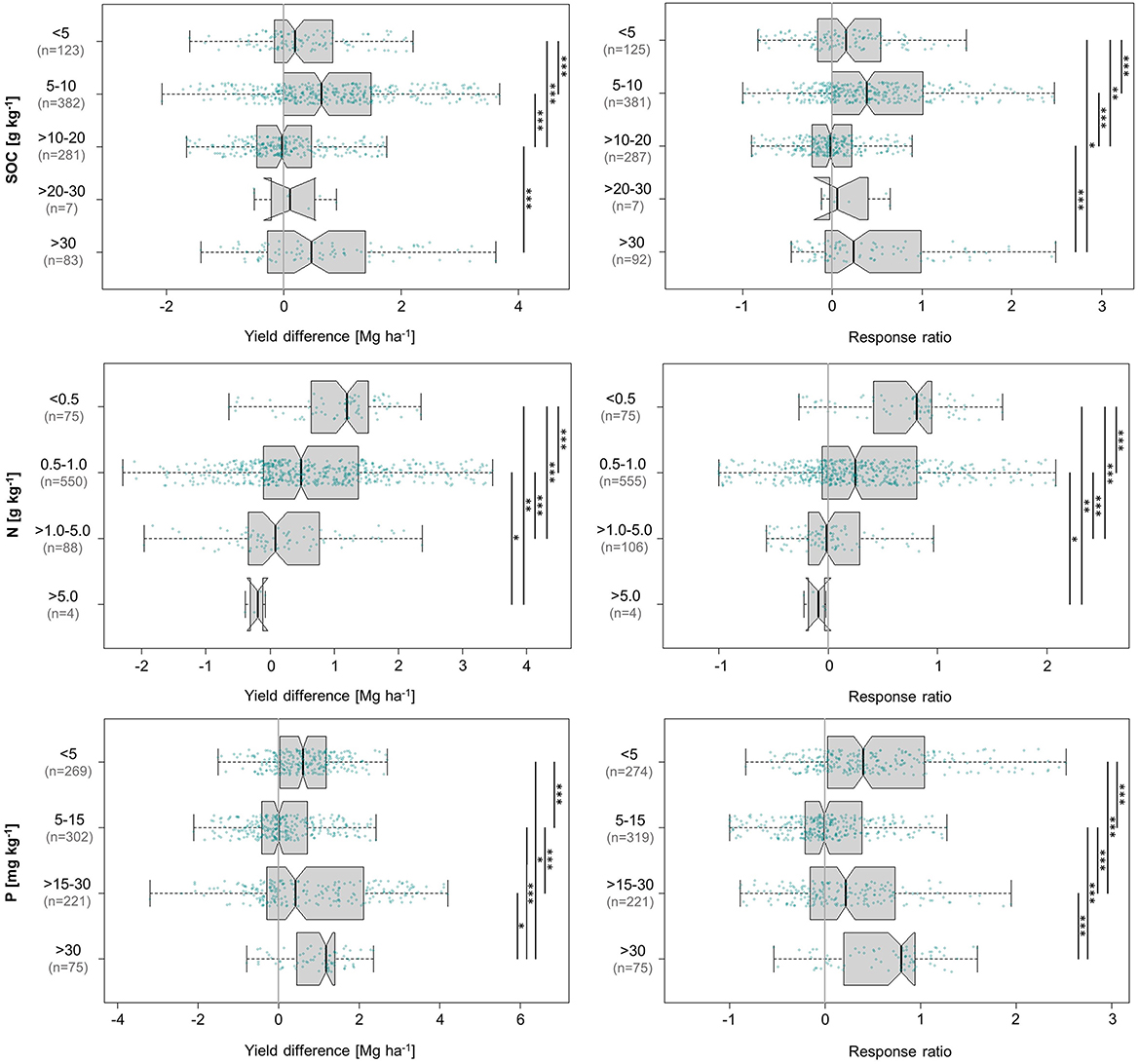
Figure 8. Influence of soil organic carbon, total soil N, and extractable soil P content on absolute (left) and relative (right) differences in maize grain yield achieved under agroforestry as compared to the control plots (sole maize). Each box contains the middle 50% of the data of a category. The median of the data is shown as a vertical solid line within the box. Notches represent the 95% confidence interval of the median. The whiskers indicate the lower and upper quartile of the data, respectively, and are limited to 1.5 times the interquartile range. Green dots represent the individual data points that were included in the calculation. Data points exceeding the whiskers were removed visually, without alteration of the underlying dataset. The number of values per group is given in parentheses, with the first one relating to the yield difference in Mg ha−1 and the second one to the response ratio. The solid black lines annotated with stars to the right of the plot indicate statistically significant differences between groups according to the Kruskal-Wallis-Test (***for p < 0.001, **for p < 0.01, and *for p < 0.05).
These results are inconsistent with those of previous research on the relationship between SOC and maize yields. In a global meta-analysis, Oldfield et al. (2019) found that maize yields, on average, increased with rising SOC content, levelling off at ~20 g kg−1 SOC. They observed the largest gains in yield between SOC contents of 1–20 g kg−1. If this classification is applied to our data, the category 1–20 g kg−1 produces a yield increase of 0.3 Mg ha−1 (48% increase, data not shown in figures), which is still distinctly lower than the response to SOC contents >30 g kg−1. Our results are also in disagreement with the critical value of >27 g kg−1 total C in soils, which has been reported by the Kenyan Ministry of Agriculture to mark the threshold above which an economic maize yield response to added C is unlikely (Munialo et al., 2020). In contrast, the fact that approximately two thirds of the worldwide area under maize cultivation currently draw on SOC contents of less than 20 g kg−1 (Oldfield et al., 2019) is indeed supported by the distribution of values in our analysis, with 89% of data entries corresponding to SOC contents of ≤ 20 g kg−1.
3.7.4. Effects of soil nitrogen content
Our analysis showed that the benefits of agroforestry for maize cultivation were highest on N-deficient soils and decreased as soil N content rose (Figure 8), which substantiates the well-established insight that agroforestry can be of particular value in relatively fertile but N-deficient soils (Nair et al., 2021). On soils containing <0.5 g kg−1 N, maize yields increased by 1.2 Mg ha−1 (81%), which highlights the significance of agroforestry for improvement of N availability. As soil N content rose, agroforestry continued to improve yields, but to less extent. Agroforestry-induced yield increases appeared to approach zero when soil N exceeded 5 g kg−1, but this could not be conclusively confirmed. The reason is that the response registered in soil with more than 5 g kg−1 N (-0.2 Mg ha−1, −9%)—the only negative response in both absolute and relative terms—is not representative, as it was based on a mere four values from one single study by Imo (2009) with the unusual combination of two different conifers, i.e., no N-fertilisation and reduced overall soil fertility improvement by trees, in the humid tropical climate of western Kenya.
A study in Indonesian cacao agroforestry has revealed higher rates of N mineralisation and ammonium uptake as well as faster turnover of the ammonium pool under agroforestry than in a neighbouring maize monoculture, suggesting that the decomposer community finds better conditions for fulfilling its nutrient cycling functions under agroforestry than in sole cropping (Tscharntke et al., 2011).
