- 1Univ. Littoral Côte d'Opale, UMRt 1158 BioEcoAgro, USC ANSES, INRAe, Univ. Artois, Univ. Lille, Univ. Picardie Jules Verne, Univ. Liège, Boulogne-sur-Mer, France
- 2Sustainable AgriFoodtech Innovation & Research (SAFIR), Arras, France
- 3Research and Development, Hayan Group, Rhenen, Netherlands
- 4Information Technology Group, Wageningen University & Research, Wageningen, Netherlands
- 5Department of Preventive Medicine and Public Health, Food Science, Toxicology and Forensic Medicine, Faculty of Pharmacy, Universitat de València, Burjassot, València, Spain
- 6National Institute of Food Science and Technology, University of Agriculture, Faisalabad, Pakistan
- 7Department of Food Nutrition and Packaging Sciences, Clemson University, Clemson, SC, United States
- 8ERA-Chair in VALORTECH, Estonian University of Life Sciences, Tartu, Estonia
- 9Department of Biological Sciences, Norwegian University of Science and Technology, Ålesund, Norway
- 10Department of Food Science, College of Agriculture and Veterinary Medicine, United Arab Emirates University, Al-Ain, United Arab Emirates
- 11Department of Food Science, Cornell University, Ithaca, NY, United States
Providing food has become more complex because of climate change and other environmental and societal stressors, such as political instability, the growth in the world population, and outbreaks of new diseases, especially the COVID-19 pandemic. In response to these challenges, the agri-food industry has increased its efforts to shift to using more digital tools and other advanced technologies. The transition toward digital has been part of the fourth industrial revolution (called Industry 4.0) innovations that have and are reshaping most industries. This literature review discusses the potential of implementing digital technologies in the agri-food industry, focusing heavily on the role of the COVID-19 pandemic in fostering the adoption of greater digitalization of food supply chains. Examples of the use of these digital innovations for various food applications, and the barriers and challenges will be highlighted. The trend toward digital solutions has gained momentum since the advent of Industry 4.0 and implementations of these solutions have been accelerated by the outbreak of the COVID-19 pandemic. Important digital technology enablers that have high potential for mitigating the negative effects of both the current global health pandemic and the environmental crisis on food systems include artificial intelligence, big data, the Internet of Things, blockchain, smart sensors, robotics, digital twins, and virtual and augmented reality. However, much remains to be done to fully harness the power of Industry 4.0 technologies and achieve widespread implementation of digitalization in the agriculture and food industries.
1. Introduction
The declaration of COVID-19 as a pandemic by the World Health Organization (WHO) on 11 March 2020 has led to unprecedented challenges to public health and the global economy, including food supply chains and food systems. Due to the severe restrictions (e.g., lockdowns and social distancing) and the resulting labor shortages throughout the food value chain, this global health crisis has disrupted the current food systems and consumer eating behaviors, highlighting the importance of adaptability and resilience for a healthier and more sustainable food supply. The safety, quality, and traceability of food have become major concerns for consumers and food producers worldwide (Barone et al., 2021; Bisoffi et al., 2021; Han et al., 2021; Hassoun et al., 2022a,f).
New ways, alternative solutions, and technological innovations have been explored to deal with the COVID-19 crisis and mitigate the impact of this pandemic (Barrett et al., 2021; Galanakis et al., 2021). One of the solutions that has received enormous attention is digitalization, and research on digital transformation has, therefore, increased rapidly in the last few years. Indeed, data obtained from the Scopus database shows that there has been a growing literature on digitalization in agri-food systems over the last decade (Figure 1). The agriculture and food industry, as did other sectors, experienced increased digitalization before the COVID-19 pandemic, but the need for digital transformations became clearer during and after this crisis. Digitalization and automation have become increasingly ubiquitous as a way to increase productivity and improve efficient resource use, consistent with the need for a more sustainable food system (Ghobakhloo, 2020; Marvin et al., 2022; Abbate et al., 2023; Hassoun et al., 2023c).
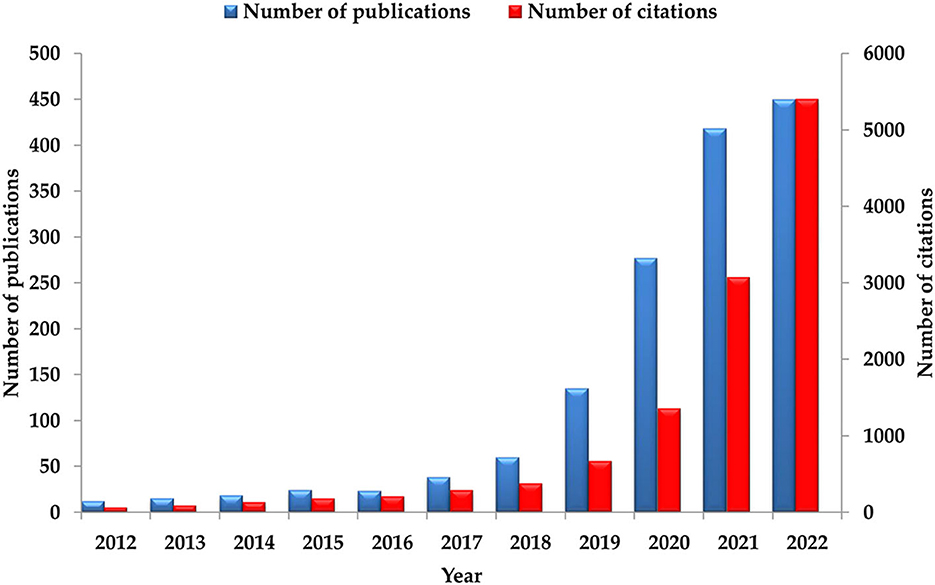
Figure 1. Number of publications and citations/year on the shift to digital in agri-foods over the last decade (search query was done using the Scopus database on 11th January 2023).
The concept of digitalization is strongly linked with Industry 4.0 (or the fourth industrial revolution). Many digital, physical, and biological domains are being combined, such as artificial intelligence (AI), the Internet of Things (IoT), big data, robotics, 3D printing, and smart sensors to create smart digital factories and farms (Lezoche et al., 2020; Hassoun et al., 2022c,e, 2023b). There is no specific classification or general agreement as to what are the Industry 4.0 technologies, but most reports within the agriculture and food industry include those mentioned above, plus blockchain, cloud computing, digital twins, and cyber-physical systems (Echegaray et al., 2022; Hassoun et al., 2022b,h). The Scopus data were analyzed using the graph network bibliometric visualization tool VOSviewer (VOSviewer.com, Leiden University, The Netherlands). According to the output, co-occurrence analysis identified >5,600 keywords, of which 111 occurred at least 10 times (Figure 2). Agriculture, digitalization, digital technologies, and digital transformation were the most frequent keywords identified (the size of the node indicates the frequency of the keyword), followed by Industry 4.0, and its elements, such as AI and IoT.
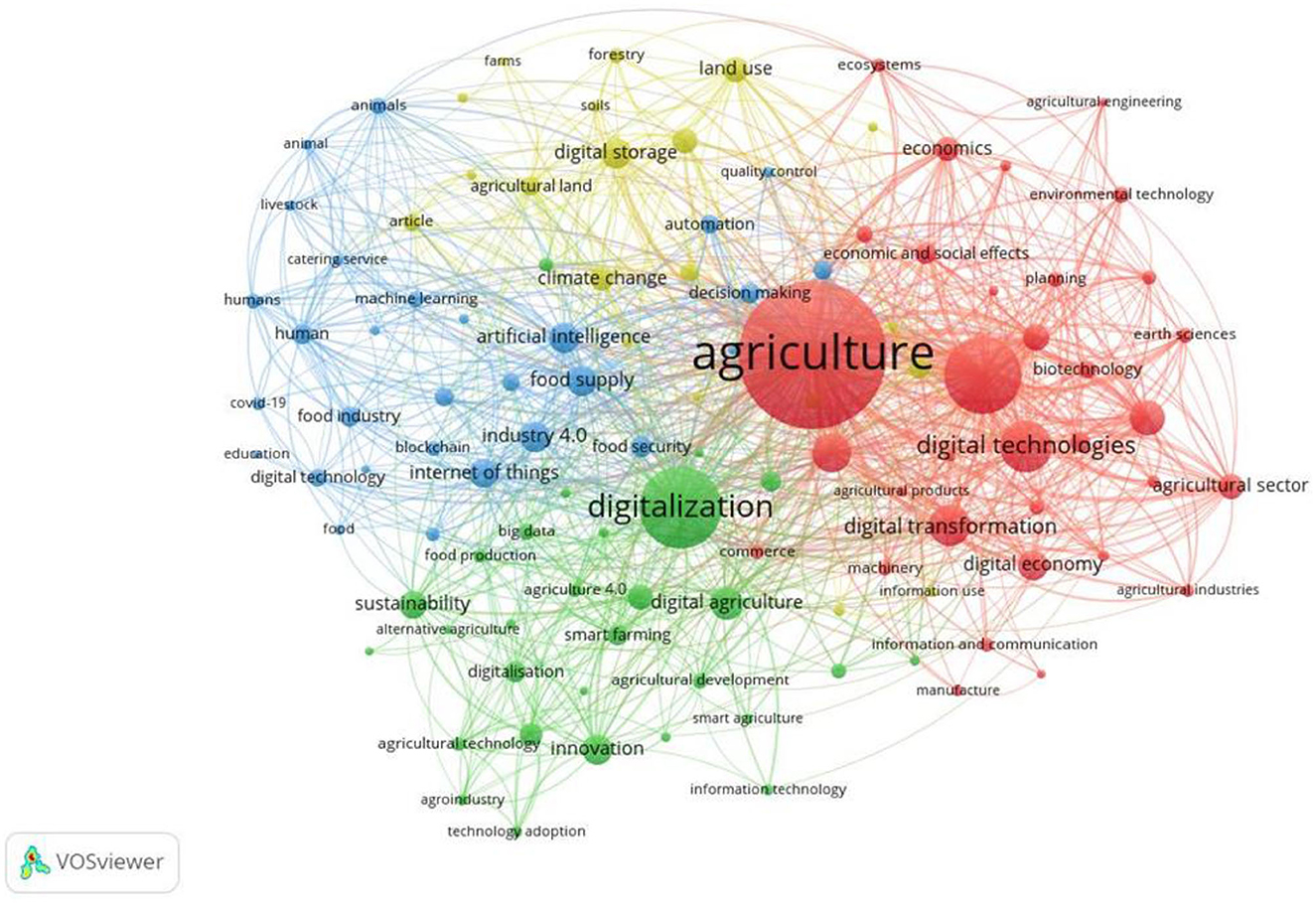
Figure 2. Network map of main keywords (with a minimum of 10 occurrences) identified using VOSviewer with the Scopus (CSV) database represented in Figure 1 (unit of analysis; all keywords, 111 words meet the threshold).
Several recent publications have reviewed the use of digital technologies and other Industry 4.0 elements to fight the COVID-19 pandemic in different fields, such as healthcare services (Chandra et al., 2022), international business (Pereira et al., 2022), and learning and teaching (Jakoet-Salie and Ramalobe, 2022). In the agri-food sector, the role of certain Industry 4.0 technologies, namely blockchain, IoT, big data analytics, and AI, in the digitalization of the food system has been highlighted (Amentae and Gebresenbet, 2021). The role of digital transition and Industry 4.0 technologies in enhancing food sustainability and achieving sustainable food systems has been also highlighted (Masi et al., 2021; Marvin et al., 2022; Abbate et al., 2023). Another recent publication has discussed the impact of Industry 4.0 technologies for a successful implementation of smart farming (Javaid et al., 2022). The significant role of digital technologies in addressing food loss and waste issues in agriculture was also recently demonstrated (Benyam et al., 2021; Lopes de Sousa Jabbour et al., 2021; Hassoun et al., 2023a). The implication of digitalization in the shift to plant-based diets (Hassoun et al., 2022d) and the dairy sector (Hassoun et al., 2023b) has also been explained. Di Vaio et al. (2020) demonstrated the significant role of AI in supporting the businesses struggling through the COVID-19 pandemic, and achieving the sustainable development goals in the water, energy, and food sectors (D'amore et al., 2022).
