- 1Charles H. Dyson School of Applied Economics and Management, Cornell University, Ithaca, NY, United States
- 2Senegal Institute of Agricultural Research, Dakar, Senegal
- 3Cultural Practice LLC, Bethesda, MD, United States
- 4School of Integrative Plant Science, Cornell University, Ithaca, NY, United States
Crop trait and varietal preferences are socially shaped, varying by gender, experience, and on-farm roles. This drives preference heterogeneity, between households but also within households. Adhering to the common practice of only interviewing the household head as a representative of households, leads to breeding programs collecting trait preferences that do not represent the experiences of other members within that household. This dearth of data on trait preferences of multiple household members could be hindered by the lack of robust and agile methods to collect this data. Here we present a method that explores intra-household differences between husbands and wives in trait preferences through choice experimentation, coupled with questions that capture decision-making, experience and time spent on farm to explore how these drive preferences. Dissecting crop management into three dimensions, we explore what drives intra-household heterogeneity in varietal preferences between husbands and wives, as well as, decision-making, crop experience and time spent working on the crop. We present preliminary results from testing this combined protocol with 270 cowpea growing households (540 respondents) in Senegal. The findings from this work hold promise to inform crop breeding programs on the value of intra-household analysis for trait priority setting, while offering a new method which is applicable by National Agricultural Research Organizations globally.
1 Introduction
Breeding programs are becoming more demand-led, yet farmers do not always accept the improved crops developed (see among others, Sheahan and Barrett, 2017; Macours, 2019; Michler et al., 2019; Takahashi et al., 2020). One of the reasons this adoption gap may occur, is that varietal traits tend to be valued differently by different household members (Tufan et al., 2018; Marimo et al., 2020; Maligalig et al., 2021; McEwan et al., 2021; Krishna and Veettil, 2022). This heterogeneity in intra-household preferences is poorly captured by existing crop trait and varietal priority setting practices, with few priority setting studies focusing on intra-household crop and trait choices (Marimo et al., 2020; Occelli et al., 2023).
Members within a household diverge in crop trait preferences when they face different constraints and have varied responsibilities and production goals (Doss, 2001; Teeken et al., 2018; Weltzien et al., 2019). Furthermore, crop management roles at different stages within the crop life cycle are recognized to affect users' acceptability of breeding products (Laborte et al., 2015; Ashby and Polar, 2019). Yet, for setting trait priorities, crop breeding programs frequently collect only the preferences of the household head (or one member of the family) which are implicitly assumed to reflect those of the entire household (Asrat et al., 2010; Pant et al., 2012; Mengistu et al., 2019). The rationale behind this trend is the assumption of the household as a single decision-making unit where there is a shared utility function among family members (Becker, 1965) despite the ample empirical evidence that rejects the unitary household model (Hoddinott and Haddad, 1995; Udry, 1996; Attanasio and Lechene, 2002; Duflo and Udry, 2004). Interviewing only one person, usually the eldest man or the head of household in the family, misses important information about other actors in the household, resulting in only a partial understanding of the adoption process (Joshi et al., 2019). This is true even if women heads of households are interviewed, as their experiences seldom represent the experiences of women who live in male headed households (Doss and Kieran, 2013; Carletto, 2021). As a result, there is a lack of literature on the influence of intra-household preferences on crop decision-making and technology adoption (Gulati, 2016; Maligalig et al., 2017).
As the recognition of the gendered division of labor and knowledge in varietal selection continues to grow (Lope-Alzina, 2007; Teeken et al., 2018; McDougall et al., 2022; Smale et al., 2022), attention should shift toward understanding the influence of intra-household dynamics on trait choices. If household members possess different preferences for crop attributes, then this should be considered by crop breeders when setting breeding objectives. By doing so, breeders can practice more gender-equitable trait and varietal development processes.
The scarcity of intra-household trait preference studies might have roots in the orthodoxy around the unitary household model, but we posit that it is also the lack of standardized data collection methods that exacerbate this persistence. Looking back at 30 years of socio-economic data collection, Doss (2021) describes how feminist economics has been at the forefront of intra-household analysis in socio-economic studies. Many examples testify that intra-household data are increasingly made available: among others, women's empowerment is measured intra-household with the Women's Empowerment in Agriculture Index (WEAI) (Alkire et al., 2013) and the World Bank Living Standard Measurement Surveys – Integrated Surveys on Agriculture (LSMS-ISA) collects plot-level data on who manages the plot. Asset ownership is another topic where intra-household data collection has been impactful (among others, see Deere and Doss, 2006; Doss et al., 2014, 2018). Studies investigating intra-household decision-making are also more numerous: among others, worth citing here Bernard et al. (2019) which uses vignette to describe how within households reasons of production and consumption are made. There is however a dearth of data on intra-household data on trait and varietal preferences from different household's members.
To close the data gap between intra-household analysis and trait preferences, exploration of methods which build on widely known data collection tools, while leveraging best practices from intra-household research are needed. In this study, we propose an intra-household discrete choice experiment method for capturing trait preferences, joining a brief survey module on intra-household decision-making, time use and years of experience in a survey administered to both husbands and wives1, combined with a standard discrete choice experiment protocol. Dissecting crop management into three dimensions, we explore what drives intra-household heterogeneity in varietal preferences between conjugal couples.
Discrete choice experiments have proven successful in quantifying farmers' preferences and produce results which are tangible for breeders and plant scientists (Anugwa et al., 2022; Miriti et al., 2022). Using a discrete choice experiment framework, bundles of attributes are evaluated. This allows an assessment of how individual choices change when one or more of the attributes varies. Whenever prices are included in a discrete choice experiment, individual choices can be expressed in terms of willingness-to-pay for one attribute rather than another. A large amount of literature has documented agricultural growers' trait preferences using choice experiments, with a particular emphasis on sub-Saharan African countries (among others, see Labarta, 2009; Waldman et al., 2017; Kimathi et al., 2022). Discrete choice experiments present non-negligible drawbacks as method, among others the susceptibility to hypothetical biases and the limitation in the number of traits that can define a crop, without risking decision fatigue with an overwhelming number of choice sets or traits per profile (Burns et al., 2022). However, the use of choice experiments does have two main benefits. First, it does not require longitudinal market data, which are hard to collect and rarely useful in disentangling the effect of each trait on farmers' choices due to correlations between traits (Miriti et al., 2022). Second, choice experiment analyses offer a means through which the nuances of decision-making can be understood by providing insights into implicit trade-offs between different traits (Khanal et al., 2017).
The quantification of trade-offs make discrete choice experiments particularly informative for studying intra-household heterogeneity in trait preferences, but the combination of choice experiments and intra-household methods have not been explored. On one hand, sex-disaggregated data on trait preferences have been collected through choice experiments. For example, Marenya et al. (2021) show that men and women farmers in Kenya have similar preferences for maize traits but showcase different trade-offs between traits. Martey et al. (2022) observe wide dispersion of willingness-to-pay among women cowpea farmers relative to men cowpea farmers in Northern Ghana, with participation in cowpea training contributing to reduce the dispersion of WTP for both men and women (for other examples, see Asrat et al., 2010; Fisher and Carr, 2015; Kassie et al., 2017). However, few studies use choice experiments with an intra-household approach and even fewer do so for crop trait preferences. Intra-household choice experiment methods have been used mainly to explore the acceptance of new agricultural technologies: see Gulati (2016) on rice transplanting technology and Maligalig (2018) on rice improved varieties. Joshi et al. (2019) combine measures of willingness-to-pay with results from the Women's Empowerment in Agriculture Index (WEAI), finding that the respective gender roles of women and men in the family and on the farm are aligned with their preferences for the labor-saving direct-seeded rice technology. Krishna and Veettil (2022) evaluated wheat preferences in 420 households in the central Indian state of Madhya Pradesh. Most women respondents were not actively involved in making decisions related to wheat cultivation, including varietal selection. However, the results indicate that women farmers were open to experimentation with new varieties, a conclusion derived from their positive willingness to pay for improved varietal traits.