3.7.5. Effects of soil phosphorous content
Our analysis did not indicate a trend regarding the relationship between extractable P content in agricultural soils and maize yields as influenced by agroforestry (Figure 8). The highest median yield increase of 1.2 Mg ha−1 (79%) was observed in soils with more than 30 mg kg−1 plant-available P and is nearly identical to the maximum absolute and relative yield increase achieved in relation to soil N content. The fact that both these nutrients caused yield increases of almost five times the grand median underlines their relevance for maize production. While plant-available P contents <5 mg kg−1 and 15–30 mg kg−1 led to medium-scale effects, the category in between recorded a near-neutral response of −0.003 Mg ha−1 (−0.6%). This suggests that maize may benefit most from agroforestry in settings of very low or very high P availability.
P is an essential nutrient for maize cultivation (Gagnon et al., 2020). Generally, P deficiency is among the most widespread nutrient deficiencies in African soils (together with N) and, as such, an important limitation to maize production due to the low native soil P content and high P fixation by Fe and Al oxides in tropical soils (Radersma and Grierson, 2004; Mukuralinda et al., 2010). This accounts for the positive response observed in settings of low P availability. Previous research has indicated that N fertilisation may have a much greater impact on maize yields than P fertilisation (Sapkota et al., 2017), which did not become evident from our analysis, given the near-identical maximum responses.
The fact that agroforestry also substantially increased maize yields in situations of comparatively high P availability may indicate that other yield-promoting influence factors of agroforestry are able to take effect if P limitation is not an issue or once the obstacle of P deficiency has been overcome. Finally, as is often the case, P acquisition and internal utilisation efficiency is also a trait that varies among genotypes (Alves et al., 2001; Parentoni and Souza Júnior, 2008), which has not been evaluated in this study.
3.8. Effects of intermediate crops
More than two thirds of all values (68% of absolute and 67% of relative values) originated from trials that did not include the cultivation of an intermediate crop between maize seasons. Among those that did, N-fixing crops such as cowpea (Vigna unguiculata) or soybean (Glycine max) were clearly preferred over species without N-fixing symbionts. Our results showed that while the overall yield difference between studies with and without intermediate crops was small from both a statistical and ecological perspective (0.19 and 0.26 Mg ha−1, corresponding to a 7 and 10% increase, respectively), the question of whether the intermediate crop species is able to fix N proved to be a very important one (Figure 9). N-fixing intermediate crops were related to a median maize yield increment of 0.3 Mg ha−1 (13%), whereas non-N-fixing reduced maize yields by almost equal proportion at a decline of 0.4 Mg ha−1 (−12%). The favourable influence of N-fixing intermediate crops is entirely consistent with the positive impact that was observed in response to N-fixing trees and the utilisation of their biomass as organic fertiliser, as discussed previously. In 61% of the values, N-fixing intermediate crops were combined with an N-fixing agroforestry tree species, which increased yields by 0.4 Mg ha−1 (19%)—more than double the amount achieved by N-fixing intermediate crops combined with tree species that do not fix N (0.2 Mg ha−1, 8%). This shows that they can be a valuable addition to existing sources of natural N fixation. The significant negative effects of the remaining intermediate crop species—which consisted of cassava (Manihot esculenta), rice, wheat, peanut (Arachis hypogaea), and millet (Panicum miliaceum)—showed that maize yields in agroforestry systems tended not to benefit from rotation with crops that lack the great advantage of prompt N enrichment (although these intermediate crops may serve other economic and or/ecological purposes).
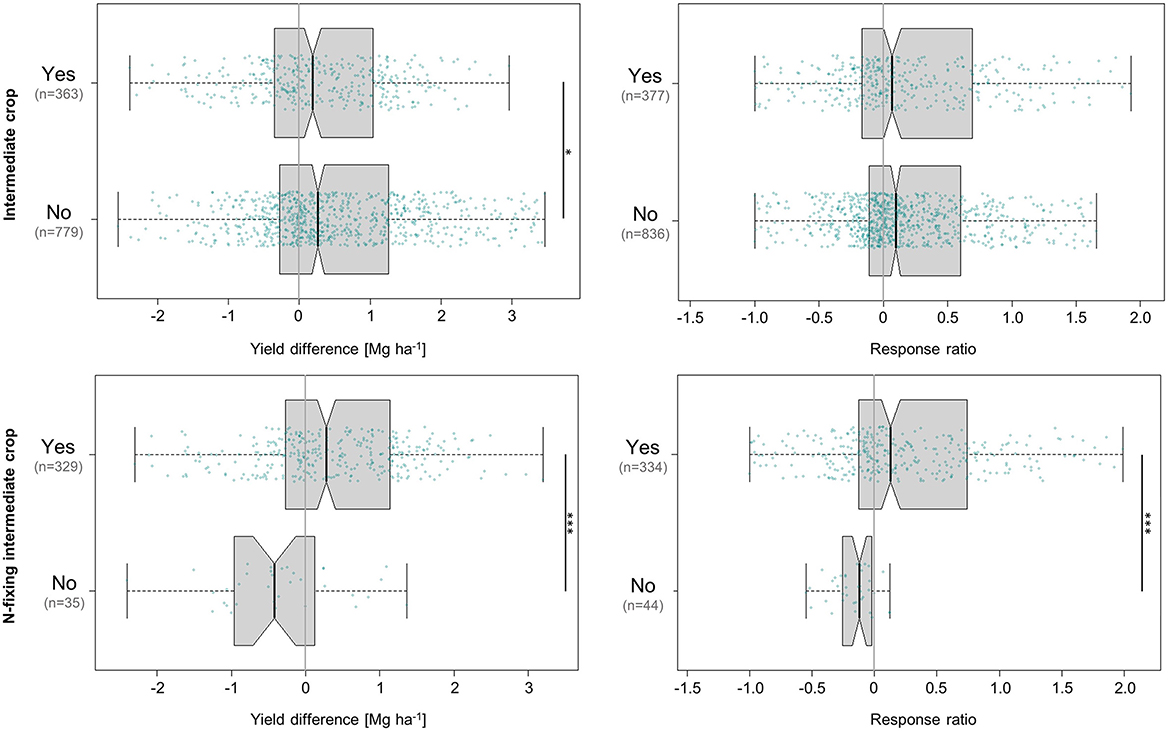
Figure 9. Influence of intermediate crops and their ability to fix N on absolute (left) and relative (right) differences in maize grain yield achieved under agroforestry as compared to the control plots (sole maize). Each box contains the middle 50% of the data of a category. The median of the data is shown as a vertical solid line within the box. Notches represent the 95% confidence interval of the median. The whiskers indicate the lower and upper quartile of the data, respectively, and are limited to 1.5 times the interquartile range. Green dots represent the individual data points that were included in the calculation. Data points exceeding the whiskers were removed visually, without alteration of the underlying dataset. The number of values per group is given in parentheses, with the first one relating to the yield difference in Mg ha−1 and the second one to the response ratio. The solid black lines annotated with stars to the right of the plot indicate statistically significant differences between groups according to the Kruskal-Wallis-Test (***for p < 0.001 and *for p < 0.05).