However, there is a gap in the literature since no comprehensive review has explored the role of all Industry 4.0 components in accelerating the shift to digital in the agri-food sector during the COVID-19 era. Indeed, little attention has been given to the COVID-19 pandemic and its critical role in driving the adoption of digital technologies in different food-related sectors. Thus, based on the recent literature (within the past 5 years), this review will identify the important enablers of digital transformation and briefly describe their implication in mitigating the negative consequences of the COVID-19 pandemic in agriculture and the food industry. In this literature review will try to bridge the aforementioned literature gap by answering the following two research questions: what are the main enablers of the digital transformation in the agri-food sector: how could digital technologies help mitigating the negative effects of the COVID-19 pandemic.
The methodology used in this literature review process can be summarized as follows: publications search, selection, and collection using leading data sources (especially Scopus and Google Scholar). The following keywords were included in the search string: TITLE-ABS-KEY (Digital transformation) OR (Digital shift) OR (Shift to digital) OR (Digitalization) AND (Agri-food) OR (Agriculture) OR (Food industry).
2. Overview of the main enablers of the digital transformation
Several emerging technologies can be considered enablers of the digital transformation in agri-food sectors (Figure 3). A short introduction to these technologies follows.
2.1. AI and big data analytics
AI and big data analytics are at the core of digital transformation. AI is a branch of computer science that simulates human thinking processes, learning ability, and knowledge storage to enable the performance of tasks that typically require human intelligence (Addanki et al., 2022; Fuentes et al., 2022). AI and data-driven innovations can address many challenges to the food supply chain related to public health and mitigation of risks, improvement of efficiency and accuracy, and implementation of sustainability initiatives. AI-based techniques, combining data from multiple sources, can have a role in providing predictive risk analysis as well as prescriptive decision-support (Lezoche et al., 2020; Marvin et al., 2022; Misra et al., 2022). The possibilities of such an approach improving food safety, food fraud detection, sustainability and quality have been recently illustrated (Bouzembrak et al., 2019; Marvin and Bouzembrak, 2020; Marvin et al., 2020; Sharma A. et al., 2021). A recent review paper has given a general overview of implications of AI in precision agriculture to analyze data from sensors, drones, and satellites and optimize many farming practices, such as irrigation, fertilization (Taneja et al., 2023).
Big data and cloud technologies have been predicted to grow along with AI, especially after COVID-19. Handling the large amount of data generated along the food supply chain is still challenging and time-consuming and requires a large computational infrastructure. Although cloud computing has emerged as a solution in other sectors, its use with the food chain has just begun (Wang and Wang, 2021; Rejeb et al., 2022b). Cloud computing might accelerate the implementation of the “lab-to-sample” approach in which food safety data is generated on-site using, for example, portable devices and sensors, and transferring it to a central cloud-based infrastructure, where it is processed and analyzed on-line. The results would then be sent back to plant operators allowing real-time decisions to assure compliance with food safety legislation and standards. This approach would further reduce costs and enhance the efficiency of food safety controls since fewer samples will be needed for the laboratory analysis. If IoT can be applied, a potential food safety risk could be detected quickly allowing actions to mitigate the risks (Jin et al., 2020; Kamble et al., 2020; Marvin et al., 2022). The use of big data in agriculture and smart farming has led to significant advantages suc has crop modeling for targeting genotypes to different environments, forecasting crop harvest and improve agriculture monitoring, and increasing efficiency of agricultural operations (Jin et al., 2020; Rejeb et al., 2022a,b).
2.2. IoT and smart sensing
IoT provides numerous options for gathering data in real-time from any stage of the food supply chain using sophisticated sensors and other devices to monitor the environment and production conditions (e.g., temperature, pressure, conductivity, pH, and color of products) starting at the farm. Other sensors can provide information on quality and machine operations both in the manufacturing plant, and during transportation and storage (Bouzembrak et al., 2019; Marvin et al., 2022). IoT sensors can be used to predict quality and shelf-life of agricultural products, detect food adulteration, and improve food safety. For example, IoT-based sensors are being developed to monitor temperature and oxygen content in frozen food and packaged products. The used sensing technologies are based on photoacoustic spectroscopy, quasi-imaging spectrometry and temperature, which are integrated in a multi sensor device for real-time monitoring of several quality parameters. Thus, IoT and smart sensors can provide full tracking of the product, along with its ingredients, properties, and production history (Misra et al., 2022; Xu et al., 2022). In agriculture, smart sensors are being increasingly applied for many purposes such as assessment of weather conditions and soil quality, and crop monitoring, achieving higher crop yields and less cost (Saiz-Rubio and Rovira-Más, 2020; Ullo and Sinha, 2021).
2.3. IoT and blockchain
Blockchain creates digital, decentralized, and distributed ledgers that store records of transactions in a peer-to-peer network in “blocks.” Each link in the supply chain adds a block that once added cannot be changed with all links having access. The use of blockchain technology combined with IoT represents a promising strategy to guarantee real-time visibility, transparency, security, immutability, irrevocability, neutrality, and reliability of the information and actions of all the supply chain actors. It can track in real-time the food's properties along the supply chain to ensure food safety and quality, especially when combined with IoT (Zhao et al., 2019; Jin et al., 2020; Bigliardi et al., 2022; Kayikci et al., 2022). The potential of blockchain in agriculture has been highlighted as a promising technology toward a transparent supply chain of food, providing significant benefits to all participants (including provider, producer, processing, distribution, retailer, and consumer) involved along the food supply chain (Kamilaris et al., 2019).
2.4. Robotics and smart machinery
Agricultural automation and robotics using automatic controls and AI can be used at all levels of the agricultural production and the following manufacturing processes (Marvin et al., 2022; Ramirez-Asis et al., 2022). Smart machines can independently complete several tasks, such as cultivation, sowing, transplanting, fertilization, spraying, feeding, irrigation, picking, and harvesting. These machines can also collect information on soil and water quality, and plant or animal growth, which provides technical support for the implementation of precision agriculture and better breeding choices (Xu et al., 2022). Robots can perform many tasks along the post-harvest supply chain, such as sorting, storing, picking and packing, and shipping (Chauhan et al., 2022). Current robotic development is focused on replacing manual operations in food handling and processing, food packaging, food delivery, and other operations along the food supply chain (Duong et al., 2020; Sulaiman et al., 2021). Advanced robotics are being increasingly involved in many agricultural practices such as sowing, planting, plant treatment, harvesting, yield estimation and phenotyping (Oliveira et al., 2021).
2.5. Digital twins and virtual or augmented reality
A digital twin is a digital representation of a real-world physical entity (i.e., a real process operation or physical system). Digital twins can be used to model activities that will lead to increased productivity, resource efficiency, energy efficiency, and cost reduction (Verboven et al., 2020; Kamble et al., 2022). Augmented reality technology is being used in several sectors, including the food sector (Rejeb et al., 2021; Chai et al., 2022). A recent study showed that virtual reality could help adults be more successful in weight management, e.g., enabling them to reduce food intake after strenuous physical activity (Chai et al., 2022). However, the use of digital twins and virtual or augmented reality in agriculture is still very limited.
2.6. Other industry 4.0 technologies
Many other Industry 4.0 smart technologies including remote sensing technologies, such as imagery from satellite sensors, unmanned aerial vehicles (UAV), and drones, as well as other advanced technologies, such as 3D food printing have been developed or are under development in smart farming and smart food factories (Bedoya et al., 2022; Boursianis et al., 2022; Javaid et al., 2022).
3. Examples of applications of digital technologies in agriculture and food sectors
The need for high-quality food and improved sustainability has accelerated the integration of digital and smart technologies in food manufacturing (smart factories), agriculture and production systems (smart/precision agriculture and farming), and other applications along the food value chain. This section will present some examples of these applications.
3.1. Precision agriculture and smart farming
Digital technologies and other advanced innovations (e.g., AI and big data, IoT, smart sensors, satellite imagery, and drones) and smart machinery are being increasingly adapted for use in agriculture, leading to the emergence of new concepts, such as precision agriculture and smart farming (Idoje et al., 2021; Kumar et al., 2021; Musa and Basir, 2021; Javaid et al., 2022; Rejeb et al., 2022a).
The use of AI with smart agriculture has been shown to be efficient in predicting agricultural performance based on present and past conditions, achieving many benefits, such as minimizing crop diseases and pest infestation and predicting the weather, soil properties, and crop yield, among others (Pathan et al., 2020; Sharma A. et al., 2021; Tomar and Kaur, 2021). Moreover, AI-based models are being investigated for the development of innovative food production systems such as vertical farming (Siregar et al., 2022). AI models are often used in combination with other cornerstones of smart farming, especially IoT (in addition to big data, smart sensors, and cloud computing). As most farming processes are becoming increasingly data-driven and data-enabled, data management can be a challenging task, which accentuates the need for innovative approaches. For example, Ouafiq et al. (2022) proposed an AI-based comprehensive architecture called Smart Farming Oriented Big-Data Architecture that includes big data technologies, IoT components, and knowledge-based systems to ensure agricultural sustainability and environmental protection, and achieve high quality, productivity, and efficiency.
IoT has been widely applied in smart farming, and can enable soil monitoring, irrigation, crop, and waste management, livestock surveillance, among others, boosting farming activities to new heights (Musa and Basir, 2021; Holzinger et al., 2022; Sinha and Dhanalakshmi, 2022). Recently, it was argued that IoT has significant potentials to speed up the transition to precision agriculture, highlighting publication trends and important topics in agricultural IoT research, as well as agricultural resources that are impacted by IoT (Rejeb et al., 2022c). The main application areas of IoT in the agricultural sector include monitoring, control, logistics and prediction (Bouzembrak et al., 2019). A recent study investigated the possibility of using IoT coupled with UAV and AI for monitoring pests in the rice field during the production (Bhoi et al., 2021). As this study focused only on rice field monitoring, the authors envisaged further investigations to develop similar models that can be used in the following rice production stages. Srivastava et al. (2020) proposed a simple and cost-effective IoT-based system involving sensors that could measure and monitor important soil parameters, such as moisture content. IoT and other advanced technologies help take preventive measures to reduce losses, increase the productivity of crops, and achieve higher yields and better-quality produce.
Drones, robotics, UAV, satellites, and other smart machinery and remote sensing technologies are being increasingly applied in the transition to smart farming and digital agriculture. With recent developments in deep learning, machine learning, and IoT, the application of drones in precision agriculture has been growing steadily over the last years, as recently highlighted by Rejeb et al. (2022a). Robotics are being used to perform many tasks that are often tedious and sometimes strenuous in plant agriculture, such as land preparation, sowing, planting, plant treatment, harvesting, yield estimation, and weeding (Lezoche et al., 2020; Sharma A. et al., 2021; Misra et al., 2022). For example, a harvester robot using dual-arm manipulation combined with an image segmentation algorithm with a dynamic planning algorithm and an occlusion algorithm was developed for automatic aubergine harvesting (Sepúlveda et al., 2020). The system had a success rate of 92% and an average cycle time of 26 s/harvested aubergine.
Precision livestock farming systems involve monitoring animals and their environment using smart sensors, AI, and other digital technologies to maintain productivity and profitability, improve animal health, and decrease the environmental footprint (Bao and Xie, 2022; Fuentes et al., 2022). For example, in smart swine farming, relevant information can be collected from different sensors (e.g., cameras, microphones, accelerometers or radio-frequency identification transponders), images, and sounds, among others, to enable non-invasive monitoring of animals and ensure good health, welfare and optimum production (Racewicz et al., 2021). Nasirahmadi et al. (2019) developed a system to detect the standing and lying postures of pigs with commercial farm conditions using a two-dimensional imaging system combined with different deep learning approaches. The system worked well with different lighting conditions, ages of animals, and skin color, offering promising opportunities to achieve real-time, continuous computer-based monitoring of livestock. In a recent study, a machine learning framework was developed to predict daily milk yield, composition (fat and protein content) and the frequency of individual cow milking needs, achieving a high accuracy of prediction (Ji et al., 2022).