Building especially on the work of Joshi et al. (2019) and Krishna and Veettil (2022), we construct an intra-household discrete choice experiment protocol to explore trade-offs and preferences among crop attributes, in relation to decision-making, experience and time spent on farm for conjugal couples. In this paper, we present a description of our method along with preliminary results from a pilot with cowpea growers in Senegal. The choice of cowpea producers in Senegal to test the method itself is a contribution to the literature, due to the scarcity of choice experiments with farmers in the region. The paper is structured as follows: Section 2 describes the method in detail, Section 3 presents preliminary results, and Section 4 discusses findings considering the protocol testing.
2 Description of the intra-household discrete choice experiment method
The method has been developed by a team of agricultural economists, breeders and gender specialists affiliated with the Feed the Future Innovation Lab for Crop Improvement, based at Cornell University (US), the Bureau of Macroeconomic Analysis at the Senegalese Institute of Agricultural Research (Senegal) and Cultural Practice, LLC (US). The method comprises a combination of two tools: a brief survey and a streamlined discrete choice experiment (see Appendix I for full tools). We summarize the steps and process in Figure 1, with each step being administered to both spouses within each household producing the crop of interest. For good practice in collecting data from men and women, both tools should be administered separate to the respondents, and where possible by enumerators who identify as the same gender category as the respondent. The method is crop and region – agnostic, but it should be adapted to the crop and region of interest whenever implemented.
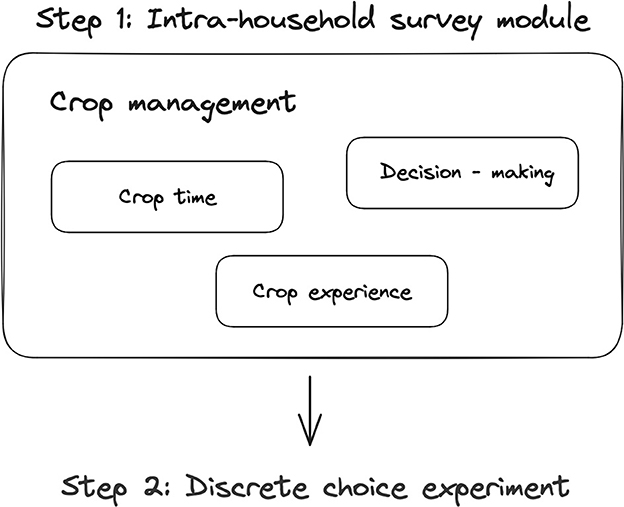
Figure 1. The intra-household discrete choice method. Both steps 1 and 2 are administered to spousal couples, or male and female adult decision makers as appropriate.
2.1 The crop management module
The survey builds on the premise that decision-making dynamics, experience with the crop, and time spent on producing and processing the crop of interest may shape trait preferences within the households. To collect enough information to test this hypothesis, the survey is composed of three modules.
The decision-making module (Section B in Appendix I) investigates who within the household make decisions on the crop of interest, at the pre-harvest, harvest and post-harvest stage. Both household members are asked who within the household makes most of the decisions on crop varieties to be planted, inputs usage, timing of cropping activities, land allocation, intercropping (if practiced) and what to do with the harvested crop. Household members can reply that they perform the activity alone, jointly with their spouses, that their spouses alone are decision-makers, or that other members of the household are making most of the decisions on a specific activity. This module maps decision-making in well-established indices such as the WEAI (Alkire et al., 2013) and it mimics tools used in the literature on intra-household bargaining outcomes and technology preferences (Chiappori et al., 2002; see, among others, Akresh, 2005; Anderson et al., 2017). We are aware of the shortcomings of directly elicited decision-making questions, and the more recent best practice around vignettes as a more effective method (Bernard et al., 2019), however for practicability we have chosen to use the current approach.
Decision-making is not the only dimension which determines intra-household trait preferences, with accumulating evidence that experience (often proxied with age and gender) influences crop management decisions within the household (Deressa et al., 2009; Amare et al., 2018). Analogously, time spent working on the plot where the crop of interest is cultivated is also important. Udry (1996) identifies time as a key element to identify the relation between gender and the agricultural production. Pierotti et al. (2022) show how time poverty limits women's role and preferences in farming. In line with these studies, we enrich our survey with a module on crop experience (Section C in Appendix I) and a module on time spent on the plot (Section D in Appendix I), where respondents are asked to list the experience (years engaged in the production of the crop) and the time spent on activities related to the crop production of each household member respectively. These three modules are accompanied by a brief introductory section (Section A in Appendix I), where interviewed household members are asked basic sociodemographic (age, gender, education level, farmers' group membership) and agronomic questions (land under cultivation of the crop of interest and income from the crop). Furthermore, each respondent interviewed is asked to compile a roster of household members, declaring whether they are involved in the production of the crop of interest.
In its entirety, the survey sheds light on the complexity behind crop roles. By eliciting respondents' own and others' perceptions on decision-making, experience and time, the survey highlights not only which household member scores higher in each module, but also the degree of intra-household agreement on crop roles. Furthermore, the interaction between decision-making, crop experience and time, cemented by gender norms, is informative of trait preference heterogeneity within the household.
2.2 The discrete choice experiment
The discrete choice experiment uses pictorial choice sets and asks respondents to choose their preferred choice among a set of predefined options. This part of the method follows closely the established literature on discrete choice experiments (McFadden, 1973, 2001) and it contributes to elicit trade-offs among diverse bundles of traits as the choices proposed to respondents represent different combinations of traits for the crop of interest. Following Kolstad (2011), Ryan et al. (2012), and Wasserman-Olin (2020), we use the discrete choice experiment part of our method also to examine the impact of variations in cowpea attributes on the likelihood of an individual adopting a particular cowpea variety. An example of a choice experiment card is reported in Section E, Appendix I. Since the choice experiment is not gamified, cheap talk and repeated opt-out reminders were given to respondents to mitigate respondents' bias (Tonsor and Shupp, 2011).
To select the traits included in the cards, along with the associated levels, the method proposes a two-step approach. First, a literature review establishes a potential list of traits that are considered relevant by the respondents' category (for example, crop producers). Whenever possible, disaggregated trait preferences based on several dimensions including gender, age and region should be considered. Second, breeders specialized on the crop of interest are consulted to inform which traits the national breeding program is actively prioritizing, ensuring that the choices proposed are appealing to the targeted category of respondents and trait levels align to existing product profiles, and are actionable by breeders.
2.3 Data analysis and synthesis
Once data are collected through the intra-household discrete choice experiment, the method allows to assess the utility of different trait bundles. Researchers can employ any of the discrete choice model suitable for this scope. We find it intuitive to employ coefficients obtained from a mixed logit model and convert them into probabilities of selection compared to the base level bundle. The base level bundle is represented by a combination of traits which are assumed to be the least desirable by a respondent (e.g., low yield, low biomass yield, long maturity, etc.).
Utilizing a mixed logit model enables researchers to relax the assumption that unobserved factors are uncorrelated over alternatives and there is the same variance across all alternatives. In the evaluation of alternative trait combinations, it is highly unlikely that different unobservable respondents' characteristics (such as attitudes, beliefs, risk perceptions) are uncorrelated over trait bundle alternatives. In a similar fashion, it is probable that some trait bundle alternatives are less appealing for the overall sample studied. therefore, the mixed logit model (or random parameter logit) is flexible enough to permit heterogeneity across individuals and time, and it overcomes the limitations of the conditional logit model by allowing for random taste variation, unrestricted substitution patterns, and correction in unobserved factors (Train, 2009). Equation 1 specifies the mixed logit utility function:
where βi is vector of mean attribute utility weights in the sample and σi is the vector representing farmer i specific deviation from the mean. Xij are trait bundle alternatives and εij is the random error component. βi ensures that the mixed logit coefficients vary among sampled respondents, generating the heterogeneity exploited by the method to investigate different intra-household probabilities of selection compared to the base level bundle.