3.9. Effects of climate and water availability
3.9.1. Effects of temperate vs. tropical agroforestry
Agroforestry in temperate and tropical climates differs in environmental production factors (e.g., soil fertility, temperature), dominating systems (e.g., shelterbelts vs. alley cropping), tree species (e.g., broadleaved vs. coniferous, N-fixing vs. non-N-fixing), management practises (e.g., pruning), and primary objective (securing livelihoods vs. environmental benefits). In the specific case of maize production, our analysis (Figure 10) showed that on the whole, the set of natural and management-related characteristics that generally apply to tropical agroforestry generated a median yield increment of 0.3 Mg ha−1 (16%). Temperate systems, on the contrary, decreased maize yields by almost the same extent in absolute terms at a median loss of 0.4 Mg ha−1 (5%). This indicates substantial ecological differences, which show agroforestry—at least how it was practised in the studies that make up this analysis—to be a viable option for maize yield improvement in the tropics and subtropics, but not in temperate regions. However, the small relative decrease of 5% recorded by temperate systems may be entirely acceptable and within reason given that the main objective of temperate agroforestry is mitigation of environmental degradation rather than enhancement of crop productivity. In these settings, the positive effects of agroforestry on soil health, biodiversity, water pollution control, and climate change mitigation may well outweigh minor reductions in crop yields—especially if these environmental benefits were compensated financially.
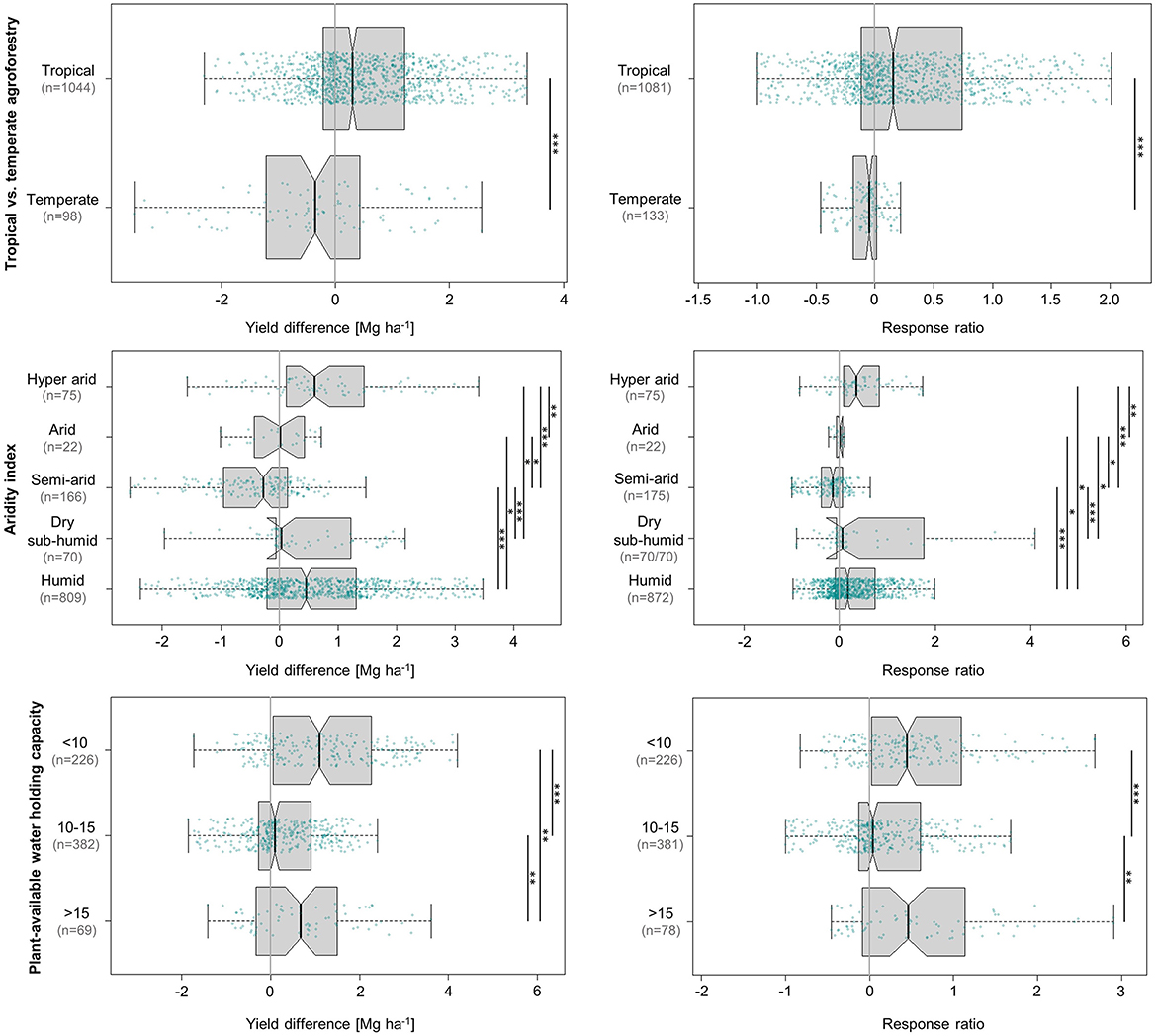
Figure 10. Influence of climate (tropical vs. temperate), aridity [index by Zomer et al. (2022)], and the plant available water holding capacity of the soil on absolute (left) and relative (right) differences in maize grain yield achieved under agroforestry as compared to the control plots (sole maize). Each box contains the middle 50% of the data of a category. The median of the data is shown as a vertical solid line within the box. Notches represent the 95% confidence interval of the median. The whiskers indicate the lower and upper quartile of the data, respectively, and are limited to 1.5 times the interquartile range. Green dots represent the individual data points that were included in the calculation. Data points exceeding the whiskers were removed visually, without alteration of the underlying dataset. The number of values per group is given in parentheses, with the first one relating to the yield difference in Mg ha−1 and the second one to the response ratio. The solid black lines annotated with stars to the right of the plot indicate statistically significant differences between groups according to the Kruskal-Wallis-Test (***for p < 0.001, **for p < 0.01, and *for p < 0.05).
3.9.2. Effects of water availability (aridity index and plant-available water holding capacity of soils)
Both the yield responses to the aridity index and to plant-available water in soils (Figure 10) show that maize production benefits the most from agroforestry in settings of pronounced water limitation, followed by environments with ample water supply. In hyper-arid regions (aridity index <0.03), maize yields increased by a median of 0.6 Mg ha−1 (35%) under the influence of agroforestry. Humid growing conditions led to median yield gains of 0.5 Mg ha−1 (17%). Similarly, soils in the lowest category of plant-available water holding capacity (<10 mm dm−1) registered the largest absolute yield increment at 1.1 Mg ha−1, followed by 0.7 Mg ha−1 at >15 mm dm−1. In this case, the relative responses were nearly identical at 44 and 46%, respectively.