However, smart farming (producing crops and raising animals) still seems to have had limited practical applications in industry due to numerous challenges that need to be solved (Musa and Basir, 2021; Fuentes et al., 2022). For example, farmers are often lacking proper infrastructure and skills needed to use digital technologies and other advanced innovations. Moreover, high costs, privacy, data security and ethical issues, and organizational barriers and regulations are still hindering the wider spread of smart farming and precision agriculture in rural and poorer areas, especially in developing countries (Demestichas et al., 2020; Musa and Basir, 2021; Rejeb et al., 2022a,c).
3.2. Digital technologies for smart factories
The “smart food factory” is based on adopting high levels of automation and digitalization to increase productivity, production efficiency, and food quality and safety (Chen et al., 2017; Shi et al., 2020; Echegaray et al., 2022). Many manufacturing industries are moving to connecting processing lines and intelligent machines to communicate with each other in an automated production chain, with minimal human involvement (Kumar et al., 2021; Watson et al., 2021; Dzedzickis et al., 2022). Among the different Industry 4.0 innovations, robots, AI, big data, cloud computing, blockchain, and smart sensors are considered the main contributors to the smart food factory (Figure 4). Growing research shows that the implementation of these advanced technologies in food manufacturing has the potential to contribute to environmental sustainability by reducing production costs and human errors, maximizing efficiency, and achieving autonomous predictive management.
Food manufacturing is becoming more complex, including several steps where robotics can help. For example, in the meat industry a human-robot collaboration can be achieved to address many challenges (e.g., heterogeneity of meat pieces and inconsistency of cutting trajectories) to help modernize the production line (Romanov et al., 2022). The rapid development of AI technologies offers unprecedented opportunities to accelerate advances in robotics technology with both hardware and software control systems (Dzedzickis et al., 2022). The most common robotic systems in the food industry do “pick and place,” i.e., they handle a specific type of object presented in a consistent, pre-defined orientation. Any variation in the object's shape and size can lead to failure to complete the pick and place task, making precise handling of irregular, naturally varying objects, such as vegetables and fruit, a greater challenge (Bader and Rahimifard, 2020; Chauhan et al., 2022). Soft robotics technology, soft grippers, and machine learning techniques have shown the potential to practically address the handling of irregular and naturally varying objects with low cost (Navas et al., 2021; Wang et al., 2022). For example, the application of automation using intelligent machines and robots to perform many tasks in red meat slaughter processes, such as carcass cutting and deboning in abattoirs was recently highlighted (de Medeiros Esper et al., 2021; Ross et al., 2022). A recent study focused on the digitalization of the supply chain of the food and beverage industry in Indonesia (Saryatmo and Sukhotu, 2021). The authors found that the adoption of digital technologies, such as robotics, big data, cloud computing, blockchain, and IoT can positively influence the quality, productivity, and cost reduction performance, and create considerable value-added and monetary gain.
AI solutions offer the potential to revolutionize different areas of the food sector, such as developing indicators that reduce or eliminate food safety risks before they appear (Kudashkina et al., 2022; Ramirez-Asis et al., 2022). A broader application of AI in the food industry can increase digitalization and real-time surveillance, leading to greater resilience and supporting the transition toward more sustainable food production systems (Kumar et al., 2021; Marvin et al., 2022). However, using AI within the food supply chain raises ethical questions related to privacy, accessibility, accountability, and responsibility that should be addressed by developing suitable protocols (Manning et al., 2022).
Another important feature of a smart food factory is the prevalence of smart sensors for real-time monitoring. For example, using smart sensors and IoT has the possibility to detect faults and quickly adjust processing conditions, such as the internal temperature and humidity of a processing line (Misra et al., 2022). The application of miniaturized smart sensors based on spectroscopy principles for monitoring food quality is another important research area (McVey et al., 2021).
3D food printing is another promising digitalized innovative technology that has been gaining growing interest in recent years and can be considered as one of the smart food factory technologies. Recent advances in AI and other digital technologies make it possible to use 3D printing to create food of complex shapes or to develop protein food from novel protein sources, such as, insects, fungi, and algae (Dankar et al., 2018; Addanki et al., 2022; Bedoya et al., 2022).
3.3. Blockchain technology in the agri-food supply chain
The agri-food supply chain is an interlinked chain with multiple actors. Different participants are involved in different tasks, such as farming, processing, shipping, distributing, and retailing. Blockchain technology could solve different problems related to food security, safety and integrity, and support increased competitiveness of small farmers if equal access to these technologies can be developed (Hassoun et al., 2022a; Kayikci et al., 2022; Zhang et al., 2022).
Tracing food products along the entire supply chain is important for achieving better management of food products. Various frameworks and traceability systems based on blockchain technology have been proposed in recent years for many applications in the agri-food sector. For example, Wang L. et al. (2022) proposed a traceability system framework integrating a decentralized blockchain-enabled data storage platform (used for data management) and a radio frequency identification (RFID) technology (used for data collection and limited data storage). A creative blockchain-based RFID tag that can be attached to a food package was designed to have a high-security level and low cost. Another interesting application of blockchain technology and IoT was suggested by Khan M. A. et al. (2022), who developed a “Shrimp Chain”: a blockchain-based transparent and traceable framework to help with the export of Bangladeshi shrimp. They proposed a scoring-based certification method for supply chain stakeholders involved with shrimp to detect fraud in packaged shrimps along the entire shrimp supply chain, and to encourage traceability and food safety. A platform based on blockchain was designed for the traceability of the supply chain of Fontina cheese produced in the Aosta Valley region in Italy, but can be used for any other dairy product (Varavallo et al., 2022). This blockchain design was characterized as “green” due to low energy consumption and minimum environmental impact. Other blockchain-based traceability systems were suggested for fruits and vegetables (Yang et al., 2021).
The combination of blockchain with other digital technologies (such as IoT and big data) can enhance transparency in food safety and quality. For example, the combination of blockchain with IoT was reported to improve business processes and customer satisfaction (Grecuccio et al., 2020). Zhang et al. (2022) reported that blockchain combined with IoT can collect and provide consumers with reliable data about when and where the fruit was picked. However, current applications of blockchain technology in the agri-food sector are still facing many challenges that need to be addressed. These include among others privacy concerns, high cost, lack of standards and regulations, as well as lack of skills (Zhao et al., 2019; Kayikci et al., 2022).
3.4. IoT for food waste management
IoT represents a promising approach and a viable solution to reduce waste since it provides transparency and the real-time status of resources consumed or wasted at various stages in the food supply chain through smart systems (Amentae and Gebresenbet, 2021; Bigliardi et al., 2022). An interesting application of IoT for food waste management was developed for the potato industry by Jagtap et al. (2019). The authors proposed an automated and real-time system to measure the overall amount of waste as well as the reasons for waste generation in real-time by using image processing and load cell technologies. The camera images were processed using a convolutional neural network (CNN) to classify the damaged and unusable potatoes into seven categories: diseased, blackened, cracked, sprouted, green, uncleaned, and cut-marked. The results could be used to implement corrective actions. In a recent study, the use of IoT in rearing black soldier fly was investigated to mitigate food waste (Cheng et al., 2022). The results showed that the implementation of sensors/devices, software, and mobile application could help to monitor this farming remotely. Another study showed the potential of using IoT in decentralized biogas plants, enabling a remote monitoring of food waste treatment (Logan et al., 2019).
4. Role of the COVID-19 pandemic in accelerating the food digital transformation
4.1. The COVID-19 pandemic and the agri-food industry
The COVID-19 pandemic has created significant challenges for humanity and caused many disruptions in different supply chains, including the agri-food industry, showing their weaknesses and vulnerability. This pandemic and its socio-economic consequences have had direct implications for food sectors, primarily affecting food safety, food security, and food sustainability (Knorr and Augustin, 2021; Rahaman et al., 2021).
The rapid spread of the COVID-19 pandemic has pushed many governments to impose lockdowns and severe restrictions of human movements that have led to labor shortages, including the food supply chain, disrupting the relatively seamless flow of raw materials in agriculture, food, and beverage industries. The impact of the crisis was visible at the beginning of the pandemic outbreak (in early 2020) with images of empty supermarket shelves appearing in mainstream media due to panic buying and stockpiling of essential goods, especially toilet paper, hand sanitizer, and masks. However, COVID-19 pandemic has been an innovation booster and digital stimulator in many fields, including the agri-food systems (Bakalis et al., 2020; Barrett et al., 2021).
New trends have emerged, including a renewed focus on locally produced foods, a shift in demand from food service to food retail, and an emphasis on reduced food waste. These trends have highlighted the need to implement radical changes and transform the existing food systems to make them more sustainable (Bisoffi et al., 2021; Knorr and Augustin, 2021).
4.2. Application of digital technologies to mitigate the COVID-19 impact on various food sectors
The pandemic has accentuated the pressing need for technological innovations and highlighted the importance of implementing new solutions to reduce human-to-human and human-to-food contact (Chitrakar et al., 2021). Many digital innovations (Figure 5) have been developed to mitigate the negative impact of the COVID-19 pandemic and accelerate the move toward more sustainable food systems.
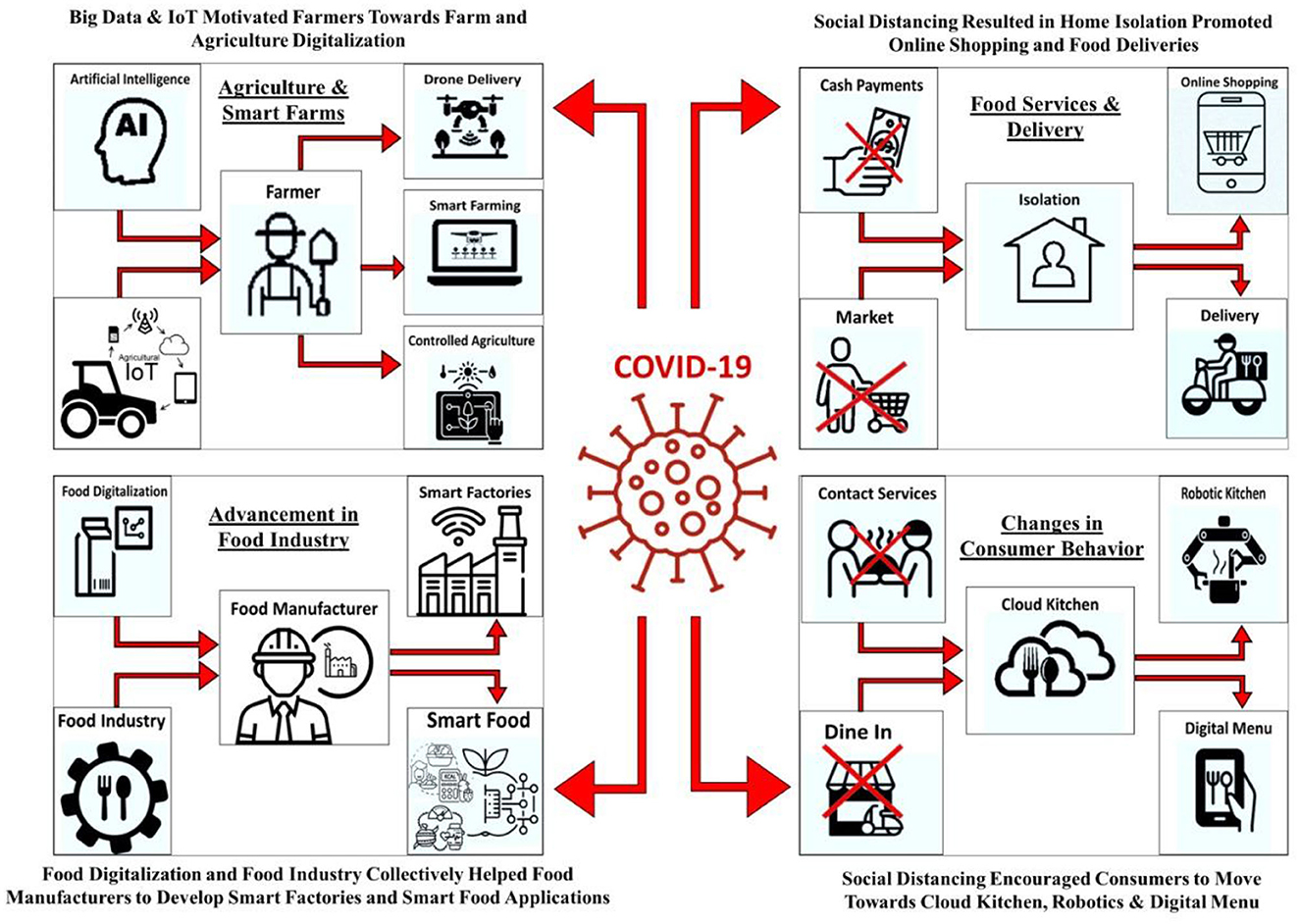
Figure 5. The role of the COVID-19 pandemic in accelerating the digitalization in many food-related areas.