By considering the base bundle as the least attractive trait bundle from the respondents' perspective, modifying just one level of the crop attribute significantly enhances the utility and selection probabilities of farmers. More specifically, the probability indicates the likelihood of selecting a specific trait bundle relative to the base bundle. The attribute categories in a trait bundle “b” are dummy variables, taking the value 1 if included and 0 if not. To find the probability of selecting each bundle relative to the base bundle, we transform the ordinal utility calculations into odds following Equation 2.
The probability of selecting a trait bundle relative to the base bundle alternative is a rather straightforward way to synthesize the data collected through the method. However, the method becomes particularly informative if probabilities are disaggregated by socially relevant variables collected through the socio-demographic or crop management modules of the survey. Following Equation 2, probabilities can be segmented by sex, by decision-making, time and knowledge dimensions. Furthermore, they can be segmented by agreement between respondents on responses to each module and question.
3 Piloting the method with cowpea growers in Senegal
The testing of the method was carried out in the Peanut Basin area, Senegal, in February-March 2023. The area includes six administrative regions (Louga, Kaolack, Fatick, Diourbel, Thies and Kaffrine) and it accounts for a sizable portion of the country's land area and population (Beye et al., 2022). We chose to focus on these regions due to their high agricultural productivity, which is critical to the country's economy (Toure and Diakhate, 2020). In these areas, crop production is primarily characterized by cereal-leguminous rotations, with millet, maize, groundnuts, and cowpea being the main crops grown for home consumption (United States Agency for International Development, 2016).
The research team agreed to test the method with households who are engaged in cowpea production for two reasons: first, the study area experienced a decrease in rainfall levels in recent years, leading to environmental degradation and loss of plant cover (Faye and Du, 2021) which his has led to cowpea cultivation becoming a strategic crop choice for farmers in the area due to its drought tolerance compared to other rainfed crops; Second, in Senegal cowpea is commonly grown by both men and women farmers (Guendel, 2009; Smale et al., 2022).
3.1 Sampling and administration of the socio-demographic and crop management modules
In July 2022, the Senegalese Institute of Agricultural Research collected an array of socioeconomic and agronomic data on cowpea producers in the Peanut Basin area. This baseline data provided useful insights on socio-demographic characteristics of cowpea producers in the area, such plot size, number of varieties produced, and top-2 ranked trait preferences by household heads. This information was used to refine the intra-household choice experiment and to guide the sample size calculation.
We used a three-stage sampling technique to select communes/municipalities, villages, and agricultural households to include in the pilot. The number of communes per region was determined by calculating the agricultural weight based on each region's 2017–18 cowpea production values, allowing us to randomly select an average of four communes from each region. Using GIS locations from the 2022 survey, we randomly chose two villages from each selected commune, resulting in a total of 45 villages where we conducted our survey. To focus on intra-household roles and preferences, we interviewed household heads and spouses from monogamous and polygamous agricultural households who produced cowpea. At the village level, we randomly selected six households from a list of cowpea producers in the region, provided by the village chief. Additionally, we chose five households from the list as proxies to replace any original households that were absent or unwilling to participate. The total number of responding households was 270, and we collected data from 540 individuals. Interviews were conducted in the homestead, separately between heads of households and spouses to minimize potential influence in responses and we randomized the order of the interviews in each household. In polygamous households, we interviewed any available spouse who was involved in cowpea production and could provide time, as we found no evidence of any established ranking among the spouses in our study areas.
The survey team consisted of six enumerators (four men, two women) from the Senegalese Institute of Agricultural Research. We initially planned to pair same sex respondents - enumerators, to avoid mistrusts and biases in responses, but during recruitment we encountered difficulties to engage women enumerators. Enumerators were selected for their prior surveying experience and proficiency in local languages, specifically Wolof, to effectively communicate with the participants. Before conducting interviews, the enumerators received a week-long training on the method objectives, on the survey and the discrete choice experiment to ensure consistent and clear survey execution and conceptual explanations.
3.2 Design of the discrete choice experiment
To design the discrete choice experiment, we followed the two-step approach previously described. Firstly, we conducted a literature review on traits and attributes for cowpea in Senegal. Our primary list of traits was inspired by the seminal paper by Kitch et al. (1998), which classified each cowpea-related trait into three categories - yield, quality and labor-related. Similarly, the baseline data collection in July 2022 conducted by Senegalese Institute of Agricultural Research in the same area informed the first list of 10 traits to be prioritized in the choice experiment.
However, to reduce the study complexity while maintaining the efficiency of the choice experiment design, we had to further restrict the number of traits. Evidence suggests that farmers make correct choices when presented with fewer attributes in a choice set, as this eliminates the tendency to ignore one or more attributes in the experiment (Hensher and Greene, 2010). Therefore, as a second step in this approach, we consulted two cowpea breeders at the Senegalese Institute of Agricultural Research. We presented them with a list of 10 traits and we co-evaluated which traits would be included in the final experiment design. Following the method, decisions were made according to producers' preferences, the ability to act on the traits by breeding programs, and according to national program priorities for the current and future breeding cycles. For example, grain yield and biomass yield were top ranked by growers in the baseline survey, cowpea breeders were highly interested in growers' trade-off between grain yield and biomass yield as cowpea is regarded as a dual-purpose crop. The relevance of these two traits for both stakeholders' groups and the fact that the national breeding program has the mandate to breed for dual-purpose cowpea in the next breeding cycle ensured that both grain yield and biomass yield were included in the choice experiment. On the contrary, taste was highly ranked by growers, but breeders did not currently have the ability to phenotype their breeding lines for taste (with neither consumer testing nor sensory evaluation). Therefore, despite its importance taste was excluded from the experiment, but noted by breeders as an area in need of methodological development for phenotypic evaluation. The five final traits were included in the discrete choice experiment are shown in Table 1. We chose to specify the level in a qualitative form (e.g., short and long cycle, instead of providing the exact number of days) since we could not find enough evidence in the literature to quantitatively construct all levels precisely. We completed the design of the choice experiment choosing pictorial representations of the traits selected. For grain yield, biomass yield and seed size we utilized pictures taken at local markets, while maturity and pod filling were exemplified through vignettes. We acknowledge that the representation of pod filling through vignette might be partially misleading for respondents.
We used JMP, a statistical software, to create the choice profiles included in the experiment based on the attributes and levels described in Table 1. A full-factorial design of 192 possible combinations (24 x 3 x 4) was obtained, given that we had 4 attributes with two levels, 1 attribute with three levels, and 1 attribute with four levels. However, since it is impractical to expect farmers to evaluate 192 choices, we used a D-optimal design using the JMP software. A D-optimal design is an algorithmic approach used in choice experiments to maximize the determinant of the information set used in the design of experiments with multiple treatments. It is designed to maximize the differences in attribute levels across alternatives, provide the best subset of all possible combinations and yield data that enables the estimation of parameters with low standard errors (Kimathi et al., 2022). Our generated design had a D-efficiency value of 99.28, indicating a high level of D-optimality (Kuhfeld, 2010). Further, we used a blocking strategy to mitigate the potential impact of presenting too many choice tasks on the respondents' decisions, as this helps to improve response efficiency by reducing the cognitive effort required from each respondent (Hanley et al., 2002; Johnson et al., 2013). A fractional factorial design with 24 choice sets was generated and put into three blocks, each consisting of 8 choice sets. Participants in the choice experiment were randomly assigned to one of the blocks and presented with 8 independent choice sets, with the sequence of the sets randomized within each block. Each choice set depicted a real market situation with two alternatives and an opt-out option, and participants were asked to choose their preferred alternative based on the attribute levels presented. In total, the study gathered 12,960 individual choices (540 farmers × 8 choice sets × 3 alternatives).