The high relative response to hyper-arid conditions is not an indication of relatively low yields in the control sites under these conditions. The pattern of response is, in fact, consistent with earlier observations and well-established knowledge. Nair et al. (2021) relate that agroforestry, specifically alley cropping, is beneficial to crop yields in sub-humid and humid environments where trees and crops do not compete for water, but detrimental to crop yields in semi-arid areas. Although this is also closely linked to the level of soil fertility (Nair et al., 2021), it agrees with our findings regarding the influence of aridity. An important aspect to be highlighted, however, is the yield improvement potential of agroforestry in arid and hyper-arid regions, or, in other words, in situations of drought stress. Makumba et al. (2006) observed that maize in agroforestry systems withstood drought stress for approximately 2 weeks during the common mid-season drought periods, whereas the maize plants of the sole crop control wilted. The authors pointed out that soils under a gliricidia-maize system had previously been found to contain 20% more soil moisture at depths >30 cm than sole maize during the driest month of the year (Makumba et al., 2006). In a drought experiment using a rainout shelter, mean maize grain yields were significantly reduced by drought in sole maize and a maize-pigeon pea (Cajanus cajan) agroforestry system, but not in maize-gliricidia or maize-gliricidia-pigeon pea systems as long as they received N and P fertilisers. Maize drought resistance in fertilised conditions was significantly higher in maize-gliricidia-pigeon pea systems than in sole maize and maize-gliricidia (Renwick et al., 2020). An important aspect that has not been mentioned yet is drought-alleviating capacities of mycorrhizal fungi (MF) associated with tree roots. Agroforestry trees can be an important source of MF inoculants to the companion crop (Hailemariam et al., 2013), and MF can enhance plants' resistance to drought (Boomsma and Vyn, 2008). These benefits become particularly evident under drought stress but are also relevant when water is not a limiting factor (Augé, 2001; Lawson et al., 2019). In addition, mulching with tree prunings—which was practised in 57% of the studies in this analysis—can also be an effective tool to conserve soil moisture (Lawson and Kang, 1990; Sharma et al., 2010).
4. Conclusions
The purpose of this meta-analysis was to gain insights into the effects of agroforestry on grain yields of maize and to what extent these effects are a function of varying environmental and management-related aspects. We demonstrated that agroforestry is able to increase maize yields, thus alleviating hunger, especially in SSA countries, and significantly contributing to SDG 2 of the United Nations. Given the complexity of biotic and abiotic interactions in agroforestry systems, our results indicate that there is no one-size-fits-all-solution to increase crop yields and maximise economic, social, or environmental benefits through agroforestry. Considering this, the present study provided evidence of how these biotic and abiotic interactions determine the benefits of agroforestry systems and thereby provided a guide to maize cultivation under agroforestry for farmers, extension workers, and agricultural decision-makers.
Data availability statement
Publicly available datasets were analysed in this study. This data can be found here: The full list of publications (i.e., original data sources) this meta-analysis is based on can be found in the Supplementary material. The dataset generated by compiling and analysing the original values extracted from these individual studies is available upon request to the corresponding author.
Author contributions
Data extraction, writing—original draught preparation, and conceptualisation: CB. Writing—review and editing: CB, BG, AG, and NT. Figures: CB and AG. Supervision: BG, NT, and AG. All authors contributed to the article and approved the submitted version.
Funding
Open Access funding enabled and organised by Projekt DEAL. Funding for this study was provided by Martin Luther University Halle-Wittenberg.
Acknowledgments
We warmly thank Dr. Daniel Holte for his valuable support with statistical analyses and data visualisation using R.
Conflict of interest
The authors declare that the research was conducted in the absence of any commercial or financial relationships that could be construed as a potential conflict of interest.
Publisher's note
All claims expressed in this article are solely those of the authors and do not necessarily represent those of their affiliated organizations, or those of the publisher, the editors and the reviewers. Any product that may be evaluated in this article, or claim that may be made by its manufacturer, is not guaranteed or endorsed by the publisher.
Supplementary material
The Supplementary Material for this article can be found online at: https://www.frontiersin.org/articles/10.3389/fsufs.2023.1167686/full#supplementary-material
References
Adeoye, G. O., and Agboola, A. A. (1985). Critical levels for soil pH, available P, K, Zn and Mn and maize ear-leaf content of P, Cu and Mn in sedimentary soils of South-Western Nigeria. Fertilizer Res. 6, 65–71. doi: 10.1007/BF01058165
Akinnifesi, F. K., Ajayi, O. C., Sileshi, G., Chirwa, P. W., and Chianu, J. (2010). Fertiliser trees for sustainable food security in the maize-based production systems of East and Southern Africa. A review. Agron. Sustain. Dev. 30, 615–629. doi: 10.1051/agro/2009058
Akinnifesi, F. K., Makumba, W., and Kwesiga, F. R. (2006). Sustainable maize production using gliricidia/maize intercropping in Southern Malawi. Ex. Agric. 42, 441–457. doi: 10.1017/S0014479706003814
Akinnifesi, F. K., Makumba, W., Sileshi, G., Ajayi, O. C., and Mweta, D. (2007). Synergistic effect of inorganic N and P fertilizers and organic inputs from Gliricidia sepium on productivity of intercropped maize in Southern Malawi. Plant Soil 294, 203–217. doi: 10.1007/s11104-007-9247-z
Akintan, C. I. (2019). Agronomic Performance of Maize (Zea mays L.) as influenced by Leaf Pruning Quality in a Cut and Carry Agroforestry System in Akure, Ondo State, Nigeria. KIU J. Humanities 4, 199–208.