4.2.1. Sustainable food systems and food supply chains
The realization of sustainable food systems from farm to fork requires a full consideration of the economic, environmental, and social dimensions of all aspects related to the production and consumption of sufficient and healthy food (Barrett et al., 2021; Bisoffi et al., 2021; Marvin et al., 2022). Marvin et al. (2022) highlighted the need for a system approach based on digitalization and AI, and the importance of data availability for actors from farmers to consumers to achieve sustainable food systems. The authors made valuable policy recommendations for a successful future implementation of AI to support the transition toward more sustainable food systems.
Big data, the cloud, and information and communication technologies are predicted to accelerate together with AI especially after the COVID-19 pandemic (Amentae and Gebresenbet, 2021; Wang and Wang, 2021). As mentioned earlier, handling the large amount of data generated along the food supply chain is still challenging and time-consuming, and requires a large computational infrastructure. Although cloud computing has emerged as a solution in other sectors, its use in the food chain remains limited (Jin et al., 2020; Rejeb et al., 2022b). Cloud computing might accelerate the implementation of the “lab-to-sample” approach in which food safety data is generated on-site (e.g., portable devices and sensors) and transferred to a central cloud-based infrastructure, where it is processed and analyzed online. The results are returned to the appropriate users allowing immediate relevant decisions that might not otherwise be possible (Jin et al., 2020; Kamble et al., 2020; Marvin et al., 2022).
Blockchain technology can also help food supply chains in controlling demand and supply shocks and providing real-time monitoring and information sharing. It can be a catalyst to improve flexibility, traceability, and develop shorter supply chains, which may assist in mitigating risk and disruption in the event of another pandemic or other disruptors (Sharma M. et al., 2021). For example, integrating satellite imagery and blockchain technology might address fish supply chain challenges to generate new opportunities for low income fisher-folks, especially in developing nations (Sengupta et al., 2021).
The implementation of digital technologies in the agri-food supply chain requires first and foremost the availability of adequate, cheap, and sustainable energy sources. The current energy crisis in many parts of the world underlines the need, at this time more than ever before, for innovative solutions to mitigate the negative consequences of the COVID-19 pandemic and achieve sustainable economic development and growth, especially in developing countries (Oruma et al., 2021). Promoting digital solutions and innovative thinking in agriculture and the food industry is undoubtedly crucial to transform agri-food systems (Hassoun et al., 2022g).
4.2.2. Inspections for food quality, safety, and traceability
Ensuring food quality, safety, and traceability during the COVID-19 pandemic has been a topmost priority. Many innovative strategies, such as smart monitoring technologies and smart detection systems have been developed and suggested as possible solutions to mitigate the negative consequences of the pandemic and to enhance food quality and safety (Chitrakar et al., 2021; Alabi and Ngwenyama, 2022). The role of packaging has been a focus, particularly with the recent development of nanocarriers that can be used as part of active packaging to increase shelf life and maintain food quality during food transportation (Pascuta and Vodnar, 2022).
Recent publications have highlighted the role of Industry 4.0 technologies to enhance food quality (Hassoun et al., 2023c) and food traceability (Hassoun et al., 2022a). For example, the use of IoT, big data, blockchain, and digital twins has been proposed to improve meat quality and ensure meat safety (Ren et al., 2022). A new framework based on IoT combined with blockchain was suggested to ensure food safety and reliable traceability of food cold chain systems (Qian et al., 2022). The proposed system can improve cold chain management, reducing the risk of contaminated products by making a rapid response to incidents possible. Digital technologies can provide full transparency across the supply chain and help consumers to trace the origin of products they purchased. For example, a blockchain-based traceability system was developed to reduce the risk of the COVID-19 transmission through the frozen meat supply chain by applying a tamper-proof tracing strategy (Iftekhar and Cui, 2021).
4.2.3. Food waste management
Food waste reduction has become one of the trendiest topics during the COVID-19 pandemic due to the increased focus on the environment and sustainability. Reducing food waste can enhance the three sustainability pillars—environmental, economic, and social (Barbar et al., 2022; Hassoun et al., 2022g). However, food waste increased early in the crisis due to panic-buying and food stockpiling by consumers, on the one hand, and the lockdown, restrictions on human movement, and closure of food production facilities, on the other hand. This led to more food wastes at the production and distribution levels along with those at the consumer level (Bhakta et al., 2020; Benyam et al., 2021; Boyacι-Gündüz et al., 2021). Therefore, a growing demand for the use of Industry 4.0 technologies (such as AI, IoT, blockchain, and smart sensors that allow real-time monitoring and tracking of food and food waste) has been seen during the COVID-19 crisis, to reduce food loss and waste (Galanakis et al., 2021). Among the various Industry 4.0 enablers, the IoT has attracted particular attention due to its ability to ensure higher levels of traceability and safety in supply chains and to reduce waste (Bigliardi et al., 2022). Moreover, the COVID-19 pandemic has also shown the importance of blockchain to ensure food safety and freshness as an additional way to reduce food waste (Khan H. H. et al., 2022).
The development of biodegradable packaging made from food wastes and by-products intensified during the pandemic (Barone et al., 2021). New extraction methods are being applied to valorize various food waste and by-products to obtain a range of bioactive compounds (Thirumdas et al., 2021; Madureira et al., 2022). The application of Industry 4.0 technologies in the food packaging (called Packaging 4.0) can provide diverse advantages, such as real-time monitoring food quality and safety, extension of the shelf life, in addition to the reduction of food waste (Sadeghi et al., 2022). Another efficient strategy that can be used to achieve a sustainable management of food waste is the development of 3D printed foods using food waste materials (Lee, 2021). This innovative technology has a huge potential in designing personalized healthy diets, reducing the dangerous effects of COVID-19 on people health, as well as mitigating the issue of food waste (Derossi et al., 2021).
4.2.4. Food service and food delivery
Food service includes commercial (e.g., restaurants, coffee shops, and hotels) and institutional (e.g., hospitals, schools, and institutions) sectors. As these sectors normally involve socializing, the social distancing regulations imposed by COVID-19 have negatively impacted the food service industry (de Souza et al., 2022). Although WHO and FAO reported that transmission of the COVID-19 through food is highly unlikely, many consumers still fear the possible transmission of the virus via food and packaging (Han et al., 2021). To cope with these concerns, the adoption of digital technologies, such as contactless digital payment, service robots, online ordering and delivery applications has increased considerably in recent years (de Souza et al., 2022).
Many restaurants, which are no longer a part of a consumer accessible facility, have started to use cloud kitchens (often referred to as ghost, virtual, dark, or cyber kitchens). These emerging business models for restaurants lead to less labor and lower capital expenditures compared to conventional restaurants (John, 2021). Moreover, the COVID-19 pandemic has boosted innovation in the development of new solutions for home delivery and take-out (de Souza et al., 2022).
The use of drones and robots (such as cooking, serving, and delivery robots) increased during the pandemic as ways to limit the spread of the virus (Choe et al., 2021). For example, an increasing number of coffee shops are using a robot serving barista. A study of 300 people using the robot barista in a coffee shop in South Korea showed that the service provided induced positive emotions and behavioral intentions (Yoo et al., 2022). However, the high price of delivery drones and robots in addition to the time needed for consumers to become familiar with their operating procedures, along with privacy and security issues need to be solved (Choe et al., 2021).
Because of the pandemic and the government imposed social distancing, many restaurants have had to change their business models by implementing innovative approaches to online food delivery (OFD) services. The rapid growth of OFD has been backed by the development of a range of user-friendly food delivery applications (such as Deliveroo, Uber Eat, and Just Eat) and an increase in smartphone users (Partridge et al., 2020; Zanetta et al., 2021).
Although these new business models have experienced booming progress, some obstacles remain. Among the challenges are developing products that travel well and packaging that preserves food quality so as to increase the experiential value perceived by consumers that, in turn, increases consumers' willingness to order food online (Gavilan et al., 2021). However, the greater use of OFD could also lead to poorer food choices (Jia et al., 2022). For example, independent takeaway outlets using Sydney's market-leading OFD platform (i.e., Uber Eats) showed that cereal-based meals such as pizzas, burgers, pies, pasta, wraps, and sandwiches were the largest category selected (Wang et al., 2021).
5. Barriers facing the adoption of digital and other new technologies
The food and agricultural sectors are complex and multidimensional, and they require digital and other advanced technologies (including AI, big data, IoT, smart sensors, robotics, blockchain, digital twins, and augmented reality) to meet the current unprecedented challenges. However, there are many obstacles and pitfalls that still limit the possibilities of a wider adoption of digitalization in agri-food systems. The main barriers include the lack of adequate infrastructure, limited institutional capacities, high costs and the investment involved, farm size, industrial set-up, lack of essential training for the personnel using the systems, and finally integrating these systems into the routines of all the decision-makers. In addition, there are the regional socio-economic-environmental-political hurdles influencing the practical applications of new technologies (Amentae and Gebresenbet, 2021; Chapman et al., 2021; Herrero et al., 2021). These challenges to digitalization existed since the emergence of Industry 4.0 but became more obvious during the COVID-19 pandemic.
Two types of obstacles that are reducing the uptake of digital technologies can be highlighted: (i) general challenges, that are valid for almost all technologies, and (ii) specific challenges that are related to each Industry 4.0 element.
The investment required to successfully adopt the new technology is among the main challenges for farmers and food companies that always need to consider profitability, and implementing digitalization depends on an increased profit after investments, i.e., a reasonable return of investment. Paudel et al. (2020) investigated the reasons behind the adoption of advanced technologies (precision technologies), based on evidence from U.S. cotton producers, and found that the profitability was the main motivation, followed by the environmental benefit and/or the desire to be at the forefront of the technology. Another general challenge is the conservative nature of the food industry, such as the silo mentality of a range of actors and organizations involved in the food supply chain that could slow the acceptance of new technology, collaboration, or data sharing (Serazetdinova et al., 2019; Hassoun et al., 2020). Moreover, lack of knowledge and digital skills, lack of familiarity with digitalization, and the absence of appropriate education and training programs can lead to a mistrust of the technology and hinder the understanding of the benefits of the use of new technologies and their incorporation into the supply chain (Amentae and Gebresenbet, 2021; Chapman et al., 2021).
Besides these common challenges, specific limitations associated with each enabler of the digital transformation have been identified. For example, ethical considerations should be taken into account when developing and deploying AI in the food and agricultural sectors (Manning et al., 2022). Big data provides large opportunities to make real-time decision, influencing the entire food supply chain, but issues related to data ownership, privacy, and security need to be addressed (Jin et al., 2020; Rejeb et al., 2022b). Blockchain has become a significant contributor to smart farming/precision agriculture and smart food factories, enabling full traceability, transparency, integrity, and secure transactions (Kayikci et al., 2022; Khan H. H. et al., 2022). However, its implementation is facing multiple barriers. Zhao et al. (2019) identified six challenges related to blockchain technology, including privacy leakage, storage capacity and scalability, lack of skills, high costs, regulatory problems, and throughput and latency issues (i.e., limited transaction capacity and the long time required for generating blocks and validating transactions). Research shows promising applications of IoT in several food-related fields, such as food safety and traceability, although issues related to inadequate security features and different standards for IoT communication protocols need to be addressed (Bouzembrak et al., 2019).