3.3 Synthesis of results and segmentation of bundle probability by a socially relevant variable
We estimated respondents' choice utility following Equation 1, including the cowpea traits of the choice experiment (i.e., grain yield, biomass yield, maturity, pod filling, seed size) as explanatory variables. The utility derived by producer i from choosing cowpea bundle j at choice occasion t is shown as:
The dependent variable is a binary variable defined as 1 if respondent i chooses cowpea alternative j in a choice set t. We encode all traits in the equation using a dummy variable. The base level for each attribute is used as the reference to compare the change in producers' utility. The base level used across all model specifications is low grain yield, low biomass yield, long maturity, fewer seeds per pod, and small seed size (Table 2).
To analyze the utility of the cowpea bundle, we use the coefficients from Equation 3 and transform them into probabilities of selection as compared to the base level. We calculate the utility of the cowpea bundle as:
where bundle utility b is the sum of the utility for each trait category. The trait categories in bundle b are dummy variables, taking the value 1 if preferred and 0 if not. To find the probability of selecting each bundle relative to the base bundle, we transform the ordinal utility calculations into odds. In this analysis, all probabilities indicate the likelihood of selecting a particular bundle in comparison to the base bundle. We consider the base bundle as a cowpea variety with low grain and biomass yield, long maturity, fewer seeds per pod, and small seed size (the least desirable baseline). To calculate the bundle probabilities, we adapt Equation 2 to our specific case under analysis (Equation 5):
As previously specified, the bundle probability analysis can be segmented using socially relevant variables. To showcase the potential of the method, we further synthesized findings from Equation 5 by (i) respondents' sex, (ii) intra-household level of agreement on who decides which variety is to be planted and by (iii) respondents' level of experience in growing cowpea. We first calculated the bundle probability analysis for women and men respondents separately. Secondly, we disaggregated bundle probabilities by the intra-household level of agreement on who decides which cowpea variety is to be planted. Whenever two members of the same household agree on who is the decision-maker for this specific cowpea pre-harvest activity, we consider that household to be in agreement on who decides which cowpea variety is to be planted. Therefore, we calculated the bundle probabilities for those households in agreement on cowpea variety decision-making with respect to households not in agreement. These results are shown in Figure 4B. Finally, we disaggregated by respondents' experience in growing cowpea: we distinguish between those with more than 20 years of experience and those with less. The 20-years cut off has been chosen since it is the mean of the variable distribution.
The three socially relevant variables we showcase in this study are just two of the possible segmentations which our method allows us to compute. The method permits disaggregation either by composite indices aggregating decision-making, time and experience into crop roles or by decision-making, time and experience individually. Furthermore, disaggregation can be made at pre-harvest, harvest and post-harvest stages.
4 Preliminary results showcasing the potential of the method
Mixed logit model coefficients from Equation 3 are shown in Table 3. These coefficients are not disaggregated and represent the influence of independent cowpea attributes on farmers' choice utility and signals their preferences for each trait.
Building on coefficients from Table 3, results from the probability analysis are shown in Figure 2. When a trait level is changed compared to the base bundle, the probability analysis shows how much more likely respondents are to choose the new variety. The base level represents the least-attractive bundle from the respondents' perspective, and it has a 50% probability to be chosen over any other bundle. Our findings reveal that respondents, in general, prioritize high grain yield as the most important attribute in cowpea varieties, followed by biomass yield and short maturity. Specifically, when a high grain yield is offered instead of a low grain yield, the likelihood of respondents selecting the new cowpea variety increases from 50% (base bundle) to 88% for the new bundle, with all other traits kept constant. Similarly, when a high biomass yield is offered instead of a low biomass yield, the probability of respondents selecting the new cowpea variety increases from 50 to 79%. Early maturity shows a similar importance, increasing the probability of selecting the new variety from 50 to 76%. More seeds per pod and large seed size do influence the probability of choosing the new variety too, but the effect is smaller compared to other traits in the bundle.
These findings build on those from the choice experiment, and they are interesting per se as there are no similar studies currently existing on cowpea in Senegal. However, they do not exploit the entire potential of the intra-household method proposed. To display how the survey can support the segmentation of the findings, we decided to present the probability analysis by respondents' sex, by intra-household level of agreement on who decides which cowpea variety to plant and by experience. In the sample of this pilot, household head respondents are always men while spouses are always women. The proportion of households in agreement regarding who decides on which cowpea variety to purchase is shown in Figure 3.
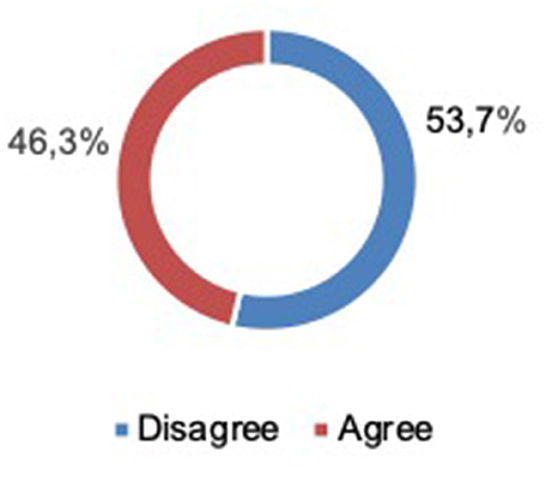
Figure 3. Proportion of households in agreement on who decides which cowpea variety is to be purchased.
The figure shows that 53.7% of intra-household respondents disagree on who the major decision maker on the variety to be planted is. Data shows that most disagreements occur when both the household heads and spouses identify themselves as the main decision-makers and choose the respondent alone option while answering the “who decides” question on planting cowpea variety. In a smaller number of cases, members identify each other as the main decision-maker (i.e., head identifies spouse or vice versa) and this is still considered a disagreement. Discussions on why these mismatches exist would require a thorough study on gender norms in Senegal and this goes beyond the scope of this methodological paper. We will just say that these discrepancies signal an intra-household heterogeneity which is frequently ignored by crop breeding programs in their priority setting analysis and that this heterogeneity might have implications for seed and marketing as well as adoption. For 53.7% of the households in our sample, the survey question “who decides which cowpea variety to be planted” will have a different answer if asked of the household head or of the spouse. A study not including an intra-household design would have missed this discrepancy, along with others driven by socially relevant variables. To demonstrate how the method is novel in this regard, we go on to regenerate (Figure 2), using responses from the intra-household module.
Figure 4 depicts bundle probability differences for three different segments: men and women respondents (Figure 4A), intra-household agreement on the “who decides” question on planting cowpea variety and intra-household disagreement (Figure 4B) and respondents' experience on growing cowpea (Figure 4C). Findings segmented by gender reveal that the probability of women respondents to select a new cowpea variety is driven predominantly by high grain yield. When a high grain yielding variety is offered, the likelihood of choosing the new cowpea variety increases from 50% (for the base bundle) to 91% for women, and the increase is significantly higher than for men. Conversely, the probability of choosing a new cowpea variety which is more biomass yielding increases to 67% for women and 86% for men.