Alves, V. M. C., Parentoni, S. N., Vasconcellos, C. A., Bahia Filho, A. F. C., Pitta, G. V. E., and Schaffert, R. E. (2001). “Mechanisms of phosphorus efficiency in maize,” in Plant Nutrition, eds. W. J. Horst, M. K. Schenk, A. Bürkert, N. Claassen, H. Flessa, W. B. Frommer, et al. (Dordrecht: Springer Netherlands) 566–567. doi: 10.1007/0-306-47624-X_274
Amelung, W., Blume, H.-P., Fleige, H., Horn, R., Kandeler, E., Kögel-Knabner, I., et al. (2018). Scheffer/Schachtschabel Lehrbuch der Bodenkunde. Berlin, Heidelberg: Springer Spektrum. doi: 10.1007/978-3-662-55871-3
Augé, R. M. (2001). Water relations, drought and vesicular-arbuscular mycorrhizal symbiosis. Mycorrhiza 11, 3–42. doi: 10.1007/s005720100097
Bagnall, D. K., Morgan, C. L. S., Cope, M., Bean, G. M., Cappellazzi, S., Greub, K., et al. (2022). Carbon-sensitive pedotransfer functions for plant available water. Soil Science Soc of Amer J 86, 612–629. doi: 10.1002/saj2.20395
Banda, A. Z., Maghembe, J. A., Ngugi, D. N., and Chome, V. A. (1994). Effect of intercropping maize and closely spacedLeucaena hedgerows on soil conservation and maize yield on a steep slope at Ntcheu, Malawi. Agroforest Syst. 27, 17–22. doi: 10.1007/BF00704831
Bayala, J., and Prieto, I. (2020). Water acquisition, sharing and redistribution by roots: applications to agroforestry systems. Plant Soil. 453, 17–28. doi: 10.1007/s11104-019-04173-z
Berge, H. F. M., Ten Hijbeek, R., van Loon, M. P., Rurinda, J., Tesfaye, K., Zingore, S., et al. (2019). Maize crop nutrient input requirements for food security in sub-Saharan Africa. Global Food Secur. 23, 9–21. doi: 10.1016/j.gfs.2019.02.001
Boomsma, C. R., and Vyn, T. J. (2008). Maize drought tolerance: Potential improvements through arbuscular mycorrhizal symbiosis? Field Crops Res. 108, 14–31. doi: 10.1016/j.fcr.2008.03.002
Campbell, B. M., Frost, P., King, J. A., Mawanza, M., and Mhlanga, L. (1994). The influence of trees on soil fertility on two contrasting semi-arid soil types at Matopos, Zimbabwe. Agroforest. Syst. 28, 159–172. doi: 10.1007/BF00704827
Cardinael, R., Mao, Z., Chenu, C., and Hinsinger, P. (2020). Belowground functioning of agroforestry systems: recent advances and perspectives. Plant Soil. 453, 1–13. doi: 10.1007/s11104-020-04633-x
Cole, M. B., Augustin, M. A., Robertson, M. J., and Manners, J. M. (2018). The science of food security. NPJ Sci. Food 2, 14. doi: 10.1038/s41538-018-0021-9
Crippa, M., Solazzo, E., Guizzardi, D., Monforti-Ferrario, F., Tubiello, F. N., and Leip, A. (2021). Food systems are responsible for a third of global anthropogenic GHG emissions. Nat. Food 2, 198–209. doi: 10.1038/s43016-021-00225-9
Cui, H., Camberato, J. J., Jin, L., and Zhang, J. (2015). Effects of shading on spike differentiation and grain yield formation of summer maize in the field. Int. J. Biometeorol. 59, 1189–1200. doi: 10.1007/s00484-014-0930-5
Dagar, J. C., Gupta, S. R., and Teketay, D. (2020). Agroforestry for Degraded Landscapes. Singapore: Springer Singapore. doi: 10.1007/978-981-15-6807-7
Dagar, J. C., and Tewari, V. P. (2017). Agroforestry. Singapore: Springer Singapore. doi: 10.1007/978-981-10-7650-3
den Biggelaar, C., Lal, R., Wiebe, K., and Breneman, V. (2003). “The Global Impact Of Soil Erosion On Productivity,” in Advances in agronomy (Elsevier) 1–48. doi: 10.1016/S0065-2113(03)81001-5
Development Initiatives (2021). 2021 Global Nutrition Report: The state of global nutrition. Bristol, UK.
Dilla, A., Smethurst, P. J., Barry, K., Parsons, D., and Denboba, M. (2018). Potential of the APSIM model to simulate impacts of shading on maize productivity. Agroforest Syst. 92, 1699–1709. doi: 10.1007/s10457-017-0119-0
Easterling, W. (1997). Modelling the effect of shelterbelts on maize productivity under climate change: An application of the EPIC model. Agric. Ecosyst. Environ. 61, 163–176. doi: 10.1016/S0167-8809(96)01098-5
El Bilali, H., Callenius, C., Strassner, C., and Probst, L. (2019). Food and nutrition security and sustainability transitions in food systems. Food Energy Secur. 8, e00154. doi: 10.1002/fes3.154
Erenstein, O., Jaleta, M., Sonder, K., Mottaleb, K., and Prasanna, B. M. (2022). Global maize production, consumption and trade: trends and RandD implications. Food Sec. 14, 1295–1319. doi: 10.1007/s12571-022-01288-7
FAO (2017). The future of food and agriculture: Trends and challenges. Rome: Food and Agriculture Organization of the United Nations.
FAO IFAD, UNICEF, WFP, and WHO. (2022). The State of Food Security and Nutrition in the World 2022. Repurposing food and agricultural policies to make healthy diets more affordable. Rome, Italy: FAO, IFAD, UNICEF, WFP, WHO.
Gagnon, B., Ziadi, N., Bélanger, G., and Parent, G. (2020). Validation and use of critical phosphorus concentration in maize. Eur. J. Agron. 120, 126147. doi: 10.1016/j.eja.2020.126147
George, T. S., Gregory, P. J., Robinson, J. S., Buresh, R. J., and Jama, B. (2002). Utilisation of soil organic P by agroforestry and crop species in the field, western Kenya. Plant Soil 246, 53–63. doi: 10.1023/A:1021575532546
Guto, S. N., Pypers, P., Vanlauwe, B., Ridder, N., and de Giller, K. E. (2011). Tillage and vegetative barrier effects on soil conservation and short-term economic benefits in the Central Kenya highlands. Field Crops Res. 122, 85–94. doi: 10.1016/j.fcr.2011.03.002
Hailemariam, M., Birhane, E., Asfaw, Z., and Zewdie, S. (2013). Arbuscular mycorrhizal association of indigenous agroforestry tree species and their infective potential with maize in the rift valley, Ethiopia. Agroforest Syst. 87, 1261–1272. doi: 10.1007/s10457-013-9634-9
Hou, G., Delang, C. O., Lu, X., and Gao, L. (2020). A meta-analysis of changes in soil organic carbon stocks after afforestation with deciduous broadleaved, sempervirent broadleaved, and conifer tree species. Ann. Forest Sci. 77, 1–13. doi: 10.1007/s13595-020-00997-3
Imo, M. (2009). Interactions amongst trees and crops in taungya systems of western Kenya. Agroforest. Syst. 76, 265–273. doi: 10.1007/s10457-008-9164-z
IPCC (2014). Climate Change 2014: Synthesis Report. Contribution of Working Groups I, II and III to the Fifth Assessment Report of the Intergovernmental Panel on Climate Change. Geneva, Switzerland.
Isaac, M. E., Harmand, J.-M., Lesueur, D., and Lelon, J. (2011). Tree age and soil phosphorus conditions influence N2-fixation rates and soil N dynamics in natural populations of Acacia senegal. Forest Ecol. Manage. 261, 582–588. doi: 10.1016/j.foreco.2010.11.011
IUSS Working Group WRB (2015). “World reference base for soil resources 2014,” in International soil classification system for naming soils and creating legends for soil maps. Update 2015.