Replacing humans with smart robots and drones may be efficient, particularly in the current pandemic era, but implementing costs and the overall maintenance can be expensive, especially for small-scale farming and production facilities (Kumar et al., 2021; Rejeb et al., 2022a). Although smart sensors have the ability to make processes more intelligent and efficient, lack of cost-effective sensing technologies capable of monitoring key parameters and converting sensor data into actionable information remain challenging (Watson et al., 2021). Limited applications of digital twins for food process operations have been reported, since many obstacles (e.g., the need for specific programming and different modeling methods) still need to be overcome (Verboven et al., 2020). Likewise, only a few examples have been found in the literature about the application of augmented reality in the agriculture and food sector. This seems to be due to technical challenges associated with the strong dependence on the coverage and speed of connection, effect of background on food recognition rate, difficulties to identify objects in the complex and dynamic world, such as real-time processing, among others (Chai et al., 2022).
While these barriers are valid, they are not insurmountable. Respect for data privacy, safeguarding of intellectual property rights, mutual trust among all stakeholders involved, overcoming cybersecurity breaches, as well as effective decision-making processes and government policies can all facilitate the adoption of digital technologies in the agri-food sector. Cross-learning, multi-stakeholder, and multi-disciplinary approaches are necessary to achieve greater sustainability and resilience in food systems. Recently, the role of collaborative practices between the different actors on the digital food supply chain has been highlighted as a way to solve problems, such as food waste (Annosi et al., 2021). Investments in innovations, especially bundling innovations, is another important success factor that should be prioritized to transform agri-food systems (Serazetdinova et al., 2019; Barrett et al., 2020; Galanakis et al., 2021).
6. Conclusions and future direction
The global challenges for economic, social, and environmental aspects of sustainable development have become more acute over the last years, especially during and in the wake of the COVID-19 pandemic. The shift to digital technologies has accelerated in many fields, including the agri-food sector, to adapt to the negative consequences of this pandemic on various aspects of food production and consumption. Herein, a comprehensive overview of the up-to-date research progress with Industry 4.0 technologies and their significant implications to achieving a digital transformation has been provided. Moreover, the role of the COVID-19 pandemic in accelerating the digital transformation in agriculture and the food industry has been highlighted.
Industry 4.0 innovations, especially IoT, AI, big data, smart sensors, blockchain, robotics, digital twins, and virtual and augmented reality have improved many sectors of agriculture and food. Even in pre-COVID-19 times, the process of adopting these technologies in agriculture and the food industry had begun including concepts, such as precision agriculture/ smart farming, and smart food factories. These technologies have the potential to enhance food quality, safety, and traceability, increase profitability, and reduce food waste and production costs. The severe disruptions caused by the pandemic due to lockdowns and travel restrictions, supply/demand shocks resulted from stockpiling and panic buying, and the stress on food transportation and distribution have accelerated the adoption of digital technologies. Growing research shows that the integration and application of digital technologies can promote precision agriculture and smart factories and contribute to building more resilient and sustainable food supply chains.
Precision agriculture/smart farming and smart food factories are the embodiment of Industry 4.0 in agriculture and the food industry, respectively. Smart farms and smart food factories are characterized by the prevalence of data analytics solutions, sensing technologies, and automated intelligent machines for a complete digitized food production environment. The new possibilities of data collection, processing and evaluation, and data storage, as well as connectivity and information exchange provide new insights and enable much higher precision in applications in automatic and robotic systems. Robots and drones could provide solutions to, among others, the labor shortage caused by the COVID-19 pandemic as well as war and conflicts in many parts of world. Nowadays, robotics is increasingly used to perform many agricultural tasks, automate operations in food processing plants, and replace humans in food service and food delivery. In addition, recent advances in IoT and smart sensors make it possible to ensure food quality and safety, reduce food waste, and track food from farm to fork. Moreover, the food supply chain security can be increased, and traceability and transparency can be enhanced using blockchain and other emerging technologies.
The pandemic is not over, and climate change and war will likely have greater disruptions to the global food supply and exacerbate food insecurity. Therefore, effective harnessing of digital technologies is expected to help overcome these extraordinary challenges. The results of our literature search show that the digital transformation holds significant potential and opens promising opportunities for accelerating the move toward enhanced food sustainability in the agri-food sector. However, compared to other industries, the adoption of digital and other advanced technologies in agriculture and food sectors is still slow. A huge gap can be noticed between Industry 4.0 achievements and practical applications in current agriculture and food industries. This is due to many obstacles (e.g., lack of investments and digital skills, fear of new technologies, and multiple technical and governance issues) that need to be overcome with future research.
The following four concrete recommendations are proposed to achieve an inclusive digital transformation and enhance food security and food sustainability:
1. Transformation of mindsets and development of digital skills are one of the important elements to foster a deeper integration of digital technologies into agriculture and the food industry. For this end, training programs and sharing knowledge and learning should be prioritized.
2. Establishment of adequate digital infrastructure and services, especially in developing countries is crucial to accelerate the digital transformation. This will require more government investment and support, as well as active involvement of political decision-making actors.
3. Interdisciplinarity in large and long-term research efforts as well as private-public partnership are required to release synergies within emerging fields. This interdisciplinary and participatory approach is in line with the core principle of industry 4.0, namely the fusion of various scientific fields and expertise. Therefore, more adoption of digital technologies in agriculture and the food industry will depend first and foremost on the wide cross-border collaboration between all actors in the agri-food system, including researchers with various expertise and backgrounds, food producers and consumers, in addition to regulatory agencies and policymakers.
4. Digital technologies have transformed food production and manufacturing industries into data producers. Effective data governance and cybersecurity strategies should be provided for the captured data to be easily shared and interpreted.
The transition to digital space is expected to continue at a fast pace in the coming few years, especially with the emergence of new technologies and the implementation of innovative strategies to overcome the current challenges. Although the worst of the COVID-19 pandemic seems to be over, there are numerous indications that the pandemic-driven digital transformations are here to stay.
Author contributions
AH: conceptualization, formal analysis, methodology, writing—original draft, and writing—review and editing. HM, YB, FB, JMC, NP, RR, RA, SP, RB, JC, and SM: writing—original draft. JR: review and editing. All authors contributed to the article and approved the submitted version.
Funding
JMC was the beneficiary of a grant (ZA21-028) for the requalification of the Spanish University system from the Ministry of Universities of the Government of Spain, modality Maria Zambrano, financed by the European Union, Next Generation EU, through the project Extraction of bioactive compounds from food matrices using innovative and sustainable technologies (EXTRABIO). NP wishes to thank the post-Ph.D. program of the University of Valencia for the requalification of the Spanish University System from the Ministry of Universities of the Government of Spain, modality Margarita Salas (MS21-045), financed by the European Union, Next Generation EU.
Acknowledgments
Thanks to all the medical staffs and food workers who worked on the front lines during the ongoing COVID-19 pandemic, and the editors and reviewers who evaluated this work.
Conflict of interest
The authors declare that the research was conducted in the absence of any commercial or financial relationships that could be construed as a potential conflict of interest.
Publisher's note
All claims expressed in this article are solely those of the authors and do not necessarily represent those of their affiliated organizations, or those of the publisher, the editors and the reviewers. Any product that may be evaluated in this article, or claim that may be made by its manufacturer, is not guaranteed or endorsed by the publisher.
References
Abbate, S., Centobelli, P., and Cerchione, R. (2023). The digital and sustainable transition of the agri-food sector. Technol. Forecast. Soc. Change 187, 122222. doi: 10.1016/j.techfore.2022.122222
Addanki, M., Patra, P., and Kandra, P. (2022). Recent advances and applications of artificial intelligence and related technologies in the food industry. Appl. Food Res. 2, 100126. doi: 10.1016/j.afres.2022.100126
Alabi, M. O., and Ngwenyama, O. (2022). Food security and disruptions of the global food supply chains during COVID-19: building smarter food supply chains for post COVID-19 era. Br. Food J. 115, 351–367. doi: 10.1108/BFJ-03-2021-0333
Amentae, T. K., and Gebresenbet, G. (2021). Digitalization and future agro-food supply chain management: a literature-based implications. Sustainability 13, 12181. doi: 10.3390/su132112181
Annosi, M. C., Brunetta, F., Bimbo, F., and Kostoula, M. (2021). Digitalization within food supply chains to prevent food waste. drivers, barriers and collaboration practices. Ind. Mark. Manag. 93, 208–220. doi: 10.1016/j.indmarman.2021.01.005
Bader, F., and Rahimifard, S. (2020). A methodology for the selection of industrial robots in food handling. Innov. Food Sci. Emerg. Technol. 64, 102379. doi: 10.1016/j.ifset.2020.102379