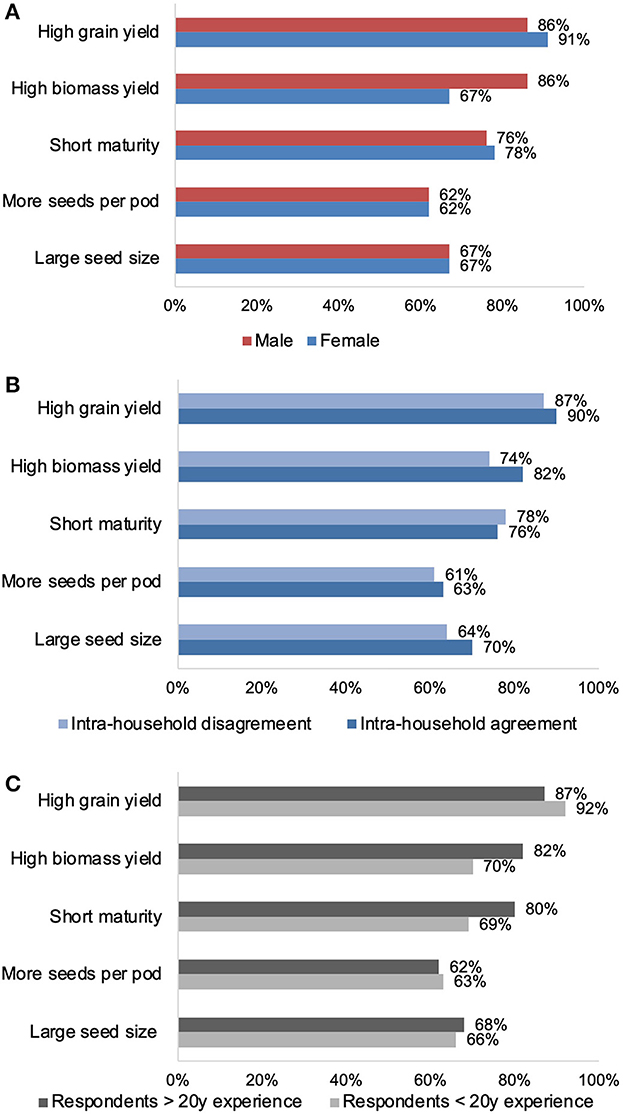
Figure 4. Bundle probability analysis by socially relevant variables. (A) represents disaggregation by gender, (B) shows disaggregation by household agreement, and (C) by respondents' years of experience.
Figure 4B highlights bundle probabilities differences according to intra-household agreement on who decides which cowpea variety is to be planted. Bundle probabilities do not differ significantly for the three traits of high grain yield, short maturity, and more seeds per pod. For respondents which show intra-household agreement the probability of choosing a new variety which has large seed size is higher than for respondents with intra-household disagreement, but the probability differences are still relatively small (+6%). The narrative is however quite different for high biomass yield: respondents which show intra-household agreement appear to have a probability of choosing a high biomass yielding variety which increases from 50 to 82%, while the one of respondents with intra-household disagreement is at 74%. Finally, Figure 4C depicts bundle probabilities by respondents' years of experience. In this case, differences in trait preferences widen with more experienced respondents preferring short maturity and high biomass yield more than respondents with relatively less experience. The stark difference in bundle probabilities especially on short maturity is very interesting, as it has not been documented previously.
In its entirety, Figure 4 stylizes how the method proposed in this study makes apparent the complexity behind trait preferences. Combining an agile intra-household survey and a streamlined choice experiment, the method gives cross-functional breeding teams the opportunity to quantitatively measure trait preference differences at the intersection between gender, crop roles, decision-making, experience and time.
5 Discussion
Overall, the signs of the two yield coefficients indicate that farmers prefer cowpea varieties with high grain and biomass yields in comparison to low yielding varieties (Table 3). These findings suggests the breeding programs the need to prioritize breeding for dual-purpose varieties in Senegal, as farmers use this crop both for human consumption and animal feed, as biomass yield as a desirable and marketable trait. Looking at the coefficients for the maturity and pod filling traits, respondents exhibit a stronger preference for short maturity compared to long maturity, and for more seeds per pod over fewer seeds per pod. The short rainy season in Senegal might be one of the contributing factors driving the preference for short maturity. Evidence shows that early maturity in crop varieties help coping with short rainy seasons (Abdou, 2021). Furthermore, early maturity in cowpea seems to allow producers to avoid pest and disease infestation that typically occurs at a later stage of cropping seasons (Owusu et al., 2021). More seeds per pod is a trait tied to yield and scholars in the past have considered more seeds per pod also as a labor-saving trait, as it reduces farmers' effort in threshing for a given quantity of cowpea (Kitch et al., 1998). Lastly, respondents seem to prefer cowpea varieties with larger or medium seed sizes over those with smaller seed sizes. In similar studies on preferences across West African countries, cowpea value chain actors have specified their preferences for larger seed size as they believe that consumers in West African regions are ready to pay a premium for such quality (Langyintuo et al., 2003; Mishili et al., 2009; Bolarinwa et al., 2021; Mohammed et al., 2021). The high significance of each trait in Table 3 suggests that each of these traits is generally preferred by respondents. However, in cases when the breeding team is not able to breed for all these traits equally, it is important to investigate trade-offs.
Figure 4 is however the one exemplifying the full potential of the method. The presentation of bundle probabilities segmented by socially-relevant variables is instrumental to study whether intra-household heterogeneity in trait preferences is driven by respondents' roles in crop choice and production. The focus on these roles transcend sex-disaggregation, to include aspects of decision-making, time, and experience on the crop. The proposed method rests on the assumption that a higher intra-household heterogeneity in trait preferences is paired with a higher intra-household heterogeneity in decision-making, experience and time spent. Furthermore, higher intra-household disagreement on crop roles might lead to higher intra-household heterogeneity in trait preferences. The method and the suggested data synthesis via bundle probabilities enable us to test both hypotheses.
For example, short maturity, more seeds per pod and large seeds are traits desirable equally for both women and men. As the Senegalese national breeding program is interested in breeding for dual-purpose cowpea, these intra-household gendered preferences on grain yield and biomass yield hint at the fact that gender entry points should be systematically integrated into the breeding pipeline, to ensure that improved dual-purpose varieties mediate women's and men's needs. However, households showing intra-household agreement present trade-offs between grain yield and biomass yield which are different from households in disagreement. Hypotheses on why this might happen are multiple: households in agreement on who decides which cowpea variety is to be planted might prefer high biomass yielding varieties because the agreement is driven by more discussion within the family and convergence of preferences or, on the contrary, heads' preferences become predominant in pre-harvest choices. Whatever the underlying mechanism, results disaggregated by intra-household agreement raise awareness on the relational nature of trait preferences, with individual choices being shaped by other household members' preferences.
6 Conclusions
This study presents a new method for exploring intra-household trait preferences using choice experimentation. Dissecting intra-household crop roles into three dimensions, we explore what drives intra-household heterogeneity in varietal preferences between gender, decision-making, crop experience and time spent working on the crop. Combining an agile intra-household survey and a streamlined choice experiment, preliminary results from the pilot exemplifies trait differences segmented by a battery of socially relevant variables. Next to findings disaggregated by gender, which contribute to a long-standing stream of literature in priority setting, the method is able to dissect preferences by less investigated – but equally relevant – variables, such respondents' years of experience and the level of intra-household agreement on who decides which variety is to be planted. Similarly, the method can be adjusted to account for other economically relevant variable which play a role in shaping farmers' trait preferences, such as land and input ownership.
The method gives cross-functional breeding teams the opportunity to quantitatively measure trade-offs in trait preferences, considering trait choices an intersection between respondents' gender, crop roles, decision making, experience, and time. By suggesting a method which combines agile and streamlined tools (i.e., a brief intra-household survey and a traditional discrete choice experiment), we supply social scientists within breeding teams with a data collection method which promotes attention to diverse market segments in setting breeding priorities.