Jalonen, R., Timonen, S., Sierra, J., and Nygren, P. (2013). Arbuscular mycorrhizal symbioses in a cut-and-carry forage production system of legume tree Gliricidia sepium and fodder grass Dichanthium aristatum. Agroforest. Syst. 87, 319–330. doi: 10.1007/s10457-012-9553-1
Jama, B., Palm, C. A., Buresh, R. J., Niang, A., Gachengo, C., Nziguheba, G., et al. (2000). Tithonia diversifolia as a green manure for soil fertility improvement in western Kenya: A review. Agroforest. Syst. 49, 201–221. doi: 10.1023/A:1006339025728
Jinger, D., Kumar, R., Kakade, V., Dinesh, D., Singh, G., Pande, V. C., et al. (2022). Agroforestry for controlling soil erosion and enhancing system productivity in ravine lands of Western India under climate change scenario. Environ. Monit. Assess. 194, 267. doi: 10.1007/s10661-022-09910-z
Kadiata, B. D., Mulongoy, K., and Isirimah, N. O. (1997). Effect of tree pruning and pruning application to trees on nitrogen fixation by Leucaena and Gliricidia. Agroforest. Syst. 39, 117–128. doi: 10.1023/A:1005957206624
Kamara, A. Y., Akobundu, I. O., Chikoye, D., and Jutzi, S. C. (2000). Selective control of weeds in an arable crop by mulches from some multipurpose trees in Southwestern Nigeria. Agroforest. Syst. 50, 17–26. doi: 10.1023/A:1006425825597
Kang, B. T., Sipkens, L., Wilson, G. F., and Nangju, D. (1981a). Leucaena (Leucaena leucocephala (Lam) de Wit) prunings as nitrogen source for maize (Zea mays L.). Fertilizer Res. 2, 279–287. doi: 10.1007/BF01050199
Kang, B. T., Wilson, G. F., and Sipkens, L. (1981b). Alley cropping maize (Zea mays L.) and leucaena (Leucaena leucocephala Lam) in southern Nigeria. Plant Soil 63, 165–179. doi: 10.1007/BF02374595
Kiepe, P. (1996). Cover and barrier effect of Cassia siamea hedgerows on soil conservation in semi-arid Kenya. Soil Technol. 9, 161–171. doi: 10.1016/S0933-3630(96)00010-4
Kim, S., Dale, B. E., and Jenkins, R. (2009). Life cycle assessment of corn grain and corn stover in the United States. Int. J. Life Cycle Assess. 14, 160–174. doi: 10.1007/s11367-008-0054-4
Kormawa, P. M., Kamara, A. Y., Jutzi, S. C., and Sanginga, N. (1999). Economic evaluation of using mulch from multi-purpose trees in maize-based production systems in South-Western Nigeria. Ex. Agric. 35, 101–109. doi: 10.1017/S0014479799001106
Koutouleas, A., Sarzynski, T., Bertrand, B., Bordeaux, M., Bosselmann, A. S., Campa, C., et al. (2022). Shade effects on yield across different Coffea arabica cultivars—how much is too much? A meta-analysis. Agron. Sustain. Dev. 42, 55. doi: 10.1007/s13593-022-00788-2
Kunhamu, T. K., Kumar, B. M., and Samuel, S. (2011). “Does Tree Management Affect Biomass and Soil Carbon Stocks of Acacia mangium Willd. Stands in Kerala, India?” in Carbon Sequestration Potential of Agroforestry Systems, eds. B. M. Kumar, and P. K. R. Nair (Dordrecht: Springer Netherlands) 217–228. doi: 10.1007/978-94-007-1630-8_12
Kuyah, S., Whitney, C. W., Jonsson, M., Sileshi, G. W., Öborn, I., Muthuri, C. W., et al. (2019). Agroforestry delivers a win-win solution for ecosystem services in sub-Saharan Africa. A meta-analysis. Agron. Sustain. Dev. 39, 1–18. doi: 10.1007/s13593-019-0589-8
Lawson, G., Dupraz, C., and Watté, J. (2019). “Can Silvoarable Systems Maintain Yield, Resilience, and Diversity in the Face of Changing Environments?” in Agroecosystem Diversity (Elsevier) 145–168. doi: 10.1016/B978-0-12-811050-8.00009-1
Lawson, T. L., and Kang, B. T. (1990). Yield of maize and cowpea in an alley cropping system in relation to available light. Agric. Forest Meteorol. 52, 347–357. doi: 10.1016/0168-1923(90)90091-J
Lim, S.-S., Baah-Acheamfour, M., Choi, W.-J., Arshad, M. A., Fatemi, F., Banerjee, S., et al. (2018). Soil organic carbon stocks in three Canadian agroforestry systems: From surface organic to deeper mineral soils. Forest Ecol. Manage. 417, 103–109. doi: 10.1016/j.foreco.2018.02.050
Liu, J., Schulz, H., Brandl, S., Miehtke, H., Huwe, B., and Glaser, B. (2012). Short-term effect of biochar and compost on soil fertility and water status of a Dystric Cambisol in NE Germany under field conditions. J. Plant Nutr. Soil Sci. 175, 698–707. doi: 10.1002/jpln.201100172
Ma, Z., Chen, H. Y. H., Bork, E. W., Carlyle, C. N., and Chang, S. X. (2020). Carbon accumulation in agroforestry systems is affected by tree species diversity, age and regional climate: A global meta-analysis. Global Ecol. Biogeogr. 29, 1817–1828. doi: 10.1111/geb.13145
Makumba W. Janssen B. Oenema O. Akinnifesi F. Mweta D. and KWESIGAF. (2006). The long-term effects of a gliricidia–maize intercropping system in Southern Malawi, on gliricidia and maize yields, and soil properties. Agric. Ecosyst. Environ. 116, 85–92. doi: 10.1016/j.agee.2006.03.012
Mayer, S., Wiesmeier, M., Sakamoto, E., Hübner, R., Cardinael, R., Kühnel, A., et al. (2022). Soil organic carbon sequestration in temperate agroforestry systems – A meta-analysis. Agric. Ecosyst. Environ. 323, 107689. doi: 10.1016/j.agee.2021.107689
McGill, R., Tukey, J. W., and Larsen, W. A. (1978). Variations of box plots. Am. Statistic. 32, 12. doi: 10.2307/2683468
Mokondoko, P., Avila-Foucat, V. S., and Galeana-Pizaña, J. M. (2022). Biophysical drivers of yield gaps and ecosystem services across different coffee-based agroforestry management types: A global meta-analysis. Agric. Ecosyst. Environ. 337, 108024. doi: 10.1016/j.agee.2022.108024
Muchane, M. N., Sileshi, G. W., Gripenberg, S., Jonsson, M., Pumariño, L., and Barrios, E. (2020). Agroforestry boosts soil health in the humid and sub-humid tropics: A meta-analysis. Agric. Ecosyst. Environ. 295, 106899. doi: 10.1016/j.agee.2020.106899
Mugendi, D. N., Nair, P. K. R., Mugwe, J. N., O'Neill, M. K., and Woomer, P. (1999). Alley cropping of maize with calliandra and leucaena in the subhumid highlands of Kenya Part 1. Soil-fertility changes and maize yield. Agroforest. Syst. 46, 39–50. doi: 10.1023/A:1006288301044
Mukuralinda, A., Tenywa, J. S., Verchot, L., Obua, J., Nabahungu, N. L., and Chianu, J. N. (2010). Phosphorus uptake and maize response to organic and inorganic fertilizer inputs in Rubona, Southern Province of Rwanda. Agroforest. Syst. 80, 211–221. doi: 10.1007/s10457-010-9324-9
Munialo, S., Dahlin, A. S., Onyango, M.C, Oluoch-Kosura, W., Marstorp, H., and Öborn, I. (2020). Soil and management-related factors contributing to maize yield gaps in western Kenya. Food Energy Secur. 9, e189. doi: 10.1002/fes3.189
Mweta, D. E., Akinifesi, F. K., Saka, J. D. K., Makumba, W. I., and Chokotho, N. (2007). Green manure from prunings and mineral fertilizer affect phosphorus adsorption and uptake by maize crop in a gliricidia-maize intercropping. Sci. Res. Essay 10, 446–453.