Bakalis, S., Valdramidis, V. P., Argyropoulos, D., Ahrne, L., Chen, J., Cullen, P. J., et al. (2020). Perspectives from CO+RE: how COVID-19 changed our food systems and food security paradigms. Curr. Res. Food Sci. 3, 166–172. doi: 10.1016/j.crfs.2020.05.003
Bao, J., and Xie, Q. (2022). Artificial intelligence in animal farming: a systematic literature review. J. Clean. Prod. 331, 129956. doi: 10.1016/j.jclepro.2021.129956
Barbar, C., Bass, P. D., Barbar, R., Bader, J., and Wondercheck, B. (2022). Artificial intelligence-driven automation is how we achieve the next level of efficiency in meat processing. Anim. Front. 12, 56–63. doi: 10.1093/af/vfac017
Barone, A. S., Matheus, J. R. V., Souza, T. S. P., Moreira, R. F. A., and Fai, A. E. C. (2021). Green-based active packaging: opportunities beyond COVID-19, food applications, and perspectives in circular economy—a brief review. Compr. Rev. Food Sci. Food Saf. 20, 4881–4905. doi: 10.1111/1541-4337.12812
Barrett, B. C., Benton, T. G., Cooper, K. A., Fanzo, J., Gandhi, R., Herrero, M., et al. (2020). Bundling innovations to transform agri-food systems. Nat. Sustain. 3, 974–976. doi: 10.1038/s41893-020-00661-8
Barrett, B. C., Fanzo, J., Herrero, M., Mason-D'Croz, D., Mathys, A., Thornton, P., et al. (2021). COVID-19 pandemic lessons for agri-food systems innovation. Environ. Res. Lett. 16, 101001. doi: 10.1088/1748-9326/ac25b9
Bedoya, M. G., Montoya, D. R., Tabilo-Munizaga, G., Pérez-Won, M., and Lemus-Mondaca, R. (2022). Promising perspectives on novel protein food sources combining artificial intelligence and 3D food printing for food industry. Trends Food Sci. Technol. 128, 38–52. doi: 10.1016/j.tifs.2022.05.013
Benyam, A., Soma, T., and Fraser, E. (2021). Digital agricultural technologies for food loss and waste prevention and reduction: global trends, adoption opportunities and barriers. J. Clean. Prod. 323, 129099. doi: 10.1016/j.jclepro.2021.129099
Bhakta, H., Raja, K., Shankar, V. R., and Prakash, V. (2020). Resources, conservation and recycling challenges, opportunities, and innovations for effective solid waste management during and post COVID-19 pandemic. Resour. Conserv. Recycl. 162, 105052. doi: 10.1016/j.resconrec.2020.105052
Bhoi, S. K., Jena, K. K., Panda, S. K., Long, H. V., Kumar, R., Subbulakshmi, P., et al. (2021). An internet of things assisted unmanned aerial vehicle based artificial intelligence model for rice pest detection. Microprocess. Microsyst. 80, 103607. doi: 10.1016/j.micpro.2020.103607
Bigliardi, B., Bottani, E., and Filippelli, S. (2022). A study on IoT application in the food industry using keywords analysis. Procedia Comput. Sci. 200, 1826–1835. doi: 10.1016/j.procs.2022.01.383
Bisoffi, S., Ahrn,é, L., Aschemann-Witzel, J., Báldi, A., Cuhls, K., DeClerck, F., et al. (2021). COVID-19 and sustainable food systems: what should we learn before the next emergency. Front. Sustain. Food Syst. 5, 53. doi: 10.3389/fsufs.2021.650987
Boursianis, D. A., Papadopoulou, M. S., Diamantoulakis, P., Liopa-Tsakalidi, A., Barouchas, P., Salahas, G., et al. (2022). Internet of things (IoT) and agricultural unmanned aerial vehicles (UAVs) in smart farming: a comprehensive review. Internet Things 18, 100187. doi: 10.1016/j.iot.2020.100187
Bouzembrak, Y., Klüche, M., Gavai, A., and Marvin, H. J. P. (2019). Internet of things in food safety: literature review and a bibliometric analysis. Trends Food Sci. Technol. 94, 54–64. doi: 10.1016/j.tifs.2019.11.002
Boyacι-Gündüz, C. P., Ibrahim, S. A., Wei, O. C., and Galanakis, C. M. (2021). Transformation of the food sector: security and resilience during the COVID-19 pandemic. Foods 10, 1–14. doi: 10.3390/foods10030497
Chai, J. J. K., O'Sullivan, C., Gowen, A. A., Rooney, B., and Xu, J.-L. (2022). Augmented/mixed reality technologies for food: a review. Trends Food Sci. Technol. 124, 182–194. doi: 10.1016/j.tifs.2022.04.021
Chandra, M., Kumar, K., Thakur, P., Chattopadhyaya, S., Alam, F., and Kumar, S. (2022). Digital technologies, healthcare and Covid-19: insights from developing and emerging nations. Health Technol. 12, 547–568. doi: 10.1007/s12553-022-00650-1
Chapman, J., Power, A., Netzel, M. E., Sultanbawa, Y., Smyth, H. E., Truong, V. K., et al. (2021). Challenges and opportunities of the fourth revolution: a brief insight into the future of food. Crit. Rev. Food Sci. Nutr. 62, 2845–2853. doi: 10.1080/10408398.2020.1863328
Chauhan, A., Brouwer, B., and Westra, E. (2022). Robotics for a quality-driven post-harvest supply chain. Curr. Robot. Rep. 3, 39–48. doi: 10.1007/s43154-022-00075-8
Chen, B., Wan, J., Shu, L., Li, P., Mukherjee, M., and Yin, B. (2017). Smart factory of industry 4.0: key technologies, application case, and challenges. IEEE Access 6, 6505–6519. doi: 10.1109/ACCESS.2017.2783682
Cheng, J., Van, F., En, P., Ren, H., and Shiong, K. (2022). Integration of internet-of-things as sustainable smart farming technology for the rearing of black soldier fly to mitigate food waste. J. Taiwan Inst. Chem. Eng. 137, 104235. doi: 10.1016/j.jtice.2022.104235
Chitrakar, B., Zhang, M., and Bhandari, B. (2021). Improvement strategies of food supply chain through novel food processing technologies during COVID-19 pandemic. Food Control 125, 108010. doi: 10.1016/j.foodcont.2021.108010
Choe, J. Y., Kim, J. J., and Hwang, J. (2021). Perceived risks from drone food delivery services before and after COVID-19. Int. J. Contemp. Hosp. Manag. 33, 1276–1296. doi: 10.1108/IJCHM-08-2020-0839
D'amore, G., Vaio, A. D., Balsalobre-Lorente, D., and Boccia, F. (2022). Artificial intelligence in the water–energy–food model: a holistic approach towards sustainable development goals. Sustainability 14, 867. doi: 10.3390/su14020867
Dankar, I., Haddarah, A., Omar, E. F. L., Sepulcre, F., and Pujolà, M. (2018). 3D printing technology: the new era for food customization and elaboration. Trends Food Sci. Technol. 75, 231–242. doi: 10.1016/j.tifs.2018.03.018
de Medeiros Esper, M., From, P. J., and Mason, A. (2021). Robotisation and intelligent systems in abattoirs. Trends Food Sci. Technol. 108, 214–222. doi: 10.1016/j.tifs.2020.11.005
de Souza, T. S. P., Miyahira, R. F., Vaz Matheus, J. R., de Brito Nogueira, T. B., Maragoni-Santos, C., Cavalcante Barros, F. F., et al. (2022). Food services in times of uncertainty: remodeling operations, changing trends, and looking into perspectives after the COVID-19 Pandemic. Trends Food Sci. Technol. 120, 301–307. doi: 10.1016/j.tifs.2022.01.005
Demestichas, K., Peppes, N., and Alexakis, T. (2020). Survey on security threats in agricultural IoT and smart farming. Sensors 20, 6458. doi: 10.3390/s20226458
Derossi, A., Bhandari, B., Bommel, K. v, Noort, M., and Severini, C. (2021). Could 3D food printing help to improve the food supply chain resilience against disruptions such as caused by pandemic crises? Int. J. Food Sci. Technol. 56, 4338–55. doi: 10.1111/ijfs.15258
Di Vaio, A. D., Boccia, F., Landriani, L., and Palladino, R. (2020). Artificial intelligence in the agri-food system: rethinking sustainable business models in the COVID-19 scenario. Sustainability 12, 4851. doi: 10.3390/su12124851
Duong, L. N. K., Al-Fadhli, M., Jagtap, S., Bader, F., Martindale, W., Swainson, H., et al. (2020). A review of robotics and autonomous systems in the food industry: from the supply chains perspective. Trends Food Sci. Technol. 106, 355–364. doi: 10.1016/j.tifs.2020.10.028
Dzedzickis, A., Subačiute-Žemaitiene, J., Šutinys, E., Samukaite-Bubniene, U., and Bučinskas, V. (2022). Advanced applications of industrial robotics: new trends and possibilities. Appl. Sci. 12, 135. doi: 10.3390/app12010135
Echegaray, N., Hassoun, A., Jagtap, S., Tetteh-Caesar, M., Kumar, M., Tomasevic, I., et al. (2022). Meat 4.0: principles and applications of industry 4.0 technologies in the meat industry. Appl. Sci. 12, 6986. doi: 10.3390/app12146986
Fuentes, S., Viejo, C. G., Tongson, E., and Dunshea, F. R. (2022). The livestock farming digital transformation: implementation of new and emerging technologies using artificial intelligence. Anim. Health Res. Rev. 23, 59–71. doi: 10.1017/S1466252321000177
Galanakis, C. M., Rizou, M., Aldawoud, T. M. S., Ucak, I., and Rowan, N. J. (2021). Innovations and technology disruptions in the food sector within the COVID-19 pandemic and post-lockdown era. Trends Food Sci. Technol. 110, 193–200. doi: 10.1016/j.tifs.2021.02.002
Gavilan, D., Balderas-Cejudo, A., Fernández-Lores, S., and Martinez-Navarro, G. (2021). Innovation in online food delivery: learnings from COVID-19. Int. J. Gastron. Food. Sci. 24, 100330. doi: 10.1016/j.ijgfs.2021.100330
Ghobakhloo, M. (2020). Industry 4.0, digitization, opportunities for sustainability. J. Clean. Prod. 252, 119869. doi: 10.1016/j.jclepro.2019.119869
Grecuccio, J., Giusto, E., Fiori, F., and Rebaudengo, M. (2020). Combining blockchain and iot: food-chain traceability and beyond. Energies 13, 3820. doi: 10.3390/en13153820
Han, S., Roy, P. K., Hossain, M. I., Byun, K. H., Choi, C., and Ha, S. D. (2021). COVID-19 pandemic crisis and food safety: implications and inactivation strategies. Trends Food Sci. Technol. 109, 25–36. doi: 10.1016/j.tifs.2021.01.004
Hassoun, A., Abdullah, N. A., Aït-kaddour, A., Beşir, A., Zannou, O., and Önal, B. (2022a). Food traceability 4.0 as part of the fourth industrial revolution: key enabling technologies. Crit. Rev. Food Sci. Nutr. 1–17. doi: 10.1080/10408398.2022.2110033
Hassoun, A., Aït-kaddour, A., Abu-mahfouz, A. M., Rathod, N. B., Bader, F., Barba, F. J., et al. (2022b). The fourth industrial revolution in the food industry — part I: industry 4.0 technologies. Crit. Rev. Food Sci. Nutr. 1–17. doi: 10.1080/10408398.2022.2034735
Hassoun, A., Bekhit, A. E.-d., Jambrak, A. R., Regenstein, J. M., Chemat, F., Morton, J. D., et al. (2022c). The fourth industrial revolution in the food industry — part II : emerging food trends trends. Crit. Rev. Food Sci. Nutr. 1−31. doi: 10.1080/10408398.2022.2106472
Hassoun, A., Boukid, F., Pasqualone, A., Bryant, C. J., Jagtap, S., Trollman, H., et al. (2022d). Current research in food science emerging trends in the agri-food sector: digitalisation and shift to plant-based diets. Curr. Res. Food Sci. 5, 2261–2269. doi: 10.1016/j.crfs.2022.11.010
Hassoun, A., Cropotova, J., Trif, M., Rusu, A. V., Bobi, O., Nayik, G. A., et al. (2022e). Consumer acceptance of new food trends resulting from the fourth industrial revolution technologies: a narrative review of literature and future perspectives. Front. Nutr. 9, 972154. doi: 10.3389/fnut.2022.972154
Hassoun, A., Cropotova, J., Trollman, H., Jagtap, S., Garcia-Garcia, G., Parra-López, C., et al. (2023a). Use of Industry 4.0 technologies to reduce and valorize seafood waste and by-products: a narrative review on current knowledge. Curr. Res. Food Sci. 6, 100505. doi: 10.1016/j.crfs.2023.100505
Hassoun, A., Garcia-garcia, G., Trollman, H., Jagtap, S., Cropotova, J., Bhat, Z., et al. (2023b). Birth of Dairy 4.0: opportunities and challenges in adoption of fourth industrial revolution technologies in the production of milk and itsderivatives birth of Dairy 4.0: opportunities and challenges in adoption of fourth industrial revolution technologie. Curr. Res. Food Sci. 7, 100535. doi: 10.1016/j.crfs.2023.100535