Data availability statement
The raw data supporting the conclusions of this article will be made available by the authors, without undue reservation.
Ethics statement
The studies involving humans were approved by the Institutional Review Board for Human Participants at Cornell University has granted this study exemption from IRB review according to Cornell IRB policy and under the Department of Health and Human Services Code of Federal Regulations 45CFR46.104(d). For questions related to this application, please contact the IRB office at irbhp@cornell.edu, specifying the protocol number IRB0146740. The studies were conducted in accordance with the local legislation and institutional requirements. The participants provided their written informed consent to participate in this study.
Author contributions
RM: Data curation, Formal analysis, Investigation, Methodology, Writing – original draft. NF: Conceptualization, Funding acquisition, Investigation, Methodology, Writing – review & editing. MB: Data curation, Investigation, Writing – review & editing. MG: Conceptualization, Funding acquisition, Supervision, Writing – review & editing. DR: Conceptualization, Methodology, Writing – original draft. HT: Conceptualization, Formal analysis, Funding acquisition, Methodology, Writing – original draft. MO: Conceptualization, Formal analysis, Methodology, Visualization, Writing – original draft.
Funding
The author(s) declare financial support was received for the research, authorship, and/or publication of this article. This publication is made possible by the generous support of the American people through the United States Agency of International Development (USAID). The contents are the responsibility of the authors and do not necessarily reflect the views of USAID or the United States Government. Program activities are funded by the United States Agency for International Development (USAID) under Cooperative Agreement no. 7200AA-19LE-00005. The study also acknowledges funding from the ABEE project - West African Breeding networks and Extension Empowerment.
Acknowledgments
We thank Eveline Compaore (INERA, Burkina Faso) for comments on the work: her insight on considering time on the plot and experience as dimensions of crop management has proven key in developing this study. Our deep gratitude goes to the field officers who collected the data. We also thank respondents in the study area: engaging in intra-household research requires an extra dose of time, patience and trust in the researchers. Finally, we are indebted to Jie Li (Cornell University) for her invaluable support in designing the choice experiment.
Conflict of interest
DR is the Director of Cultural Practice, LLC.
The remaining authors declare that the research was conducted in the absence of any commercial or financial relationships that could be construed as a potential conflict of interest.
Publisher's note
All claims expressed in this article are solely those of the authors and do not necessarily represent those of their affiliated organizations, or those of the publisher, the editors and the reviewers. Any product that may be evaluated in this article, or claim that may be made by its manufacturer, is not guaranteed or endorsed by the publisher.
Supplementary material
The Supplementary Material for this article can be found online at: https://www.frontiersin.org/articles/10.3389/fsufs.2023.1257076/full#supplementary-material
Footnotes
1. ^If the household is not composed of a head and a single spouse (for example, it is a polygamous household or a household headed by a widow), respondents should be the adult man or woman agricultural decision maker.
References
Abdou, S. (2021). Grain and fodder yields of early-maturity cowpea (Vigna unguiculata L. Walp) lines in Niger Republic. J. Appl. Biosci. 164, 16931–16942.
Akresh, R. (2005). Understanding pareto inefficient intra-household allocations. IZA Dis. Paper 12, 1858. doi: 10.2139/ssrn.866885
Alkire, S., Meinzen-Dick, R., Peterman, A., Quisumbing, A., Seymour, G., Vaz, A., et al. (2013). The women's empowerment in agriculture index. World Dev. 52, 71–91. doi: 10.1016/j.worlddev.2013.06.007
Amare, Z. Y., Ayoade, J. O., Adelekan, I. O., and Zeleke, M. T. (2018). Barriers to and determinants of the choice of crop management strategies to combat climate change in Dejen District, Nile Basin of Ethiopia. Agric. Food Secur. 7, 1–11. doi: 10.1186/s40066-018-0188-y
Anderson, C. L., Reynolds, T. W., and Gugerty, M. K. (2017). Husband and wife perspectives on farm household decision-making authority and evidence on intra-household accord in rural Tanzania. World Dev. 90, 169–183. doi: 10.1016/j.worlddev.2016.09.005
Anugwa, I. Q., Onwubuya, E. A., Chah, J. M., Abonyi, C. C., and Nduka, E. K. (2022). Farmers' preferences and willingness to pay for climate-smart agricultural technologies on rice production in Nigeria. Clim. Policy 22, 112–131. doi: 10.1080/14693062.2021.1953435
Ashby, J. A., and Polar, V. (2019). The Implications of Gender Relations for Modern Approaches to Crop Improvement and Plant Breeding. Gender, Agriculture and Agrarian Transformations. London: Routledge, 11–34.
Asrat, S., Yesuf, M., Carlsson, F., and Wale, E. (2010). Farmers' preferences for crop variety traits: lessons for on-farm conservation and technology adoption. Ecol. Econ. 69, 2394–2401. doi: 10.1016/j.ecolecon.2010.07.006
Attanasio, O., and Lechene, V. (2002). Tests of income pooling in household decisions. Rev. Econ. Dyn. 5, 720–748. doi: 10.1006/redy.2002.0191
Becker, G. S. (1965). A theory of the allocation of time. Econ. J. 75, 493–517. doi: 10.2307/2228949
Bernard, T., Doss, C., Hidrobo, M., Hoel, J., and Kieran, C. (2019). Ask me why: patterns of intrahousehold decision-making. World Dev. 125, 104671. doi: 10.1016/j.worlddev.2019.104671
Beye, A., Diakhate, P. B., Diouf, O., Faye, A., Obour, A. K., Stewart, Z. P., et al. (2022). Socio-economic constraints of adopting new cowpea varieties in three agro-ecological zones in the Senegalese peanut basin. Sustainability 14, 14550. doi: 10.3390/su142114550
Bolarinwa, K. A., Ogunkanmi, L. A., Ogundipe, O. T., Agboola, O. O., and Amusa, O. D. (2021). An investigation of cowpea production constraints and preferences among small holder farmers in Nigeria. Geo J. 24, 1–13. doi: 10.1007/s10708-021-10405-6
Burns, J. G., Eory, V., Butler, A., Simm, G., and Wall, E. (2022). Preference elicitation methods for appropriate breeding objectives. Animal 16, 100535. doi: 10.1016/j.animal.2022.100535
Carletto, C. (2021). Better data, higher impact: improving agricultural data systems for societal change. Eur. Rev. Agric. Econ. 48, 719–740. doi: 10.1093/erae/jbab030
Chiappori, P. A., Fortin, B., and Lacroix, G. (2002). Marriage market, divorce legislation, and household labor supply. J. Polit. Econ. 110, 37–72. doi: 10.1086/324385
Deere, C. D., and Doss, C. R. (2006). The gender asset gap: What do we know and why does it matter? Femin. Econ. 12, 1–50. doi: 10.1080/13545700500508056
Deressa, T. T., Hassan, R. M., Ringler, C., Alemu, T., and Yesuf, M. (2009). Determinants of farmers' choice of adaptation methods to climate change in the Nile Basin of Ethiopia. Glob. Environ. Change 19, 248–255. doi: 10.1016/j.gloenvcha.2009.01.002
Doss, C. (2001). How does gender affect the adoption of agricultural innovations? The case of improved maize technology in Ghana. Agric. Econ. 25, 27–39. doi: 10.1016/S0169-5150(00)00096-7
Doss, C., and Kieran, C. (2013). Standards for Collecting Sex-Disaggregated Data for Gender Analysis: A Guide for CGIAR Researchers In Workshop on Methods and Standards for Research on Gender and Agriculture (Consultative Group for International Agricultural Research). Available online at: https://cgspace.cgiar.org/handle/10947/3072 (accessed May 31, 2023).