Nair, P. K. R., Kumar, B. M., and Nair, V. D. (2021). An Introduction to Agroforestry. Cham: Springer International Publishing. doi: 10.1007/978-3-030-75358-0
Neba, D. A., Suh, C., Mbarga, A. M., Ngome, A. S. F., Fornkwa, V. Y., and Lendzemo, V. (2022). Effect of tree hedgerow pruning on maize yield in santa, cameroon. Int. J. Agric. Innov. Res. 3, 1750–1756.
Niether, W., Jacobi, J., Blaser, W. J., Andres, C., and Armengot, L. (2020). Cocoa agroforestry systems versus monocultures: a multi-dimensional meta-analysis. Environ. Res. Lett. 15, 104085. doi: 10.1088/1748-9326/abb053
Nuss, E. T., and Tanumihardjo, S. A. (2010). Maize: A paramount staple crop in the context of global nutrition. Compr. Rev. Food Sci. Food Saf. 9, 417–436. doi: 10.1111/j.1541-4337.2010.00117.x
Odhiambo, H. O., Ong, C. K., Deans, J. D., Wilson, J., Khan, A. A. H., and Sprent, J. I. (2001). Roots, soil water and crop yield: tree crop interactions in a semi-arid agroforestry system in Kenya. Plant Soil 235, 221–233. doi: 10.1023/A:1011959805622
Oelbermann, M., Paul Voroney, R., and Gordon, A. M. (2004). Carbon sequestration in tropical and temperate agroforestry systems: a review with examples from Costa Rica and southern Canada. Agric. Ecosyst. Environ. 104, 359–377. doi: 10.1016/j.agee.2004.04.001
Oldfield, E. E., Bradford, M. A., and Wood, S. A. (2019). Global meta-analysis of the relationship between soil organic matter and crop yields. SOIL 5, 15–32. doi: 10.5194/soil-5-15-2019
O'Neill, M. K., Angima, S. D., Duinker, B., and Okoba, B. (2002). Fodder Production from Contour Hedges in the Central Kenyan Highlands. J. Sustain. Agric. 20, 57–67. doi: 10.1300/J064v20n03_06
Orwa, C., Mutua, A., Kindt, R., Jamnadass, R., and Simons, A. (2022). Agroforestree Database: A tree reference and selection guide. Version 4.0. Available online at: https://apps.worldagroforestry.org/treedb2/speciesprofile.php?Spid=18109 (accessed November 19, 2022).
Osgood-Zimmerman, A., Millear, A. I., Stubbs, R. W., Shields, C., Pickering, B. V., Earl, L., et al. (2018). Mapping child growth failure in Africa between 2000 and 2015. Nature 555, 41–47. doi: 10.1038/nature25760
Parentoni, S. N., and Souza Júnior, C. L. (2008). Phosphorus acquisition and internal utilization efficiency in tropical maize genotypes. Pesq. Agropec. Bras. 43, 893–901. doi: 10.1590/S0100-204X2008000700014
Piato, K., Lefort, F., Subía, C., Caicedo, C., Calderón, D., Pico, J., et al. (2020). Effects of shade trees on robusta coffee growth, yield and quality. A meta-analysis. Agron. Sustain. Dev. 40, 1–13. doi: 10.1007/s13593-020-00642-3
Prescott, C. E., Blevins, L. L., and Staley, C. (2005). Litter decomposition in British Columbia forests: Controlling factors and influences of forestry activities. Discussion paper. doi: 10.22230/jem.2005v5n2a298
Prescott, C. E., Zabek, L. M., Staley, C. L., and Kabzems, R. (2000). Decomposition of broadleaf and needle litter in forests of British Columbia: influences of litter type, forest type, and litter mixtures. Can. J. For. Res. 30, 1742–1750. doi: 10.1139/x00-097
QGIS Development Team (2020). QGIS Geographic Information System Version 3.16. Open Source Geospatial Foundation Project.
Radersma, S., and Grierson, P. F. (2004). Phosphorus mobilization in agroforestry: Organic anions, phosphatase activity and phosphorus fractions in the rhizosphere. Plant Soil 259, 209–219. doi: 10.1023/B:PLSO.0000020970.40167.40
Rao, M. R., Nair, P. K. R., and Ong, C. K. (1997). Biophysical interactions in tropical agroforestry systems. Agroforest. Syst. 38, 3–50. doi: 10.1023/A:1005971525590
Renwick, L. L. R., Kimaro, A. A., Hafner, J. M., Rosenstock, T. S., and Gaudin, A. C. M. (2020). Maize-pigeonpea intercropping outperforms monocultures under drought. Front. Sustain. Food Syst. 4, 562663. doi: 10.3389/fsufs.2020.562663
Rivest, D., and Vézina, A. (2015). Maize yield patterns on the leeward side of tree windbreaks are site-specific and depend on rainfall conditions in eastern Canada. Agroforest. Syst. 89, 237–246. doi: 10.1007/s10457-014-9758-6
Rockström, J., Edenhofer, O., Gaertner, J., and DeClerck, F. (2020). Planet-proofing the global food system. Nat. Food 1, 3–5. doi: 10.1038/s43016-019-0010-4
Rowe, E. C., van Noordwijk, M., Suprayogo, D., and Cadisch, G. (2005). Nitrogen use efficiency of monoculture and hedgerow intercropping in the humid tropics. Plant Soil. 268, 61–74. doi: 10.1007/s11104-004-0227-2
RStudio Team (2021). RStudio: Integrated Development Environment for R. Boston, MA, USA: RStudio, PBC.