Hassoun, A., Gudjónsdóttir, M., Prieto, M. A., Garcia-Oliveira, P., Simal-Gandara, J., Marini, F., et al. (2020). Application of novel techniques for monitoring quality changes in meat and fish products during traditional processing processes: reconciling novelty and tradition. Processes 8, 988. doi: 10.3390/pr8080988
Hassoun, A., Harastani, R., Jagtap, S., Trollman, H., Awad, N. M. H., Zannou, O., et al. (2022f). Truths and myths about superfoods in the era of the COVID-19 pandemic. Crit. Rev. Food Sci. Nutr. 1−18. doi: 10.1080/10408398.2022.2106939
Hassoun, A., Jagtap, S., Garcia-garcia, G., Trollman, H., Pateiro, M., Lorenzo, M., et al. (2023c). Food Quality 4.0: from traditional approaches to digitalized automated analysis. J. Food Eng. 337, 111216. doi: 10.1016/j.jfoodeng.2022.111216
Hassoun, A., Prieto, M. A., Carpena, M., Bouzembrak, Y., Marvin, H. J. P., Pallar, N., et al. (2022g). Exploring the role of green and Industry 4.0 technologies in achieving sustainable development goals in food sectors. Food Res. Int. 162, 112068. doi: 10.1016/j.foodres.2022.112068
Hassoun, A., Siddiqui, S. A., Smaoui, S., Ucak, I., Arshad, R. N., Garcia-Oliveira, P., et al. (2022h). Seafood processing, preservation, and analytical techniques in the age of Industry 4.0. Appl. Sci. 12, 1703. doi: 10.3390/app12031703
Herrero, M., Thornton, P. K., Mason-D'Croz, D., Palmer, J., Bodirsky, B. L., Pradhan, P., et al. (2021). Articulating the effect of food systems innovation on the sustainable development goals. Lancet Planet. Health 5, e50–e62. doi: 10.1016/S2542-5196(20)30277-1
Holzinger, A., Saranti, A., Angerschmid, A., Retzlaff, C. O., Gronauer, A., Pejakovic, V., et al. (2022). Digital transformation in smart farm and forest operations needs human-centered AI: challenges and future directions. Sensors 22, 1–35. doi: 10.3390/s22083043
Idoje, G., Dagiuklas, T., and Iqbal, M. (2021). Survey for smart farming technologies: challenges and issues. Comput. Electr. Eng. 92, 107104. doi: 10.1016/j.compeleceng.2021.107104
Iftekhar, A., and Cui, X. (2021). Blockchain-based traceability system that ensures food safety measures to protect consumer safety and COVID-19 free supply chains. Foods 10, 1289. doi: 10.3390/foods10061289
Jagtap, S., Bhatt, C., Thik, J., and Rahimifard, S. (2019). Monitoring potato waste in food manufacturing using image processing and internet of things approach. Sustainability 11, 3173. doi: 10.3390/su11113173
Jakoet-Salie, A., and Ramalobe, K. (2022). The digitalization of learning and teaching practices in higher education institutions during the Covid-19 pandemic. Teach. Public Adm. 41, 014473942210922. doi: 10.1177/01447394221092275
Javaid, M., Haleem, A., Singh, R. P., and Suman, R. (2022). Enhancing smart farming through the applications of Agriculture 4.0 technologies. Int. J. Intell. Netw. 3, 150–164. doi: 10.1016/j.ijin.2022.09.004
Ji, B., Banhazi, T., Phillips, C. J. C., and Wang, C. (2022). A machine learning framework to predict the next month's daily milk yield, milk composition and milking frequency for cows in a robotic dairy farm. Biosyst. Eng. 216, 186–197. doi: 10.1016/j.biosystemseng.2022.02.013
Jia, S. S., Gibson, A. A., Ding, D., Allman-Farinelli, M., Phongsavan, P., Redfern, J., et al. (2022). Perspective: are online food delivery services emerging as another obstacle to achieving the 2030 united nations sustainable development goals? Front. Nutr. 9, 858475. doi: 10.3389/fnut.2022.858475
Jin, C., Bouzembrak, Y., Zhou, J., Liang, Q., van den Bulk, L. M., Gavai, A., et al. (2020). Big data in food safety- a review. Curr. Opin. Food Sci. 36, 24–32. doi: 10.1016/j.cofs.2020.11.006
John, K. T. (2021). Digital disruption: the hyperlocal delivery and cloud kitchen driven future of food services in post-COVID India. Int. Hosp. Rev. 37, 161–187. doi: 10.1108/IHR-06-2021-0045
Kamble, S. S., Gunasekaran, A., and Gawankar, S. A. (2020). Achieving sustainable performance in a data-driven agriculture supply chain: a review for research and applications. Int. J. Prod. Econ. 219, 179–194. doi: 10.1016/j.ijpe.2019.05.022
Kamble, S. S., Gunasekaran, A., Parekh, H., Mani, V., Belhadi, A., and Sharma, R. (2022). Digital twin for sustainable manufacturing supply chains: current trends, future perspectives, and an implementation framework. Technol. Forecast. Soc. Change 176, 121448. doi: 10.1016/j.techfore.2021.121448
Kamilaris, A., Fonts, A., and Prenafeta-Boldú, F. X. (2019). The rise of blockchain technology in agriculture and food supply chains. Trend. Food Sci. Technol. 91, 640–652. doi: 10.1016/j.tifs.2019.07.034
Kayikci, Y., Subramanian, N., Dora, M., and Bhatia, M. S. (2022). Food supply chain in the era of Industry 4.0: blockchain technology implementation opportunities and impediments from the perspective of people, process, performance, and technology. Prod. Plan. Control 33, 301–321. doi: 10.1080/09537287.2020.1810757
Khan, H. H., Malik, M. N., Konečná, Z., Chofreh, A. G., Goni, F. A., and Klemeš, J. J. (2022). Blockchain technology for agricultural supply chains during the COVID-19 pandemic: benefits and cleaner solutions. J. Clean. Prod. 347, 131268. doi: 10.1016/j.jclepro.2022.131268
Khan, M. A., Hossain, E., Shahaab, A., and Khan, I. (2022). ShrimpChain: a blockchain-based transparent and traceable framework to enhance the export potentiality of Bangladeshi Shrimp. Smart Agric. Technol. 2, 100041. doi: 10.1016/j.atech.2022.100041
Knorr, D., and Augustin, M. A. (2021). From value chains to food webs: the quest for lasting food systems. Trends Food Sci. Technol. 110, 812–821. doi: 10.1016/j.tifs.2021.02.037
Kudashkina, K., Corradini, M. G., Thirunathan, P., Yada, R. Y., and Fraser, E. D. G. (2022). Artificial intelligence technology in food safety: a behavioral approach. Trends Food Sci. Technol. 123, 376–381. doi: 10.1016/j.tifs.2022.03.021
Kumar, I., Rawat, J., Mohd, N., and Husain, S. (2021). Opportunities of artificial intelligence and machine learning in the food industry. J. Food Qual. 2021, 4535567. doi: 10.1155/2021/4535567
Lee, J. (2021). A 3D food printing process for the new normal era: a review. Processes 9, 1495. doi: 10.3390/pr9091495
Lezoche, M., Panetto, H., Kacprzyk, J., Hernandez, J. E., and Eva Alemany Díaz, M. M. (2020). Agri-Food 4.0: a survey of the supply chains and technologies for the future agriculture. Comput. Ind. 117, 103187. doi: 10.1016/j.compind.2020.103187
Logan, M., Safi, M., Lens, P., and Visvanathan, C. (2019). Investigating the performance of internet of things based anaerobic digestion of food waste. Process Saf. Environ. Prot. 127, 277–287. doi: 10.1016/j.psep.2019.05.025
Lopes de Sousa Jabbour, L., A. B, de Oliveira Frascareli, F. C., Santibanez Gonzalez, E. D. R., and Chiappetta Jabbour, C. J. (2021). Are food supply chains taking advantage of the circular economy? A research agenda on tackling food waste based on Industry 4.0 technologies. Prod. Plan. Control 34, 967–983. doi: 10.1080/09537287.2021.1980903
Madureira, J., Margaça, F. M. A., Santos-Buelga, C., Ferreira, I. C. F. R., Verde, S. C., and Barros, L. (2022). Applications of bioactive compounds extracted from olive industry wastes: a review. Compr. Rev. Food Sci. Food Saf. 21, 453–476. doi: 10.1111/1541-4337.12861
Manning, L., Brewer, S., Craigon, P. J., Frey, J., Gutierrez, A., Jacobs, N., et al. (2022). Artificial intelligence and ethics within the food sector: developing a common language for technology adoption across the supply chain. Trends Food Sci. Technol. 125, 33–42. doi: 10.1016/j.tifs.2022.04.025
Marvin, H. J. P., and Bouzembrak, Y. (2020). A system approach towards prediction of food safety hazards: impact of climate and agrichemical use on the occurrence of food safety hazards. Agric. Syst. 178, 102760. doi: 10.1016/j.agsy.2019.102760
Marvin, H. J. P., Bouzembrak, Y., van der Fels-Klerx, H. J., Kempenaar, C., Veerkamp, R., Chauhan, A., et al. (2022). Digitalisation and artificial intelligence for sustainable food systems. Trends Food Sci. Technol. 120, 344–348. doi: 10.1016/j.tifs.2022.01.020
Marvin, H. J. P., van Asselt, E., Kleter, G., Meijer, N., Lorentzen, G., Johansen, L. H., et al. (2020). Expert-driven methodology to assess and predict the effects of drivers of change on vulnerabilities in a food supply chain: aquaculture of atlantic salmon in norway as a showcase. Trends Food Sci. Technol. 103, 49–56. doi: 10.1016/j.tifs.2020.06.022
Masi, A., Ciccullo, F., and Pero, M. (2021). “Digitalizing agri-food supply chains to achieve sustainable development goals: a systematic literature review,” in 2021 IEEE International Conference on Engineering, Technology and Innovation, ICE/ITMC 2021 – Proceedings (Cardiff: IEEE), 1–8. doi: 10.1109/ICE/ITMC52061.2021.9570237
McVey, C., Elliott, C. T., Cannavan, A., Kelly, S. D., Petchkongkaew, A., and Haughey, S. A. (2021). Portable spectroscopy for high throughput food authenticity screening: advancements in technology and integration into digital traceability systems. Trends Food Sci. Technol. 118, 777–790. doi: 10.1016/j.tifs.2021.11.003
Misra, N. N., Dixit, Y., Al-Mallahi, A., Bhullar, M. S., Upadhyay, R., and Martynenko, A. (2022). IoT, big data and artificial intelligence in agriculture and food industry. IEEE Internet Things J. 9, 1–1. doi: 10.1109/JIOT.2020.29985
Musa, S. F. P. D., and Basir, K. H. (2021). Smart farming: towards a sustainable agri-food system. Br. Food J. 123, 3085–3099. doi: 10.1108/BFJ-03-2021-0325
Nasirahmadi, A., Sturm, B., Edwards, S., Jeppsson, K.-H., Olsson, A.-C., Müller, S., et al. (2019). Deep learning and machine vision approaches for posture detection of individual pigs. Sensors 19, 3738. doi: 10.3390/s19173738
Navas, E., Fernández, R., Sepúlveda, D., Armada, M., and Gonzalez-de-santos, P. (2021). Soft grippers for automatic crop harvesting: a review. Sensors 21, 2689. doi: 10.3390/s21082689
Oliveira, L. F. P., Moreira, A. P., and Silva, M. F. (2021). Advances in agriculture robotics: A state-of-the-art review and challenges ahead. Robotics 10, 52. doi: 10.3390/robotics10020052
Oruma, S. O., Misra, S., and Fernandez-Sanz, L. (2021). Agriculture 4.0: an implementation framework for food security attainment in nigeria's post-COVID-19 Era. IEEE Access 9, 83592–83627. doi: 10.1109/ACCESS.2021.3086453
Ouafiq, E. M., Saadane, R., and Chehri, A. (2022). Data management and integration of low power consumption embedded devices IoT for transforming smart agriculture into actionable knowledge. Agriculture 12, 329. doi: 10.3390/agriculture12030329
Partridge, S. R., Gibson, A. A., Roy, R., Malloy, J. A., Raeside, R., Jia, S. S., et al. (2020). Junk food on demand: a cross-sectional analysis of the nutritional quality of popular online food delivery outlets in Australia and New Zealand. Nutrients 12, 3107. doi: 10.3390/nu12103107
Pascuta, M. S., and Vodnar, D. C. (2022). Nanocarriers for sustainable active packaging: an overview during and post COVID-19. Coatings 12, 102. doi: 10.3390/coatings12010102