Doss, C. R. (2021). Diffusion and dilution: the power and perils of integrating feminist perspectives into household economics. Femin. Econ. 27, 1–20. doi: 10.1080/13545701.2021.1883701
Doss, C. R., Catanzarite, Z., Baah-Boateng, W., Swaminathan, H., Deere, C. D., Boakye-Yiadom, L., et al. (2018). Do men and women estimate property values differently? World Dev. 107, 75–86. doi: 10.1016/j.worlddev.2018.02.012
Doss, C. R., Deere, C. D., Oduro, A. D., and Swaminathan, H. (2014). The gender asset and wealth gaps. World Dev. 57, 400–409. doi: 10.1057/dev.2015.10
Duflo, E., and Udry, C. R. (2004). Intrahousehold Resource Allocation in Cote d'Ivoire: Social Norms, Separate Accounts and Consumption Choices. NBER Working Paper No. w10498. Available online at: https://ssrn.com/abstract=552103
Faye, B., and Du, G. (2021). Agricultural land transition in the “groundnut basin” of Senegal: 2009 to 2018. Land 10, 996. doi: 10.3390/land10100996
Fisher, M., and Carr, E. R. (2015). The influence of gendered roles and responsibilities on the adoption of technologies that mitigate drought risk: the case of drought-tolerant maize seed in eastern Uganda. Glob. Environ. Change 35, 82–92. doi: 10.1016/j.gloenvcha.2015.08.009
Guendel, S. (2009). What Are 'Women'Scrops', and Why? Food and Agriculture Organization of the United Nations. Available online at: https://www.fao.fao./gender/insights/detail/en/c/36003/
Gulati, K. (2016). Who's the Boss? Intrahousehold Valuation, Preference Heterogeneity, and Demand for an Agricultural Technology in India. (job market paper). Available online at: https://economics.ucr.edu/pacdev/pacdev-papers/who_is_the_boss_intrahousehold.pdf
Hanley, N., Wright, R. E., and Koop, G. (2002). Modeling recreation demand using choice experiments: climbing in Scotland. Environ. Res. Econ. 22, 449–466. doi: 10.1023/A:1016077425039
Hensher, D. A., and Greene, W. (2010). Non-attendance and dual processing of common-metric attributes in choice analysis: a latent class specification. Empir. Econ. 39, 413–426. doi: 10.1007/s00181009-0310-x
Hoddinott, J., and Haddad, L. (1995). Does female income share influence household expenditures? Evidence from Côte d'Ivoire. Oxford Bullet. Econ. Stat. 57, 77–96. doi: 10.1111/j.1468-0084.1995.tb00028.x
Johnson, F. R., Lancsar, E., Marshall, D., Kilambi, V., Bs, B. A., Mu, A., et al. (2013). Constructing experimental designs for discrete-choice experiments: report of the ISPOR conjoint analysis experimental design good research practices task force. Value Health 61, 3–13. doi: 10.1016/j.jval.2012.08.2223
Joshi, P. K., Khan, M. T., and Kishore, A. (2019). Heterogeneity in male and female farmers' preference for a profit-enhancing and labor-saving technology: the case of direct-seeded rice (DSR) in India. Can. J. Agric. Econ. 67, 303–320. doi: 10.1111/cjag.12205
Kassie, G. T., Abdulai, A., Greene, W. H., Shiferaw, B., Abate, T., Tarekegne, A., et al. (2017). Modeling preference and willingness to pay for drought tolerance (DT) in maize in rural Zimbabwe. World Dev. 94, 465–477. doi: 10.1016/j.worlddev.2017.02.008
Khanal, U., Adhikari, A., and Wilson, C. (2017). Evaluating smallholder farmers' demand for rice variety attributes in Nepal. J. Crop Imp. 31, 438–452. doi: 10.1080/15427528.2017.1311286
Kimathi, S. M., Ayuya, O. I., and Mutai, B. (2022). Stated Farmers' Preferences and Willingness to Pay for Climate Resilient Potato Varieties in Kenya: A Discrete Choice Experiment. Available online at: https://ageconsearch.umn.edu/record/321220/ (accessed May 31, 2023).
Kitch, L. W., Boukar, O., Endondo, C., and Murdock, L. L. (1998). Farmer acceptability criteria in breeding cowpea. Exp. Agric. 34, 475–486.
Kolstad, J. R. (2011). How to make rural jobs more attractive to health workers. Findings from a discrete choice experiment in Tanzania. Health Econ. 20, 196–211. doi: 10.1002/hec.1581
Krishna, V. V., and Veettil, P. C. (2022). Gender, caste, and heterogeneous farmer preferences for wheat varietal traits in rural India. PLoS ONE 17, e0272126. doi: 10.1371/journal.pone.0272126
Kuhfeld, F. W. (2010). Marketing Research Methods in SAS: SAS 9, 2nd Edn. Arlington: SAS Institute Inc.
Labarta, R. A. (2009). Are Small Sub-Sahara African Farmers Willing to Pay for Vegetative Propagated Orange Fleshed Sweet Potato Planting Material? Evidence from Central Mozambique (No. 319-2016-9823). Available online at: https://ageconsearch.umn.edu/record/49447/ (accessed May 31, 2023.).
Laborte, A. G., Paguirigan, N. C., Moya, P. F., Nelson, A., Sparks, A. H., Gregorio, G. B., et al. (2015). Farmers' preference for rice traits: insights from farm surveys in Central Luzon, Philippines. PLoS ONE 10. e0136562. doi: 10.1371/journal.pone.0136562
Langyintuo, A. S., Lowenberg-DeBoer, J., Faye, M., Lambert, D., Ibro, G., Moussa, B., Kergna, A., Kushwaha, S., Musa, S., and Ntoukam, G. (2003). Cowpea supply and demand in West Africa. Field Crops Res. 82, 215–231. doi: 10.1016/S0378-4290(03)00039-X
Lope-Alzina, D. G. (2007). Gendered production spaces and crop varietal selection: case study in Yucatán, Mexico. Singapore J. Trop. Geography 28, 21–38. doi: 10.1111/j.1467-9493.2006.00274.x
Macours, K. (2019). Farmers' demand and the traits and diffusion of agricultural innovations in developing countries. Ann. Rev. Res. Econ. 11, 483–499. doi: 10.1146/annurev-resource-100518-094045
Maligalig, R., Demont, M., Umberger, W. J., and Peralta, A. (2021). Understanding Filipino rice farmer preference heterogeneity for varietal trait improvements: a latent class analysis. J. Agric. Econ. 72, 134–157. doi: 10.1111/1477-9552.12392
Maligalig, R. L. (2018). Eliciting farmer preferences for rice varietal trait improvements using an experimental methodology based on investment games (Doctoral dissertation). Available online at: https://hdl.handle.net/2440/113577
Maligalig, R. L., Demont, M., Umberger, W. J., and Peralta, A. (2017). “Intrahousehold decision-making on rice varietal trait improvements: using experiments to estimate gender influence,” Agricultural and Applied Economics Association 2017 Annual Meeting, No 258522.
Marenya, P. P., Wanyama, R., Alemu, S., and Woyengo, V. (2021). Trait preference trade-offs among maize farmers in western Kenya. Heliyon 7, e06389. doi: 10.1016/j.heliyon.2021.e06389
Marimo, P., Caron, C., Van den Bergh, I., Crichton, R., Weltzien, E., Ortiz, R., et al. (2020). Gender and trait preferences for banana cultivation and use in Sub-Saharan Africa: a literature review. Econ. Bot. 74, 226–241. doi: 10.1007/s12231-020-09496-y
Martey, E., Etwire, P. M., Adogoba, D. S., and Tengey, T. K. (2022). Farmers' preferences for climate-smart cowpea varieties: implications for crop breeding programmes. Climate Dev. 14, 105–120. doi: 10.1080/17565529.2021.1889949
McDougall, C., Kariuki, J., Lenjiso, B. M., Marimo, P., Mehar, M., Murphy, S., et al. (2022). Understanding gendered trait preferences: Implications for client-responsive breeding programs. PLOS Sust. Trans. 1, e0000025. doi: 10.1371/journal.pstr.0000025
McEwan, M., Mulwa, C. K., Mussa, H., and Ogero, K. (2021). “Gender-differentiated trait preferences for sweet potato varieties in Tanzania,” in Focus Group Discussion Survey-Final Report (Lima: International Potato Center), 33.