Sanchez, P. A., Shepherd, K. D., Soule, M. J., Place, F. M., Buresh, R. J., Izac, A.-M. N., et al. (1997). “Soil Fertility Replenishment in Africa: An Investment in Natural Resource Capital,” in Replenishing Soil Fertility in Africa, eds. R. J. Buresh, P. A. Sanchez, and F. Calhoun (Madison, WI, USA: Soil Science Society of America American Society of Agronomy) 1–46. doi: 10.2136/sssaspecpub51.c1
Sapkota, A., Shrestha, R. K., and Chalise, D. (2017). Response of Maize to the Soil Application of Nitrogen and Phosphorous Fertilizers. Int. J. Appl. Sci. Biotechnol. 5, 537–541. doi: 10.3126/ijasbt.v5i4.18777
Schulte, L. A., Dale, B. E., Bozzetto, S., Liebman, M., Souza, G. M., Haddad, N., et al. (2022). Meeting global challenges with regenerative agriculture producing food and energy. Nat. Sustain. 5, 384–388. doi: 10.1038/s41893-021-00827-y
Sharma, A. R., Singh, R., Dhyani, S. K., and Dube, R. K. (2010). Moisture conservation and nitrogen recycling through legume mulching in rainfed maize (Zea mays)–wheat (Triticum aestivum) cropping system. Fertil. Res. 87, 187–197. doi: 10.1007/s10705-009-9327-y
Shiferaw, B., Prasanna, B. M., Hellin, J., and Bänziger, M. (2011). Crops that feed the world 6. Past successes and future challenges to the role played by maize in global food security. Food Sec. 3, 307–327. doi: 10.1007/s12571-011-0140-5
Sileshi, G. W., Debusho, L. K., and Akinnifesi, F. K. (2012). Can Integration of Legume Trees Increase Yield Stability in Rainfed Maize Cropping Systems in Southern Africa? Agron. J. 104, 1392–1398. doi: 10.2134/agronj2012.0063
Sileshi, G. W., Mafongoya, P. L., Akinnifesi, F. K., Phiri, E., Chirwa, P., Beedy, T., et al. (2014). “Agroforestry: Fertilizer Trees,” in Encyclopedia of Agriculture and Food Systems (Elsevier) 222–234. doi: 10.1016/B978-0-444-52512-3.00022-X
Song, L., Jin, J., and He, J. (2019). Effects of severe water stress on maize growth processes in the field. Sustainability 11, 5086. doi: 10.3390/su11185086
Suryanto, P., Putra, E. T. S., Kurniawan, S., Suwignyo, B., and Sukirno, D. A. P. (2014). Maize Response at Three Levels of Shade and its Improvement with Intensive Agro Forestry Regimes in Gunung Kidul, Java, Indonesia. Proc. Environ. Sci. 20, 370–376. doi: 10.1016/j.proenv.2014.03.047
Takle, E. S. (2015). “Agricultural meteorology and climatology,” in Encyclopedia of Atmospheric Sciences (Elsevier) 92–97. doi: 10.1016/B978-0-12-382225-3.00009-8
Tanumihardjo, S. A., McCulley, L., Roh, R., Lopez-Ridaura, S., Palacios-Rojas, N., and Gunaratna, N. S. (2020). Maize agro-food systems to ensure food and nutrition security in reference to the Sustainable Development Goals. Global Food Secur. 25, 100327. doi: 10.1016/j.gfs.2019.100327
Thevs, N., Strenge, E., Aliev, K., Eraaliev, M., Lang, P., Baibagysov, A., et al. (2017). Tree shelterbelts as an element to improve water resource management in central asia. Water 9, 842. doi: 10.3390/w9110842
Thomas, G. W., and Hargrove, W. L. (1984). “The Chemistry of Soil Acidity,” in Soil Acidity and Liming, ed. F. Adams (Madison, WI, USA: American Society of Agronomy, Crop Science Society of America, Soil Science Society of America) 3–56. doi: 10.2134/agronmonogr12.2ed.c1
Tiwari, T. P., Brook, R. M., Wagstaff, P., and Sinclair, F. L. (2012). Effects of light environment on maize in hillside agroforestry systems of Nepal. Food Sec. 4, 103–114. doi: 10.1007/s12571-012-0165-4
Tscharntke, T., Clough, Y., Bhagwat, S. A., Buchori, D., Faust, H., Hertel, D., et al. (2011). Multifunctional shade-tree management in tropical agroforestry landscapes - a review. J. Appl. Ecol. 48, 619–629. doi: 10.1111/j.1365-2664.2010.01939.x
Udawatta, R. P., and Jose, S. (2021). Agroforestry and Ecosystem Services. Cham: Springer International Publishing. doi: 10.1007/978-3-030-80060-4
Vanlauwe, B., Aihou, K., Houngnandan, P., Diels, J., Sanginga, N., and Merckx, R. (2001). Nitrogen management in ‘adequate' input maize-based agriculture in the derived savanna benchmark zone of Benin Republic. Plant Soil. 228, 61–71. doi: 10.1023/A:1004847623249
Vogel, E., Deumlich, D., and Kaupenjohann, M. (2016). Bioenergy maize and soil erosion — Risk assessment and erosion control concepts. Geoderma 261, 80–92. doi: 10.1016/j.geoderma.2015.06.020
Vyamana, V. G., Chamshama, S. A. O., and Andrew, S. M. (2021). Soil nutrients and maize yields responses to indigenous agroforestry tree post-fallows management in Tanzania. Trees, Forests People 6, 100164. doi: 10.1016/j.tfp.2021.100164
Waldron, A., Garrity, D., Malhi, Y., Girardin, C., Miller, D. C., and Seddon, N. (2017). Agroforestry can enhance food security while meeting other sustainable development goals. Trop. Conserv. Sci. 10, 194008291772066. doi: 10.1177/1940082917720667
Wong, M. T. F., Gibbs, P., Nortcliff, S., and Swift, R. S. (2000). Measurement of the acid neutralizing capacity of agroforestry tree prunings added to tropical soils. J. Agric. Sci. 134, 269–276. doi: 10.1017/S0021859699007388
World Agroforestry (2021). What is Agroforestry? Available online at: http://worldagroforestry.org/about/agroforestry (accessed April 01, 2023).
Keywords: agroforestry, alley cropping, maize (Zea mays L.), corn, relative yields
Citation: Baier C, Gross A, Thevs N and Glaser B (2023) Effects of agroforestry on grain yield of maize (Zea mays L.)—A global meta-analysis. Front. Sustain. Food Syst. 7:1167686. doi: 10.3389/fsufs.2023.1167686
Received: 16 February 2023; Accepted: 28 April 2023;
Published: 17 May 2023.
Edited by:
B. Mohan Kumar, Arunachal University of Studies, IndiaReviewed by:
Rashmita Sharma, Jawaharlal Nehru University, IndiaAjay Sharma, University of Florida, United States
Copyright © 2023 Baier, Gross, Thevs and Glaser. This is an open-access article distributed under the terms of the Creative Commons Attribution License (CC BY). The use, distribution or reproduction in other forums is permitted, provided the original author(s) and the copyright owner(s) are credited and that the original publication in this journal is cited, in accordance with accepted academic practice. No use, distribution or reproduction is permitted which does not comply with these terms.
*Correspondence: Bruno Glaser, bruno.glaser@landw.uni-halle.de