Pathan, M., Patel, N., Yagnik, H., and Shah, M. (2020). Artificial cognition for applications in smart agriculture: a comprehensive review. Artif. Intell. Agric. 4, 81–95. doi: 10.1016/j.aiia.2020.06.001
Paudel, K. P., Mishra, A. K., Pandit, M., Larkin, S., Rejesus, R., and Velandia, M. (2020). Modeling multiple reasons for adopting precision technologies: evidence from U.S. cotton producers. Comput. Electron. Agric. 175, 105625. doi: 10.1016/j.compag.2020.105625
Pereira, C. S., Veloso, B., Durão, N., and Moreira, F. (2022). The influence of technological innovations on international business strategy before and during COVID-19 pandemic. Procedia Comput. Sci. 196, 44–51. doi: 10.1016/j.procs.2021.11.071
Qian, J., Yu, Q., Jiang, L., Yang, H., and Wu, W. (2022). Food cold chain management improvement: a conjoint analysis on COVID-19 and food cold chain systems. Food Control 137, 108940. doi: 10.1016/j.foodcont.2022.108940
Racewicz, P., Ludwiczak, A., Skrzypczak, E., Składanowska-Baryza, J., Biesiada, H., Nowak, T., et al. (2021). Welfare health and productivity in commercial pig herds. Animals 11, 1176. doi: 10.3390/ani11041176
Rahaman, A., Kumari, A., Zeng, X. A., Khalifa, I., Farooq, M. A., Singh, N., et al. (2021). The increasing hunger concern and current need in the development of sustainable food security in the developing countries. Trends Food Sci. Technol. 113, 423–429. doi: 10.1016/j.tifs.2021.04.048
Ramirez-Asis, E., Vilchez-Carcamo, J., Thakar, C. M., Phasinam, K., Kassanuk, T., and Naved, M. (2022). A review on role of artificial intelligence in food processing and manufacturing industry. Mater. Today Proc. 51, 2462–2465. doi: 10.1016/j.matpr.2021.11.616
Rejeb, A., Abdollahi, A., Rejeb, K., and Treiblmaier, H. (2022a). Drones in agriculture: a review and bibliometric analysis. Comput. Electr. Agric. 198, 107017. doi: 10.1016/j.compag.2022.107017
Rejeb, A., Keogh, J. G., and Rejeb, K. (2022b). Big data in the food supply chain: a literature review. J. Data Inf. Manag. 4, 33–47. doi: 10.1007/s42488-021-00064-0
Rejeb, A., Rejeb, K., Abdollahi, A., Al-Turjman, F., and Treiblmaier, H. (2022c). The interplay between the internet of things and agriculture: a bibliometric analysis and research agenda. Internet Things 19, 100580. doi: 10.1016/j.iot.2022.100580
Rejeb, A., Rejeb, K., and Keogh, J. G. (2021). Enablers of augmented reality in the food supply chain: a systematic literature review. J. Foodserv. Bus. Res. 24, 415–444. doi: 10.1080/15378020.2020.1859973
Ren, Q. S., Fang, K., Yang, X. T., and Han, J. W. (2022). Ensuring the quality of meat in cold chain logistics: a comprehensive review. Trends Food Sci. Technol. 119, 133–151. doi: 10.1016/j.tifs.2021.12.006
Romanov, D., Korostynska, O., Lekang, O. I., and Mason, A. (2022). Towards human-robot collaboration in meat processing: challenges and possibilities. J. Food Eng. 331, 111117. doi: 10.1016/j.jfoodeng.2022.111117
Ross, S., Korostynska, O., Cordova-Lopez, L. E., and Mason, A. (2022). A review of unilateral grippers for meat industry automation. Trends Food Sci. Technol. 119, 309–319. doi: 10.1016/j.tifs.2021.12.017
Sadeghi, K., Kim, J., and Seo, J. (2022). Packaging 4.0: the threshold of an intelligent approach. Compr. Rev. Food Sci. Food Saf. 21, 2615–2638. doi: 10.1111/1541-4337.12932
Saiz-Rubio, V., and Rovira-Más, F. (2020). From smart farming towards agriculture 5.0: A review on crop data management. Agronomy 10, 207. doi: 10.3390/agronomy10020207
Saryatmo, M. A., and Sukhotu, V. (2021). The influence of the digital supply chain on operational performance: a study of the food and beverage industry in Indonesia. Sustainability 13, 5109. doi: 10.3390/su13095109
Sengupta, T., Narayanamurthy, G., Moser, R., Pereira, V., and Bhattacharjee, D. (2021). Disruptive technologies for achieving supply chain resilience in COVID-19 Era: an implementation case study of satellite imagery and blockchain technologies in fish supply chain. Inf. Syst. Front. 24, 1107–1123. doi: 10.1007/s10796-021-10228-3
Sepúlveda, D., Fernández, R., Navas, E., Armada, M., and González-de-santos, P. (2020). Robotic aubergine harvesting using dual-arm manipulation. IEEE Access 8, 121889–121904. doi: 10.1109/ACCESS.2020.3006919
Serazetdinova, L., Garratt, J., Baylis, A., Stergiadis, S., Collison, M., and Davis, S. (2019). How should we turn data into decisions in agrifood? J. Sci. Food Agric. 99, 3213–3219. doi: 10.1002/jsfa.9545
Sharma, A., Jain, A., Gupta, P., and Chowdary, V. (2021). Machine learning applications for precision agriculture: a comprehensive review. IEEE Access 9, 4843–4873. doi: 10.1109/ACCESS.2020.3048415
Sharma, M., Joshi, S., Luthra, S., and Kumar, A. (2021). Managing disruptions and risks amidst COVID-19 outbreaks: role of blockchain technology in developing resilient food supply chains. Oper. Manag. Res. 15, 268–281. doi: 10.1007/s12063-021-00198-9
Shi, Z., Xie, Y., Xue, W., Chen, Y., Fu, L., and Xu, X. (2020). Smart factory in Industry 4.0. Syst. Res. Behav. Sci. 37, 607–617. doi: 10.1002/sres.2704
Sinha, B. B., and Dhanalakshmi, R. (2022). Recent advancements and challenges of internet of things in smart agriculture: a survey. Future Gener. Comput. Syst. 126, 169–184. doi: 10.1016/j.future.2021.08.006
Siregar, R. R. A., Seminar, K. B., Wahjuni, S., and Santosa, E. (2022). Vertical farming perspectives in support of precision agriculture using artificial intelligence: a review. Computers 11, 135. doi: 10.3390/computers11090135
Srivastava, A., Das, D. K., and Kumar, R. (2020). “Monitoring of soil parameters and controlling of soil moisture through iot based smart agriculture,” in 2020 IEEE Students' Conference on Engineering and Systems, SCES (Prayagraj: IEEE), 1–6. doi: 10.1109/SCES50439.2020.9236764
Sulaiman, N. B., Mahmud, N. P. N. B., Nazir, U., Latib, S. K. K. B. A., Hafidz, H. F. B. M., and Kamran Abid, S. (2021). The role of autonomous robots in fourth industrial revolution (4IR) as an approach of sustainable development goals (SDG9): industry, innovation and infrastructure in handling the effect of COVID-19 outbreak. IOP Conf. Ser. Earth Environ. Sci. 775, 012017. doi: 10.1088/1755-1315/775/1/012017
Taneja, A., Nair, G., Joshi, M., Sharma, S., Sharma, S., Jambraj, A. R., et al. (2023). Artificial intelligence: Implications for the agri-food sector. Agronomy. 13, 1397. doi: 10.3390/agronomy13051397
Thirumdas, R., Kothakota, A., Pandiselvam, R., Bahrami, A., and Barba, F. J. (2021). Role of food nutrients and supplementation in fighting against viral infections and boosting immunity: a review. Trends Food Sci. Technol. 110, 66–77. doi: 10.1016/j.tifs.2021.01.069
Tomar, P., and Kaur, G. (2021). Artificial Intelligence and IoT-Based Technologies for Sustainable Farming and Smart Agriculture. (Hershey, PA: IGI Global). doi: 10.4018/978-1-7998-1722-2
Ullo, S. L., and Sinha, G. R. (2021). Advances in IoT and smart sensors for remote sensing and agriculture applications. Remot. Sens. 13, 2585. doi: 10.3390/rs13132585
Varavallo, G., Caragnano, G., Bertone, F., Vernetti-Prot, L., and Terzo, O. (2022). Traceability platform based on green blockchain: an application case study in dairy supply chain. Sustainability 14, 3321. doi: 10.3390/su14063321
Verboven, P., Defraeye, T., Datta, A. K., and Nicolai, B. (2020). Digital twins of food process operations: the next step for food process models? Curr. Opin. Food Sci. 35, 79–87. doi: 10.1016/j.cofs.2020.03.002
Wang, C., Korai, A., Jia, S. S., Allman-Farinelli, M., Chan, V., Raeside, R., et al. (2021). Hunger for home delivery: cross-sectional analysis of the nutritional quality of complete menus on an online food delivery platform in Australia. Nutrients 13, 1–14. doi: 10.3390/nu13030905
Wang, L., He, Y., and Wu, Z. (2022). Design of a blockchain-enabled traceability system framework for food supply chains. Foods 11, 744. doi: 10.3390/foods11050744
Wang, X. V., and Wang, L. (2021). A literature survey of the robotic technologies during the COVID-19 pandemic. J. Manuf. Syst. 60, 823–836. doi: 10.1016/j.jmsy.2021.02.005
Wang, Z., Hirai, S., and Kawamura, S. (2022). Challenges and opportunities in robotic food handling: a review. Front. Robot. AI 8, 433. doi: 10.3389/frobt.2021.789107
Watson, N. J., Bowler, A. L., Rady, A., Fisher, O. J., Simeone, A., Escrig, J., et al. (2021). Intelligent sensors for sustainable food and drink manufacturing. Front. Sustain. Food Syst. 5, 408. doi: 10.3389/fsufs.2021.642786
Xu, J., Gu, B., and Tian, G. (2022). Review of agricultural iot technology. Artif. Intell. Agric. 6, 10–22. doi: 10.1016/j.aiia.2022.01.001
Yang, X., Li, M., Yu, H., Wang, M., Xu, D., and Sun, C. (2021). A trusted blockchain-based traceability system for fruit and vegetable agricultural products. IEEE Access 9, 36282–36293. doi: 10.1109/ACCESS.2021.3062845
Yoo, S. R., Kim, S. H., and Jeon, H. M. (2022). How does experiential value toward robot barista service affect emotions, storytelling, and behavioral intention in the context of COVID-19? Sustainability 14, 450. doi: 10.3390/su14010450
Zanetta, L. D. A, Hakim, M. P., Gastaldi, G. B., Alverne Jucá Seabra, L. M., Rolim, P. M., Nascimento, L. G. P., et al. (2021). The use of food delivery apps during the COVID-19 pandemic in Brazil: the role of solidarity, perceived risk, and regional aspects. Food Res. Int. 149, 110671. doi: 10.1016/j.foodres.2021.110671
Zhang, Y., Chen, L., Battino, M., Farag, M. A., Xiao, J., Simal-Gandara, J., et al. (2022). Blockchain: an emerging novel technology to upgrade the current fresh fruit supply chain. Trends Food Sci. Technol. 124, 1–12. doi: 10.1016/j.tifs.2022.03.030
Keywords: artificial intelligence, automation, digitalization, Industry 4.0, robots, smart factory, precision agriculture
Citation: Hassoun A, Marvin HJP, Bouzembrak Y, Barba FJ, Castagnini JM, Pallarés N, Rabail R, Aadil RM, Bangar SP, Bhat R, Cropotova J, Maqsood S and Regenstein JM (2023) Digital transformation in the agri-food industry: recent applications and the role of the COVID-19 pandemic. Front. Sustain. Food Syst. 7:1217813. doi: 10.3389/fsufs.2023.1217813
Received: 05 May 2023; Accepted: 03 July 2023;
Published: 18 July 2023.
Edited by:
Flavio Boccia, University of Naples Parthenope, ItalyReviewed by:
Nikheel Rathod, Dr. Balasaheb Sawant Konkan Krishi Vidyapeeth, IndiaCengiz Gokbulut, Balikesir University, Türkiye
Ferdaous Boughattas, UniLaSalle, France
Letizia Alvino, University of Twente, Netherlands
Copyright © 2023 Hassoun, Marvin, Bouzembrak, Barba, Castagnini, Pallarés, Rabail, Aadil, Bangar, Bhat, Cropotova, Maqsood and Regenstein. This is an open-access article distributed under the terms of the Creative Commons Attribution License (CC BY). The use, distribution or reproduction in other forums is permitted, provided the original author(s) and the copyright owner(s) are credited and that the original publication in this journal is cited, in accordance with accepted academic practice. No use, distribution or reproduction is permitted which does not comply with these terms.
*Correspondence: Abdo Hassoun, YS5oYXNzb3VuQHNhZi1pci5jb20=; Janna Cropotova, amFubmEuY3JvcG90b3ZhQG50bnUubm8=