McFadden, D. (1973). “Chapter 4: Conditional logit analysis of qualitative choice behavior,” in Frontiers in Economics, ed P. Zarambka (New York, NY: Academic Press), 105–142.
Mengistu, G., Shimelis, H., Laing, M., and Lule, D. (2019). Assessment of farmers' perceptions of production constraints, and their trait preferences of sorghum in western Ethiopia: implications for anthracnose resistance breeding. Acta Agric. Scand. Soil Plant Sci. 69, 241–249. doi: 10.1080/09064710.2018.1541190
Michler, J. D., Tjernström, E., Verkaart, S., and Mausch, K. (2019). Money matters: the role of yields and profits in agricultural technology adoption. Am. J. Agric. Econ. 101, 710–731. doi: 10.1093/ajae/aay050
Miriti, P., Regassa, M. D., Ojiewo, C. O., and Melesse, M. B. (2022). Farmers' preferences and willingness to pay for traits of sorghum varieties: informing product development and breeding programs in Tanzania. J. Crop Imp. 23, 1–20. doi: 10.1080/15427528.2022.2079038
Mishili, F. G., Fulton, J., Shehu, M., Kushwaha, S., Marfo, K., Jamal, M., et al. (2009). Consumer preferences for quality characteristics along the cowpea value chain in Nigeria, Ghana and Mali. J. Agribus. 25, 16–35. doi: 10.1002/agr.20184
Mohammed, S. B., Dzidzienyo, D. K., Umar, M. L., Ishiyaku, M. F., Tongoona, P. B., Gracen, V., et al. (2021). Appraisal of cowpea cropping systems and farmers' perceptions of production constraints and preferences in the dry savannah areas of Nigeria. AgriRxiv 9, 20210152201. doi: 10.31220/agriRxiv.2021.00046
Occelli, M., Mukerjee, R., Miller, C., Porciello, J., Puerto, S., Garner, E., et al. (2023). Trait Prioritization in Crop Breeding Programs: A Scoping Review on Tools and Methods. Available online at: https://doi.org/10.21203/rs.3.rs-2548847/v1 (accessed May 31, 2023).
Owusu, E. Y., Karikari, B., Kusi, F., Haruna, M., Amoah, R. A., Attamah, P., et al. (2021). Genetic variability, heritability and correlation analysis among maturity and yield traits in Cowpea (Vigna unguiculata (L) Walp) in Northern Ghana. Heliyon 7, e07890. doi: 10.1016/j.heliyon.2021.e07890
Pant, K. P., Gautam, J. C., and Wale, E. (2012). Valuation of Rice Diversity in Nepal: A Trait-Based Approach. The Economics of Managing Crop Diversity On-Farm. London: Routledge, 45–64.
Pierotti, R. S., Friedson-Ridenour, S., and Olayiwola, O. (2022). Women farm what they can manage: how time constraints affect the quantity and quality of labor for married women's agricultural production in southwestern Nigeria. World Dev. 152, 105800. doi: 10.1016/j.worlddev.2021.105800
Ryan, M., Kolstad, J. R., Rockers, P. C., and Dolea, C. (2012). How to Conduct a Discrete Choice Experiment for Health Workforce Recruitment and Retention in Remote and Rural Areas: A User Guide With Case Studies (English). Washington, DC: World Bank Group.
Sheahan, M., and Barrett, C. B. (2017). Ten striking facts about agricultural input use in Sub-Saharan Africa. Food Policy 67, 12–25. doi: 10.1016/j.foodpol.2016.09.010
Smale, M., Theriault, V., Allen, A., and Sissoko, M. (2022). Is cowpea a ‘women's crop'in Mali? Implications for value chain development. Afr. J. Agric. Res. Econ. 17, 92–105. doi: 10.22004/ag.econ.333971
Takahashi, K., Muraoka, R., and Otsuka, K. (2020). Technology adoption, impact, and extension in developing countries' agriculture: a review of the recent literature. Agric. Econ. 51, 31–45. doi: 10.1111/agec.12539
Teeken, B., Olaosebikan, O., Haleegoah, J., Oladejo, E., Madu, T., Bello, A., et al. (2018). Cassava trait preferences of men and women farmers in Nigeria: implications for breeding. Econ. Bot. 72, 263–277. doi: 10.1007/s12231-018-9421-7
Tonsor, G. T., and Shupp, R. S. (2011). Cheap talk scripts and online choice experiments: “looking beyond the mean”. Am. J. Agric. Econ. 93, 1015–1031. doi: 10.1093/ajae/aar036
Toure, A. K., and Diakhate, M. (2020). Descriptive Analysis of the Influence of Rainfall and Temperature Indicators on Agricultural Yields in Senegal. Available online at: https://www.researchgate.net/publicatioN/338698842_Analyse_descriptive_de_l%27
Train, K. E. (2009). Discrete Choice Methods With Simulation. Cambridge: Cambridge University Press.
Tufan, H. A., Grando, S., and Meola, C. (2018). State of the Knowledge for Gender in Breeding: Case Studies for Practitioners. Working Paper. No. 3. Lima: CGIAR Gender and Breeding Initiative. Available online at: https://cgspace.cgiar.org/handle/10568/92819
Udry, C. (1996). Gender, agricultural production, and the theory of the household. J. Polit. Econ. 104, 1010–1046. doi: 10.1086/262050
United States Agency for International Development (2016). Feed the Future Senegal Naatal Mbay Project: Women's Economic Empowerment Strategy.
Waldman, K. B., Ortega, D. L., Richardson, R. B., and Snapp, S. S. (2017). Estimating demand for perennial pigeon pea in Malawi using choice experiments. Ecol. Econ. 131, 222–230. doi: 10.1016/j.ecolecon.2016.09.006
Wasserman-Olin, R. (2020). Marketing decisions of farmers via wholesalers: a choice experiment (doctoral thesis). Available online at: https://ecommons.cornell.edu/server/api/core/bitstreams/ac77e030-c6b8-49fc-a04c-7c473bc089b3/content
Keywords: intra-household analysis, Senegal, cowpea, discrete choice experiment, gender
Citation: Mukerjee R, Faye NF, Badji MJ, Gomez M, Rubin D, Tufan HA and Occelli M (2023) Intra-household discrete choice experiment for trait preferences: a new method. Front. Sustain. Food Syst. 7:1257076. doi: 10.3389/fsufs.2023.1257076
Received: 10 August 2023; Accepted: 27 October 2023;
Published: 28 November 2023.
Edited by:
Donato Morea, University of Cagliari, ItalyReviewed by:
Edward Martey, CSIR-Savanna Agricultural Research Institute, GhanaTheophilus Tengey, CSIR-Savanna Agricultural Research Institute, Ghana
Copyright © 2023 Mukerjee, Faye, Badji, Gomez, Rubin, Tufan and Occelli. This is an open-access article distributed under the terms of the Creative Commons Attribution License (CC BY). The use, distribution or reproduction in other forums is permitted, provided the original author(s) and the copyright owner(s) are credited and that the original publication in this journal is cited, in accordance with accepted academic practice. No use, distribution or reproduction is permitted which does not comply with these terms.
*Correspondence: Martina Occelli, mo386@cornell.edu